Data Analysis & Forecasting: A Sleeping Hours Statistical Report
VerifiedAdded on 2023/06/18
|12
|1571
|479
Report
AI Summary
This report provides a comprehensive analysis of sleeping hours using numeracy and data analysis techniques. It includes arranging the data in a tabular format, presenting it in pictorial formats like bar and line charts, and performing calculations to determine the mean (8.5 hours), median (8.25), ...
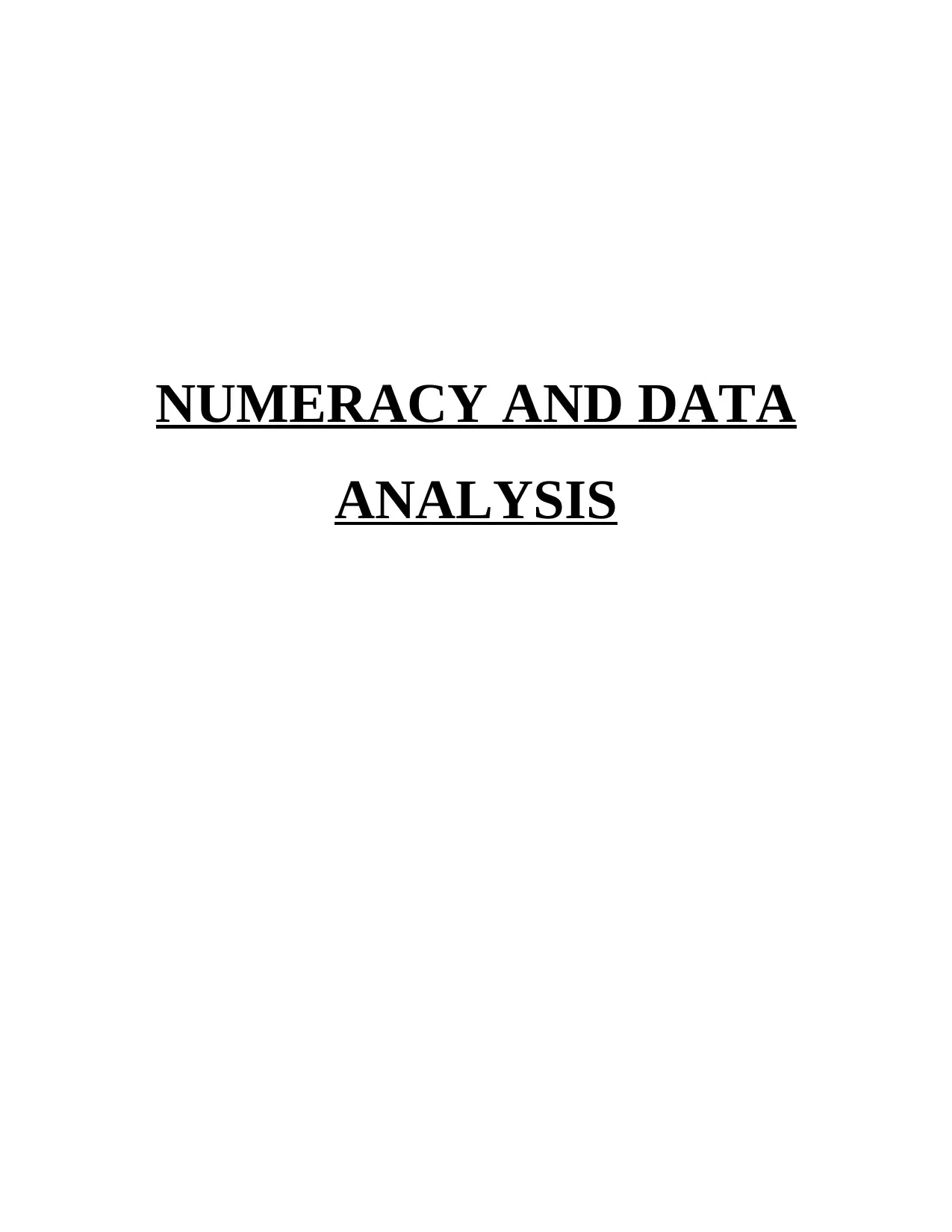
NUMERACY AND DATA
ANALYSIS
ANALYSIS
Paraphrase This Document
Need a fresh take? Get an instant paraphrase of this document with our AI Paraphraser
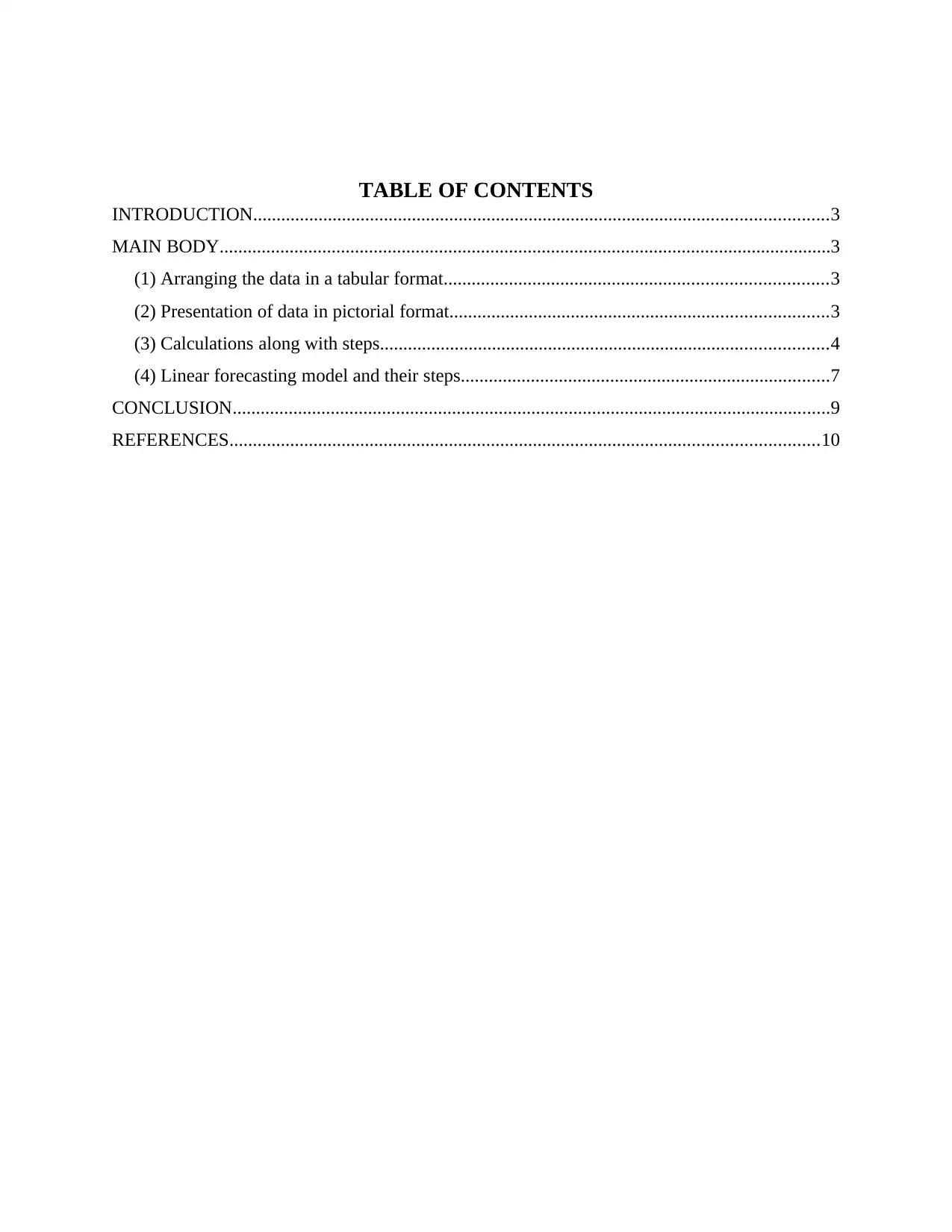
TABLE OF CONTENTS
INTRODUCTION...........................................................................................................................3
MAIN BODY...................................................................................................................................3
(1) Arranging the data in a tabular format..................................................................................3
(2) Presentation of data in pictorial format.................................................................................3
(3) Calculations along with steps................................................................................................4
(4) Linear forecasting model and their steps...............................................................................7
CONCLUSION................................................................................................................................9
REFERENCES..............................................................................................................................10
INTRODUCTION...........................................................................................................................3
MAIN BODY...................................................................................................................................3
(1) Arranging the data in a tabular format..................................................................................3
