PID Controller Design and Tuning Methods for Industrial Automation
VerifiedAdded on 2023/06/03
|28
|5000
|119
AI Summary
This guide provides an overview of industrial automation and the importance of PID controllers. It discusses different tuning methods, including manual tuning and the Ziegler-Nichols method. The guide also includes a detailed design methodology and simulation using MATLAB software. Access solved assignments, essays, and dissertations on Desklib.
Contribute Materials
Your contribution can guide someone’s learning journey. Share your
documents today.
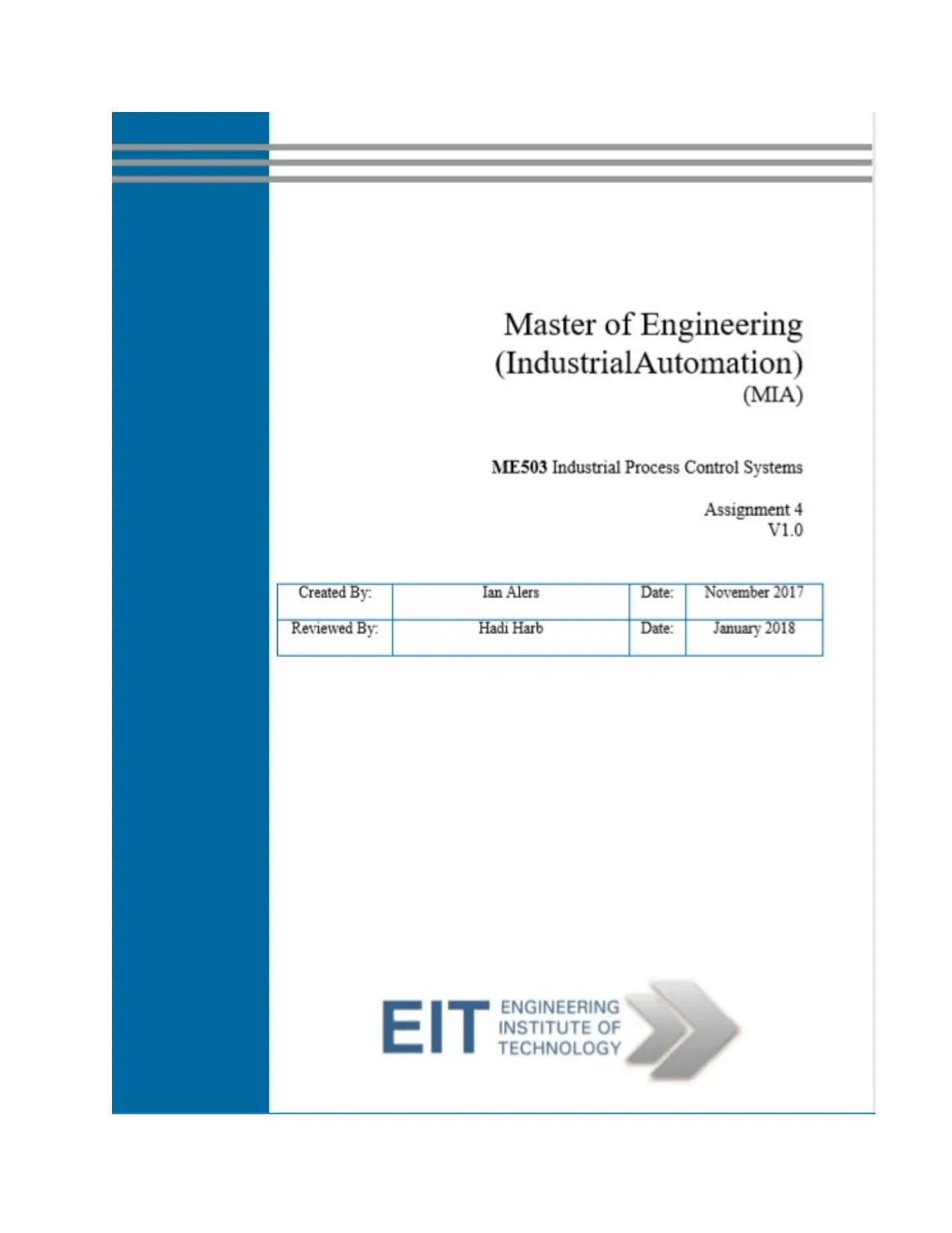
Secure Best Marks with AI Grader
Need help grading? Try our AI Grader for instant feedback on your assignments.
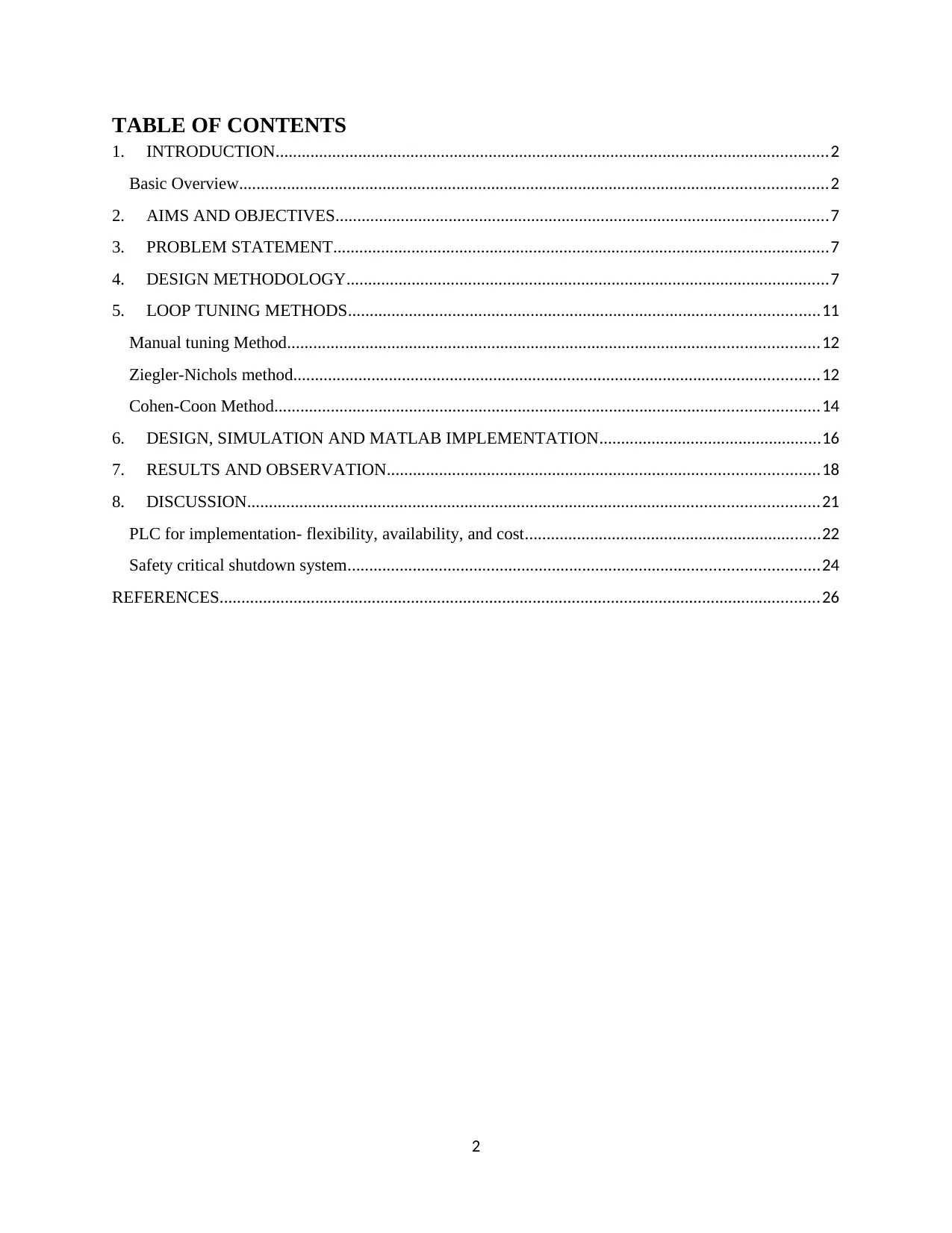
TABLE OF CONTENTS
1. INTRODUCTION...............................................................................................................................2
Basic Overview.......................................................................................................................................2
2. AIMS AND OBJECTIVES.................................................................................................................7
3. PROBLEM STATEMENT..................................................................................................................7
4. DESIGN METHODOLOGY...............................................................................................................7
5. LOOP TUNING METHODS............................................................................................................11
Manual tuning Method..........................................................................................................................12
Ziegler-Nichols method.........................................................................................................................12
Cohen-Coon Method.............................................................................................................................14
6. DESIGN, SIMULATION AND MATLAB IMPLEMENTATION...................................................16
7. RESULTS AND OBSERVATION...................................................................................................18
8. DISCUSSION...................................................................................................................................21
PLC for implementation- flexibility, availability, and cost....................................................................22
Safety critical shutdown system............................................................................................................24
REFERENCES..........................................................................................................................................26
2
1. INTRODUCTION...............................................................................................................................2
Basic Overview.......................................................................................................................................2
2. AIMS AND OBJECTIVES.................................................................................................................7
3. PROBLEM STATEMENT..................................................................................................................7
4. DESIGN METHODOLOGY...............................................................................................................7
5. LOOP TUNING METHODS............................................................................................................11
Manual tuning Method..........................................................................................................................12
Ziegler-Nichols method.........................................................................................................................12
Cohen-Coon Method.............................................................................................................................14
6. DESIGN, SIMULATION AND MATLAB IMPLEMENTATION...................................................16
7. RESULTS AND OBSERVATION...................................................................................................18
8. DISCUSSION...................................................................................................................................21
PLC for implementation- flexibility, availability, and cost....................................................................22
Safety critical shutdown system............................................................................................................24
REFERENCES..........................................................................................................................................26
2
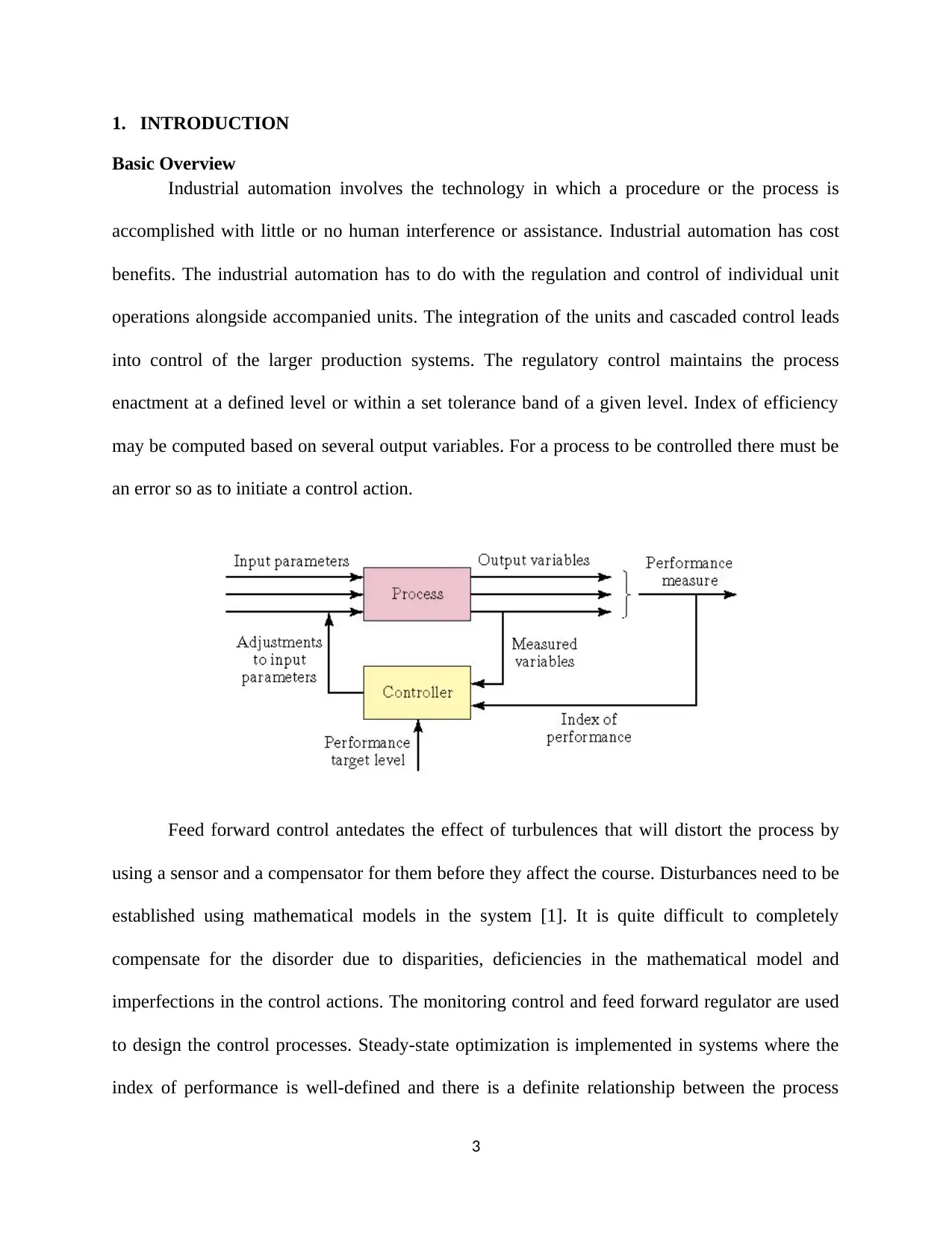
1. INTRODUCTION
Basic Overview
Industrial automation involves the technology in which a procedure or the process is
accomplished with little or no human interference or assistance. Industrial automation has cost
benefits. The industrial automation has to do with the regulation and control of individual unit
operations alongside accompanied units. The integration of the units and cascaded control leads
into control of the larger production systems. The regulatory control maintains the process
enactment at a defined level or within a set tolerance band of a given level. Index of efficiency
may be computed based on several output variables. For a process to be controlled there must be
an error so as to initiate a control action.
Feed forward control antedates the effect of turbulences that will distort the process by
using a sensor and a compensator for them before they affect the course. Disturbances need to be
established using mathematical models in the system [1]. It is quite difficult to completely
compensate for the disorder due to disparities, deficiencies in the mathematical model and
imperfections in the control actions. The monitoring control and feed forward regulator are used
to design the control processes. Steady-state optimization is implemented in systems where the
index of performance is well-defined and there is a definite relationship between the process
3
Basic Overview
Industrial automation involves the technology in which a procedure or the process is
accomplished with little or no human interference or assistance. Industrial automation has cost
benefits. The industrial automation has to do with the regulation and control of individual unit
operations alongside accompanied units. The integration of the units and cascaded control leads
into control of the larger production systems. The regulatory control maintains the process
enactment at a defined level or within a set tolerance band of a given level. Index of efficiency
may be computed based on several output variables. For a process to be controlled there must be
an error so as to initiate a control action.
Feed forward control antedates the effect of turbulences that will distort the process by
using a sensor and a compensator for them before they affect the course. Disturbances need to be
established using mathematical models in the system [1]. It is quite difficult to completely
compensate for the disorder due to disparities, deficiencies in the mathematical model and
imperfections in the control actions. The monitoring control and feed forward regulator are used
to design the control processes. Steady-state optimization is implemented in systems where the
index of performance is well-defined and there is a definite relationship between the process
3
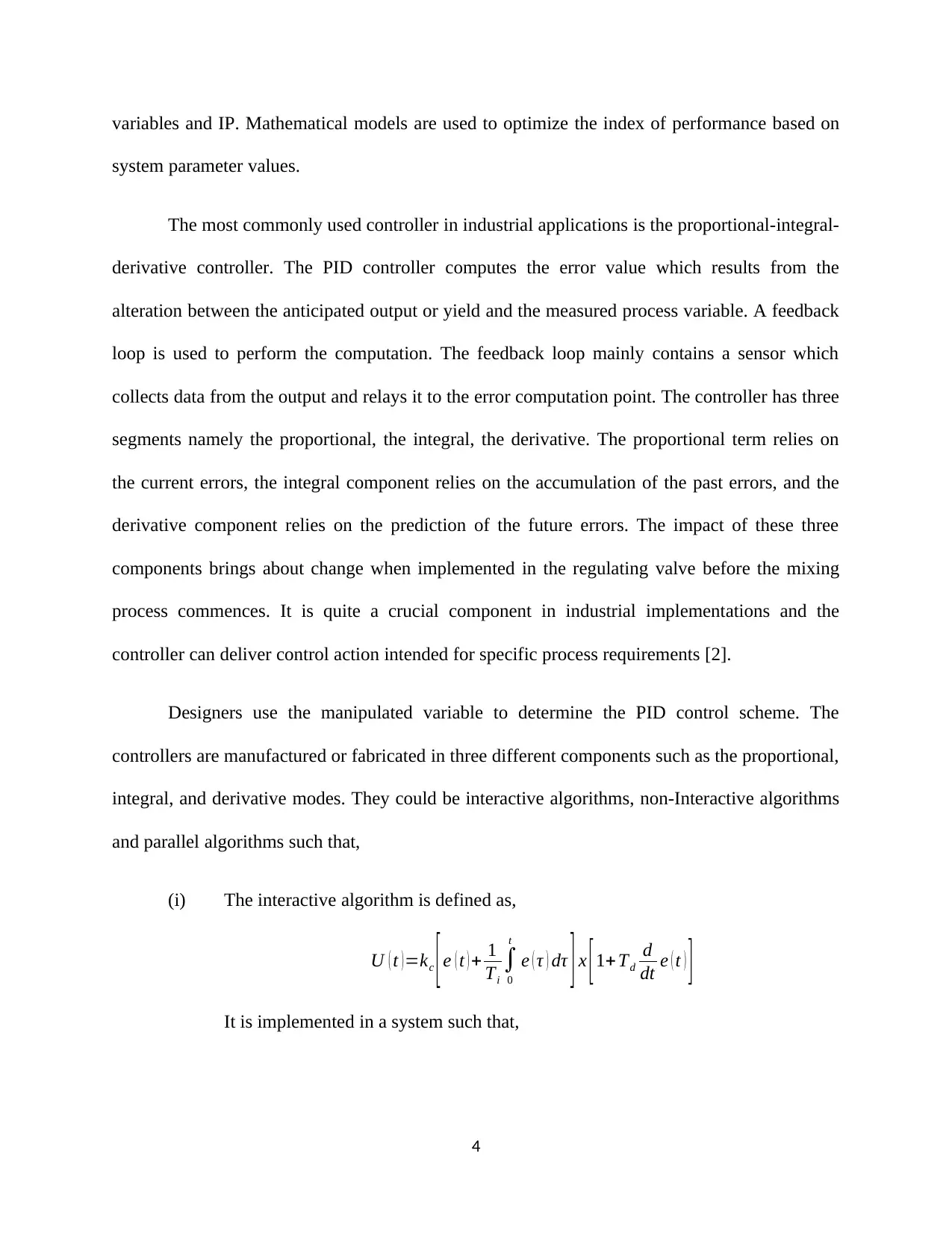
variables and IP. Mathematical models are used to optimize the index of performance based on
system parameter values.
