Remote Sensing in Plant Sciences: A Comprehensive Analysis
VerifiedAdded on 2022/09/07
|19
|5644
|17
Report
AI Summary
This report examines the application of remote sensing in plant sciences, emphasizing its role in modern agriculture and crop management. It explores the evolution of remote sensing, from early satellite imagery to advanced techniques using various sensors and spectral bands. The report highlights the use of remote sensing for crop yield projections, crop condition assessments, and crop type classification. It delves into specific indices like NDVI and LSWI, and how they are used to monitor plant health, soil moisture, and vegetation stress. The study further discusses the capabilities of different satellite systems, including MODIS and Sentinel-2, and their contributions to precision agriculture. It underscores the importance of rapid data turnaround and the integration of remote sensing with ecological and surface data for comprehensive crop assessment. The report concludes by emphasizing the potential of remote sensing to improve crop yields, identify plant diseases, and support sustainable farming practices.
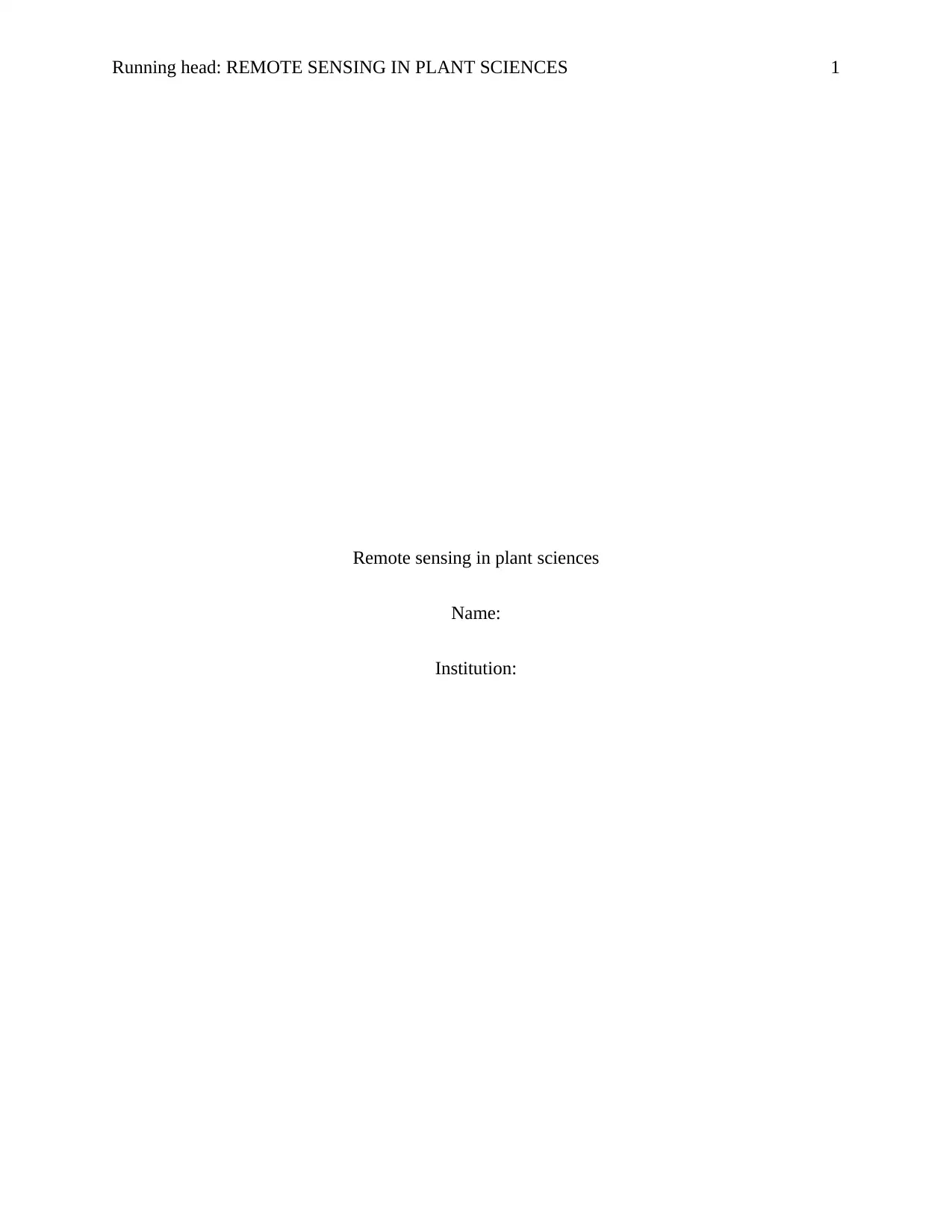
Running head: REMOTE SENSING IN PLANT SCIENCES 1
Remote sensing in plant sciences
Name:
Institution:
Remote sensing in plant sciences
Name:
Institution:
Paraphrase This Document
Need a fresh take? Get an instant paraphrase of this document with our AI Paraphraser
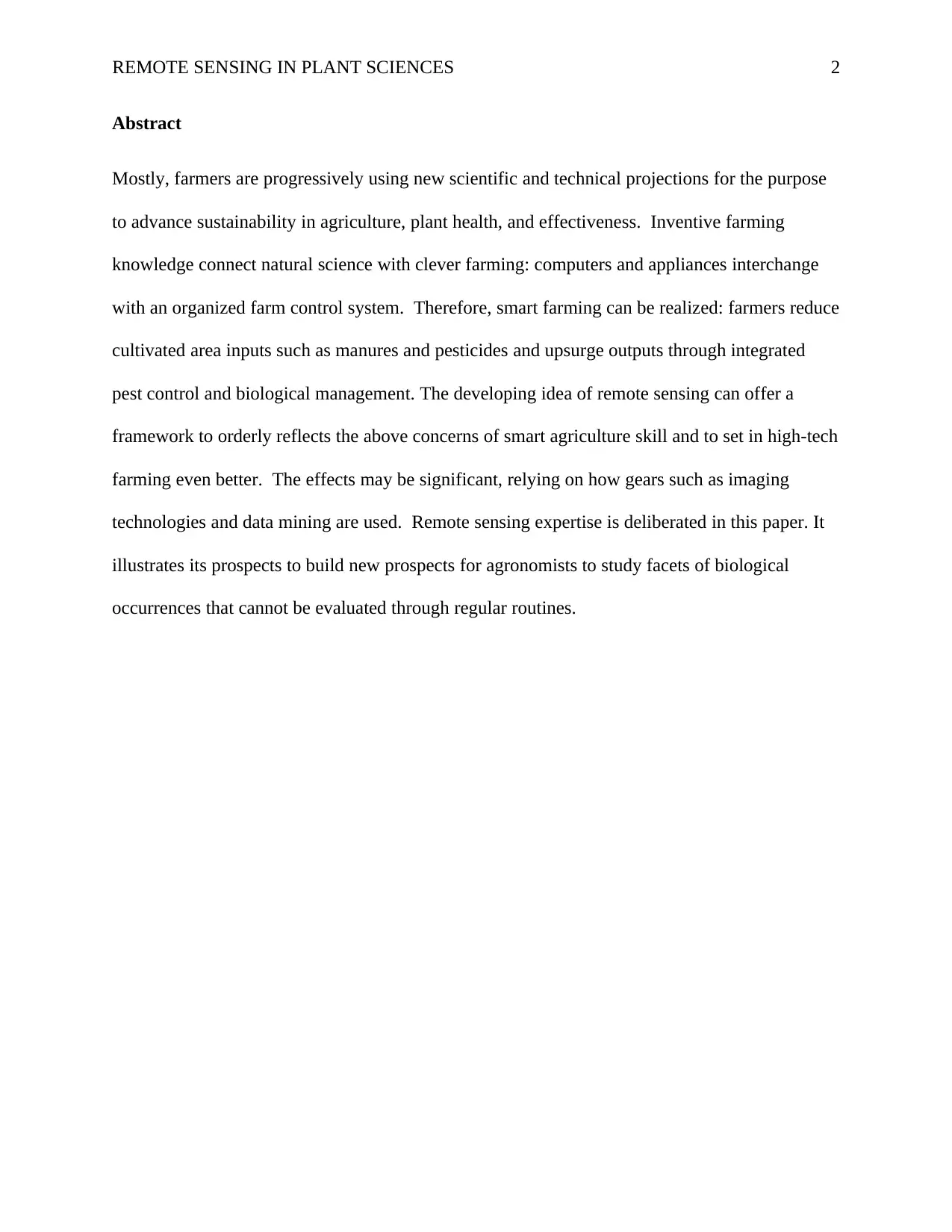
REMOTE SENSING IN PLANT SCIENCES 2
Abstract
Mostly, farmers are progressively using new scientific and technical projections for the purpose
to advance sustainability in agriculture, plant health, and effectiveness. Inventive farming
knowledge connect natural science with clever farming: computers and appliances interchange
with an organized farm control system. Therefore, smart farming can be realized: farmers reduce
cultivated area inputs such as manures and pesticides and upsurge outputs through integrated
pest control and biological management. The developing idea of remote sensing can offer a
framework to orderly reflects the above concerns of smart agriculture skill and to set in high-tech
farming even better. The effects may be significant, relying on how gears such as imaging
technologies and data mining are used. Remote sensing expertise is deliberated in this paper. It
illustrates its prospects to build new prospects for agronomists to study facets of biological
occurrences that cannot be evaluated through regular routines.
Abstract
Mostly, farmers are progressively using new scientific and technical projections for the purpose
to advance sustainability in agriculture, plant health, and effectiveness. Inventive farming
knowledge connect natural science with clever farming: computers and appliances interchange
with an organized farm control system. Therefore, smart farming can be realized: farmers reduce
cultivated area inputs such as manures and pesticides and upsurge outputs through integrated
pest control and biological management. The developing idea of remote sensing can offer a
framework to orderly reflects the above concerns of smart agriculture skill and to set in high-tech
farming even better. The effects may be significant, relying on how gears such as imaging
technologies and data mining are used. Remote sensing expertise is deliberated in this paper. It
illustrates its prospects to build new prospects for agronomists to study facets of biological
occurrences that cannot be evaluated through regular routines.
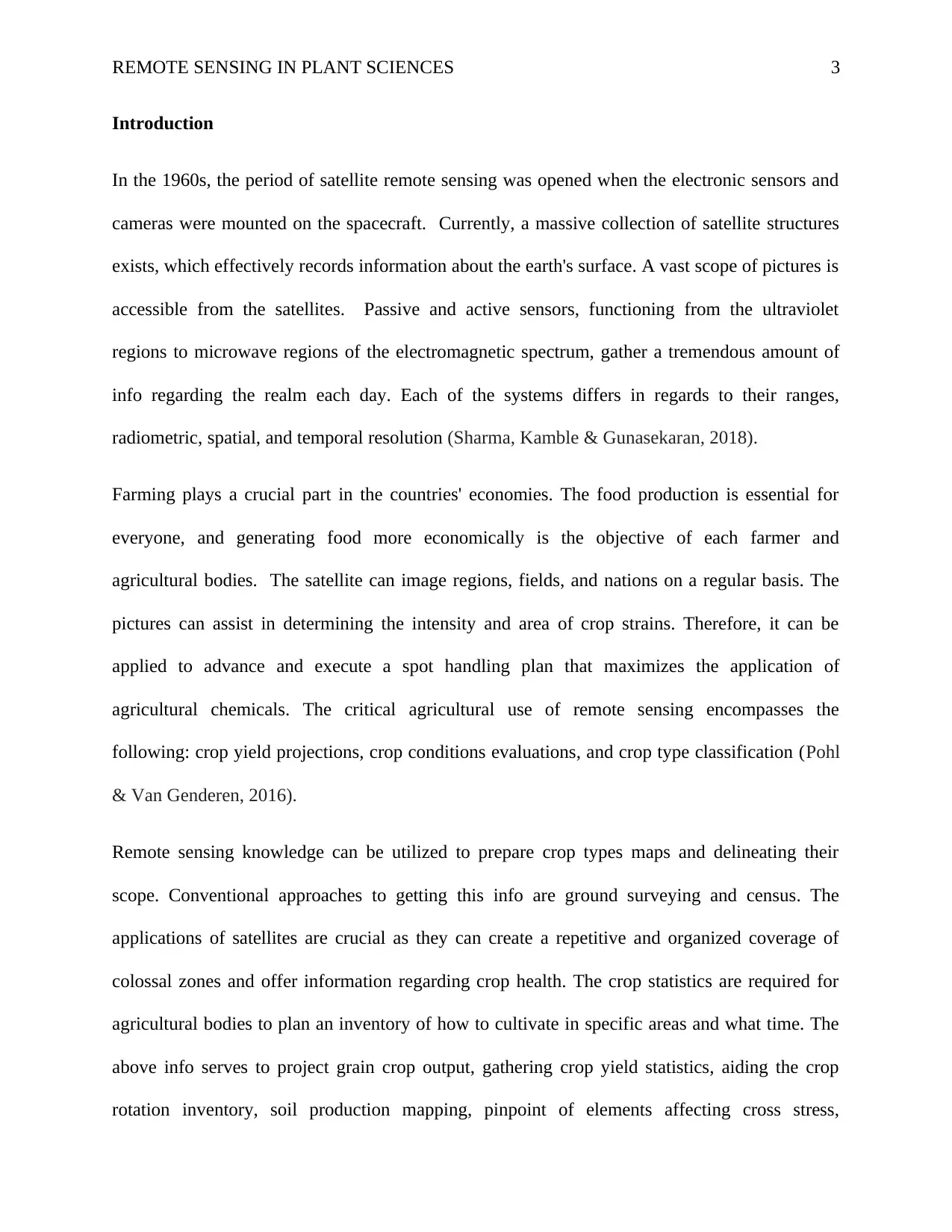
REMOTE SENSING IN PLANT SCIENCES 3
Introduction
In the 1960s, the period of satellite remote sensing was opened when the electronic sensors and
cameras were mounted on the spacecraft. Currently, a massive collection of satellite structures
exists, which effectively records information about the earth's surface. A vast scope of pictures is
accessible from the satellites. Passive and active sensors, functioning from the ultraviolet
regions to microwave regions of the electromagnetic spectrum, gather a tremendous amount of
info regarding the realm each day. Each of the systems differs in regards to their ranges,
radiometric, spatial, and temporal resolution (Sharma, Kamble & Gunasekaran, 2018).
Farming plays a crucial part in the countries' economies. The food production is essential for
everyone, and generating food more economically is the objective of each farmer and
agricultural bodies. The satellite can image regions, fields, and nations on a regular basis. The
pictures can assist in determining the intensity and area of crop strains. Therefore, it can be
applied to advance and execute a spot handling plan that maximizes the application of
agricultural chemicals. The critical agricultural use of remote sensing encompasses the
following: crop yield projections, crop conditions evaluations, and crop type classification (Pohl
& Van Genderen, 2016).
Remote sensing knowledge can be utilized to prepare crop types maps and delineating their
scope. Conventional approaches to getting this info are ground surveying and census. The
applications of satellites are crucial as they can create a repetitive and organized coverage of
colossal zones and offer information regarding crop health. The crop statistics are required for
agricultural bodies to plan an inventory of how to cultivate in specific areas and what time. The
above info serves to project grain crop output, gathering crop yield statistics, aiding the crop
rotation inventory, soil production mapping, pinpoint of elements affecting cross stress,
Introduction
In the 1960s, the period of satellite remote sensing was opened when the electronic sensors and
cameras were mounted on the spacecraft. Currently, a massive collection of satellite structures
exists, which effectively records information about the earth's surface. A vast scope of pictures is
accessible from the satellites. Passive and active sensors, functioning from the ultraviolet
regions to microwave regions of the electromagnetic spectrum, gather a tremendous amount of
info regarding the realm each day. Each of the systems differs in regards to their ranges,
radiometric, spatial, and temporal resolution (Sharma, Kamble & Gunasekaran, 2018).
