Improving the Regression Model for ICU Hours
VerifiedAdded on 2019/10/01
|15
|3286
|326
Report
AI Summary
The analysis examined the relationship between ICU hours and length of stay for patients, including the effects of marital status. It found a significant difference in average length of stay between males and females. The regression equation showed that ICU hours are significantly related to length of stay, married status, and single status. The model explained 19.6% variation in ICU hours, which is considered a poor fit. The analysis also found that the assumption of normality of error terms and homogeneity of residuals were not satisfied. It was recommended that additional demographic variables, such as age, income, nationality, and family history, be included in the model to improve its fit.
Contribute Materials
Your contribution can guide someone’s learning journey. Share your
documents today.
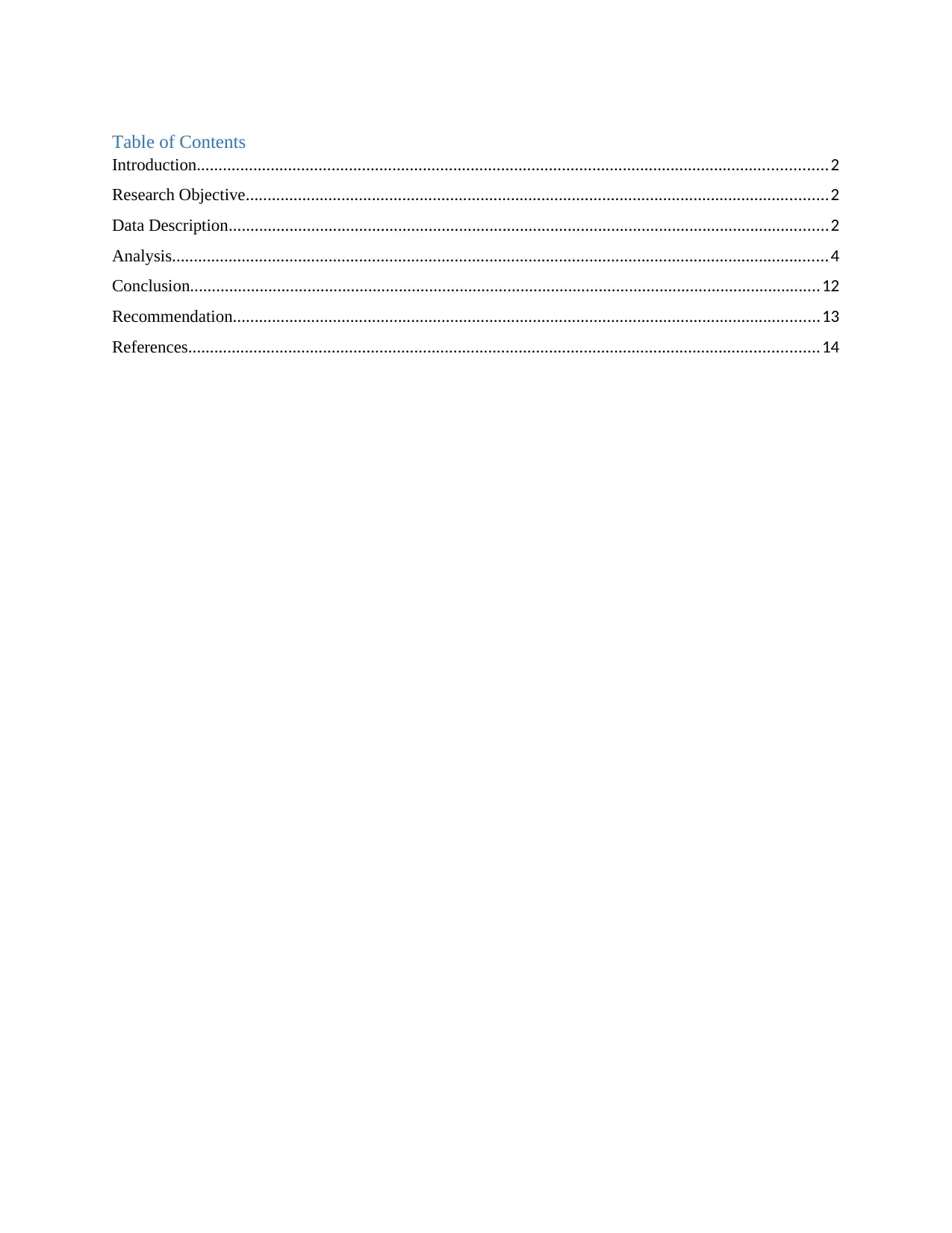
Table of Contents
Introduction.................................................................................................................................................2
Research Objective......................................................................................................................................2
Data Description..........................................................................................................................................2
Analysis.......................................................................................................................................................4
Conclusion.................................................................................................................................................12
Recommendation.......................................................................................................................................13
References.................................................................................................................................................14
Introduction.................................................................................................................................................2
Research Objective......................................................................................................................................2
Data Description..........................................................................................................................................2
Analysis.......................................................................................................................................................4
Conclusion.................................................................................................................................................12
Recommendation.......................................................................................................................................13
References.................................................................................................................................................14
Secure Best Marks with AI Grader
Need help grading? Try our AI Grader for instant feedback on your assignments.
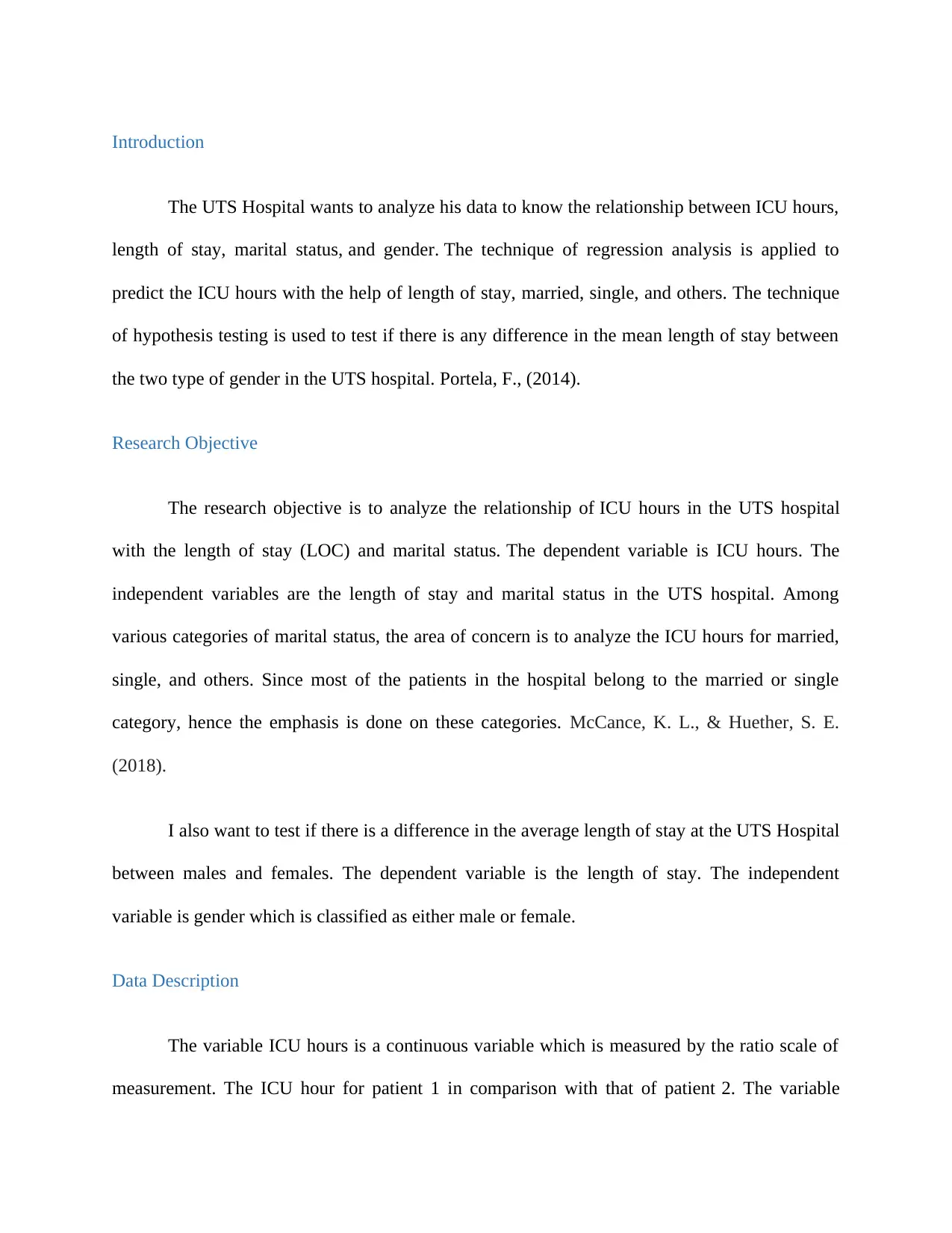
Introduction
The UTS Hospital wants to analyze his data to know the relationship between ICU hours,
length of stay, marital status, and gender. The technique of regression analysis is applied to
predict the ICU hours with the help of length of stay, married, single, and others. The technique
of hypothesis testing is used to test if there is any difference in the mean length of stay between
the two type of gender in the UTS hospital. Portela, F., (2014).
Research Objective
The research objective is to analyze the relationship of ICU hours in the UTS hospital
with the length of stay (LOC) and marital status. The dependent variable is ICU hours. The
independent variables are the length of stay and marital status in the UTS hospital. Among
various categories of marital status, the area of concern is to analyze the ICU hours for married,
single, and others. Since most of the patients in the hospital belong to the married or single
category, hence the emphasis is done on these categories. McCance, K. L., & Huether, S. E.
(2018).
I also want to test if there is a difference in the average length of stay at the UTS Hospital
between males and females. The dependent variable is the length of stay. The independent
variable is gender which is classified as either male or female.
Data Description
The variable ICU hours is a continuous variable which is measured by the ratio scale of
measurement. The ICU hour for patient 1 in comparison with that of patient 2. The variable
The UTS Hospital wants to analyze his data to know the relationship between ICU hours,
length of stay, marital status, and gender. The technique of regression analysis is applied to
predict the ICU hours with the help of length of stay, married, single, and others. The technique
of hypothesis testing is used to test if there is any difference in the mean length of stay between
the two type of gender in the UTS hospital. Portela, F., (2014).
Research Objective
The research objective is to analyze the relationship of ICU hours in the UTS hospital
with the length of stay (LOC) and marital status. The dependent variable is ICU hours. The
independent variables are the length of stay and marital status in the UTS hospital. Among
various categories of marital status, the area of concern is to analyze the ICU hours for married,
single, and others. Since most of the patients in the hospital belong to the married or single
category, hence the emphasis is done on these categories. McCance, K. L., & Huether, S. E.
