Pearson Correlation in Quantitative Reasoning
VerifiedAdded on 2023/06/04
|8
|1598
|497
AI Summary
This article explains Pearson Correlation in Quantitative Reasoning. It defines Pearson's correlation coefficient and its possible values. It also discusses the meaning of output items like Statistic, t, Degree of freedom, p-value, Alternative hypothesis, and 95% confidence interval. The article explains how to perform a hypothesis test and interpret the results. It concludes that the sample data is not sufficient to make significant statistical decisions on the correlation between X and Y.
Contribute Materials
Your contribution can guide someone’s learning journey. Share your
documents today.
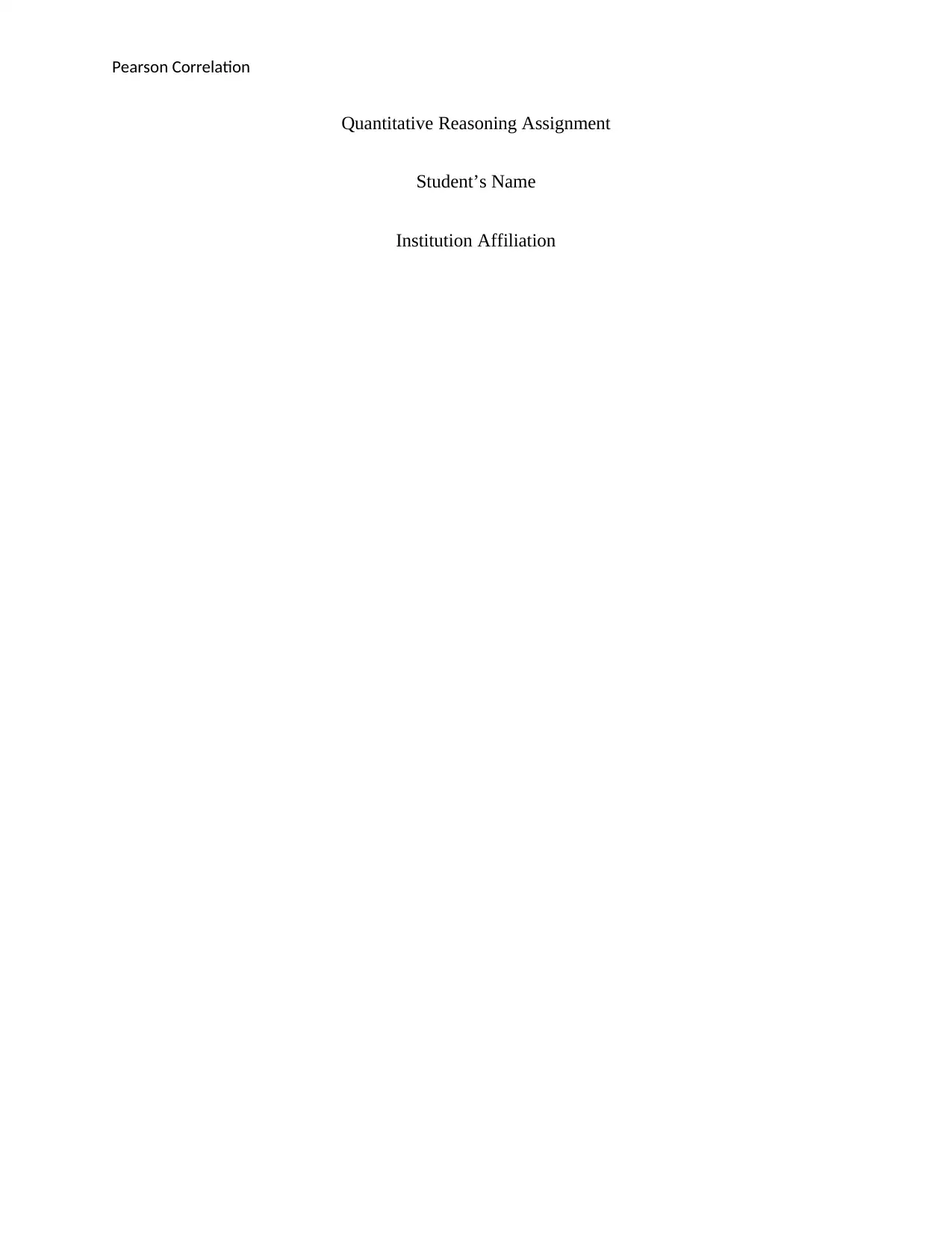
Pearson Correlation
Quantitative Reasoning Assignment
Student’s Name
Institution Affiliation
Quantitative Reasoning Assignment
Student’s Name
Institution Affiliation
Secure Best Marks with AI Grader
Need help grading? Try our AI Grader for instant feedback on your assignments.