Farming plays a crucial part in the countries' economies. The food production is essential for
everyone, and generating food more economically is the objective of each farmer and
agricultural bodies. The satellite can image regions, fields, and nations on a regular basis. The
pictures can assist in determining the intensity and area of crop strains. Therefore, it can be
applied to advance and execute a spot handling plan that maximizes the application of
agricultural chemicals. The critical agricultural use of remote sensing encompasses the
following: crop yield projections, crop conditions evaluations, and crop type classification (Pohl
& Van Genderen, 2016).
Remote sensing knowledge can be utilized to prepare crop types maps and delineating their
scope. Conventional approaches to getting this info are ground surveying and census. The
applications of satellites are crucial as they can create a repetitive and organized coverage of
colossal zones and offer information regarding crop health. The crop statistics are required for
agricultural bodies to plan an inventory of how to cultivate in specific areas and what time. The
above info serves to project grain crop output, gathering crop yield statistics, aiding the crop
rotation inventory, soil production mapping, pinpoint of elements affecting cross stress,
You're viewing a preview
Unlock full access by subscribing today!
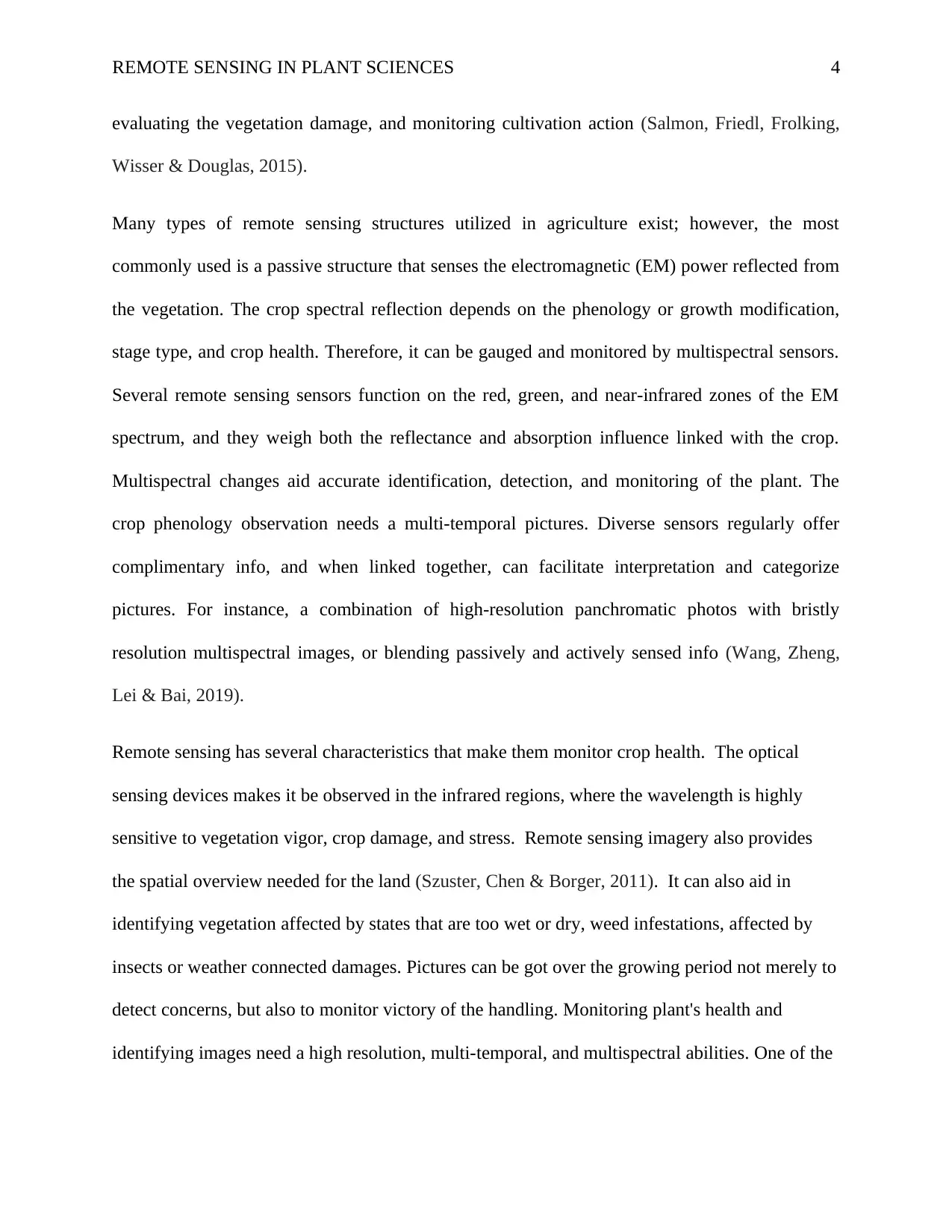
REMOTE SENSING IN PLANT SCIENCES 4
evaluating the vegetation damage, and monitoring cultivation action (Salmon, Friedl, Frolking,
Wisser & Douglas, 2015).
Many types of remote sensing structures utilized in agriculture exist; however, the most
commonly used is a passive structure that senses the electromagnetic (EM) power reflected from
the vegetation. The crop spectral reflection depends on the phenology or growth modification,
stage type, and crop health. Therefore, it can be gauged and monitored by multispectral sensors.
Several remote sensing sensors function on the red, green, and near-infrared zones of the EM
spectrum, and they weigh both the reflectance and absorption influence linked with the crop.
Multispectral changes aid accurate identification, detection, and monitoring of the plant. The
crop phenology observation needs a multi-temporal pictures. Diverse sensors regularly offer
complimentary info, and when linked together, can facilitate interpretation and categorize
pictures. For instance, a combination of high-resolution panchromatic photos with bristly
resolution multispectral images, or blending passively and actively sensed info (Wang, Zheng,
Lei & Bai, 2019).
Remote sensing has several characteristics that make them monitor crop health. The optical
sensing devices makes it be observed in the infrared regions, where the wavelength is highly
sensitive to vegetation vigor, crop damage, and stress. Remote sensing imagery also provides
the spatial overview needed for the land (Szuster, Chen & Borger, 2011). It can also aid in
identifying vegetation affected by states that are too wet or dry, weed infestations, affected by
insects or weather connected damages. Pictures can be got over the growing period not merely to
detect concerns, but also to monitor victory of the handling. Monitoring plant's health and
identifying images need a high resolution, multi-temporal, and multispectral abilities. One of the
evaluating the vegetation damage, and monitoring cultivation action (Salmon, Friedl, Frolking,
Wisser & Douglas, 2015).
Many types of remote sensing structures utilized in agriculture exist; however, the most
commonly used is a passive structure that senses the electromagnetic (EM) power reflected from
the vegetation. The crop spectral reflection depends on the phenology or growth modification,
stage type, and crop health. Therefore, it can be gauged and monitored by multispectral sensors.
Several remote sensing sensors function on the red, green, and near-infrared zones of the EM
spectrum, and they weigh both the reflectance and absorption influence linked with the crop.
Multispectral changes aid accurate identification, detection, and monitoring of the plant. The
crop phenology observation needs a multi-temporal pictures. Diverse sensors regularly offer
complimentary info, and when linked together, can facilitate interpretation and categorize
pictures. For instance, a combination of high-resolution panchromatic photos with bristly
resolution multispectral images, or blending passively and actively sensed info (Wang, Zheng,
Lei & Bai, 2019).
Remote sensing has several characteristics that make them monitor crop health. The optical
sensing devices makes it be observed in the infrared regions, where the wavelength is highly
sensitive to vegetation vigor, crop damage, and stress. Remote sensing imagery also provides
the spatial overview needed for the land (Szuster, Chen & Borger, 2011). It can also aid in
identifying vegetation affected by states that are too wet or dry, weed infestations, affected by
insects or weather connected damages. Pictures can be got over the growing period not merely to
detect concerns, but also to monitor victory of the handling. Monitoring plant's health and
identifying images need a high resolution, multi-temporal, and multispectral abilities. One of the
Paraphrase This Document
Need a fresh take? Get an instant paraphrase of this document with our AI Paraphraser
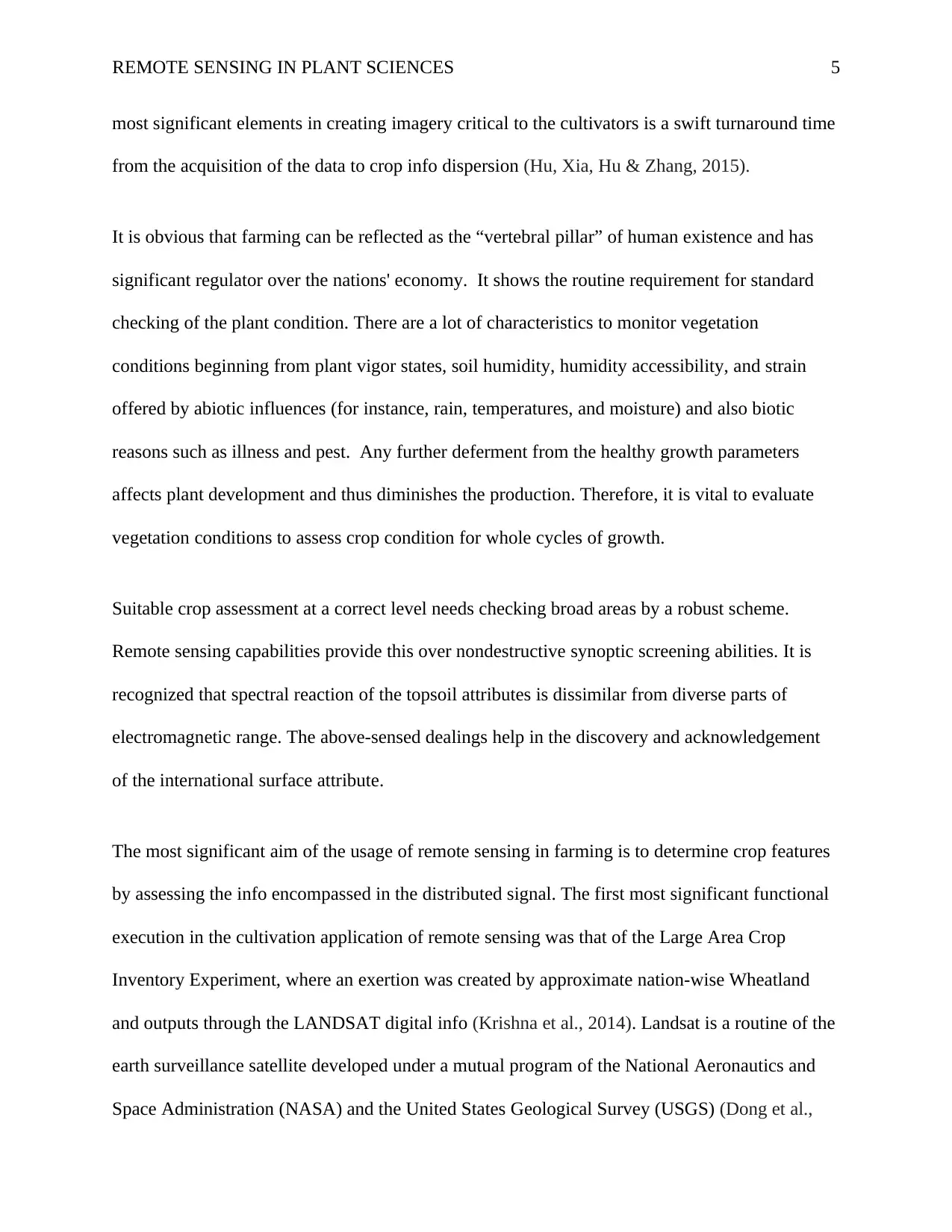
REMOTE SENSING IN PLANT SCIENCES 5
most significant elements in creating imagery critical to the cultivators is a swift turnaround time
from the acquisition of the data to crop info dispersion (Hu, Xia, Hu & Zhang, 2015).
It is obvious that farming can be reflected as the “vertebral pillar” of human existence and has
significant regulator over the nations' economy. It shows the routine requirement for standard
checking of the plant condition. There are a lot of characteristics to monitor vegetation
conditions beginning from plant vigor states, soil humidity, humidity accessibility, and strain
offered by abiotic influences (for instance, rain, temperatures, and moisture) and also biotic
reasons such as illness and pest. Any further deferment from the healthy growth parameters
affects plant development and thus diminishes the production. Therefore, it is vital to evaluate
vegetation conditions to assess crop condition for whole cycles of growth.
Suitable crop assessment at a correct level needs checking broad areas by a robust scheme.
Remote sensing capabilities provide this over nondestructive synoptic screening abilities. It is
recognized that spectral reaction of the topsoil attributes is dissimilar from diverse parts of
electromagnetic range. The above-sensed dealings help in the discovery and acknowledgement
of the international surface attribute.
The most significant aim of the usage of remote sensing in farming is to determine crop features
by assessing the info encompassed in the distributed signal. The first most significant functional
execution in the cultivation application of remote sensing was that of the Large Area Crop
Inventory Experiment, where an exertion was created by approximate nation-wise Wheatland
and outputs through the LANDSAT digital info (Krishna et al., 2014). Landsat is a routine of the
earth surveillance satellite developed under a mutual program of the National Aeronautics and
Space Administration (NASA) and the United States Geological Survey (USGS) (Dong et al.,
most significant elements in creating imagery critical to the cultivators is a swift turnaround time
from the acquisition of the data to crop info dispersion (Hu, Xia, Hu & Zhang, 2015).
It is obvious that farming can be reflected as the “vertebral pillar” of human existence and has
significant regulator over the nations' economy. It shows the routine requirement for standard
checking of the plant condition. There are a lot of characteristics to monitor vegetation
conditions beginning from plant vigor states, soil humidity, humidity accessibility, and strain
offered by abiotic influences (for instance, rain, temperatures, and moisture) and also biotic
reasons such as illness and pest. Any further deferment from the healthy growth parameters
affects plant development and thus diminishes the production. Therefore, it is vital to evaluate
vegetation conditions to assess crop condition for whole cycles of growth.
Suitable crop assessment at a correct level needs checking broad areas by a robust scheme.
Remote sensing capabilities provide this over nondestructive synoptic screening abilities. It is
recognized that spectral reaction of the topsoil attributes is dissimilar from diverse parts of
electromagnetic range. The above-sensed dealings help in the discovery and acknowledgement
of the international surface attribute.