(2018).
I also want to test if there is a difference in the average length of stay at the UTS Hospital
between males and females. The dependent variable is the length of stay. The independent
variable is gender which is classified as either male or female.
Data Description
The variable ICU hours is a continuous variable which is measured by the ratio scale of
measurement. The ICU hour for patient 1 in comparison with that of patient 2. The variable
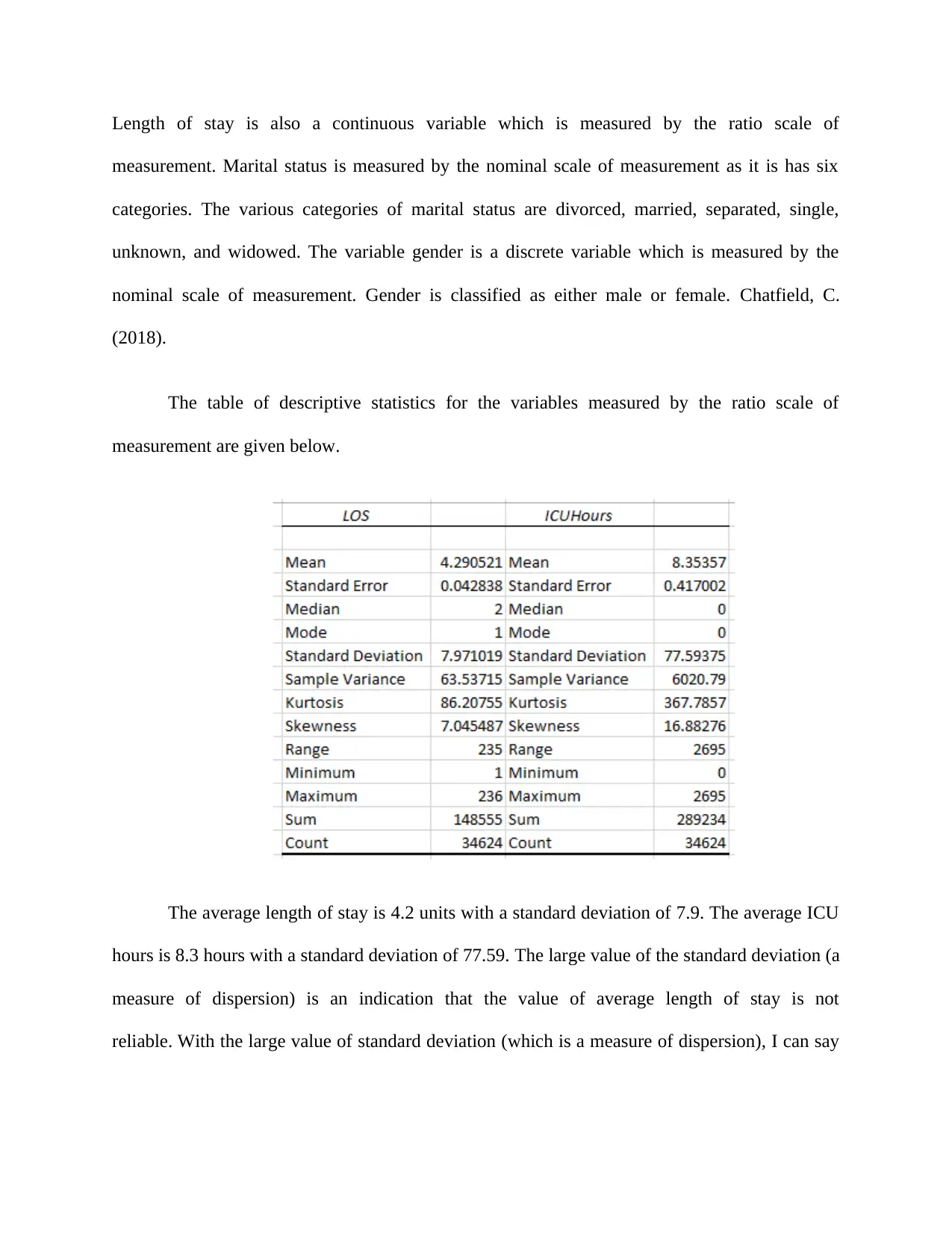
Length of stay is also a continuous variable which is measured by the ratio scale of
measurement. Marital status is measured by the nominal scale of measurement as it is has six
categories. The various categories of marital status are divorced, married, separated, single,
unknown, and widowed. The variable gender is a discrete variable which is measured by the
nominal scale of measurement. Gender is classified as either male or female. Chatfield, C.
(2018).
The table of descriptive statistics for the variables measured by the ratio scale of
measurement are given below.
The average length of stay is 4.2 units with a standard deviation of 7.9. The average ICU
hours is 8.3 hours with a standard deviation of 77.59. The large value of the standard deviation (a
measure of dispersion) is an indication that the value of average length of stay is not
reliable. With the large value of standard deviation (which is a measure of dispersion), I can say
measurement. Marital status is measured by the nominal scale of measurement as it is has six
categories. The various categories of marital status are divorced, married, separated, single,
unknown, and widowed. The variable gender is a discrete variable which is measured by the
nominal scale of measurement. Gender is classified as either male or female. Chatfield, C.
(2018).
The table of descriptive statistics for the variables measured by the ratio scale of
measurement are given below.
The average length of stay is 4.2 units with a standard deviation of 7.9. The average ICU
hours is 8.3 hours with a standard deviation of 77.59. The large value of the standard deviation (a
measure of dispersion) is an indication that the value of average length of stay is not
reliable. With the large value of standard deviation (which is a measure of dispersion), I can say
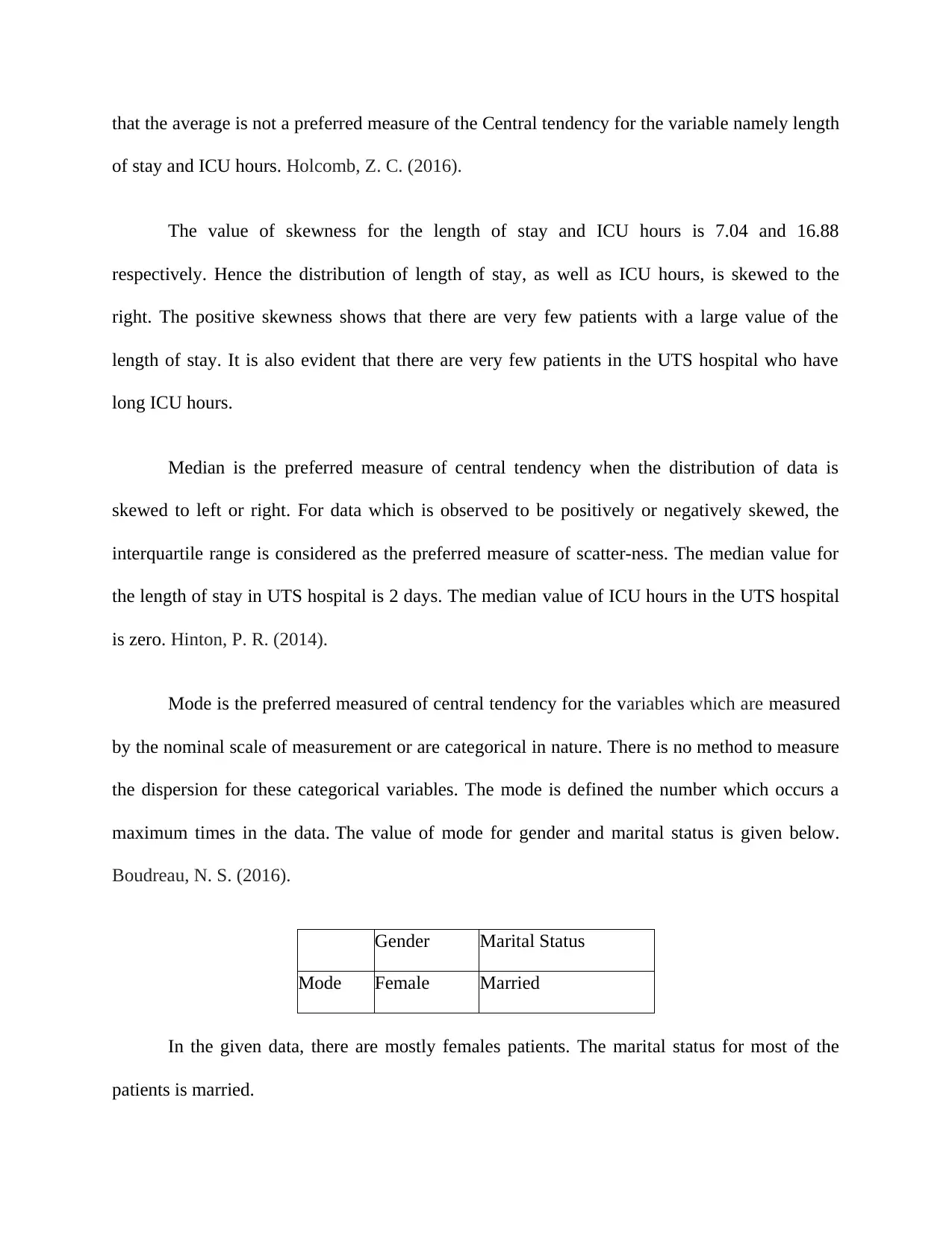
that the average is not a preferred measure of the Central tendency for the variable namely length
of stay and ICU hours. Holcomb, Z. C. (2016).
The value of skewness for the length of stay and ICU hours is 7.04 and 16.88
respectively. Hence the distribution of length of stay, as well as ICU hours, is skewed to the
right. The positive skewness shows that there are very few patients with a large value of the
length of stay. It is also evident that there are very few patients in the UTS hospital who have
long ICU hours.
Median is the preferred measure of central tendency when the distribution of data is
skewed to left or right. For data which is observed to be positively or negatively skewed, the
interquartile range is considered as the preferred measure of scatter-ness. The median value for
the length of stay in UTS hospital is 2 days. The median value of ICU hours in the UTS hospital
is zero. Hinton, P. R. (2014).
Mode is the preferred measured of central tendency for the variables which are measured
by the nominal scale of measurement or are categorical in nature. There is no method to measure
the dispersion for these categorical variables. The mode is defined the number which occurs a
maximum times in the data. The value of mode for gender and marital status is given below.
Boudreau, N. S. (2016).
Gender Marital Status
Mode Female Married
In the given data, there are mostly females patients. The marital status for most of the
patients is married.
of stay and ICU hours. Holcomb, Z. C. (2016).
The value of skewness for the length of stay and ICU hours is 7.04 and 16.88
respectively. Hence the distribution of length of stay, as well as ICU hours, is skewed to the
right. The positive skewness shows that there are very few patients with a large value of the
length of stay. It is also evident that there are very few patients in the UTS hospital who have
long ICU hours.