The most commonly used controller in industrial applications is the proportional-integral-
derivative controller. The PID controller computes the error value which results from the
alteration between the anticipated output or yield and the measured process variable. A feedback
loop is used to perform the computation. The feedback loop mainly contains a sensor which
collects data from the output and relays it to the error computation point. The controller has three
segments namely the proportional, the integral, the derivative. The proportional term relies on
the current errors, the integral component relies on the accumulation of the past errors, and the
derivative component relies on the prediction of the future errors. The impact of these three
components brings about change when implemented in the regulating valve before the mixing
process commences. It is quite a crucial component in industrial implementations and the
controller can deliver control action intended for specific process requirements [2].
Designers use the manipulated variable to determine the PID control scheme. The
controllers are manufactured or fabricated in three different components such as the proportional,
integral, and derivative modes. They could be interactive algorithms, non-Interactive algorithms
and parallel algorithms such that,
(i) The interactive algorithm is defined as,
U ( t ) =kc [ e ( t ) + 1
Ti
∫
0
t
e ( τ ) dτ ] x [ 1+ Td
d
dt e ( t ) ]
It is implemented in a system such that,
4
system parameter values.
The most commonly used controller in industrial applications is the proportional-integral-
derivative controller. The PID controller computes the error value which results from the
alteration between the anticipated output or yield and the measured process variable. A feedback
loop is used to perform the computation. The feedback loop mainly contains a sensor which
collects data from the output and relays it to the error computation point. The controller has three
segments namely the proportional, the integral, the derivative. The proportional term relies on
the current errors, the integral component relies on the accumulation of the past errors, and the
derivative component relies on the prediction of the future errors. The impact of these three
components brings about change when implemented in the regulating valve before the mixing
process commences. It is quite a crucial component in industrial implementations and the
controller can deliver control action intended for specific process requirements [2].
Designers use the manipulated variable to determine the PID control scheme. The
controllers are manufactured or fabricated in three different components such as the proportional,
integral, and derivative modes. They could be interactive algorithms, non-Interactive algorithms
and parallel algorithms such that,
(i) The interactive algorithm is defined as,
U ( t ) =kc [ e ( t ) + 1
Ti
∫
0
t
e ( τ ) dτ ] x [ 1+ Td
d
dt e ( t ) ]
It is implemented in a system such that,
4
Paraphrase This Document
Need a fresh take? Get an instant paraphrase of this document with our AI Paraphraser
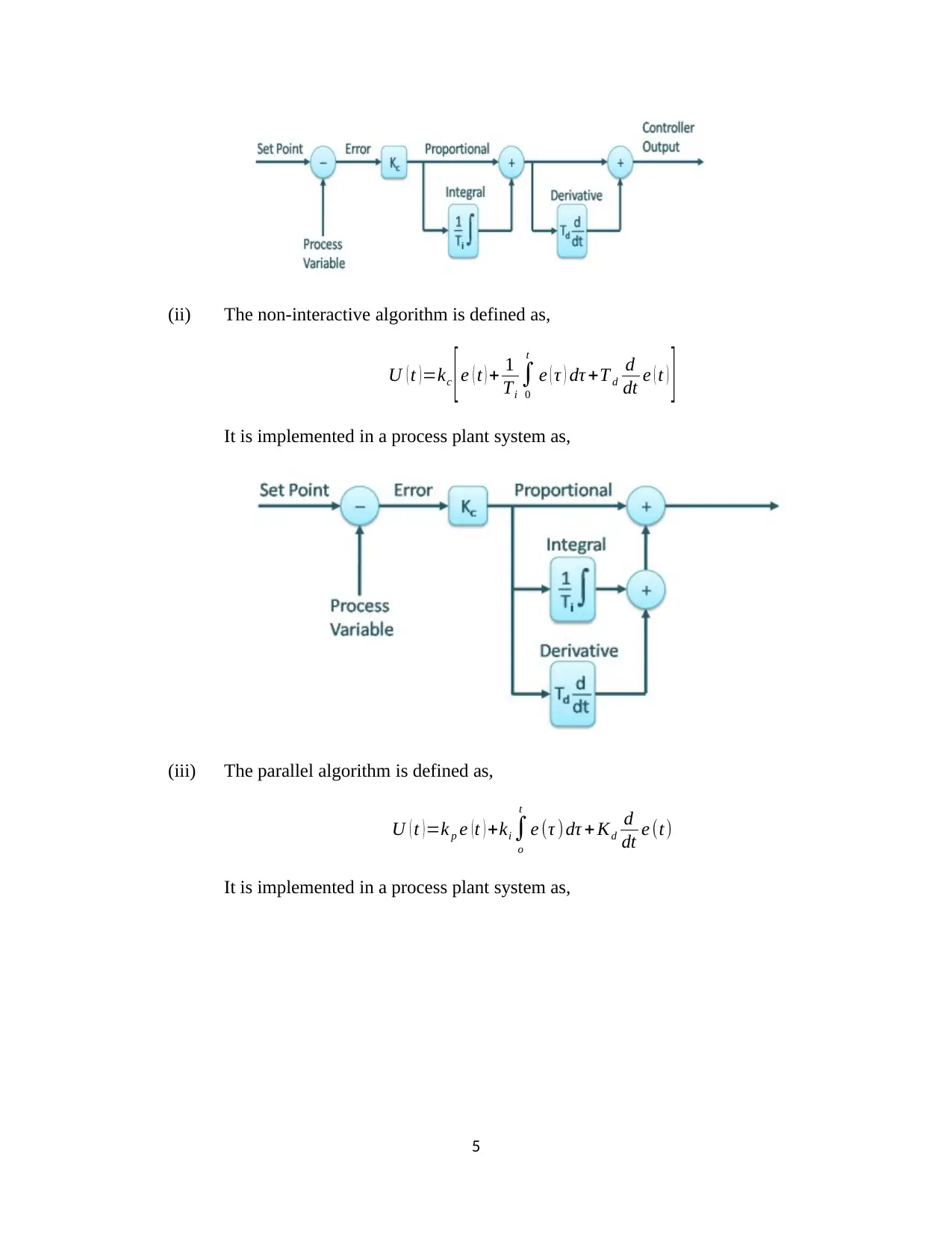
(ii) The non-interactive algorithm is defined as,
U ( t )=kc [e ( t ) + 1
Ti
∫
0
t
e ( τ ) dτ +T d
d
dt e ( t ) ]
It is implemented in a process plant system as,
(iii) The parallel algorithm is defined as,
U ( t )=k p e (t ) +ki ∫
o
t
e (τ )dτ + Kd
d
dt e (t)
It is implemented in a process plant system as,
5
U ( t )=kc [e ( t ) + 1
Ti
∫
0
t
e ( τ ) dτ +T d
d
dt e ( t ) ]
It is implemented in a process plant system as,
(iii) The parallel algorithm is defined as,
U ( t )=k p e (t ) +ki ∫
o
t
e (τ )dτ + Kd
d
dt e (t)
It is implemented in a process plant system as,
5
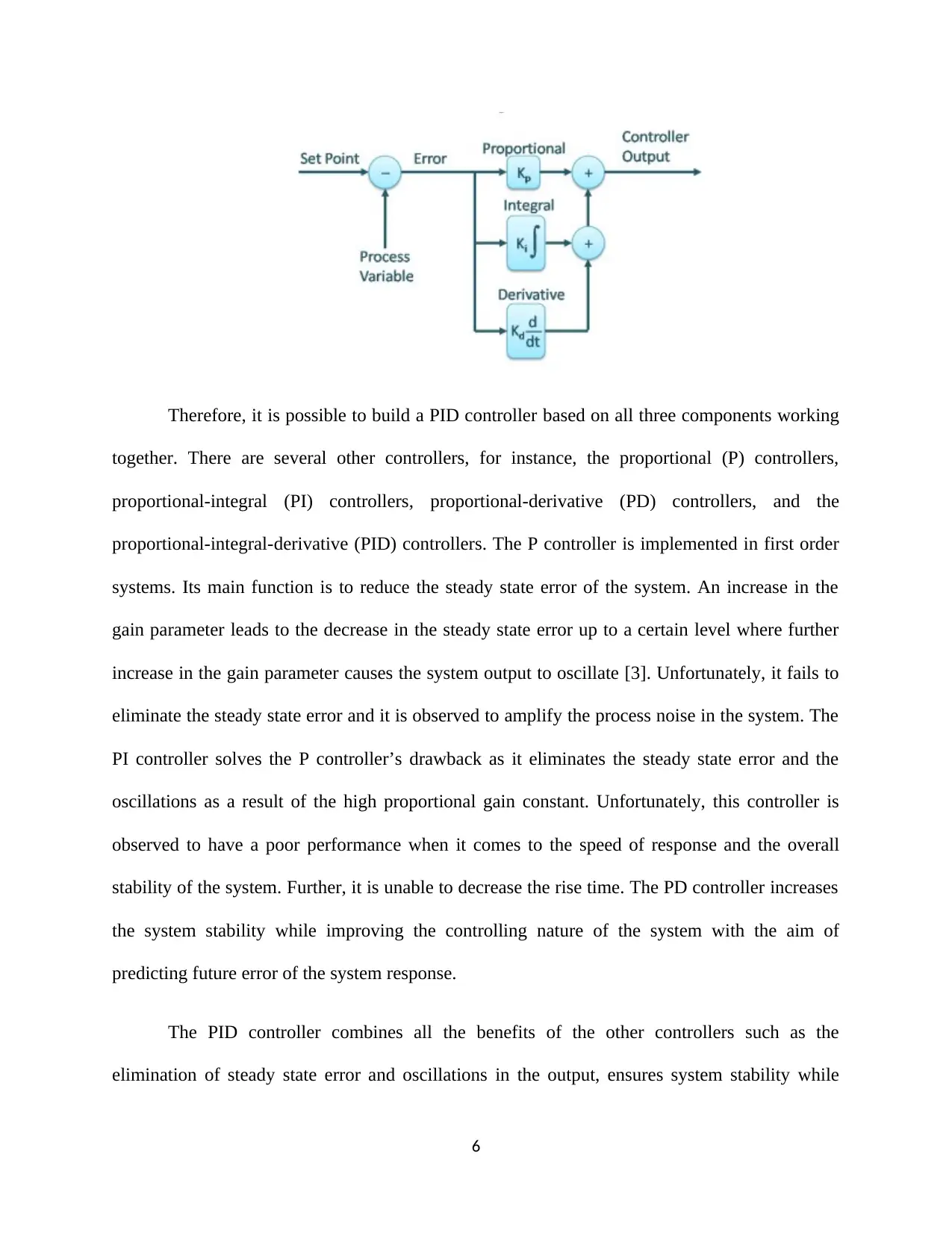
Therefore, it is possible to build a PID controller based on all three components working
together. There are several other controllers, for instance, the proportional (P) controllers,
proportional-integral (PI) controllers, proportional-derivative (PD) controllers, and the
proportional-integral-derivative (PID) controllers. The P controller is implemented in first order
systems. Its main function is to reduce the steady state error of the system. An increase in the
gain parameter leads to the decrease in the steady state error up to a certain level where further
increase in the gain parameter causes the system output to oscillate [3]. Unfortunately, it fails to
eliminate the steady state error and it is observed to amplify the process noise in the system. The
PI controller solves the P controller’s drawback as it eliminates the steady state error and the
oscillations as a result of the high proportional gain constant. Unfortunately, this controller is
observed to have a poor performance when it comes to the speed of response and the overall
stability of the system. Further, it is unable to decrease the rise time. The PD controller increases
the system stability while improving the controlling nature of the system with the aim of
predicting future error of the system response.
The PID controller combines all the benefits of the other controllers such as the
elimination of steady state error and oscillations in the output, ensures system stability while
6
together. There are several other controllers, for instance, the proportional (P) controllers,
proportional-integral (PI) controllers, proportional-derivative (PD) controllers, and the
proportional-integral-derivative (PID) controllers. The P controller is implemented in first order
systems. Its main function is to reduce the steady state error of the system. An increase in the
gain parameter leads to the decrease in the steady state error up to a certain level where further
increase in the gain parameter causes the system output to oscillate [3]. Unfortunately, it fails to
eliminate the steady state error and it is observed to amplify the process noise in the system. The
PI controller solves the P controller’s drawback as it eliminates the steady state error and the
oscillations as a result of the high proportional gain constant. Unfortunately, this controller is
observed to have a poor performance when it comes to the speed of response and the overall
stability of the system. Further, it is unable to decrease the rise time. The PD controller increases
the system stability while improving the controlling nature of the system with the aim of
predicting future error of the system response.
The PID controller combines all the benefits of the other controllers such as the
elimination of steady state error and oscillations in the output, ensures system stability while
6
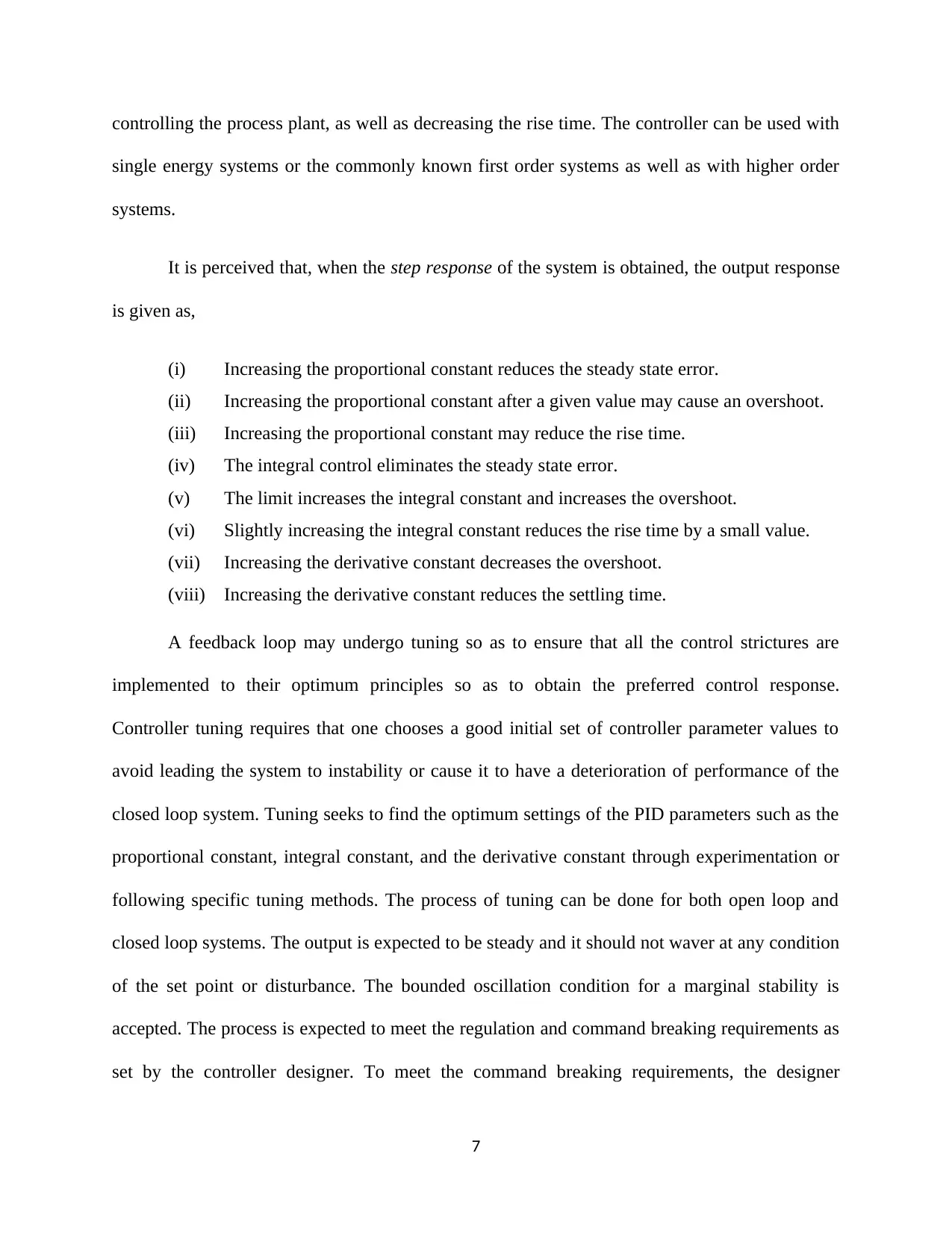
controlling the process plant, as well as decreasing the rise time. The controller can be used with
single energy systems or the commonly known first order systems as well as with higher order
systems.
It is perceived that, when the step response of the system is obtained, the output response
is given as,
(i) Increasing the proportional constant reduces the steady state error.
(ii) Increasing the proportional constant after a given value may cause an overshoot.
(iii) Increasing the proportional constant may reduce the rise time.
(iv) The integral control eliminates the steady state error.
(v) The limit increases the integral constant and increases the overshoot.
(vi) Slightly increasing the integral constant reduces the rise time by a small value.
(vii) Increasing the derivative constant decreases the overshoot.
(viii) Increasing the derivative constant reduces the settling time.
A feedback loop may undergo tuning so as to ensure that all the control strictures are
implemented to their optimum principles so as to obtain the preferred control response.
Controller tuning requires that one chooses a good initial set of controller parameter values to
avoid leading the system to instability or cause it to have a deterioration of performance of the
closed loop system. Tuning seeks to find the optimum settings of the PID parameters such as the
proportional constant, integral constant, and the derivative constant through experimentation or
following specific tuning methods. The process of tuning can be done for both open loop and
closed loop systems. The output is expected to be steady and it should not waver at any condition
of the set point or disturbance. The bounded oscillation condition for a marginal stability is
accepted. The process is expected to meet the regulation and command breaking requirements as
set by the controller designer. To meet the command breaking requirements, the designer
7
single energy systems or the commonly known first order systems as well as with higher order
systems.
It is perceived that, when the step response of the system is obtained, the output response
is given as,
(i) Increasing the proportional constant reduces the steady state error.
(ii) Increasing the proportional constant after a given value may cause an overshoot.
(iii) Increasing the proportional constant may reduce the rise time.
(iv) The integral control eliminates the steady state error.