(2) Presentation of data in pictorial format.................................................................................3
(3) Calculations along with steps................................................................................................4
(4) Linear forecasting model and their steps...............................................................................7
CONCLUSION................................................................................................................................9
REFERENCES..............................................................................................................................10
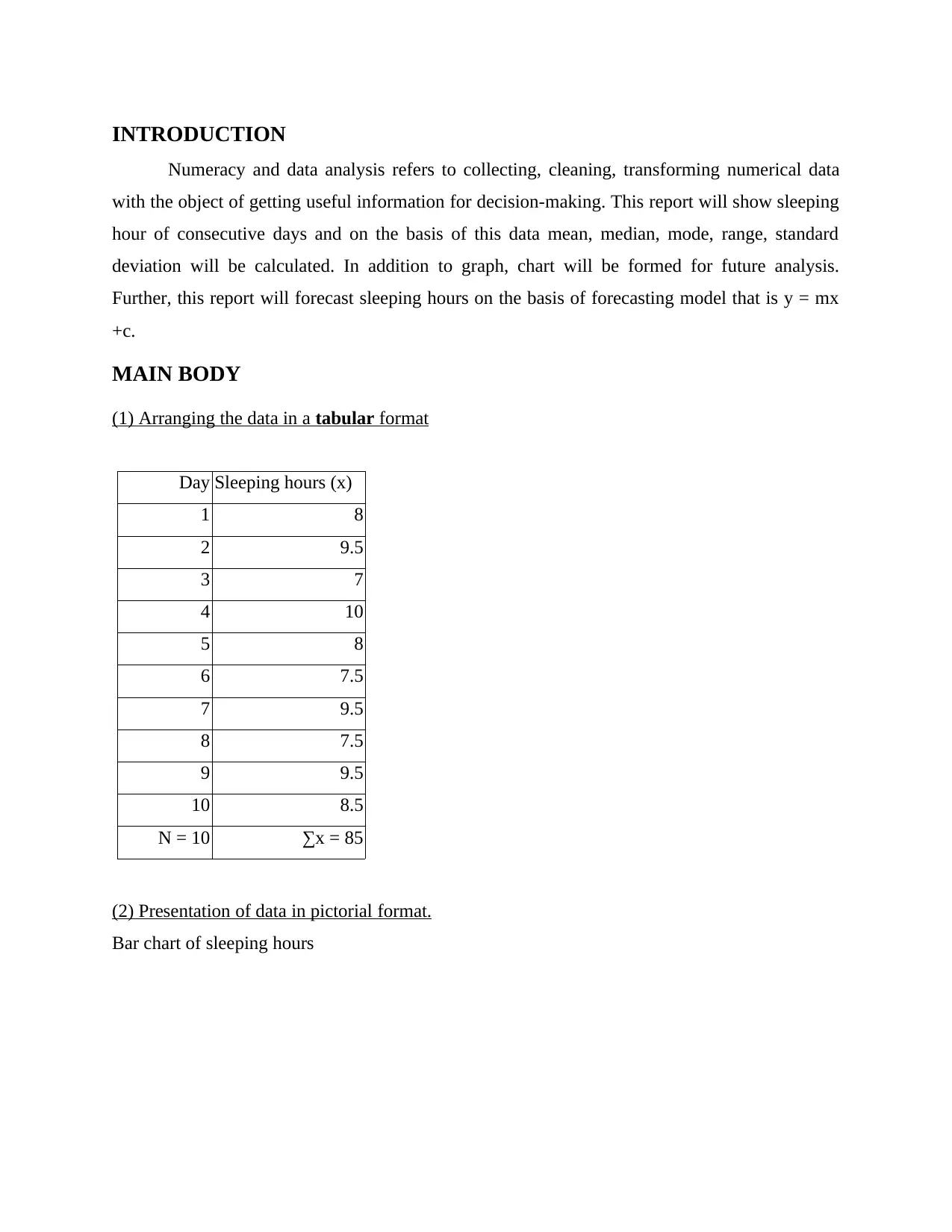
INTRODUCTION
Numeracy and data analysis refers to collecting, cleaning, transforming numerical data
with the object of getting useful information for decision-making. This report will show sleeping
hour of consecutive days and on the basis of this data mean, median, mode, range, standard
deviation will be calculated. In addition to graph, chart will be formed for future analysis.
Further, this report will forecast sleeping hours on the basis of forecasting model that is y = mx
+c.
MAIN BODY
(1) Arranging the data in a tabular format
Day Sleeping hours (x)
1 8
2 9.5
3 7
4 10
5 8
6 7.5
7 9.5
8 7.5
9 9.5
10 8.5
N = 10 ∑x = 85
(2) Presentation of data in pictorial format.
Bar chart of sleeping hours
Numeracy and data analysis refers to collecting, cleaning, transforming numerical data
with the object of getting useful information for decision-making. This report will show sleeping
hour of consecutive days and on the basis of this data mean, median, mode, range, standard
deviation will be calculated. In addition to graph, chart will be formed for future analysis.
Further, this report will forecast sleeping hours on the basis of forecasting model that is y = mx
+c.
MAIN BODY
(1) Arranging the data in a tabular format
Day Sleeping hours (x)
1 8
2 9.5
3 7
4 10
5 8
6 7.5
7 9.5
8 7.5
9 9.5
10 8.5
N = 10 ∑x = 85
(2) Presentation of data in pictorial format.
Bar chart of sleeping hours
⊘ This is a preview!⊘
Do you want full access?
Subscribe today to unlock all pages.

