Numeracy and Data Analysis
VerifiedAdded on 2023/01/11
|8
|1342
|80
AI Summary
This study focuses on data analysis and forecasting using various statistical methods. It covers topics such as arranging data in table format, presenting data using different charts, calculating mean, median, mode, range, and standard deviation, and using linear forecasting models. The study also includes a practical example of forecasting expenses using a linear forecasting model.
Contribute Materials
Your contribution can guide someone’s learning journey. Share your
documents today.
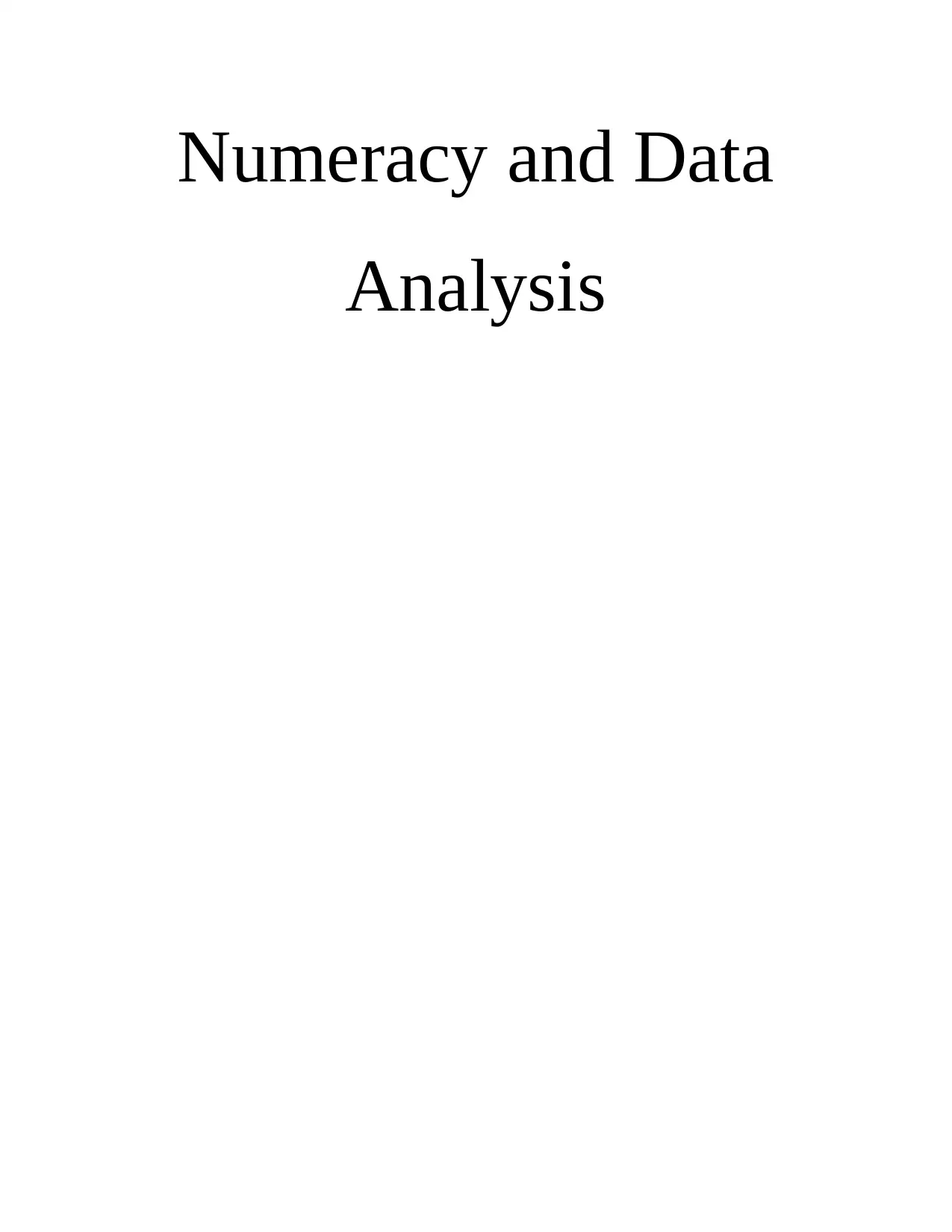
Numeracy and Data
Analysis
Analysis
Secure Best Marks with AI Grader
Need help grading? Try our AI Grader for instant feedback on your assignments.
