MSc Project: Should We Trust Z-Score: UK Private Companies Analysis
VerifiedAdded on 2023/01/11
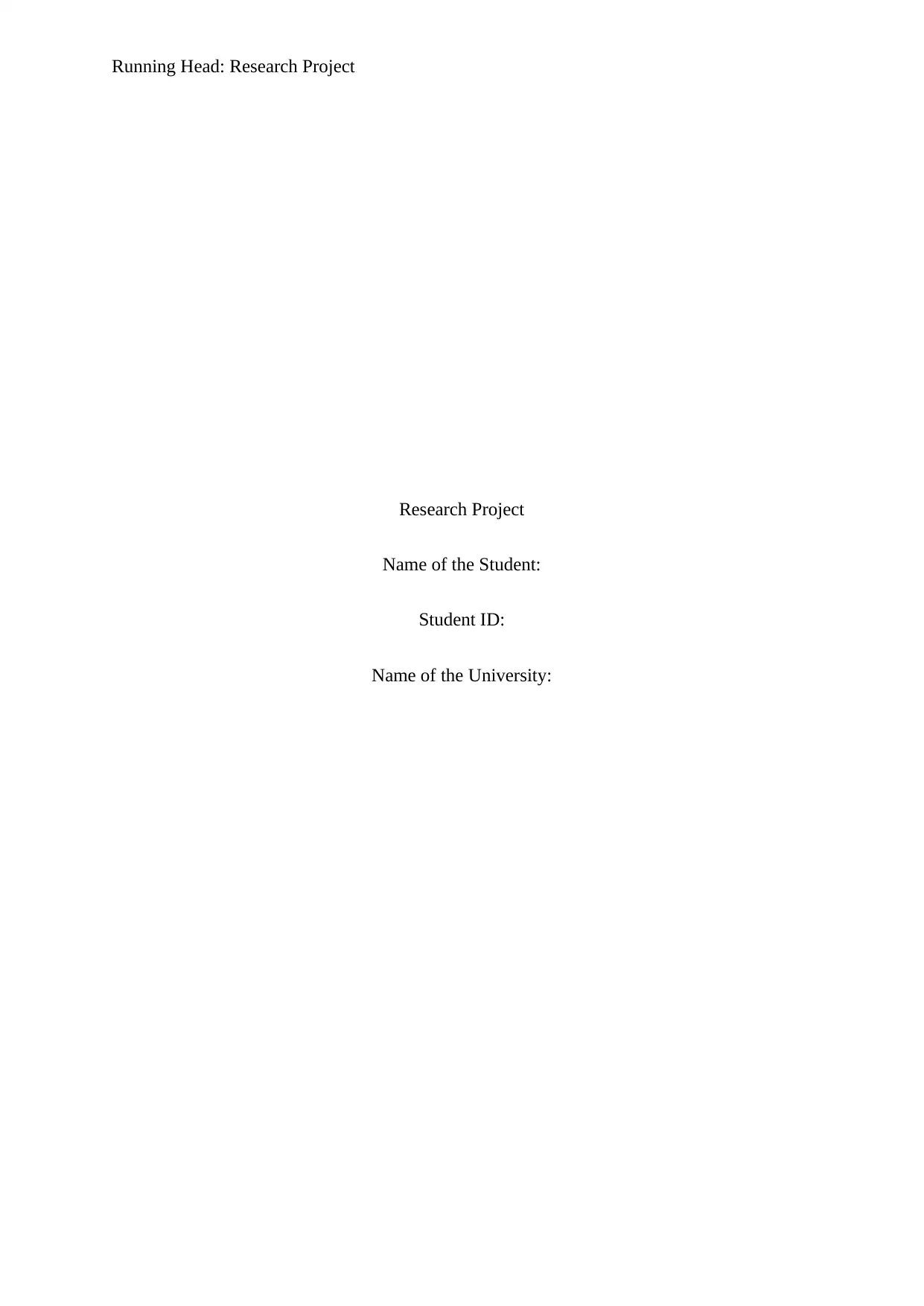
Research Project
Name of the Student:
Student ID:
Name of the University:
Paraphrase This Document
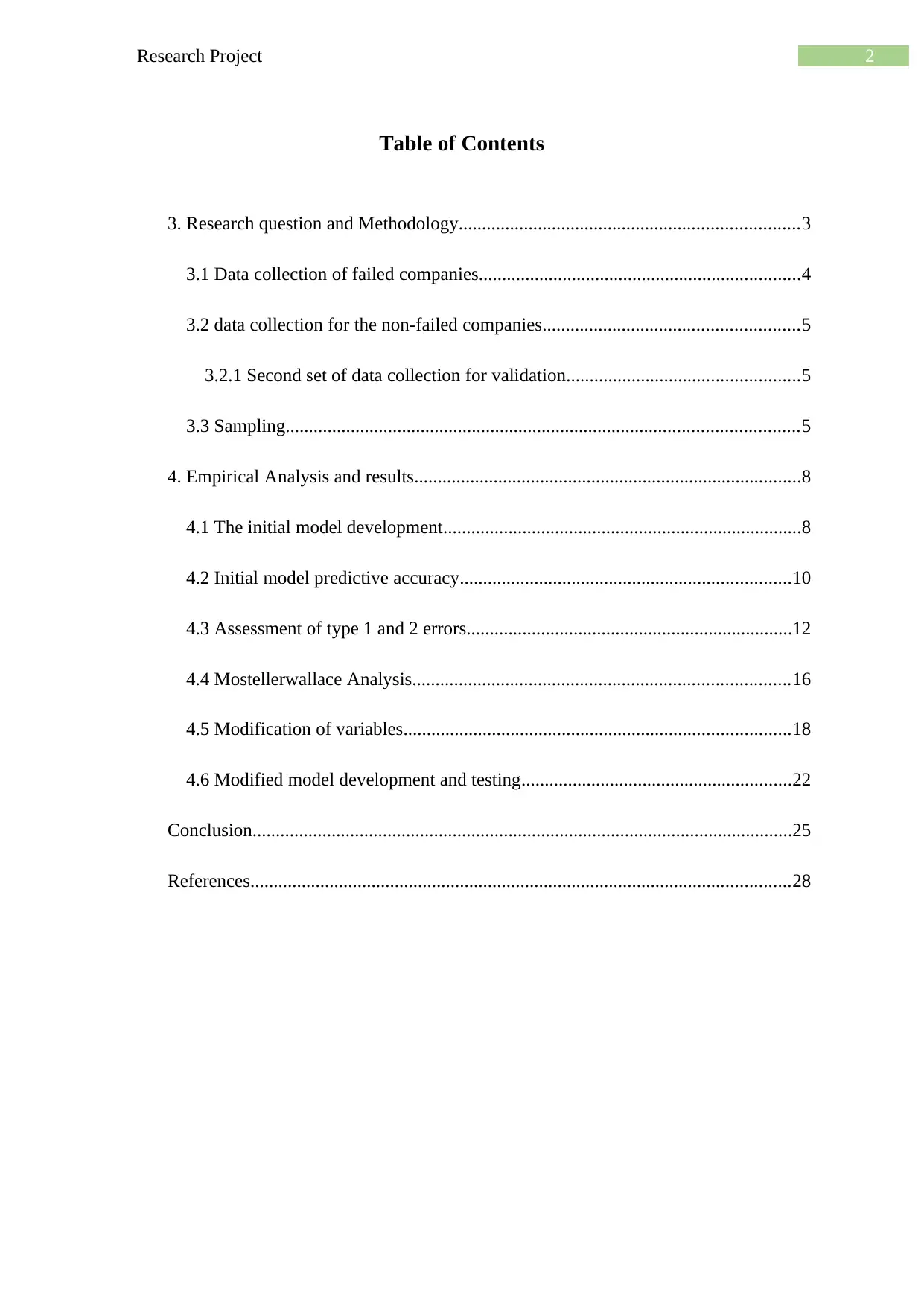
Table of Contents
3. Research question and Methodology.........................................................................3
3.1 Data collection of failed companies.....................................................................4
3.2 data collection for the non-failed companies.......................................................5
3.2.1 Second set of data collection for validation..................................................5
3.3 Sampling..............................................................................................................5
4. Empirical Analysis and results...................................................................................8
4.1 The initial model development.............................................................................8
4.2 Initial model predictive accuracy.......................................................................10
4.3 Assessment of type 1 and 2 errors......................................................................12
4.4 Mostellerwallace Analysis.................................................................................16
4.5 Modification of variables...................................................................................18
4.6 Modified model development and testing..........................................................22
Conclusion....................................................................................................................25
References....................................................................................................................28
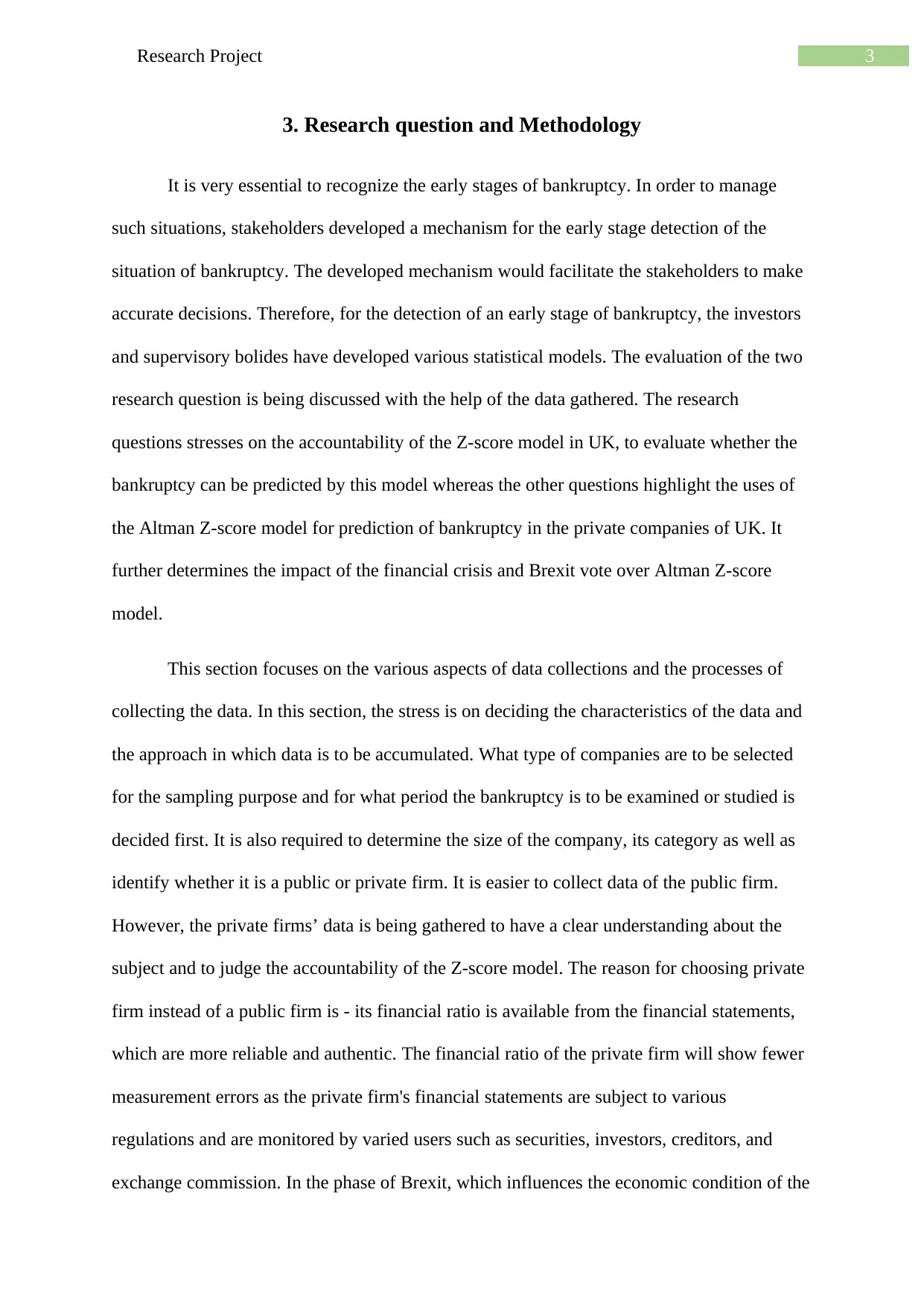
3. Research question and Methodology
It is very essential to recognize the early stages of bankruptcy. In order to manage
such situations, stakeholders developed a mechanism for the early stage detection of the
situation of bankruptcy. The developed mechanism would facilitate the stakeholders to make
accurate decisions. Therefore, for the detection of an early stage of bankruptcy, the investors
and supervisory bolides have developed various statistical models. The evaluation of the two
research question is being discussed with the help of the data gathered. The research
questions stresses on the accountability of the Z-score model in UK, to evaluate whether the
bankruptcy can be predicted by this model whereas the other questions highlight the uses of
the Altman Z-score model for prediction of bankruptcy in the private companies of UK. It
further determines the impact of the financial crisis and Brexit vote over Altman Z-score
model.