The most significant aim of the usage of remote sensing in farming is to determine crop features
by assessing the info encompassed in the distributed signal. The first most significant functional
execution in the cultivation application of remote sensing was that of the Large Area Crop
Inventory Experiment, where an exertion was created by approximate nation-wise Wheatland
and outputs through the LANDSAT digital info (Krishna et al., 2014). Landsat is a routine of the
earth surveillance satellite developed under a mutual program of the National Aeronautics and
Space Administration (NASA) and the United States Geological Survey (USGS) (Dong et al.,
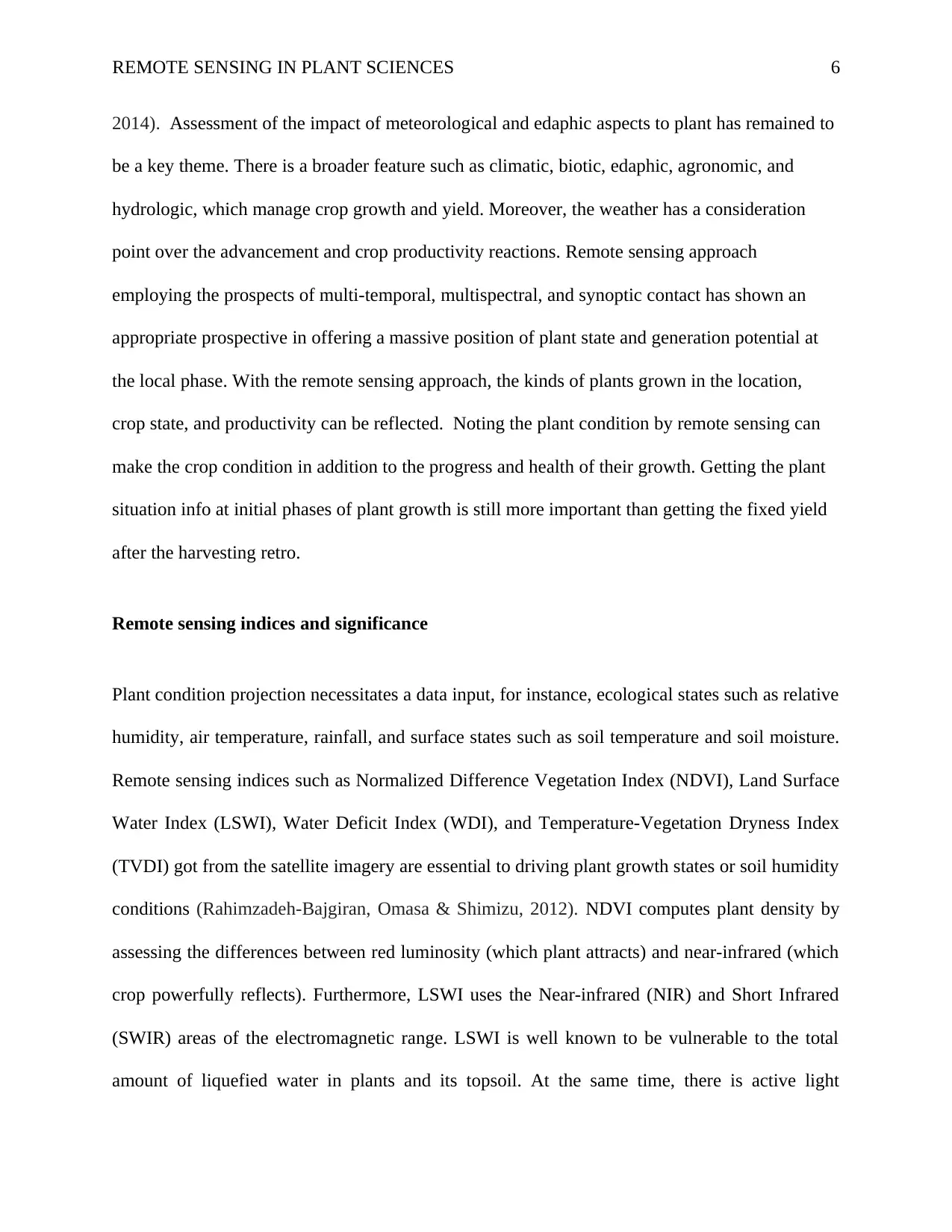
REMOTE SENSING IN PLANT SCIENCES 6
2014). Assessment of the impact of meteorological and edaphic aspects to plant has remained to
be a key theme. There is a broader feature such as climatic, biotic, edaphic, agronomic, and
hydrologic, which manage crop growth and yield. Moreover, the weather has a consideration
point over the advancement and crop productivity reactions. Remote sensing approach
employing the prospects of multi-temporal, multispectral, and synoptic contact has shown an
appropriate prospective in offering a massive position of plant state and generation potential at
the local phase. With the remote sensing approach, the kinds of plants grown in the location,
crop state, and productivity can be reflected. Noting the plant condition by remote sensing can
make the crop condition in addition to the progress and health of their growth. Getting the plant
situation info at initial phases of plant growth is still more important than getting the fixed yield
after the harvesting retro.
Remote sensing indices and significance
Plant condition projection necessitates a data input, for instance, ecological states such as relative
humidity, air temperature, rainfall, and surface states such as soil temperature and soil moisture.
Remote sensing indices such as Normalized Difference Vegetation Index (NDVI), Land Surface
Water Index (LSWI), Water Deficit Index (WDI), and Temperature-Vegetation Dryness Index
(TVDI) got from the satellite imagery are essential to driving plant growth states or soil humidity
conditions (Rahimzadeh-Bajgiran, Omasa & Shimizu, 2012). NDVI computes plant density by
assessing the differences between red luminosity (which plant attracts) and near-infrared (which
crop powerfully reflects). Furthermore, LSWI uses the Near-infrared (NIR) and Short Infrared
(SWIR) areas of the electromagnetic range. LSWI is well known to be vulnerable to the total
amount of liquefied water in plants and its topsoil. At the same time, there is active light
2014). Assessment of the impact of meteorological and edaphic aspects to plant has remained to
be a key theme. There is a broader feature such as climatic, biotic, edaphic, agronomic, and
hydrologic, which manage crop growth and yield. Moreover, the weather has a consideration
point over the advancement and crop productivity reactions. Remote sensing approach
employing the prospects of multi-temporal, multispectral, and synoptic contact has shown an
appropriate prospective in offering a massive position of plant state and generation potential at
the local phase. With the remote sensing approach, the kinds of plants grown in the location,
crop state, and productivity can be reflected. Noting the plant condition by remote sensing can
make the crop condition in addition to the progress and health of their growth. Getting the plant
situation info at initial phases of plant growth is still more important than getting the fixed yield
after the harvesting retro.
Remote sensing indices and significance
Plant condition projection necessitates a data input, for instance, ecological states such as relative
humidity, air temperature, rainfall, and surface states such as soil temperature and soil moisture.
Remote sensing indices such as Normalized Difference Vegetation Index (NDVI), Land Surface
Water Index (LSWI), Water Deficit Index (WDI), and Temperature-Vegetation Dryness Index
(TVDI) got from the satellite imagery are essential to driving plant growth states or soil humidity
conditions (Rahimzadeh-Bajgiran, Omasa & Shimizu, 2012). NDVI computes plant density by
assessing the differences between red luminosity (which plant attracts) and near-infrared (which
crop powerfully reflects). Furthermore, LSWI uses the Near-infrared (NIR) and Short Infrared
(SWIR) areas of the electromagnetic range. LSWI is well known to be vulnerable to the total
amount of liquefied water in plants and its topsoil. At the same time, there is active light
You're viewing a preview
Unlock full access by subscribing today!
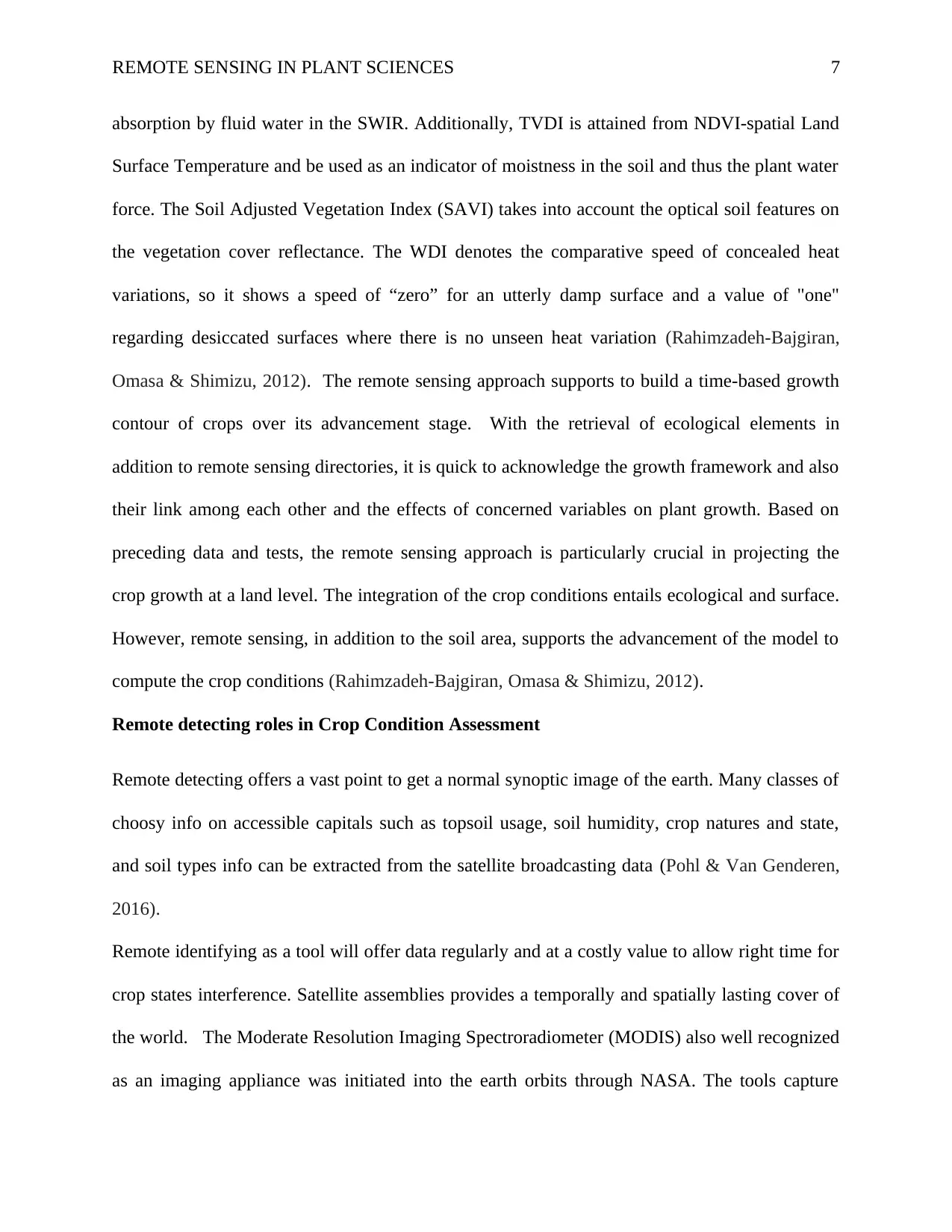
REMOTE SENSING IN PLANT SCIENCES 7
absorption by fluid water in the SWIR. Additionally, TVDI is attained from NDVI-spatial Land
Surface Temperature and be used as an indicator of moistness in the soil and thus the plant water
force. The Soil Adjusted Vegetation Index (SAVI) takes into account the optical soil features on
the vegetation cover reflectance. The WDI denotes the comparative speed of concealed heat
variations, so it shows a speed of “zero” for an utterly damp surface and a value of "one"
regarding desiccated surfaces where there is no unseen heat variation (Rahimzadeh-Bajgiran,
Omasa & Shimizu, 2012). The remote sensing approach supports to build a time-based growth
contour of crops over its advancement stage. With the retrieval of ecological elements in
addition to remote sensing directories, it is quick to acknowledge the growth framework and also
their link among each other and the effects of concerned variables on plant growth. Based on
preceding data and tests, the remote sensing approach is particularly crucial in projecting the
crop growth at a land level. The integration of the crop conditions entails ecological and surface.
However, remote sensing, in addition to the soil area, supports the advancement of the model to
compute the crop conditions (Rahimzadeh-Bajgiran, Omasa & Shimizu, 2012).
Remote detecting roles in Crop Condition Assessment
Remote detecting offers a vast point to get a normal synoptic image of the earth. Many classes of
choosy info on accessible capitals such as topsoil usage, soil humidity, crop natures and state,
and soil types info can be extracted from the satellite broadcasting data (Pohl & Van Genderen,
2016).
Remote identifying as a tool will offer data regularly and at a costly value to allow right time for
crop states interference. Satellite assemblies provides a temporally and spatially lasting cover of
the world. The Moderate Resolution Imaging Spectroradiometer (MODIS) also well recognized
as an imaging appliance was initiated into the earth orbits through NASA. The tools capture
absorption by fluid water in the SWIR. Additionally, TVDI is attained from NDVI-spatial Land
Surface Temperature and be used as an indicator of moistness in the soil and thus the plant water
force. The Soil Adjusted Vegetation Index (SAVI) takes into account the optical soil features on
the vegetation cover reflectance. The WDI denotes the comparative speed of concealed heat
variations, so it shows a speed of “zero” for an utterly damp surface and a value of "one"
regarding desiccated surfaces where there is no unseen heat variation (Rahimzadeh-Bajgiran,
Omasa & Shimizu, 2012). The remote sensing approach supports to build a time-based growth
contour of crops over its advancement stage. With the retrieval of ecological elements in
addition to remote sensing directories, it is quick to acknowledge the growth framework and also
their link among each other and the effects of concerned variables on plant growth. Based on
preceding data and tests, the remote sensing approach is particularly crucial in projecting the
crop growth at a land level. The integration of the crop conditions entails ecological and surface.
However, remote sensing, in addition to the soil area, supports the advancement of the model to
compute the crop conditions (Rahimzadeh-Bajgiran, Omasa & Shimizu, 2012).
Remote detecting roles in Crop Condition Assessment
Remote detecting offers a vast point to get a normal synoptic image of the earth. Many classes of
choosy info on accessible capitals such as topsoil usage, soil humidity, crop natures and state,
and soil types info can be extracted from the satellite broadcasting data (Pohl & Van Genderen,
2016).
Remote identifying as a tool will offer data regularly and at a costly value to allow right time for
crop states interference. Satellite assemblies provides a temporally and spatially lasting cover of
the world. The Moderate Resolution Imaging Spectroradiometer (MODIS) also well recognized
as an imaging appliance was initiated into the earth orbits through NASA. The tools capture
Paraphrase This Document
Need a fresh take? Get an instant paraphrase of this document with our AI Paraphraser
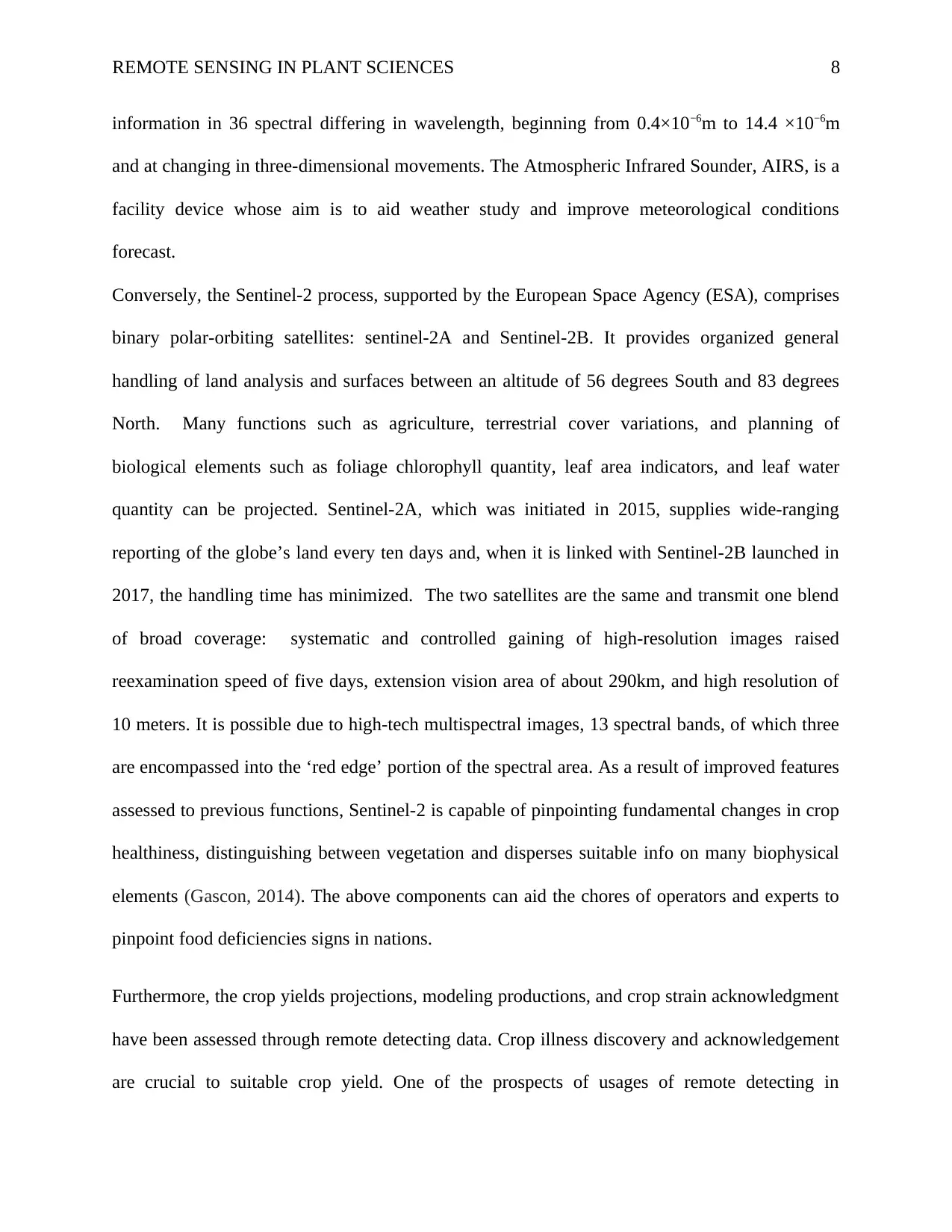
REMOTE SENSING IN PLANT SCIENCES 8
information in 36 spectral differing in wavelength, beginning from 0.4×10−6m to 14.4 ×10−6m
and at changing in three-dimensional movements. The Atmospheric Infrared Sounder, AIRS, is a
facility device whose aim is to aid weather study and improve meteorological conditions
forecast.
