Recommendation System for the E-Commerce Businesses
VerifiedAdded on  2022/10/01
|15
|3700
|211
AI Summary
This document provides a survey on the recommendation system and its importance for e-commerce organizations. It discusses the working procedures, methods, and contribution to business intelligence. The three types of methods are content-based, collaborative, and hybrid recommendation systems.
Contribute Materials
Your contribution can guide someone’s learning journey. Share your
documents today.
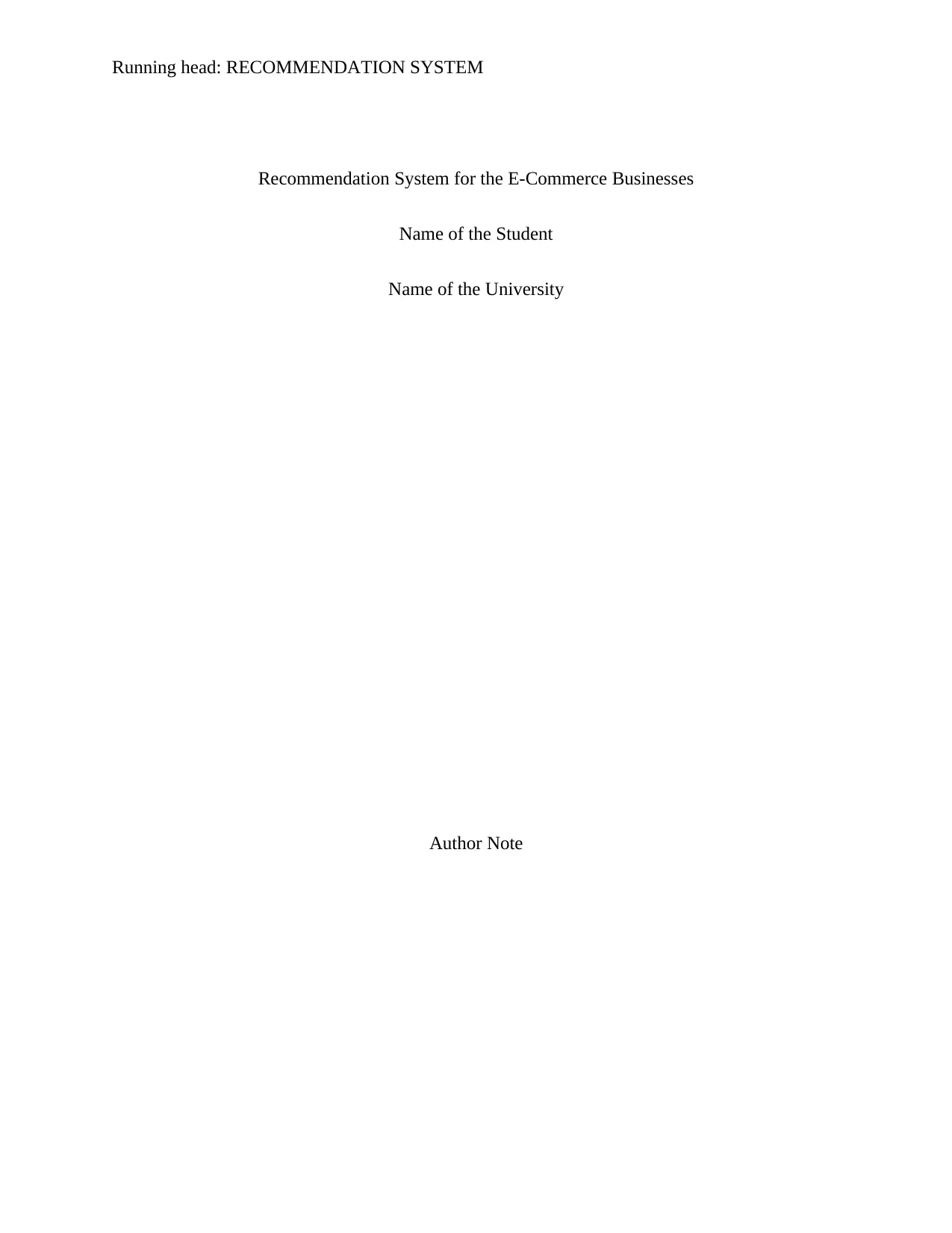
Running head: RECOMMENDATION SYSTEM
Recommendation System for the E-Commerce Businesses
Name of the Student
Name of the University
Author Note
Recommendation System for the E-Commerce Businesses
Name of the Student
Name of the University
Author Note
Secure Best Marks with AI Grader
Need help grading? Try our AI Grader for instant feedback on your assignments.
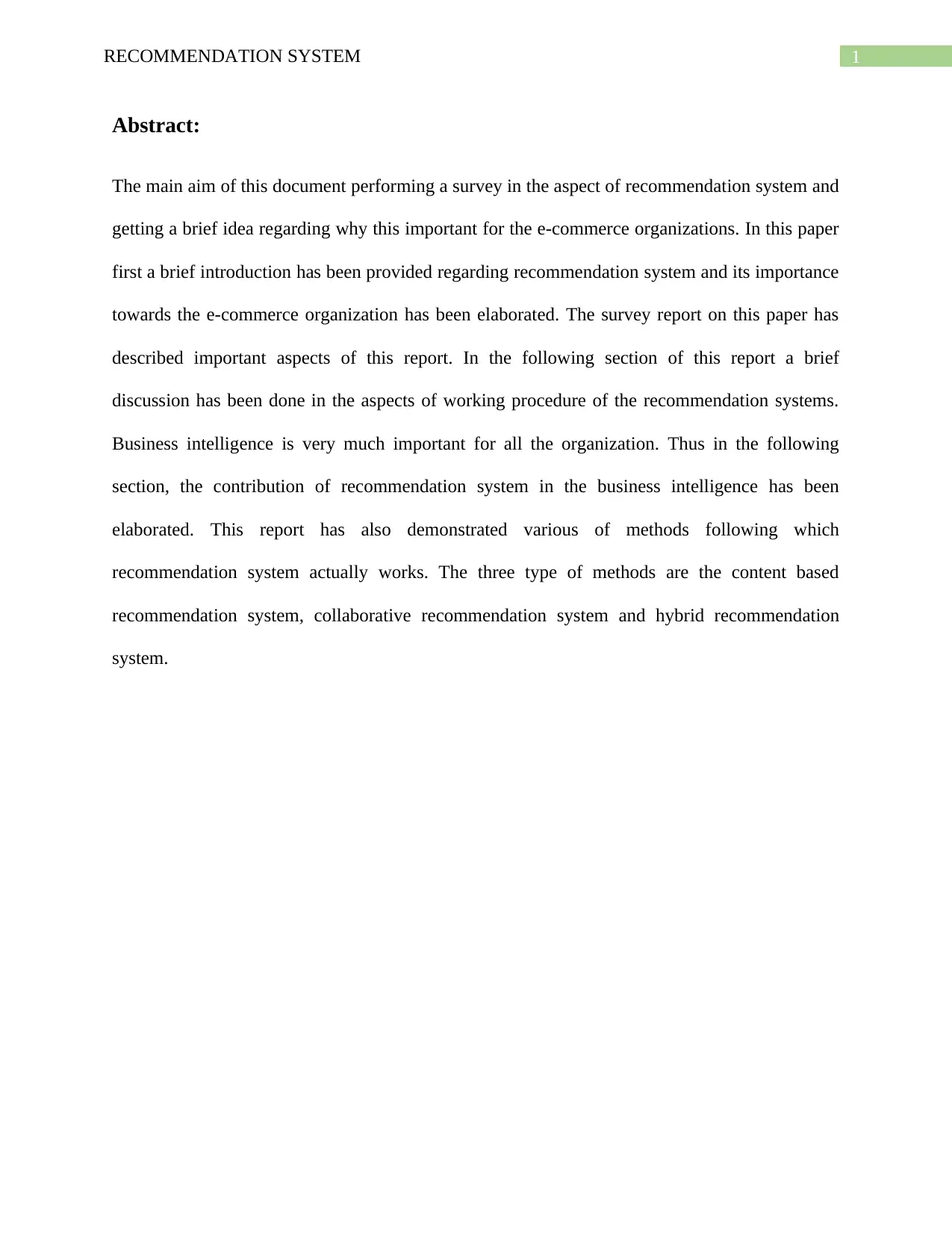
1RECOMMENDATION SYSTEM
Abstract:
The main aim of this document performing a survey in the aspect of recommendation system and
getting a brief idea regarding why this important for the e-commerce organizations. In this paper
first a brief introduction has been provided regarding recommendation system and its importance
towards the e-commerce organization has been elaborated. The survey report on this paper has
described important aspects of this report. In the following section of this report a brief
discussion has been done in the aspects of working procedure of the recommendation systems.
Business intelligence is very much important for all the organization. Thus in the following
section, the contribution of recommendation system in the business intelligence has been
elaborated. This report has also demonstrated various of methods following which
recommendation system actually works. The three type of methods are the content based
recommendation system, collaborative recommendation system and hybrid recommendation
system.
Abstract:
The main aim of this document performing a survey in the aspect of recommendation system and
getting a brief idea regarding why this important for the e-commerce organizations. In this paper
first a brief introduction has been provided regarding recommendation system and its importance
towards the e-commerce organization has been elaborated. The survey report on this paper has
described important aspects of this report. In the following section of this report a brief
discussion has been done in the aspects of working procedure of the recommendation systems.
Business intelligence is very much important for all the organization. Thus in the following
section, the contribution of recommendation system in the business intelligence has been
elaborated. This report has also demonstrated various of methods following which
recommendation system actually works. The three type of methods are the content based
recommendation system, collaborative recommendation system and hybrid recommendation
system.