This section focuses on the various aspects of data collections and the processes of
collecting the data. In this section, the stress is on deciding the characteristics of the data and
the approach in which data is to be accumulated. What type of companies are to be selected
for the sampling purpose and for what period the bankruptcy is to be examined or studied is
decided first. It is also required to determine the size of the company, its category as well as
identify whether it is a public or private firm. It is easier to collect data of the public firm.
However, the private firms’ data is being gathered to have a clear understanding about the
subject and to judge the accountability of the Z-score model. The reason for choosing private
firm instead of a public firm is - its financial ratio is available from the financial statements,
which are more reliable and authentic. The financial ratio of the private firm will show fewer
measurement errors as the private firm's financial statements are subject to various
regulations and are monitored by varied users such as securities, investors, creditors, and
exchange commission. In the phase of Brexit, which influences the economic condition of the
⊘ This is a preview!⊘
Do you want full access?
Subscribe today to unlock all pages.

Trusted by 1+ million students worldwide
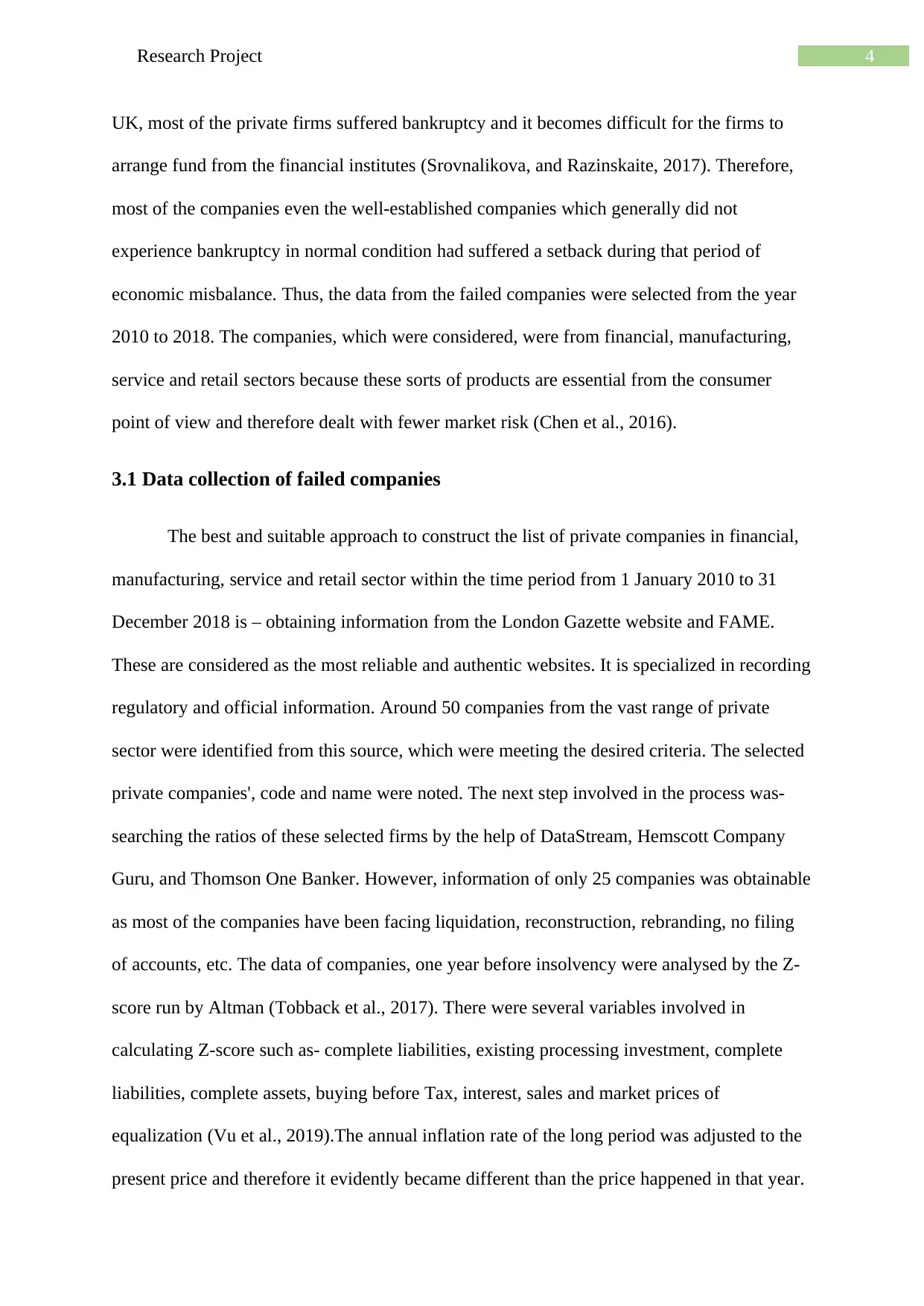
UK, most of the private firms suffered bankruptcy and it becomes difficult for the firms to
arrange fund from the financial institutes (Srovnalikova, and Razinskaite, 2017). Therefore,
most of the companies even the well-established companies which generally did not
experience bankruptcy in normal condition had suffered a setback during that period of
economic misbalance. Thus, the data from the failed companies were selected from the year
2010 to 2018. The companies, which were considered, were from financial, manufacturing,
service and retail sectors because these sorts of products are essential from the consumer
point of view and therefore dealt with fewer market risk (Chen et al., 2016).
3.1 Data collection of failed companies
The best and suitable approach to construct the list of private companies in financial,
manufacturing, service and retail sector within the time period from 1 January 2010 to 31
December 2018 is – obtaining information from the London Gazette website and FAME.
These are considered as the most reliable and authentic websites. It is specialized in recording
regulatory and official information. Around 50 companies from the vast range of private
sector were identified from this source, which were meeting the desired criteria. The selected
private companies', code and name were noted. The next step involved in the process was-
searching the ratios of these selected firms by the help of DataStream, Hemscott Company
Guru, and Thomson One Banker. However, information of only 25 companies was obtainable
as most of the companies have been facing liquidation, reconstruction, rebranding, no filing
of accounts, etc. The data of companies, one year before insolvency were analysed by the Z-
score run by Altman (Tobback et al., 2017). There were several variables involved in
calculating Z-score such as- complete liabilities, existing processing investment, complete
liabilities, complete assets, buying before Tax, interest, sales and market prices of
equalization (Vu et al., 2019).The annual inflation rate of the long period was adjusted to the
present price and therefore it evidently became different than the price happened in that year.
Paraphrase This Document
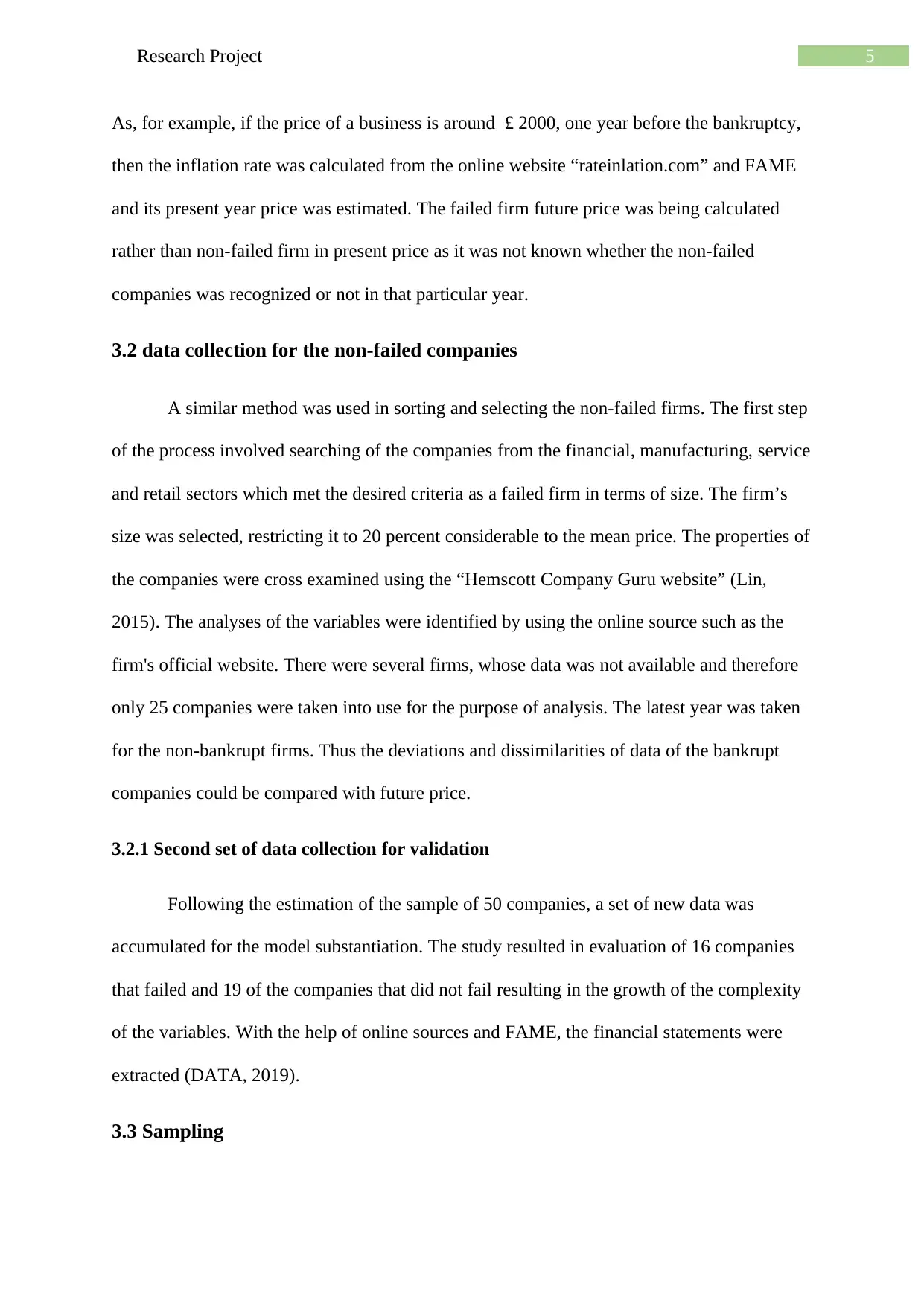
As, for example, if the price of a business is around £ 2000, one year before the bankruptcy,
then the inflation rate was calculated from the online website “rateinlation.com” and FAME
and its present year price was estimated. The failed firm future price was being calculated
rather than non-failed firm in present price as it was not known whether the non-failed
companies was recognized or not in that particular year.