Conversely, the Sentinel-2 process, supported by the European Space Agency (ESA), comprises
binary polar-orbiting satellites: sentinel-2A and Sentinel-2B. It provides organized general
handling of land analysis and surfaces between an altitude of 56 degrees South and 83 degrees
North. Many functions such as agriculture, terrestrial cover variations, and planning of
biological elements such as foliage chlorophyll quantity, leaf area indicators, and leaf water
quantity can be projected. Sentinel-2A, which was initiated in 2015, supplies wide-ranging
reporting of the globe’s land every ten days and, when it is linked with Sentinel-2B launched in
2017, the handling time has minimized. The two satellites are the same and transmit one blend
of broad coverage: systematic and controlled gaining of high-resolution images raised
reexamination speed of five days, extension vision area of about 290km, and high resolution of
10 meters. It is possible due to high-tech multispectral images, 13 spectral bands, of which three
are encompassed into the ‘red edge’ portion of the spectral area. As a result of improved features
assessed to previous functions, Sentinel-2 is capable of pinpointing fundamental changes in crop
healthiness, distinguishing between vegetation and disperses suitable info on many biophysical
elements (Gascon, 2014). The above components can aid the chores of operators and experts to
pinpoint food deficiencies signs in nations.
Furthermore, the crop yields projections, modeling productions, and crop strain acknowledgment
have been assessed through remote detecting data. Crop illness discovery and acknowledgement
are crucial to suitable crop yield. One of the prospects of usages of remote detecting in
information in 36 spectral differing in wavelength, beginning from 0.4×10−6m to 14.4 ×10−6m
and at changing in three-dimensional movements. The Atmospheric Infrared Sounder, AIRS, is a
facility device whose aim is to aid weather study and improve meteorological conditions
forecast.
Conversely, the Sentinel-2 process, supported by the European Space Agency (ESA), comprises
binary polar-orbiting satellites: sentinel-2A and Sentinel-2B. It provides organized general
handling of land analysis and surfaces between an altitude of 56 degrees South and 83 degrees
North. Many functions such as agriculture, terrestrial cover variations, and planning of
biological elements such as foliage chlorophyll quantity, leaf area indicators, and leaf water
quantity can be projected. Sentinel-2A, which was initiated in 2015, supplies wide-ranging
reporting of the globe’s land every ten days and, when it is linked with Sentinel-2B launched in
2017, the handling time has minimized. The two satellites are the same and transmit one blend
of broad coverage: systematic and controlled gaining of high-resolution images raised
reexamination speed of five days, extension vision area of about 290km, and high resolution of
10 meters. It is possible due to high-tech multispectral images, 13 spectral bands, of which three
are encompassed into the ‘red edge’ portion of the spectral area. As a result of improved features
assessed to previous functions, Sentinel-2 is capable of pinpointing fundamental changes in crop
healthiness, distinguishing between vegetation and disperses suitable info on many biophysical
elements (Gascon, 2014). The above components can aid the chores of operators and experts to
pinpoint food deficiencies signs in nations.
Furthermore, the crop yields projections, modeling productions, and crop strain acknowledgment
have been assessed through remote detecting data. Crop illness discovery and acknowledgement
are crucial to suitable crop yield. One of the prospects of usages of remote detecting in
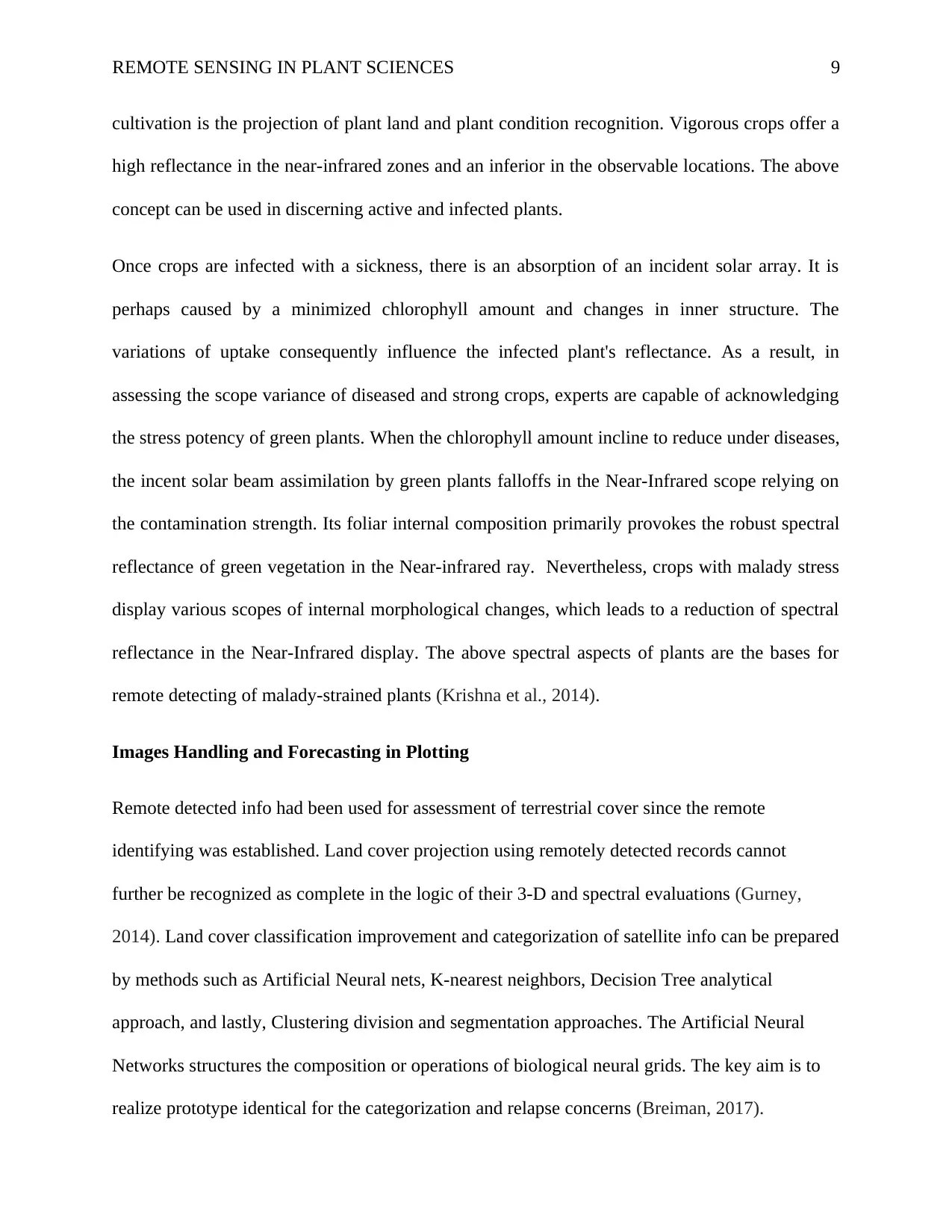
REMOTE SENSING IN PLANT SCIENCES 9
cultivation is the projection of plant land and plant condition recognition. Vigorous crops offer a
high reflectance in the near-infrared zones and an inferior in the observable locations. The above
concept can be used in discerning active and infected plants.
Once crops are infected with a sickness, there is an absorption of an incident solar array. It is
perhaps caused by a minimized chlorophyll amount and changes in inner structure. The
variations of uptake consequently influence the infected plant's reflectance. As a result, in
assessing the scope variance of diseased and strong crops, experts are capable of acknowledging
the stress potency of green plants. When the chlorophyll amount incline to reduce under diseases,
the incent solar beam assimilation by green plants falloffs in the Near-Infrared scope relying on
the contamination strength. Its foliar internal composition primarily provokes the robust spectral
reflectance of green vegetation in the Near-infrared ray. Nevertheless, crops with malady stress
display various scopes of internal morphological changes, which leads to a reduction of spectral
reflectance in the Near-Infrared display. The above spectral aspects of plants are the bases for
remote detecting of malady-strained plants (Krishna et al., 2014).
Images Handling and Forecasting in Plotting
Remote detected info had been used for assessment of terrestrial cover since the remote
identifying was established. Land cover projection using remotely detected records cannot
further be recognized as complete in the logic of their 3-D and spectral evaluations (Gurney,
2014). Land cover classification improvement and categorization of satellite info can be prepared
by methods such as Artificial Neural nets, K-nearest neighbors, Decision Tree analytical
approach, and lastly, Clustering division and segmentation approaches. The Artificial Neural
Networks structures the composition or operations of biological neural grids. The key aim is to
realize prototype identical for the categorization and relapse concerns (Breiman, 2017).
cultivation is the projection of plant land and plant condition recognition. Vigorous crops offer a
high reflectance in the near-infrared zones and an inferior in the observable locations. The above
concept can be used in discerning active and infected plants.
Once crops are infected with a sickness, there is an absorption of an incident solar array. It is
perhaps caused by a minimized chlorophyll amount and changes in inner structure. The
variations of uptake consequently influence the infected plant's reflectance. As a result, in
assessing the scope variance of diseased and strong crops, experts are capable of acknowledging
the stress potency of green plants. When the chlorophyll amount incline to reduce under diseases,
the incent solar beam assimilation by green plants falloffs in the Near-Infrared scope relying on
the contamination strength. Its foliar internal composition primarily provokes the robust spectral
reflectance of green vegetation in the Near-infrared ray. Nevertheless, crops with malady stress
display various scopes of internal morphological changes, which leads to a reduction of spectral
reflectance in the Near-Infrared display. The above spectral aspects of plants are the bases for
remote detecting of malady-strained plants (Krishna et al., 2014).
Images Handling and Forecasting in Plotting
Remote detected info had been used for assessment of terrestrial cover since the remote
identifying was established. Land cover projection using remotely detected records cannot
further be recognized as complete in the logic of their 3-D and spectral evaluations (Gurney,
2014). Land cover classification improvement and categorization of satellite info can be prepared
by methods such as Artificial Neural nets, K-nearest neighbors, Decision Tree analytical
approach, and lastly, Clustering division and segmentation approaches. The Artificial Neural
Networks structures the composition or operations of biological neural grids. The key aim is to
realize prototype identical for the categorization and relapse concerns (Breiman, 2017).
You're viewing a preview
Unlock full access by subscribing today!
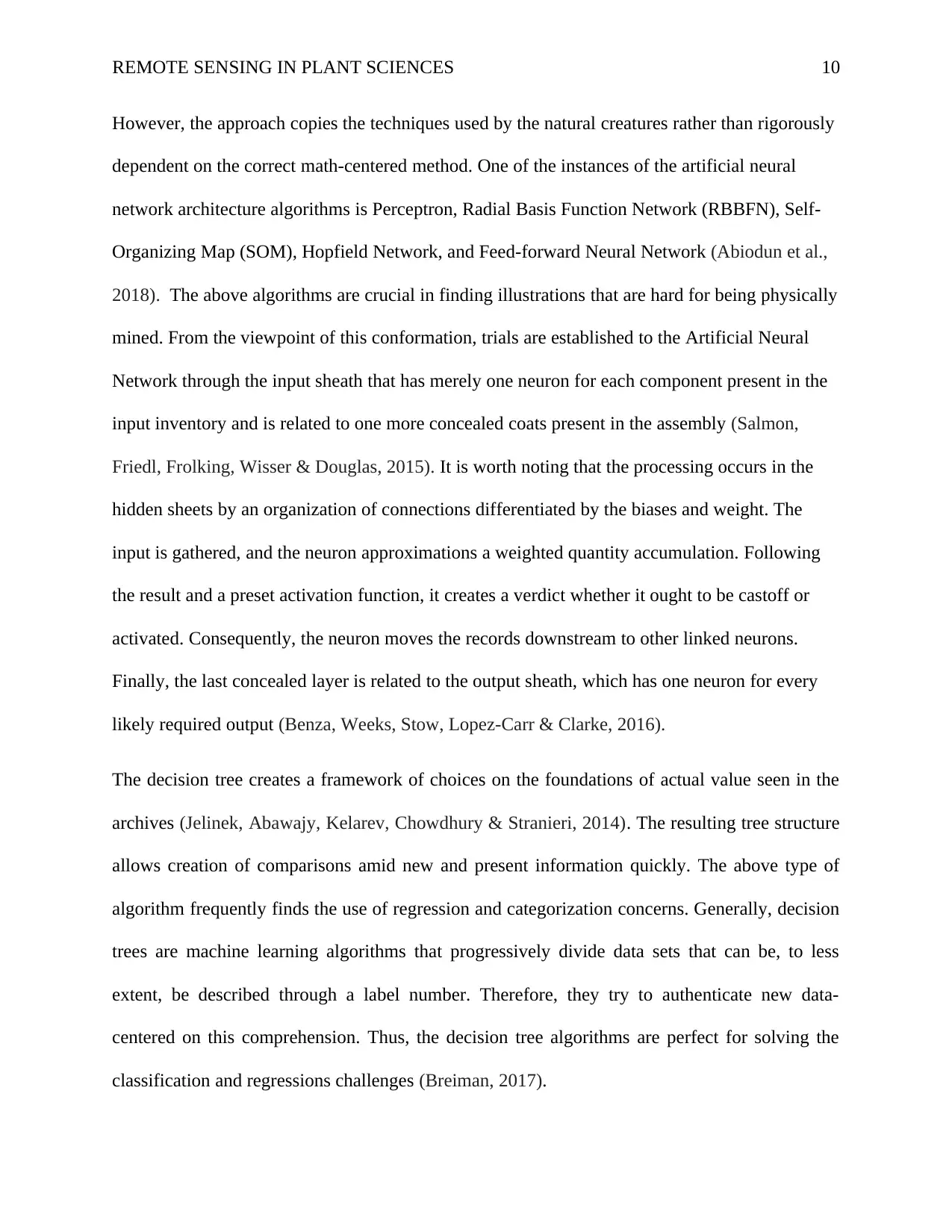
REMOTE SENSING IN PLANT SCIENCES 10
However, the approach copies the techniques used by the natural creatures rather than rigorously
dependent on the correct math-centered method. One of the instances of the artificial neural
network architecture algorithms is Perceptron, Radial Basis Function Network (RBBFN), Self-
Organizing Map (SOM), Hopfield Network, and Feed-forward Neural Network (Abiodun et al.,
2018). The above algorithms are crucial in finding illustrations that are hard for being physically
mined. From the viewpoint of this conformation, trials are established to the Artificial Neural
Network through the input sheath that has merely one neuron for each component present in the
input inventory and is related to one more concealed coats present in the assembly (Salmon,
Friedl, Frolking, Wisser & Douglas, 2015). It is worth noting that the processing occurs in the
hidden sheets by an organization of connections differentiated by the biases and weight. The
input is gathered, and the neuron approximations a weighted quantity accumulation. Following
the result and a preset activation function, it creates a verdict whether it ought to be castoff or
activated. Consequently, the neuron moves the records downstream to other linked neurons.
Finally, the last concealed layer is related to the output sheath, which has one neuron for every
likely required output (Benza, Weeks, Stow, Lopez-Carr & Clarke, 2016).