Median is the preferred measure of central tendency when the distribution of data is
skewed to left or right. For data which is observed to be positively or negatively skewed, the
interquartile range is considered as the preferred measure of scatter-ness. The median value for
the length of stay in UTS hospital is 2 days. The median value of ICU hours in the UTS hospital
is zero. Hinton, P. R. (2014).
Mode is the preferred measured of central tendency for the variables which are measured
by the nominal scale of measurement or are categorical in nature. There is no method to measure
the dispersion for these categorical variables. The mode is defined the number which occurs a
maximum times in the data. The value of mode for gender and marital status is given below.
Boudreau, N. S. (2016).
Gender Marital Status
Mode Female Married
In the given data, there are mostly females patients. The marital status for most of the
patients is married.
Paraphrase This Document
Need a fresh take? Get an instant paraphrase of this document with our AI Paraphraser
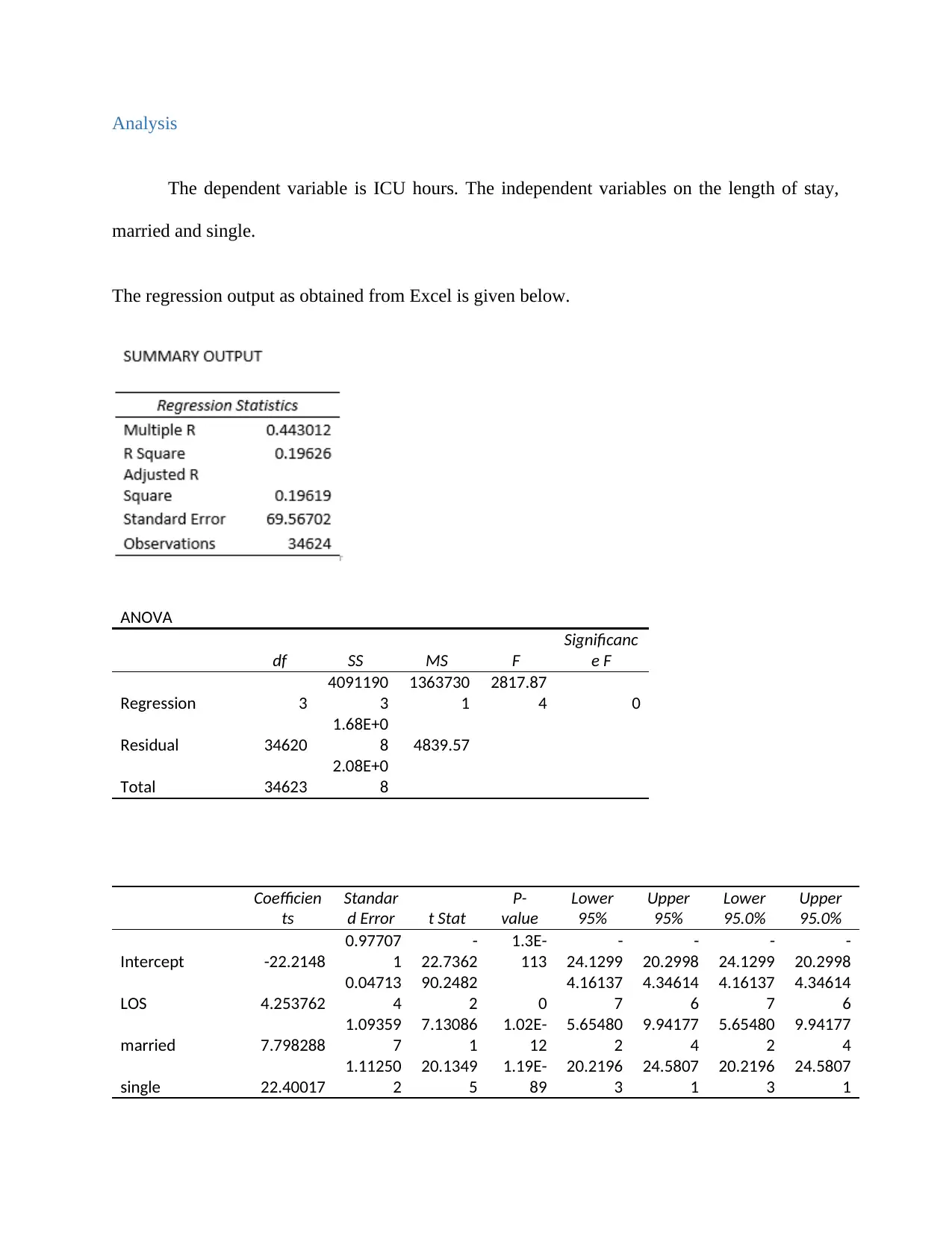
Analysis
The dependent variable is ICU hours. The independent variables on the length of stay,
married and single.
The regression output as obtained from Excel is given below.
ANOVA
df SS MS F
Significanc
e F
Regression 3
4091190
3
1363730
1
2817.87
4 0
Residual 34620
1.68E+0
8 4839.57
Total 34623
2.08E+0
8
Coefficien
ts
Standar
d Error t Stat
P-
value
Lower
95%
Upper
95%
Lower
95.0%
Upper
95.0%
Intercept -22.2148
0.97707
1
-
22.7362
1.3E-
113
-
24.1299
-
20.2998
-
24.1299
-
20.2998
LOS 4.253762
0.04713
4
90.2482
2 0
4.16137
7
4.34614
6
4.16137
7
4.34614
6
married 7.798288
1.09359
7
7.13086
1
1.02E-
12
5.65480
2
9.94177
4
5.65480
2
9.94177
4
single 22.40017
1.11250
2
20.1349
5
1.19E-
89
20.2196
3
24.5807
1
20.2196
3
24.5807
1
The dependent variable is ICU hours. The independent variables on the length of stay,
married and single.
The regression output as obtained from Excel is given below.
ANOVA
df SS MS F
Significanc
e F
Regression 3
4091190
3
1363730
1
2817.87
4 0
Residual 34620
1.68E+0
8 4839.57
Total 34623
2.08E+0
8
Coefficien
ts
Standar
d Error t Stat
P-
value
Lower
95%
Upper
95%
Lower
95.0%
Upper
95.0%
Intercept -22.2148
0.97707
1
-
22.7362
1.3E-
113
-
24.1299
-
20.2998
-
24.1299
-
20.2998
LOS 4.253762
0.04713
4
90.2482
2 0
4.16137
7
4.34614
6
4.16137
7
4.34614
6
married 7.798288
1.09359
7
7.13086
1
1.02E-
12
5.65480
2
9.94177
4
5.65480
2
9.94177
4
single 22.40017
1.11250
2
20.1349
5
1.19E-
89
20.2196
3
24.5807
1
20.2196
3
24.5807
1
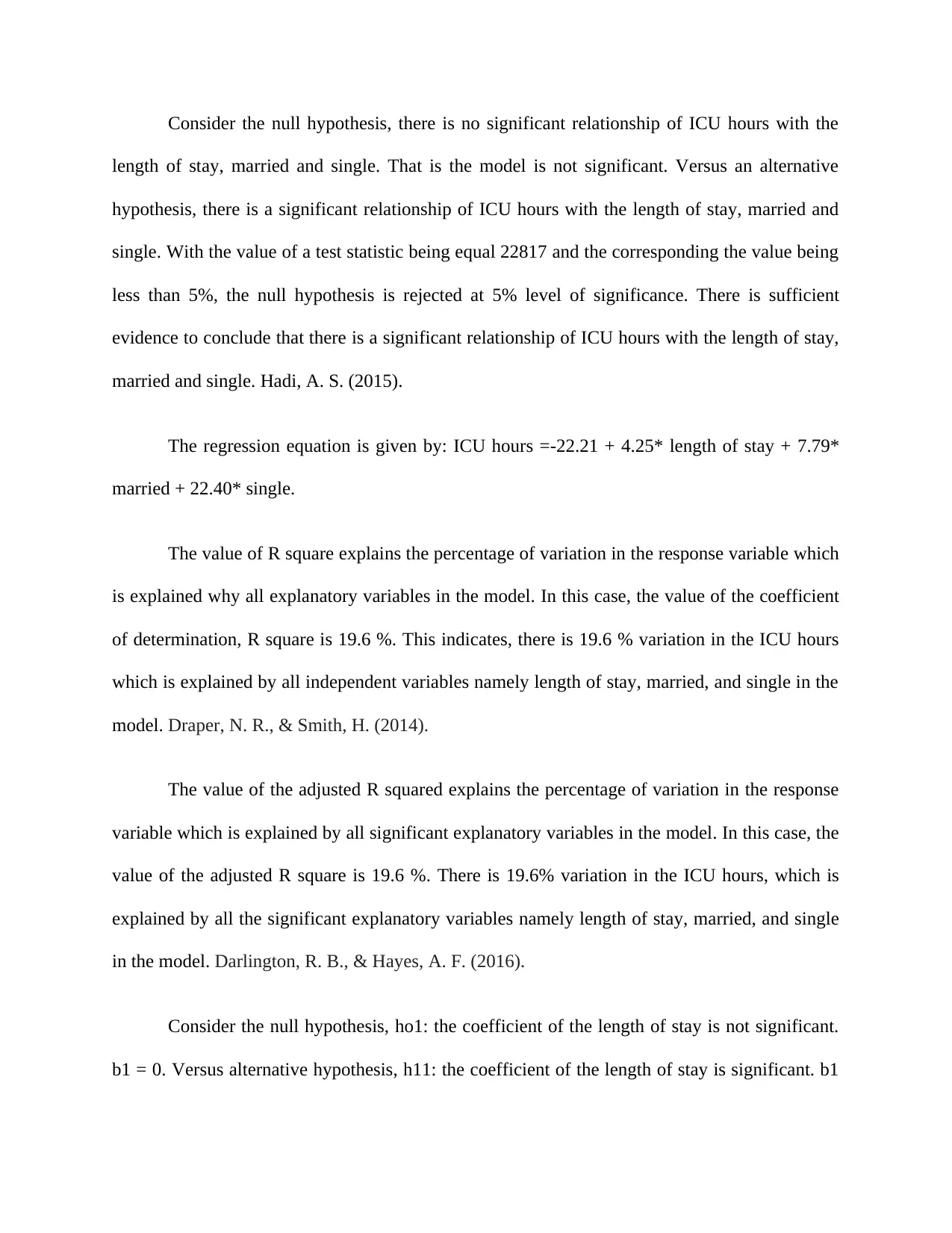
Consider the null hypothesis, there is no significant relationship of ICU hours with the
length of stay, married and single. That is the model is not significant. Versus an alternative
hypothesis, there is a significant relationship of ICU hours with the length of stay, married and
single. With the value of a test statistic being equal 22817 and the corresponding the value being
less than 5%, the null hypothesis is rejected at 5% level of significance. There is sufficient
evidence to conclude that there is a significant relationship of ICU hours with the length of stay,
married and single. Hadi, A. S. (2015).