3.2 data collection for the non-failed companies
A similar method was used in sorting and selecting the non-failed firms. The first step
of the process involved searching of the companies from the financial, manufacturing, service
and retail sectors which met the desired criteria as a failed firm in terms of size. The firm’s
size was selected, restricting it to 20 percent considerable to the mean price. The properties of
the companies were cross examined using the “Hemscott Company Guru website” (Lin,
2015). The analyses of the variables were identified by using the online source such as the
firm's official website. There were several firms, whose data was not available and therefore
only 25 companies were taken into use for the purpose of analysis. The latest year was taken
for the non-bankrupt firms. Thus the deviations and dissimilarities of data of the bankrupt
companies could be compared with future price.
3.2.1 Second set of data collection for validation
Following the estimation of the sample of 50 companies, a set of new data was
accumulated for the model substantiation. The study resulted in evaluation of 16 companies
that failed and 19 of the companies that did not fail resulting in the growth of the complexity
of the variables. With the help of online sources and FAME, the financial statements were
extracted (DATA, 2019).
3.3 Sampling
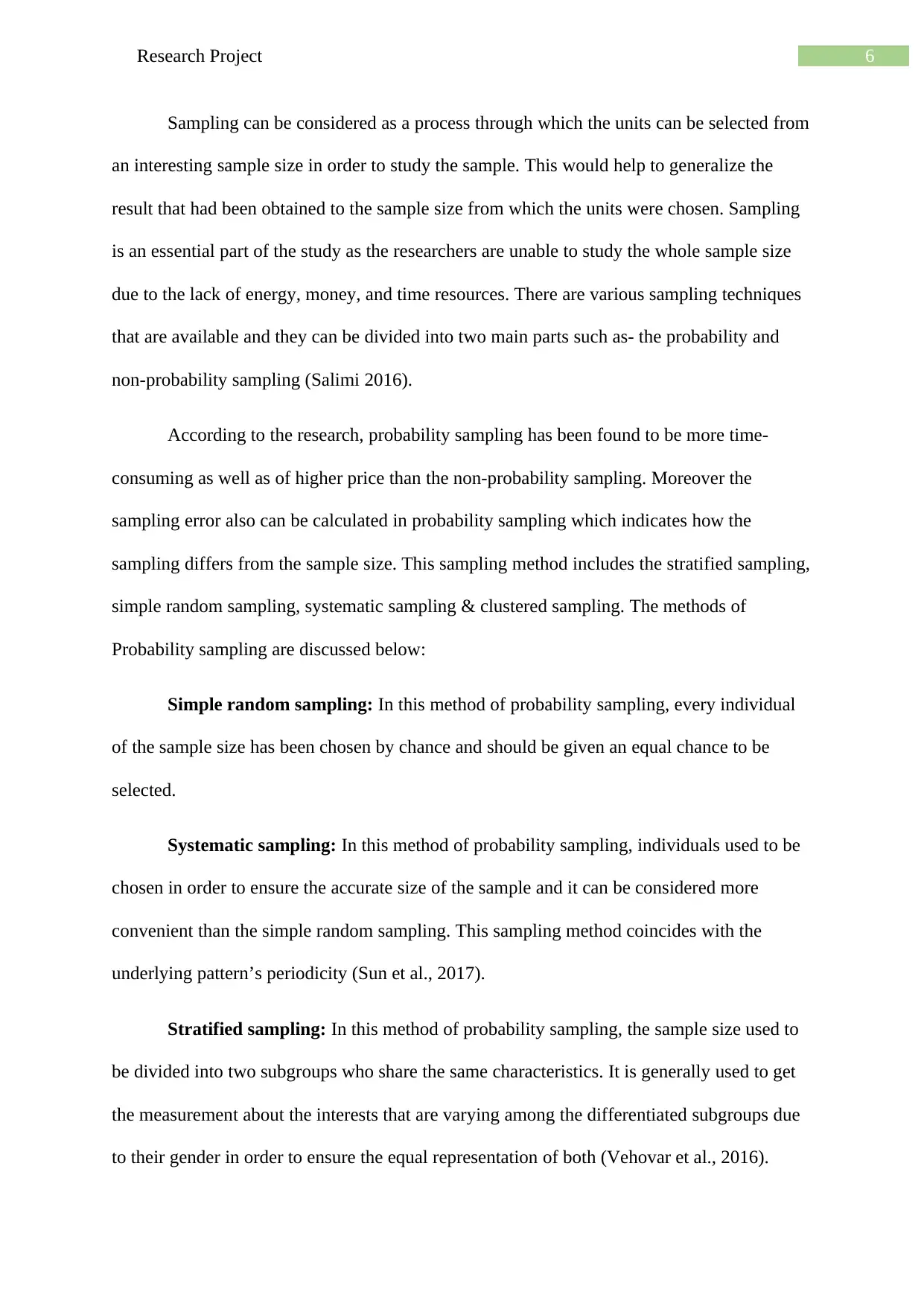
Sampling can be considered as a process through which the units can be selected from
an interesting sample size in order to study the sample. This would help to generalize the
result that had been obtained to the sample size from which the units were chosen. Sampling
is an essential part of the study as the researchers are unable to study the whole sample size
due to the lack of energy, money, and time resources. There are various sampling techniques
that are available and they can be divided into two main parts such as- the probability and
non-probability sampling (Salimi 2016).
According to the research, probability sampling has been found to be more time-
consuming as well as of higher price than the non-probability sampling. Moreover the
sampling error also can be calculated in probability sampling which indicates how the
sampling differs from the sample size. This sampling method includes the stratified sampling,
simple random sampling, systematic sampling & clustered sampling. The methods of
Probability sampling are discussed below:
Simple random sampling: In this method of probability sampling, every individual
of the sample size has been chosen by chance and should be given an equal chance to be
selected.
Systematic sampling: In this method of probability sampling, individuals used to be
chosen in order to ensure the accurate size of the sample and it can be considered more
convenient than the simple random sampling. This sampling method coincides with the
underlying pattern’s periodicity (Sun et al., 2017).
Stratified sampling: In this method of probability sampling, the sample size used to
be divided into two subgroups who share the same characteristics. It is generally used to get
the measurement about the interests that are varying among the differentiated subgroups due
to their gender in order to ensure the equal representation of both (Vehovar et al., 2016).
⊘ This is a preview!⊘
Do you want full access?
Subscribe today to unlock all pages.

Trusted by 1+ million students worldwide
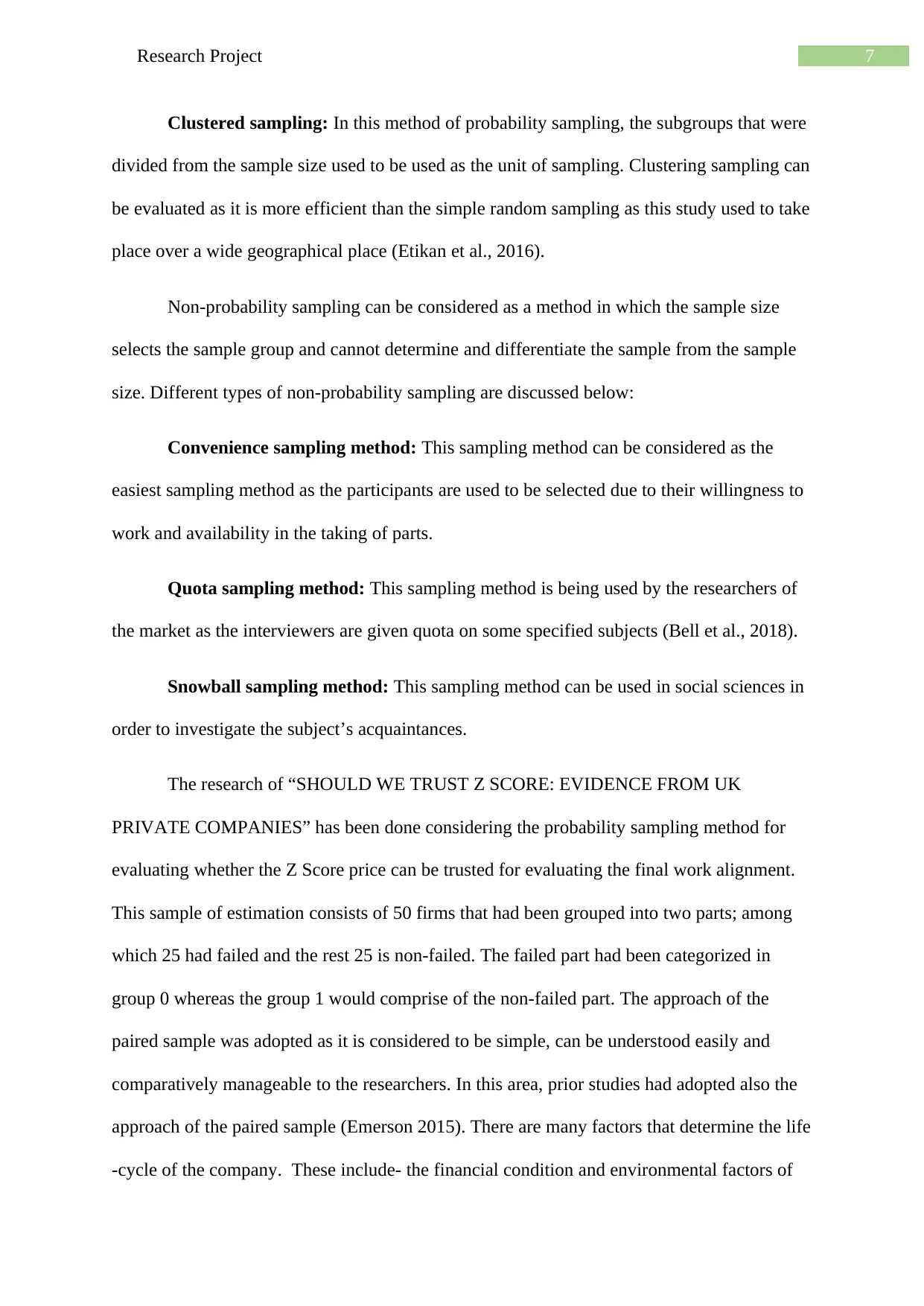
Clustered sampling: In this method of probability sampling, the subgroups that were
divided from the sample size used to be used as the unit of sampling. Clustering sampling can
be evaluated as it is more efficient than the simple random sampling as this study used to take
place over a wide geographical place (Etikan et al., 2016).