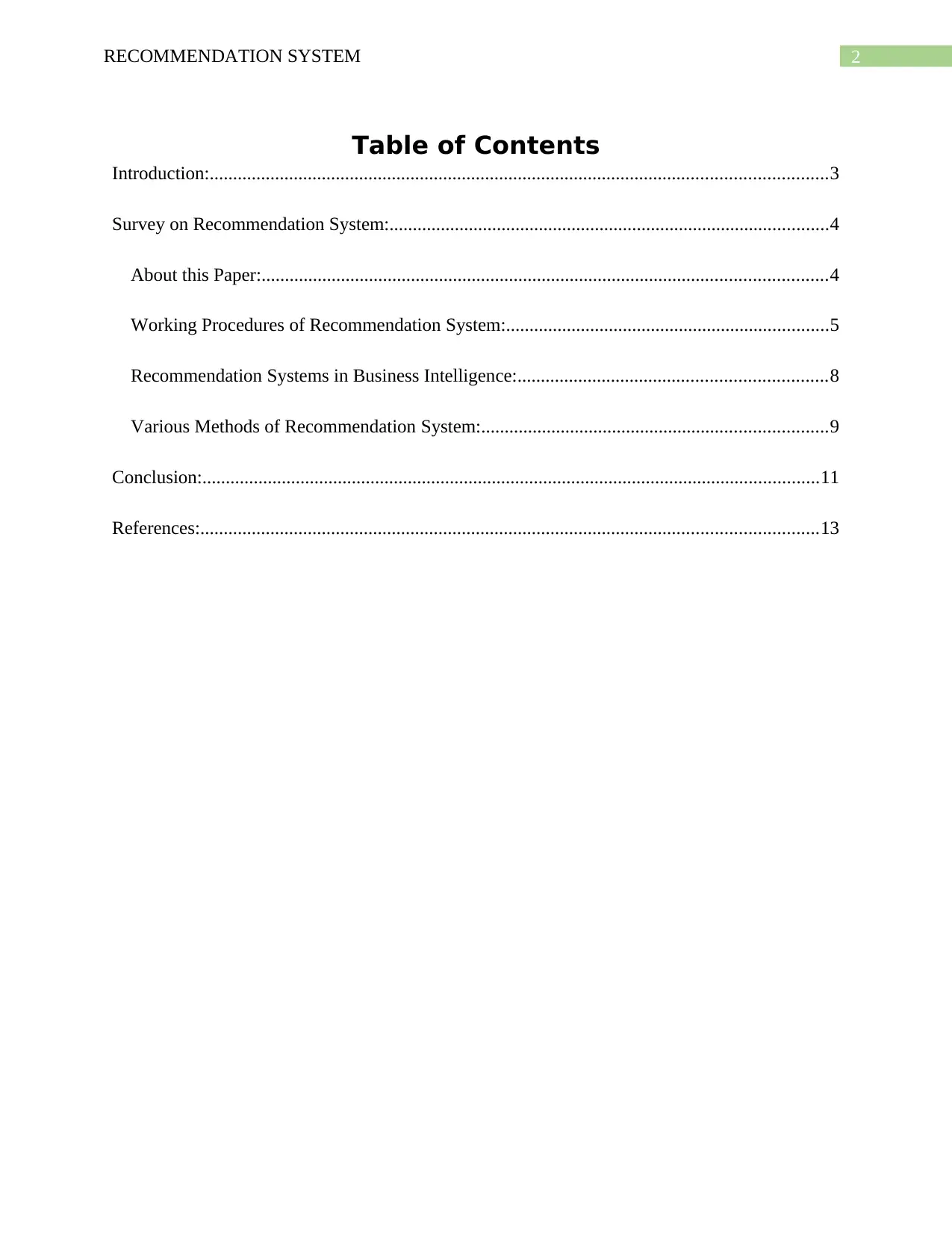
2RECOMMENDATION SYSTEM
Table of Contents
Introduction:....................................................................................................................................3
Survey on Recommendation System:..............................................................................................4
About this Paper:.........................................................................................................................4
Working Procedures of Recommendation System:.....................................................................5
Recommendation Systems in Business Intelligence:..................................................................8
Various Methods of Recommendation System:..........................................................................9
Conclusion:....................................................................................................................................11
References:....................................................................................................................................13
Table of Contents
Introduction:....................................................................................................................................3
Survey on Recommendation System:..............................................................................................4
About this Paper:.........................................................................................................................4
Working Procedures of Recommendation System:.....................................................................5
Recommendation Systems in Business Intelligence:..................................................................8
Various Methods of Recommendation System:..........................................................................9
Conclusion:....................................................................................................................................11
References:....................................................................................................................................13
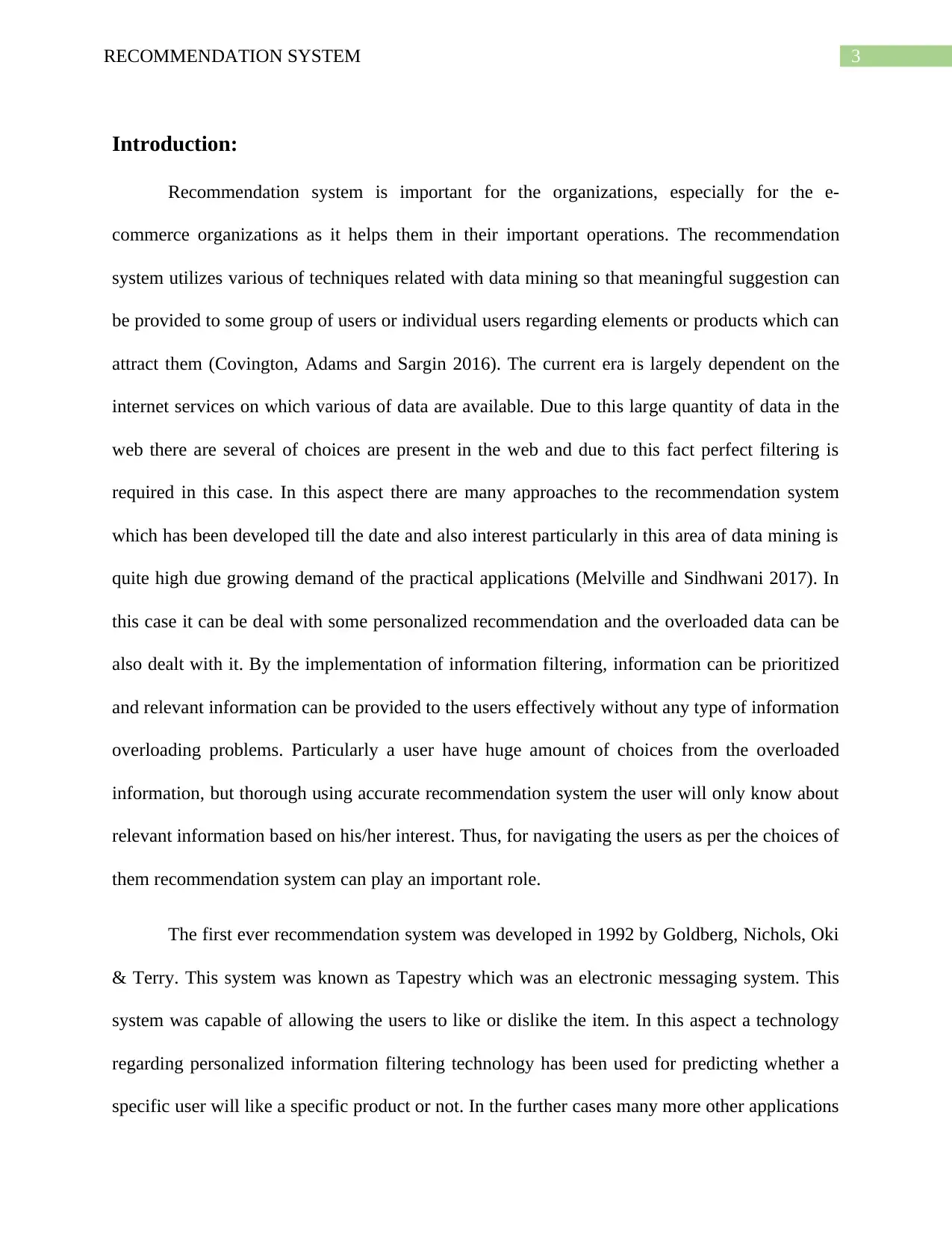
3RECOMMENDATION SYSTEM
Introduction:
Recommendation system is important for the organizations, especially for the e-
commerce organizations as it helps them in their important operations. The recommendation
system utilizes various of techniques related with data mining so that meaningful suggestion can
be provided to some group of users or individual users regarding elements or products which can
attract them (Covington, Adams and Sargin 2016). The current era is largely dependent on the
internet services on which various of data are available. Due to this large quantity of data in the
web there are several of choices are present in the web and due to this fact perfect filtering is
required in this case. In this aspect there are many approaches to the recommendation system
which has been developed till the date and also interest particularly in this area of data mining is
quite high due growing demand of the practical applications (Melville and Sindhwani 2017). In
this case it can be deal with some personalized recommendation and the overloaded data can be
also dealt with it. By the implementation of information filtering, information can be prioritized
and relevant information can be provided to the users effectively without any type of information
overloading problems. Particularly a user have huge amount of choices from the overloaded
information, but thorough using accurate recommendation system the user will only know about
relevant information based on his/her interest. Thus, for navigating the users as per the choices of
them recommendation system can play an important role.
The first ever recommendation system was developed in 1992 by Goldberg, Nichols, Oki
& Terry. This system was known as Tapestry which was an electronic messaging system. This
system was capable of allowing the users to like or dislike the item. In this aspect a technology
regarding personalized information filtering technology has been used for predicting whether a
specific user will like a specific product or not. In the further cases many more other applications
Introduction:
Recommendation system is important for the organizations, especially for the e-
commerce organizations as it helps them in their important operations. The recommendation
system utilizes various of techniques related with data mining so that meaningful suggestion can
be provided to some group of users or individual users regarding elements or products which can
attract them (Covington, Adams and Sargin 2016). The current era is largely dependent on the
internet services on which various of data are available. Due to this large quantity of data in the
web there are several of choices are present in the web and due to this fact perfect filtering is
required in this case. In this aspect there are many approaches to the recommendation system
which has been developed till the date and also interest particularly in this area of data mining is
quite high due growing demand of the practical applications (Melville and Sindhwani 2017). In
this case it can be deal with some personalized recommendation and the overloaded data can be
also dealt with it. By the implementation of information filtering, information can be prioritized
and relevant information can be provided to the users effectively without any type of information
overloading problems. Particularly a user have huge amount of choices from the overloaded
information, but thorough using accurate recommendation system the user will only know about
relevant information based on his/her interest. Thus, for navigating the users as per the choices of
them recommendation system can play an important role.
The first ever recommendation system was developed in 1992 by Goldberg, Nichols, Oki
& Terry. This system was known as Tapestry which was an electronic messaging system. This
system was capable of allowing the users to like or dislike the item. In this aspect a technology
regarding personalized information filtering technology has been used for predicting whether a
specific user will like a specific product or not. In the further cases many more other applications
Secure Best Marks with AI Grader
Need help grading? Try our AI Grader for instant feedback on your assignments.