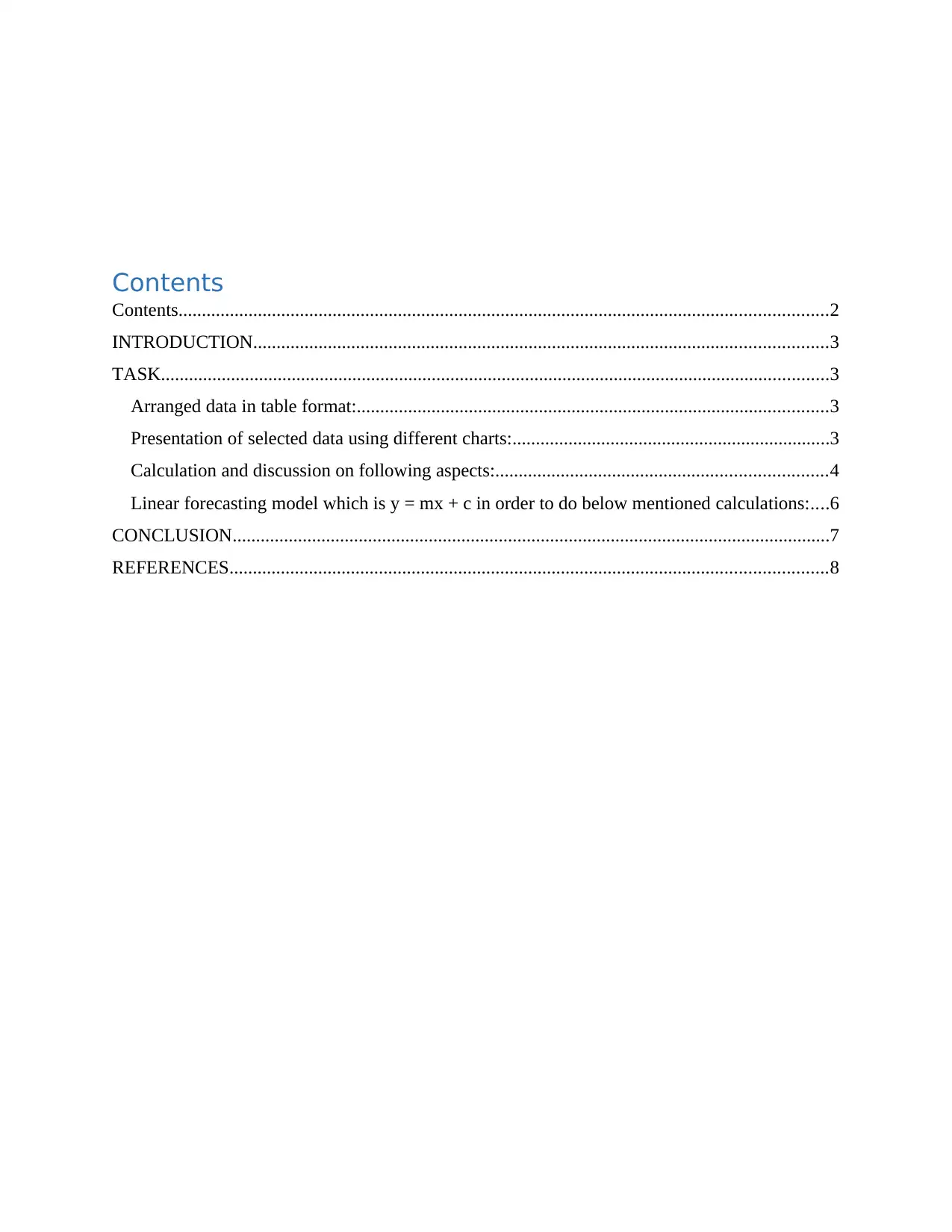
Contents
Contents...........................................................................................................................................2
INTRODUCTION...........................................................................................................................3
TASK...............................................................................................................................................3
Arranged data in table format:.....................................................................................................3
Presentation of selected data using different charts:....................................................................3
Calculation and discussion on following aspects:.......................................................................4
Linear forecasting model which is y = mx + c in order to do below mentioned calculations:....6
CONCLUSION................................................................................................................................7
REFERENCES................................................................................................................................8
Contents...........................................................................................................................................2
INTRODUCTION...........................................................................................................................3
TASK...............................................................................................................................................3
Arranged data in table format:.....................................................................................................3
Presentation of selected data using different charts:....................................................................3
Calculation and discussion on following aspects:.......................................................................4
Linear forecasting model which is y = mx + c in order to do below mentioned calculations:....6
CONCLUSION................................................................................................................................7
REFERENCES................................................................................................................................8
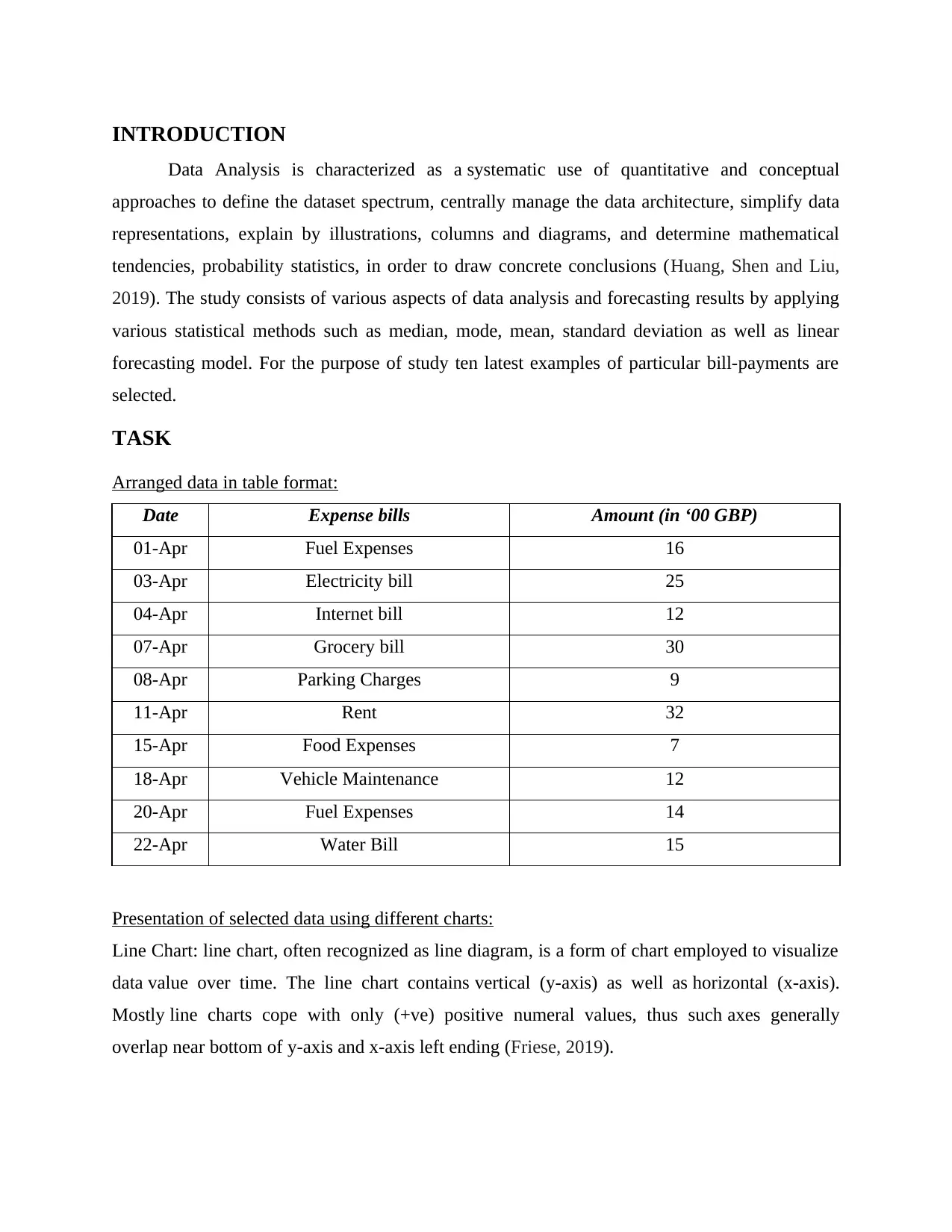
INTRODUCTION
Data Analysis is characterized as a systematic use of quantitative and conceptual
approaches to define the dataset spectrum, centrally manage the data architecture, simplify data