Non-probability sampling can be considered as a method in which the sample size
selects the sample group and cannot determine and differentiate the sample from the sample
size. Different types of non-probability sampling are discussed below:
Convenience sampling method: This sampling method can be considered as the
easiest sampling method as the participants are used to be selected due to their willingness to
work and availability in the taking of parts.
Quota sampling method: This sampling method is being used by the researchers of
the market as the interviewers are given quota on some specified subjects (Bell et al., 2018).
Snowball sampling method: This sampling method can be used in social sciences in
order to investigate the subject’s acquaintances.
The research of “SHOULD WE TRUST Z SCORE: EVIDENCE FROM UK
PRIVATE COMPANIES” has been done considering the probability sampling method for
evaluating whether the Z Score price can be trusted for evaluating the final work alignment.
This sample of estimation consists of 50 firms that had been grouped into two parts; among
which 25 had failed and the rest 25 is non-failed. The failed part had been categorized in
group 0 whereas the group 1 would comprise of the non-failed part. The approach of the
paired sample was adopted as it is considered to be simple, can be understood easily and
comparatively manageable to the researchers. In this area, prior studies had adopted also the
approach of the paired sample (Emerson 2015). There are many factors that determine the life
-cycle of the company. These include- the financial condition and environmental factors of
Paraphrase This Document
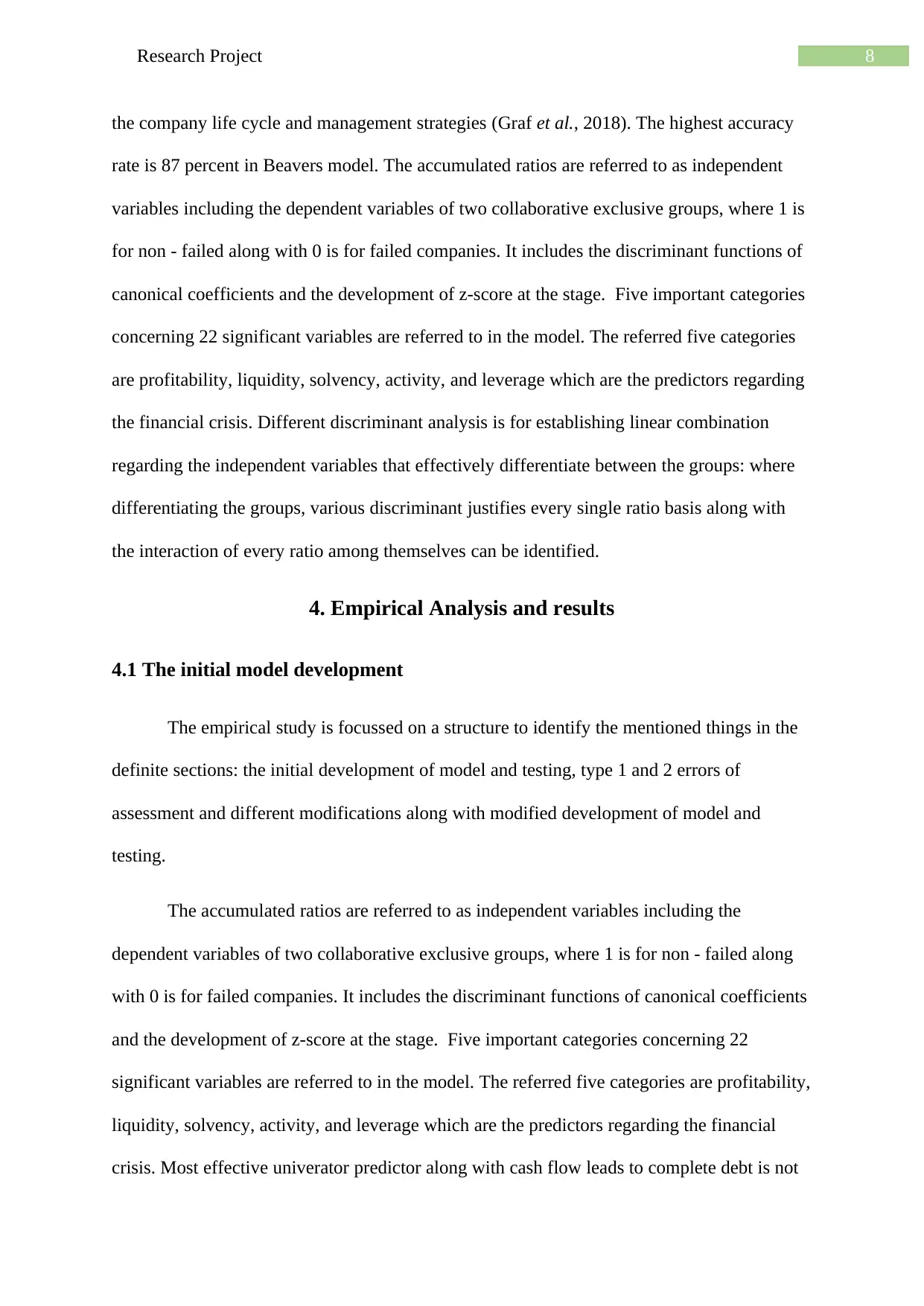
the company life cycle and management strategies (Graf et al., 2018). The highest accuracy
rate is 87 percent in Beavers model. The accumulated ratios are referred to as independent
variables including the dependent variables of two collaborative exclusive groups, where 1 is
for non - failed along with 0 is for failed companies. It includes the discriminant functions of
canonical coefficients and the development of z-score at the stage. Five important categories
concerning 22 significant variables are referred to in the model. The referred five categories
are profitability, liquidity, solvency, activity, and leverage which are the predictors regarding
the financial crisis. Different discriminant analysis is for establishing linear combination
regarding the independent variables that effectively differentiate between the groups: where
differentiating the groups, various discriminant justifies every single ratio basis along with
the interaction of every ratio among themselves can be identified.
4. Empirical Analysis and results
4.1 The initial model development
The empirical study is focussed on a structure to identify the mentioned things in the
definite sections: the initial development of model and testing, type 1 and 2 errors of
assessment and different modifications along with modified development of model and
testing.
The accumulated ratios are referred to as independent variables including the
dependent variables of two collaborative exclusive groups, where 1 is for non - failed along
with 0 is for failed companies. It includes the discriminant functions of canonical coefficients
and the development of z-score at the stage. Five important categories concerning 22
significant variables are referred to in the model. The referred five categories are profitability,
liquidity, solvency, activity, and leverage which are the predictors regarding the financial
crisis. Most effective univerator predictor along with cash flow leads to complete debt is not
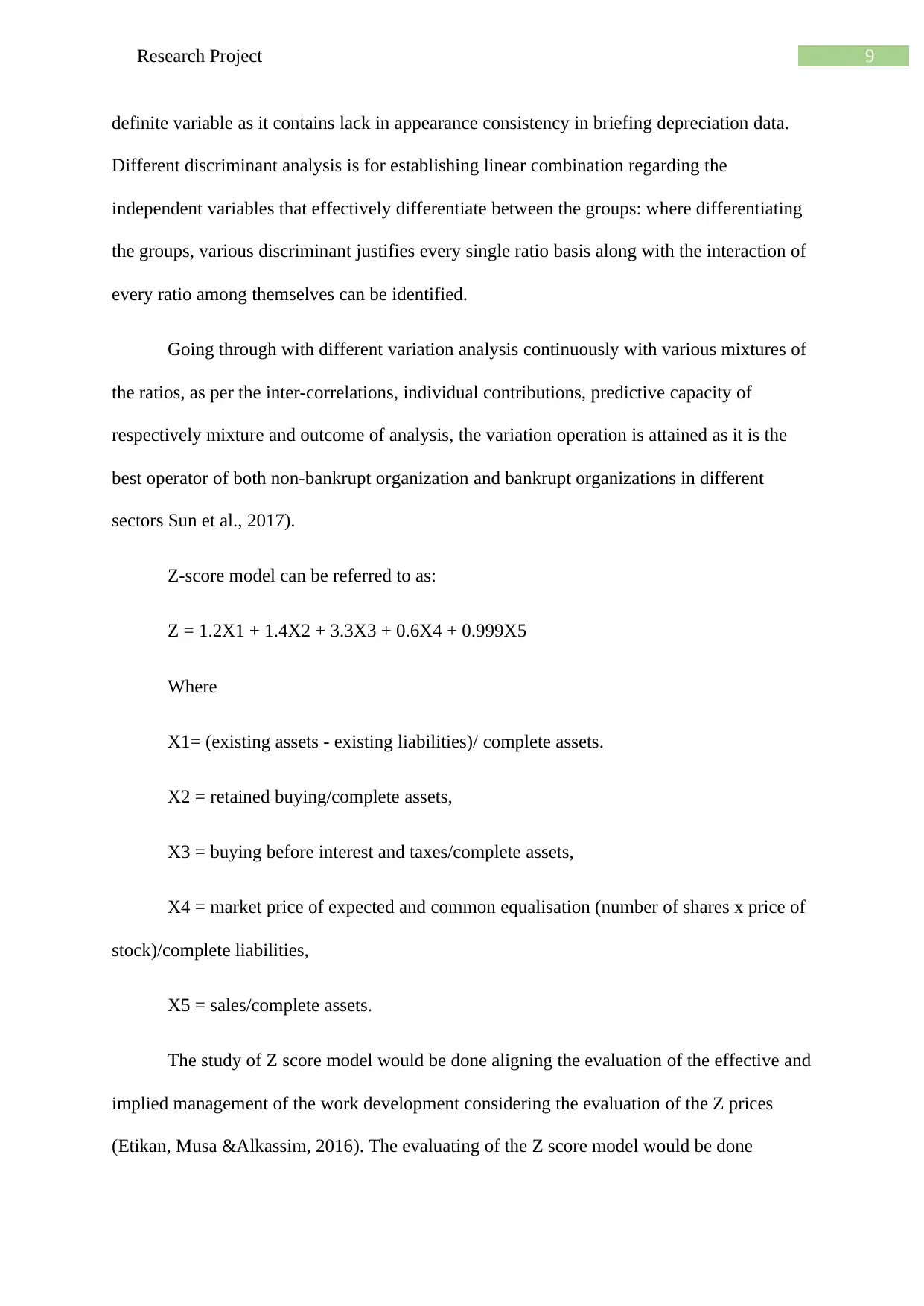
definite variable as it contains lack in appearance consistency in briefing depreciation data.
Different discriminant analysis is for establishing linear combination regarding the
independent variables that effectively differentiate between the groups: where differentiating
the groups, various discriminant justifies every single ratio basis along with the interaction of
every ratio among themselves can be identified.