The decision tree creates a framework of choices on the foundations of actual value seen in the
archives (Jelinek, Abawajy, Kelarev, Chowdhury & Stranieri, 2014). The resulting tree structure
allows creation of comparisons amid new and present information quickly. The above type of
algorithm frequently finds the use of regression and categorization concerns. Generally, decision
trees are machine learning algorithms that progressively divide data sets that can be, to less
extent, be described through a label number. Therefore, they try to authenticate new data-
centered on this comprehension. Thus, the decision tree algorithms are perfect for solving the
classification and regressions challenges (Breiman, 2017).
However, the approach copies the techniques used by the natural creatures rather than rigorously
dependent on the correct math-centered method. One of the instances of the artificial neural
network architecture algorithms is Perceptron, Radial Basis Function Network (RBBFN), Self-
Organizing Map (SOM), Hopfield Network, and Feed-forward Neural Network (Abiodun et al.,
2018). The above algorithms are crucial in finding illustrations that are hard for being physically
mined. From the viewpoint of this conformation, trials are established to the Artificial Neural
Network through the input sheath that has merely one neuron for each component present in the
input inventory and is related to one more concealed coats present in the assembly (Salmon,
Friedl, Frolking, Wisser & Douglas, 2015). It is worth noting that the processing occurs in the
hidden sheets by an organization of connections differentiated by the biases and weight. The
input is gathered, and the neuron approximations a weighted quantity accumulation. Following
the result and a preset activation function, it creates a verdict whether it ought to be castoff or
activated. Consequently, the neuron moves the records downstream to other linked neurons.
Finally, the last concealed layer is related to the output sheath, which has one neuron for every
likely required output (Benza, Weeks, Stow, Lopez-Carr & Clarke, 2016).
The decision tree creates a framework of choices on the foundations of actual value seen in the
archives (Jelinek, Abawajy, Kelarev, Chowdhury & Stranieri, 2014). The resulting tree structure
allows creation of comparisons amid new and present information quickly. The above type of
algorithm frequently finds the use of regression and categorization concerns. Generally, decision
trees are machine learning algorithms that progressively divide data sets that can be, to less
extent, be described through a label number. Therefore, they try to authenticate new data-
centered on this comprehension. Thus, the decision tree algorithms are perfect for solving the
classification and regressions challenges (Breiman, 2017).
Paraphrase This Document
Need a fresh take? Get an instant paraphrase of this document with our AI Paraphraser
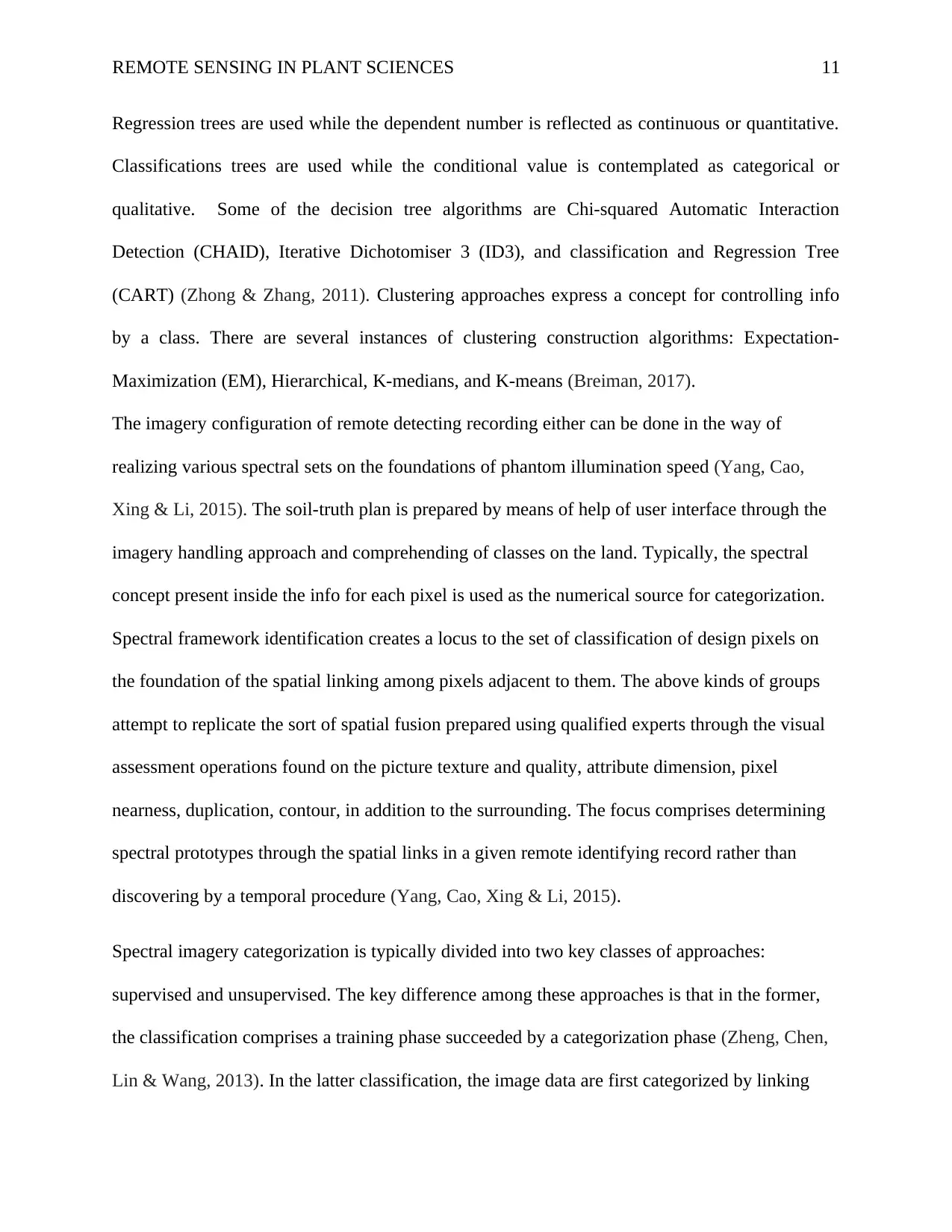
REMOTE SENSING IN PLANT SCIENCES 11
Regression trees are used while the dependent number is reflected as continuous or quantitative.
Classifications trees are used while the conditional value is contemplated as categorical or
qualitative. Some of the decision tree algorithms are Chi-squared Automatic Interaction
Detection (CHAID), Iterative Dichotomiser 3 (ID3), and classification and Regression Tree
(CART) (Zhong & Zhang, 2011). Clustering approaches express a concept for controlling info
by a class. There are several instances of clustering construction algorithms: Expectation-
Maximization (EM), Hierarchical, K-medians, and K-means (Breiman, 2017).
The imagery configuration of remote detecting recording either can be done in the way of
realizing various spectral sets on the foundations of phantom illumination speed (Yang, Cao,
Xing & Li, 2015). The soil-truth plan is prepared by means of help of user interface through the
imagery handling approach and comprehending of classes on the land. Typically, the spectral
concept present inside the info for each pixel is used as the numerical source for categorization.
Spectral framework identification creates a locus to the set of classification of design pixels on
the foundation of the spatial linking among pixels adjacent to them. The above kinds of groups
attempt to replicate the sort of spatial fusion prepared using qualified experts through the visual
assessment operations found on the picture texture and quality, attribute dimension, pixel
nearness, duplication, contour, in addition to the surrounding. The focus comprises determining
spectral prototypes through the spatial links in a given remote identifying record rather than
discovering by a temporal procedure (Yang, Cao, Xing & Li, 2015).
Spectral imagery categorization is typically divided into two key classes of approaches:
supervised and unsupervised. The key difference among these approaches is that in the former,
the classification comprises a training phase succeeded by a categorization phase (Zheng, Chen,
Lin & Wang, 2013). In the latter classification, the image data are first categorized by linking
Regression trees are used while the dependent number is reflected as continuous or quantitative.
Classifications trees are used while the conditional value is contemplated as categorical or
qualitative. Some of the decision tree algorithms are Chi-squared Automatic Interaction
Detection (CHAID), Iterative Dichotomiser 3 (ID3), and classification and Regression Tree
(CART) (Zhong & Zhang, 2011). Clustering approaches express a concept for controlling info
by a class. There are several instances of clustering construction algorithms: Expectation-
Maximization (EM), Hierarchical, K-medians, and K-means (Breiman, 2017).
The imagery configuration of remote detecting recording either can be done in the way of
realizing various spectral sets on the foundations of phantom illumination speed (Yang, Cao,
Xing & Li, 2015). The soil-truth plan is prepared by means of help of user interface through the
imagery handling approach and comprehending of classes on the land. Typically, the spectral
concept present inside the info for each pixel is used as the numerical source for categorization.
Spectral framework identification creates a locus to the set of classification of design pixels on
the foundation of the spatial linking among pixels adjacent to them. The above kinds of groups
attempt to replicate the sort of spatial fusion prepared using qualified experts through the visual
assessment operations found on the picture texture and quality, attribute dimension, pixel
nearness, duplication, contour, in addition to the surrounding. The focus comprises determining
spectral prototypes through the spatial links in a given remote identifying record rather than
discovering by a temporal procedure (Yang, Cao, Xing & Li, 2015).
Spectral imagery categorization is typically divided into two key classes of approaches:
supervised and unsupervised. The key difference among these approaches is that in the former,
the classification comprises a training phase succeeded by a categorization phase (Zheng, Chen,
Lin & Wang, 2013). In the latter classification, the image data are first categorized by linking
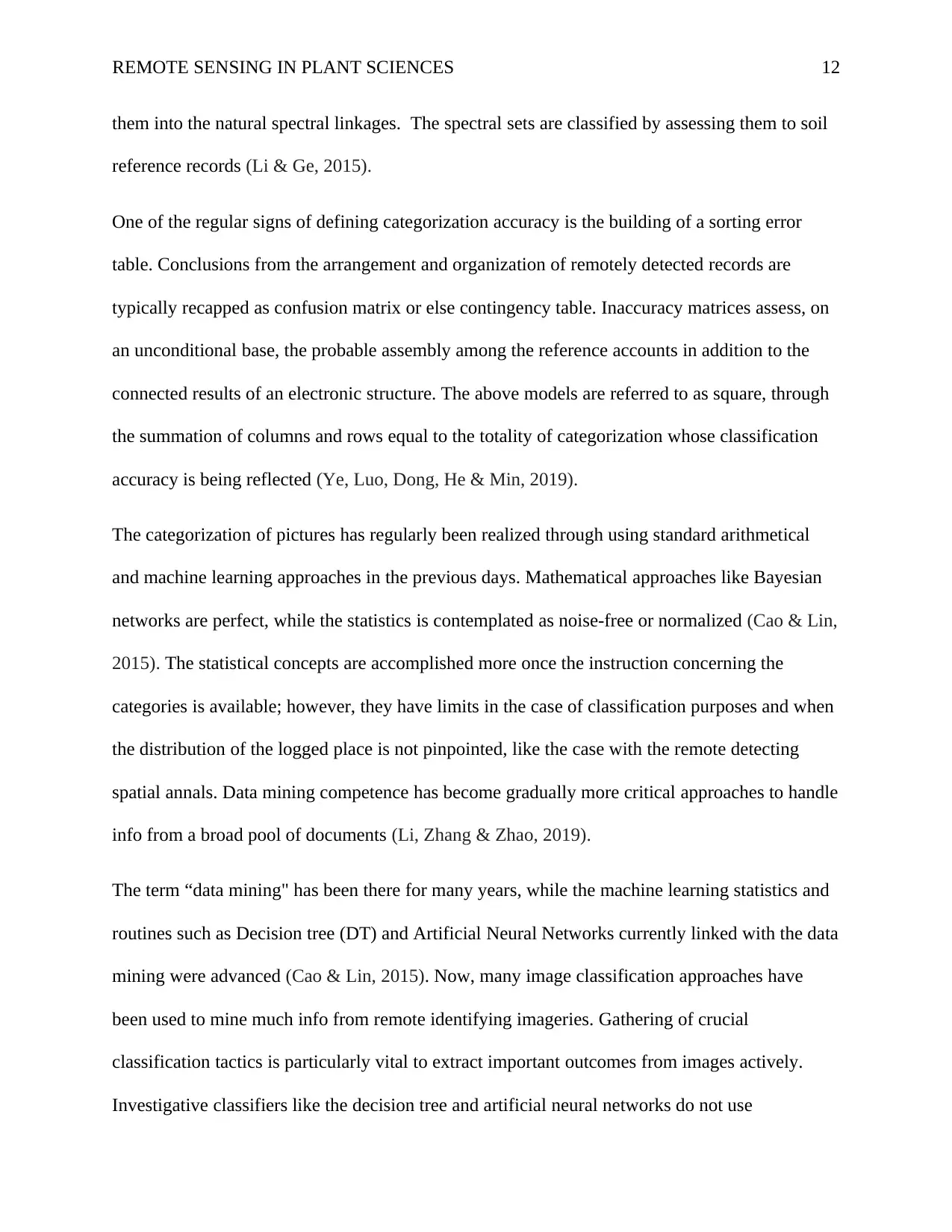
REMOTE SENSING IN PLANT SCIENCES 12
them into the natural spectral linkages. The spectral sets are classified by assessing them to soil
reference records (Li & Ge, 2015).
One of the regular signs of defining categorization accuracy is the building of a sorting error
table. Conclusions from the arrangement and organization of remotely detected records are
typically recapped as confusion matrix or else contingency table. Inaccuracy matrices assess, on
an unconditional base, the probable assembly among the reference accounts in addition to the
connected results of an electronic structure. The above models are referred to as square, through
the summation of columns and rows equal to the totality of categorization whose classification
accuracy is being reflected (Ye, Luo, Dong, He & Min, 2019).
The categorization of pictures has regularly been realized through using standard arithmetical
and machine learning approaches in the previous days. Mathematical approaches like Bayesian
networks are perfect, while the statistics is contemplated as noise-free or normalized (Cao & Lin,
2015). The statistical concepts are accomplished more once the instruction concerning the
categories is available; however, they have limits in the case of classification purposes and when
the distribution of the logged place is not pinpointed, like the case with the remote detecting
spatial annals. Data mining competence has become gradually more critical approaches to handle
info from a broad pool of documents (Li, Zhang & Zhao, 2019).
The term “data mining" has been there for many years, while the machine learning statistics and
routines such as Decision tree (DT) and Artificial Neural Networks currently linked with the data
mining were advanced (Cao & Lin, 2015). Now, many image classification approaches have
been used to mine much info from remote identifying imageries. Gathering of crucial
classification tactics is particularly vital to extract important outcomes from images actively.
Investigative classifiers like the decision tree and artificial neural networks do not use
them into the natural spectral linkages. The spectral sets are classified by assessing them to soil
reference records (Li & Ge, 2015).
One of the regular signs of defining categorization accuracy is the building of a sorting error
table. Conclusions from the arrangement and organization of remotely detected records are
typically recapped as confusion matrix or else contingency table. Inaccuracy matrices assess, on
an unconditional base, the probable assembly among the reference accounts in addition to the
connected results of an electronic structure. The above models are referred to as square, through
the summation of columns and rows equal to the totality of categorization whose classification
accuracy is being reflected (Ye, Luo, Dong, He & Min, 2019).
The categorization of pictures has regularly been realized through using standard arithmetical
and machine learning approaches in the previous days. Mathematical approaches like Bayesian
networks are perfect, while the statistics is contemplated as noise-free or normalized (Cao & Lin,
2015). The statistical concepts are accomplished more once the instruction concerning the
categories is available; however, they have limits in the case of classification purposes and when
the distribution of the logged place is not pinpointed, like the case with the remote detecting
spatial annals. Data mining competence has become gradually more critical approaches to handle
info from a broad pool of documents (Li, Zhang & Zhao, 2019).
The term “data mining" has been there for many years, while the machine learning statistics and
routines such as Decision tree (DT) and Artificial Neural Networks currently linked with the data
mining were advanced (Cao & Lin, 2015). Now, many image classification approaches have
been used to mine much info from remote identifying imageries. Gathering of crucial
classification tactics is particularly vital to extract important outcomes from images actively.
Investigative classifiers like the decision tree and artificial neural networks do not use
You're viewing a preview
Unlock full access by subscribing today!