Trusted by 1+ million students worldwide
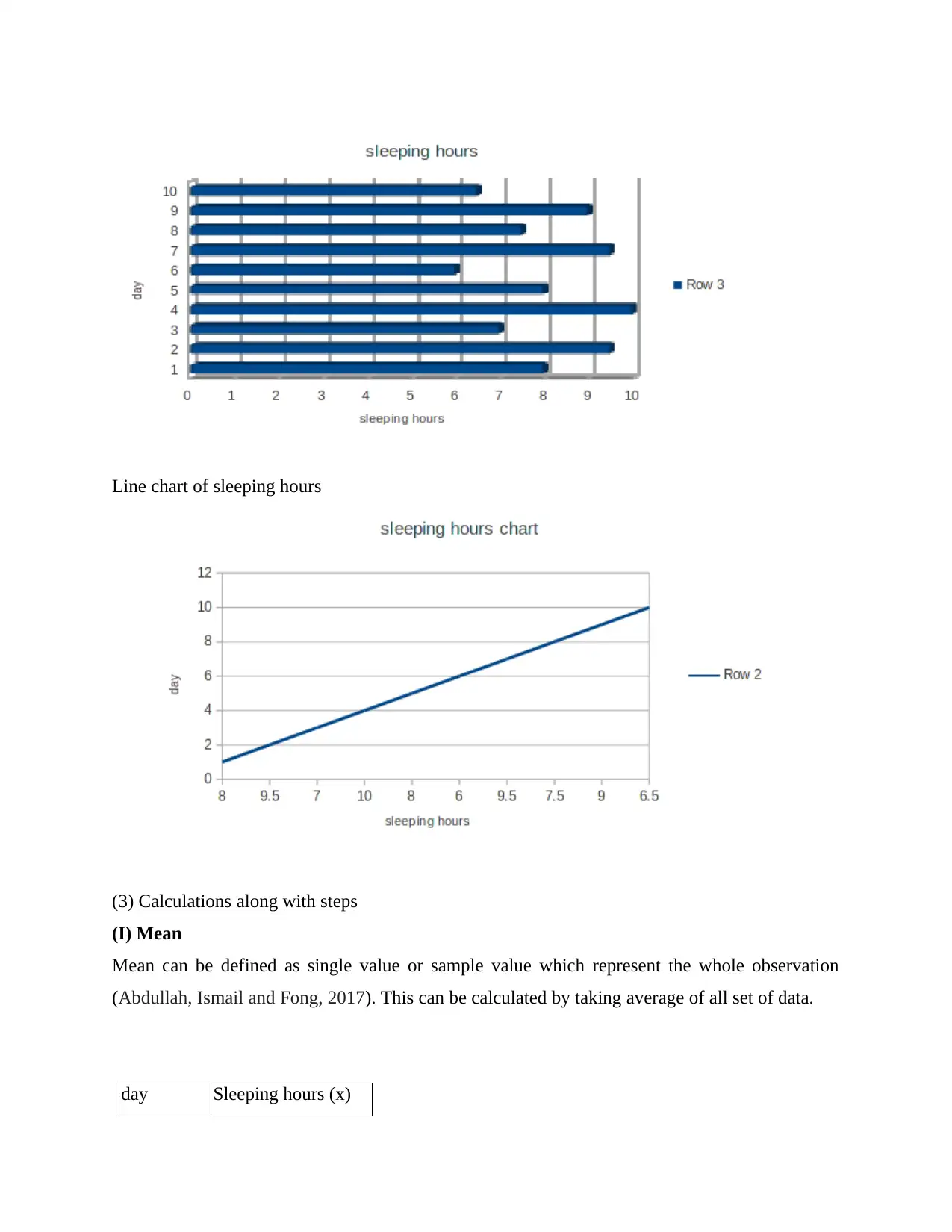
Line chart of sleeping hours
(3) Calculations along with steps
(I) Mean
Mean can be defined as single value or sample value which represent the whole observation
(Abdullah, Ismail and Fong, 2017). This can be calculated by taking average of all set of data.
day Sleeping hours (x)
(3) Calculations along with steps
(I) Mean
Mean can be defined as single value or sample value which represent the whole observation
(Abdullah, Ismail and Fong, 2017). This can be calculated by taking average of all set of data.
day Sleeping hours (x)
Paraphrase This Document
Need a fresh take? Get an instant paraphrase of this document with our AI Paraphraser
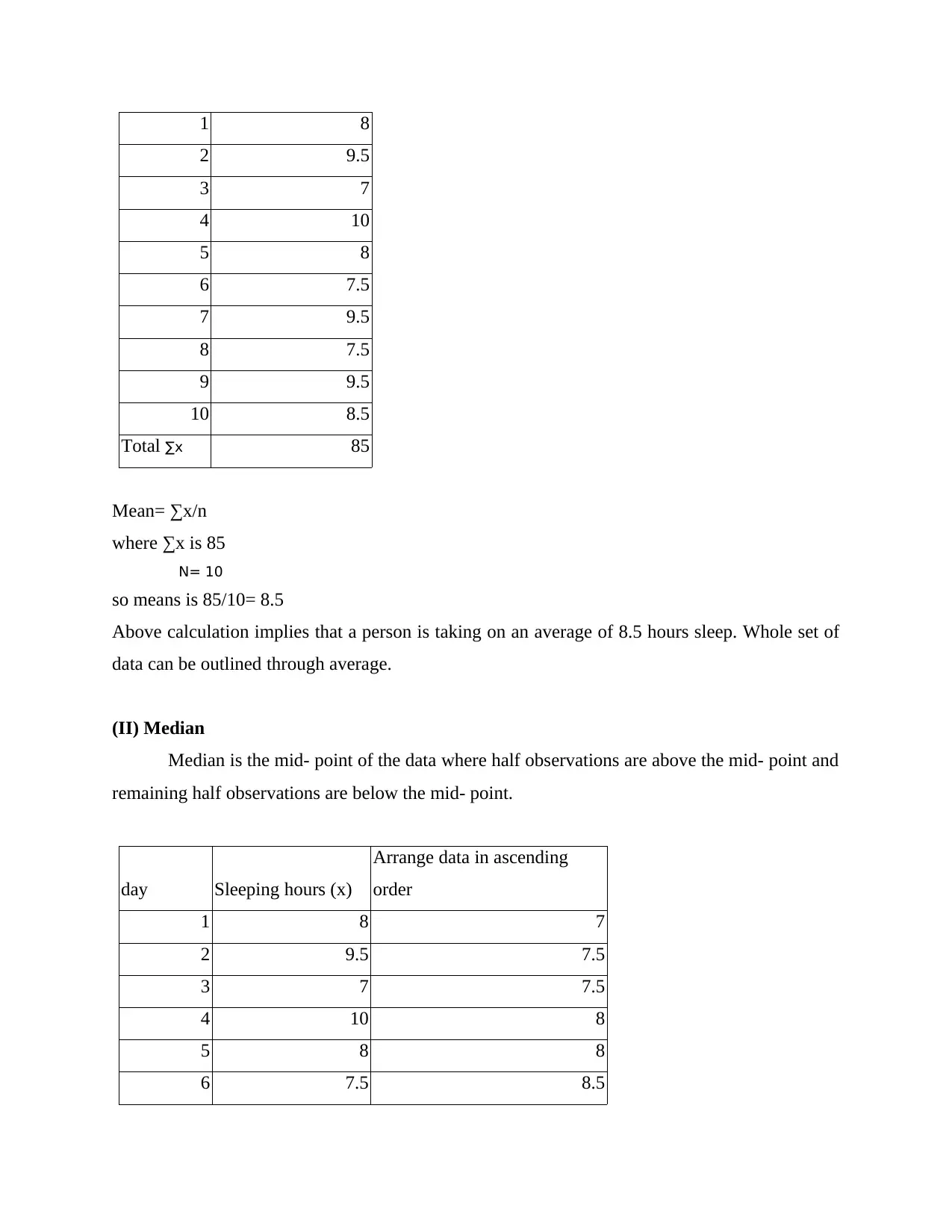
1 8
2 9.5
3 7
4 10
5 8
6 7.5
7 9.5
8 7.5
9 9.5
10 8.5
Total ∑x 85
Mean= ∑x/n
where ∑x is 85
N= 10
so means is 85/10= 8.5
Above calculation implies that a person is taking on an average of 8.5 hours sleep. Whole set of
data can be outlined through average.
(II) Median
Median is the mid- point of the data where half observations are above the mid- point and
remaining half observations are below the mid- point.
day Sleeping hours (x)
Arrange data in ascending
order
1 8 7
2 9.5 7.5
3 7 7.5
4 10 8
5 8 8
6 7.5 8.5
2 9.5
3 7
4 10
5 8
6 7.5
7 9.5
8 7.5
9 9.5
10 8.5
Total ∑x 85
Mean= ∑x/n
where ∑x is 85
N= 10
so means is 85/10= 8.5
Above calculation implies that a person is taking on an average of 8.5 hours sleep. Whole set of
data can be outlined through average.