Going through with different variation analysis continuously with various mixtures of
the ratios, as per the inter-correlations, individual contributions, predictive capacity of
respectively mixture and outcome of analysis, the variation operation is attained as it is the
best operator of both non-bankrupt organization and bankrupt organizations in different
sectors Sun et al., 2017).
Z-score model can be referred to as:
Z = 1.2X1 + 1.4X2 + 3.3X3 + 0.6X4 + 0.999X5
Where
X1= (existing assets - existing liabilities)/ complete assets.
X2 = retained buying/complete assets,
X3 = buying before interest and taxes/complete assets,
X4 = market price of expected and common equalisation (number of shares x price of
stock)/complete liabilities,
X5 = sales/complete assets.
The study of Z score model would be done aligning the evaluation of the effective and
implied management of the work development considering the evaluation of the Z prices
(Etikan, Musa &Alkassim, 2016). The evaluating of the Z score model would be done
⊘ This is a preview!⊘
Do you want full access?
Subscribe today to unlock all pages.

Trusted by 1+ million students worldwide
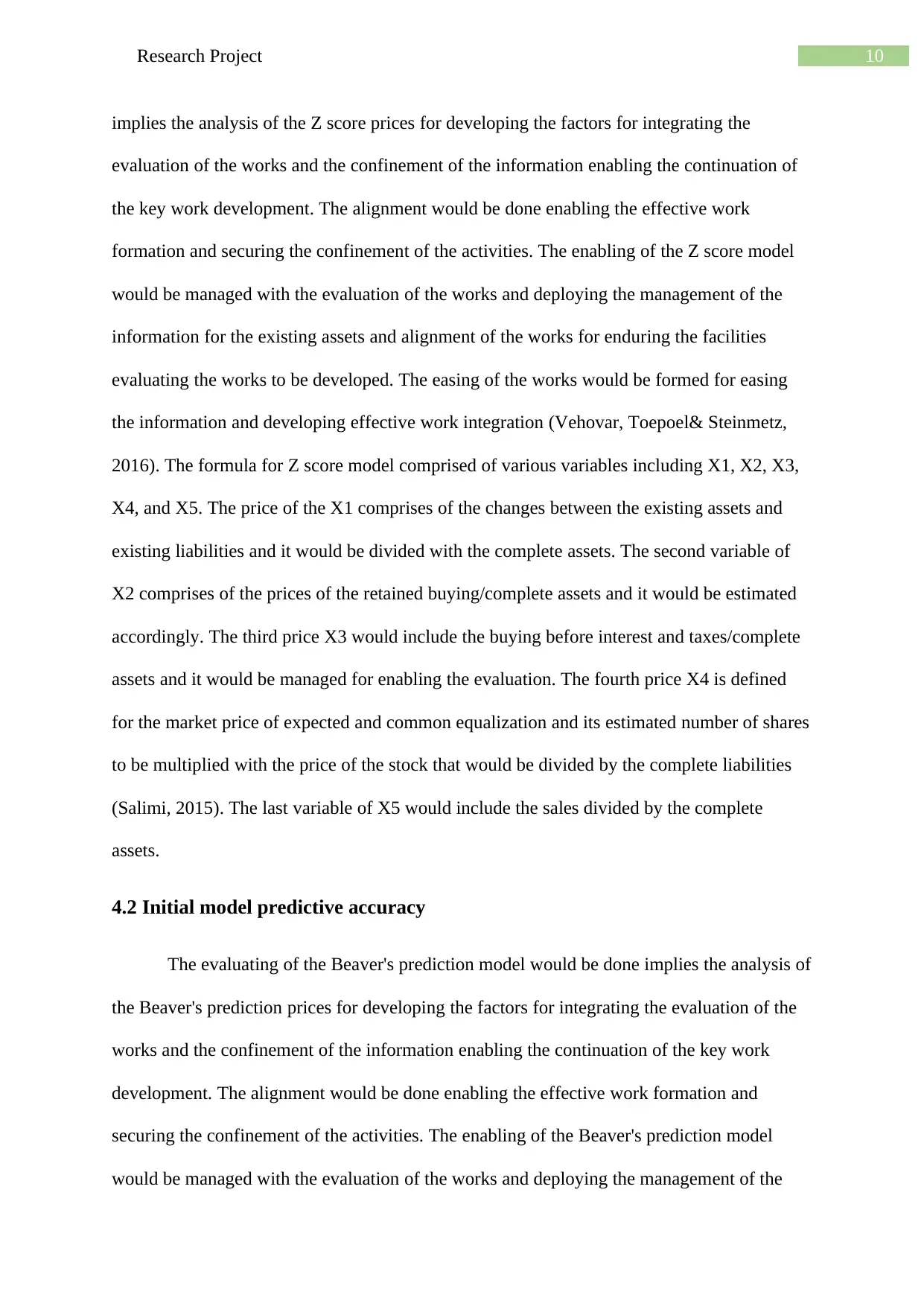
implies the analysis of the Z score prices for developing the factors for integrating the
evaluation of the works and the confinement of the information enabling the continuation of
the key work development. The alignment would be done enabling the effective work
formation and securing the confinement of the activities. The enabling of the Z score model
would be managed with the evaluation of the works and deploying the management of the
information for the existing assets and alignment of the works for enduring the facilities
evaluating the works to be developed. The easing of the works would be formed for easing
the information and developing effective work integration (Vehovar, Toepoel& Steinmetz,
2016). The formula for Z score model comprised of various variables including X1, X2, X3,
X4, and X5. The price of the X1 comprises of the changes between the existing assets and
existing liabilities and it would be divided with the complete assets. The second variable of
X2 comprises of the prices of the retained buying/complete assets and it would be estimated
accordingly. The third price X3 would include the buying before interest and taxes/complete
assets and it would be managed for enabling the evaluation. The fourth price X4 is defined
for the market price of expected and common equalization and its estimated number of shares
to be multiplied with the price of the stock that would be divided by the complete liabilities
(Salimi, 2015). The last variable of X5 would include the sales divided by the complete
assets.
4.2 Initial model predictive accuracy
The evaluating of the Beaver's prediction model would be done implies the analysis of
the Beaver's prediction prices for developing the factors for integrating the evaluation of the
works and the confinement of the information enabling the continuation of the key work
development. The alignment would be done enabling the effective work formation and
securing the confinement of the activities. The enabling of the Beaver's prediction model
would be managed with the evaluation of the works and deploying the management of the
Paraphrase This Document
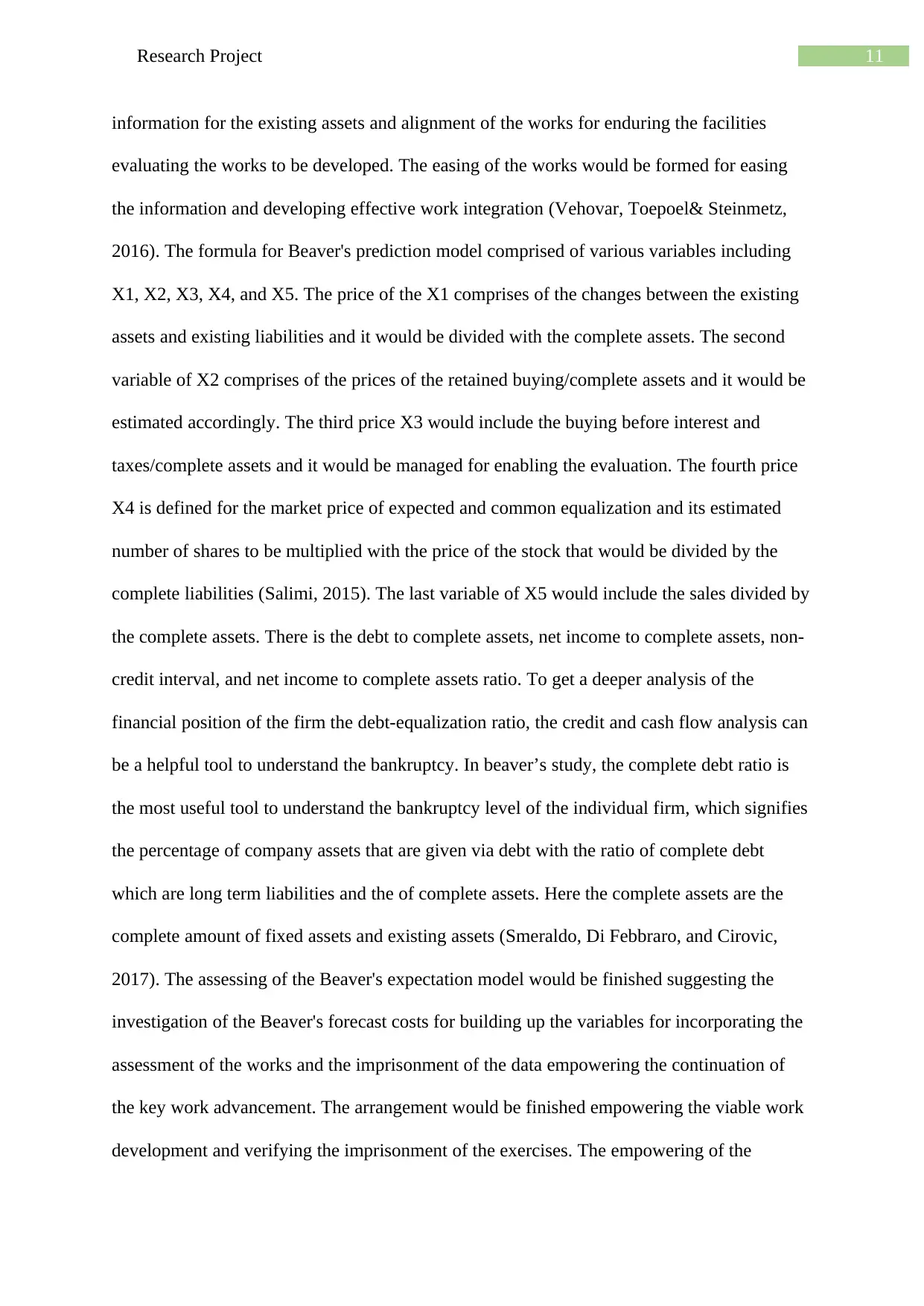
information for the existing assets and alignment of the works for enduring the facilities
evaluating the works to be developed. The easing of the works would be formed for easing
the information and developing effective work integration (Vehovar, Toepoel& Steinmetz,
2016). The formula for Beaver's prediction model comprised of various variables including
X1, X2, X3, X4, and X5. The price of the X1 comprises of the changes between the existing
assets and existing liabilities and it would be divided with the complete assets. The second
variable of X2 comprises of the prices of the retained buying/complete assets and it would be
estimated accordingly. The third price X3 would include the buying before interest and
taxes/complete assets and it would be managed for enabling the evaluation. The fourth price