The regression equation is given by: ICU hours =-22.21 + 4.25* length of stay + 7.79*
married + 22.40* single.
The value of R square explains the percentage of variation in the response variable which
is explained why all explanatory variables in the model. In this case, the value of the coefficient
of determination, R square is 19.6 %. This indicates, there is 19.6 % variation in the ICU hours
which is explained by all independent variables namely length of stay, married, and single in the
model. Draper, N. R., & Smith, H. (2014).
The value of the adjusted R squared explains the percentage of variation in the response
variable which is explained by all significant explanatory variables in the model. In this case, the
value of the adjusted R square is 19.6 %. There is 19.6% variation in the ICU hours, which is
explained by all the significant explanatory variables namely length of stay, married, and single
in the model. Darlington, R. B., & Hayes, A. F. (2016).
Consider the null hypothesis, ho1: the coefficient of the length of stay is not significant.
b1 = 0. Versus alternative hypothesis, h11: the coefficient of the length of stay is significant. b1
length of stay, married and single. That is the model is not significant. Versus an alternative
hypothesis, there is a significant relationship of ICU hours with the length of stay, married and
single. With the value of a test statistic being equal 22817 and the corresponding the value being
less than 5%, the null hypothesis is rejected at 5% level of significance. There is sufficient
evidence to conclude that there is a significant relationship of ICU hours with the length of stay,
married and single. Hadi, A. S. (2015).
The regression equation is given by: ICU hours =-22.21 + 4.25* length of stay + 7.79*
married + 22.40* single.
The value of R square explains the percentage of variation in the response variable which
is explained why all explanatory variables in the model. In this case, the value of the coefficient
of determination, R square is 19.6 %. This indicates, there is 19.6 % variation in the ICU hours
which is explained by all independent variables namely length of stay, married, and single in the
model. Draper, N. R., & Smith, H. (2014).
The value of the adjusted R squared explains the percentage of variation in the response
variable which is explained by all significant explanatory variables in the model. In this case, the
value of the adjusted R square is 19.6 %. There is 19.6% variation in the ICU hours, which is
explained by all the significant explanatory variables namely length of stay, married, and single
in the model. Darlington, R. B., & Hayes, A. F. (2016).
Consider the null hypothesis, ho1: the coefficient of the length of stay is not significant.
b1 = 0. Versus alternative hypothesis, h11: the coefficient of the length of stay is significant. b1
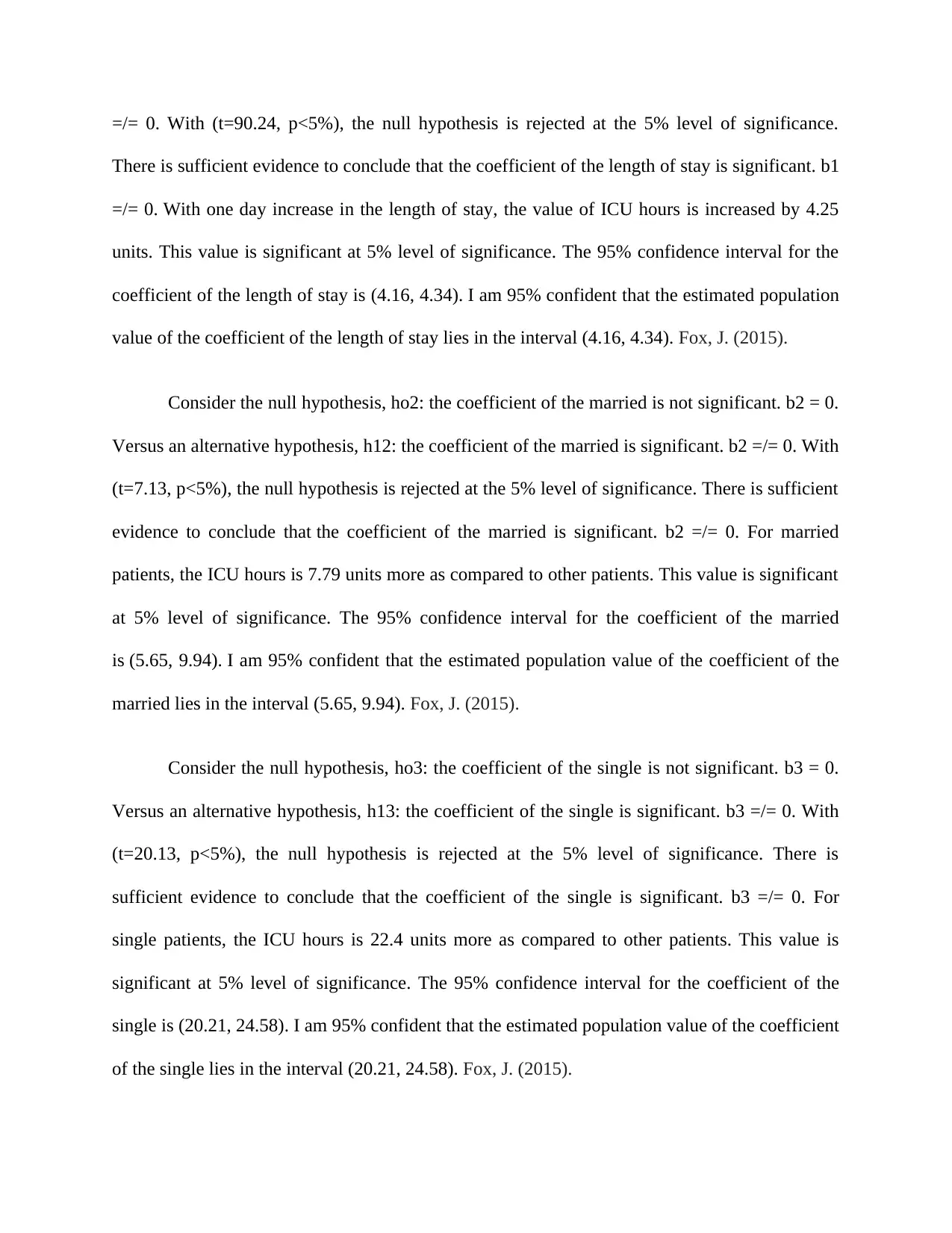
=/= 0. With (t=90.24, p<5%), the null hypothesis is rejected at the 5% level of significance.
There is sufficient evidence to conclude that the coefficient of the length of stay is significant. b1
=/= 0. With one day increase in the length of stay, the value of ICU hours is increased by 4.25
units. This value is significant at 5% level of significance. The 95% confidence interval for the
coefficient of the length of stay is (4.16, 4.34). I am 95% confident that the estimated population
value of the coefficient of the length of stay lies in the interval (4.16, 4.34). Fox, J. (2015).
Consider the null hypothesis, ho2: the coefficient of the married is not significant. b2 = 0.
Versus an alternative hypothesis, h12: the coefficient of the married is significant. b2 =/= 0. With
(t=7.13, p<5%), the null hypothesis is rejected at the 5% level of significance. There is sufficient
evidence to conclude that the coefficient of the married is significant. b2 =/= 0. For married
patients, the ICU hours is 7.79 units more as compared to other patients. This value is significant
at 5% level of significance. The 95% confidence interval for the coefficient of the married
is (5.65, 9.94). I am 95% confident that the estimated population value of the coefficient of the
married lies in the interval (5.65, 9.94). Fox, J. (2015).
Consider the null hypothesis, ho3: the coefficient of the single is not significant. b3 = 0.
Versus an alternative hypothesis, h13: the coefficient of the single is significant. b3 =/= 0. With
(t=20.13, p<5%), the null hypothesis is rejected at the 5% level of significance. There is
sufficient evidence to conclude that the coefficient of the single is significant. b3 =/= 0. For
single patients, the ICU hours is 22.4 units more as compared to other patients. This value is
significant at 5% level of significance. The 95% confidence interval for the coefficient of the
single is (20.21, 24.58). I am 95% confident that the estimated population value of the coefficient
of the single lies in the interval (20.21, 24.58). Fox, J. (2015).
There is sufficient evidence to conclude that the coefficient of the length of stay is significant. b1
=/= 0. With one day increase in the length of stay, the value of ICU hours is increased by 4.25
units. This value is significant at 5% level of significance. The 95% confidence interval for the
coefficient of the length of stay is (4.16, 4.34). I am 95% confident that the estimated population
value of the coefficient of the length of stay lies in the interval (4.16, 4.34). Fox, J. (2015).
Consider the null hypothesis, ho2: the coefficient of the married is not significant. b2 = 0.
Versus an alternative hypothesis, h12: the coefficient of the married is significant. b2 =/= 0. With
(t=7.13, p<5%), the null hypothesis is rejected at the 5% level of significance. There is sufficient
evidence to conclude that the coefficient of the married is significant. b2 =/= 0. For married
patients, the ICU hours is 7.79 units more as compared to other patients. This value is significant
at 5% level of significance. The 95% confidence interval for the coefficient of the married
is (5.65, 9.94). I am 95% confident that the estimated population value of the coefficient of the
married lies in the interval (5.65, 9.94). Fox, J. (2015).
Consider the null hypothesis, ho3: the coefficient of the single is not significant. b3 = 0.
Versus an alternative hypothesis, h13: the coefficient of the single is significant. b3 =/= 0. With
(t=20.13, p<5%), the null hypothesis is rejected at the 5% level of significance. There is
sufficient evidence to conclude that the coefficient of the single is significant. b3 =/= 0. For
single patients, the ICU hours is 22.4 units more as compared to other patients. This value is
significant at 5% level of significance. The 95% confidence interval for the coefficient of the
single is (20.21, 24.58). I am 95% confident that the estimated population value of the coefficient
of the single lies in the interval (20.21, 24.58). Fox, J. (2015).
Secure Best Marks with AI Grader
Need help grading? Try our AI Grader for instant feedback on your assignments.