(II) Median
Median is the mid- point of the data where half observations are above the mid- point and
remaining half observations are below the mid- point.
day Sleeping hours (x)
Arrange data in ascending
order
1 8 7
2 9.5 7.5
3 7 7.5
4 10 8
5 8 8
6 7.5 8.5
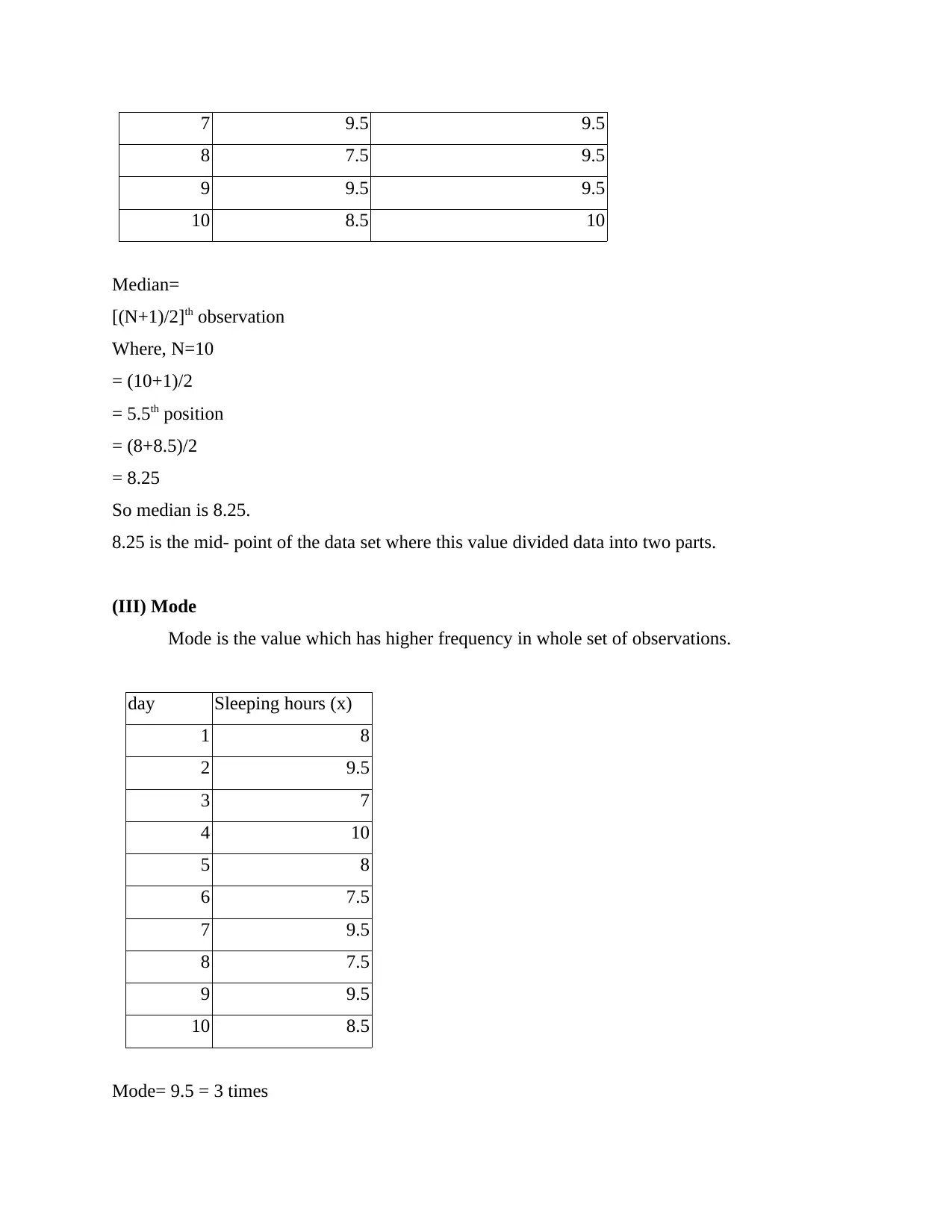
7 9.5 9.5
8 7.5 9.5
9 9.5 9.5
10 8.5 10
Median=
[(N+1)/2]th observation
Where, N=10
= (10+1)/2
= 5.5th position
= (8+8.5)/2
= 8.25
So median is 8.25.
8.25 is the mid- point of the data set where this value divided data into two parts.
(III) Mode
Mode is the value which has higher frequency in whole set of observations.
day Sleeping hours (x)
1 8
2 9.5
3 7
4 10
5 8
6 7.5
7 9.5
8 7.5
9 9.5
10 8.5
Mode= 9.5 = 3 times
8 7.5 9.5
9 9.5 9.5
10 8.5 10
Median=
[(N+1)/2]th observation
Where, N=10
= (10+1)/2
= 5.5th position
= (8+8.5)/2
= 8.25
So median is 8.25.
8.25 is the mid- point of the data set where this value divided data into two parts.
(III) Mode
Mode is the value which has higher frequency in whole set of observations.
day Sleeping hours (x)
1 8
2 9.5
3 7
4 10
5 8
6 7.5
7 9.5
8 7.5
9 9.5
10 8.5
Mode= 9.5 = 3 times
⊘ This is a preview!⊘
Do you want full access?
Subscribe today to unlock all pages.

Trusted by 1+ million students worldwide
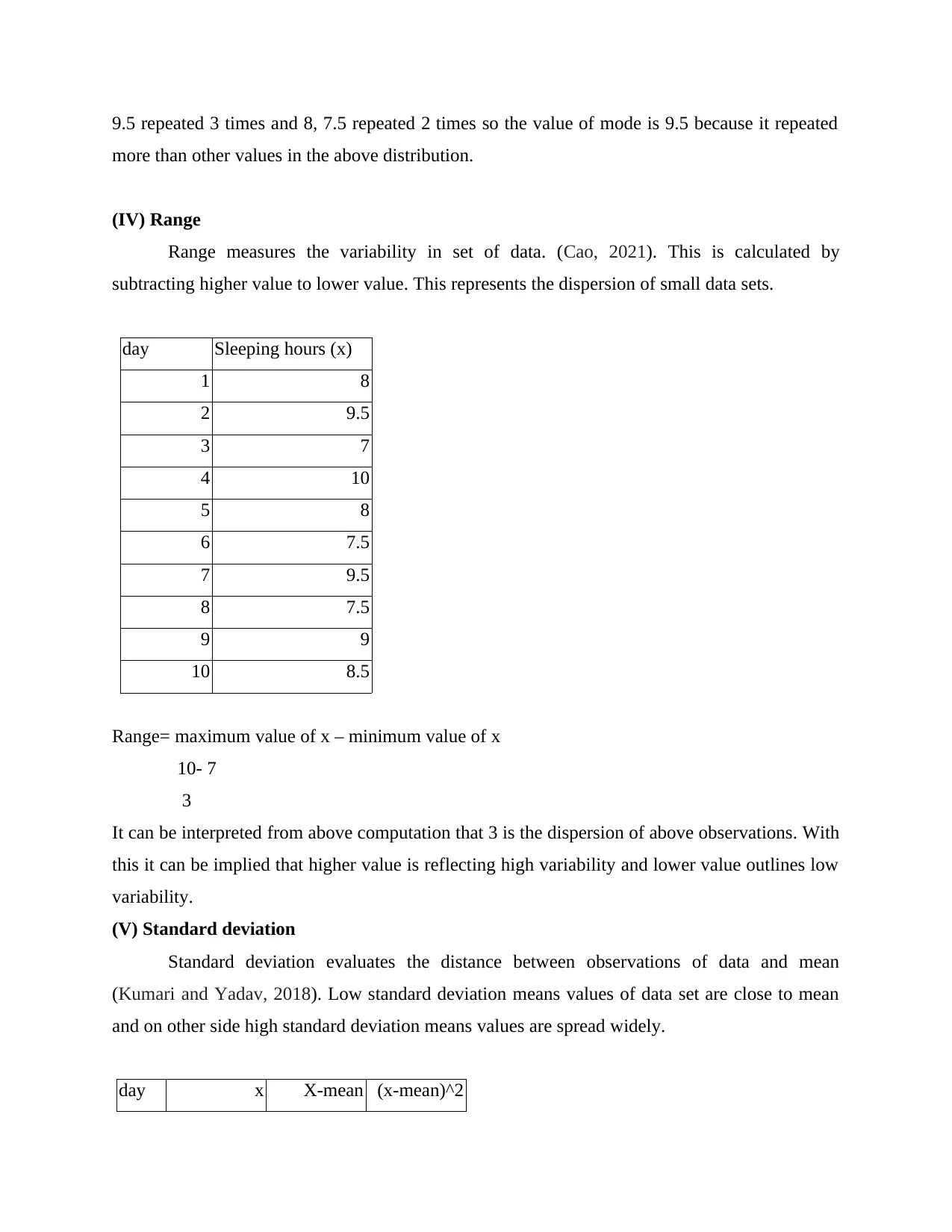
9.5 repeated 3 times and 8, 7.5 repeated 2 times so the value of mode is 9.5 because it repeated
more than other values in the above distribution.