X4 is defined for the market price of expected and common equalization and its estimated
number of shares to be multiplied with the price of the stock that would be divided by the
complete liabilities (Salimi, 2015). The last variable of X5 would include the sales divided by
the complete assets. There is the debt to complete assets, net income to complete assets, non-
credit interval, and net income to complete assets ratio. To get a deeper analysis of the
financial position of the firm the debt-equalization ratio, the credit and cash flow analysis can
be a helpful tool to understand the bankruptcy. In beaver’s study, the complete debt ratio is
the most useful tool to understand the bankruptcy level of the individual firm, which signifies
the percentage of company assets that are given via debt with the ratio of complete debt
which are long term liabilities and the of complete assets. Here the complete assets are the
complete amount of fixed assets and existing assets (Smeraldo, Di Febbraro, and Cirovic,
2017). The assessing of the Beaver's expectation model would be finished suggesting the
investigation of the Beaver's forecast costs for building up the variables for incorporating the
assessment of the works and the imprisonment of the data empowering the continuation of
the key work advancement. The arrangement would be finished empowering the viable work
development and verifying the imprisonment of the exercises. The empowering of the
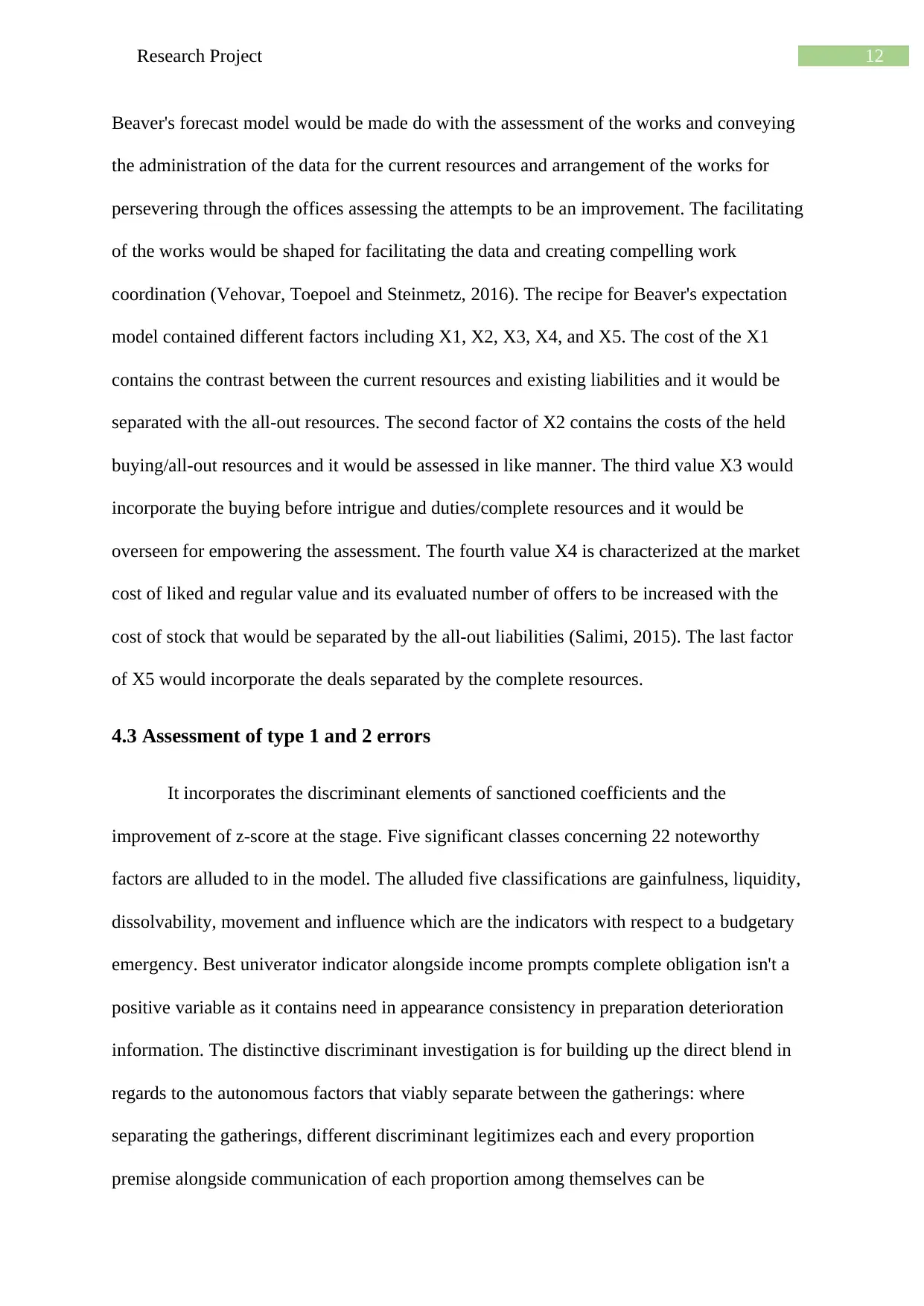
Beaver's forecast model would be made do with the assessment of the works and conveying
the administration of the data for the current resources and arrangement of the works for
persevering through the offices assessing the attempts to be an improvement. The facilitating
of the works would be shaped for facilitating the data and creating compelling work
coordination (Vehovar, Toepoel and Steinmetz, 2016). The recipe for Beaver's expectation
model contained different factors including X1, X2, X3, X4, and X5. The cost of the X1
contains the contrast between the current resources and existing liabilities and it would be
separated with the all-out resources. The second factor of X2 contains the costs of the held
buying/all-out resources and it would be assessed in like manner. The third value X3 would
incorporate the buying before intrigue and duties/complete resources and it would be
overseen for empowering the assessment. The fourth value X4 is characterized at the market
cost of liked and regular value and its evaluated number of offers to be increased with the
cost of stock that would be separated by the all-out liabilities (Salimi, 2015). The last factor
of X5 would incorporate the deals separated by the complete resources.
4.3 Assessment of type 1 and 2 errors
It incorporates the discriminant elements of sanctioned coefficients and the
improvement of z-score at the stage. Five significant classes concerning 22 noteworthy
factors are alluded to in the model. The alluded five classifications are gainfulness, liquidity,
dissolvability, movement and influence which are the indicators with respect to a budgetary
emergency. Best univerator indicator alongside income prompts complete obligation isn't a
positive variable as it contains need in appearance consistency in preparation deterioration
information. The distinctive discriminant investigation is for building up the direct blend in
regards to the autonomous factors that viably separate between the gatherings: where
separating the gatherings, different discriminant legitimizes each and every proportion
premise alongside communication of each proportion among themselves can be
⊘ This is a preview!⊘
Do you want full access?
Subscribe today to unlock all pages.

Trusted by 1+ million students worldwide
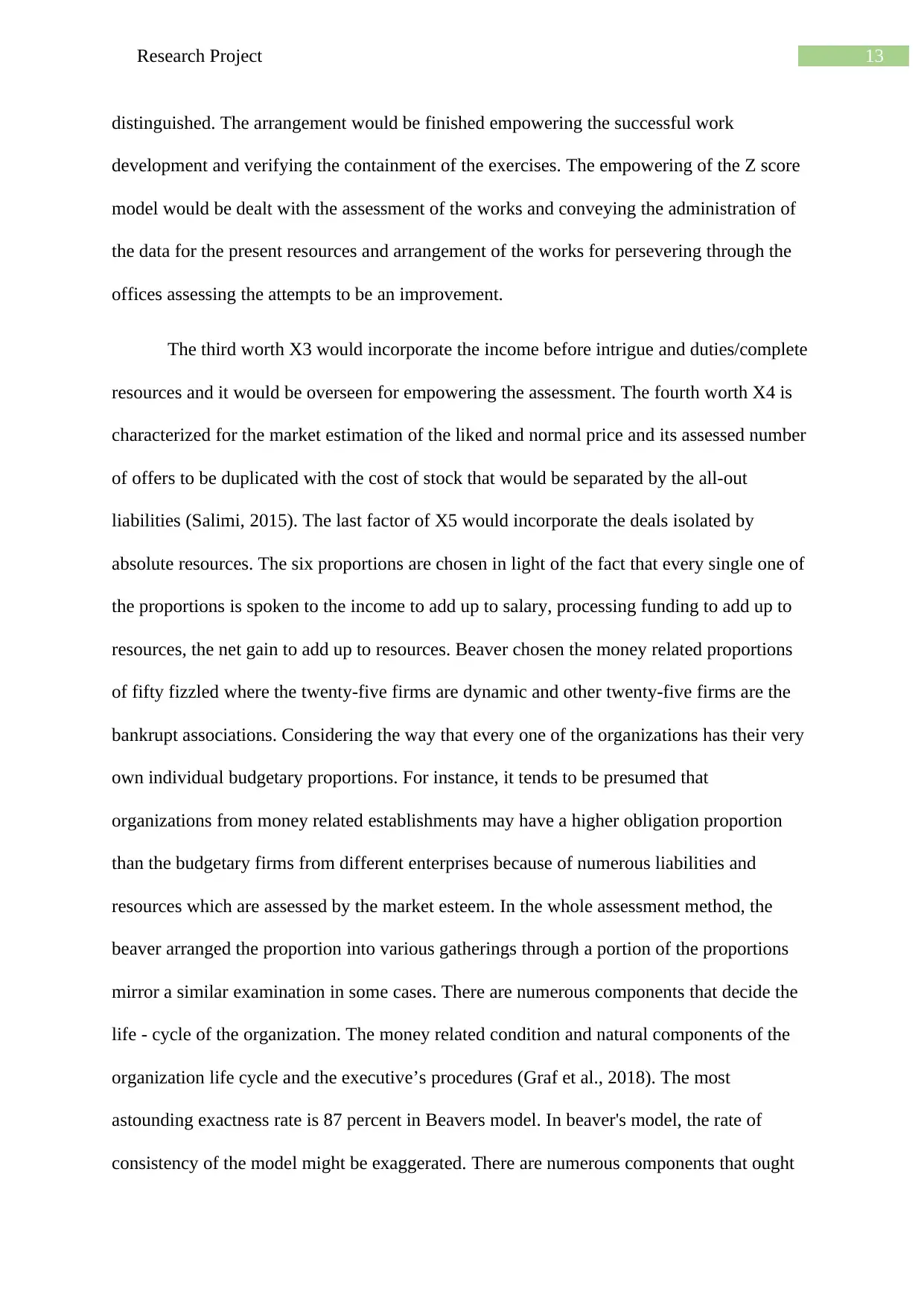
distinguished. The arrangement would be finished empowering the successful work
development and verifying the containment of the exercises. The empowering of the Z score
model would be dealt with the assessment of the works and conveying the administration of
the data for the present resources and arrangement of the works for persevering through the
offices assessing the attempts to be an improvement.