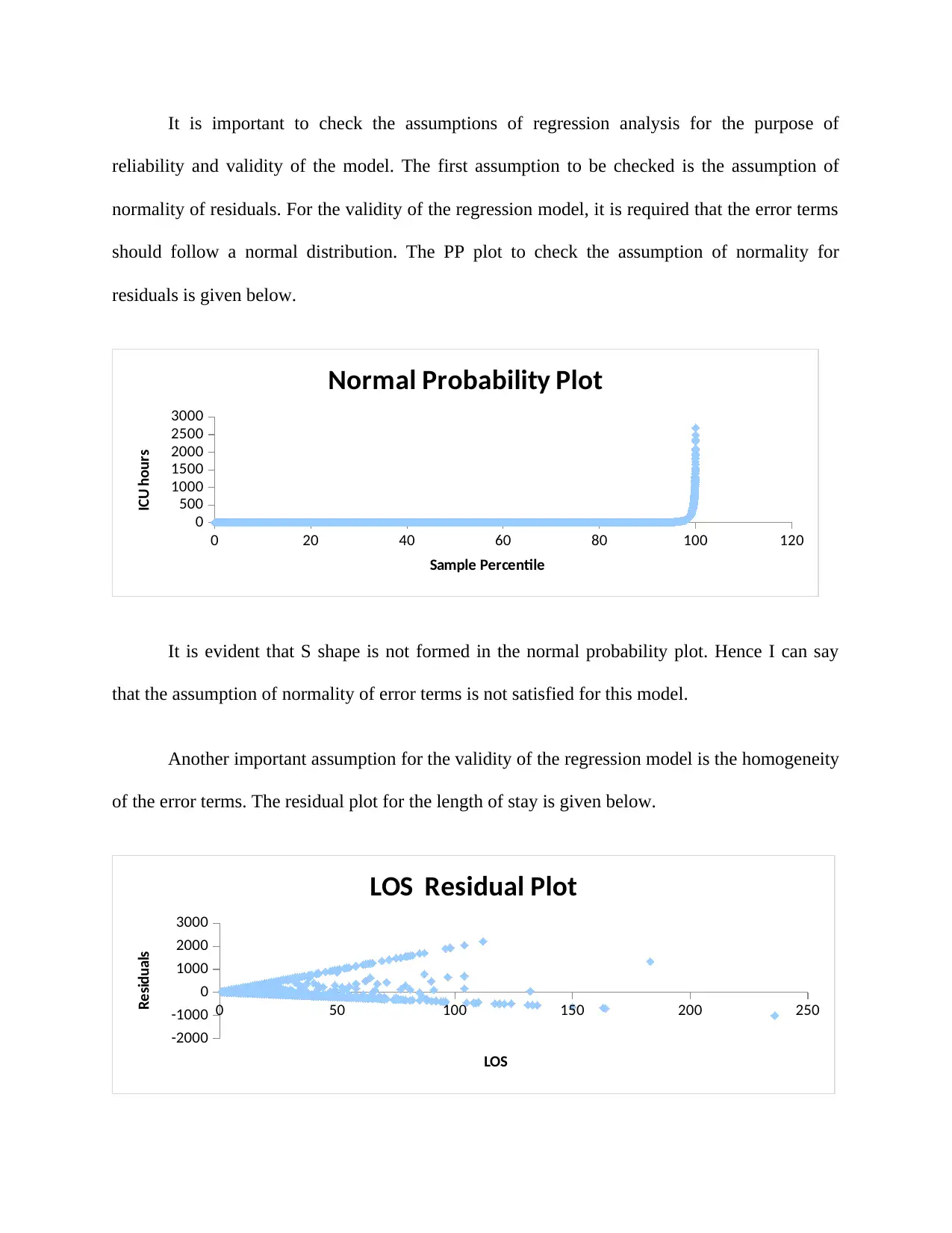
It is important to check the assumptions of regression analysis for the purpose of
reliability and validity of the model. The first assumption to be checked is the assumption of
normality of residuals. For the validity of the regression model, it is required that the error terms
should follow a normal distribution. The PP plot to check the assumption of normality for
residuals is given below.
0 20 40 60 80 100 120
0
500
1000
1500
2000
2500
3000
Normal Probability Plot
Sample Percentile
ICU hours
It is evident that S shape is not formed in the normal probability plot. Hence I can say
that the assumption of normality of error terms is not satisfied for this model.
Another important assumption for the validity of the regression model is the homogeneity
of the error terms. The residual plot for the length of stay is given below.
0 50 100 150 200 250
-2000
-1000
0
1000
2000
3000
LOS Residual Plot
LOS
Residuals
reliability and validity of the model. The first assumption to be checked is the assumption of
normality of residuals. For the validity of the regression model, it is required that the error terms
should follow a normal distribution. The PP plot to check the assumption of normality for
residuals is given below.
0 20 40 60 80 100 120
0
500
1000
1500
2000
2500
3000
Normal Probability Plot
Sample Percentile
ICU hours
It is evident that S shape is not formed in the normal probability plot. Hence I can say
that the assumption of normality of error terms is not satisfied for this model.
Another important assumption for the validity of the regression model is the homogeneity
of the error terms. The residual plot for the length of stay is given below.
0 50 100 150 200 250
-2000
-1000
0
1000
2000
3000
LOS Residual Plot
LOS
Residuals
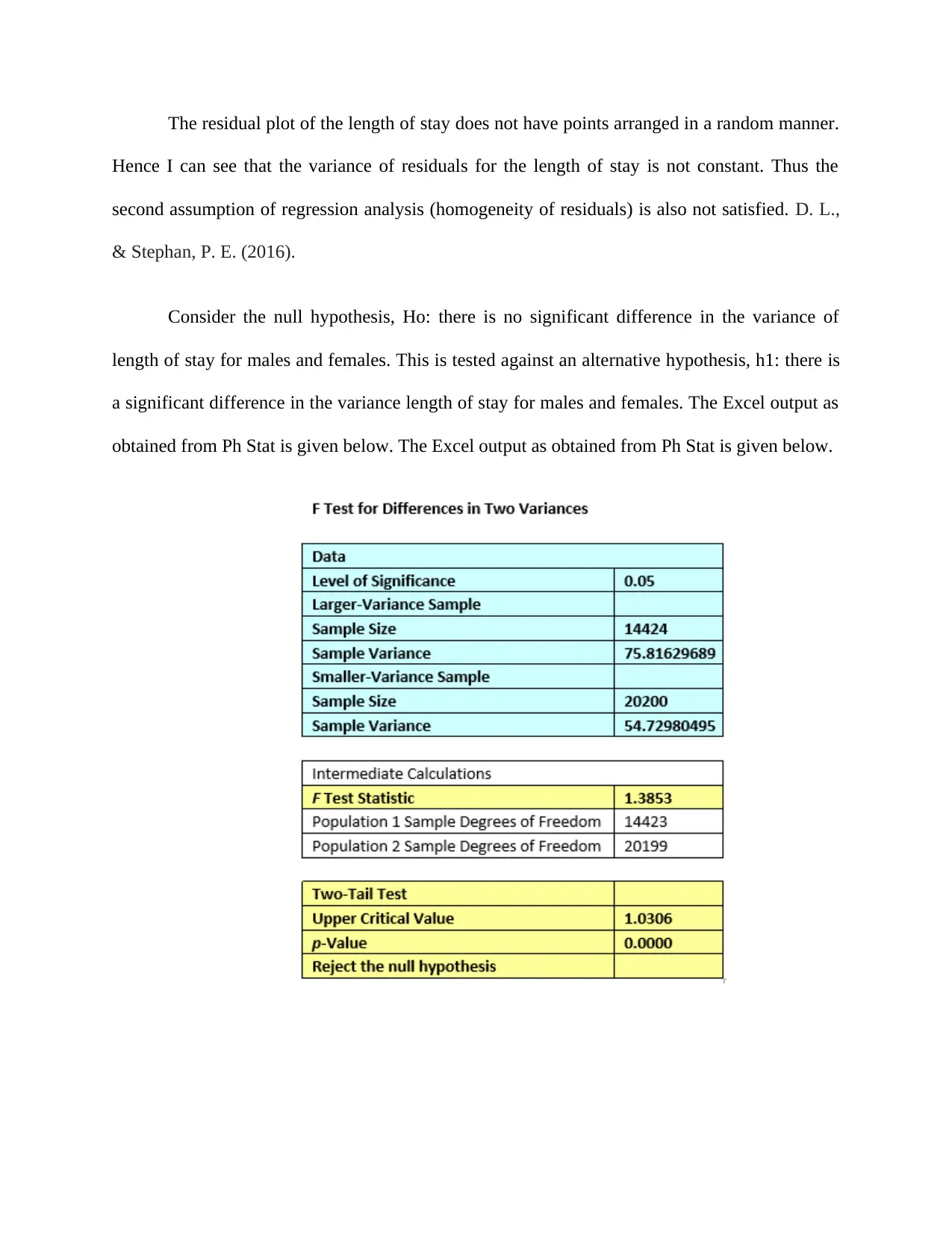
The residual plot of the length of stay does not have points arranged in a random manner.
Hence I can see that the variance of residuals for the length of stay is not constant. Thus the
second assumption of regression analysis (homogeneity of residuals) is also not satisfied. D. L.,
& Stephan, P. E. (2016).
Consider the null hypothesis, Ho: there is no significant difference in the variance of
length of stay for males and females. This is tested against an alternative hypothesis, h1: there is
a significant difference in the variance length of stay for males and females. The Excel output as
obtained from Ph Stat is given below. The Excel output as obtained from Ph Stat is given below.
Hence I can see that the variance of residuals for the length of stay is not constant. Thus the
second assumption of regression analysis (homogeneity of residuals) is also not satisfied. D. L.,
& Stephan, P. E. (2016).
Consider the null hypothesis, Ho: there is no significant difference in the variance of
length of stay for males and females. This is tested against an alternative hypothesis, h1: there is
a significant difference in the variance length of stay for males and females. The Excel output as
obtained from Ph Stat is given below. The Excel output as obtained from Ph Stat is given below.