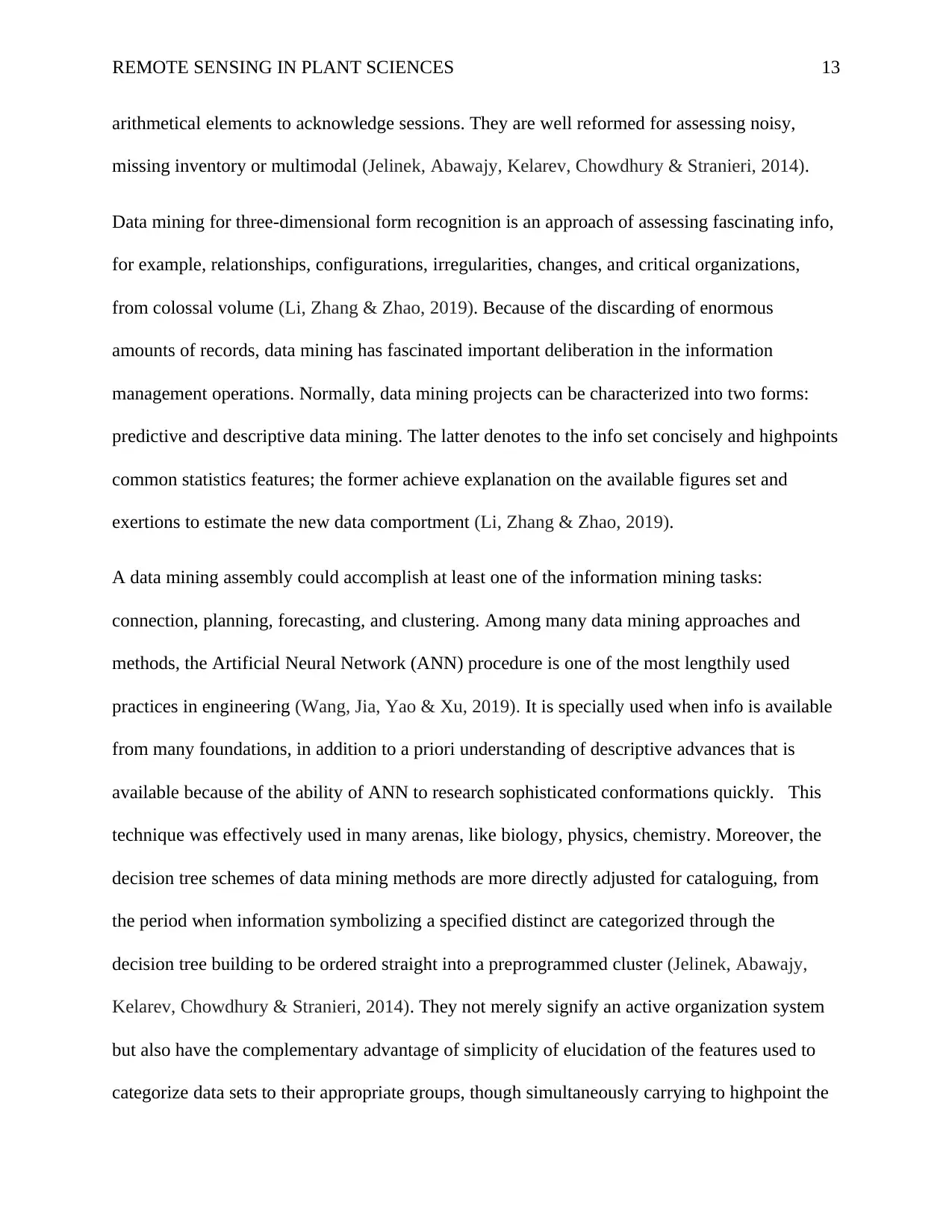
REMOTE SENSING IN PLANT SCIENCES 13
arithmetical elements to acknowledge sessions. They are well reformed for assessing noisy,
missing inventory or multimodal (Jelinek, Abawajy, Kelarev, Chowdhury & Stranieri, 2014).
Data mining for three-dimensional form recognition is an approach of assessing fascinating info,
for example, relationships, configurations, irregularities, changes, and critical organizations,
from colossal volume (Li, Zhang & Zhao, 2019). Because of the discarding of enormous
amounts of records, data mining has fascinated important deliberation in the information
management operations. Normally, data mining projects can be characterized into two forms:
predictive and descriptive data mining. The latter denotes to the info set concisely and highpoints
common statistics features; the former achieve explanation on the available figures set and
exertions to estimate the new data comportment (Li, Zhang & Zhao, 2019).
A data mining assembly could accomplish at least one of the information mining tasks:
connection, planning, forecasting, and clustering. Among many data mining approaches and
methods, the Artificial Neural Network (ANN) procedure is one of the most lengthily used
practices in engineering (Wang, Jia, Yao & Xu, 2019). It is specially used when info is available
from many foundations, in addition to a priori understanding of descriptive advances that is
available because of the ability of ANN to research sophisticated conformations quickly. This
technique was effectively used in many arenas, like biology, physics, chemistry. Moreover, the
decision tree schemes of data mining methods are more directly adjusted for cataloguing, from
the period when information symbolizing a specified distinct are categorized through the
decision tree building to be ordered straight into a preprogrammed cluster (Jelinek, Abawajy,
Kelarev, Chowdhury & Stranieri, 2014). They not merely signify an active organization system
but also have the complementary advantage of simplicity of elucidation of the features used to
categorize data sets to their appropriate groups, though simultaneously carrying to highpoint the
arithmetical elements to acknowledge sessions. They are well reformed for assessing noisy,
missing inventory or multimodal (Jelinek, Abawajy, Kelarev, Chowdhury & Stranieri, 2014).
Data mining for three-dimensional form recognition is an approach of assessing fascinating info,
for example, relationships, configurations, irregularities, changes, and critical organizations,
from colossal volume (Li, Zhang & Zhao, 2019). Because of the discarding of enormous
amounts of records, data mining has fascinated important deliberation in the information
management operations. Normally, data mining projects can be characterized into two forms:
predictive and descriptive data mining. The latter denotes to the info set concisely and highpoints
common statistics features; the former achieve explanation on the available figures set and
exertions to estimate the new data comportment (Li, Zhang & Zhao, 2019).
A data mining assembly could accomplish at least one of the information mining tasks:
connection, planning, forecasting, and clustering. Among many data mining approaches and
methods, the Artificial Neural Network (ANN) procedure is one of the most lengthily used
practices in engineering (Wang, Jia, Yao & Xu, 2019). It is specially used when info is available
from many foundations, in addition to a priori understanding of descriptive advances that is
available because of the ability of ANN to research sophisticated conformations quickly. This
technique was effectively used in many arenas, like biology, physics, chemistry. Moreover, the
decision tree schemes of data mining methods are more directly adjusted for cataloguing, from
the period when information symbolizing a specified distinct are categorized through the
decision tree building to be ordered straight into a preprogrammed cluster (Jelinek, Abawajy,
Kelarev, Chowdhury & Stranieri, 2014). They not merely signify an active organization system
but also have the complementary advantage of simplicity of elucidation of the features used to
categorize data sets to their appropriate groups, though simultaneously carrying to highpoint the
Paraphrase This Document
Need a fresh take? Get an instant paraphrase of this document with our AI Paraphraser
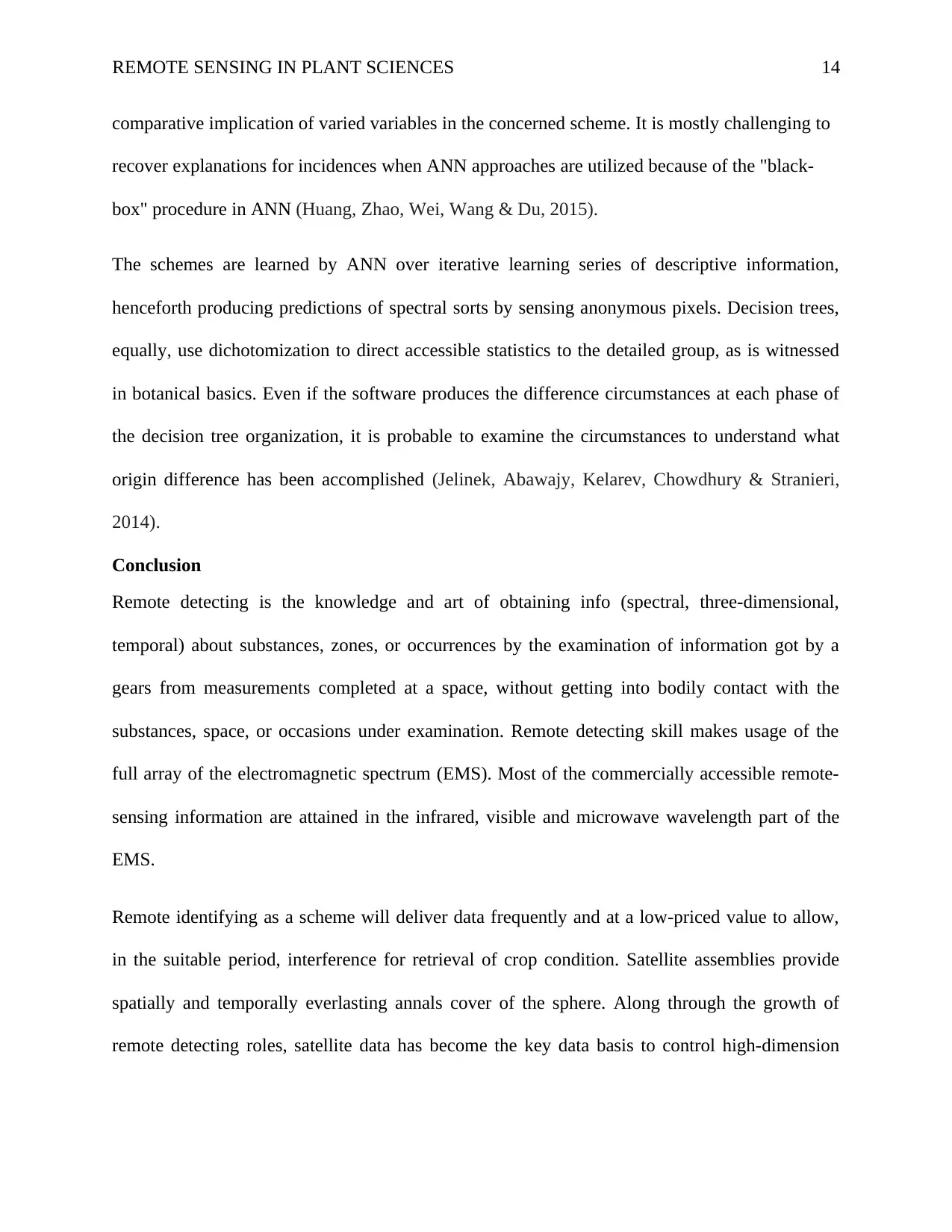
REMOTE SENSING IN PLANT SCIENCES 14
comparative implication of varied variables in the concerned scheme. It is mostly challenging to
recover explanations for incidences when ANN approaches are utilized because of the "black-
box" procedure in ANN (Huang, Zhao, Wei, Wang & Du, 2015).
The schemes are learned by ANN over iterative learning series of descriptive information,
henceforth producing predictions of spectral sorts by sensing anonymous pixels. Decision trees,
equally, use dichotomization to direct accessible statistics to the detailed group, as is witnessed
in botanical basics. Even if the software produces the difference circumstances at each phase of
the decision tree organization, it is probable to examine the circumstances to understand what
origin difference has been accomplished (Jelinek, Abawajy, Kelarev, Chowdhury & Stranieri,
2014).
Conclusion
Remote detecting is the knowledge and art of obtaining info (spectral, three-dimensional,
temporal) about substances, zones, or occurrences by the examination of information got by a
gears from measurements completed at a space, without getting into bodily contact with the
substances, space, or occasions under examination. Remote detecting skill makes usage of the
full array of the electromagnetic spectrum (EMS). Most of the commercially accessible remote-
sensing information are attained in the infrared, visible and microwave wavelength part of the
EMS.
Remote identifying as a scheme will deliver data frequently and at a low-priced value to allow,
in the suitable period, interference for retrieval of crop condition. Satellite assemblies provide
spatially and temporally everlasting annals cover of the sphere. Along through the growth of
remote detecting roles, satellite data has become the key data basis to control high-dimension
comparative implication of varied variables in the concerned scheme. It is mostly challenging to
recover explanations for incidences when ANN approaches are utilized because of the "black-
box" procedure in ANN (Huang, Zhao, Wei, Wang & Du, 2015).
The schemes are learned by ANN over iterative learning series of descriptive information,
henceforth producing predictions of spectral sorts by sensing anonymous pixels. Decision trees,
equally, use dichotomization to direct accessible statistics to the detailed group, as is witnessed
in botanical basics. Even if the software produces the difference circumstances at each phase of
the decision tree organization, it is probable to examine the circumstances to understand what
origin difference has been accomplished (Jelinek, Abawajy, Kelarev, Chowdhury & Stranieri,
2014).
Conclusion
Remote detecting is the knowledge and art of obtaining info (spectral, three-dimensional,
temporal) about substances, zones, or occurrences by the examination of information got by a
gears from measurements completed at a space, without getting into bodily contact with the
substances, space, or occasions under examination. Remote detecting skill makes usage of the
full array of the electromagnetic spectrum (EMS). Most of the commercially accessible remote-
sensing information are attained in the infrared, visible and microwave wavelength part of the
EMS.
Remote identifying as a scheme will deliver data frequently and at a low-priced value to allow,
in the suitable period, interference for retrieval of crop condition. Satellite assemblies provide
spatially and temporally everlasting annals cover of the sphere. Along through the growth of
remote detecting roles, satellite data has become the key data basis to control high-dimension
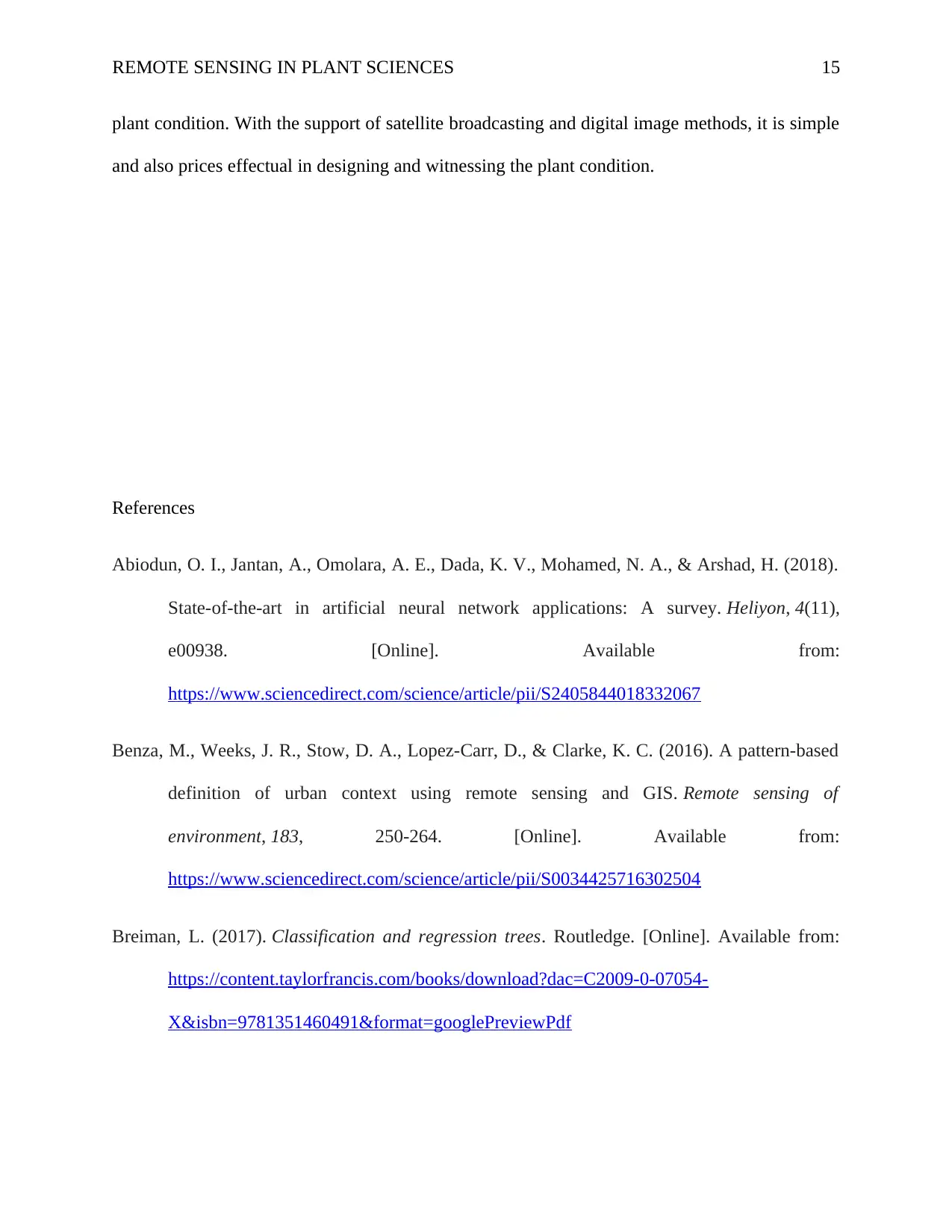
REMOTE SENSING IN PLANT SCIENCES 15
plant condition. With the support of satellite broadcasting and digital image methods, it is simple
and also prices effectual in designing and witnessing the plant condition.