representations, explain by illustrations, columns and diagrams, and determine mathematical
tendencies, probability statistics, in order to draw concrete conclusions (Huang, Shen and Liu,
2019). The study consists of various aspects of data analysis and forecasting results by applying
various statistical methods such as median, mode, mean, standard deviation as well as linear
forecasting model. For the purpose of study ten latest examples of particular bill-payments are
selected.
TASK
Arranged data in table format:
Date Expense bills Amount (in ‘00 GBP)
01-Apr Fuel Expenses 16
03-Apr Electricity bill 25
04-Apr Internet bill 12
07-Apr Grocery bill 30
08-Apr Parking Charges 9
11-Apr Rent 32
15-Apr Food Expenses 7
18-Apr Vehicle Maintenance 12
20-Apr Fuel Expenses 14
22-Apr Water Bill 15
Presentation of selected data using different charts:
Line Chart: line chart, often recognized as line diagram, is a form of chart employed to visualize
data value over time. The line chart contains vertical (y-axis) as well as horizontal (x-axis).
Mostly line charts cope with only (+ve) positive numeral values, thus such axes generally
overlap near bottom of y-axis and x-axis left ending (Friese, 2019).
Data Analysis is characterized as a systematic use of quantitative and conceptual
approaches to define the dataset spectrum, centrally manage the data architecture, simplify data
representations, explain by illustrations, columns and diagrams, and determine mathematical
tendencies, probability statistics, in order to draw concrete conclusions (Huang, Shen and Liu,
2019). The study consists of various aspects of data analysis and forecasting results by applying
various statistical methods such as median, mode, mean, standard deviation as well as linear
forecasting model. For the purpose of study ten latest examples of particular bill-payments are
selected.
TASK
Arranged data in table format:
Date Expense bills Amount (in ‘00 GBP)
01-Apr Fuel Expenses 16
03-Apr Electricity bill 25
04-Apr Internet bill 12
07-Apr Grocery bill 30
08-Apr Parking Charges 9
11-Apr Rent 32
15-Apr Food Expenses 7
18-Apr Vehicle Maintenance 12
20-Apr Fuel Expenses 14
22-Apr Water Bill 15
Presentation of selected data using different charts:
Line Chart: line chart, often recognized as line diagram, is a form of chart employed to visualize
data value over time. The line chart contains vertical (y-axis) as well as horizontal (x-axis).
Mostly line charts cope with only (+ve) positive numeral values, thus such axes generally
overlap near bottom of y-axis and x-axis left ending (Friese, 2019).