The third worth X3 would incorporate the income before intrigue and duties/complete
resources and it would be overseen for empowering the assessment. The fourth worth X4 is
characterized for the market estimation of the liked and normal price and its assessed number
of offers to be duplicated with the cost of stock that would be separated by the all-out
liabilities (Salimi, 2015). The last factor of X5 would incorporate the deals isolated by
absolute resources. The six proportions are chosen in light of the fact that every single one of
the proportions is spoken to the income to add up to salary, processing funding to add up to
resources, the net gain to add up to resources. Beaver chosen the money related proportions
of fifty fizzled where the twenty-five firms are dynamic and other twenty-five firms are the
bankrupt associations. Considering the way that every one of the organizations has their very
own individual budgetary proportions. For instance, it tends to be presumed that
organizations from money related establishments may have a higher obligation proportion
than the budgetary firms from different enterprises because of numerous liabilities and
resources which are assessed by the market esteem. In the whole assessment method, the
beaver arranged the proportion into various gatherings through a portion of the proportions
mirror a similar examination in some cases. There are numerous components that decide the
life - cycle of the organization. The money related condition and natural components of the
organization life cycle and the executive’s procedures (Graf et al., 2018). The most
astounding exactness rate is 87 percent in Beavers model. In beaver's model, the rate of
consistency of the model might be exaggerated. There are numerous components that ought
Paraphrase This Document
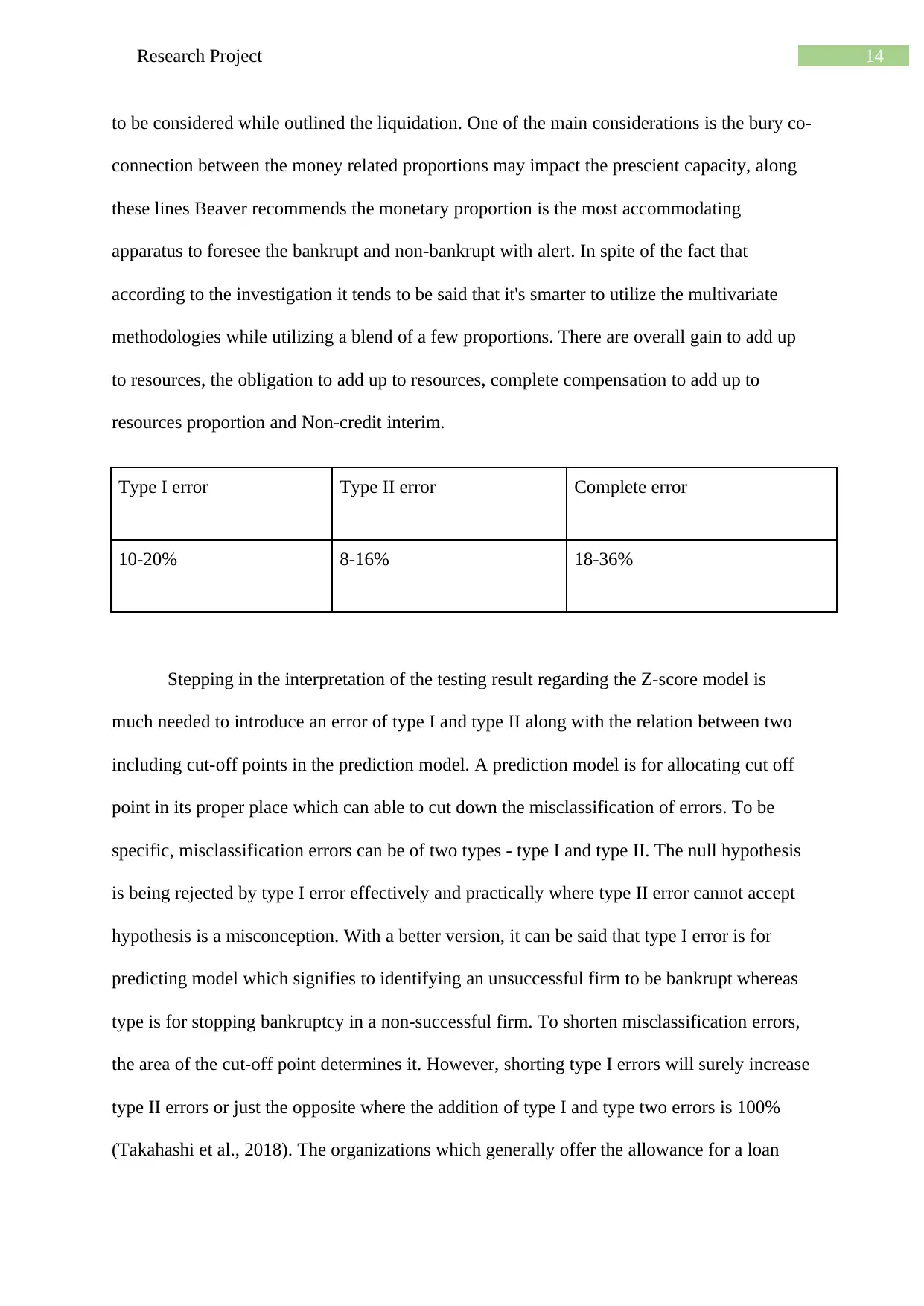
to be considered while outlined the liquidation. One of the main considerations is the bury co-
connection between the money related proportions may impact the prescient capacity, along
these lines Beaver recommends the monetary proportion is the most accommodating
apparatus to foresee the bankrupt and non-bankrupt with alert. In spite of the fact that
according to the investigation it tends to be said that it's smarter to utilize the multivariate
methodologies while utilizing a blend of a few proportions. There are overall gain to add up
to resources, the obligation to add up to resources, complete compensation to add up to
resources proportion and Non-credit interim.
Type I error Type II error Complete error
10-20% 8-16% 18-36%
Stepping in the interpretation of the testing result regarding the Z-score model is
much needed to introduce an error of type I and type II along with the relation between two
including cut-off points in the prediction model. A prediction model is for allocating cut off
point in its proper place which can able to cut down the misclassification of errors. To be
specific, misclassification errors can be of two types - type I and type II. The null hypothesis
is being rejected by type I error effectively and practically where type II error cannot accept
hypothesis is a misconception. With a better version, it can be said that type I error is for
predicting model which signifies to identifying an unsuccessful firm to be bankrupt whereas
type is for stopping bankruptcy in a non-successful firm. To shorten misclassification errors,
the area of the cut-off point determines it. However, shorting type I errors will surely increase
type II errors or just the opposite where the addition of type I and type two errors is 100%
(Takahashi et al., 2018). The organizations which generally offer the allowance for a loan
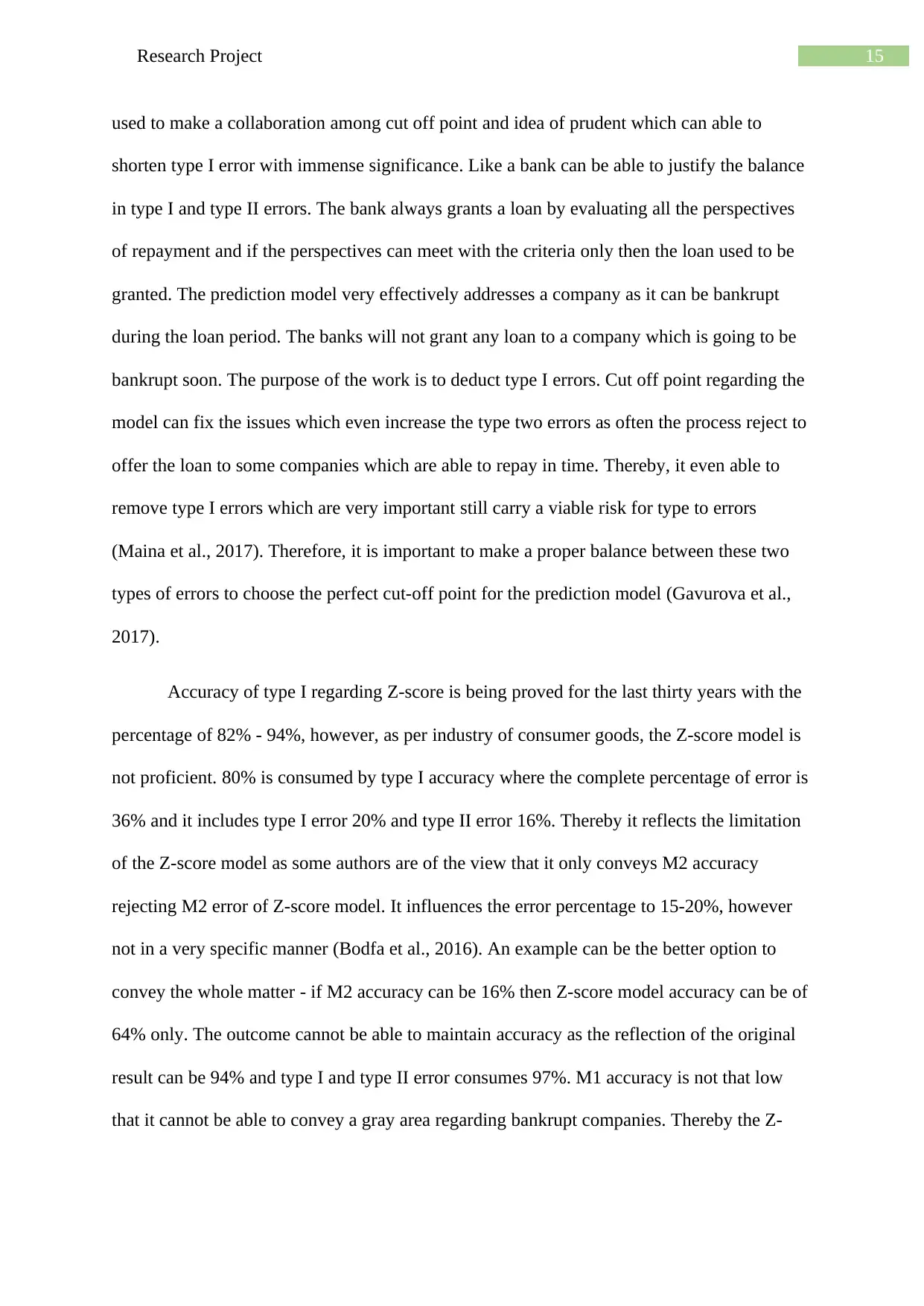
used to make a collaboration among cut off point and idea of prudent which can able to
shorten type I error with immense significance. Like a bank can be able to justify the balance
in type I and type II errors. The bank always grants a loan by evaluating all the perspectives
of repayment and if the perspectives can meet with the criteria only then the loan used to be
granted. The prediction model very effectively addresses a company as it can be bankrupt
during the loan period. The banks will not grant any loan to a company which is going to be
bankrupt soon. The purpose of the work is to deduct type I errors. Cut off point regarding the
model can fix the issues which even increase the type two errors as often the process reject to
offer the loan to some companies which are able to repay in time. Thereby, it even able to
remove type I errors which are very important still carry a viable risk for type to errors
(Maina et al., 2017). Therefore, it is important to make a proper balance between these two
types of errors to choose the perfect cut-off point for the prediction model (Gavurova et al.,
2017).
Accuracy of type I regarding Z-score is being proved for the last thirty years with the
percentage of 82% - 94%, however, as per industry of consumer goods, the Z-score model is
not proficient. 80% is consumed by type I accuracy where the complete percentage of error is
36% and it includes type I error 20% and type II error 16%. Thereby it reflects the limitation
of the Z-score model as some authors are of the view that it only conveys M2 accuracy
rejecting M2 error of Z-score model. It influences the error percentage to 15-20%, however
not in a very specific manner (Bodfa et al., 2016). An example can be the better option to
convey the whole matter - if M2 accuracy can be 16% then Z-score model accuracy can be of
64% only. The outcome cannot be able to maintain accuracy as the reflection of the original
result can be 94% and type I and type II error consumes 97%. M1 accuracy is not that low
that it cannot be able to convey a gray area regarding bankrupt companies. Thereby the Z-
⊘ This is a preview!⊘
Do you want full access?