(v) The limit increases the integral constant and increases the overshoot.
(vi) Slightly increasing the integral constant reduces the rise time by a small value.
(vii) Increasing the derivative constant decreases the overshoot.
(viii) Increasing the derivative constant reduces the settling time.
A feedback loop may undergo tuning so as to ensure that all the control strictures are
implemented to their optimum principles so as to obtain the preferred control response.
Controller tuning requires that one chooses a good initial set of controller parameter values to
avoid leading the system to instability or cause it to have a deterioration of performance of the
closed loop system. Tuning seeks to find the optimum settings of the PID parameters such as the
proportional constant, integral constant, and the derivative constant through experimentation or
following specific tuning methods. The process of tuning can be done for both open loop and
closed loop systems. The output is expected to be steady and it should not waver at any condition
of the set point or disturbance. The bounded oscillation condition for a marginal stability is
accepted. The process is expected to meet the regulation and command breaking requirements as
set by the controller designer. To meet the command breaking requirements, the designer
7
Secure Best Marks with AI Grader
Need help grading? Try our AI Grader for instant feedback on your assignments.
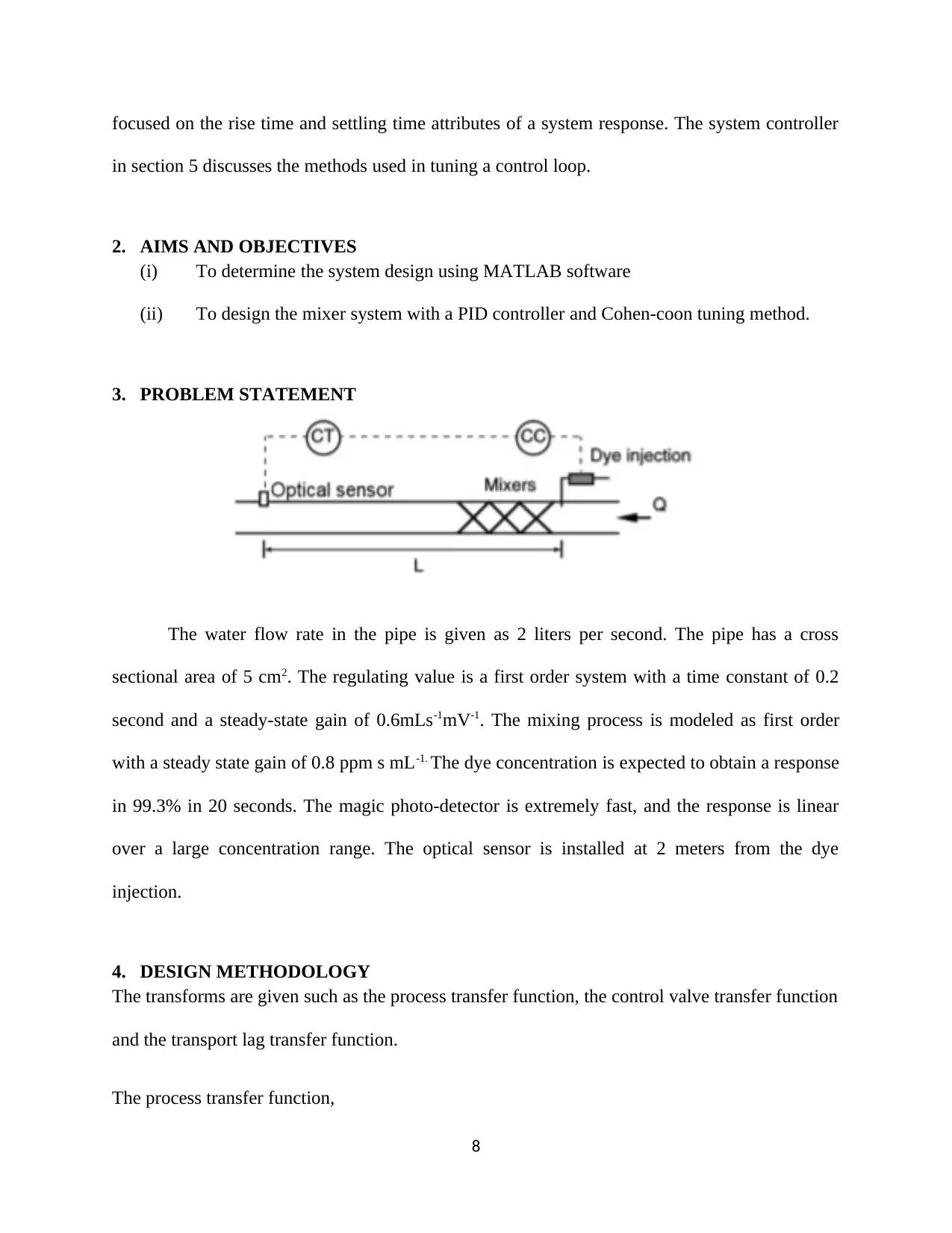
focused on the rise time and settling time attributes of a system response. The system controller
in section 5 discusses the methods used in tuning a control loop.
2. AIMS AND OBJECTIVES
(i) To determine the system design using MATLAB software
(ii) To design the mixer system with a PID controller and Cohen-coon tuning method.
3. PROBLEM STATEMENT
The water flow rate in the pipe is given as 2 liters per second. The pipe has a cross
sectional area of 5 cm2. The regulating value is a first order system with a time constant of 0.2
second and a steady-state gain of 0.6mLs-1mV-1. The mixing process is modeled as first order
with a steady state gain of 0.8 ppm s mL-1. The dye concentration is expected to obtain a response
in 99.3% in 20 seconds. The magic photo-detector is extremely fast, and the response is linear
over a large concentration range. The optical sensor is installed at 2 meters from the dye
injection.
4. DESIGN METHODOLOGY
The transforms are given such as the process transfer function, the control valve transfer function
and the transport lag transfer function.
The process transfer function,
8
in section 5 discusses the methods used in tuning a control loop.
2. AIMS AND OBJECTIVES
(i) To determine the system design using MATLAB software
(ii) To design the mixer system with a PID controller and Cohen-coon tuning method.
3. PROBLEM STATEMENT
The water flow rate in the pipe is given as 2 liters per second. The pipe has a cross
sectional area of 5 cm2. The regulating value is a first order system with a time constant of 0.2
second and a steady-state gain of 0.6mLs-1mV-1. The mixing process is modeled as first order
with a steady state gain of 0.8 ppm s mL-1. The dye concentration is expected to obtain a response
in 99.3% in 20 seconds. The magic photo-detector is extremely fast, and the response is linear
over a large concentration range. The optical sensor is installed at 2 meters from the dye
injection.
4. DESIGN METHODOLOGY
The transforms are given such as the process transfer function, the control valve transfer function
and the transport lag transfer function.
The process transfer function,
8
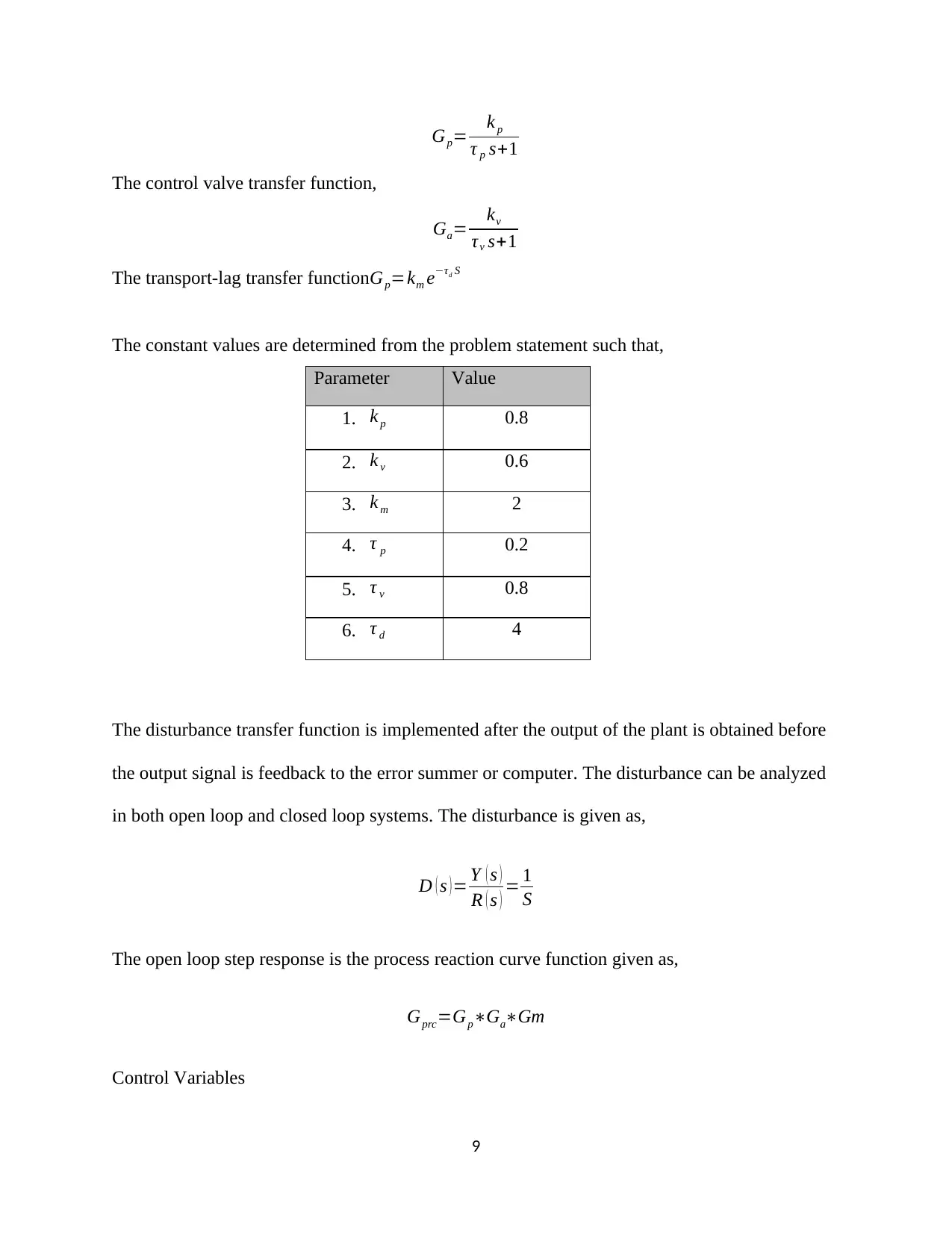
Gp= k p
τ p s+1
The control valve transfer function,
Ga= kv
τv s+1
The transport-lag transfer functionGp=km e−τd S
The constant values are determined from the problem statement such that,
Parameter Value
1. k p 0.8
2. k v 0.6
3. k m 2
4. τ p 0.2
5. τ v 0.8
6. τ d 4
The disturbance transfer function is implemented after the output of the plant is obtained before
the output signal is feedback to the error summer or computer. The disturbance can be analyzed
in both open loop and closed loop systems. The disturbance is given as,
D ( s )= Y ( s )
R ( s ) = 1
S
The open loop step response is the process reaction curve function given as,
Gprc=Gp∗Ga∗Gm
Control Variables
9
τ p s+1
The control valve transfer function,
Ga= kv
τv s+1
The transport-lag transfer functionGp=km e−τd S
The constant values are determined from the problem statement such that,
Parameter Value
1. k p 0.8
2. k v 0.6
3. k m 2
4. τ p 0.2
5. τ v 0.8
6. τ d 4
The disturbance transfer function is implemented after the output of the plant is obtained before
the output signal is feedback to the error summer or computer. The disturbance can be analyzed
in both open loop and closed loop systems. The disturbance is given as,
D ( s )= Y ( s )
R ( s ) = 1
S
The open loop step response is the process reaction curve function given as,
Gprc=Gp∗Ga∗Gm
Control Variables
9
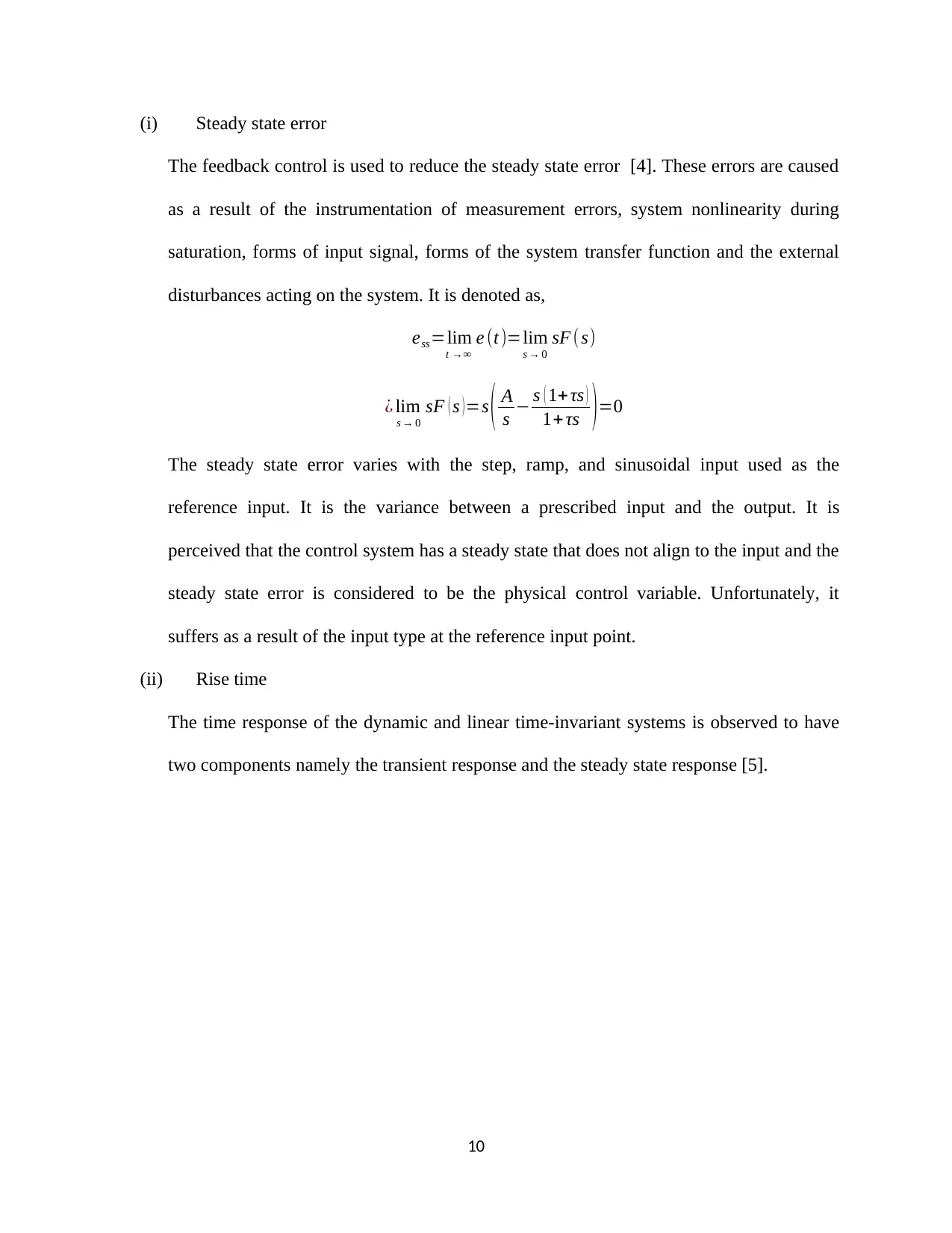
(i) Steady state error
The feedback control is used to reduce the steady state error [4]. These errors are caused
as a result of the instrumentation of measurement errors, system nonlinearity during
saturation, forms of input signal, forms of the system transfer function and the external
disturbances acting on the system. It is denoted as,
ess=lim
t →∞
e (t)=lim
s → 0
sF (s)
¿ lim
s → 0
sF ( s )=s ( A
s − s ( 1+ τs )
1+ τs )=0
The steady state error varies with the step, ramp, and sinusoidal input used as the
reference input. It is the variance between a prescribed input and the output. It is
perceived that the control system has a steady state that does not align to the input and the
steady state error is considered to be the physical control variable. Unfortunately, it
suffers as a result of the input type at the reference input point.
(ii) Rise time
The time response of the dynamic and linear time-invariant systems is observed to have
two components namely the transient response and the steady state response [5].
10
The feedback control is used to reduce the steady state error [4]. These errors are caused
as a result of the instrumentation of measurement errors, system nonlinearity during
saturation, forms of input signal, forms of the system transfer function and the external
disturbances acting on the system. It is denoted as,
ess=lim
t →∞
e (t)=lim
s → 0
sF (s)
¿ lim
s → 0
sF ( s )=s ( A
s − s ( 1+ τs )
1+ τs )=0
The steady state error varies with the step, ramp, and sinusoidal input used as the
reference input. It is the variance between a prescribed input and the output. It is
perceived that the control system has a steady state that does not align to the input and the
steady state error is considered to be the physical control variable. Unfortunately, it
suffers as a result of the input type at the reference input point.
(ii) Rise time
The time response of the dynamic and linear time-invariant systems is observed to have
two components namely the transient response and the steady state response [5].