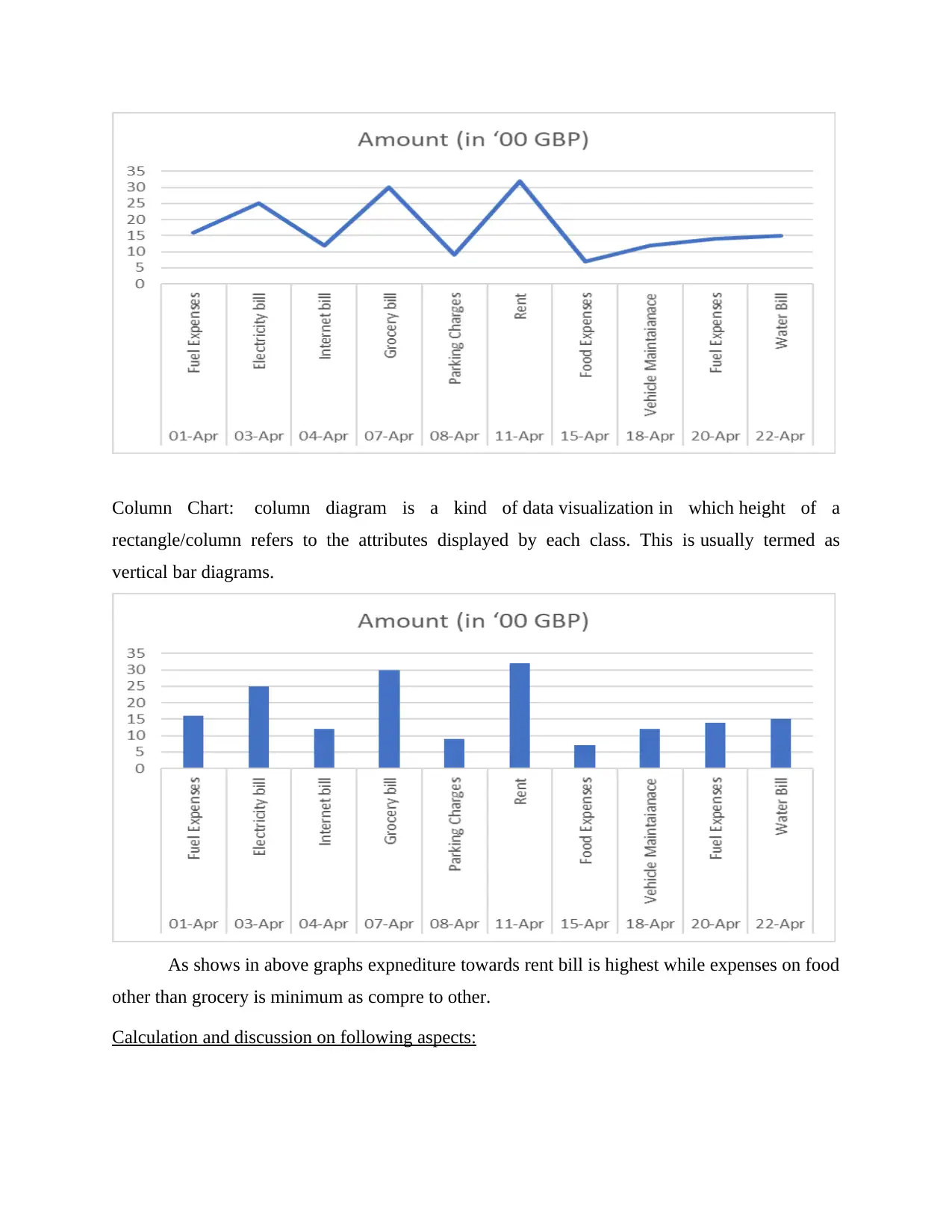
Column Chart: column diagram is a kind of data visualization in which height of a
rectangle/column refers to the attributes displayed by each class. This is usually termed as
vertical bar diagrams.
As shows in above graphs expnediture towards rent bill is highest while expenses on food
other than grocery is minimum as compre to other.
Calculation and discussion on following aspects:
rectangle/column refers to the attributes displayed by each class. This is usually termed as
vertical bar diagrams.
As shows in above graphs expnediture towards rent bill is highest while expenses on food
other than grocery is minimum as compre to other.
Calculation and discussion on following aspects:
Secure Best Marks with AI Grader
Need help grading? Try our AI Grader for instant feedback on your assignments.
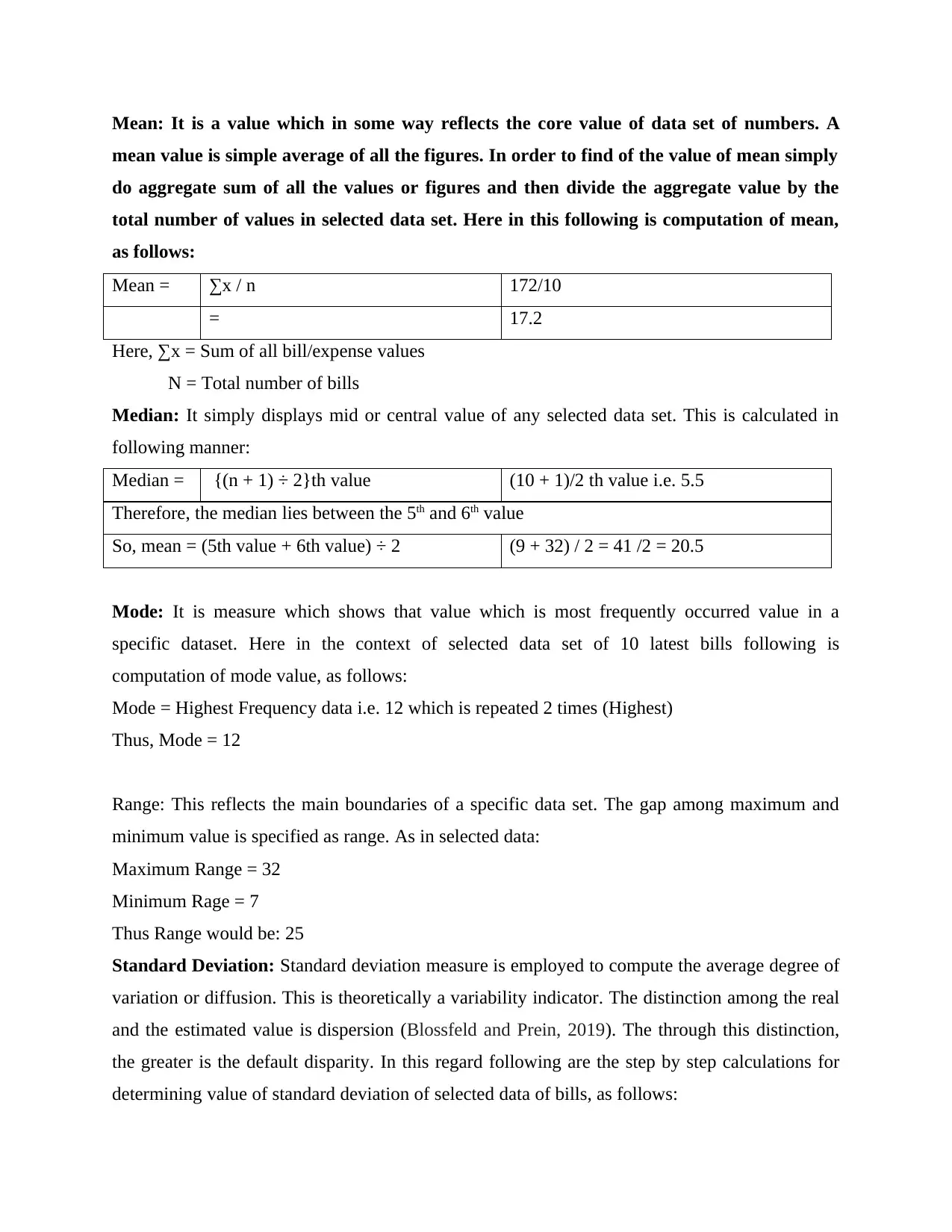
Mean: It is a value which in some way reflects the core value of data set of numbers. A
mean value is simple average of all the figures. In order to find of the value of mean simply
do aggregate sum of all the values or figures and then divide the aggregate value by the
total number of values in selected data set. Here in this following is computation of mean,
as follows:
Mean = ∑x / n 172/10
= 17.2
Here, ∑x = Sum of all bill/expense values
N = Total number of bills
Median: It simply displays mid or central value of any selected data set. This is calculated in
following manner:
Median = {(n + 1) ÷ 2}th value (10 + 1)/2 th value i.e. 5.5
Therefore, the median lies between the 5th and 6th value
So, mean = (5th value + 6th value) ÷ 2 (9 + 32) / 2 = 41 /2 = 20.5
Mode: It is measure which shows that value which is most frequently occurred value in a
specific dataset. Here in the context of selected data set of 10 latest bills following is
computation of mode value, as follows:
Mode = Highest Frequency data i.e. 12 which is repeated 2 times (Highest)
Thus, Mode = 12
Range: This reflects the main boundaries of a specific data set. The gap among maximum and