References
Abiodun, O. I., Jantan, A., Omolara, A. E., Dada, K. V., Mohamed, N. A., & Arshad, H. (2018).
State-of-the-art in artificial neural network applications: A survey. Heliyon, 4(11),
e00938. [Online]. Available from:
https://www.sciencedirect.com/science/article/pii/S2405844018332067
Benza, M., Weeks, J. R., Stow, D. A., Lopez-Carr, D., & Clarke, K. C. (2016). A pattern-based
definition of urban context using remote sensing and GIS. Remote sensing of
environment, 183, 250-264. [Online]. Available from:
https://www.sciencedirect.com/science/article/pii/S0034425716302504
Breiman, L. (2017). Classification and regression trees. Routledge. [Online]. Available from:
https://content.taylorfrancis.com/books/download?dac=C2009-0-07054-
X&isbn=9781351460491&format=googlePreviewPdf
plant condition. With the support of satellite broadcasting and digital image methods, it is simple
and also prices effectual in designing and witnessing the plant condition.
References
Abiodun, O. I., Jantan, A., Omolara, A. E., Dada, K. V., Mohamed, N. A., & Arshad, H. (2018).
State-of-the-art in artificial neural network applications: A survey. Heliyon, 4(11),
e00938. [Online]. Available from:
https://www.sciencedirect.com/science/article/pii/S2405844018332067
Benza, M., Weeks, J. R., Stow, D. A., Lopez-Carr, D., & Clarke, K. C. (2016). A pattern-based
definition of urban context using remote sensing and GIS. Remote sensing of
environment, 183, 250-264. [Online]. Available from:
https://www.sciencedirect.com/science/article/pii/S0034425716302504
Breiman, L. (2017). Classification and regression trees. Routledge. [Online]. Available from:
https://content.taylorfrancis.com/books/download?dac=C2009-0-07054-
X&isbn=9781351460491&format=googlePreviewPdf
You're viewing a preview
Unlock full access by subscribing today!
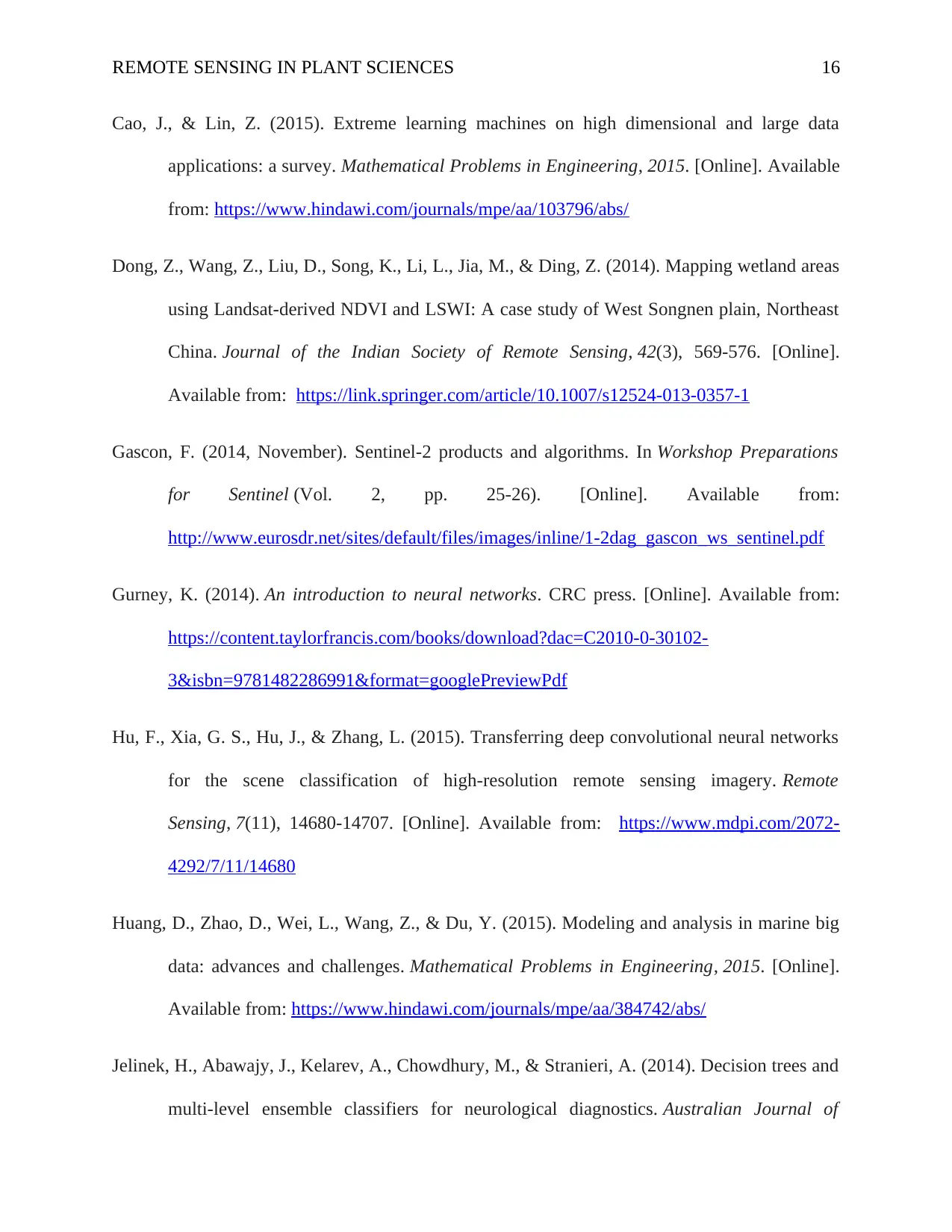
REMOTE SENSING IN PLANT SCIENCES 16
Cao, J., & Lin, Z. (2015). Extreme learning machines on high dimensional and large data
applications: a survey. Mathematical Problems in Engineering, 2015. [Online]. Available
from: https://www.hindawi.com/journals/mpe/aa/103796/abs/
Dong, Z., Wang, Z., Liu, D., Song, K., Li, L., Jia, M., & Ding, Z. (2014). Mapping wetland areas
using Landsat-derived NDVI and LSWI: A case study of West Songnen plain, Northeast
China. Journal of the Indian Society of Remote Sensing, 42(3), 569-576. [Online].
Available from: https://link.springer.com/article/10.1007/s12524-013-0357-1
Gascon, F. (2014, November). Sentinel-2 products and algorithms. In Workshop Preparations
for Sentinel (Vol. 2, pp. 25-26). [Online]. Available from:
http://www.eurosdr.net/sites/default/files/images/inline/1-2dag_gascon_ws_sentinel.pdf
Gurney, K. (2014). An introduction to neural networks. CRC press. [Online]. Available from:
https://content.taylorfrancis.com/books/download?dac=C2010-0-30102-
3&isbn=9781482286991&format=googlePreviewPdf
Hu, F., Xia, G. S., Hu, J., & Zhang, L. (2015). Transferring deep convolutional neural networks
for the scene classification of high-resolution remote sensing imagery. Remote
Sensing, 7(11), 14680-14707. [Online]. Available from: https://www.mdpi.com/2072-
4292/7/11/14680
Huang, D., Zhao, D., Wei, L., Wang, Z., & Du, Y. (2015). Modeling and analysis in marine big
data: advances and challenges. Mathematical Problems in Engineering, 2015. [Online].
Available from: https://www.hindawi.com/journals/mpe/aa/384742/abs/
Jelinek, H., Abawajy, J., Kelarev, A., Chowdhury, M., & Stranieri, A. (2014). Decision trees and
multi-level ensemble classifiers for neurological diagnostics. Australian Journal of
Cao, J., & Lin, Z. (2015). Extreme learning machines on high dimensional and large data
applications: a survey. Mathematical Problems in Engineering, 2015. [Online]. Available
from: https://www.hindawi.com/journals/mpe/aa/103796/abs/
Dong, Z., Wang, Z., Liu, D., Song, K., Li, L., Jia, M., & Ding, Z. (2014). Mapping wetland areas
using Landsat-derived NDVI and LSWI: A case study of West Songnen plain, Northeast
China. Journal of the Indian Society of Remote Sensing, 42(3), 569-576. [Online].
Available from: https://link.springer.com/article/10.1007/s12524-013-0357-1
Gascon, F. (2014, November). Sentinel-2 products and algorithms. In Workshop Preparations
for Sentinel (Vol. 2, pp. 25-26). [Online]. Available from:
http://www.eurosdr.net/sites/default/files/images/inline/1-2dag_gascon_ws_sentinel.pdf
Gurney, K. (2014). An introduction to neural networks. CRC press. [Online]. Available from:
https://content.taylorfrancis.com/books/download?dac=C2010-0-30102-
3&isbn=9781482286991&format=googlePreviewPdf
Hu, F., Xia, G. S., Hu, J., & Zhang, L. (2015). Transferring deep convolutional neural networks
for the scene classification of high-resolution remote sensing imagery. Remote
Sensing, 7(11), 14680-14707. [Online]. Available from: https://www.mdpi.com/2072-
4292/7/11/14680
Huang, D., Zhao, D., Wei, L., Wang, Z., & Du, Y. (2015). Modeling and analysis in marine big
data: advances and challenges. Mathematical Problems in Engineering, 2015. [Online].
Available from: https://www.hindawi.com/journals/mpe/aa/384742/abs/
Jelinek, H., Abawajy, J., Kelarev, A., Chowdhury, M., & Stranieri, A. (2014). Decision trees and
multi-level ensemble classifiers for neurological diagnostics. Australian Journal of
Paraphrase This Document
Need a fresh take? Get an instant paraphrase of this document with our AI Paraphraser
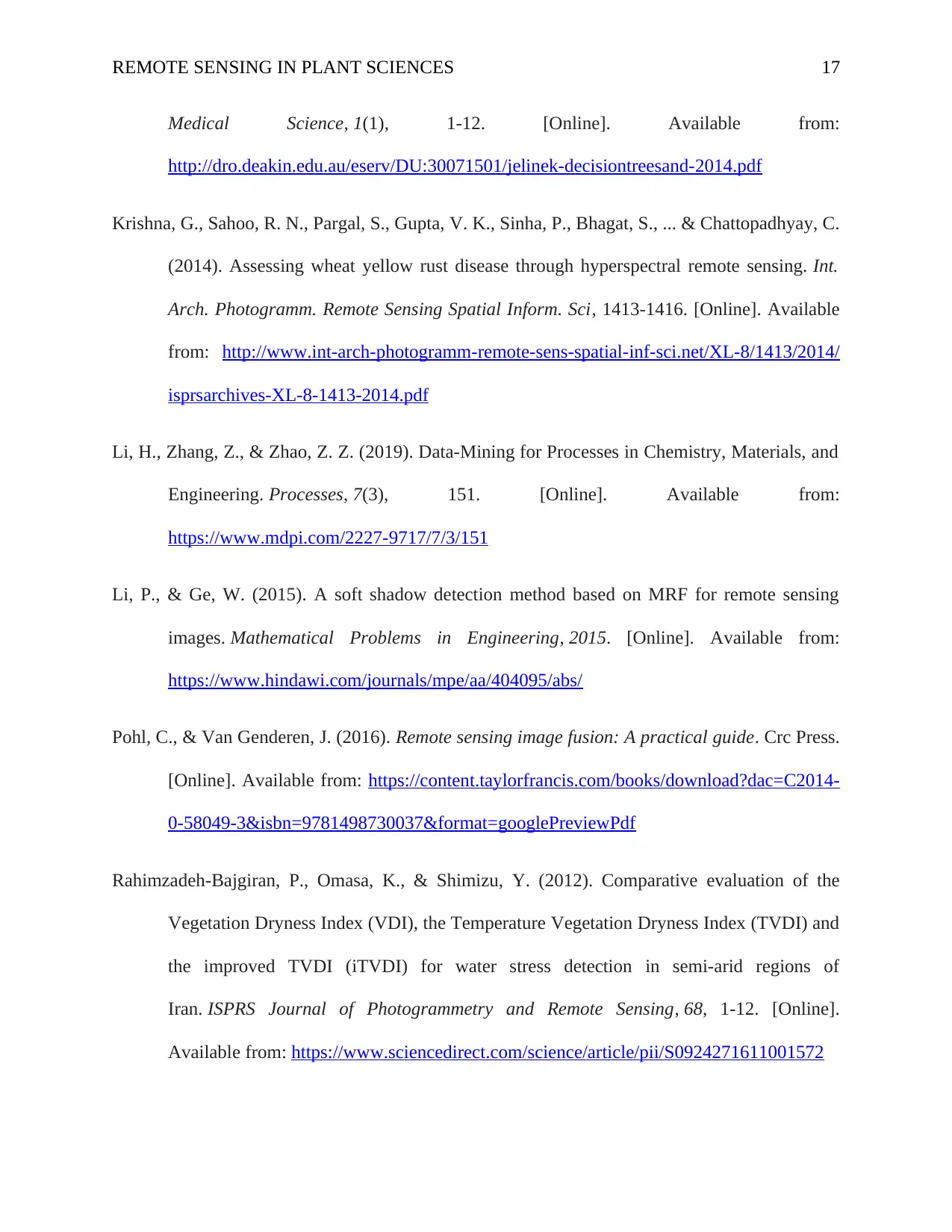
REMOTE SENSING IN PLANT SCIENCES 17
Medical Science, 1(1), 1-12. [Online]. Available from:
http://dro.deakin.edu.au/eserv/DU:30071501/jelinek-decisiontreesand-2014.pdf
Krishna, G., Sahoo, R. N., Pargal, S., Gupta, V. K., Sinha, P., Bhagat, S., ... & Chattopadhyay, C.
(2014). Assessing wheat yellow rust disease through hyperspectral remote sensing. Int.
Arch. Photogramm. Remote Sensing Spatial Inform. Sci, 1413-1416. [Online]. Available
from: http://www.int-arch-photogramm-remote-sens-spatial-inf-sci.net/XL-8/1413/2014/
isprsarchives-XL-8-1413-2014.pdf
Li, H., Zhang, Z., & Zhao, Z. Z. (2019). Data-Mining for Processes in Chemistry, Materials, and
Engineering. Processes, 7(3), 151. [Online]. Available from:
https://www.mdpi.com/2227-9717/7/3/151
Li, P., & Ge, W. (2015). A soft shadow detection method based on MRF for remote sensing
images. Mathematical Problems in Engineering, 2015. [Online]. Available from:
https://www.hindawi.com/journals/mpe/aa/404095/abs/
Pohl, C., & Van Genderen, J. (2016). Remote sensing image fusion: A practical guide. Crc Press.