(IV) Range
Range measures the variability in set of data. (Cao, 2021). This is calculated by
subtracting higher value to lower value. This represents the dispersion of small data sets.
day Sleeping hours (x)
1 8
2 9.5
3 7
4 10
5 8
6 7.5
7 9.5
8 7.5
9 9
10 8.5
Range= maximum value of x – minimum value of x
10- 7
3
It can be interpreted from above computation that 3 is the dispersion of above observations. With
this it can be implied that higher value is reflecting high variability and lower value outlines low
variability.
(V) Standard deviation
Standard deviation evaluates the distance between observations of data and mean
(Kumari and Yadav, 2018). Low standard deviation means values of data set are close to mean
and on other side high standard deviation means values are spread widely.
day x X-mean (x-mean)^2
more than other values in the above distribution.
(IV) Range
Range measures the variability in set of data. (Cao, 2021). This is calculated by
subtracting higher value to lower value. This represents the dispersion of small data sets.
day Sleeping hours (x)
1 8
2 9.5
3 7
4 10
5 8
6 7.5
7 9.5
8 7.5
9 9
10 8.5
Range= maximum value of x – minimum value of x
10- 7
3
It can be interpreted from above computation that 3 is the dispersion of above observations. With
this it can be implied that higher value is reflecting high variability and lower value outlines low
variability.
(V) Standard deviation
Standard deviation evaluates the distance between observations of data and mean
(Kumari and Yadav, 2018). Low standard deviation means values of data set are close to mean
and on other side high standard deviation means values are spread widely.
day x X-mean (x-mean)^2
Paraphrase This Document
Need a fresh take? Get an instant paraphrase of this document with our AI Paraphraser
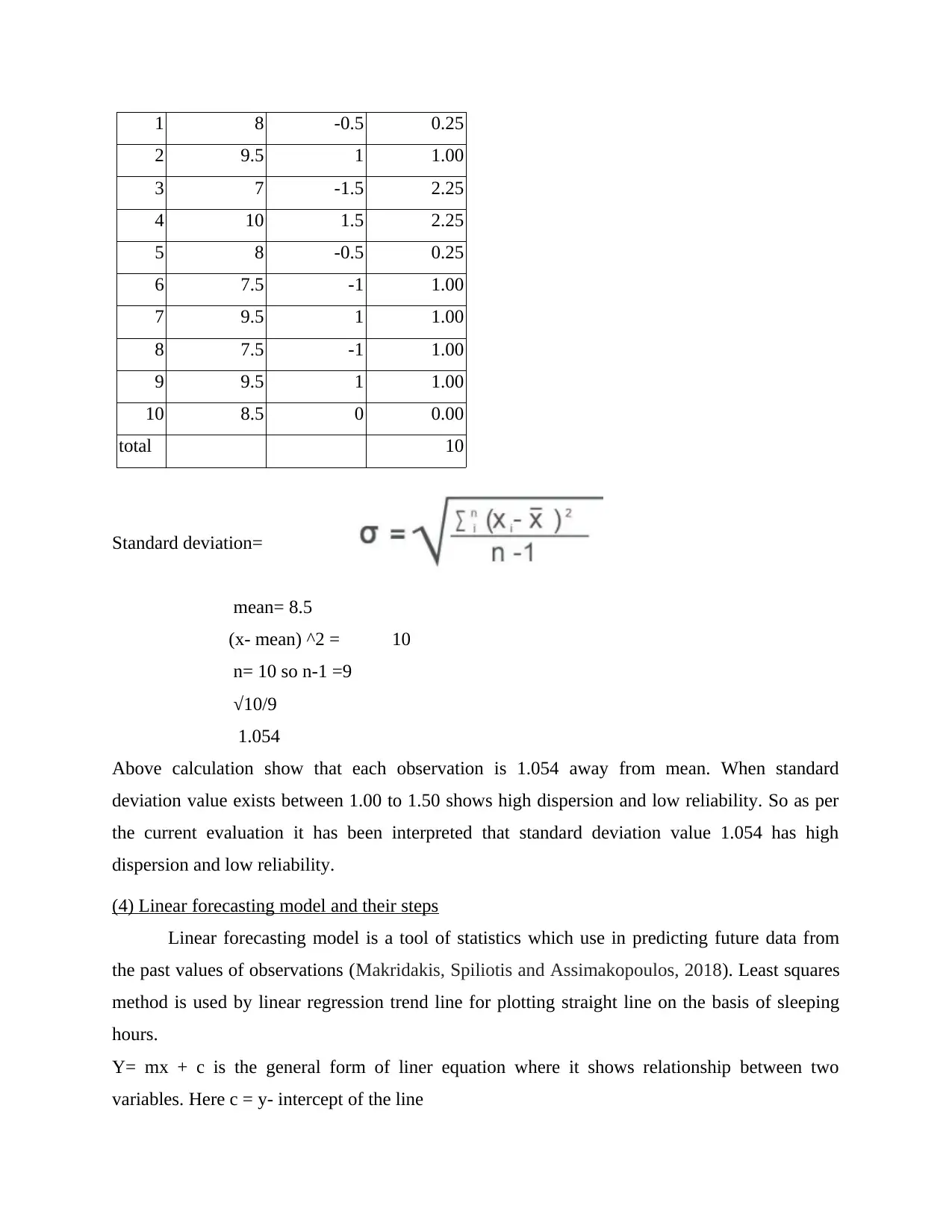
1 8 -0.5 0.25
2 9.5 1 1.00
3 7 -1.5 2.25
4 10 1.5 2.25
5 8 -0.5 0.25
6 7.5 -1 1.00
7 9.5 1 1.00
8 7.5 -1 1.00
9 9.5 1 1.00
10 8.5 0 0.00
total 10
Standard deviation=
mean= 8.5
(x- mean) ^2 = 10
n= 10 so n-1 =9
√10/9
1.054
Above calculation show that each observation is 1.054 away from mean. When standard
deviation value exists between 1.00 to 1.50 shows high dispersion and low reliability. So as per
the current evaluation it has been interpreted that standard deviation value 1.054 has high
dispersion and low reliability.
(4) Linear forecasting model and their steps
Linear forecasting model is a tool of statistics which use in predicting future data from
the past values of observations (Makridakis, Spiliotis and Assimakopoulos, 2018). Least squares
method is used by linear regression trend line for plotting straight line on the basis of sleeping
hours.