10
Paraphrase This Document
Need a fresh take? Get an instant paraphrase of this document with our AI Paraphraser
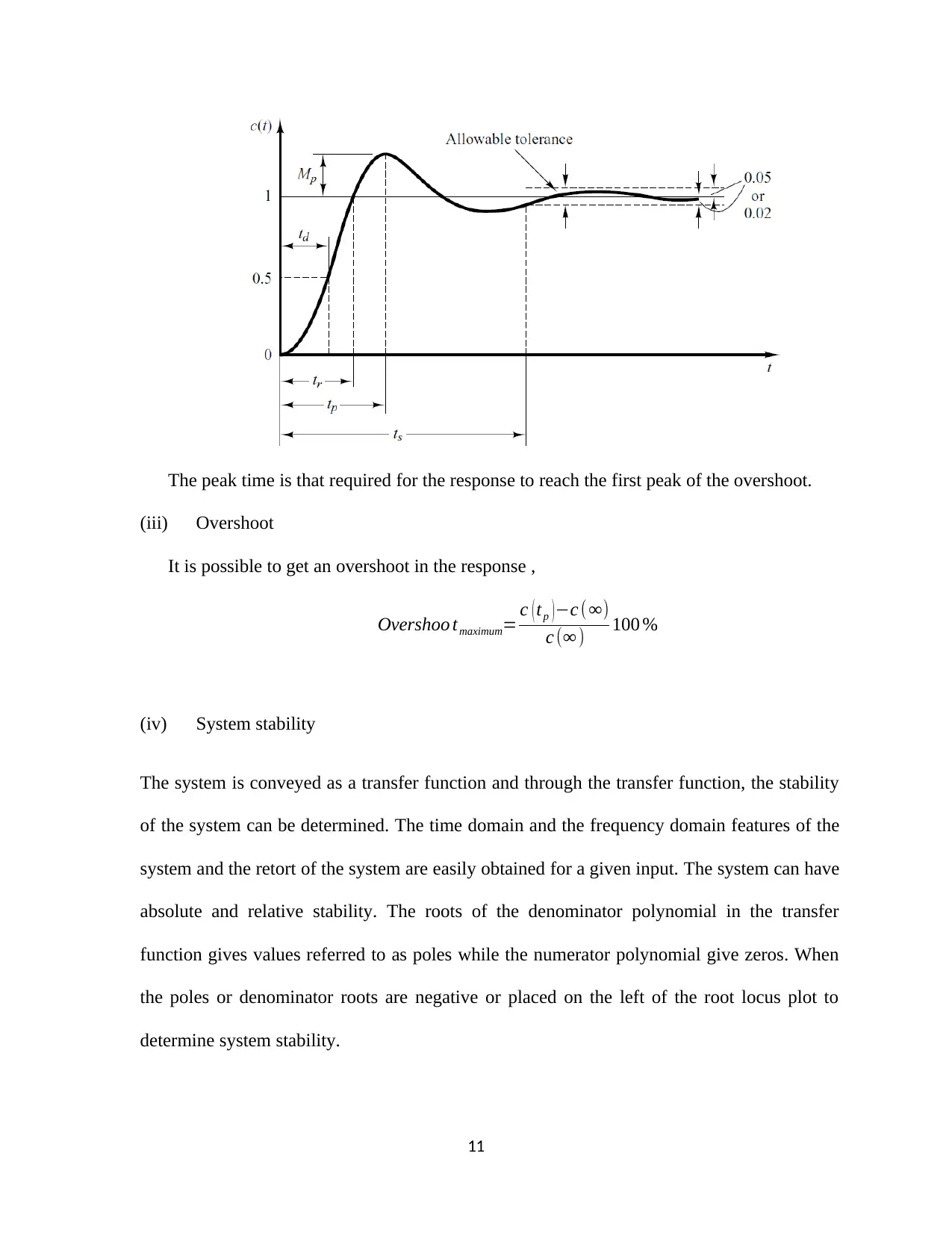
The peak time is that required for the response to reach the first peak of the overshoot.
(iii) Overshoot
It is possible to get an overshoot in the response ,
Overshoo tmaximum= c ( tp ) −c (∞)
c (∞ ) 100 %
(iv) System stability
The system is conveyed as a transfer function and through the transfer function, the stability
of the system can be determined. The time domain and the frequency domain features of the
system and the retort of the system are easily obtained for a given input. The system can have
absolute and relative stability. The roots of the denominator polynomial in the transfer
function gives values referred to as poles while the numerator polynomial give zeros. When
the poles or denominator roots are negative or placed on the left of the root locus plot to
determine system stability.
11
(iii) Overshoot
It is possible to get an overshoot in the response ,
Overshoo tmaximum= c ( tp ) −c (∞)
c (∞ ) 100 %
(iv) System stability
The system is conveyed as a transfer function and through the transfer function, the stability
of the system can be determined. The time domain and the frequency domain features of the
system and the retort of the system are easily obtained for a given input. The system can have
absolute and relative stability. The roots of the denominator polynomial in the transfer
function gives values referred to as poles while the numerator polynomial give zeros. When
the poles or denominator roots are negative or placed on the left of the root locus plot to
determine system stability.
11
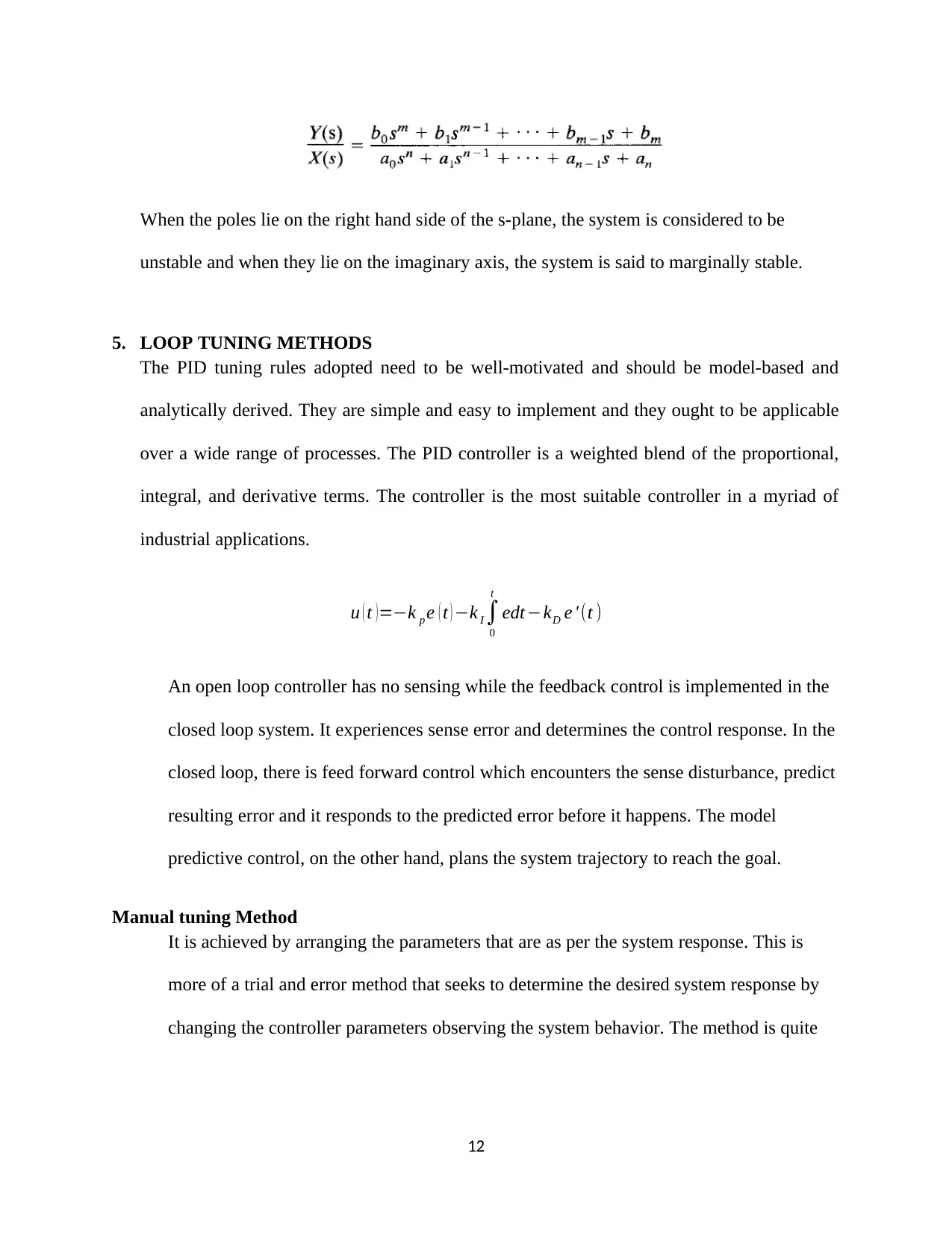
When the poles lie on the right hand side of the s-plane, the system is considered to be
unstable and when they lie on the imaginary axis, the system is said to marginally stable.
5. LOOP TUNING METHODS
The PID tuning rules adopted need to be well-motivated and should be model-based and
analytically derived. They are simple and easy to implement and they ought to be applicable
over a wide range of processes. The PID controller is a weighted blend of the proportional,
integral, and derivative terms. The controller is the most suitable controller in a myriad of
industrial applications.
u ( t ) =−k p e ( t ) −k I∫
0
t
edt −kD e ' (t )
An open loop controller has no sensing while the feedback control is implemented in the
closed loop system. It experiences sense error and determines the control response. In the
closed loop, there is feed forward control which encounters the sense disturbance, predict
resulting error and it responds to the predicted error before it happens. The model
predictive control, on the other hand, plans the system trajectory to reach the goal.
Manual tuning Method
It is achieved by arranging the parameters that are as per the system response. This is
more of a trial and error method that seeks to determine the desired system response by
changing the controller parameters observing the system behavior. The method is quite
12
unstable and when they lie on the imaginary axis, the system is said to marginally stable.
5. LOOP TUNING METHODS
The PID tuning rules adopted need to be well-motivated and should be model-based and
analytically derived. They are simple and easy to implement and they ought to be applicable
over a wide range of processes. The PID controller is a weighted blend of the proportional,
integral, and derivative terms. The controller is the most suitable controller in a myriad of
industrial applications.
u ( t ) =−k p e ( t ) −k I∫
0
t
edt −kD e ' (t )
An open loop controller has no sensing while the feedback control is implemented in the
closed loop system. It experiences sense error and determines the control response. In the
closed loop, there is feed forward control which encounters the sense disturbance, predict
resulting error and it responds to the predicted error before it happens. The model
predictive control, on the other hand, plans the system trajectory to reach the goal.
Manual tuning Method
It is achieved by arranging the parameters that are as per the system response. This is
more of a trial and error method that seeks to determine the desired system response by
changing the controller parameters observing the system behavior. The method is quite
12
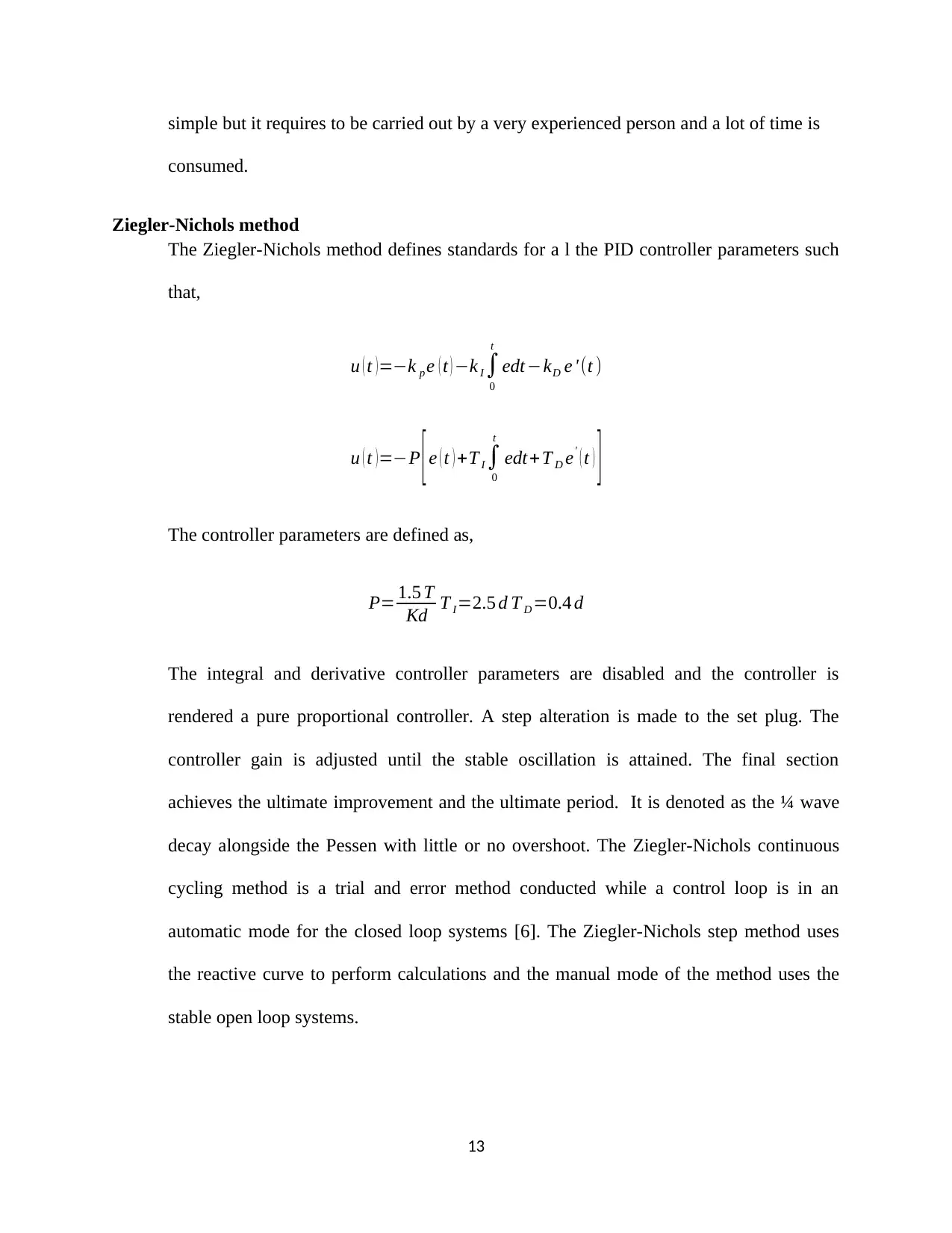
simple but it requires to be carried out by a very experienced person and a lot of time is
consumed.
Ziegler-Nichols method
The Ziegler-Nichols method defines standards for a l the PID controller parameters such
that,
u ( t )=−k p e ( t ) −k I∫
0
t
edt−kD e ' (t )
u ( t )=−P [e ( t ) +T I∫
0
t
edt+T D e' ( t ) ]
The controller parameters are defined as,
P= 1.5 T
Kd T I=2.5 d T D =0.4 d
The integral and derivative controller parameters are disabled and the controller is
rendered a pure proportional controller. A step alteration is made to the set plug. The
controller gain is adjusted until the stable oscillation is attained. The final section
achieves the ultimate improvement and the ultimate period. It is denoted as the ¼ wave
decay alongside the Pessen with little or no overshoot. The Ziegler-Nichols continuous
cycling method is a trial and error method conducted while a control loop is in an
automatic mode for the closed loop systems [6]. The Ziegler-Nichols step method uses
the reactive curve to perform calculations and the manual mode of the method uses the
stable open loop systems.
13
consumed.
Ziegler-Nichols method
The Ziegler-Nichols method defines standards for a l the PID controller parameters such
that,
u ( t )=−k p e ( t ) −k I∫
0
t
edt−kD e ' (t )
u ( t )=−P [e ( t ) +T I∫
0
t
edt+T D e' ( t ) ]
The controller parameters are defined as,
P= 1.5 T
Kd T I=2.5 d T D =0.4 d
The integral and derivative controller parameters are disabled and the controller is
rendered a pure proportional controller. A step alteration is made to the set plug. The
controller gain is adjusted until the stable oscillation is attained. The final section
achieves the ultimate improvement and the ultimate period. It is denoted as the ¼ wave
decay alongside the Pessen with little or no overshoot. The Ziegler-Nichols continuous
cycling method is a trial and error method conducted while a control loop is in an
automatic mode for the closed loop systems [6]. The Ziegler-Nichols step method uses
the reactive curve to perform calculations and the manual mode of the method uses the
stable open loop systems.
13
Secure Best Marks with AI Grader
Need help grading? Try our AI Grader for instant feedback on your assignments.
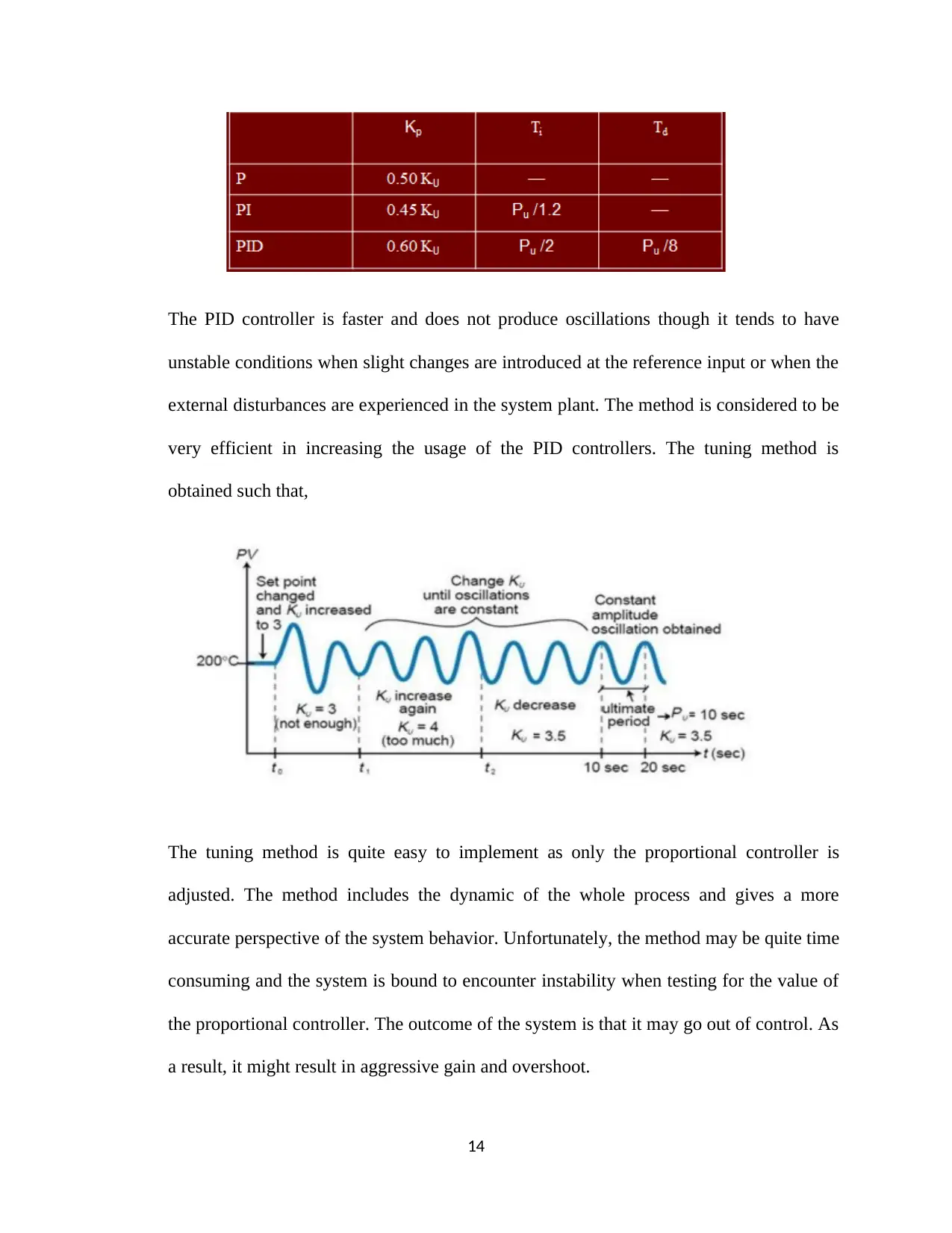
The PID controller is faster and does not produce oscillations though it tends to have
unstable conditions when slight changes are introduced at the reference input or when the
external disturbances are experienced in the system plant. The method is considered to be
very efficient in increasing the usage of the PID controllers. The tuning method is
obtained such that,
The tuning method is quite easy to implement as only the proportional controller is
adjusted. The method includes the dynamic of the whole process and gives a more
accurate perspective of the system behavior. Unfortunately, the method may be quite time
consuming and the system is bound to encounter instability when testing for the value of
the proportional controller. The outcome of the system is that it may go out of control. As
a result, it might result in aggressive gain and overshoot.