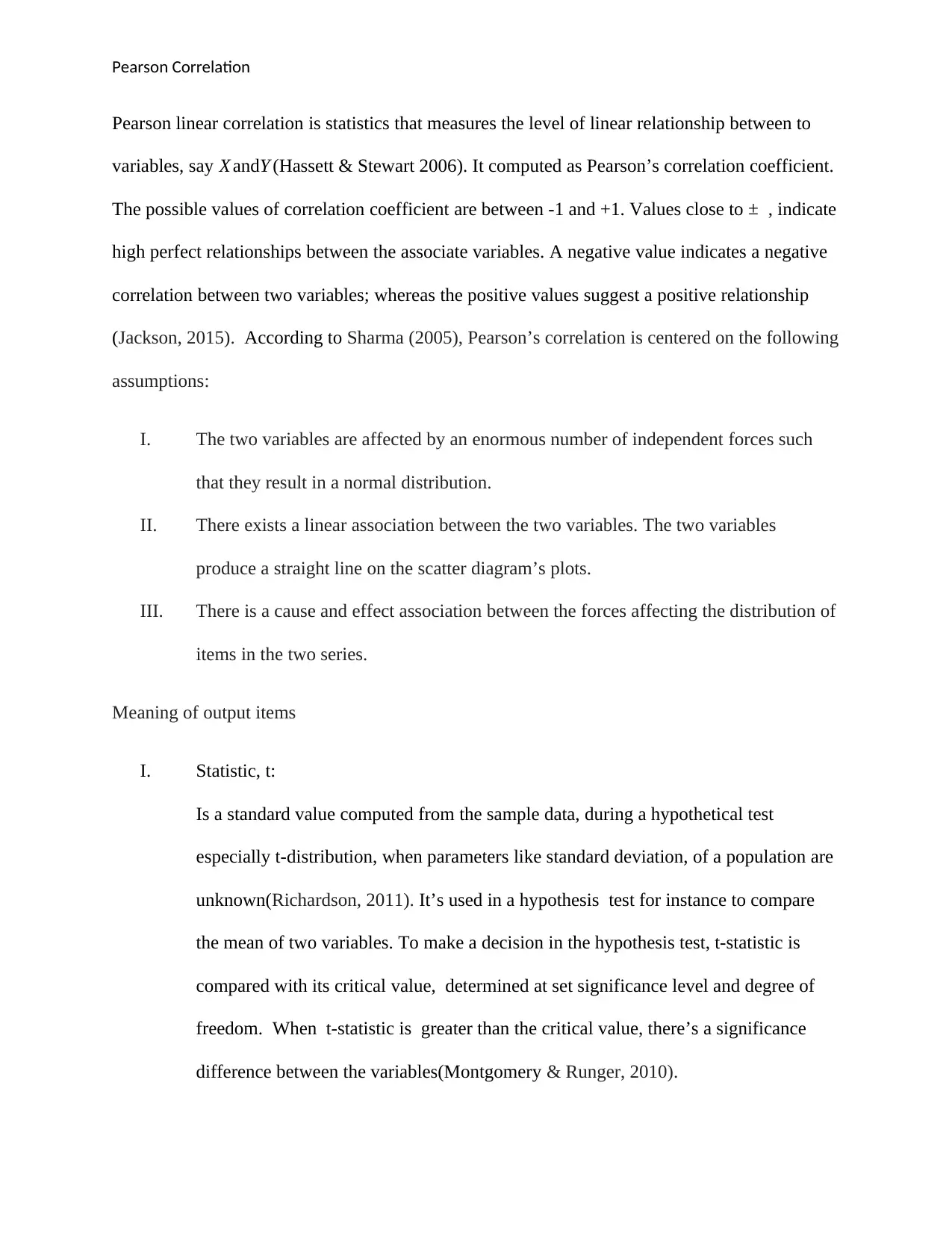
Pearson Correlation
Pearson linear correlation is statistics that measures the level of linear relationship between to
variables, say X andY (Hassett & Stewart 2006). It computed as Pearson’s correlation coefficient.
The possible values of correlation coefficient are between -1 and +1. Values close to ± , indicate
high perfect relationships between the associate variables. A negative value indicates a negative
correlation between two variables; whereas the positive values suggest a positive relationship
(Jackson, 2015). According to Sharma (2005), Pearson’s correlation is centered on the following
assumptions:
I. The two variables are affected by an enormous number of independent forces such
that they result in a normal distribution.
II. There exists a linear association between the two variables. The two variables
produce a straight line on the scatter diagram’s plots.
III. There is a cause and effect association between the forces affecting the distribution of
items in the two series.
Meaning of output items
I. Statistic, t:
Is a standard value computed from the sample data, during a hypothetical test
especially t-distribution, when parameters like standard deviation, of a population are
unknown(Richardson, 2011). It’s used in a hypothesis test for instance to compare
the mean of two variables. To make a decision in the hypothesis test, t-statistic is
compared with its critical value, determined at set significance level and degree of
freedom. When t-statistic is greater than the critical value, there’s a significance
difference between the variables(Montgomery & Runger, 2010).
Pearson linear correlation is statistics that measures the level of linear relationship between to
variables, say X andY (Hassett & Stewart 2006). It computed as Pearson’s correlation coefficient.
The possible values of correlation coefficient are between -1 and +1. Values close to ± , indicate
high perfect relationships between the associate variables. A negative value indicates a negative
correlation between two variables; whereas the positive values suggest a positive relationship
(Jackson, 2015). According to Sharma (2005), Pearson’s correlation is centered on the following
assumptions:
I. The two variables are affected by an enormous number of independent forces such
that they result in a normal distribution.
II. There exists a linear association between the two variables. The two variables
produce a straight line on the scatter diagram’s plots.
III. There is a cause and effect association between the forces affecting the distribution of
items in the two series.
Meaning of output items
I. Statistic, t:
Is a standard value computed from the sample data, during a hypothetical test
especially t-distribution, when parameters like standard deviation, of a population are
unknown(Richardson, 2011). It’s used in a hypothesis test for instance to compare
the mean of two variables. To make a decision in the hypothesis test, t-statistic is
compared with its critical value, determined at set significance level and degree of
freedom. When t-statistic is greater than the critical value, there’s a significance
difference between the variables(Montgomery & Runger, 2010).