minimum value is specified as range. As in selected data:
Maximum Range = 32
Minimum Rage = 7
Thus Range would be: 25
Standard Deviation: Standard deviation measure is employed to compute the average degree of
variation or diffusion. This is theoretically a variability indicator. The distinction among the real
and the estimated value is dispersion (Blossfeld and Prein, 2019). The through this distinction,
the greater is the default disparity. In this regard following are the step by step calculations for
determining value of standard deviation of selected data of bills, as follows:
mean value is simple average of all the figures. In order to find of the value of mean simply
do aggregate sum of all the values or figures and then divide the aggregate value by the
total number of values in selected data set. Here in this following is computation of mean,
as follows:
Mean = ∑x / n 172/10
= 17.2
Here, ∑x = Sum of all bill/expense values
N = Total number of bills
Median: It simply displays mid or central value of any selected data set. This is calculated in
following manner:
Median = {(n + 1) ÷ 2}th value (10 + 1)/2 th value i.e. 5.5
Therefore, the median lies between the 5th and 6th value
So, mean = (5th value + 6th value) ÷ 2 (9 + 32) / 2 = 41 /2 = 20.5
Mode: It is measure which shows that value which is most frequently occurred value in a
specific dataset. Here in the context of selected data set of 10 latest bills following is
computation of mode value, as follows:
Mode = Highest Frequency data i.e. 12 which is repeated 2 times (Highest)
Thus, Mode = 12
Range: This reflects the main boundaries of a specific data set. The gap among maximum and
minimum value is specified as range. As in selected data:
Maximum Range = 32
Minimum Rage = 7
Thus Range would be: 25
Standard Deviation: Standard deviation measure is employed to compute the average degree of
variation or diffusion. This is theoretically a variability indicator. The distinction among the real
and the estimated value is dispersion (Blossfeld and Prein, 2019). The through this distinction,