14
unstable conditions when slight changes are introduced at the reference input or when the
external disturbances are experienced in the system plant. The method is considered to be
very efficient in increasing the usage of the PID controllers. The tuning method is
obtained such that,
The tuning method is quite easy to implement as only the proportional controller is
adjusted. The method includes the dynamic of the whole process and gives a more
accurate perspective of the system behavior. Unfortunately, the method may be quite time
consuming and the system is bound to encounter instability when testing for the value of
the proportional controller. The outcome of the system is that it may go out of control. As
a result, it might result in aggressive gain and overshoot.
14
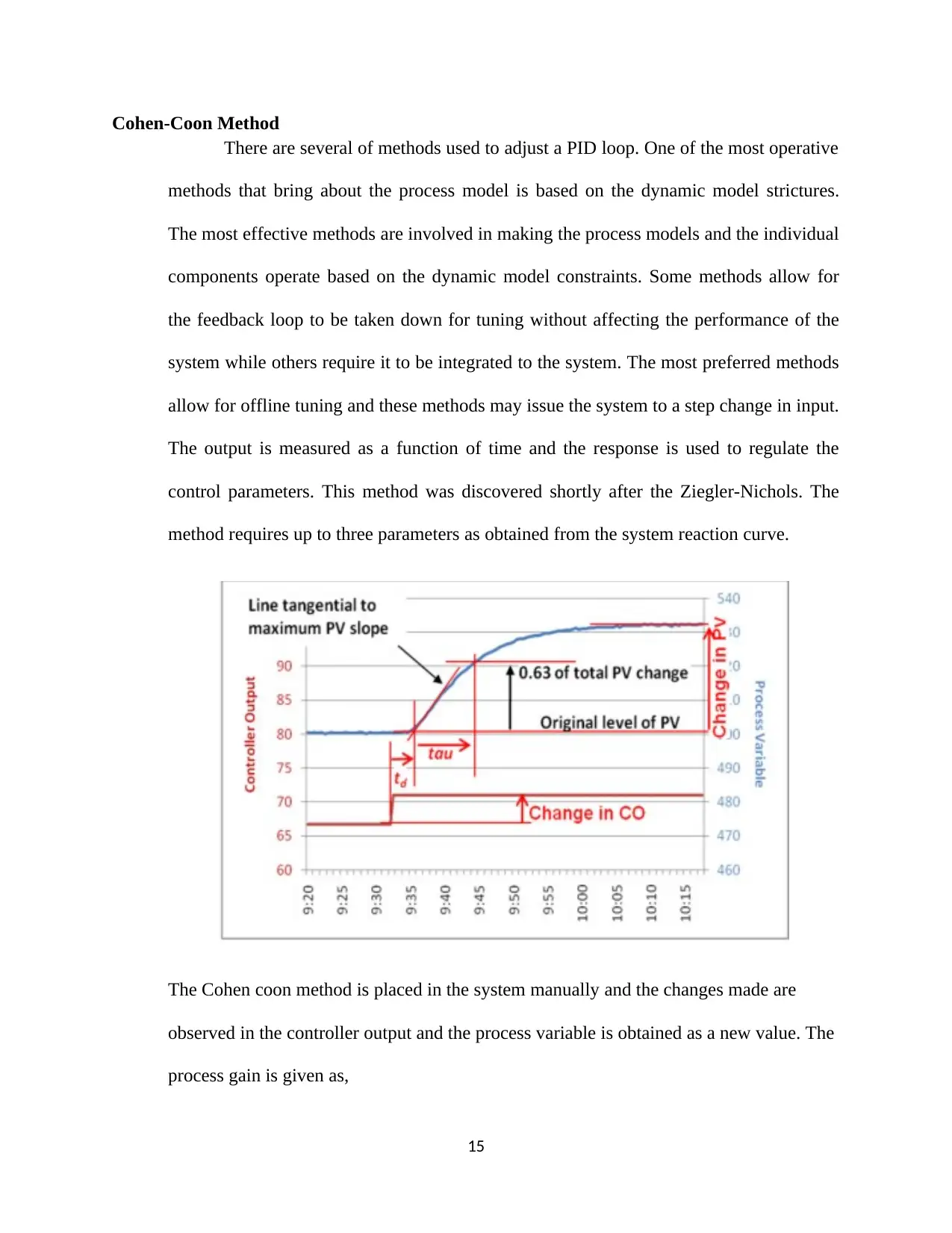
Cohen-Coon Method
There are several of methods used to adjust a PID loop. One of the most operative
methods that bring about the process model is based on the dynamic model strictures.
The most effective methods are involved in making the process models and the individual
components operate based on the dynamic model constraints. Some methods allow for
the feedback loop to be taken down for tuning without affecting the performance of the
system while others require it to be integrated to the system. The most preferred methods
allow for offline tuning and these methods may issue the system to a step change in input.
The output is measured as a function of time and the response is used to regulate the
control parameters. This method was discovered shortly after the Ziegler-Nichols. The
method requires up to three parameters as obtained from the system reaction curve.
The Cohen coon method is placed in the system manually and the changes made are
observed in the controller output and the process variable is obtained as a new value. The
process gain is given as,
15
There are several of methods used to adjust a PID loop. One of the most operative
methods that bring about the process model is based on the dynamic model strictures.
The most effective methods are involved in making the process models and the individual
components operate based on the dynamic model constraints. Some methods allow for
the feedback loop to be taken down for tuning without affecting the performance of the
system while others require it to be integrated to the system. The most preferred methods
allow for offline tuning and these methods may issue the system to a step change in input.
The output is measured as a function of time and the response is used to regulate the
control parameters. This method was discovered shortly after the Ziegler-Nichols. The
method requires up to three parameters as obtained from the system reaction curve.
The Cohen coon method is placed in the system manually and the changes made are
observed in the controller output and the process variable is obtained as a new value. The
process gain is given as,
15
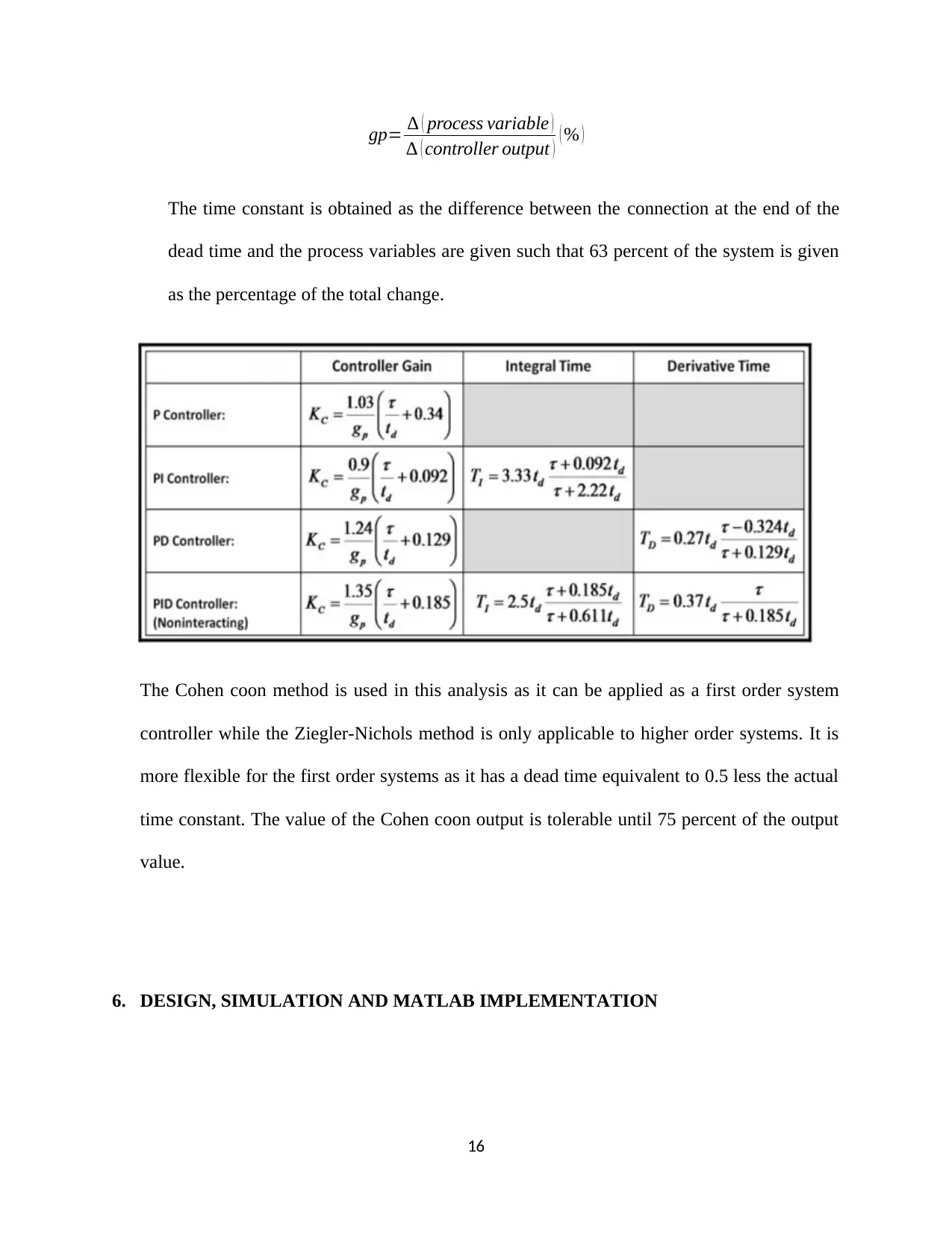
gp= ∆ ( process variable )
∆ ( controller output ) ( % )
The time constant is obtained as the difference between the connection at the end of the
dead time and the process variables are given such that 63 percent of the system is given
as the percentage of the total change.
The Cohen coon method is used in this analysis as it can be applied as a first order system
controller while the Ziegler-Nichols method is only applicable to higher order systems. It is
more flexible for the first order systems as it has a dead time equivalent to 0.5 less the actual
time constant. The value of the Cohen coon output is tolerable until 75 percent of the output
value.
6. DESIGN, SIMULATION AND MATLAB IMPLEMENTATION
16
∆ ( controller output ) ( % )
The time constant is obtained as the difference between the connection at the end of the
dead time and the process variables are given such that 63 percent of the system is given
as the percentage of the total change.
The Cohen coon method is used in this analysis as it can be applied as a first order system
controller while the Ziegler-Nichols method is only applicable to higher order systems. It is
more flexible for the first order systems as it has a dead time equivalent to 0.5 less the actual
time constant. The value of the Cohen coon output is tolerable until 75 percent of the output
value.
6. DESIGN, SIMULATION AND MATLAB IMPLEMENTATION
16
Paraphrase This Document
Need a fresh take? Get an instant paraphrase of this document with our AI Paraphraser
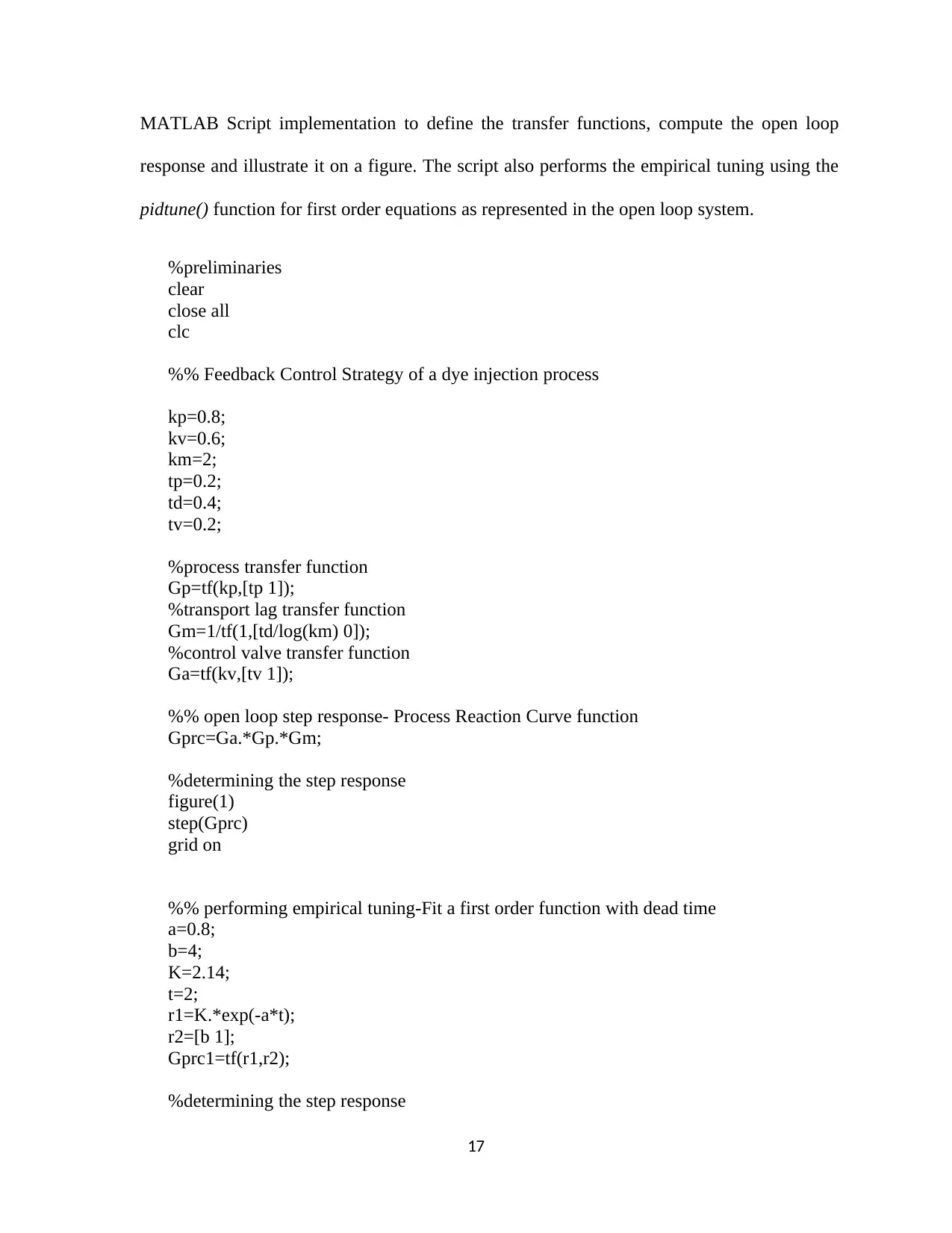
MATLAB Script implementation to define the transfer functions, compute the open loop
response and illustrate it on a figure. The script also performs the empirical tuning using the
pidtune() function for first order equations as represented in the open loop system.
%preliminaries
clear
close all
clc
%% Feedback Control Strategy of a dye injection process
kp=0.8;
kv=0.6;
km=2;
tp=0.2;
td=0.4;
tv=0.2;
%process transfer function
Gp=tf(kp,[tp 1]);
%transport lag transfer function
Gm=1/tf(1,[td/log(km) 0]);
%control valve transfer function
Ga=tf(kv,[tv 1]);
%% open loop step response- Process Reaction Curve function
Gprc=Ga.*Gp.*Gm;
%determining the step response
figure(1)
step(Gprc)
grid on
%% performing empirical tuning-Fit a first order function with dead time
a=0.8;
b=4;
K=2.14;
t=2;
r1=K.*exp(-a*t);
r2=[b 1];
Gprc1=tf(r1,r2);
%determining the step response
17
response and illustrate it on a figure. The script also performs the empirical tuning using the
pidtune() function for first order equations as represented in the open loop system.