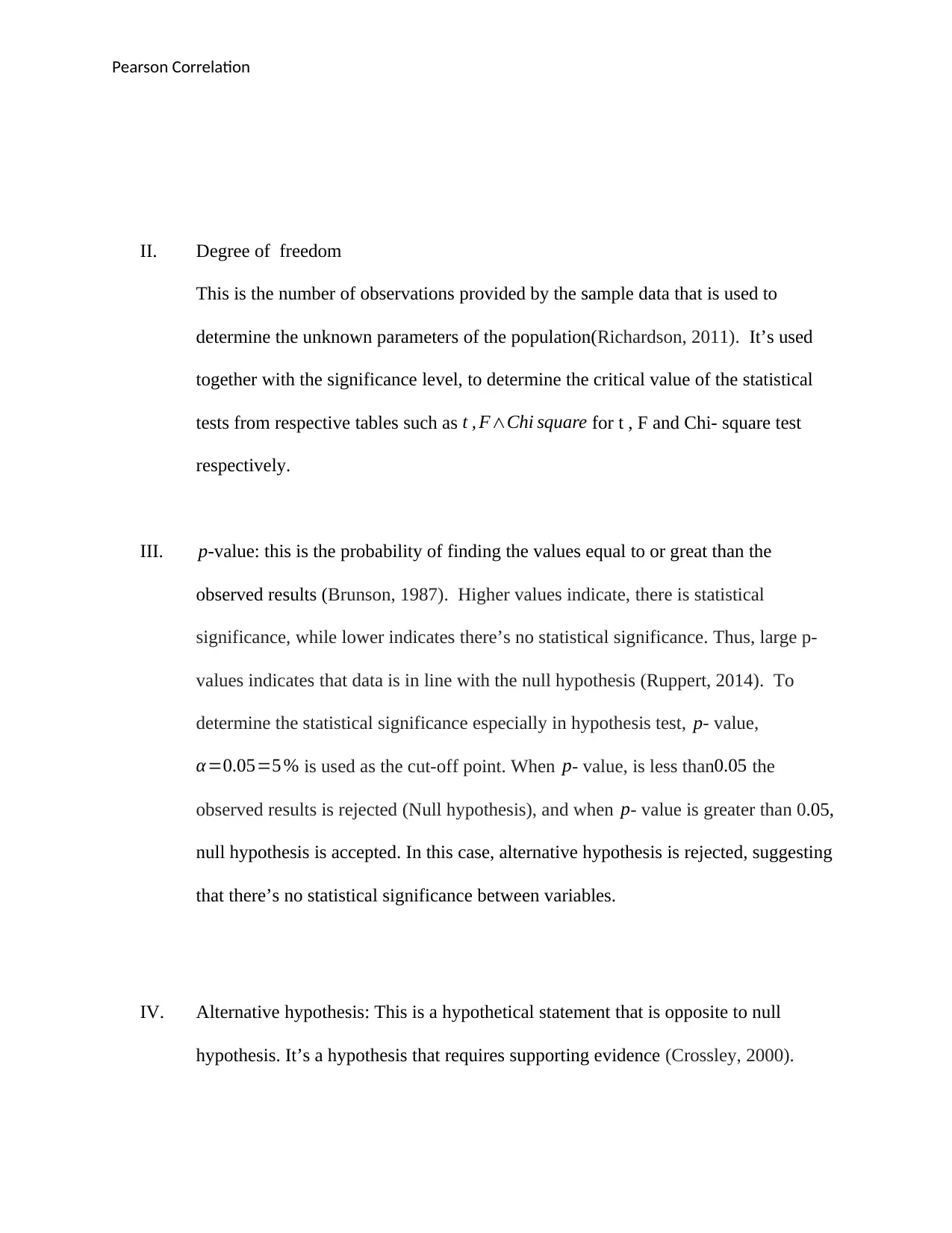
Pearson Correlation
II. Degree of freedom
This is the number of observations provided by the sample data that is used to
determine the unknown parameters of the population(Richardson, 2011). It’s used
together with the significance level, to determine the critical value of the statistical
tests from respective tables such as t , F∧Chi square for t , F and Chi- square test
respectively.
III. p-value: this is the probability of finding the values equal to or great than the
observed results (Brunson, 1987). Higher values indicate, there is statistical
significance, while lower indicates there’s no statistical significance. Thus, large p-
values indicates that data is in line with the null hypothesis (Ruppert, 2014). To
determine the statistical significance especially in hypothesis test, p- value,
α =0.05=5 % is used as the cut-off point. When p- value, is less than0.05 the
observed results is rejected (Null hypothesis), and when p- value is greater than 0.05,
null hypothesis is accepted. In this case, alternative hypothesis is rejected, suggesting
that there’s no statistical significance between variables.
IV. Alternative hypothesis: This is a hypothetical statement that is opposite to null
hypothesis. It’s a hypothesis that requires supporting evidence (Crossley, 2000).
II. Degree of freedom
This is the number of observations provided by the sample data that is used to
determine the unknown parameters of the population(Richardson, 2011). It’s used
together with the significance level, to determine the critical value of the statistical
tests from respective tables such as t , F∧Chi square for t , F and Chi- square test
respectively.
III. p-value: this is the probability of finding the values equal to or great than the
observed results (Brunson, 1987). Higher values indicate, there is statistical
significance, while lower indicates there’s no statistical significance. Thus, large p-
values indicates that data is in line with the null hypothesis (Ruppert, 2014). To
determine the statistical significance especially in hypothesis test, p- value,
α =0.05=5 % is used as the cut-off point. When p- value, is less than0.05 the
observed results is rejected (Null hypothesis), and when p- value is greater than 0.05,
null hypothesis is accepted. In this case, alternative hypothesis is rejected, suggesting
that there’s no statistical significance between variables.
IV. Alternative hypothesis: This is a hypothetical statement that is opposite to null
hypothesis. It’s a hypothesis that requires supporting evidence (Crossley, 2000).