the greater is the default disparity. In this regard following are the step by step calculations for
determining value of standard deviation of selected data of bills, as follows:
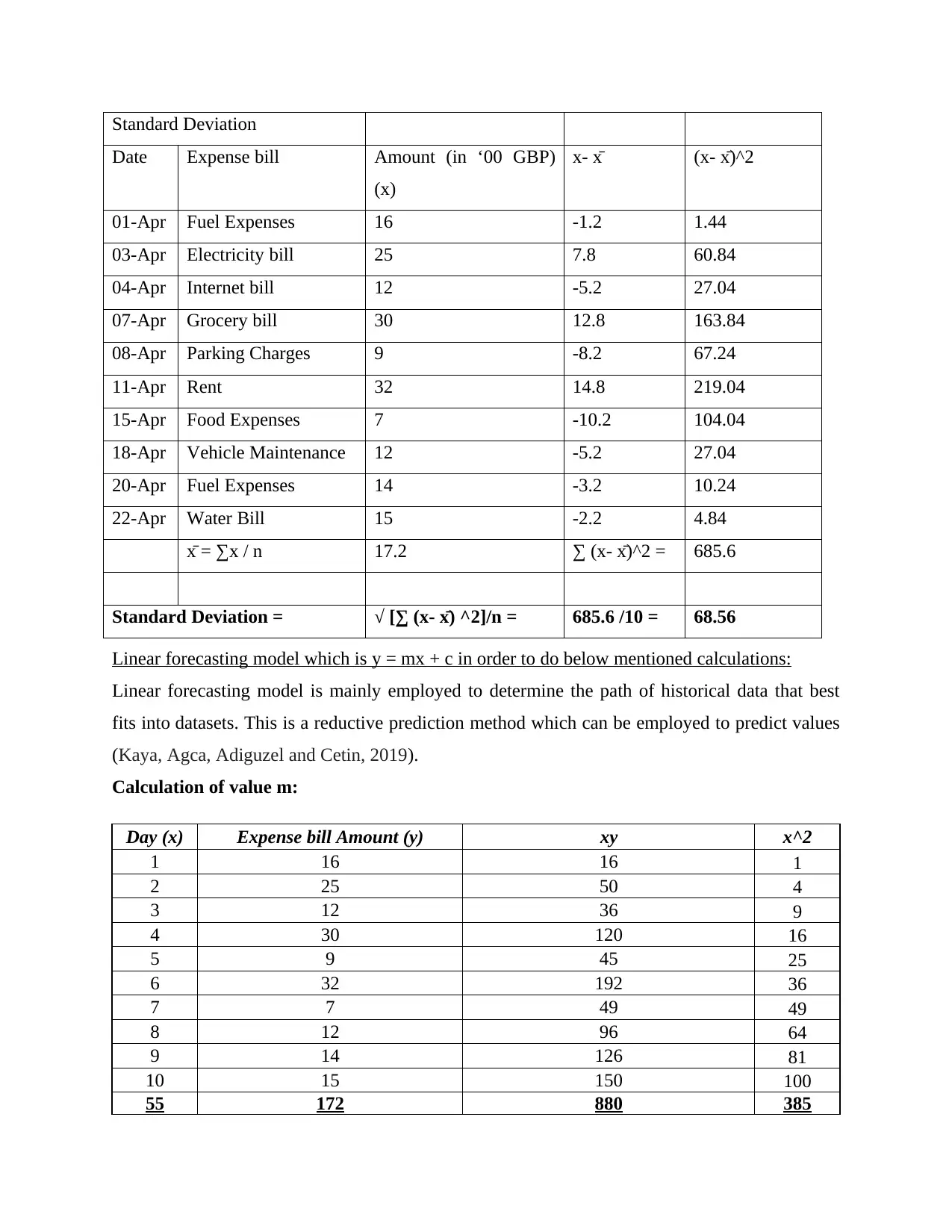
Standard Deviation
Date Expense bill Amount (in ‘00 GBP)
(x)
x- x̄ (x- x̄)^2
01-Apr Fuel Expenses 16 -1.2 1.44
03-Apr Electricity bill 25 7.8 60.84
04-Apr Internet bill 12 -5.2 27.04
07-Apr Grocery bill 30 12.8 163.84
08-Apr Parking Charges 9 -8.2 67.24
11-Apr Rent 32 14.8 219.04
15-Apr Food Expenses 7 -10.2 104.04
18-Apr Vehicle Maintenance 12 -5.2 27.04
20-Apr Fuel Expenses 14 -3.2 10.24
22-Apr Water Bill 15 -2.2 4.84
x̄ = ∑x / n 17.2 ∑ (x- x̄)^2 = 685.6
Standard Deviation = √ [∑ (x- x̄) ^2]/n = 685.6 /10 = 68.56
Linear forecasting model which is y = mx + c in order to do below mentioned calculations:
Linear forecasting model is mainly employed to determine the path of historical data that best
fits into datasets. This is a reductive prediction method which can be employed to predict values
(Kaya, Agca, Adiguzel and Cetin, 2019).
Calculation of value m:
Day (x) Expense bill Amount (y) xy x^2
1 16 16 1
2 25 50 4
3 12 36 9
4 30 120 16
5 9 45 25
6 32 192 36
7 7 49 49
8 12 96 64
9 14 126 81
10 15 150 100
55 172 880 385
Date Expense bill Amount (in ‘00 GBP)
(x)
x- x̄ (x- x̄)^2
01-Apr Fuel Expenses 16 -1.2 1.44
03-Apr Electricity bill 25 7.8 60.84
04-Apr Internet bill 12 -5.2 27.04
07-Apr Grocery bill 30 12.8 163.84
08-Apr Parking Charges 9 -8.2 67.24
11-Apr Rent 32 14.8 219.04
15-Apr Food Expenses 7 -10.2 104.04
18-Apr Vehicle Maintenance 12 -5.2 27.04
20-Apr Fuel Expenses 14 -3.2 10.24
22-Apr Water Bill 15 -2.2 4.84
x̄ = ∑x / n 17.2 ∑ (x- x̄)^2 = 685.6
Standard Deviation = √ [∑ (x- x̄) ^2]/n = 685.6 /10 = 68.56
Linear forecasting model which is y = mx + c in order to do below mentioned calculations:
Linear forecasting model is mainly employed to determine the path of historical data that best
fits into datasets. This is a reductive prediction method which can be employed to predict values
(Kaya, Agca, Adiguzel and Cetin, 2019).