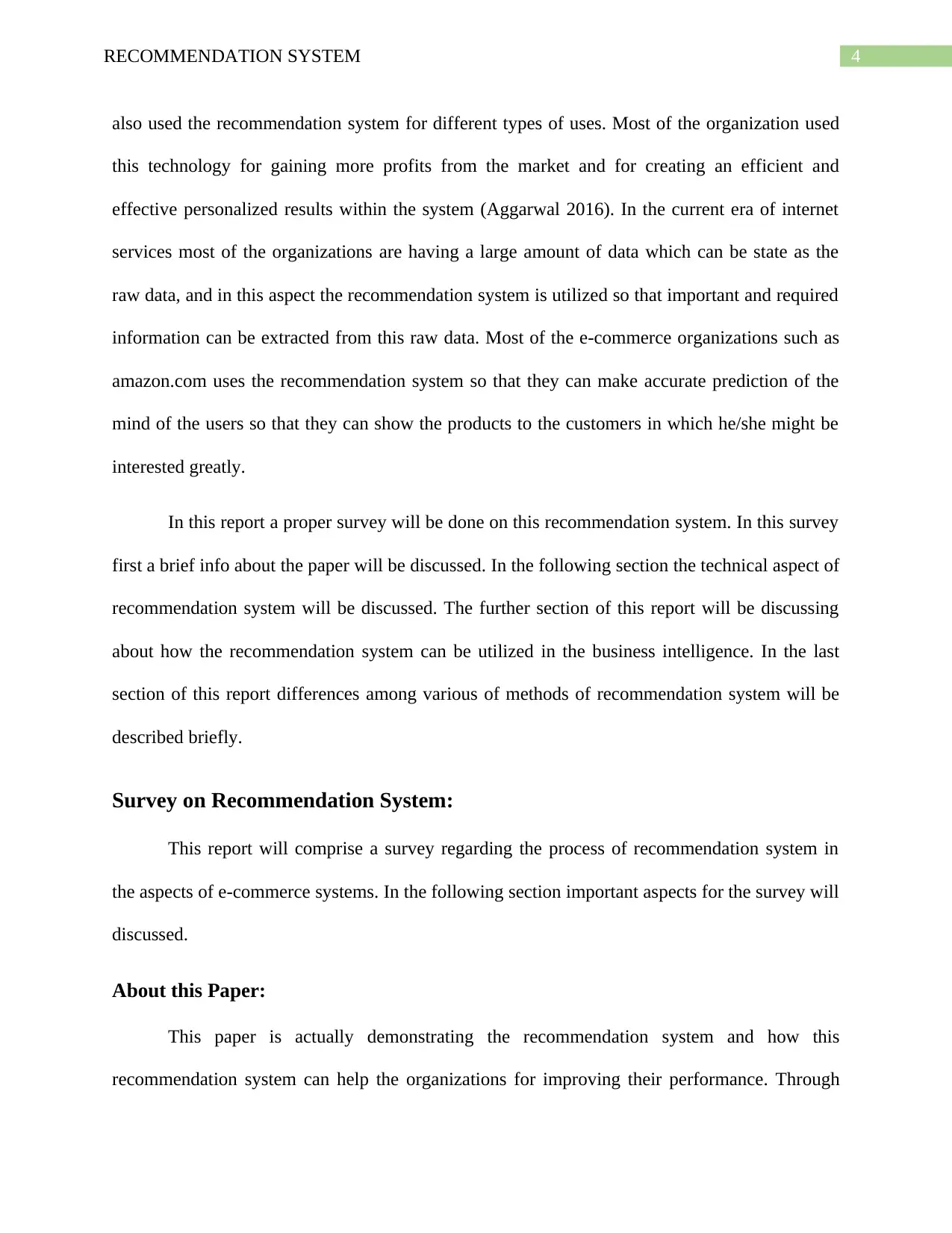
4RECOMMENDATION SYSTEM
also used the recommendation system for different types of uses. Most of the organization used
this technology for gaining more profits from the market and for creating an efficient and
effective personalized results within the system (Aggarwal 2016). In the current era of internet
services most of the organizations are having a large amount of data which can be state as the
raw data, and in this aspect the recommendation system is utilized so that important and required
information can be extracted from this raw data. Most of the e-commerce organizations such as
amazon.com uses the recommendation system so that they can make accurate prediction of the
mind of the users so that they can show the products to the customers in which he/she might be
interested greatly.
In this report a proper survey will be done on this recommendation system. In this survey
first a brief info about the paper will be discussed. In the following section the technical aspect of
recommendation system will be discussed. The further section of this report will be discussing
about how the recommendation system can be utilized in the business intelligence. In the last
section of this report differences among various of methods of recommendation system will be
described briefly.
Survey on Recommendation System:
This report will comprise a survey regarding the process of recommendation system in
the aspects of e-commerce systems. In the following section important aspects for the survey will
discussed.
About this Paper:
This paper is actually demonstrating the recommendation system and how this
recommendation system can help the organizations for improving their performance. Through
also used the recommendation system for different types of uses. Most of the organization used
this technology for gaining more profits from the market and for creating an efficient and
effective personalized results within the system (Aggarwal 2016). In the current era of internet
services most of the organizations are having a large amount of data which can be state as the
raw data, and in this aspect the recommendation system is utilized so that important and required
information can be extracted from this raw data. Most of the e-commerce organizations such as
amazon.com uses the recommendation system so that they can make accurate prediction of the
mind of the users so that they can show the products to the customers in which he/she might be
interested greatly.
In this report a proper survey will be done on this recommendation system. In this survey
first a brief info about the paper will be discussed. In the following section the technical aspect of
recommendation system will be discussed. The further section of this report will be discussing
about how the recommendation system can be utilized in the business intelligence. In the last
section of this report differences among various of methods of recommendation system will be
described briefly.
Survey on Recommendation System:
This report will comprise a survey regarding the process of recommendation system in
the aspects of e-commerce systems. In the following section important aspects for the survey will
discussed.
About this Paper:
This paper is actually demonstrating the recommendation system and how this
recommendation system can help the organizations for improving their performance. Through
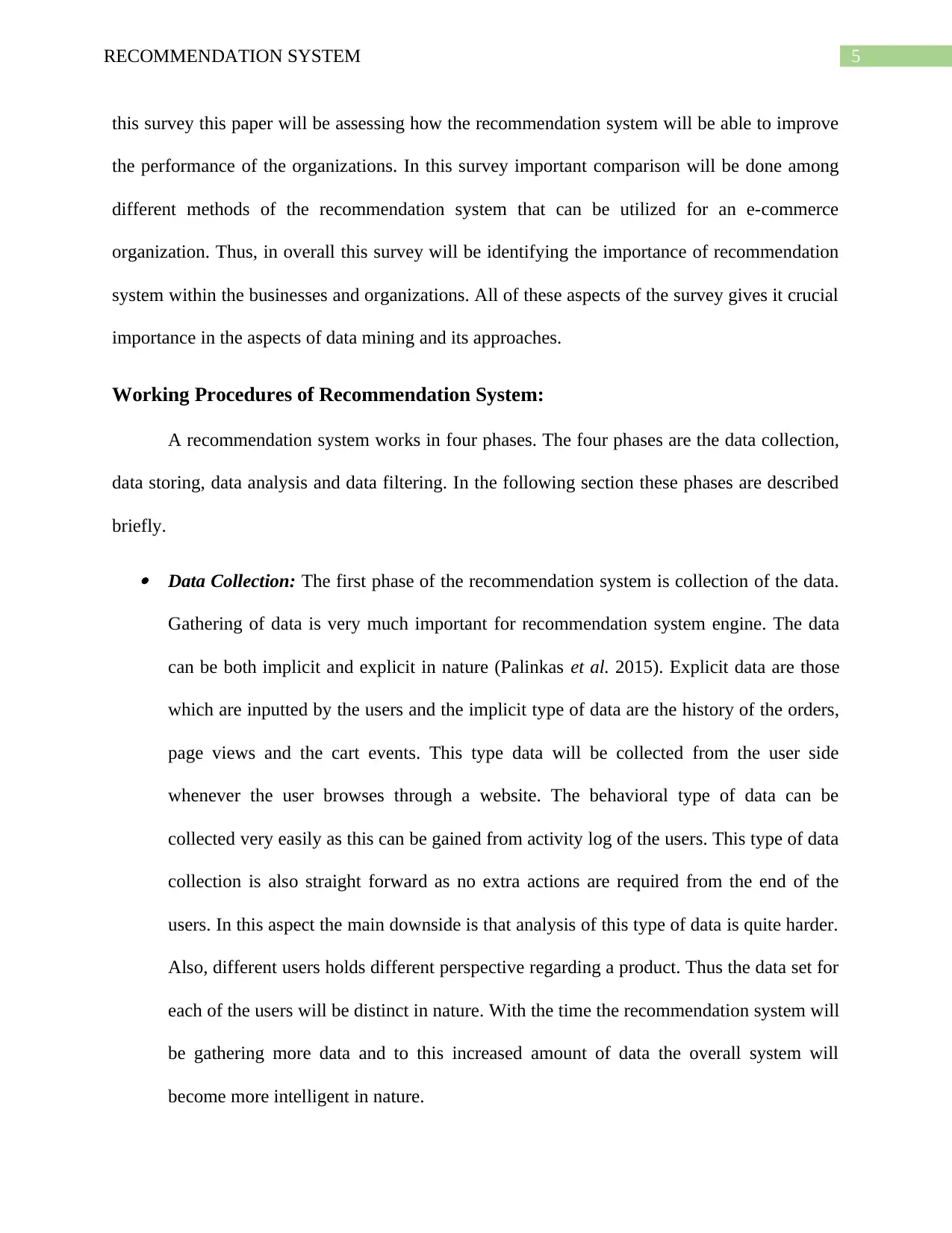
5RECOMMENDATION SYSTEM
this survey this paper will be assessing how the recommendation system will be able to improve
the performance of the organizations. In this survey important comparison will be done among
different methods of the recommendation system that can be utilized for an e-commerce
organization. Thus, in overall this survey will be identifying the importance of recommendation
system within the businesses and organizations. All of these aspects of the survey gives it crucial
importance in the aspects of data mining and its approaches.
Working Procedures of Recommendation System:
A recommendation system works in four phases. The four phases are the data collection,
data storing, data analysis and data filtering. In the following section these phases are described
briefly.
ï‚· Data Collection: The first phase of the recommendation system is collection of the data.
Gathering of data is very much important for recommendation system engine. The data
can be both implicit and explicit in nature (Palinkas et al. 2015). Explicit data are those
which are inputted by the users and the implicit type of data are the history of the orders,
page views and the cart events. This type data will be collected from the user side
whenever the user browses through a website. The behavioral type of data can be
collected very easily as this can be gained from activity log of the users. This type of data
collection is also straight forward as no extra actions are required from the end of the
users. In this aspect the main downside is that analysis of this type of data is quite harder.
Also, different users holds different perspective regarding a product. Thus the data set for
each of the users will be distinct in nature. With the time the recommendation system will
be gathering more data and to this increased amount of data the overall system will
become more intelligent in nature.
this survey this paper will be assessing how the recommendation system will be able to improve
the performance of the organizations. In this survey important comparison will be done among
different methods of the recommendation system that can be utilized for an e-commerce
organization. Thus, in overall this survey will be identifying the importance of recommendation
system within the businesses and organizations. All of these aspects of the survey gives it crucial
importance in the aspects of data mining and its approaches.
Working Procedures of Recommendation System:
A recommendation system works in four phases. The four phases are the data collection,
data storing, data analysis and data filtering. In the following section these phases are described
briefly.
ï‚· Data Collection: The first phase of the recommendation system is collection of the data.
Gathering of data is very much important for recommendation system engine. The data
can be both implicit and explicit in nature (Palinkas et al. 2015). Explicit data are those
which are inputted by the users and the implicit type of data are the history of the orders,
page views and the cart events. This type data will be collected from the user side
whenever the user browses through a website. The behavioral type of data can be
collected very easily as this can be gained from activity log of the users. This type of data
collection is also straight forward as no extra actions are required from the end of the
users. In this aspect the main downside is that analysis of this type of data is quite harder.
Also, different users holds different perspective regarding a product. Thus the data set for
each of the users will be distinct in nature. With the time the recommendation system will
be gathering more data and to this increased amount of data the overall system will
become more intelligent in nature.