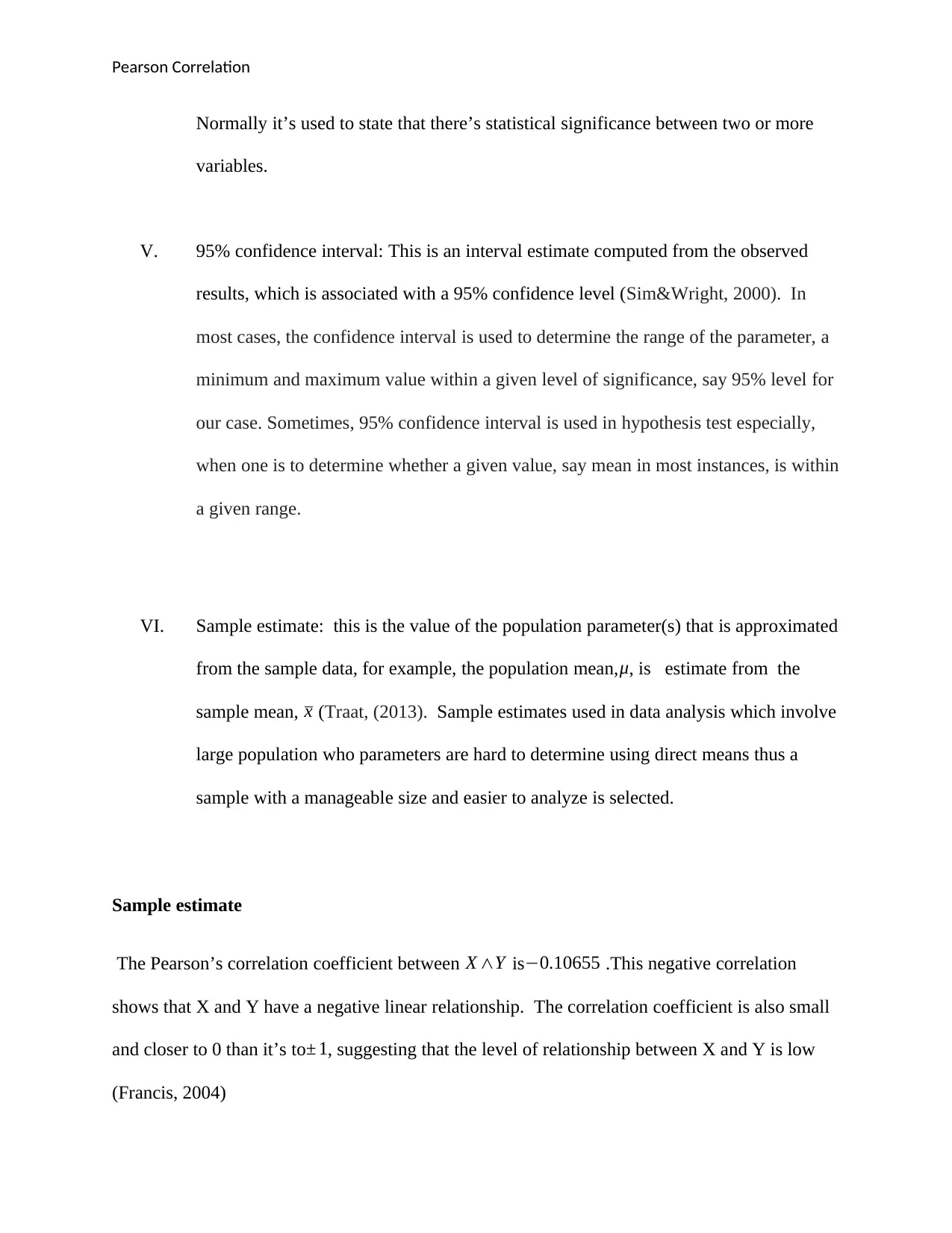
Pearson Correlation
Normally it’s used to state that there’s statistical significance between two or more
variables.
V. 95% confidence interval: This is an interval estimate computed from the observed
results, which is associated with a 95% confidence level (Sim&Wright, 2000). In
most cases, the confidence interval is used to determine the range of the parameter, a
minimum and maximum value within a given level of significance, say 95% level for
our case. Sometimes, 95% confidence interval is used in hypothesis test especially,
when one is to determine whether a given value, say mean in most instances, is within
a given range.
VI. Sample estimate: this is the value of the population parameter(s) that is approximated
from the sample data, for example, the population mean, μ, is estimate from the
sample mean, x (Traat, (2013). Sample estimates used in data analysis which involve
large population who parameters are hard to determine using direct means thus a
sample with a manageable size and easier to analyze is selected.
Sample estimate
The Pearson’s correlation coefficient between X ∧Y is−0.10655 .This negative correlation
shows that X and Y have a negative linear relationship. The correlation coefficient is also small
and closer to 0 than it’s to ± 1, suggesting that the level of relationship between X and Y is low
(Francis, 2004)
Normally it’s used to state that there’s statistical significance between two or more
variables.
V. 95% confidence interval: This is an interval estimate computed from the observed
results, which is associated with a 95% confidence level (Sim&Wright, 2000). In
most cases, the confidence interval is used to determine the range of the parameter, a
minimum and maximum value within a given level of significance, say 95% level for
our case. Sometimes, 95% confidence interval is used in hypothesis test especially,
when one is to determine whether a given value, say mean in most instances, is within
a given range.
VI. Sample estimate: this is the value of the population parameter(s) that is approximated
from the sample data, for example, the population mean, μ, is estimate from the
sample mean, x (Traat, (2013). Sample estimates used in data analysis which involve
large population who parameters are hard to determine using direct means thus a
sample with a manageable size and easier to analyze is selected.
Sample estimate
The Pearson’s correlation coefficient between X ∧Y is−0.10655 .This negative correlation
shows that X and Y have a negative linear relationship. The correlation coefficient is also small
and closer to 0 than it’s to ± 1, suggesting that the level of relationship between X and Y is low
(Francis, 2004)
Secure Best Marks with AI Grader
Need help grading? Try our AI Grader for instant feedback on your assignments.