Calculation of value m:
Day (x) Expense bill Amount (y) xy x^2
1 16 16 1
2 25 50 4
3 12 36 9
4 30 120 16
5 9 45 25
6 32 192 36
7 7 49 49
8 12 96 64
9 14 126 81
10 15 150 100
55 172 880 385
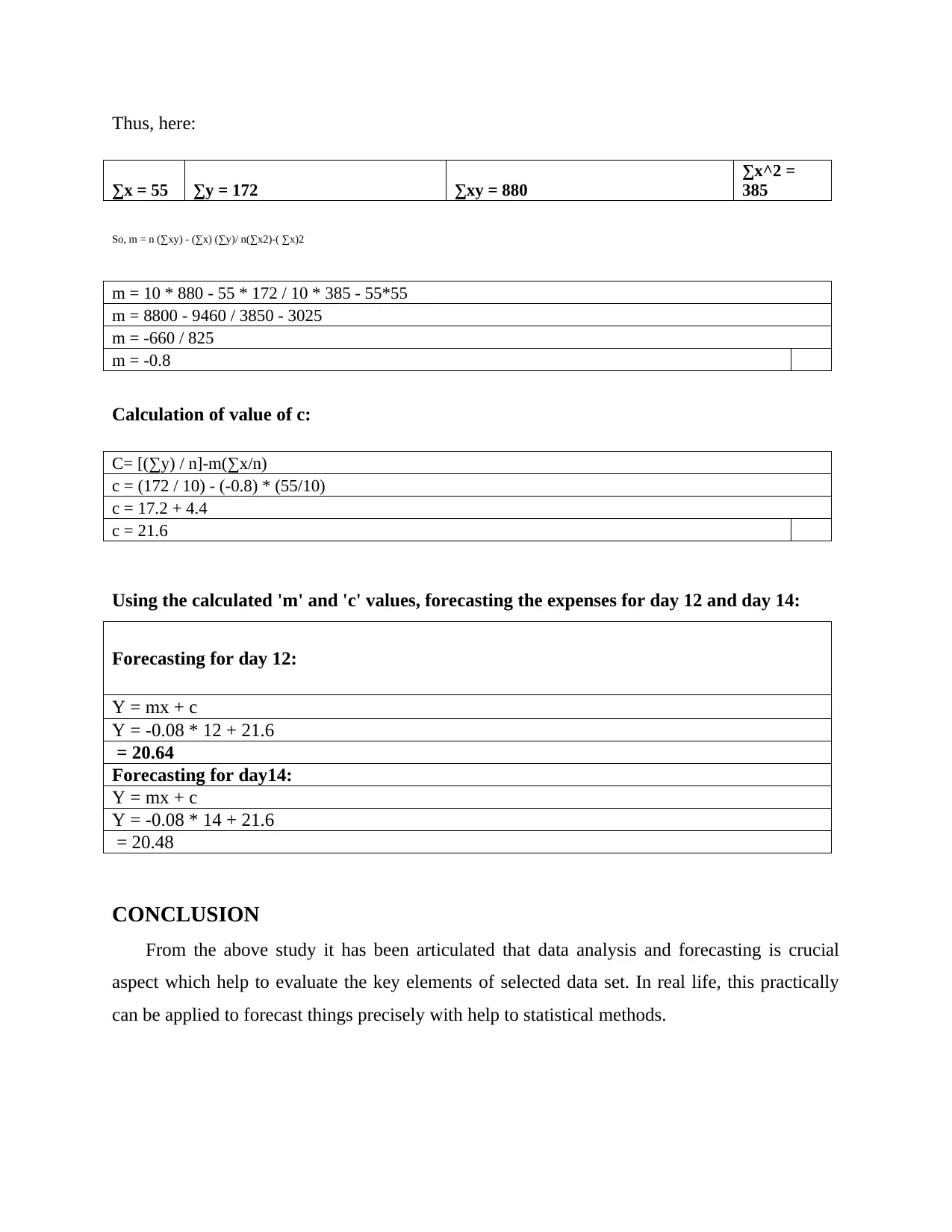
Thus, here:
∑x = 55 ∑y = 172 ∑xy = 880
∑x^2 =
385
So, m = n (∑xy) - (∑x) (∑y)/ n(∑x2)-( ∑x)2
m = 10 * 880 - 55 * 172 / 10 * 385 - 55*55
m = 8800 - 9460 / 3850 - 3025
m = -660 / 825
m = -0.8
Calculation of value of c:
C= [(∑y) / n]-m(∑x/n)
c = (172 / 10) - (-0.8) * (55/10)
c = 17.2 + 4.4
c = 21.6
Using the calculated 'm' and 'c' values, forecasting the expenses for day 12 and day 14:
Forecasting for day 12:
Y = mx + c
Y = -0.08 * 12 + 21.6
= 20.64
Forecasting for day14:
Y = mx + c
Y = -0.08 * 14 + 21.6
= 20.48
CONCLUSION
From the above study it has been articulated that data analysis and forecasting is crucial
aspect which help to evaluate the key elements of selected data set. In real life, this practically
can be applied to forecast things precisely with help to statistical methods.
∑x = 55 ∑y = 172 ∑xy = 880
∑x^2 =
385
So, m = n (∑xy) - (∑x) (∑y)/ n(∑x2)-( ∑x)2
m = 10 * 880 - 55 * 172 / 10 * 385 - 55*55
m = 8800 - 9460 / 3850 - 3025
m = -660 / 825
m = -0.8
Calculation of value of c:
C= [(∑y) / n]-m(∑x/n)
c = (172 / 10) - (-0.8) * (55/10)
c = 17.2 + 4.4
c = 21.6
Using the calculated 'm' and 'c' values, forecasting the expenses for day 12 and day 14:
Forecasting for day 12:
Y = mx + c
Y = -0.08 * 12 + 21.6
= 20.64
Forecasting for day14:
Y = mx + c
Y = -0.08 * 14 + 21.6
= 20.48
CONCLUSION
From the above study it has been articulated that data analysis and forecasting is crucial
aspect which help to evaluate the key elements of selected data set. In real life, this practically
can be applied to forecast things precisely with help to statistical methods.