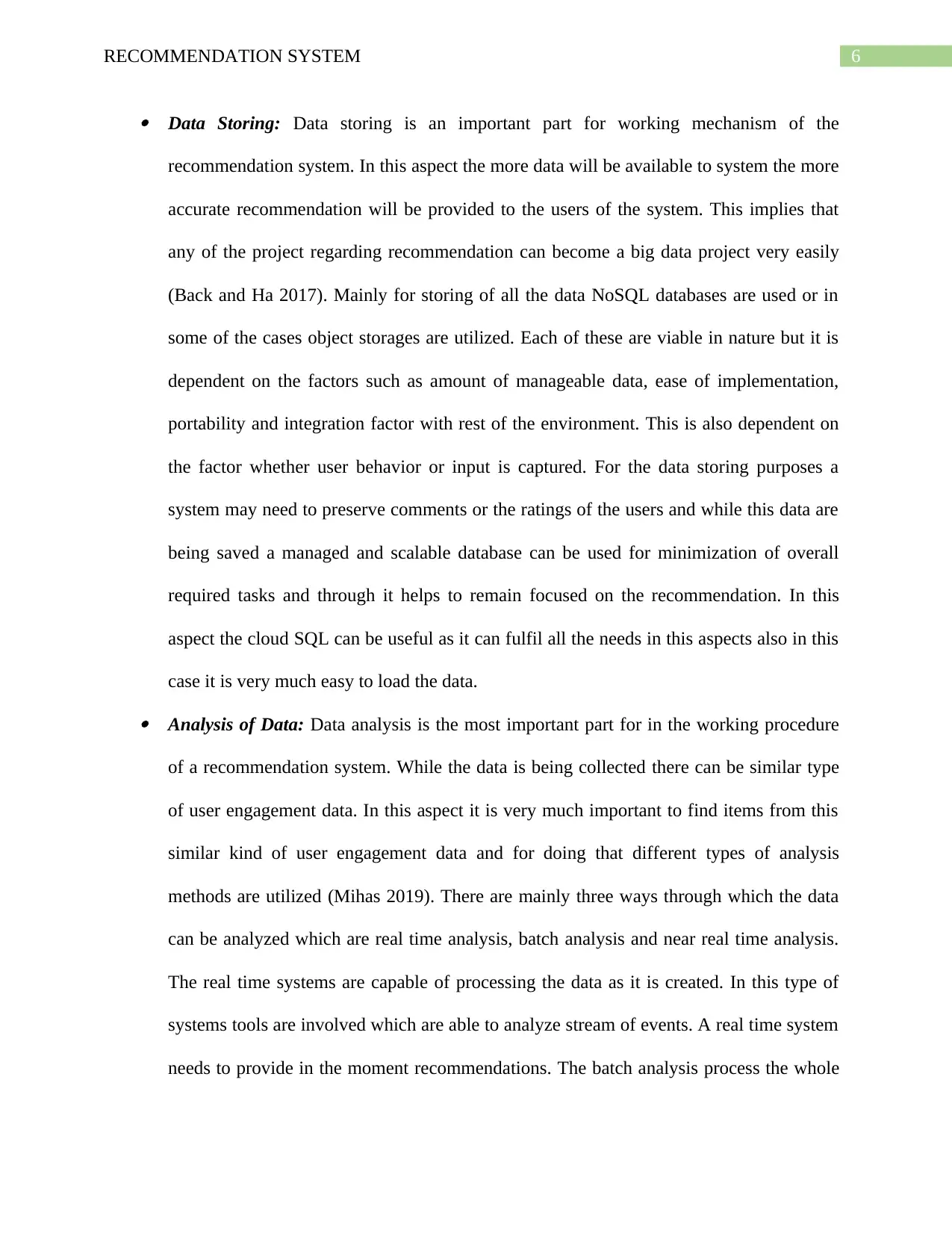
6RECOMMENDATION SYSTEM
ï‚· Data Storing: Data storing is an important part for working mechanism of the
recommendation system. In this aspect the more data will be available to system the more
accurate recommendation will be provided to the users of the system. This implies that
any of the project regarding recommendation can become a big data project very easily
(Back and Ha 2017). Mainly for storing of all the data NoSQL databases are used or in
some of the cases object storages are utilized. Each of these are viable in nature but it is
dependent on the factors such as amount of manageable data, ease of implementation,
portability and integration factor with rest of the environment. This is also dependent on
the factor whether user behavior or input is captured. For the data storing purposes a
system may need to preserve comments or the ratings of the users and while this data are
being saved a managed and scalable database can be used for minimization of overall
required tasks and through it helps to remain focused on the recommendation. In this
aspect the cloud SQL can be useful as it can fulfil all the needs in this aspects also in this
case it is very much easy to load the data.ï‚· Analysis of Data: Data analysis is the most important part for in the working procedure
of a recommendation system. While the data is being collected there can be similar type
of user engagement data. In this aspect it is very much important to find items from this
similar kind of user engagement data and for doing that different types of analysis
methods are utilized (Mihas 2019). There are mainly three ways through which the data
can be analyzed which are real time analysis, batch analysis and near real time analysis.
The real time systems are capable of processing the data as it is created. In this type of
systems tools are involved which are able to analyze stream of events. A real time system
needs to provide in the moment recommendations. The batch analysis process the whole
ï‚· Data Storing: Data storing is an important part for working mechanism of the
recommendation system. In this aspect the more data will be available to system the more
accurate recommendation will be provided to the users of the system. This implies that
any of the project regarding recommendation can become a big data project very easily
(Back and Ha 2017). Mainly for storing of all the data NoSQL databases are used or in
some of the cases object storages are utilized. Each of these are viable in nature but it is
dependent on the factors such as amount of manageable data, ease of implementation,
portability and integration factor with rest of the environment. This is also dependent on
the factor whether user behavior or input is captured. For the data storing purposes a
system may need to preserve comments or the ratings of the users and while this data are
being saved a managed and scalable database can be used for minimization of overall
required tasks and through it helps to remain focused on the recommendation. In this
aspect the cloud SQL can be useful as it can fulfil all the needs in this aspects also in this
case it is very much easy to load the data.ï‚· Analysis of Data: Data analysis is the most important part for in the working procedure
of a recommendation system. While the data is being collected there can be similar type
of user engagement data. In this aspect it is very much important to find items from this
similar kind of user engagement data and for doing that different types of analysis
methods are utilized (Mihas 2019). There are mainly three ways through which the data
can be analyzed which are real time analysis, batch analysis and near real time analysis.
The real time systems are capable of processing the data as it is created. In this type of
systems tools are involved which are able to analyze stream of events. A real time system
needs to provide in the moment recommendations. The batch analysis process the whole
Paraphrase This Document
Need a fresh take? Get an instant paraphrase of this document with our AI Paraphraser
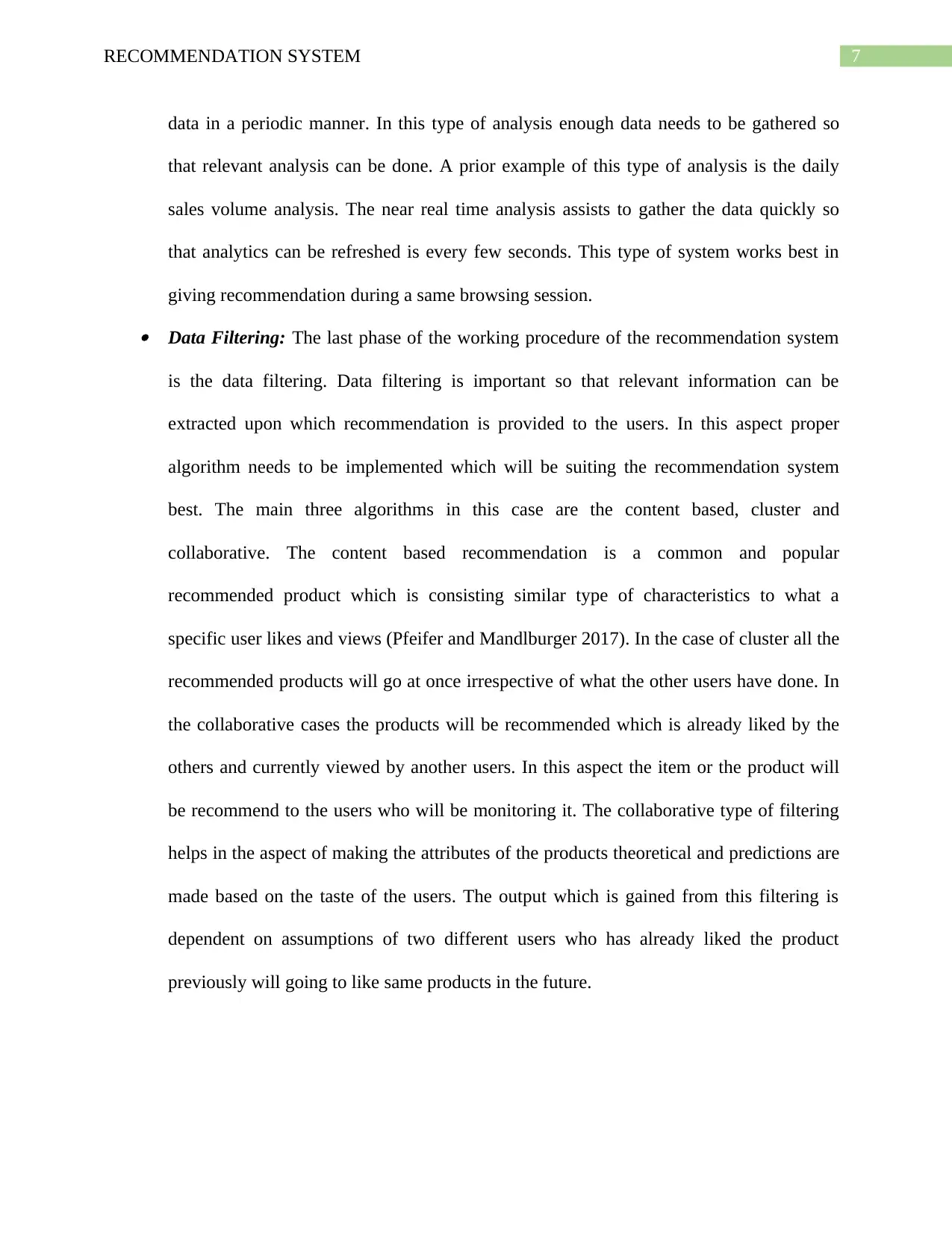
7RECOMMENDATION SYSTEM
data in a periodic manner. In this type of analysis enough data needs to be gathered so
that relevant analysis can be done. A prior example of this type of analysis is the daily
sales volume analysis. The near real time analysis assists to gather the data quickly so
that analytics can be refreshed is every few seconds. This type of system works best in
giving recommendation during a same browsing session.ï‚· Data Filtering: The last phase of the working procedure of the recommendation system
is the data filtering. Data filtering is important so that relevant information can be
extracted upon which recommendation is provided to the users. In this aspect proper
algorithm needs to be implemented which will be suiting the recommendation system
best. The main three algorithms in this case are the content based, cluster and
collaborative. The content based recommendation is a common and popular
recommended product which is consisting similar type of characteristics to what a
specific user likes and views (Pfeifer and Mandlburger 2017). In the case of cluster all the
recommended products will go at once irrespective of what the other users have done. In
the collaborative cases the products will be recommended which is already liked by the
others and currently viewed by another users. In this aspect the item or the product will
be recommend to the users who will be monitoring it. The collaborative type of filtering
helps in the aspect of making the attributes of the products theoretical and predictions are
made based on the taste of the users. The output which is gained from this filtering is
dependent on assumptions of two different users who has already liked the product
previously will going to like same products in the future.
data in a periodic manner. In this type of analysis enough data needs to be gathered so
that relevant analysis can be done. A prior example of this type of analysis is the daily
sales volume analysis. The near real time analysis assists to gather the data quickly so
that analytics can be refreshed is every few seconds. This type of system works best in
giving recommendation during a same browsing session.ï‚· Data Filtering: The last phase of the working procedure of the recommendation system
is the data filtering. Data filtering is important so that relevant information can be
extracted upon which recommendation is provided to the users. In this aspect proper
algorithm needs to be implemented which will be suiting the recommendation system
best. The main three algorithms in this case are the content based, cluster and
collaborative. The content based recommendation is a common and popular
recommended product which is consisting similar type of characteristics to what a
specific user likes and views (Pfeifer and Mandlburger 2017). In the case of cluster all the
recommended products will go at once irrespective of what the other users have done. In
the collaborative cases the products will be recommended which is already liked by the
others and currently viewed by another users. In this aspect the item or the product will
be recommend to the users who will be monitoring it. The collaborative type of filtering
helps in the aspect of making the attributes of the products theoretical and predictions are
made based on the taste of the users. The output which is gained from this filtering is
dependent on assumptions of two different users who has already liked the product
previously will going to like same products in the future.