Y= mx + c is the general form of liner equation where it shows relationship between two
variables. Here c = y- intercept of the line
2 9.5 1 1.00
3 7 -1.5 2.25
4 10 1.5 2.25
5 8 -0.5 0.25
6 7.5 -1 1.00
7 9.5 1 1.00
8 7.5 -1 1.00
9 9.5 1 1.00
10 8.5 0 0.00
total 10
Standard deviation=
mean= 8.5
(x- mean) ^2 = 10
n= 10 so n-1 =9
√10/9
1.054
Above calculation show that each observation is 1.054 away from mean. When standard
deviation value exists between 1.00 to 1.50 shows high dispersion and low reliability. So as per
the current evaluation it has been interpreted that standard deviation value 1.054 has high
dispersion and low reliability.
(4) Linear forecasting model and their steps
Linear forecasting model is a tool of statistics which use in predicting future data from
the past values of observations (Makridakis, Spiliotis and Assimakopoulos, 2018). Least squares
method is used by linear regression trend line for plotting straight line on the basis of sleeping
hours.
Y= mx + c is the general form of liner equation where it shows relationship between two
variables. Here c = y- intercept of the line
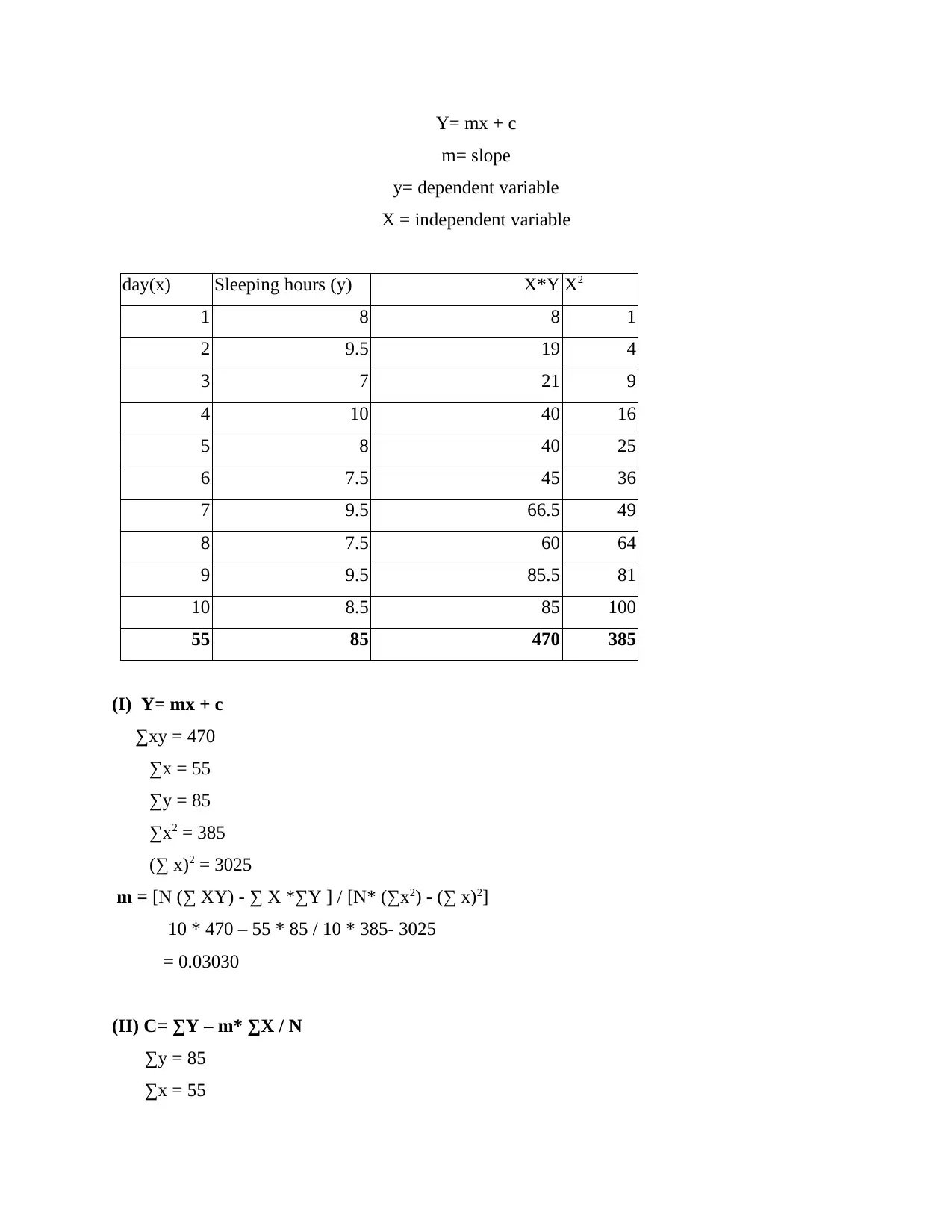
Y= mx + c
m= slope
y= dependent variable
X = independent variable
day(x) Sleeping hours (y) X*Y X2
1 8 8 1
2 9.5 19 4
3 7 21 9
4 10 40 16
5 8 40 25
6 7.5 45 36
7 9.5 66.5 49
8 7.5 60 64
9 9.5 85.5 81
10 8.5 85 100
55 85 470 385
(I) Y= mx + c
∑xy = 470
∑x = 55
∑y = 85
∑x2 = 385
(∑ x)2 = 3025
m = [N (∑ XY) - ∑ X *∑Y ] / [N* (∑x2) - (∑ x)2]
10 * 470 – 55 * 85 / 10 * 385- 3025
= 0.03030
(II) C= ∑Y – m* ∑X / N
∑y = 85
∑x = 55
m= slope
y= dependent variable
X = independent variable
day(x) Sleeping hours (y) X*Y X2
1 8 8 1
2 9.5 19 4
3 7 21 9
4 10 40 16
5 8 40 25
6 7.5 45 36
7 9.5 66.5 49
8 7.5 60 64
9 9.5 85.5 81
10 8.5 85 100
55 85 470 385
(I) Y= mx + c
∑xy = 470
∑x = 55
∑y = 85
∑x2 = 385
(∑ x)2 = 3025
m = [N (∑ XY) - ∑ X *∑Y ] / [N* (∑x2) - (∑ x)2]
10 * 470 – 55 * 85 / 10 * 385- 3025
= 0.03030
(II) C= ∑Y – m* ∑X / N
∑y = 85
∑x = 55
⊘ This is a preview!⊘
Do you want full access?
Subscribe today to unlock all pages.