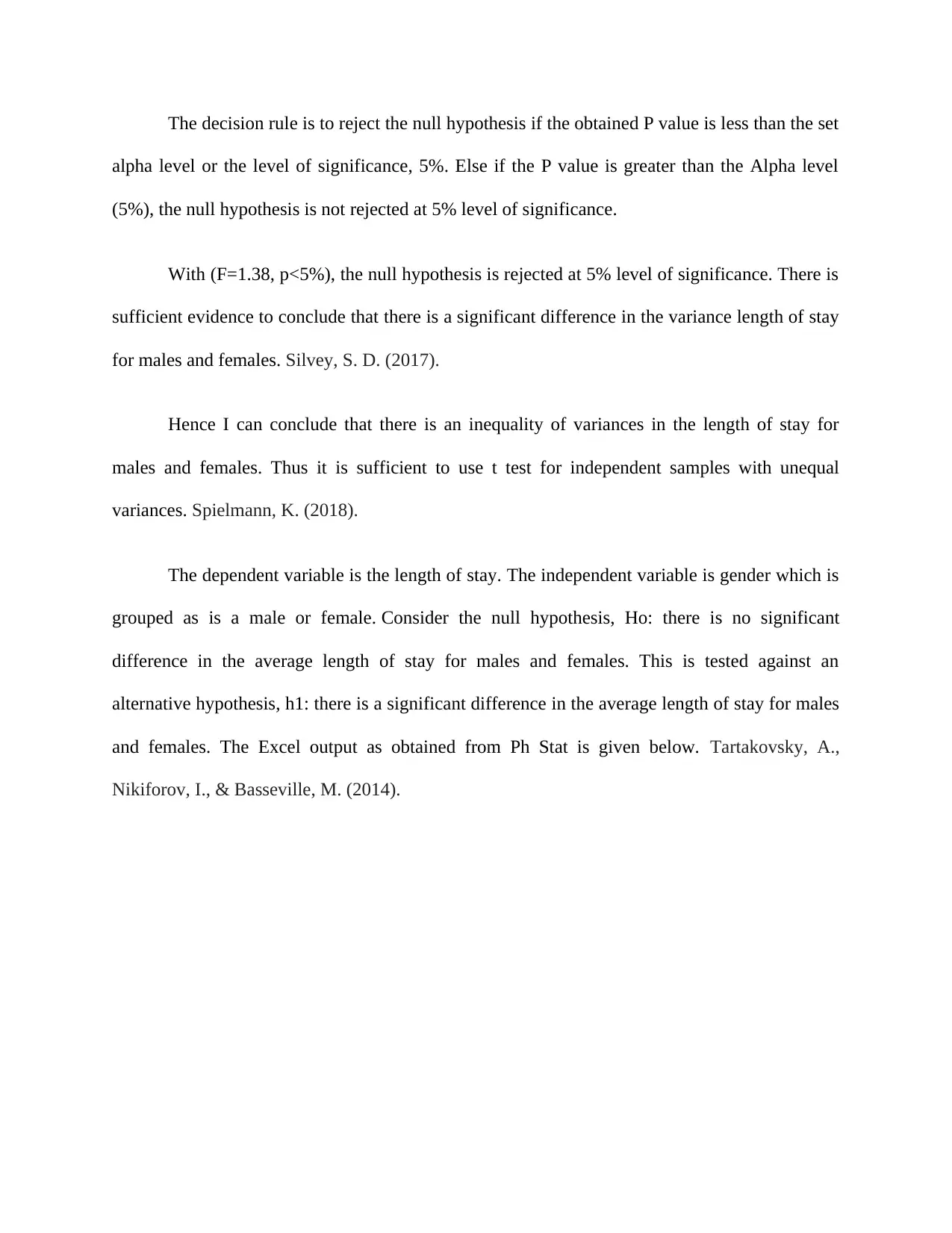
The decision rule is to reject the null hypothesis if the obtained P value is less than the set
alpha level or the level of significance, 5%. Else if the P value is greater than the Alpha level
(5%), the null hypothesis is not rejected at 5% level of significance.
With (F=1.38, p<5%), the null hypothesis is rejected at 5% level of significance. There is
sufficient evidence to conclude that there is a significant difference in the variance length of stay
for males and females. Silvey, S. D. (2017).
Hence I can conclude that there is an inequality of variances in the length of stay for
males and females. Thus it is sufficient to use t test for independent samples with unequal
variances. Spielmann, K. (2018).
The dependent variable is the length of stay. The independent variable is gender which is
grouped as is a male or female. Consider the null hypothesis, Ho: there is no significant
difference in the average length of stay for males and females. This is tested against an
alternative hypothesis, h1: there is a significant difference in the average length of stay for males
and females. The Excel output as obtained from Ph Stat is given below. Tartakovsky, A.,
Nikiforov, I., & Basseville, M. (2014).
alpha level or the level of significance, 5%. Else if the P value is greater than the Alpha level
(5%), the null hypothesis is not rejected at 5% level of significance.
With (F=1.38, p<5%), the null hypothesis is rejected at 5% level of significance. There is
sufficient evidence to conclude that there is a significant difference in the variance length of stay
for males and females. Silvey, S. D. (2017).
Hence I can conclude that there is an inequality of variances in the length of stay for
males and females. Thus it is sufficient to use t test for independent samples with unequal
variances. Spielmann, K. (2018).
The dependent variable is the length of stay. The independent variable is gender which is
grouped as is a male or female. Consider the null hypothesis, Ho: there is no significant
difference in the average length of stay for males and females. This is tested against an
alternative hypothesis, h1: there is a significant difference in the average length of stay for males
and females. The Excel output as obtained from Ph Stat is given below. Tartakovsky, A.,
Nikiforov, I., & Basseville, M. (2014).
Paraphrase This Document
Need a fresh take? Get an instant paraphrase of this document with our AI Paraphraser
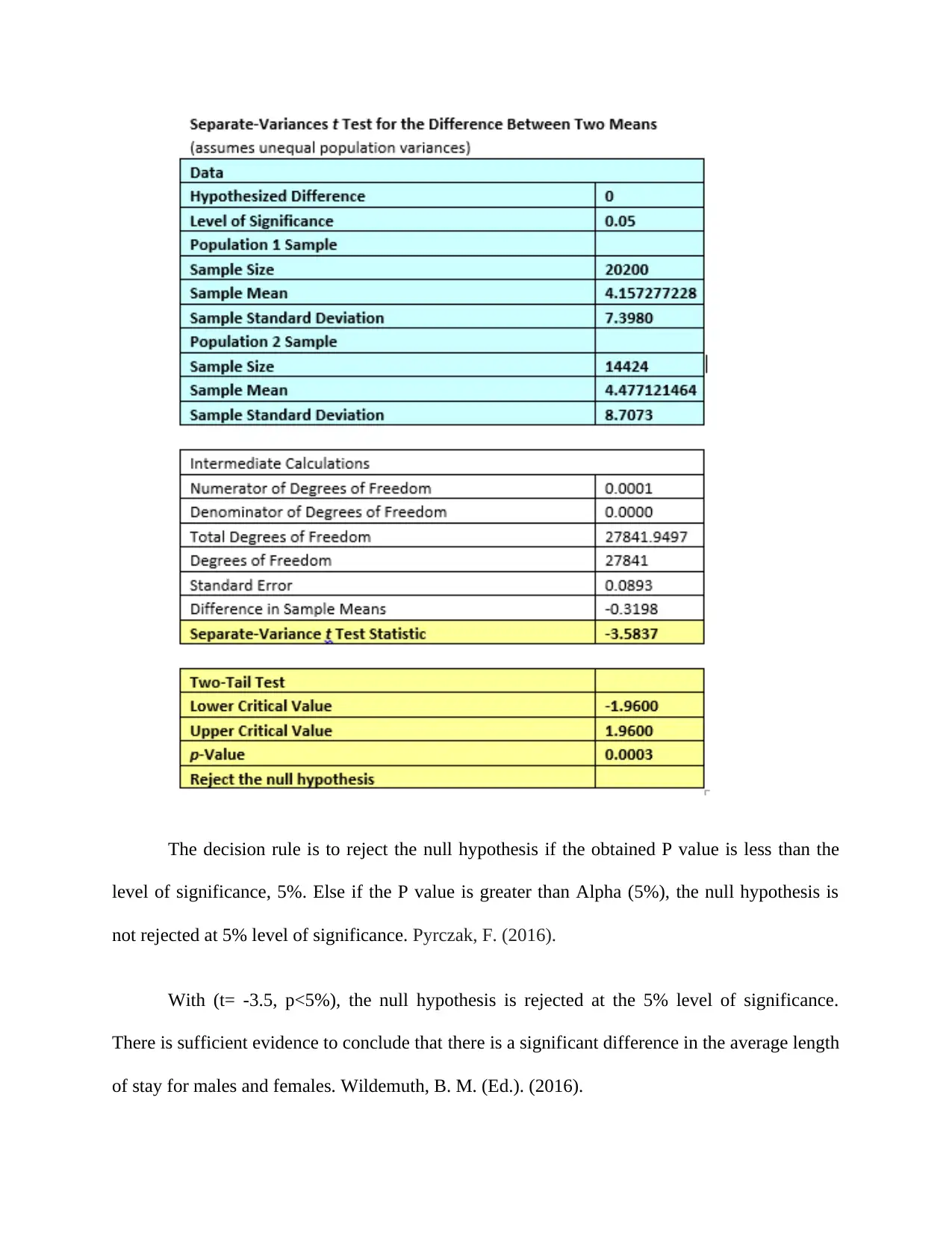
The decision rule is to reject the null hypothesis if the obtained P value is less than the
level of significance, 5%. Else if the P value is greater than Alpha (5%), the null hypothesis is
not rejected at 5% level of significance. Pyrczak, F. (2016).
With (t= -3.5, p<5%), the null hypothesis is rejected at the 5% level of significance.
There is sufficient evidence to conclude that there is a significant difference in the average length
of stay for males and females. Wildemuth, B. M. (Ed.). (2016).
level of significance, 5%. Else if the P value is greater than Alpha (5%), the null hypothesis is
not rejected at 5% level of significance. Pyrczak, F. (2016).
With (t= -3.5, p<5%), the null hypothesis is rejected at the 5% level of significance.
There is sufficient evidence to conclude that there is a significant difference in the average length
of stay for males and females. Wildemuth, B. M. (Ed.). (2016).