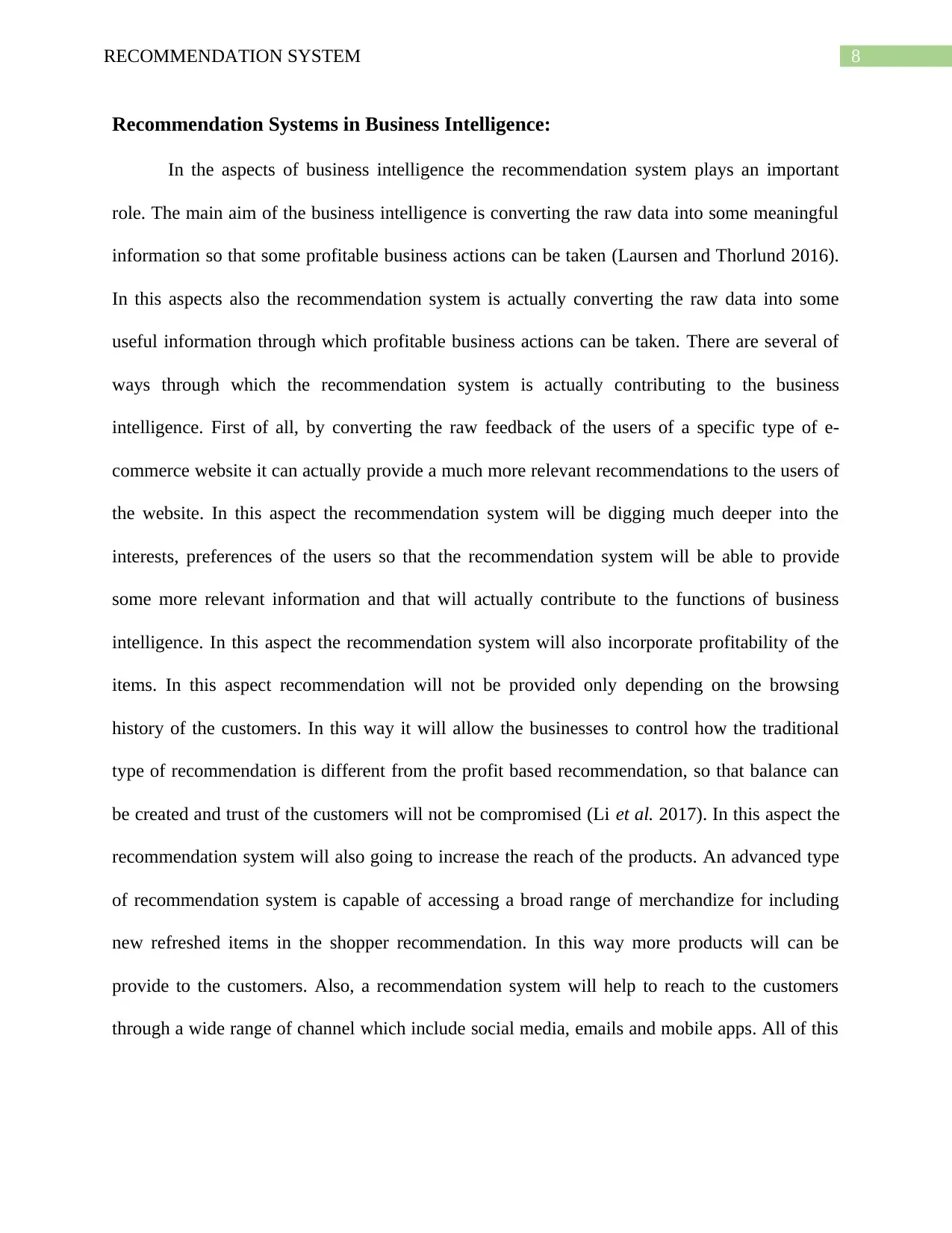
8RECOMMENDATION SYSTEM
Recommendation Systems in Business Intelligence:
In the aspects of business intelligence the recommendation system plays an important
role. The main aim of the business intelligence is converting the raw data into some meaningful
information so that some profitable business actions can be taken (Laursen and Thorlund 2016).
In this aspects also the recommendation system is actually converting the raw data into some
useful information through which profitable business actions can be taken. There are several of
ways through which the recommendation system is actually contributing to the business
intelligence. First of all, by converting the raw feedback of the users of a specific type of e-
commerce website it can actually provide a much more relevant recommendations to the users of
the website. In this aspect the recommendation system will be digging much deeper into the
interests, preferences of the users so that the recommendation system will be able to provide
some more relevant information and that will actually contribute to the functions of business
intelligence. In this aspect the recommendation system will also incorporate profitability of the
items. In this aspect recommendation will not be provided only depending on the browsing
history of the customers. In this way it will allow the businesses to control how the traditional
type of recommendation is different from the profit based recommendation, so that balance can
be created and trust of the customers will not be compromised (Li et al. 2017). In this aspect the
recommendation system will also going to increase the reach of the products. An advanced type
of recommendation system is capable of accessing a broad range of merchandize for including
new refreshed items in the shopper recommendation. In this way more products will can be
provide to the customers. Also, a recommendation system will help to reach to the customers
through a wide range of channel which include social media, emails and mobile apps. All of this
Recommendation Systems in Business Intelligence:
In the aspects of business intelligence the recommendation system plays an important
role. The main aim of the business intelligence is converting the raw data into some meaningful
information so that some profitable business actions can be taken (Laursen and Thorlund 2016).
In this aspects also the recommendation system is actually converting the raw data into some
useful information through which profitable business actions can be taken. There are several of
ways through which the recommendation system is actually contributing to the business
intelligence. First of all, by converting the raw feedback of the users of a specific type of e-
commerce website it can actually provide a much more relevant recommendations to the users of
the website. In this aspect the recommendation system will be digging much deeper into the
interests, preferences of the users so that the recommendation system will be able to provide
some more relevant information and that will actually contribute to the functions of business
intelligence. In this aspect the recommendation system will also incorporate profitability of the
items. In this aspect recommendation will not be provided only depending on the browsing
history of the customers. In this way it will allow the businesses to control how the traditional
type of recommendation is different from the profit based recommendation, so that balance can
be created and trust of the customers will not be compromised (Li et al. 2017). In this aspect the
recommendation system will also going to increase the reach of the products. An advanced type
of recommendation system is capable of accessing a broad range of merchandize for including
new refreshed items in the shopper recommendation. In this way more products will can be
provide to the customers. Also, a recommendation system will help to reach to the customers
through a wide range of channel which include social media, emails and mobile apps. All of this
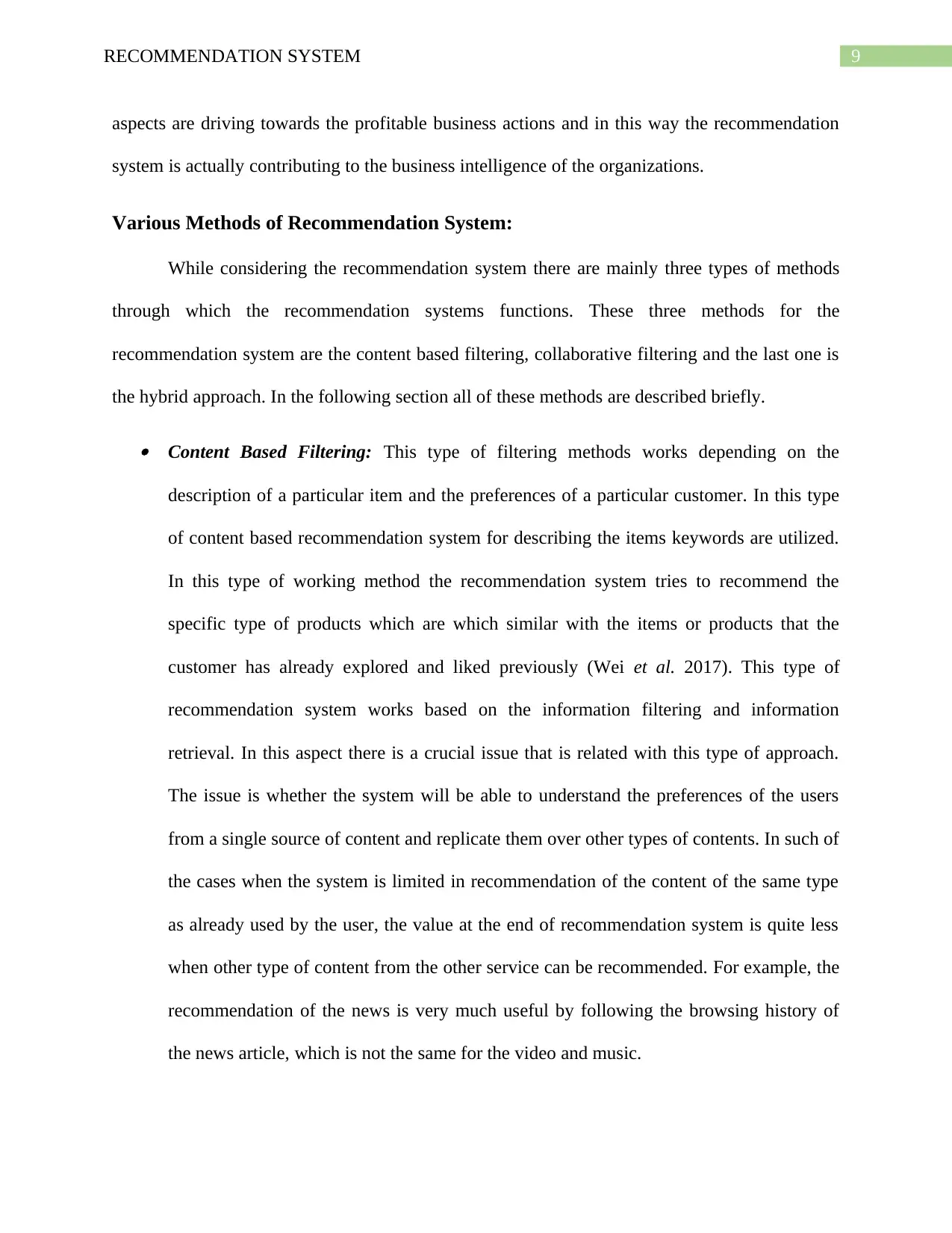
9RECOMMENDATION SYSTEM
aspects are driving towards the profitable business actions and in this way the recommendation
system is actually contributing to the business intelligence of the organizations.