Trusted by 1+ million students worldwide
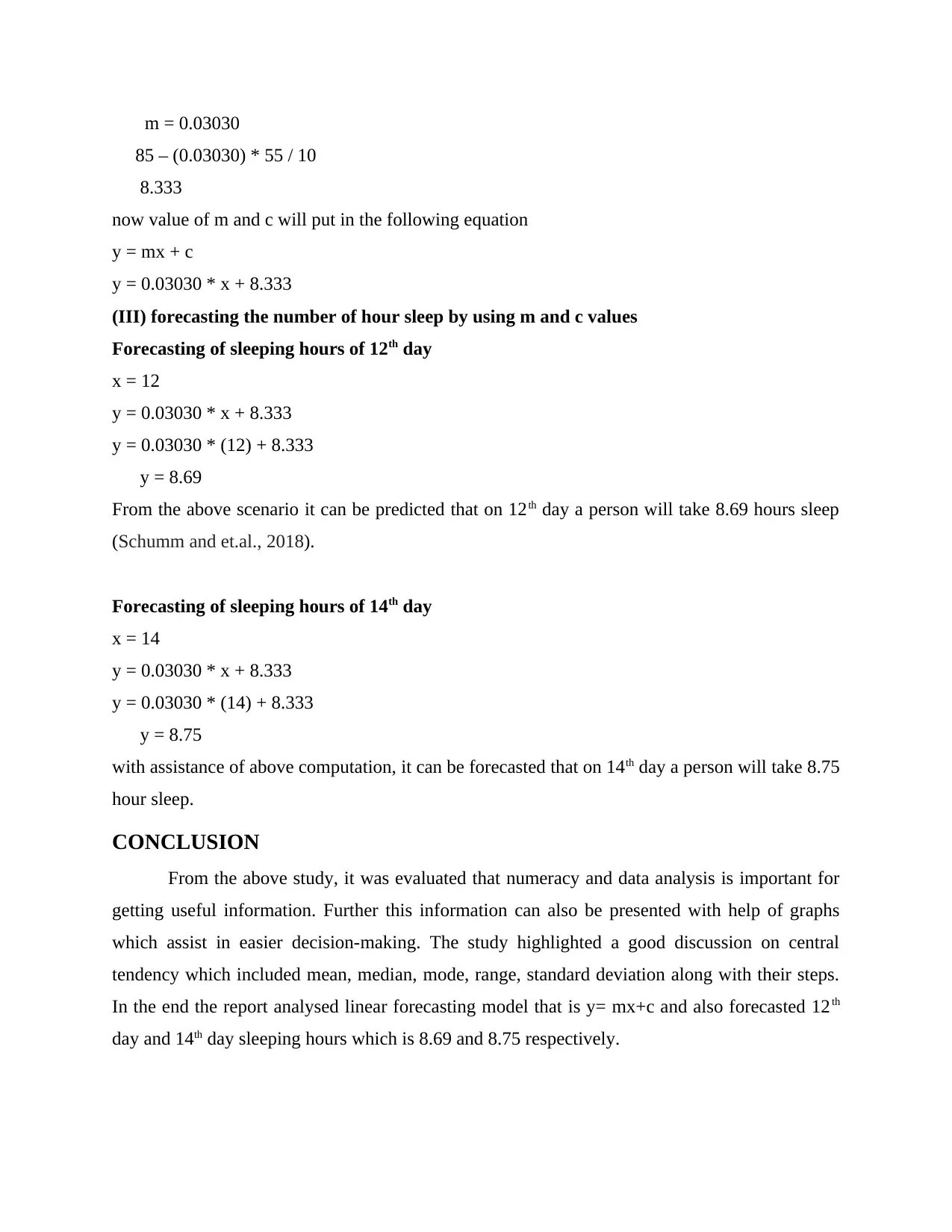
m = 0.03030
85 – (0.03030) * 55 / 10
8.333
now value of m and c will put in the following equation
y = mx + c
y = 0.03030 * x + 8.333
(III) forecasting the number of hour sleep by using m and c values
Forecasting of sleeping hours of 12th day
x = 12
y = 0.03030 * x + 8.333
y = 0.03030 * (12) + 8.333
y = 8.69
From the above scenario it can be predicted that on 12th day a person will take 8.69 hours sleep
(Schumm and et.al., 2018).
Forecasting of sleeping hours of 14th day
x = 14
y = 0.03030 * x + 8.333
y = 0.03030 * (14) + 8.333
y = 8.75
with assistance of above computation, it can be forecasted that on 14th day a person will take 8.75
hour sleep.
CONCLUSION
From the above study, it was evaluated that numeracy and data analysis is important for
getting useful information. Further this information can also be presented with help of graphs
which assist in easier decision-making. The study highlighted a good discussion on central
tendency which included mean, median, mode, range, standard deviation along with their steps.
In the end the report analysed linear forecasting model that is y= mx+c and also forecasted 12th
day and 14th day sleeping hours which is 8.69 and 8.75 respectively.
85 – (0.03030) * 55 / 10
8.333
now value of m and c will put in the following equation
y = mx + c
y = 0.03030 * x + 8.333
(III) forecasting the number of hour sleep by using m and c values
Forecasting of sleeping hours of 12th day
x = 12
y = 0.03030 * x + 8.333
y = 0.03030 * (12) + 8.333
y = 8.69
From the above scenario it can be predicted that on 12th day a person will take 8.69 hours sleep
(Schumm and et.al., 2018).
Forecasting of sleeping hours of 14th day
x = 14
y = 0.03030 * x + 8.333
y = 0.03030 * (14) + 8.333
y = 8.75
with assistance of above computation, it can be forecasted that on 14th day a person will take 8.75
hour sleep.
CONCLUSION
From the above study, it was evaluated that numeracy and data analysis is important for
getting useful information. Further this information can also be presented with help of graphs
which assist in easier decision-making. The study highlighted a good discussion on central
tendency which included mean, median, mode, range, standard deviation along with their steps.
In the end the report analysed linear forecasting model that is y= mx+c and also forecasted 12th
day and 14th day sleeping hours which is 8.69 and 8.75 respectively.
Paraphrase This Document
Need a fresh take? Get an instant paraphrase of this document with our AI Paraphraser
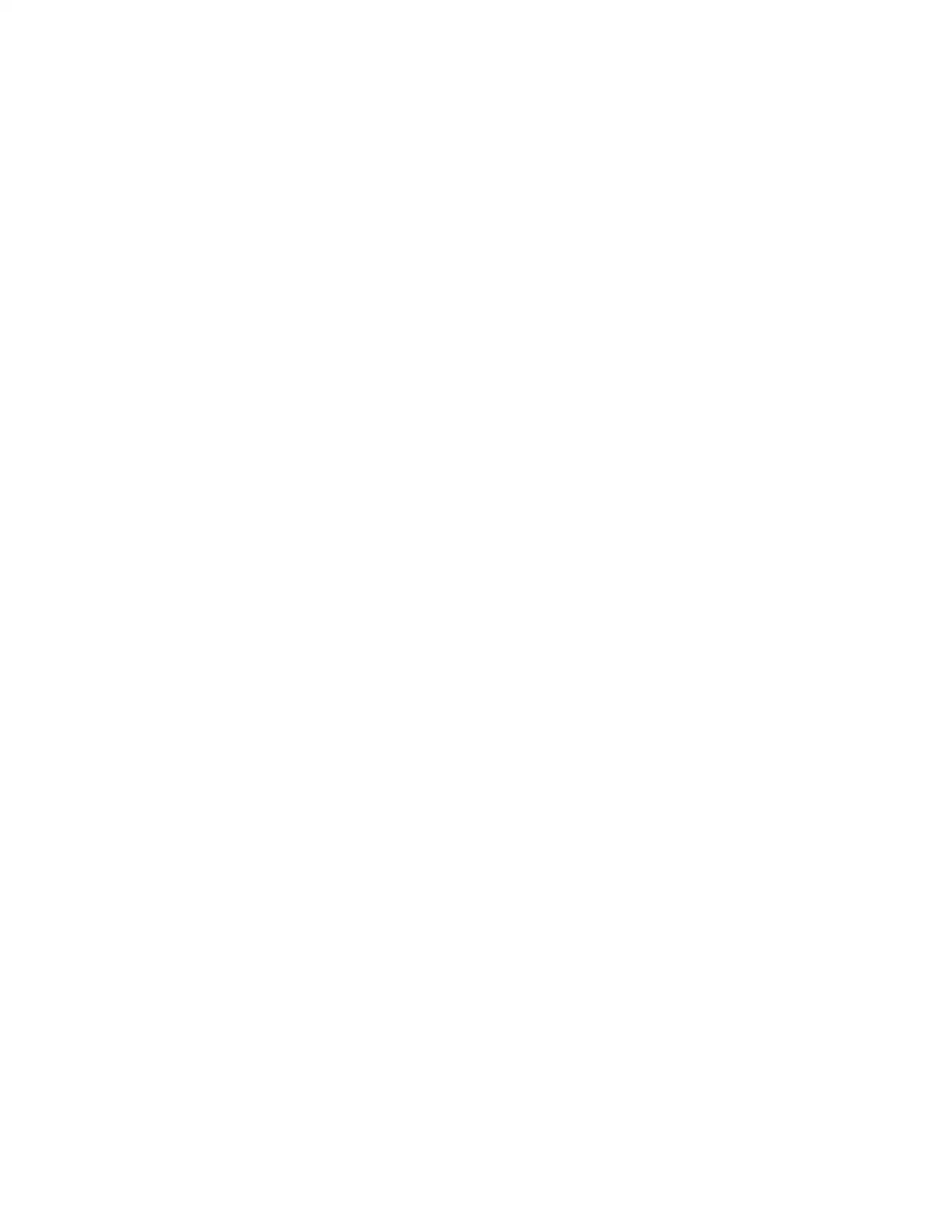
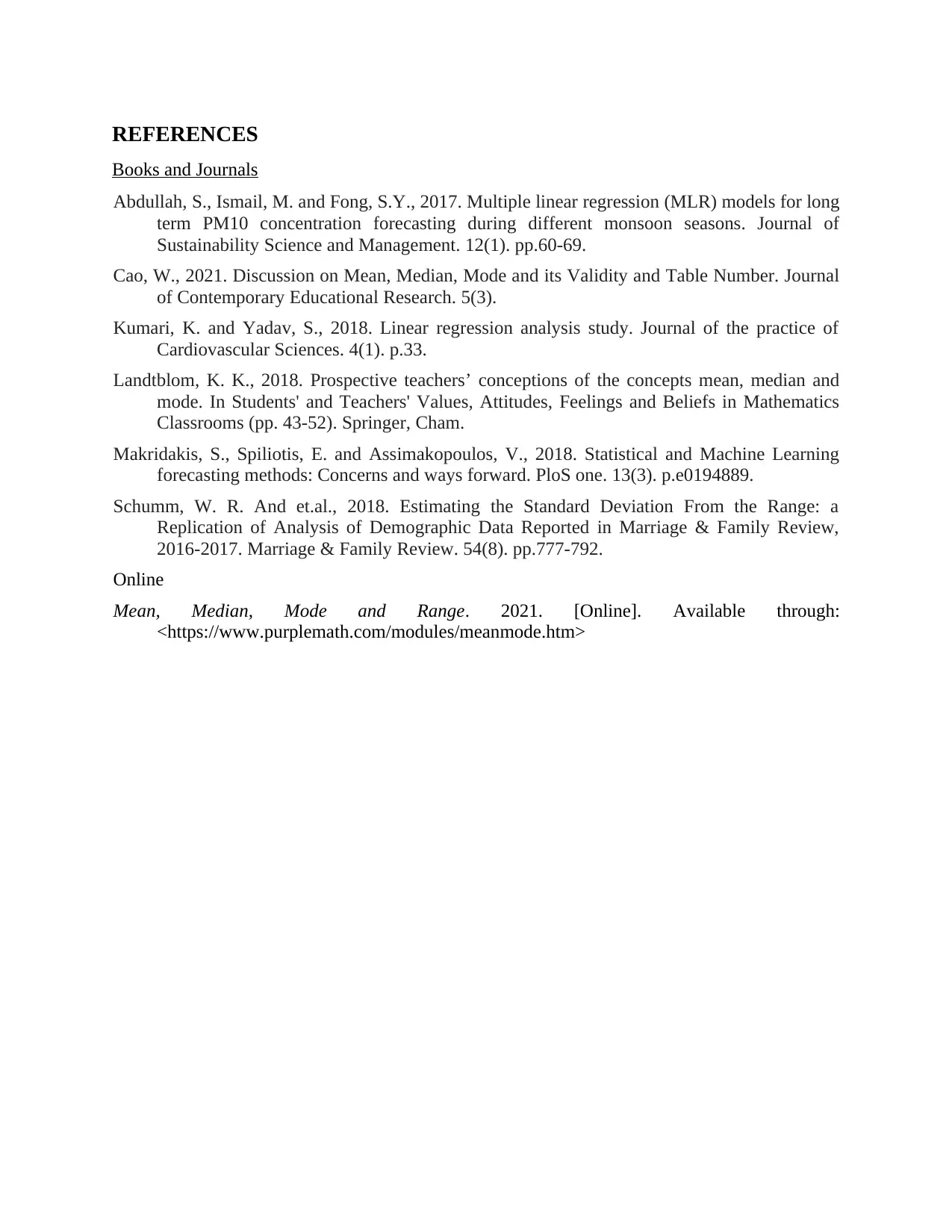
REFERENCES
Books and Journals
Abdullah, S., Ismail, M. and Fong, S.Y., 2017. Multiple linear regression (MLR) models for long