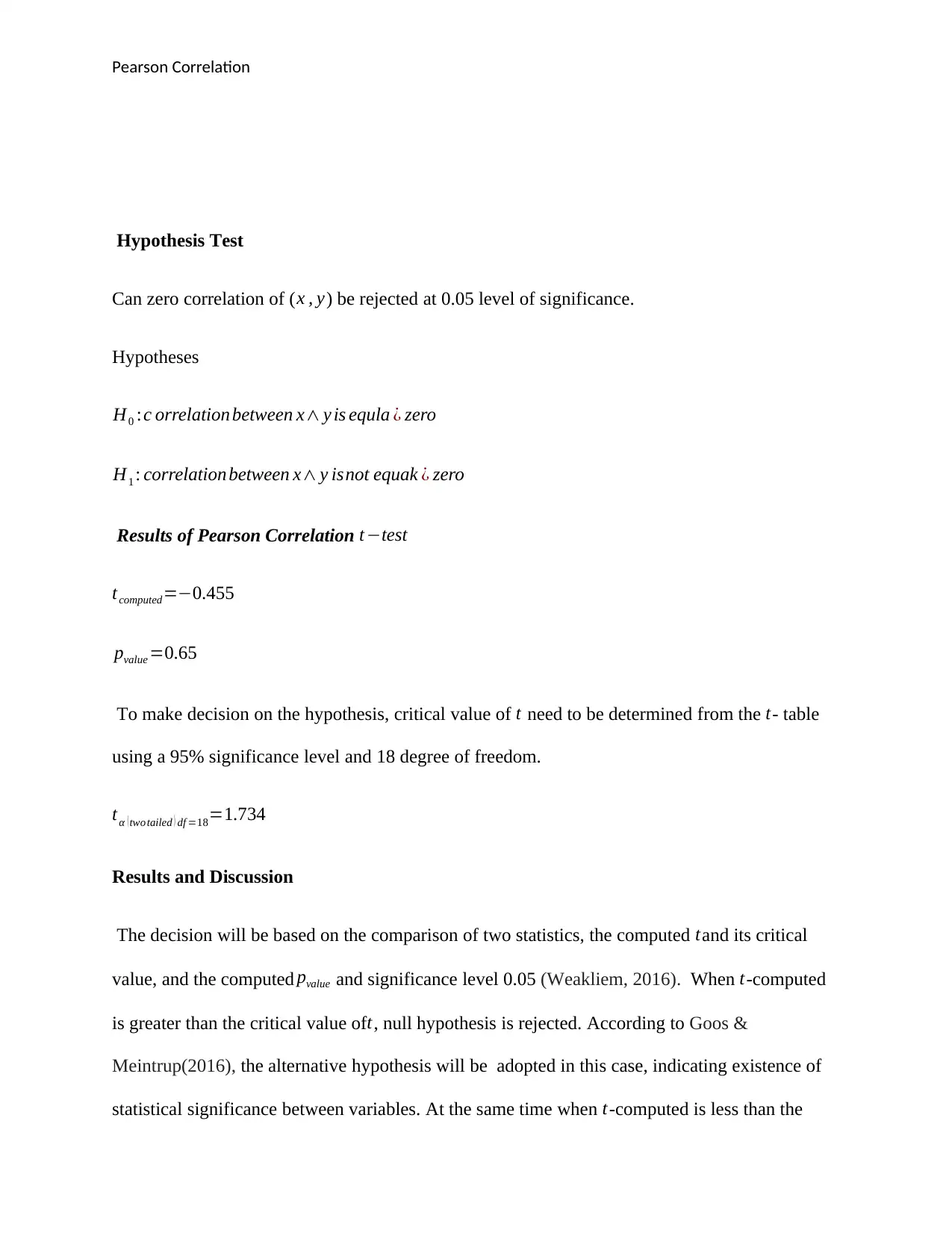
Pearson Correlation
Hypothesis Test
Can zero correlation of (x , y) be rejected at 0.05 level of significance.
Hypotheses
H0 :c orrelation between x∧ y is equla ¿ zero
H1 : correlation between x∧ y isnot equak ¿ zero
Results of Pearson Correlation t−test
tcomputed=−0.455
pvalue =0.65
To make decision on the hypothesis, critical value of t need to be determined from the t- table
using a 95% significance level and 18 degree of freedom.
tα ( two tailed ) df =18=1.734
Results and Discussion
The decision will be based on the comparison of two statistics, the computed tand its critical
value, and the computed pvalue and significance level 0.05 (Weakliem, 2016). When t-computed
is greater than the critical value oft, null hypothesis is rejected. According to Goos &
Meintrup(2016), the alternative hypothesis will be adopted in this case, indicating existence of
statistical significance between variables. At the same time when t-computed is less than the
Hypothesis Test
Can zero correlation of (x , y) be rejected at 0.05 level of significance.
Hypotheses
H0 :c orrelation between x∧ y is equla ¿ zero
H1 : correlation between x∧ y isnot equak ¿ zero
Results of Pearson Correlation t−test
tcomputed=−0.455
pvalue =0.65
To make decision on the hypothesis, critical value of t need to be determined from the t- table
using a 95% significance level and 18 degree of freedom.