Various Methods of Recommendation System:
While considering the recommendation system there are mainly three types of methods
through which the recommendation systems functions. These three methods for the
recommendation system are the content based filtering, collaborative filtering and the last one is
the hybrid approach. In the following section all of these methods are described briefly.
ï‚· Content Based Filtering: This type of filtering methods works depending on the
description of a particular item and the preferences of a particular customer. In this type
of content based recommendation system for describing the items keywords are utilized.
In this type of working method the recommendation system tries to recommend the
specific type of products which are which similar with the items or products that the
customer has already explored and liked previously (Wei et al. 2017). This type of
recommendation system works based on the information filtering and information
retrieval. In this aspect there is a crucial issue that is related with this type of approach.
The issue is whether the system will be able to understand the preferences of the users
from a single source of content and replicate them over other types of contents. In such of
the cases when the system is limited in recommendation of the content of the same type
as already used by the user, the value at the end of recommendation system is quite less
when other type of content from the other service can be recommended. For example, the
recommendation of the news is very much useful by following the browsing history of
the news article, which is not the same for the video and music.
aspects are driving towards the profitable business actions and in this way the recommendation
system is actually contributing to the business intelligence of the organizations.
Various Methods of Recommendation System:
While considering the recommendation system there are mainly three types of methods
through which the recommendation systems functions. These three methods for the
recommendation system are the content based filtering, collaborative filtering and the last one is
the hybrid approach. In the following section all of these methods are described briefly.
ï‚· Content Based Filtering: This type of filtering methods works depending on the
description of a particular item and the preferences of a particular customer. In this type
of content based recommendation system for describing the items keywords are utilized.
In this type of working method the recommendation system tries to recommend the
specific type of products which are which similar with the items or products that the
customer has already explored and liked previously (Wei et al. 2017). This type of
recommendation system works based on the information filtering and information
retrieval. In this aspect there is a crucial issue that is related with this type of approach.
The issue is whether the system will be able to understand the preferences of the users
from a single source of content and replicate them over other types of contents. In such of
the cases when the system is limited in recommendation of the content of the same type
as already used by the user, the value at the end of recommendation system is quite less
when other type of content from the other service can be recommended. For example, the
recommendation of the news is very much useful by following the browsing history of
the news article, which is not the same for the video and music.
Secure Best Marks with AI Grader
Need help grading? Try our AI Grader for instant feedback on your assignments.
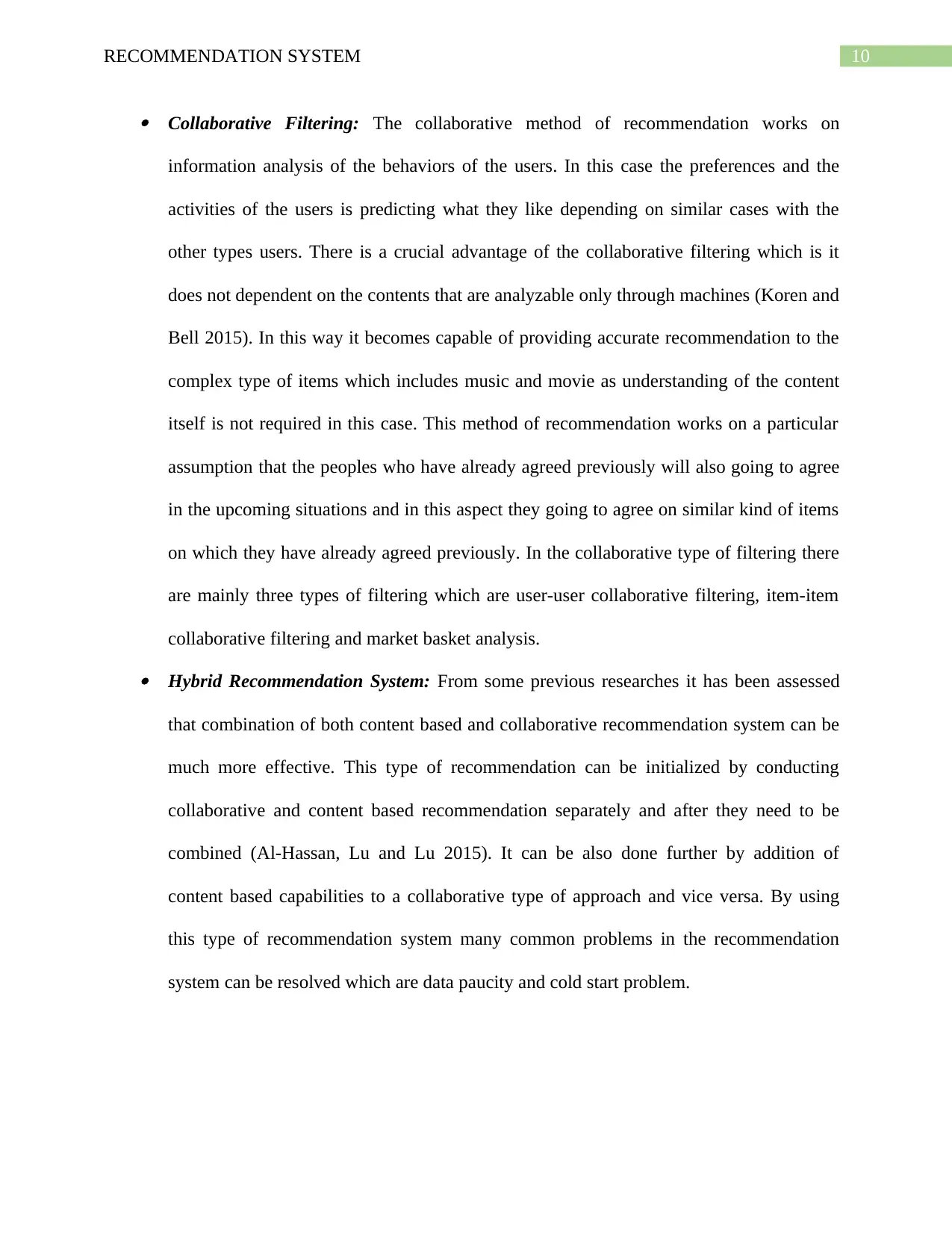
10RECOMMENDATION SYSTEM
ï‚· Collaborative Filtering: The collaborative method of recommendation works on
information analysis of the behaviors of the users. In this case the preferences and the
activities of the users is predicting what they like depending on similar cases with the
other types users. There is a crucial advantage of the collaborative filtering which is it
does not dependent on the contents that are analyzable only through machines (Koren and
Bell 2015). In this way it becomes capable of providing accurate recommendation to the
complex type of items which includes music and movie as understanding of the content
itself is not required in this case. This method of recommendation works on a particular
assumption that the peoples who have already agreed previously will also going to agree
in the upcoming situations and in this aspect they going to agree on similar kind of items
on which they have already agreed previously. In the collaborative type of filtering there
are mainly three types of filtering which are user-user collaborative filtering, item-item
collaborative filtering and market basket analysis.ï‚· Hybrid Recommendation System: From some previous researches it has been assessed
that combination of both content based and collaborative recommendation system can be
much more effective. This type of recommendation can be initialized by conducting
collaborative and content based recommendation separately and after they need to be
combined (Al-Hassan, Lu and Lu 2015). It can be also done further by addition of
content based capabilities to a collaborative type of approach and vice versa. By using
this type of recommendation system many common problems in the recommendation
system can be resolved which are data paucity and cold start problem.
ï‚· Collaborative Filtering: The collaborative method of recommendation works on
information analysis of the behaviors of the users. In this case the preferences and the
activities of the users is predicting what they like depending on similar cases with the
other types users. There is a crucial advantage of the collaborative filtering which is it
does not dependent on the contents that are analyzable only through machines (Koren and
Bell 2015). In this way it becomes capable of providing accurate recommendation to the
complex type of items which includes music and movie as understanding of the content
itself is not required in this case. This method of recommendation works on a particular
assumption that the peoples who have already agreed previously will also going to agree
in the upcoming situations and in this aspect they going to agree on similar kind of items
on which they have already agreed previously. In the collaborative type of filtering there
are mainly three types of filtering which are user-user collaborative filtering, item-item
collaborative filtering and market basket analysis.ï‚· Hybrid Recommendation System: From some previous researches it has been assessed
that combination of both content based and collaborative recommendation system can be
much more effective. This type of recommendation can be initialized by conducting
collaborative and content based recommendation separately and after they need to be
combined (Al-Hassan, Lu and Lu 2015). It can be also done further by addition of
content based capabilities to a collaborative type of approach and vice versa. By using
this type of recommendation system many common problems in the recommendation
system can be resolved which are data paucity and cold start problem.