%preliminaries
clear
close all
clc
%% Feedback Control Strategy of a dye injection process
kp=0.8;
kv=0.6;
km=2;
tp=0.2;
td=0.4;
tv=0.2;
%process transfer function
Gp=tf(kp,[tp 1]);
%transport lag transfer function
Gm=1/tf(1,[td/log(km) 0]);
%control valve transfer function
Ga=tf(kv,[tv 1]);
%% open loop step response- Process Reaction Curve function
Gprc=Ga.*Gp.*Gm;
%determining the step response
figure(1)
step(Gprc)
grid on
%% performing empirical tuning-Fit a first order function with dead time
a=0.8;
b=4;
K=2.14;
t=2;
r1=K.*exp(-a*t);
r2=[b 1];
Gprc1=tf(r1,r2);
%determining the step response
17
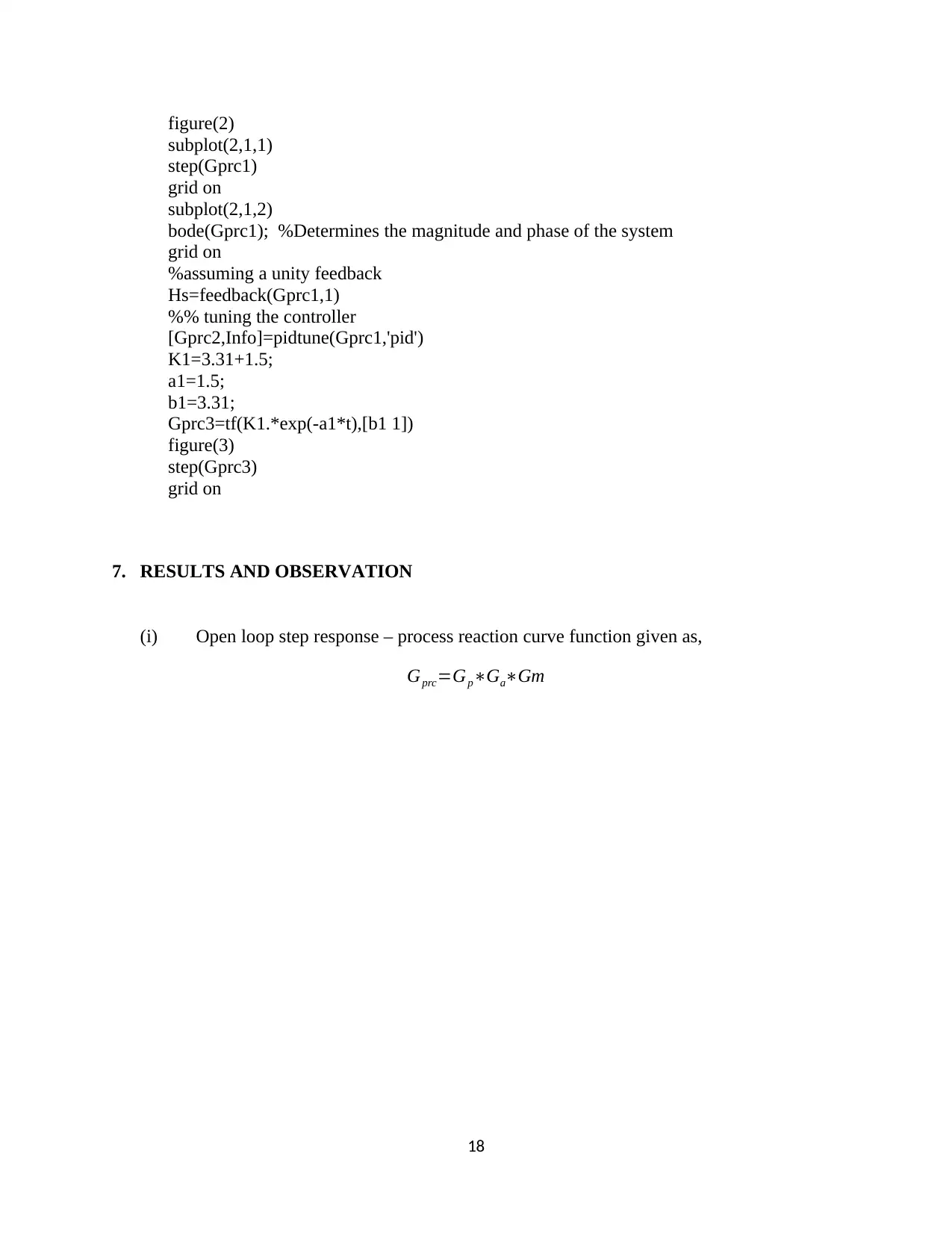
figure(2)
subplot(2,1,1)
step(Gprc1)
grid on
subplot(2,1,2)
bode(Gprc1); %Determines the magnitude and phase of the system
grid on
%assuming a unity feedback
Hs=feedback(Gprc1,1)
%% tuning the controller
[Gprc2,Info]=pidtune(Gprc1,'pid')
K1=3.31+1.5;
a1=1.5;
b1=3.31;
Gprc3=tf(K1.*exp(-a1*t),[b1 1])
figure(3)
step(Gprc3)
grid on
7. RESULTS AND OBSERVATION
(i) Open loop step response – process reaction curve function given as,
Gprc=Gp∗Ga∗Gm
18
subplot(2,1,1)
step(Gprc1)
grid on
subplot(2,1,2)
bode(Gprc1); %Determines the magnitude and phase of the system
grid on
%assuming a unity feedback
Hs=feedback(Gprc1,1)
%% tuning the controller
[Gprc2,Info]=pidtune(Gprc1,'pid')
K1=3.31+1.5;
a1=1.5;
b1=3.31;
Gprc3=tf(K1.*exp(-a1*t),[b1 1])
figure(3)
step(Gprc3)
grid on
7. RESULTS AND OBSERVATION
(i) Open loop step response – process reaction curve function given as,
Gprc=Gp∗Ga∗Gm
18
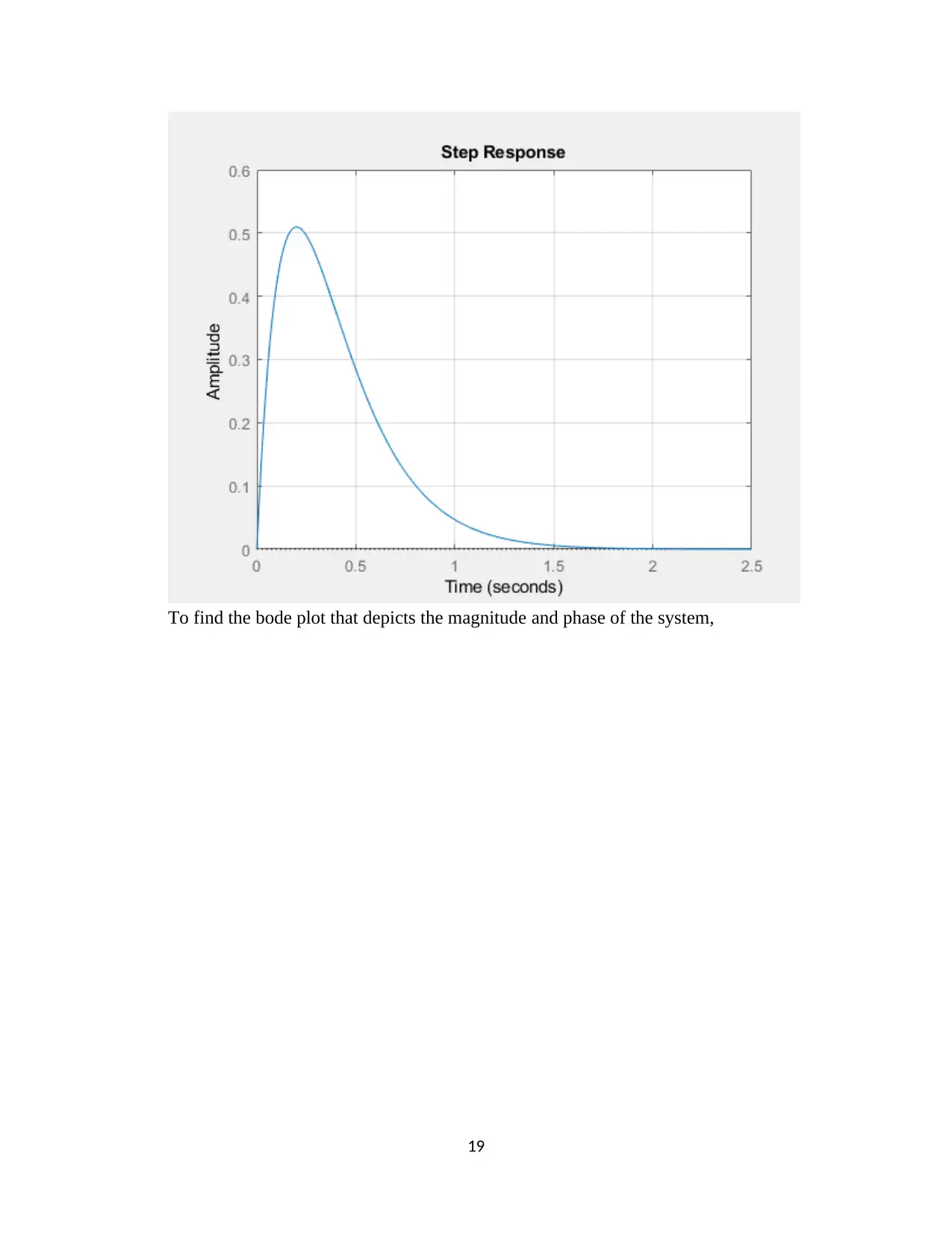
To find the bode plot that depicts the magnitude and phase of the system,
19
19
Secure Best Marks with AI Grader
Need help grading? Try our AI Grader for instant feedback on your assignments.
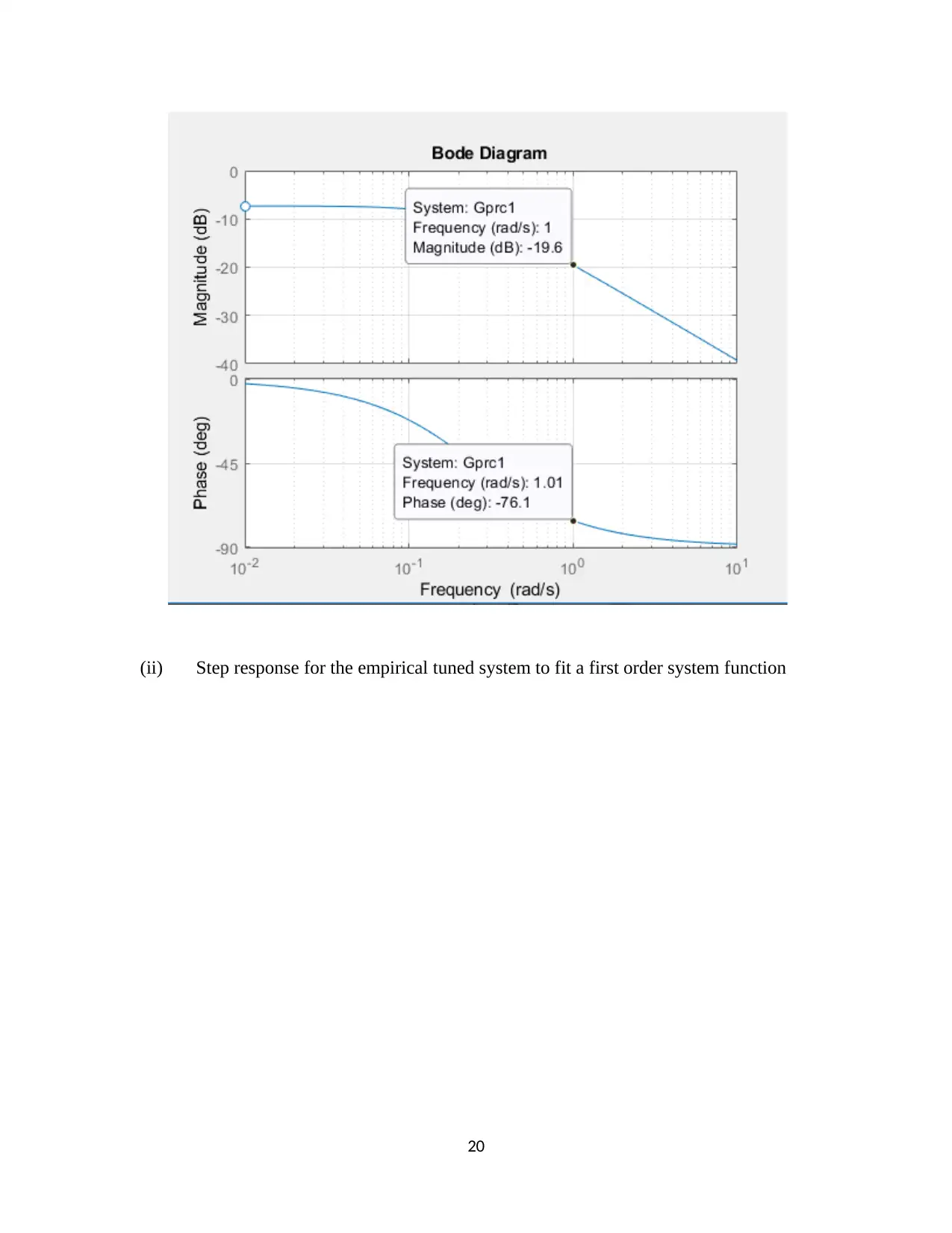
(ii) Step response for the empirical tuned system to fit a first order system function
20
20
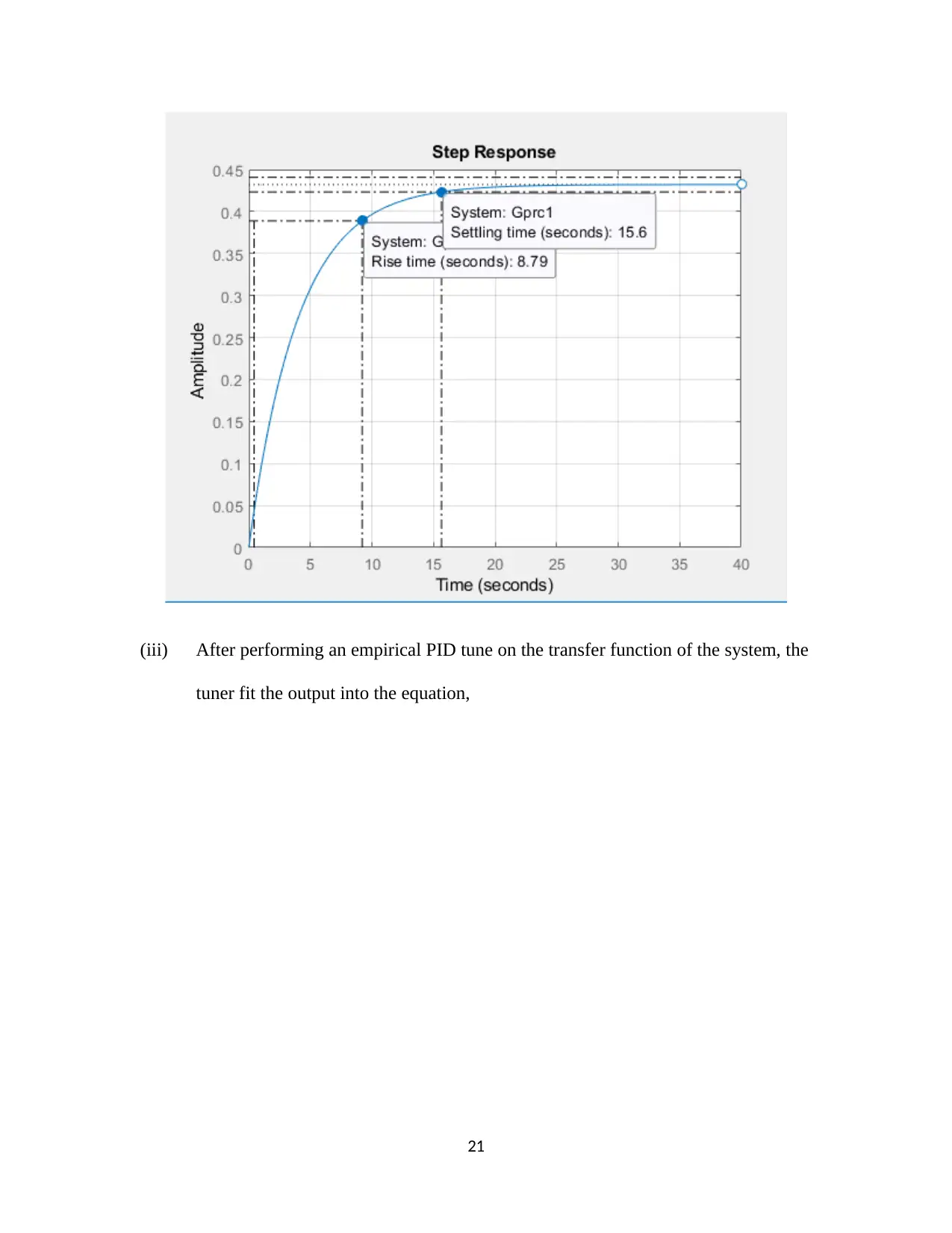
(iii) After performing an empirical PID tune on the transfer function of the system, the
tuner fit the output into the equation,
21
tuner fit the output into the equation,
21
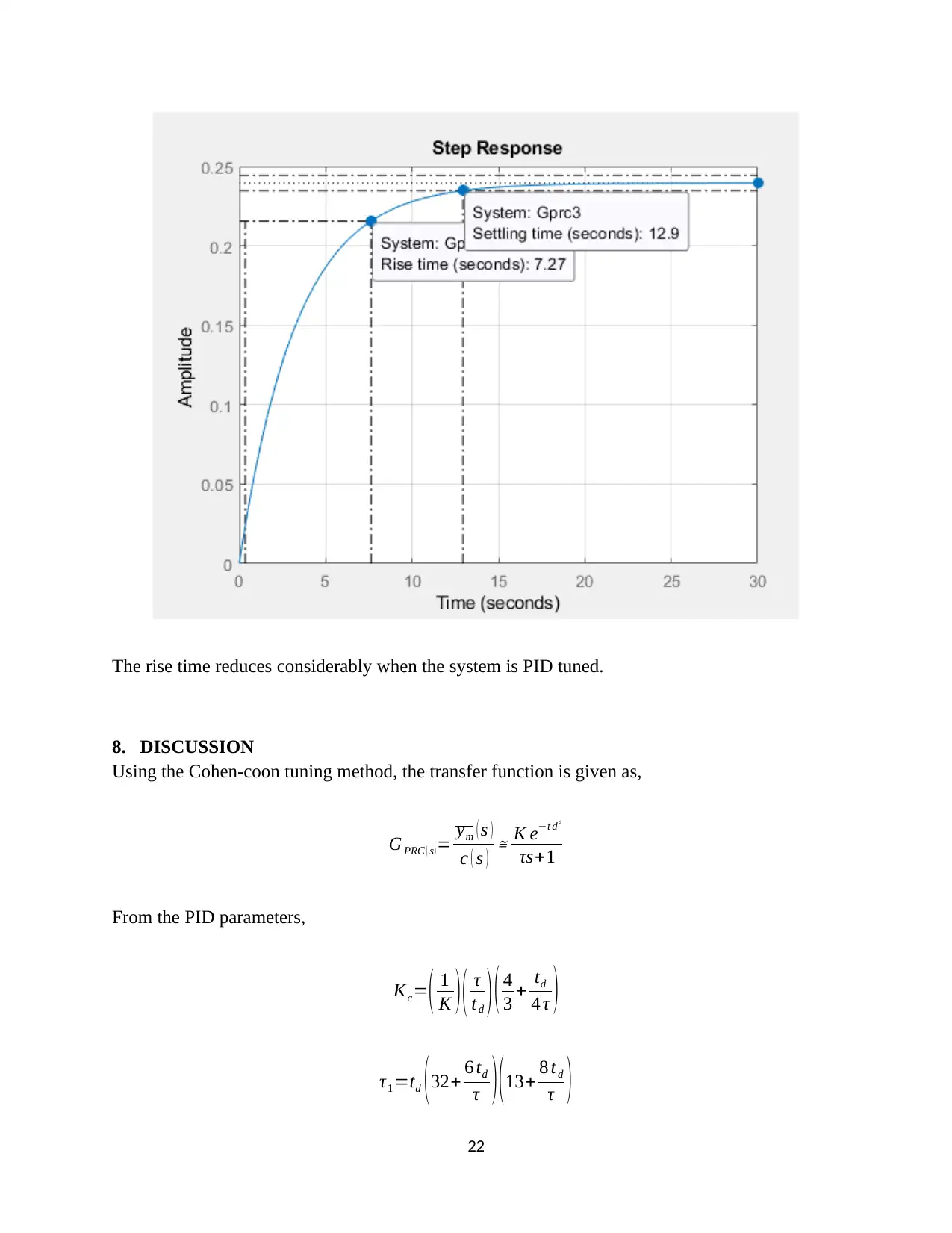
The rise time reduces considerably when the system is PID tuned.