tα ( two tailed ) df =18=1.734
Results and Discussion
The decision will be based on the comparison of two statistics, the computed tand its critical
value, and the computed pvalue and significance level 0.05 (Weakliem, 2016). When t-computed
is greater than the critical value oft, null hypothesis is rejected. According to Goos &
Meintrup(2016), the alternative hypothesis will be adopted in this case, indicating existence of
statistical significance between variables. At the same time when t-computed is less than the
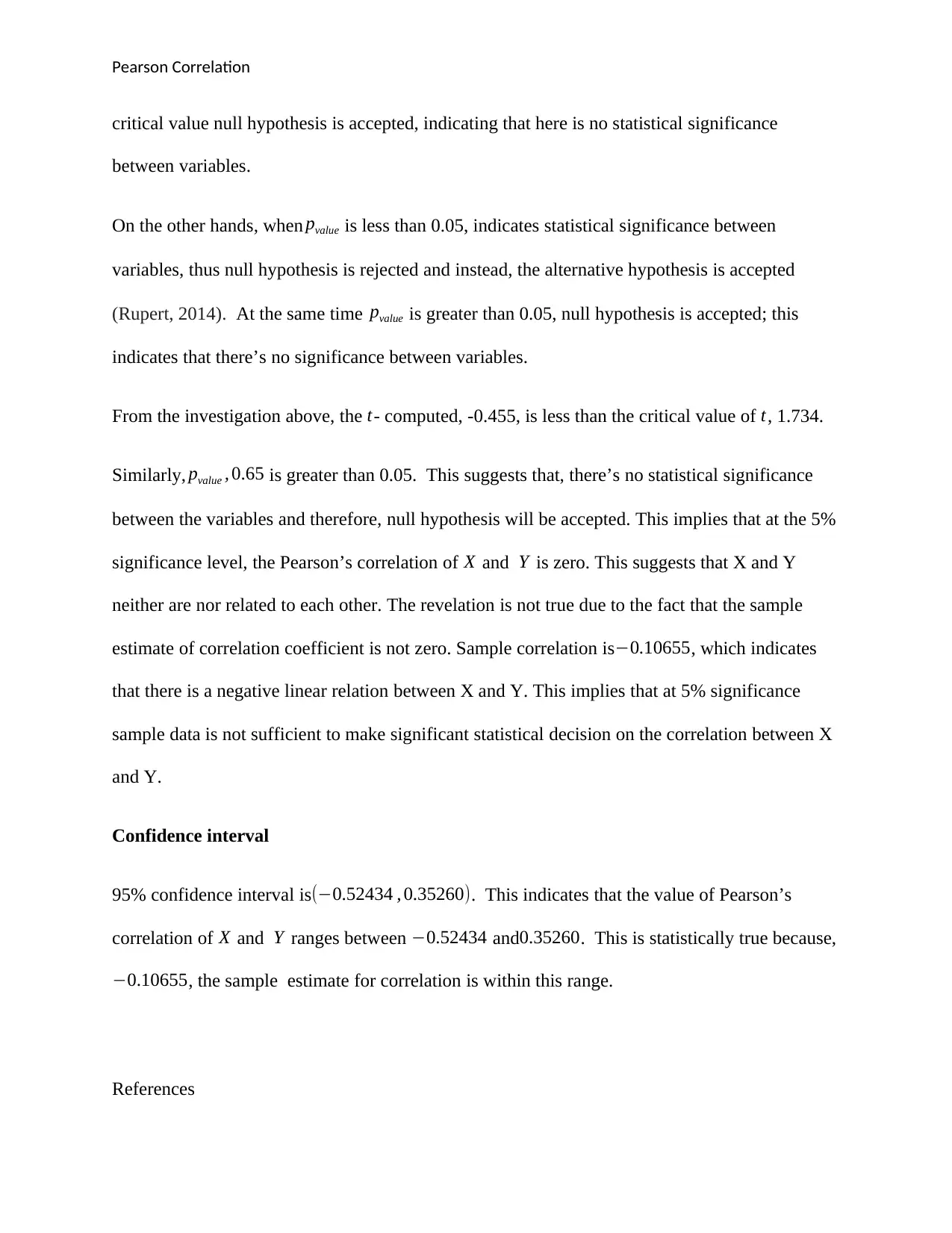
Pearson Correlation
critical value null hypothesis is accepted, indicating that here is no statistical significance
between variables.
On the other hands, when pvalue is less than 0.05, indicates statistical significance between
variables, thus null hypothesis is rejected and instead, the alternative hypothesis is accepted
(Rupert, 2014). At the same time pvalue is greater than 0.05, null hypothesis is accepted; this
indicates that there’s no significance between variables.
From the investigation above, the t- computed, -0.455, is less than the critical value of t, 1.734.
Similarly, pvalue , 0.65 is greater than 0.05. This suggests that, there’s no statistical significance
between the variables and therefore, null hypothesis will be accepted. This implies that at the 5%
significance level, the Pearson’s correlation of X and Y is zero. This suggests that X and Y
neither are nor related to each other. The revelation is not true due to the fact that the sample
estimate of correlation coefficient is not zero. Sample correlation is −0.10655, which indicates
that there is a negative linear relation between X and Y. This implies that at 5% significance
sample data is not sufficient to make significant statistical decision on the correlation between X
and Y.
Confidence interval
95% confidence interval is(−0.52434 , 0.35260). This indicates that the value of Pearson’s
correlation of X and Y ranges between −0.52434 and0.35260. This is statistically true because,
−0.10655, the sample estimate for correlation is within this range.
References
critical value null hypothesis is accepted, indicating that here is no statistical significance
between variables.
On the other hands, when pvalue is less than 0.05, indicates statistical significance between
variables, thus null hypothesis is rejected and instead, the alternative hypothesis is accepted
(Rupert, 2014). At the same time pvalue is greater than 0.05, null hypothesis is accepted; this
indicates that there’s no significance between variables.
From the investigation above, the t- computed, -0.455, is less than the critical value of t, 1.734.
Similarly, pvalue , 0.65 is greater than 0.05. This suggests that, there’s no statistical significance
between the variables and therefore, null hypothesis will be accepted. This implies that at the 5%
significance level, the Pearson’s correlation of X and Y is zero. This suggests that X and Y
neither are nor related to each other. The revelation is not true due to the fact that the sample
estimate of correlation coefficient is not zero. Sample correlation is −0.10655, which indicates
that there is a negative linear relation between X and Y. This implies that at 5% significance
sample data is not sufficient to make significant statistical decision on the correlation between X
and Y.