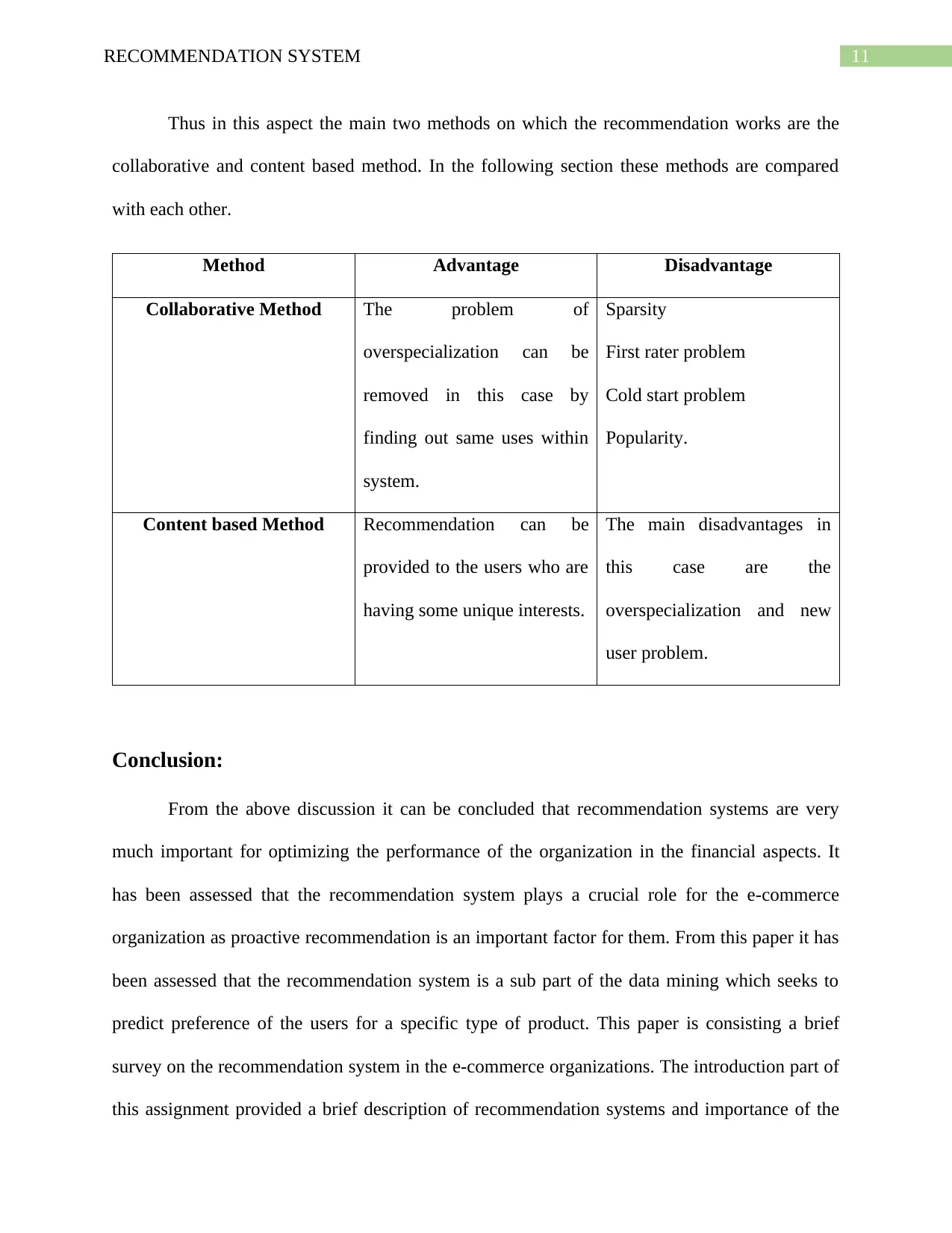
11RECOMMENDATION SYSTEM
Thus in this aspect the main two methods on which the recommendation works are the
collaborative and content based method. In the following section these methods are compared
with each other.
Method Advantage Disadvantage
Collaborative Method The problem of
overspecialization can be
removed in this case by
finding out same uses within
system.
Sparsity
First rater problem
Cold start problem
Popularity.
Content based Method Recommendation can be
provided to the users who are
having some unique interests.
The main disadvantages in
this case are the
overspecialization and new
user problem.
Conclusion:
From the above discussion it can be concluded that recommendation systems are very
much important for optimizing the performance of the organization in the financial aspects. It
has been assessed that the recommendation system plays a crucial role for the e-commerce
organization as proactive recommendation is an important factor for them. From this paper it has
been assessed that the recommendation system is a sub part of the data mining which seeks to
predict preference of the users for a specific type of product. This paper is consisting a brief
survey on the recommendation system in the e-commerce organizations. The introduction part of
this assignment provided a brief description of recommendation systems and importance of the
Thus in this aspect the main two methods on which the recommendation works are the
collaborative and content based method. In the following section these methods are compared
with each other.
Method Advantage Disadvantage
Collaborative Method The problem of
overspecialization can be
removed in this case by
finding out same uses within
system.
Sparsity
First rater problem
Cold start problem
Popularity.
Content based Method Recommendation can be
provided to the users who are
having some unique interests.
The main disadvantages in
this case are the
overspecialization and new
user problem.
Conclusion:
From the above discussion it can be concluded that recommendation systems are very
much important for optimizing the performance of the organization in the financial aspects. It
has been assessed that the recommendation system plays a crucial role for the e-commerce
organization as proactive recommendation is an important factor for them. From this paper it has
been assessed that the recommendation system is a sub part of the data mining which seeks to
predict preference of the users for a specific type of product. This paper is consisting a brief
survey on the recommendation system in the e-commerce organizations. The introduction part of
this assignment provided a brief description of recommendation systems and importance of the
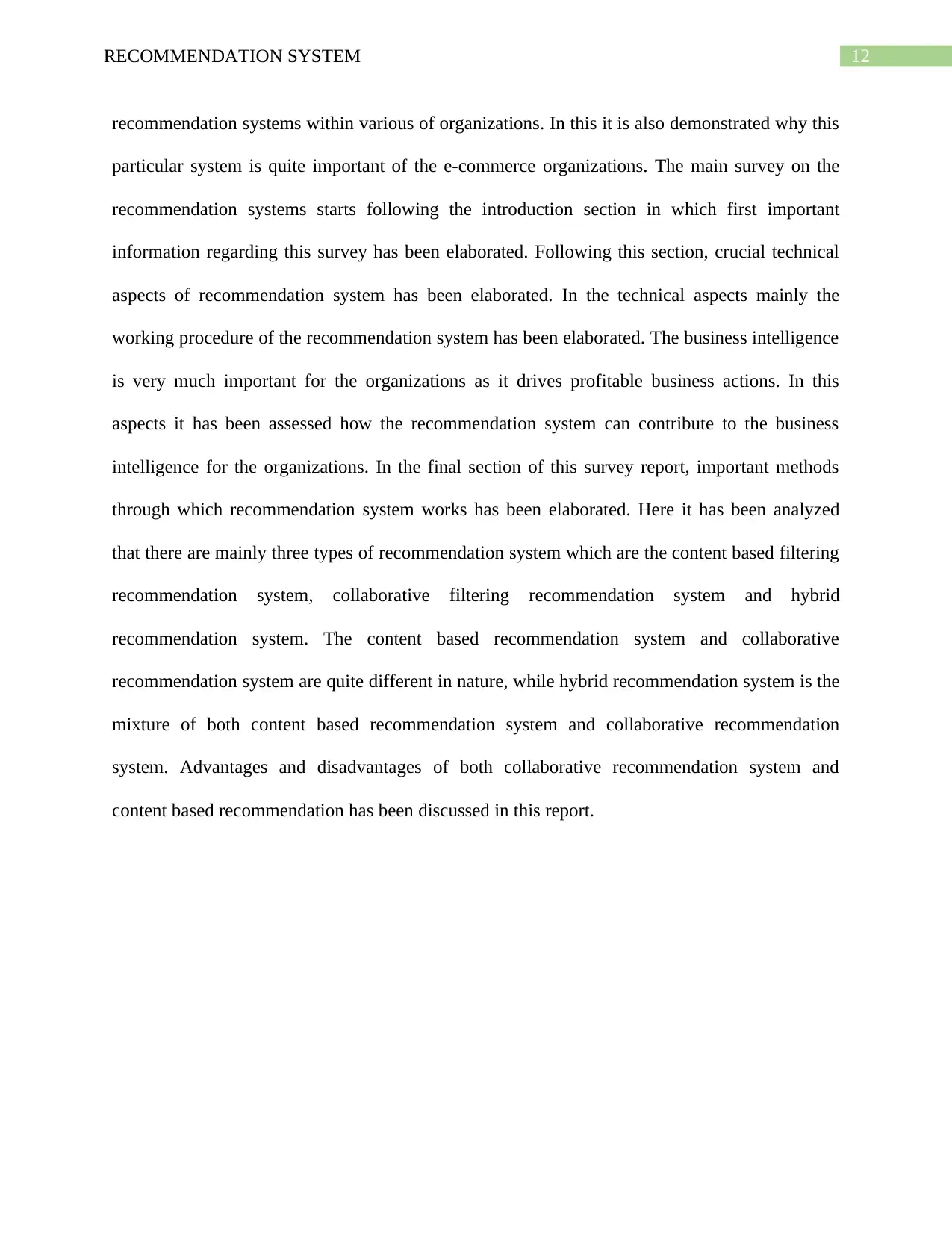
12RECOMMENDATION SYSTEM
recommendation systems within various of organizations. In this it is also demonstrated why this
particular system is quite important of the e-commerce organizations. The main survey on the
recommendation systems starts following the introduction section in which first important
information regarding this survey has been elaborated. Following this section, crucial technical
aspects of recommendation system has been elaborated. In the technical aspects mainly the
working procedure of the recommendation system has been elaborated. The business intelligence
is very much important for the organizations as it drives profitable business actions. In this
aspects it has been assessed how the recommendation system can contribute to the business
intelligence for the organizations. In the final section of this survey report, important methods
through which recommendation system works has been elaborated. Here it has been analyzed
that there are mainly three types of recommendation system which are the content based filtering
recommendation system, collaborative filtering recommendation system and hybrid
recommendation system. The content based recommendation system and collaborative
recommendation system are quite different in nature, while hybrid recommendation system is the
mixture of both content based recommendation system and collaborative recommendation
system. Advantages and disadvantages of both collaborative recommendation system and
content based recommendation has been discussed in this report.
recommendation systems within various of organizations. In this it is also demonstrated why this
particular system is quite important of the e-commerce organizations. The main survey on the
recommendation systems starts following the introduction section in which first important
information regarding this survey has been elaborated. Following this section, crucial technical
aspects of recommendation system has been elaborated. In the technical aspects mainly the
working procedure of the recommendation system has been elaborated. The business intelligence
is very much important for the organizations as it drives profitable business actions. In this
aspects it has been assessed how the recommendation system can contribute to the business
intelligence for the organizations. In the final section of this survey report, important methods
through which recommendation system works has been elaborated. Here it has been analyzed
that there are mainly three types of recommendation system which are the content based filtering
recommendation system, collaborative filtering recommendation system and hybrid
recommendation system. The content based recommendation system and collaborative
recommendation system are quite different in nature, while hybrid recommendation system is the
mixture of both content based recommendation system and collaborative recommendation
system. Advantages and disadvantages of both collaborative recommendation system and
content based recommendation has been discussed in this report.