8. DISCUSSION
Using the Cohen-coon tuning method, the transfer function is given as,
GPRC ( s ) = ym ( s )
c ( s ) ≅ K e−t d s
τs+1
From the PID parameters,
Kc= ( 1
K ) ( τ
td )( 4
3 + td
4 τ )
τ1 =td (32+ 6 td
τ )(13+ 8 td
τ )
22
8. DISCUSSION
Using the Cohen-coon tuning method, the transfer function is given as,
GPRC ( s ) = ym ( s )
c ( s ) ≅ K e−t d s
τs+1
From the PID parameters,
Kc= ( 1
K ) ( τ
td )( 4
3 + td
4 τ )
τ1 =td (32+ 6 td
τ )(13+ 8 td
τ )
22
Paraphrase This Document
Need a fresh take? Get an instant paraphrase of this document with our AI Paraphraser
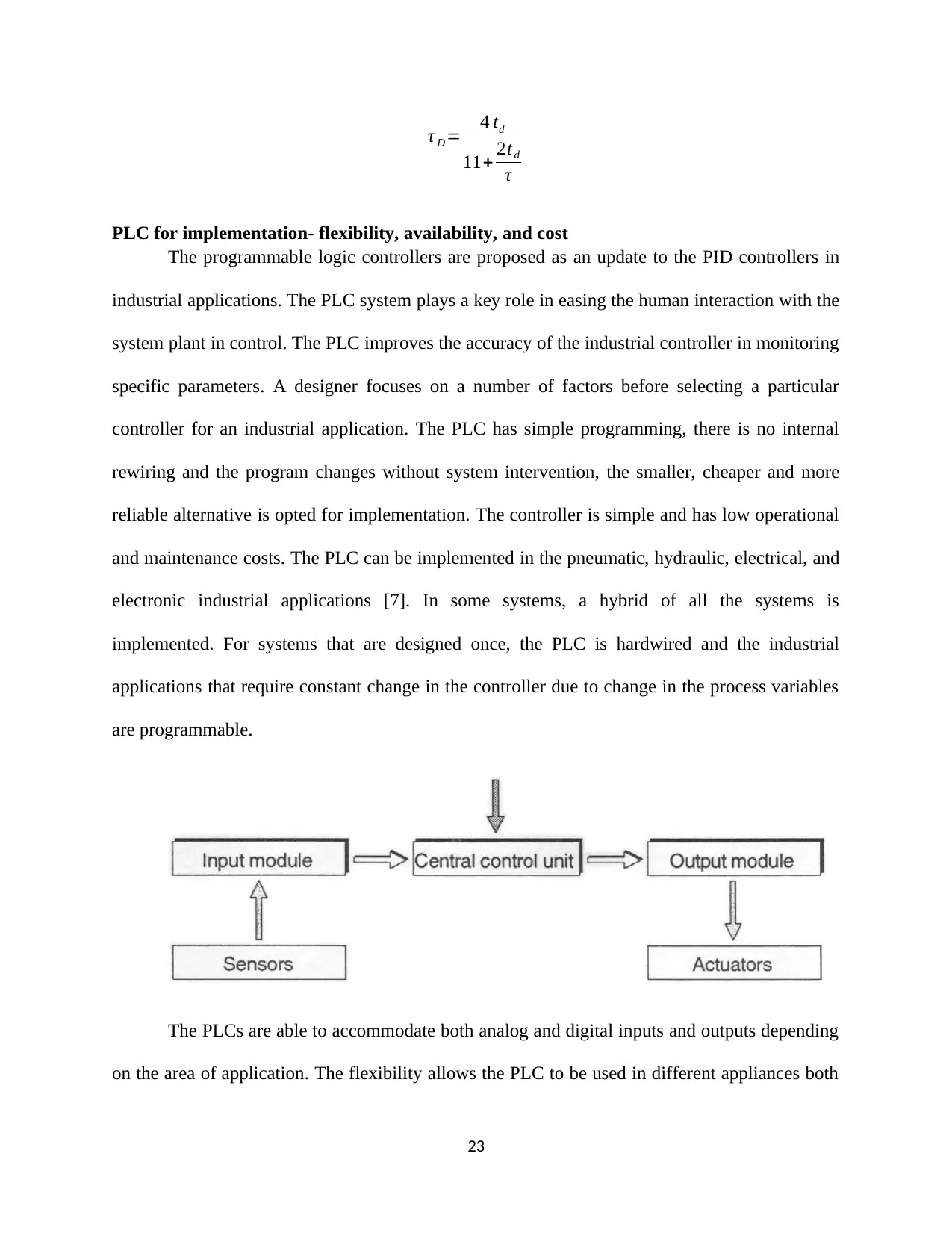
τ D = 4 td
11+ 2td
τ
PLC for implementation- flexibility, availability, and cost
The programmable logic controllers are proposed as an update to the PID controllers in
industrial applications. The PLC system plays a key role in easing the human interaction with the
system plant in control. The PLC improves the accuracy of the industrial controller in monitoring
specific parameters. A designer focuses on a number of factors before selecting a particular
controller for an industrial application. The PLC has simple programming, there is no internal
rewiring and the program changes without system intervention, the smaller, cheaper and more
reliable alternative is opted for implementation. The controller is simple and has low operational
and maintenance costs. The PLC can be implemented in the pneumatic, hydraulic, electrical, and
electronic industrial applications [7]. In some systems, a hybrid of all the systems is
implemented. For systems that are designed once, the PLC is hardwired and the industrial
applications that require constant change in the controller due to change in the process variables
are programmable.
The PLCs are able to accommodate both analog and digital inputs and outputs depending
on the area of application. The flexibility allows the PLC to be used in different appliances both
23
11+ 2td
τ
PLC for implementation- flexibility, availability, and cost
The programmable logic controllers are proposed as an update to the PID controllers in
industrial applications. The PLC system plays a key role in easing the human interaction with the
system plant in control. The PLC improves the accuracy of the industrial controller in monitoring
specific parameters. A designer focuses on a number of factors before selecting a particular
controller for an industrial application. The PLC has simple programming, there is no internal
rewiring and the program changes without system intervention, the smaller, cheaper and more
reliable alternative is opted for implementation. The controller is simple and has low operational
and maintenance costs. The PLC can be implemented in the pneumatic, hydraulic, electrical, and
electronic industrial applications [7]. In some systems, a hybrid of all the systems is
implemented. For systems that are designed once, the PLC is hardwired and the industrial
applications that require constant change in the controller due to change in the process variables
are programmable.
The PLCs are able to accommodate both analog and digital inputs and outputs depending
on the area of application. The flexibility allows the PLC to be used in different appliances both
23
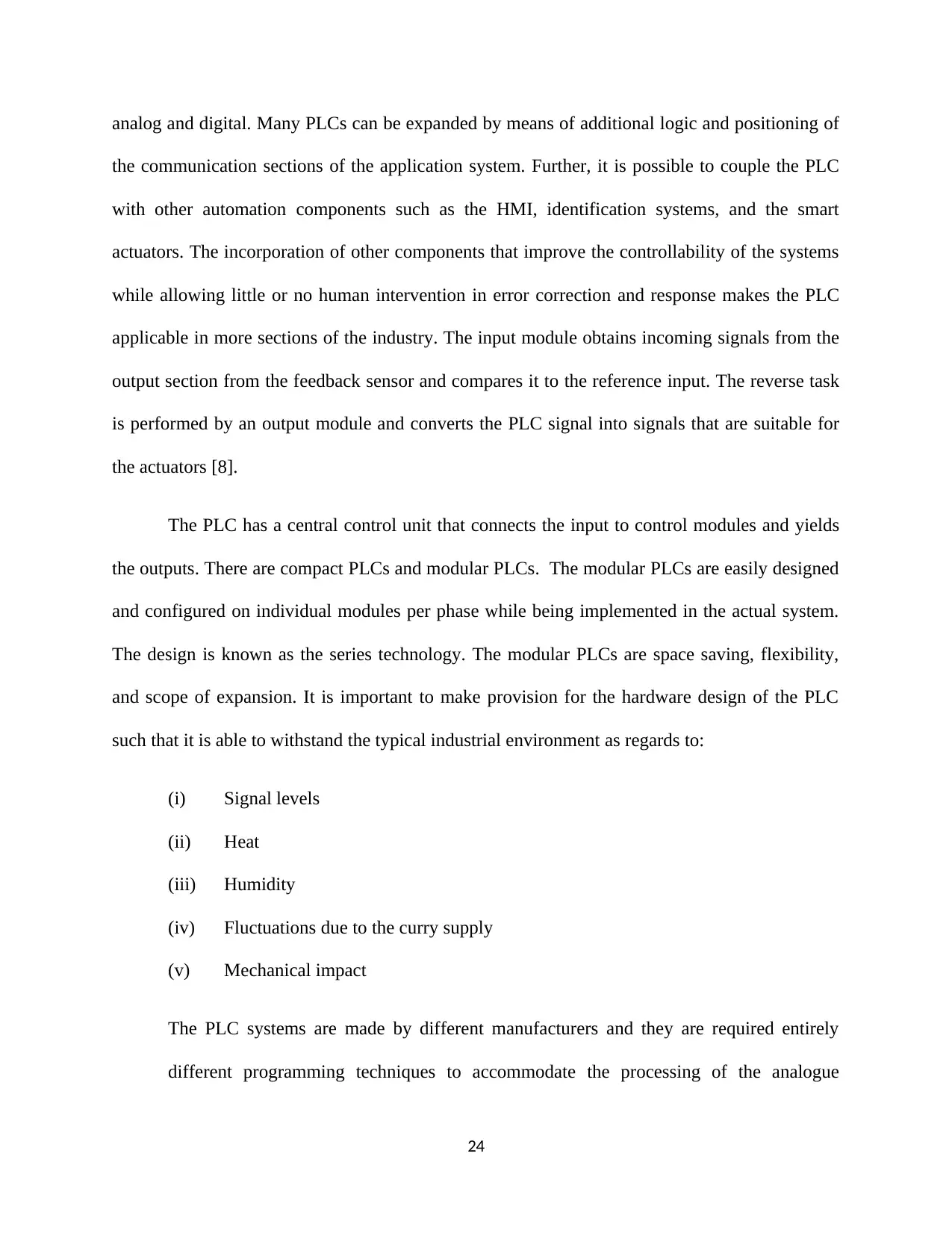
analog and digital. Many PLCs can be expanded by means of additional logic and positioning of
the communication sections of the application system. Further, it is possible to couple the PLC
with other automation components such as the HMI, identification systems, and the smart
actuators. The incorporation of other components that improve the controllability of the systems
while allowing little or no human intervention in error correction and response makes the PLC
applicable in more sections of the industry. The input module obtains incoming signals from the
output section from the feedback sensor and compares it to the reference input. The reverse task
is performed by an output module and converts the PLC signal into signals that are suitable for
the actuators [8].
The PLC has a central control unit that connects the input to control modules and yields
the outputs. There are compact PLCs and modular PLCs. The modular PLCs are easily designed
and configured on individual modules per phase while being implemented in the actual system.
The design is known as the series technology. The modular PLCs are space saving, flexibility,
and scope of expansion. It is important to make provision for the hardware design of the PLC
such that it is able to withstand the typical industrial environment as regards to:
(i) Signal levels
(ii) Heat
(iii) Humidity
(iv) Fluctuations due to the curry supply
(v) Mechanical impact
The PLC systems are made by different manufacturers and they are required entirely
different programming techniques to accommodate the processing of the analogue
24
the communication sections of the application system. Further, it is possible to couple the PLC
with other automation components such as the HMI, identification systems, and the smart
actuators. The incorporation of other components that improve the controllability of the systems
while allowing little or no human intervention in error correction and response makes the PLC
applicable in more sections of the industry. The input module obtains incoming signals from the
output section from the feedback sensor and compares it to the reference input. The reverse task
is performed by an output module and converts the PLC signal into signals that are suitable for
the actuators [8].
The PLC has a central control unit that connects the input to control modules and yields
the outputs. There are compact PLCs and modular PLCs. The modular PLCs are easily designed
and configured on individual modules per phase while being implemented in the actual system.
The design is known as the series technology. The modular PLCs are space saving, flexibility,
and scope of expansion. It is important to make provision for the hardware design of the PLC
such that it is able to withstand the typical industrial environment as regards to:
(i) Signal levels
(ii) Heat
(iii) Humidity
(iv) Fluctuations due to the curry supply
(v) Mechanical impact
The PLC systems are made by different manufacturers and they are required entirely
different programming techniques to accommodate the processing of the analogue
24
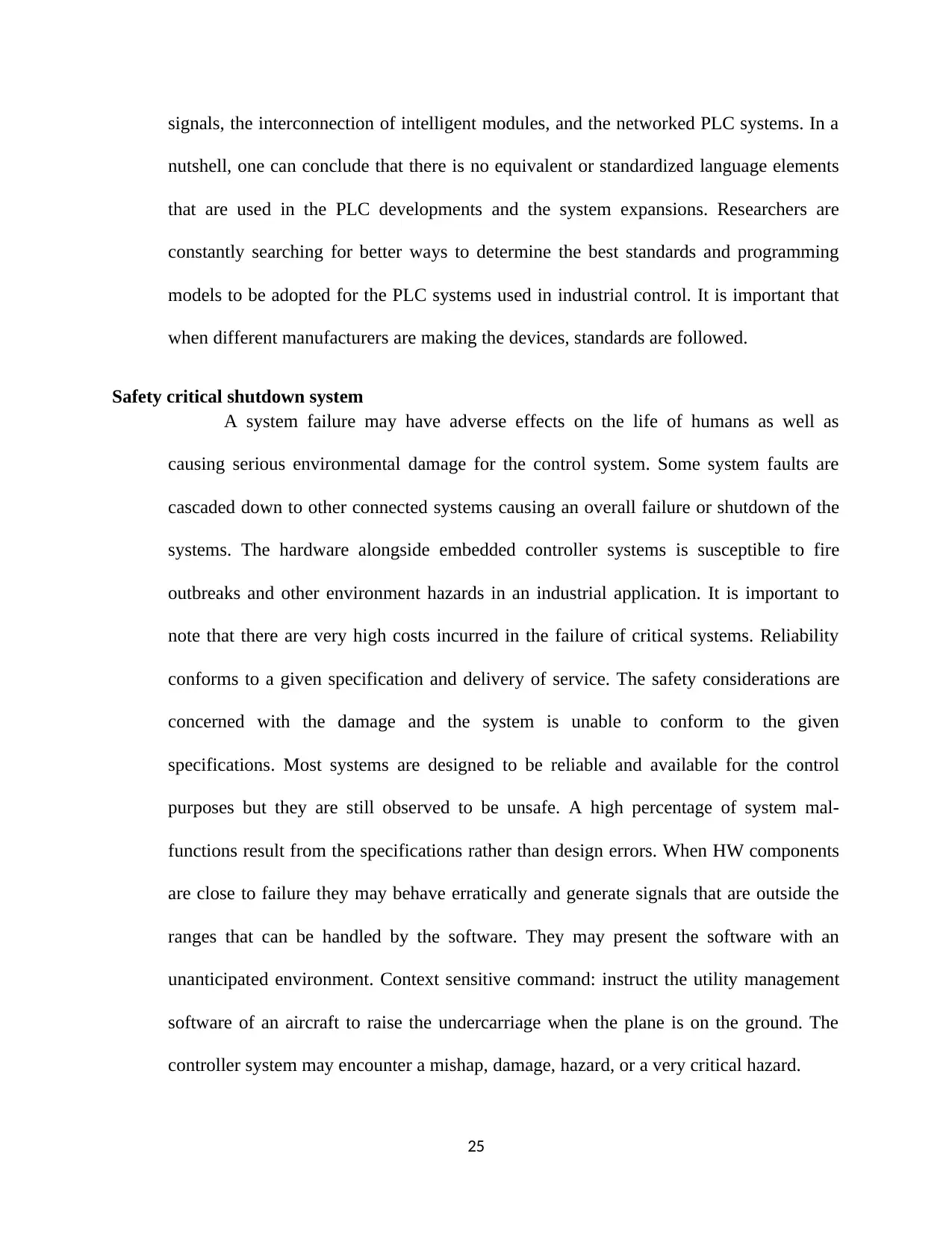
signals, the interconnection of intelligent modules, and the networked PLC systems. In a
nutshell, one can conclude that there is no equivalent or standardized language elements
that are used in the PLC developments and the system expansions. Researchers are
constantly searching for better ways to determine the best standards and programming
models to be adopted for the PLC systems used in industrial control. It is important that
when different manufacturers are making the devices, standards are followed.
Safety critical shutdown system
A system failure may have adverse effects on the life of humans as well as
causing serious environmental damage for the control system. Some system faults are
cascaded down to other connected systems causing an overall failure or shutdown of the
systems. The hardware alongside embedded controller systems is susceptible to fire
outbreaks and other environment hazards in an industrial application. It is important to
note that there are very high costs incurred in the failure of critical systems. Reliability
conforms to a given specification and delivery of service. The safety considerations are
concerned with the damage and the system is unable to conform to the given
specifications. Most systems are designed to be reliable and available for the control
purposes but they are still observed to be unsafe. A high percentage of system mal-
functions result from the specifications rather than design errors. When HW components
are close to failure they may behave erratically and generate signals that are outside the
ranges that can be handled by the software. They may present the software with an
unanticipated environment. Context sensitive command: instruct the utility management
software of an aircraft to raise the undercarriage when the plane is on the ground. The
controller system may encounter a mishap, damage, hazard, or a very critical hazard.
25
nutshell, one can conclude that there is no equivalent or standardized language elements
that are used in the PLC developments and the system expansions. Researchers are
constantly searching for better ways to determine the best standards and programming
models to be adopted for the PLC systems used in industrial control. It is important that
when different manufacturers are making the devices, standards are followed.
Safety critical shutdown system
A system failure may have adverse effects on the life of humans as well as
causing serious environmental damage for the control system. Some system faults are
cascaded down to other connected systems causing an overall failure or shutdown of the
systems. The hardware alongside embedded controller systems is susceptible to fire
outbreaks and other environment hazards in an industrial application. It is important to
note that there are very high costs incurred in the failure of critical systems. Reliability
conforms to a given specification and delivery of service. The safety considerations are
concerned with the damage and the system is unable to conform to the given
specifications. Most systems are designed to be reliable and available for the control
purposes but they are still observed to be unsafe. A high percentage of system mal-
functions result from the specifications rather than design errors. When HW components
are close to failure they may behave erratically and generate signals that are outside the
ranges that can be handled by the software. They may present the software with an
unanticipated environment. Context sensitive command: instruct the utility management
software of an aircraft to raise the undercarriage when the plane is on the ground. The
controller system may encounter a mishap, damage, hazard, or a very critical hazard.