Confidence interval
95% confidence interval is(−0.52434 , 0.35260). This indicates that the value of Pearson’s
correlation of X and Y ranges between −0.52434 and0.35260. This is statistically true because,
−0.10655, the sample estimate for correlation is within this range.
References
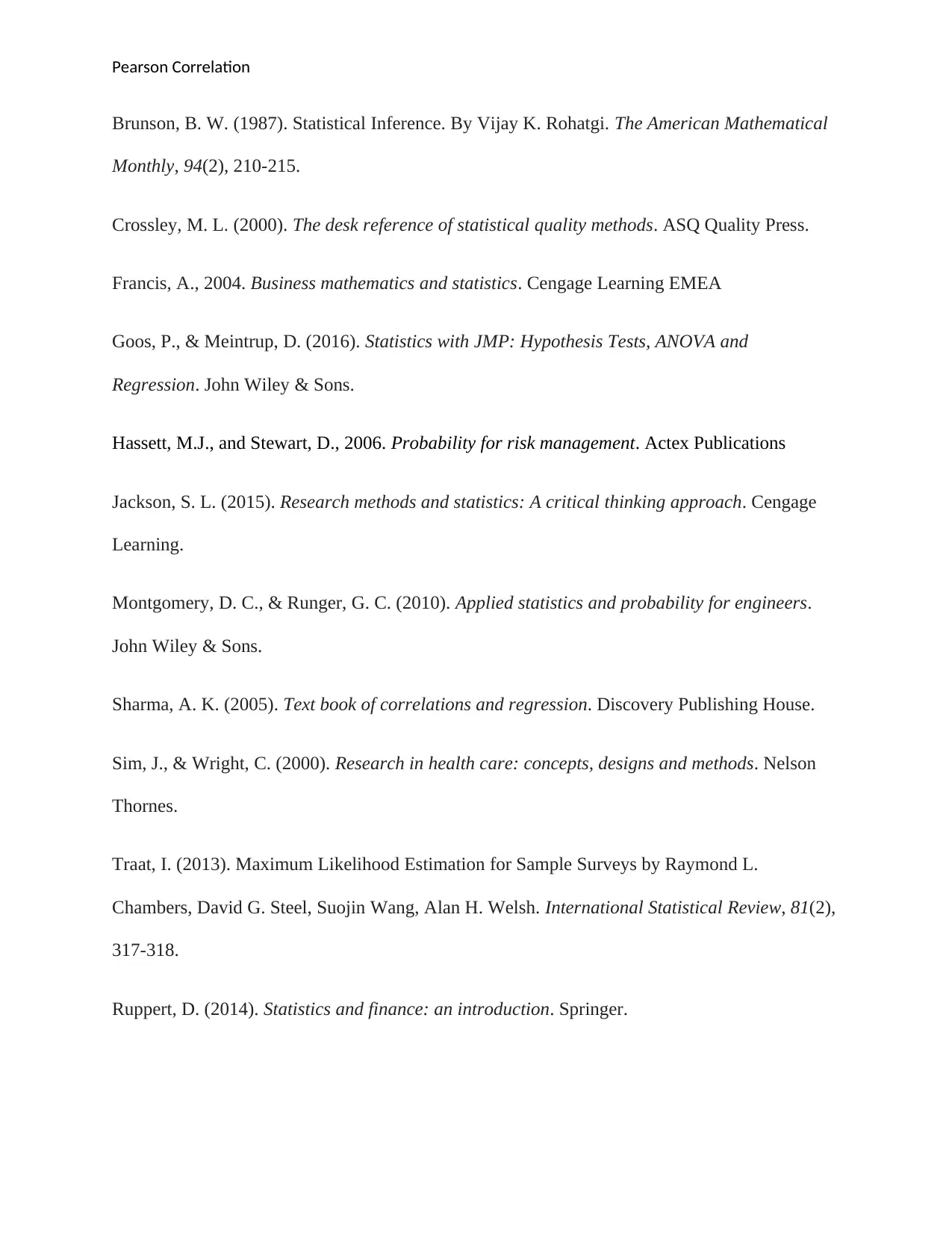
Pearson Correlation
Brunson, B. W. (1987). Statistical Inference. By Vijay K. Rohatgi. The American Mathematical
Monthly, 94(2), 210-215.
Crossley, M. L. (2000). The desk reference of statistical quality methods. ASQ Quality Press.
Francis, A., 2004. Business mathematics and statistics. Cengage Learning EMEA
Goos, P., & Meintrup, D. (2016). Statistics with JMP: Hypothesis Tests, ANOVA and
Regression. John Wiley & Sons.
Hassett, M.J., and Stewart, D., 2006. Probability for risk management. Actex Publications
Jackson, S. L. (2015). Research methods and statistics: A critical thinking approach. Cengage
Learning.
Montgomery, D. C., & Runger, G. C. (2010). Applied statistics and probability for engineers.
John Wiley & Sons.
Sharma, A. K. (2005). Text book of correlations and regression. Discovery Publishing House.
Sim, J., & Wright, C. (2000). Research in health care: concepts, designs and methods. Nelson
Thornes.
Traat, I. (2013). Maximum Likelihood Estimation for Sample Surveys by Raymond L.
Chambers, David G. Steel, Suojin Wang, Alan H. Welsh. International Statistical Review, 81(2),
317-318.
Ruppert, D. (2014). Statistics and finance: an introduction. Springer.
Brunson, B. W. (1987). Statistical Inference. By Vijay K. Rohatgi. The American Mathematical
Monthly, 94(2), 210-215.
Crossley, M. L. (2000). The desk reference of statistical quality methods. ASQ Quality Press.