Paraphrase This Document
Need a fresh take? Get an instant paraphrase of this document with our AI Paraphraser
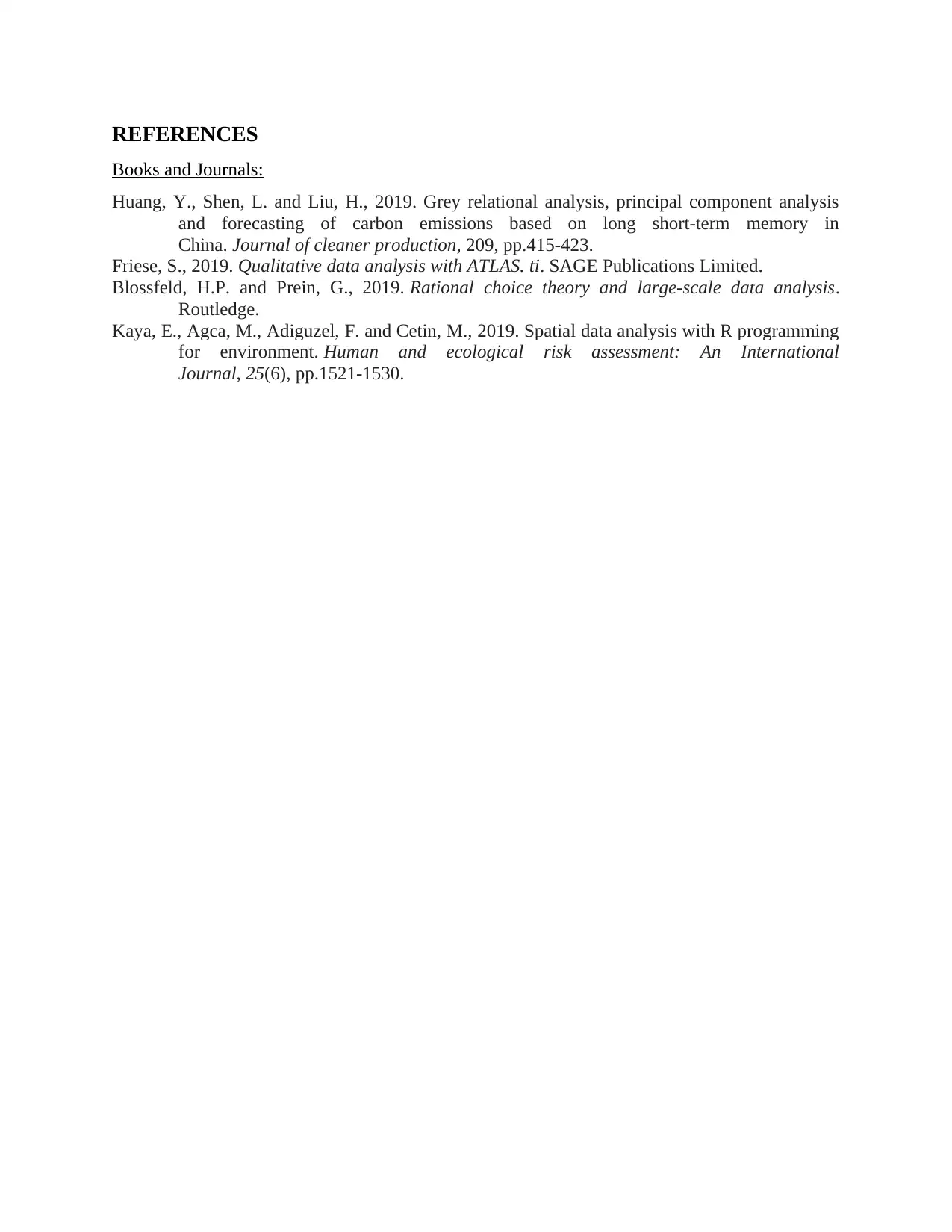
REFERENCES
Books and Journals:
Huang, Y., Shen, L. and Liu, H., 2019. Grey relational analysis, principal component analysis
and forecasting of carbon emissions based on long short-term memory in
China. Journal of cleaner production, 209, pp.415-423.
Friese, S., 2019. Qualitative data analysis with ATLAS. ti. SAGE Publications Limited.
Blossfeld, H.P. and Prein, G., 2019. Rational choice theory and large-scale data analysis.
Routledge.
Kaya, E., Agca, M., Adiguzel, F. and Cetin, M., 2019. Spatial data analysis with R programming
for environment. Human and ecological risk assessment: An International
Journal, 25(6), pp.1521-1530.
Books and Journals:
Huang, Y., Shen, L. and Liu, H., 2019. Grey relational analysis, principal component analysis
and forecasting of carbon emissions based on long short-term memory in
China. Journal of cleaner production, 209, pp.415-423.
Friese, S., 2019. Qualitative data analysis with ATLAS. ti. SAGE Publications Limited.
Blossfeld, H.P. and Prein, G., 2019. Rational choice theory and large-scale data analysis.
Routledge.
Kaya, E., Agca, M., Adiguzel, F. and Cetin, M., 2019. Spatial data analysis with R programming
for environment. Human and ecological risk assessment: An International
Journal, 25(6), pp.1521-1530.
1 out of 8
Related Documents
![[object Object]](/_next/image/?url=%2F_next%2Fstatic%2Fmedia%2Flogo.6d15ce61.png&w=640&q=75)
Your All-in-One AI-Powered Toolkit for Academic Success.
+13062052269
info@desklib.com
Available 24*7 on WhatsApp / Email
Unlock your academic potential
© 2024 | Zucol Services PVT LTD | All rights reserved.