25
Secure Best Marks with AI Grader
Need help grading? Try our AI Grader for instant feedback on your assignments.
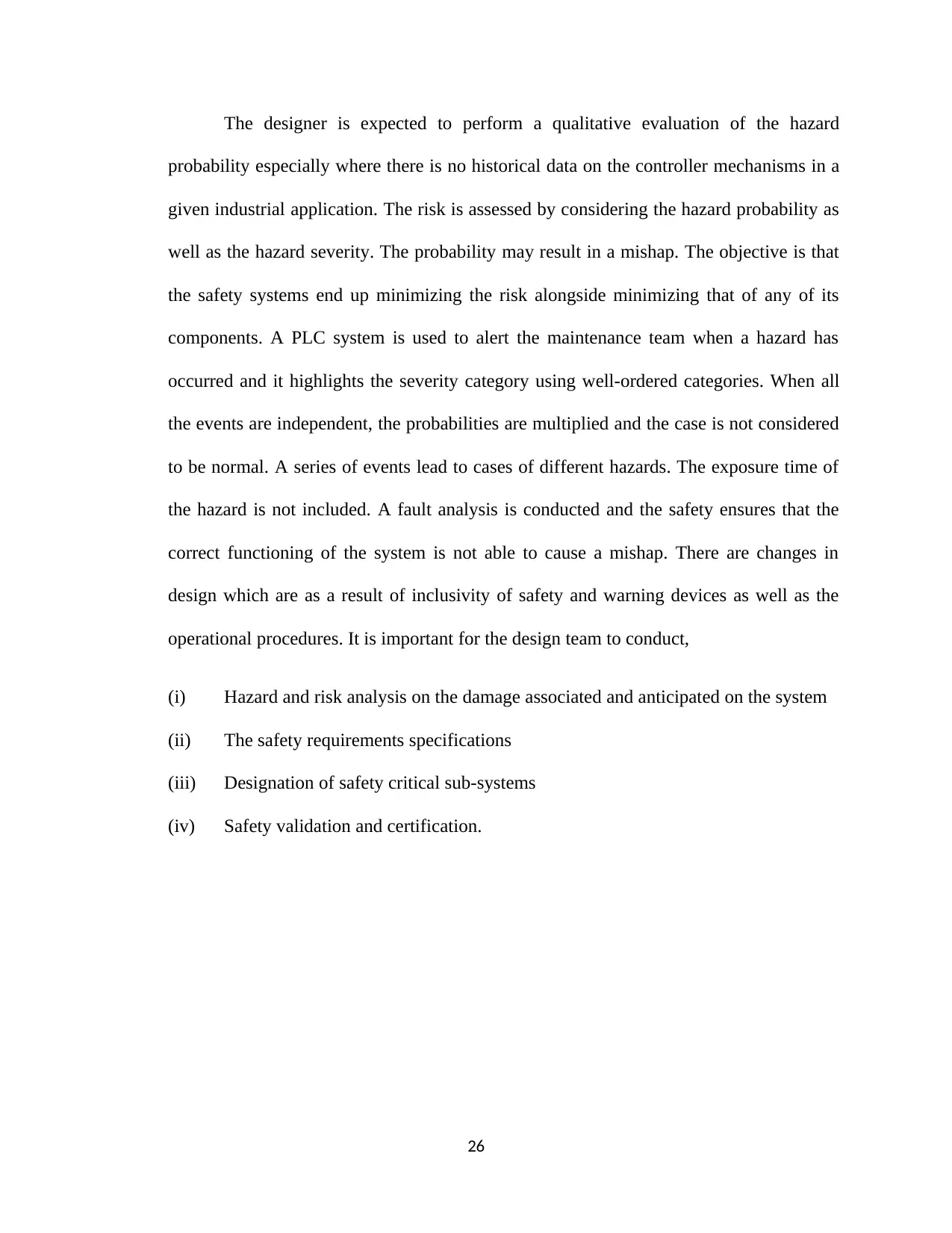
The designer is expected to perform a qualitative evaluation of the hazard
probability especially where there is no historical data on the controller mechanisms in a
given industrial application. The risk is assessed by considering the hazard probability as
well as the hazard severity. The probability may result in a mishap. The objective is that
the safety systems end up minimizing the risk alongside minimizing that of any of its
components. A PLC system is used to alert the maintenance team when a hazard has
occurred and it highlights the severity category using well-ordered categories. When all
the events are independent, the probabilities are multiplied and the case is not considered
to be normal. A series of events lead to cases of different hazards. The exposure time of
the hazard is not included. A fault analysis is conducted and the safety ensures that the
correct functioning of the system is not able to cause a mishap. There are changes in
design which are as a result of inclusivity of safety and warning devices as well as the
operational procedures. It is important for the design team to conduct,
(i) Hazard and risk analysis on the damage associated and anticipated on the system
(ii) The safety requirements specifications
(iii) Designation of safety critical sub-systems
(iv) Safety validation and certification.
26
probability especially where there is no historical data on the controller mechanisms in a
given industrial application. The risk is assessed by considering the hazard probability as
well as the hazard severity. The probability may result in a mishap. The objective is that
the safety systems end up minimizing the risk alongside minimizing that of any of its
components. A PLC system is used to alert the maintenance team when a hazard has
occurred and it highlights the severity category using well-ordered categories. When all
the events are independent, the probabilities are multiplied and the case is not considered
to be normal. A series of events lead to cases of different hazards. The exposure time of
the hazard is not included. A fault analysis is conducted and the safety ensures that the
correct functioning of the system is not able to cause a mishap. There are changes in
design which are as a result of inclusivity of safety and warning devices as well as the
operational procedures. It is important for the design team to conduct,
(i) Hazard and risk analysis on the damage associated and anticipated on the system
(ii) The safety requirements specifications
(iii) Designation of safety critical sub-systems
(iv) Safety validation and certification.
26
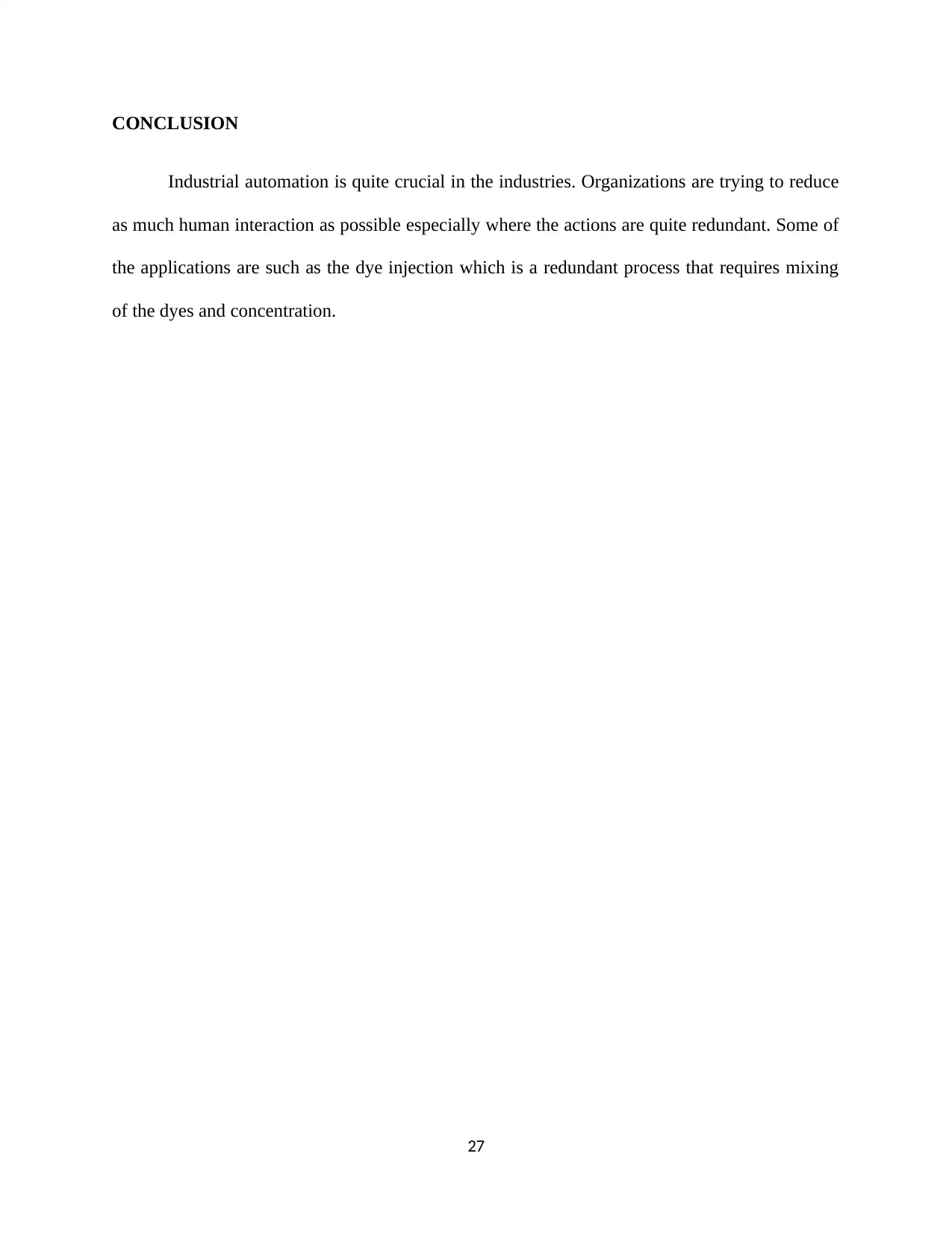
CONCLUSION
Industrial automation is quite crucial in the industries. Organizations are trying to reduce
as much human interaction as possible especially where the actions are quite redundant. Some of
the applications are such as the dye injection which is a redundant process that requires mixing
of the dyes and concentration.
27
Industrial automation is quite crucial in the industries. Organizations are trying to reduce
as much human interaction as possible especially where the actions are quite redundant. Some of
the applications are such as the dye injection which is a redundant process that requires mixing
of the dyes and concentration.
27
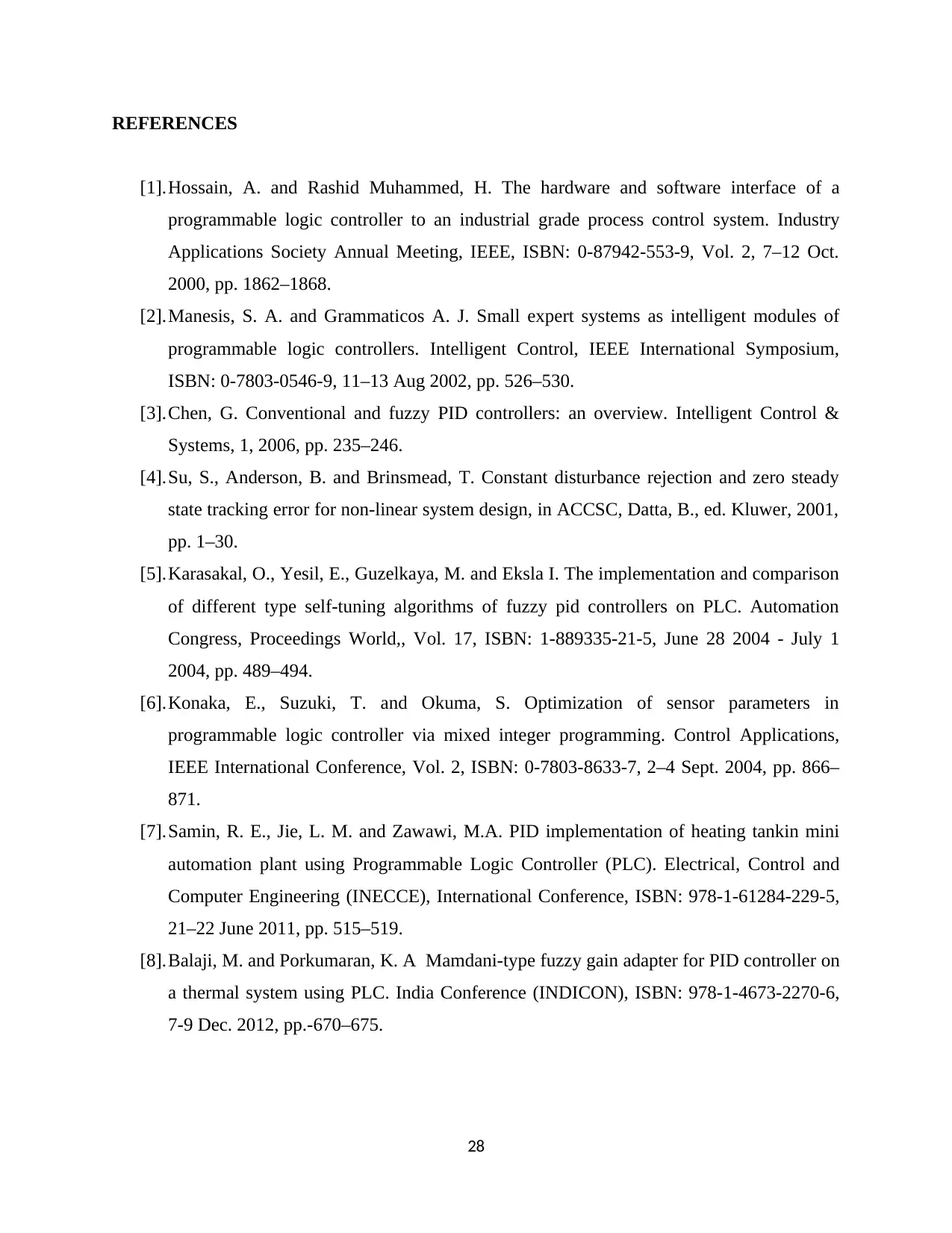
REFERENCES
[1].Hossain, A. and Rashid Muhammed, H. The hardware and software interface of a
programmable logic controller to an industrial grade process control system. Industry
Applications Society Annual Meeting, IEEE, ISBN: 0-87942-553-9, Vol. 2, 7–12 Oct.
2000, pp. 1862–1868.
[2].Manesis, S. A. and Grammaticos A. J. Small expert systems as intelligent modules of
programmable logic controllers. Intelligent Control, IEEE International Symposium,
ISBN: 0-7803-0546-9, 11–13 Aug 2002, pp. 526–530.
[3].Chen, G. Conventional and fuzzy PID controllers: an overview. Intelligent Control &
Systems, 1, 2006, pp. 235–246.
[4].Su, S., Anderson, B. and Brinsmead, T. Constant disturbance rejection and zero steady
state tracking error for non-linear system design, in ACCSC, Datta, B., ed. Kluwer, 2001,
pp. 1–30.
[5].Karasakal, O., Yesil, E., Guzelkaya, M. and Eksla I. The implementation and comparison
of different type self-tuning algorithms of fuzzy pid controllers on PLC. Automation
Congress, Proceedings World,, Vol. 17, ISBN: 1-889335-21-5, June 28 2004 - July 1
2004, pp. 489–494.
[6].Konaka, E., Suzuki, T. and Okuma, S. Optimization of sensor parameters in
programmable logic controller via mixed integer programming. Control Applications,
IEEE International Conference, Vol. 2, ISBN: 0-7803-8633-7, 2–4 Sept. 2004, pp. 866–
871.
[7].Samin, R. E., Jie, L. M. and Zawawi, M.A. PID implementation of heating tankin mini
automation plant using Programmable Logic Controller (PLC). Electrical, Control and
Computer Engineering (INECCE), International Conference, ISBN: 978-1-61284-229-5,
21–22 June 2011, pp. 515–519.
[8].Balaji, M. and Porkumaran, K. A Mamdani-type fuzzy gain adapter for PID controller on
a thermal system using PLC. India Conference (INDICON), ISBN: 978-1-4673-2270-6,
7-9 Dec. 2012, pp.-670–675.
28
[1].Hossain, A. and Rashid Muhammed, H. The hardware and software interface of a
programmable logic controller to an industrial grade process control system. Industry
Applications Society Annual Meeting, IEEE, ISBN: 0-87942-553-9, Vol. 2, 7–12 Oct.
2000, pp. 1862–1868.
[2].Manesis, S. A. and Grammaticos A. J. Small expert systems as intelligent modules of
programmable logic controllers. Intelligent Control, IEEE International Symposium,
ISBN: 0-7803-0546-9, 11–13 Aug 2002, pp. 526–530.
[3].Chen, G. Conventional and fuzzy PID controllers: an overview. Intelligent Control &
Systems, 1, 2006, pp. 235–246.
[4].Su, S., Anderson, B. and Brinsmead, T. Constant disturbance rejection and zero steady
state tracking error for non-linear system design, in ACCSC, Datta, B., ed. Kluwer, 2001,
pp. 1–30.
[5].Karasakal, O., Yesil, E., Guzelkaya, M. and Eksla I. The implementation and comparison
of different type self-tuning algorithms of fuzzy pid controllers on PLC. Automation
Congress, Proceedings World,, Vol. 17, ISBN: 1-889335-21-5, June 28 2004 - July 1
2004, pp. 489–494.
[6].Konaka, E., Suzuki, T. and Okuma, S. Optimization of sensor parameters in
programmable logic controller via mixed integer programming. Control Applications,
IEEE International Conference, Vol. 2, ISBN: 0-7803-8633-7, 2–4 Sept. 2004, pp. 866–
871.
[7].Samin, R. E., Jie, L. M. and Zawawi, M.A. PID implementation of heating tankin mini
automation plant using Programmable Logic Controller (PLC). Electrical, Control and
Computer Engineering (INECCE), International Conference, ISBN: 978-1-61284-229-5,
21–22 June 2011, pp. 515–519.
[8].Balaji, M. and Porkumaran, K. A Mamdani-type fuzzy gain adapter for PID controller on
a thermal system using PLC. India Conference (INDICON), ISBN: 978-1-4673-2270-6,
7-9 Dec. 2012, pp.-670–675.
28
1 out of 28
Related Documents
![[object Object]](/_next/image/?url=%2F_next%2Fstatic%2Fmedia%2Flogo.6d15ce61.png&w=640&q=75)
Your All-in-One AI-Powered Toolkit for Academic Success.
+13062052269
info@desklib.com
Available 24*7 on WhatsApp / Email
Unlock your academic potential
© 2024 | Zucol Services PVT LTD | All rights reserved.