term PM10 concentration forecasting during different monsoon seasons. Journal of
Sustainability Science and Management. 12(1). pp.60-69.
Cao, W., 2021. Discussion on Mean, Median, Mode and its Validity and Table Number. Journal
of Contemporary Educational Research. 5(3).
Kumari, K. and Yadav, S., 2018. Linear regression analysis study. Journal of the practice of
Cardiovascular Sciences. 4(1). p.33.
Landtblom, K. K., 2018. Prospective teachers’ conceptions of the concepts mean, median and
mode. In Students' and Teachers' Values, Attitudes, Feelings and Beliefs in Mathematics
Classrooms (pp. 43-52). Springer, Cham.
Makridakis, S., Spiliotis, E. and Assimakopoulos, V., 2018. Statistical and Machine Learning
forecasting methods: Concerns and ways forward. PloS one. 13(3). p.e0194889.
Schumm, W. R. And et.al., 2018. Estimating the Standard Deviation From the Range: a
Replication of Analysis of Demographic Data Reported in Marriage & Family Review,
2016-2017. Marriage & Family Review. 54(8). pp.777-792.
Online
Mean, Median, Mode and Range. 2021. [Online]. Available through:
<https://www.purplemath.com/modules/meanmode.htm>
Books and Journals
Abdullah, S., Ismail, M. and Fong, S.Y., 2017. Multiple linear regression (MLR) models for long
term PM10 concentration forecasting during different monsoon seasons. Journal of
Sustainability Science and Management. 12(1). pp.60-69.
Cao, W., 2021. Discussion on Mean, Median, Mode and its Validity and Table Number. Journal
of Contemporary Educational Research. 5(3).
Kumari, K. and Yadav, S., 2018. Linear regression analysis study. Journal of the practice of
Cardiovascular Sciences. 4(1). p.33.
Landtblom, K. K., 2018. Prospective teachers’ conceptions of the concepts mean, median and
mode. In Students' and Teachers' Values, Attitudes, Feelings and Beliefs in Mathematics
Classrooms (pp. 43-52). Springer, Cham.
Makridakis, S., Spiliotis, E. and Assimakopoulos, V., 2018. Statistical and Machine Learning
forecasting methods: Concerns and ways forward. PloS one. 13(3). p.e0194889.
Schumm, W. R. And et.al., 2018. Estimating the Standard Deviation From the Range: a
Replication of Analysis of Demographic Data Reported in Marriage & Family Review,
2016-2017. Marriage & Family Review. 54(8). pp.777-792.
Online
Mean, Median, Mode and Range. 2021. [Online]. Available through:
<https://www.purplemath.com/modules/meanmode.htm>
⊘ This is a preview!⊘
Do you want full access?
Subscribe today to unlock all pages.

Trusted by 1+ million students worldwide
1 out of 12
Related Documents

Your All-in-One AI-Powered Toolkit for Academic Success.
+13062052269
info@desklib.com
Available 24*7 on WhatsApp / Email
Unlock your academic potential
© 2024 | Zucol Services PVT LTD | All rights reserved.