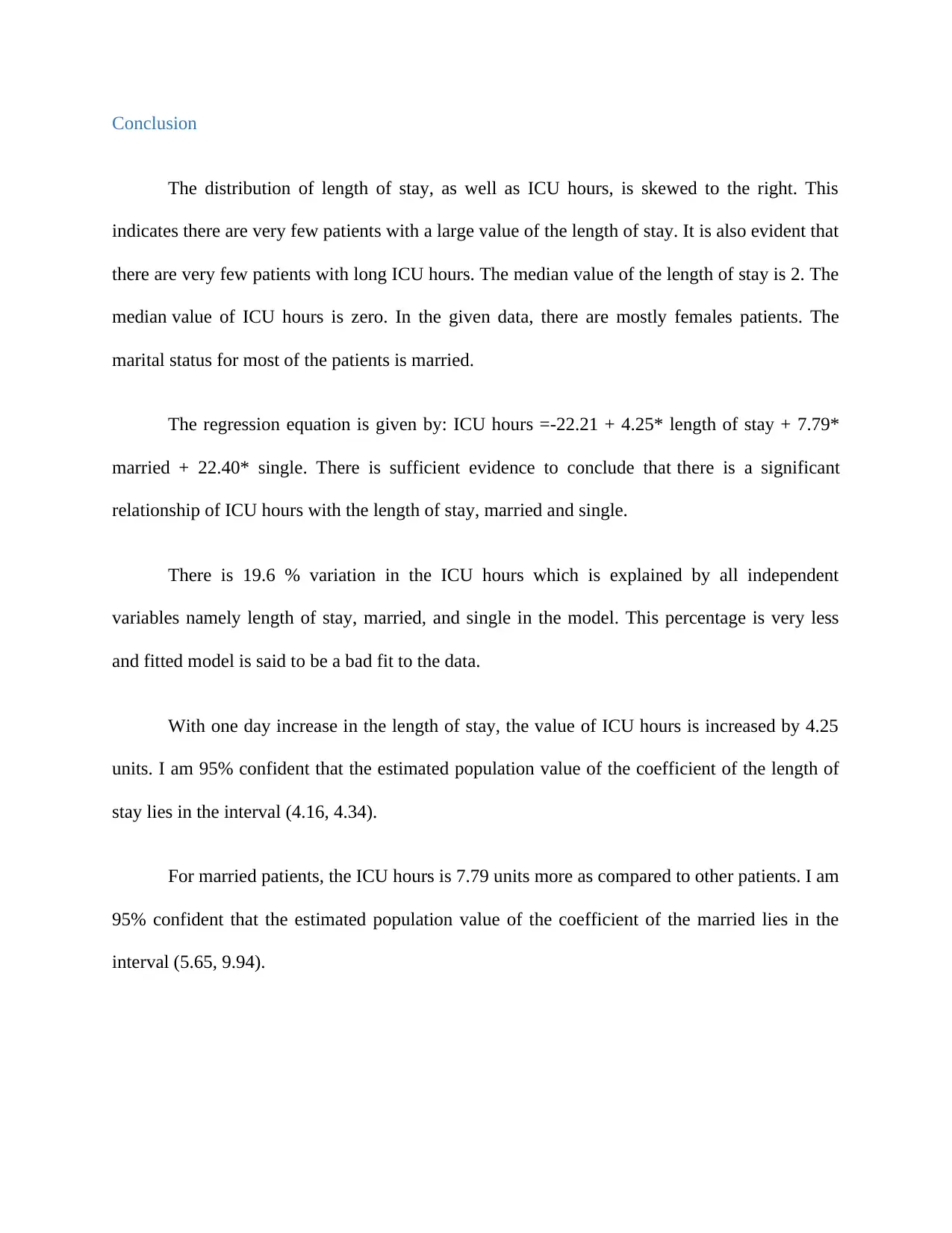
Conclusion
The distribution of length of stay, as well as ICU hours, is skewed to the right. This
indicates there are very few patients with a large value of the length of stay. It is also evident that
there are very few patients with long ICU hours. The median value of the length of stay is 2. The
median value of ICU hours is zero. In the given data, there are mostly females patients. The
marital status for most of the patients is married.
The regression equation is given by: ICU hours =-22.21 + 4.25* length of stay + 7.79*
married + 22.40* single. There is sufficient evidence to conclude that there is a significant
relationship of ICU hours with the length of stay, married and single.
There is 19.6 % variation in the ICU hours which is explained by all independent
variables namely length of stay, married, and single in the model. This percentage is very less
and fitted model is said to be a bad fit to the data.
With one day increase in the length of stay, the value of ICU hours is increased by 4.25
units. I am 95% confident that the estimated population value of the coefficient of the length of
stay lies in the interval (4.16, 4.34).
For married patients, the ICU hours is 7.79 units more as compared to other patients. I am
95% confident that the estimated population value of the coefficient of the married lies in the
interval (5.65, 9.94).
The distribution of length of stay, as well as ICU hours, is skewed to the right. This
indicates there are very few patients with a large value of the length of stay. It is also evident that
there are very few patients with long ICU hours. The median value of the length of stay is 2. The
median value of ICU hours is zero. In the given data, there are mostly females patients. The
marital status for most of the patients is married.
The regression equation is given by: ICU hours =-22.21 + 4.25* length of stay + 7.79*
married + 22.40* single. There is sufficient evidence to conclude that there is a significant
relationship of ICU hours with the length of stay, married and single.
There is 19.6 % variation in the ICU hours which is explained by all independent
variables namely length of stay, married, and single in the model. This percentage is very less
and fitted model is said to be a bad fit to the data.
With one day increase in the length of stay, the value of ICU hours is increased by 4.25
units. I am 95% confident that the estimated population value of the coefficient of the length of
stay lies in the interval (4.16, 4.34).
For married patients, the ICU hours is 7.79 units more as compared to other patients. I am
95% confident that the estimated population value of the coefficient of the married lies in the
interval (5.65, 9.94).
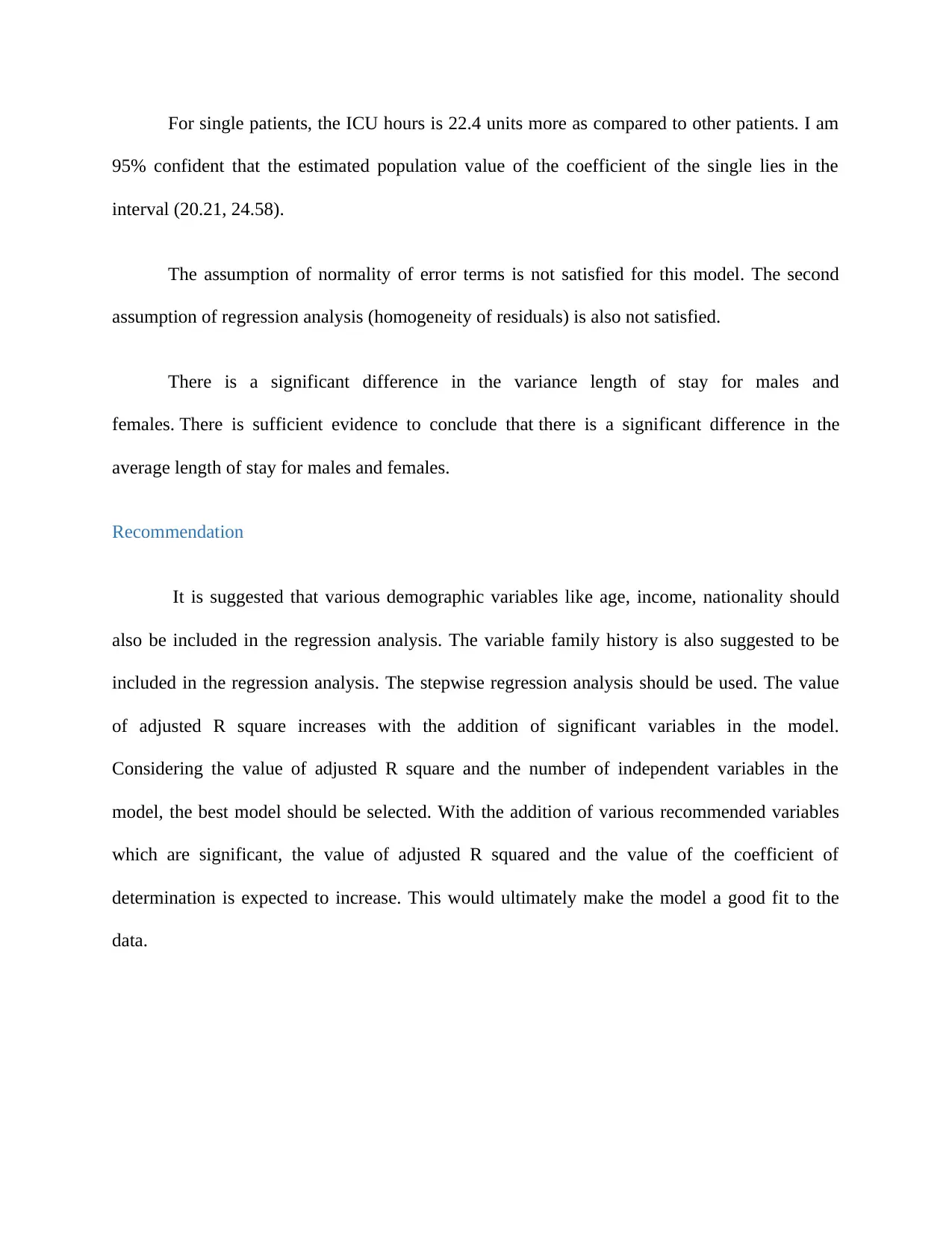
For single patients, the ICU hours is 22.4 units more as compared to other patients. I am
95% confident that the estimated population value of the coefficient of the single lies in the
interval (20.21, 24.58).
The assumption of normality of error terms is not satisfied for this model. The second
assumption of regression analysis (homogeneity of residuals) is also not satisfied.
There is a significant difference in the variance length of stay for males and
females. There is sufficient evidence to conclude that there is a significant difference in the
average length of stay for males and females.
Recommendation
It is suggested that various demographic variables like age, income, nationality should
also be included in the regression analysis. The variable family history is also suggested to be
included in the regression analysis. The stepwise regression analysis should be used. The value
of adjusted R square increases with the addition of significant variables in the model.
Considering the value of adjusted R square and the number of independent variables in the
model, the best model should be selected. With the addition of various recommended variables
which are significant, the value of adjusted R squared and the value of the coefficient of
determination is expected to increase. This would ultimately make the model a good fit to the
data.
95% confident that the estimated population value of the coefficient of the single lies in the
interval (20.21, 24.58).
The assumption of normality of error terms is not satisfied for this model. The second
assumption of regression analysis (homogeneity of residuals) is also not satisfied.
There is a significant difference in the variance length of stay for males and
females. There is sufficient evidence to conclude that there is a significant difference in the
average length of stay for males and females.
Recommendation
It is suggested that various demographic variables like age, income, nationality should
also be included in the regression analysis. The variable family history is also suggested to be
included in the regression analysis. The stepwise regression analysis should be used. The value
of adjusted R square increases with the addition of significant variables in the model.
Considering the value of adjusted R square and the number of independent variables in the
model, the best model should be selected. With the addition of various recommended variables
which are significant, the value of adjusted R squared and the value of the coefficient of
determination is expected to increase. This would ultimately make the model a good fit to the
data.
Secure Best Marks with AI Grader
Need help grading? Try our AI Grader for instant feedback on your assignments.