[Online]. Available from: https://content.taylorfrancis.com/books/download?dac=C2014-
0-58049-3&isbn=9781498730037&format=googlePreviewPdf
Rahimzadeh-Bajgiran, P., Omasa, K., & Shimizu, Y. (2012). Comparative evaluation of the
Vegetation Dryness Index (VDI), the Temperature Vegetation Dryness Index (TVDI) and
the improved TVDI (iTVDI) for water stress detection in semi-arid regions of
Iran. ISPRS Journal of Photogrammetry and Remote Sensing, 68, 1-12. [Online].
Available from: https://www.sciencedirect.com/science/article/pii/S0924271611001572
Medical Science, 1(1), 1-12. [Online]. Available from:
http://dro.deakin.edu.au/eserv/DU:30071501/jelinek-decisiontreesand-2014.pdf
Krishna, G., Sahoo, R. N., Pargal, S., Gupta, V. K., Sinha, P., Bhagat, S., ... & Chattopadhyay, C.
(2014). Assessing wheat yellow rust disease through hyperspectral remote sensing. Int.
Arch. Photogramm. Remote Sensing Spatial Inform. Sci, 1413-1416. [Online]. Available
from: http://www.int-arch-photogramm-remote-sens-spatial-inf-sci.net/XL-8/1413/2014/
isprsarchives-XL-8-1413-2014.pdf
Li, H., Zhang, Z., & Zhao, Z. Z. (2019). Data-Mining for Processes in Chemistry, Materials, and
Engineering. Processes, 7(3), 151. [Online]. Available from:
https://www.mdpi.com/2227-9717/7/3/151
Li, P., & Ge, W. (2015). A soft shadow detection method based on MRF for remote sensing
images. Mathematical Problems in Engineering, 2015. [Online]. Available from:
https://www.hindawi.com/journals/mpe/aa/404095/abs/
Pohl, C., & Van Genderen, J. (2016). Remote sensing image fusion: A practical guide. Crc Press.
[Online]. Available from: https://content.taylorfrancis.com/books/download?dac=C2014-
0-58049-3&isbn=9781498730037&format=googlePreviewPdf
Rahimzadeh-Bajgiran, P., Omasa, K., & Shimizu, Y. (2012). Comparative evaluation of the
Vegetation Dryness Index (VDI), the Temperature Vegetation Dryness Index (TVDI) and
the improved TVDI (iTVDI) for water stress detection in semi-arid regions of
Iran. ISPRS Journal of Photogrammetry and Remote Sensing, 68, 1-12. [Online].
Available from: https://www.sciencedirect.com/science/article/pii/S0924271611001572
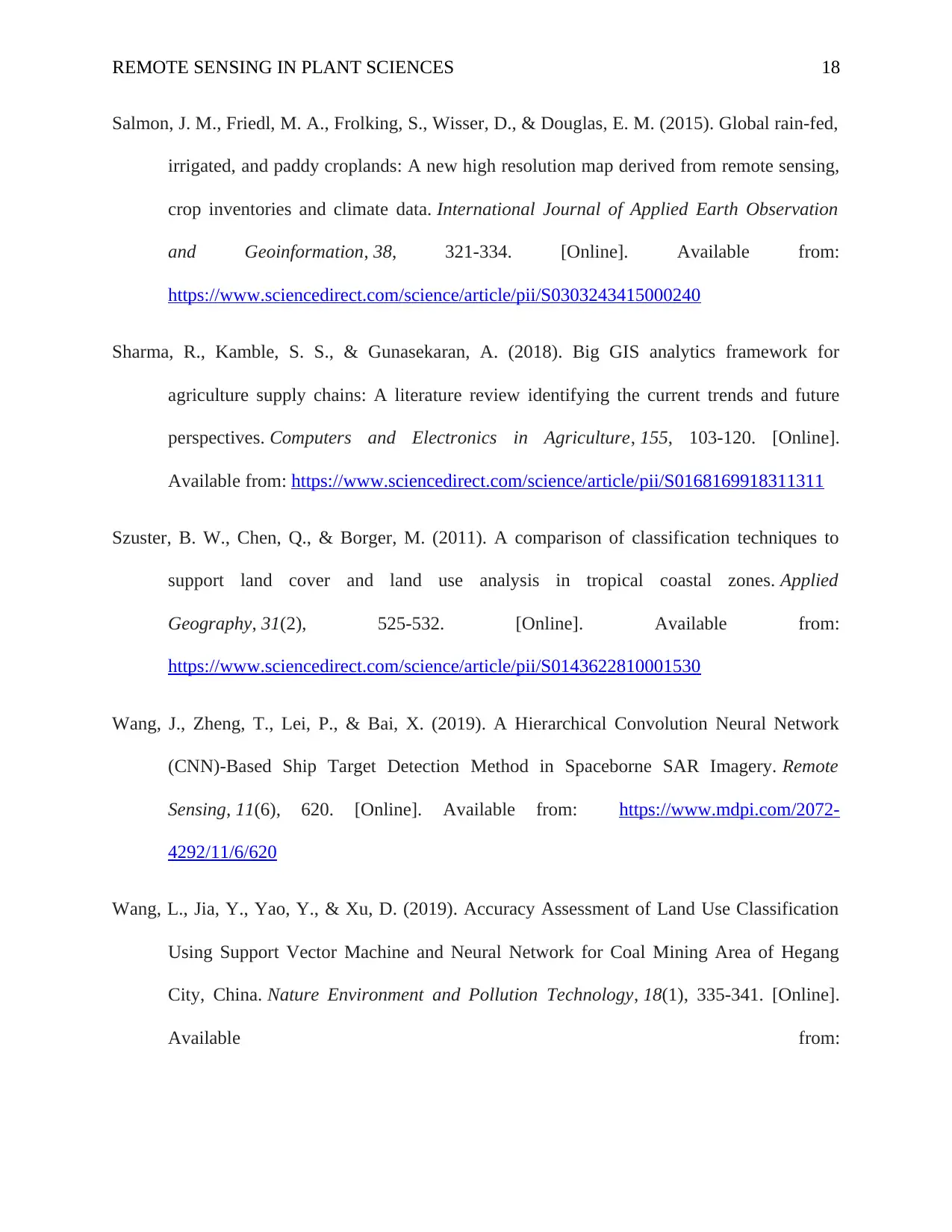
REMOTE SENSING IN PLANT SCIENCES 18
Salmon, J. M., Friedl, M. A., Frolking, S., Wisser, D., & Douglas, E. M. (2015). Global rain-fed,
irrigated, and paddy croplands: A new high resolution map derived from remote sensing,
crop inventories and climate data. International Journal of Applied Earth Observation
and Geoinformation, 38, 321-334. [Online]. Available from:
https://www.sciencedirect.com/science/article/pii/S0303243415000240
Sharma, R., Kamble, S. S., & Gunasekaran, A. (2018). Big GIS analytics framework for
agriculture supply chains: A literature review identifying the current trends and future
perspectives. Computers and Electronics in Agriculture, 155, 103-120. [Online].
Available from: https://www.sciencedirect.com/science/article/pii/S0168169918311311
Szuster, B. W., Chen, Q., & Borger, M. (2011). A comparison of classification techniques to
support land cover and land use analysis in tropical coastal zones. Applied
Geography, 31(2), 525-532. [Online]. Available from:
https://www.sciencedirect.com/science/article/pii/S0143622810001530
Wang, J., Zheng, T., Lei, P., & Bai, X. (2019). A Hierarchical Convolution Neural Network
(CNN)-Based Ship Target Detection Method in Spaceborne SAR Imagery. Remote
Sensing, 11(6), 620. [Online]. Available from: https://www.mdpi.com/2072-
4292/11/6/620
Wang, L., Jia, Y., Yao, Y., & Xu, D. (2019). Accuracy Assessment of Land Use Classification
Using Support Vector Machine and Neural Network for Coal Mining Area of Hegang
City, China. Nature Environment and Pollution Technology, 18(1), 335-341. [Online].
Available from:
Salmon, J. M., Friedl, M. A., Frolking, S., Wisser, D., & Douglas, E. M. (2015). Global rain-fed,
irrigated, and paddy croplands: A new high resolution map derived from remote sensing,
crop inventories and climate data. International Journal of Applied Earth Observation
and Geoinformation, 38, 321-334. [Online]. Available from:
https://www.sciencedirect.com/science/article/pii/S0303243415000240
Sharma, R., Kamble, S. S., & Gunasekaran, A. (2018). Big GIS analytics framework for
agriculture supply chains: A literature review identifying the current trends and future
perspectives. Computers and Electronics in Agriculture, 155, 103-120. [Online].
Available from: https://www.sciencedirect.com/science/article/pii/S0168169918311311
Szuster, B. W., Chen, Q., & Borger, M. (2011). A comparison of classification techniques to
support land cover and land use analysis in tropical coastal zones. Applied
Geography, 31(2), 525-532. [Online]. Available from:
https://www.sciencedirect.com/science/article/pii/S0143622810001530
Wang, J., Zheng, T., Lei, P., & Bai, X. (2019). A Hierarchical Convolution Neural Network
(CNN)-Based Ship Target Detection Method in Spaceborne SAR Imagery. Remote
Sensing, 11(6), 620. [Online]. Available from: https://www.mdpi.com/2072-
4292/11/6/620
Wang, L., Jia, Y., Yao, Y., & Xu, D. (2019). Accuracy Assessment of Land Use Classification
Using Support Vector Machine and Neural Network for Coal Mining Area of Hegang
City, China. Nature Environment and Pollution Technology, 18(1), 335-341. [Online].
Available from:
You're viewing a preview
Unlock full access by subscribing today!
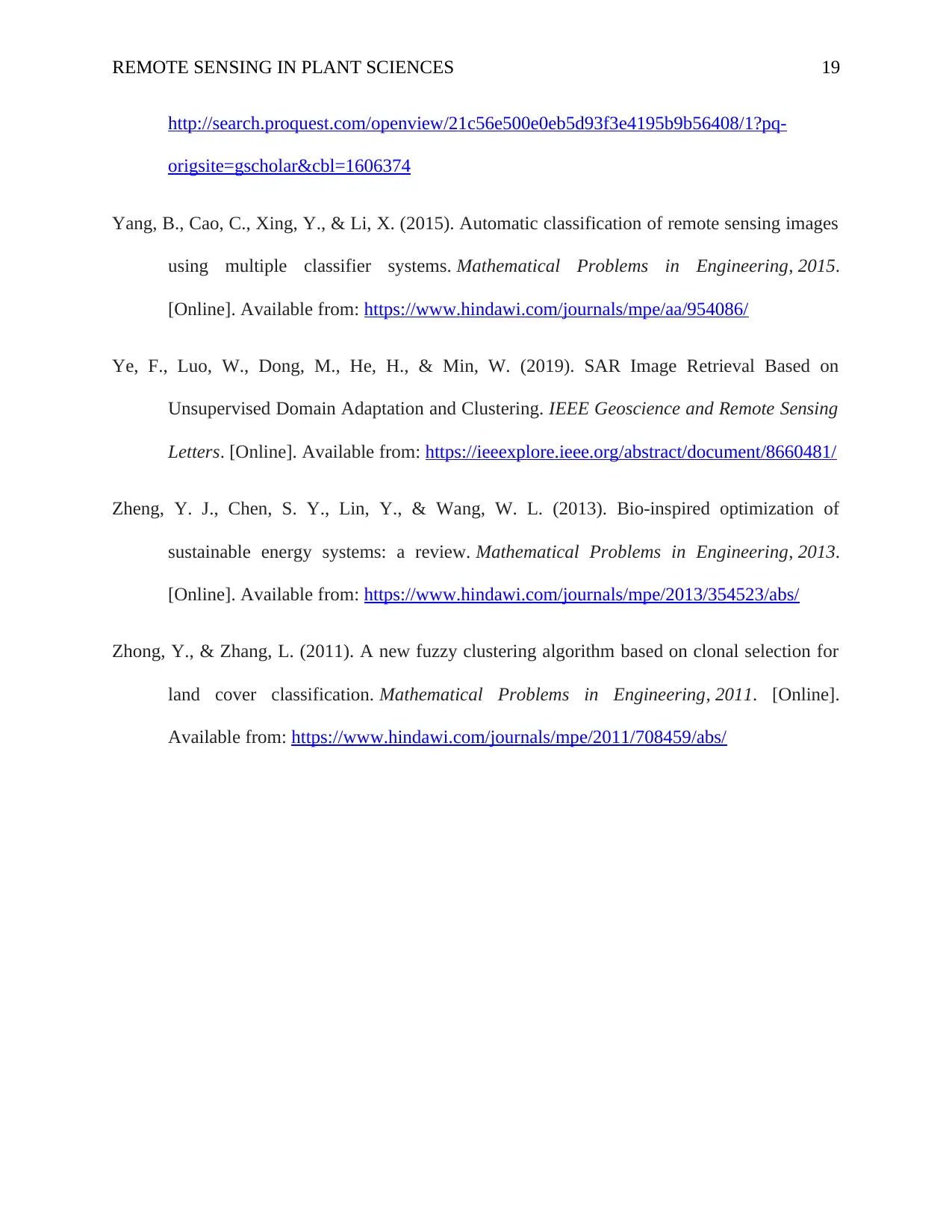
REMOTE SENSING IN PLANT SCIENCES 19
http://search.proquest.com/openview/21c56e500e0eb5d93f3e4195b9b56408/1?pq-
origsite=gscholar&cbl=1606374
Yang, B., Cao, C., Xing, Y., & Li, X. (2015). Automatic classification of remote sensing images
using multiple classifier systems. Mathematical Problems in Engineering, 2015.
[Online]. Available from: https://www.hindawi.com/journals/mpe/aa/954086/
Ye, F., Luo, W., Dong, M., He, H., & Min, W. (2019). SAR Image Retrieval Based on
Unsupervised Domain Adaptation and Clustering. IEEE Geoscience and Remote Sensing
Letters. [Online]. Available from: https://ieeexplore.ieee.org/abstract/document/8660481/
Zheng, Y. J., Chen, S. Y., Lin, Y., & Wang, W. L. (2013). Bio-inspired optimization of
sustainable energy systems: a review. Mathematical Problems in Engineering, 2013.
[Online]. Available from: https://www.hindawi.com/journals/mpe/2013/354523/abs/
Zhong, Y., & Zhang, L. (2011). A new fuzzy clustering algorithm based on clonal selection for
land cover classification. Mathematical Problems in Engineering, 2011. [Online].
Available from: https://www.hindawi.com/journals/mpe/2011/708459/abs/
http://search.proquest.com/openview/21c56e500e0eb5d93f3e4195b9b56408/1?pq-
origsite=gscholar&cbl=1606374
Yang, B., Cao, C., Xing, Y., & Li, X. (2015). Automatic classification of remote sensing images
using multiple classifier systems. Mathematical Problems in Engineering, 2015.
[Online]. Available from: https://www.hindawi.com/journals/mpe/aa/954086/
Ye, F., Luo, W., Dong, M., He, H., & Min, W. (2019). SAR Image Retrieval Based on
Unsupervised Domain Adaptation and Clustering. IEEE Geoscience and Remote Sensing
Letters. [Online]. Available from: https://ieeexplore.ieee.org/abstract/document/8660481/
Zheng, Y. J., Chen, S. Y., Lin, Y., & Wang, W. L. (2013). Bio-inspired optimization of
sustainable energy systems: a review. Mathematical Problems in Engineering, 2013.
[Online]. Available from: https://www.hindawi.com/journals/mpe/2013/354523/abs/
Zhong, Y., & Zhang, L. (2011). A new fuzzy clustering algorithm based on clonal selection for
land cover classification. Mathematical Problems in Engineering, 2011. [Online].
Available from: https://www.hindawi.com/journals/mpe/2011/708459/abs/
1 out of 19

Your All-in-One AI-Powered Toolkit for Academic Success.
+13062052269
info@desklib.com
Available 24*7 on WhatsApp / Email
Unlock your academic potential
© 2024 | Zucol Services PVT LTD | All rights reserved.