Francis, A., 2004. Business mathematics and statistics. Cengage Learning EMEA
Goos, P., & Meintrup, D. (2016). Statistics with JMP: Hypothesis Tests, ANOVA and
Regression. John Wiley & Sons.
Hassett, M.J., and Stewart, D., 2006. Probability for risk management. Actex Publications
Jackson, S. L. (2015). Research methods and statistics: A critical thinking approach. Cengage
Learning.
Montgomery, D. C., & Runger, G. C. (2010). Applied statistics and probability for engineers.
John Wiley & Sons.
Sharma, A. K. (2005). Text book of correlations and regression. Discovery Publishing House.
Sim, J., & Wright, C. (2000). Research in health care: concepts, designs and methods. Nelson
Thornes.
Traat, I. (2013). Maximum Likelihood Estimation for Sample Surveys by Raymond L.
Chambers, David G. Steel, Suojin Wang, Alan H. Welsh. International Statistical Review, 81(2),
317-318.
Ruppert, D. (2014). Statistics and finance: an introduction. Springer.
Paraphrase This Document
Need a fresh take? Get an instant paraphrase of this document with our AI Paraphraser
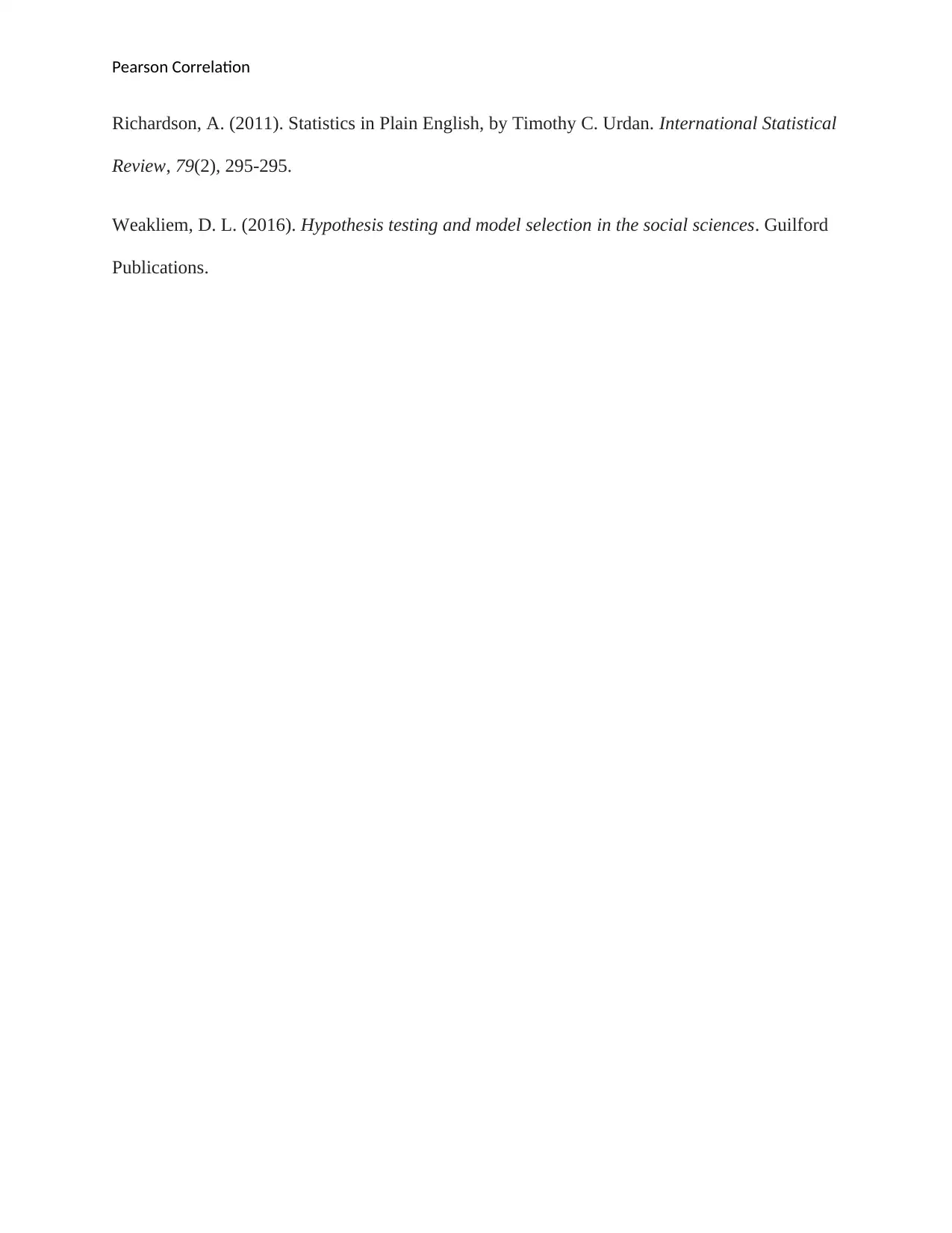
Pearson Correlation
Richardson, A. (2011). Statistics in Plain English, by Timothy C. Urdan. International Statistical
Review, 79(2), 295-295.
Weakliem, D. L. (2016). Hypothesis testing and model selection in the social sciences. Guilford
Publications.
Richardson, A. (2011). Statistics in Plain English, by Timothy C. Urdan. International Statistical
Review, 79(2), 295-295.
Weakliem, D. L. (2016). Hypothesis testing and model selection in the social sciences. Guilford
Publications.
1 out of 8
Related Documents
![[object Object]](/_next/image/?url=%2F_next%2Fstatic%2Fmedia%2Flogo.6d15ce61.png&w=640&q=75)
Your All-in-One AI-Powered Toolkit for Academic Success.
+13062052269
info@desklib.com
Available 24*7 on WhatsApp / Email
Unlock your academic potential
© 2024 | Zucol Services PVT LTD | All rights reserved.