Paraphrase This Document
Need a fresh take? Get an instant paraphrase of this document with our AI Paraphraser
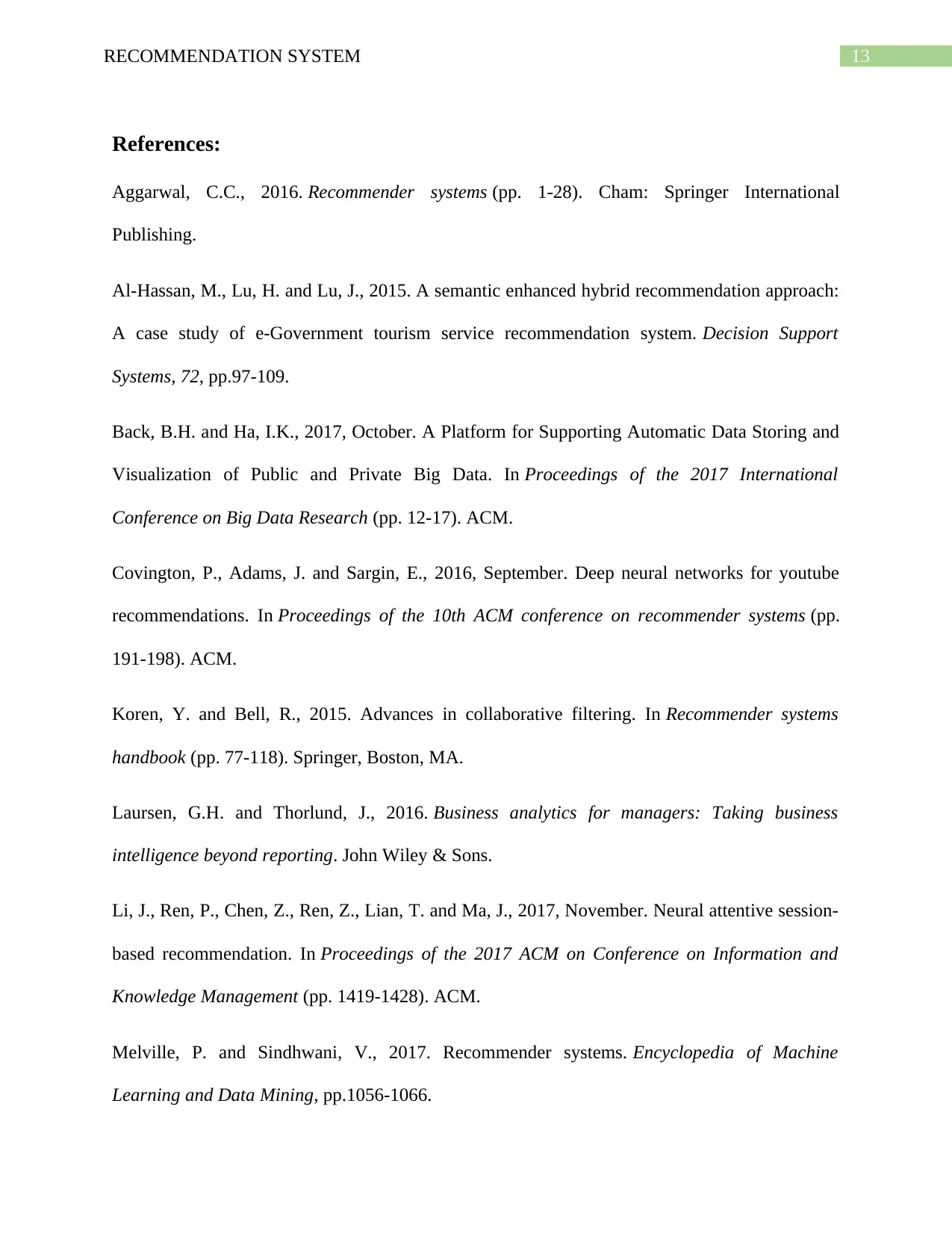
13RECOMMENDATION SYSTEM
References:
Aggarwal, C.C., 2016. Recommender systems (pp. 1-28). Cham: Springer International
Publishing.
Al-Hassan, M., Lu, H. and Lu, J., 2015. A semantic enhanced hybrid recommendation approach:
A case study of e-Government tourism service recommendation system. Decision Support
Systems, 72, pp.97-109.
Back, B.H. and Ha, I.K., 2017, October. A Platform for Supporting Automatic Data Storing and
Visualization of Public and Private Big Data. In Proceedings of the 2017 International
Conference on Big Data Research (pp. 12-17). ACM.
Covington, P., Adams, J. and Sargin, E., 2016, September. Deep neural networks for youtube
recommendations. In Proceedings of the 10th ACM conference on recommender systems (pp.
191-198). ACM.
Koren, Y. and Bell, R., 2015. Advances in collaborative filtering. In Recommender systems
handbook (pp. 77-118). Springer, Boston, MA.
Laursen, G.H. and Thorlund, J., 2016. Business analytics for managers: Taking business
intelligence beyond reporting. John Wiley & Sons.
Li, J., Ren, P., Chen, Z., Ren, Z., Lian, T. and Ma, J., 2017, November. Neural attentive session-
based recommendation. In Proceedings of the 2017 ACM on Conference on Information and
Knowledge Management (pp. 1419-1428). ACM.
Melville, P. and Sindhwani, V., 2017. Recommender systems. Encyclopedia of Machine
Learning and Data Mining, pp.1056-1066.
References:
Aggarwal, C.C., 2016. Recommender systems (pp. 1-28). Cham: Springer International
Publishing.
Al-Hassan, M., Lu, H. and Lu, J., 2015. A semantic enhanced hybrid recommendation approach:
A case study of e-Government tourism service recommendation system. Decision Support
Systems, 72, pp.97-109.
Back, B.H. and Ha, I.K., 2017, October. A Platform for Supporting Automatic Data Storing and
Visualization of Public and Private Big Data. In Proceedings of the 2017 International
Conference on Big Data Research (pp. 12-17). ACM.
Covington, P., Adams, J. and Sargin, E., 2016, September. Deep neural networks for youtube
recommendations. In Proceedings of the 10th ACM conference on recommender systems (pp.
191-198). ACM.
Koren, Y. and Bell, R., 2015. Advances in collaborative filtering. In Recommender systems
handbook (pp. 77-118). Springer, Boston, MA.
Laursen, G.H. and Thorlund, J., 2016. Business analytics for managers: Taking business
intelligence beyond reporting. John Wiley & Sons.
Li, J., Ren, P., Chen, Z., Ren, Z., Lian, T. and Ma, J., 2017, November. Neural attentive session-
based recommendation. In Proceedings of the 2017 ACM on Conference on Information and
Knowledge Management (pp. 1419-1428). ACM.
Melville, P. and Sindhwani, V., 2017. Recommender systems. Encyclopedia of Machine
Learning and Data Mining, pp.1056-1066.
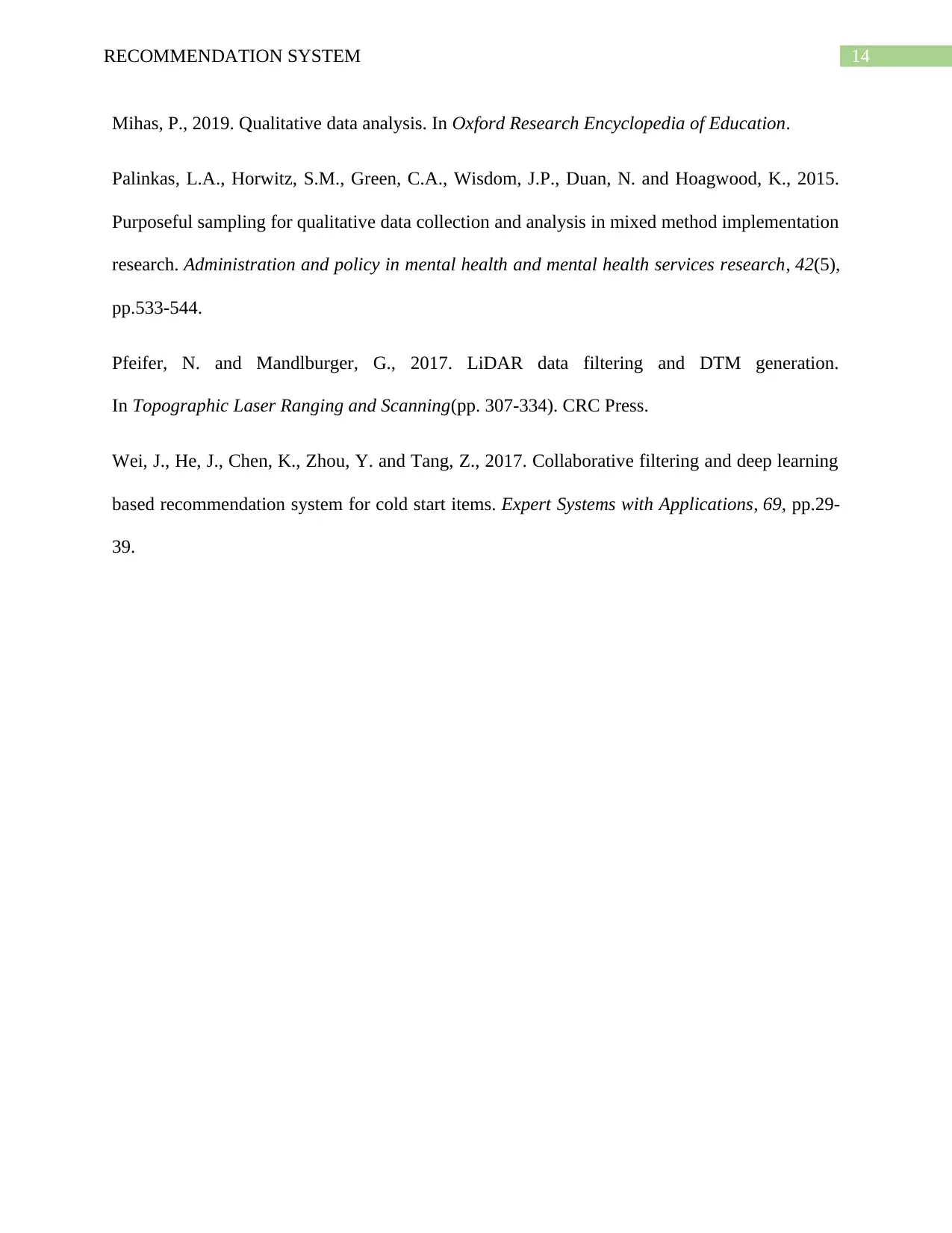
14RECOMMENDATION SYSTEM
Mihas, P., 2019. Qualitative data analysis. In Oxford Research Encyclopedia of Education.
Palinkas, L.A., Horwitz, S.M., Green, C.A., Wisdom, J.P., Duan, N. and Hoagwood, K., 2015.
Purposeful sampling for qualitative data collection and analysis in mixed method implementation
research. Administration and policy in mental health and mental health services research, 42(5),
pp.533-544.
Pfeifer, N. and Mandlburger, G., 2017. LiDAR data filtering and DTM generation.
In Topographic Laser Ranging and Scanning(pp. 307-334). CRC Press.
Wei, J., He, J., Chen, K., Zhou, Y. and Tang, Z., 2017. Collaborative filtering and deep learning
based recommendation system for cold start items. Expert Systems with Applications, 69, pp.29-
39.
Mihas, P., 2019. Qualitative data analysis. In Oxford Research Encyclopedia of Education.
Palinkas, L.A., Horwitz, S.M., Green, C.A., Wisdom, J.P., Duan, N. and Hoagwood, K., 2015.
Purposeful sampling for qualitative data collection and analysis in mixed method implementation
research. Administration and policy in mental health and mental health services research, 42(5),
pp.533-544.
Pfeifer, N. and Mandlburger, G., 2017. LiDAR data filtering and DTM generation.
In Topographic Laser Ranging and Scanning(pp. 307-334). CRC Press.
Wei, J., He, J., Chen, K., Zhou, Y. and Tang, Z., 2017. Collaborative filtering and deep learning
based recommendation system for cold start items. Expert Systems with Applications, 69, pp.29-
39.
1 out of 15
Related Documents
![[object Object]](/_next/image/?url=%2F_next%2Fstatic%2Fmedia%2Flogo.6d15ce61.png&w=640&q=75)
Your All-in-One AI-Powered Toolkit for Academic Success.
 +13062052269
info@desklib.com
Available 24*7 on WhatsApp / Email
Unlock your academic potential
© 2024  |  Zucol Services PVT LTD  |  All rights reserved.