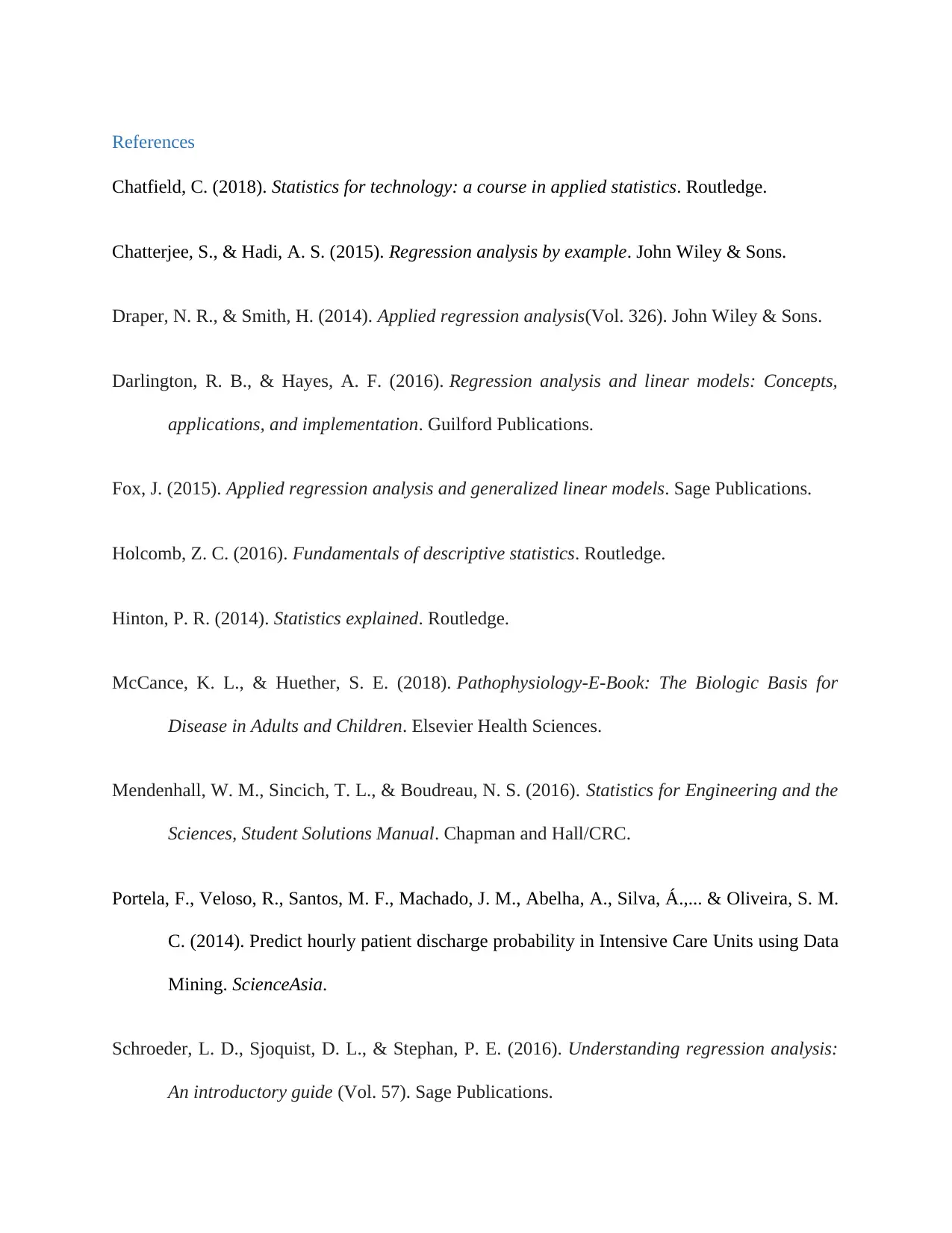
References
Chatfield, C. (2018). Statistics for technology: a course in applied statistics. Routledge.
Chatterjee, S., & Hadi, A. S. (2015). Regression analysis by example. John Wiley & Sons.
Draper, N. R., & Smith, H. (2014). Applied regression analysis(Vol. 326). John Wiley & Sons.
Darlington, R. B., & Hayes, A. F. (2016). Regression analysis and linear models: Concepts,
applications, and implementation. Guilford Publications.
Fox, J. (2015). Applied regression analysis and generalized linear models. Sage Publications.
Holcomb, Z. C. (2016). Fundamentals of descriptive statistics. Routledge.
Hinton, P. R. (2014). Statistics explained. Routledge.
McCance, K. L., & Huether, S. E. (2018). Pathophysiology-E-Book: The Biologic Basis for
Disease in Adults and Children. Elsevier Health Sciences.
Mendenhall, W. M., Sincich, T. L., & Boudreau, N. S. (2016). Statistics for Engineering and the
Sciences, Student Solutions Manual. Chapman and Hall/CRC.
Portela, F., Veloso, R., Santos, M. F., Machado, J. M., Abelha, A., Silva, Á.,... & Oliveira, S. M.
C. (2014). Predict hourly patient discharge probability in Intensive Care Units using Data
Mining. ScienceAsia.
Schroeder, L. D., Sjoquist, D. L., & Stephan, P. E. (2016). Understanding regression analysis:
An introductory guide (Vol. 57). Sage Publications.
Chatfield, C. (2018). Statistics for technology: a course in applied statistics. Routledge.
Chatterjee, S., & Hadi, A. S. (2015). Regression analysis by example. John Wiley & Sons.
Draper, N. R., & Smith, H. (2014). Applied regression analysis(Vol. 326). John Wiley & Sons.
Darlington, R. B., & Hayes, A. F. (2016). Regression analysis and linear models: Concepts,
applications, and implementation. Guilford Publications.
Fox, J. (2015). Applied regression analysis and generalized linear models. Sage Publications.
Holcomb, Z. C. (2016). Fundamentals of descriptive statistics. Routledge.
Hinton, P. R. (2014). Statistics explained. Routledge.
McCance, K. L., & Huether, S. E. (2018). Pathophysiology-E-Book: The Biologic Basis for
Disease in Adults and Children. Elsevier Health Sciences.
Mendenhall, W. M., Sincich, T. L., & Boudreau, N. S. (2016). Statistics for Engineering and the
Sciences, Student Solutions Manual. Chapman and Hall/CRC.
Portela, F., Veloso, R., Santos, M. F., Machado, J. M., Abelha, A., Silva, Á.,... & Oliveira, S. M.
C. (2014). Predict hourly patient discharge probability in Intensive Care Units using Data
Mining. ScienceAsia.
Schroeder, L. D., Sjoquist, D. L., & Stephan, P. E. (2016). Understanding regression analysis:
An introductory guide (Vol. 57). Sage Publications.
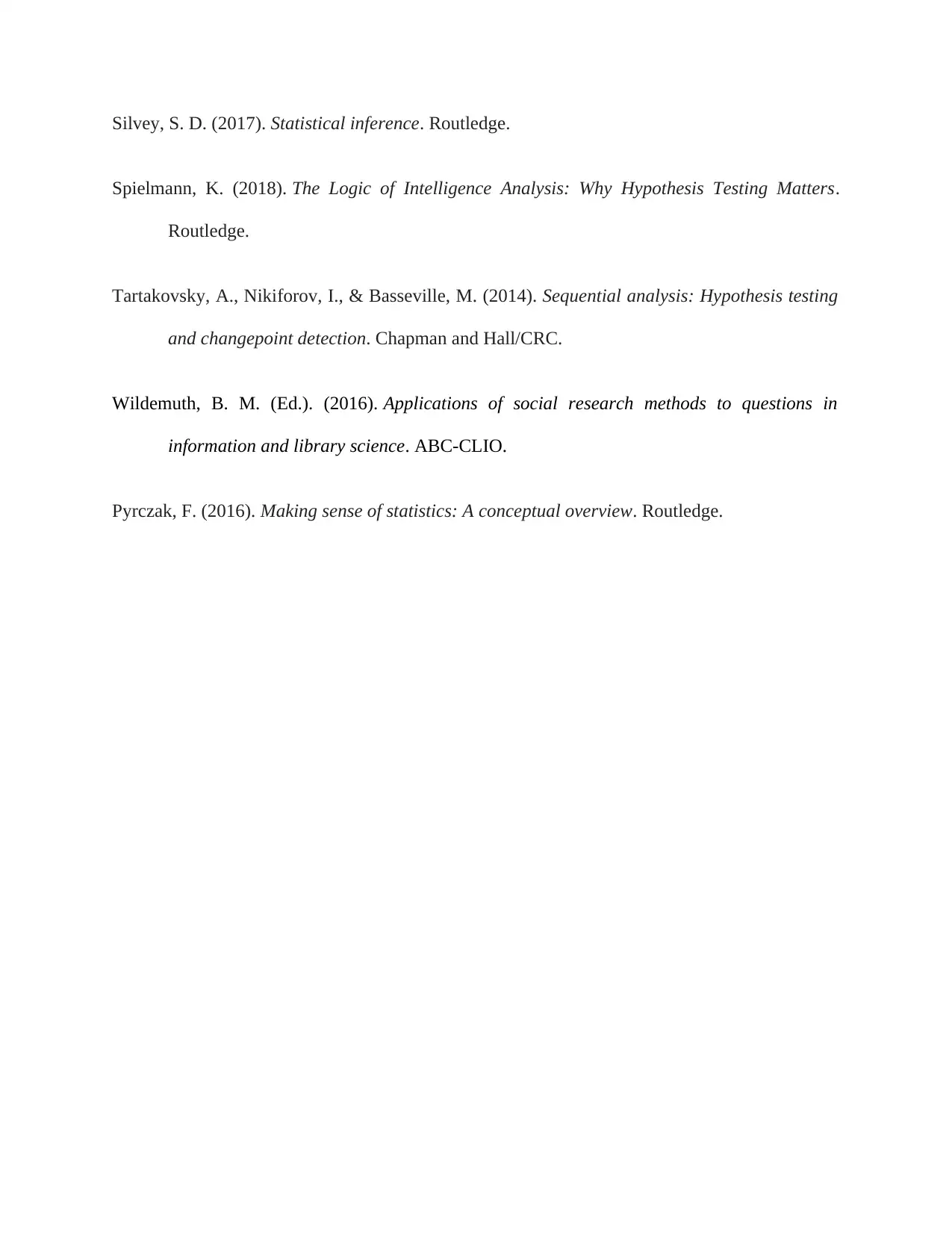
Silvey, S. D. (2017). Statistical inference. Routledge.
Spielmann, K. (2018). The Logic of Intelligence Analysis: Why Hypothesis Testing Matters.
Routledge.
Tartakovsky, A., Nikiforov, I., & Basseville, M. (2014). Sequential analysis: Hypothesis testing
and changepoint detection. Chapman and Hall/CRC.
Wildemuth, B. M. (Ed.). (2016). Applications of social research methods to questions in
information and library science. ABC-CLIO.
Pyrczak, F. (2016). Making sense of statistics: A conceptual overview. Routledge.
Spielmann, K. (2018). The Logic of Intelligence Analysis: Why Hypothesis Testing Matters.
Routledge.
Tartakovsky, A., Nikiforov, I., & Basseville, M. (2014). Sequential analysis: Hypothesis testing
and changepoint detection. Chapman and Hall/CRC.
Wildemuth, B. M. (Ed.). (2016). Applications of social research methods to questions in
information and library science. ABC-CLIO.
Pyrczak, F. (2016). Making sense of statistics: A conceptual overview. Routledge.
1 out of 15
Related Documents
![[object Object]](/_next/image/?url=%2F_next%2Fstatic%2Fmedia%2Flogo.6d15ce61.png&w=640&q=75)
Your All-in-One AI-Powered Toolkit for Academic Success.
+13062052269
info@desklib.com
Available 24*7 on WhatsApp / Email
Unlock your academic potential
© 2024 | Zucol Services PVT LTD | All rights reserved.