Subscribe today to unlock all pages.

Trusted by 1+ million students worldwide
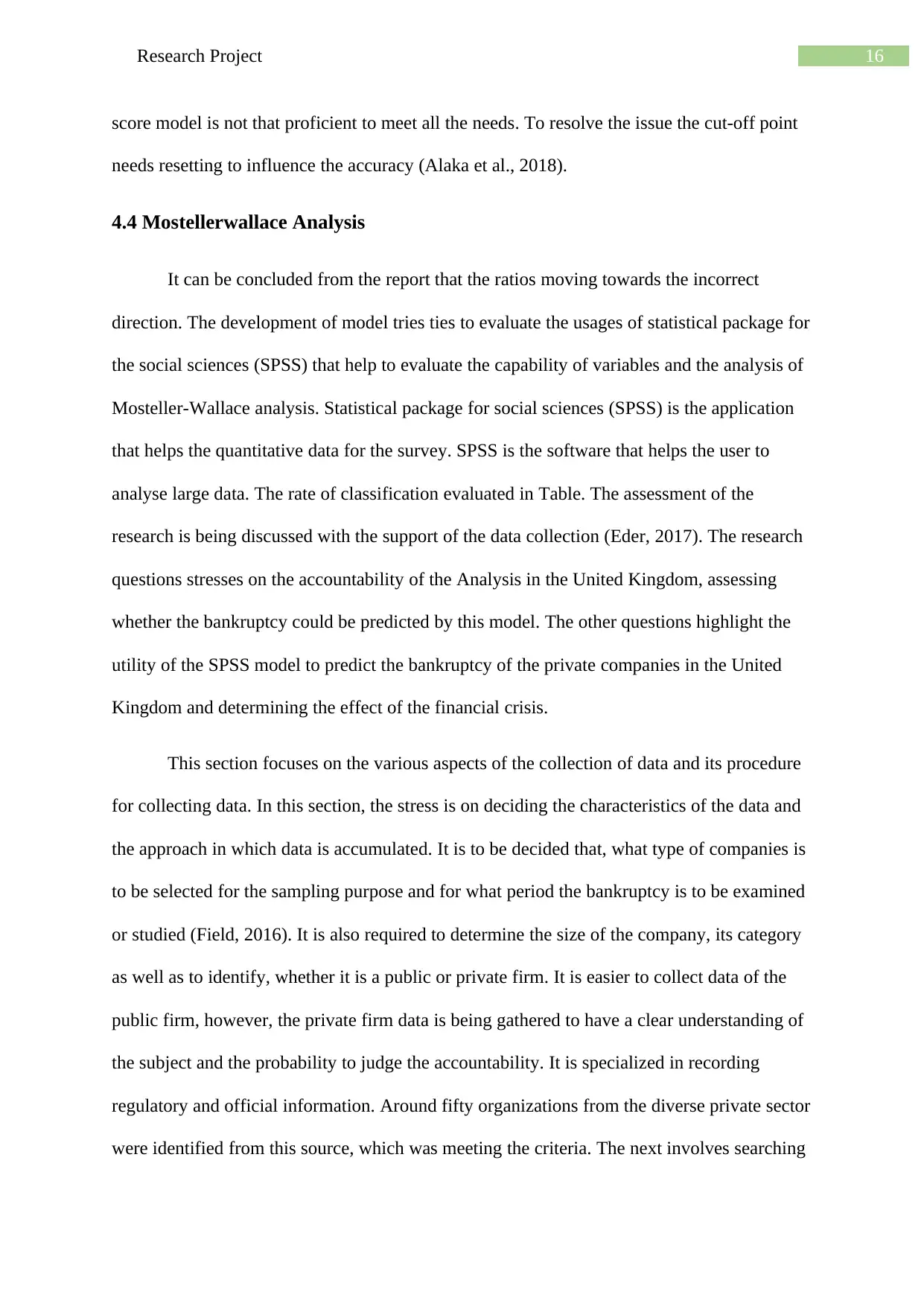
score model is not that proficient to meet all the needs. To resolve the issue the cut-off point
needs resetting to influence the accuracy (Alaka et al., 2018).
4.4 Mostellerwallace Analysis
It can be concluded from the report that the ratios moving towards the incorrect
direction. The development of model tries ties to evaluate the usages of statistical package for
the social sciences (SPSS) that help to evaluate the capability of variables and the analysis of
Mosteller-Wallace analysis. Statistical package for social sciences (SPSS) is the application
that helps the quantitative data for the survey. SPSS is the software that helps the user to
analyse large data. The rate of classification evaluated in Table. The assessment of the
research is being discussed with the support of the data collection (Eder, 2017). The research
questions stresses on the accountability of the Analysis in the United Kingdom, assessing
whether the bankruptcy could be predicted by this model. The other questions highlight the
utility of the SPSS model to predict the bankruptcy of the private companies in the United
Kingdom and determining the effect of the financial crisis.
This section focuses on the various aspects of the collection of data and its procedure
for collecting data. In this section, the stress is on deciding the characteristics of the data and
the approach in which data is accumulated. It is to be decided that, what type of companies is
to be selected for the sampling purpose and for what period the bankruptcy is to be examined
or studied (Field, 2016). It is also required to determine the size of the company, its category
as well as to identify, whether it is a public or private firm. It is easier to collect data of the
public firm, however, the private firm data is being gathered to have a clear understanding of
the subject and the probability to judge the accountability. It is specialized in recording
regulatory and official information. Around fifty organizations from the diverse private sector
were identified from this source, which was meeting the criteria. The next involves searching
Paraphrase This Document
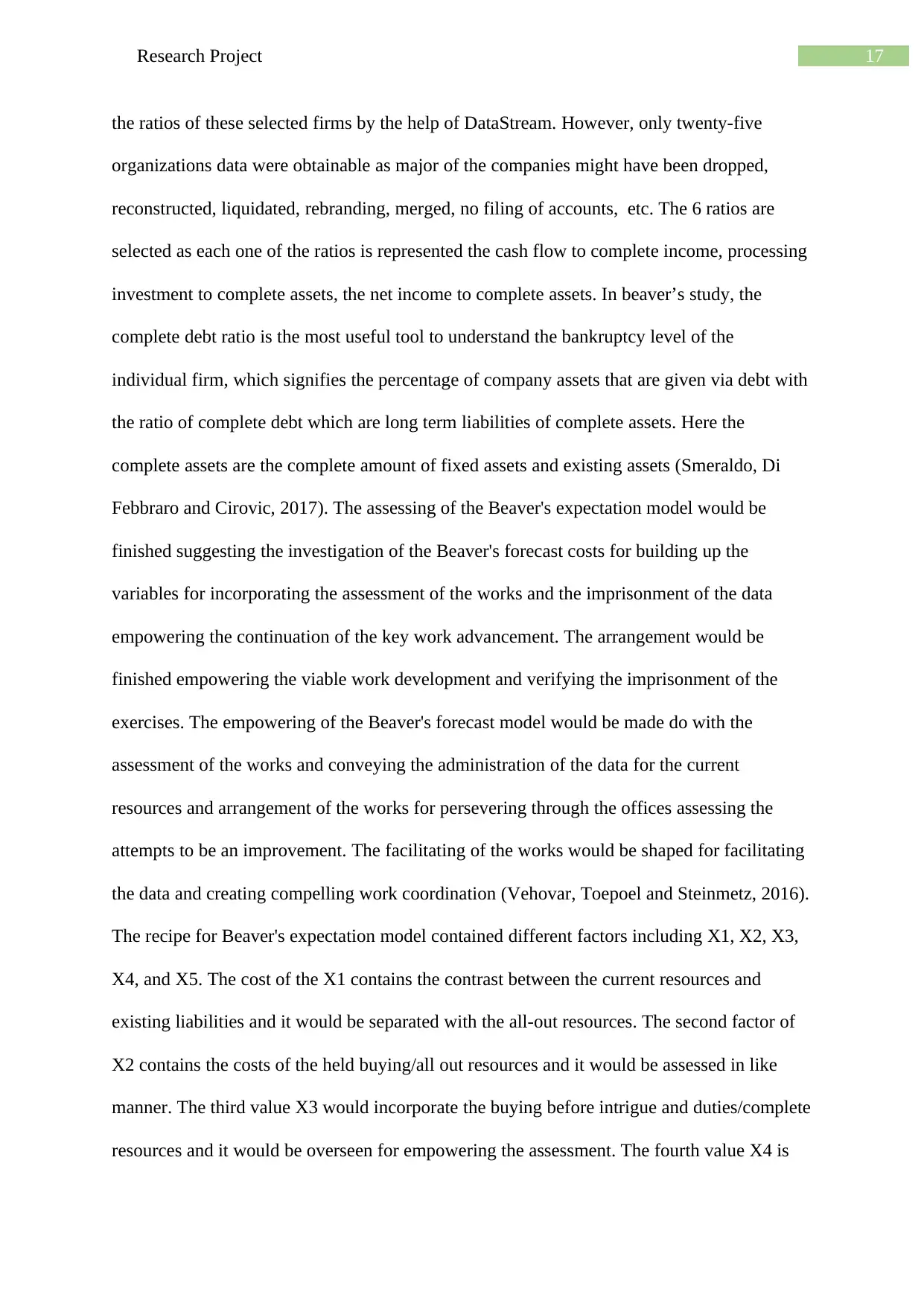
the ratios of these selected firms by the help of DataStream. However, only twenty-five
organizations data were obtainable as major of the companies might have been dropped,
reconstructed, liquidated, rebranding, merged, no filing of accounts, etc. The 6 ratios are
selected as each one of the ratios is represented the cash flow to complete income, processing
investment to complete assets, the net income to complete assets. In beaver’s study, the
complete debt ratio is the most useful tool to understand the bankruptcy level of the
individual firm, which signifies the percentage of company assets that are given via debt with
the ratio of complete debt which are long term liabilities of complete assets. Here the
complete assets are the complete amount of fixed assets and existing assets (Smeraldo, Di
Febbraro and Cirovic, 2017). The assessing of the Beaver's expectation model would be
finished suggesting the investigation of the Beaver's forecast costs for building up the
variables for incorporating the assessment of the works and the imprisonment of the data
empowering the continuation of the key work advancement. The arrangement would be
finished empowering the viable work development and verifying the imprisonment of the
exercises. The empowering of the Beaver's forecast model would be made do with the
assessment of the works and conveying the administration of the data for the current
resources and arrangement of the works for persevering through the offices assessing the
attempts to be an improvement. The facilitating of the works would be shaped for facilitating
the data and creating compelling work coordination (Vehovar, Toepoel and Steinmetz, 2016).
The recipe for Beaver's expectation model contained different factors including X1, X2, X3,
X4, and X5. The cost of the X1 contains the contrast between the current resources and
existing liabilities and it would be separated with the all-out resources. The second factor of
X2 contains the costs of the held buying/all out resources and it would be assessed in like
manner. The third value X3 would incorporate the buying before intrigue and duties/complete
resources and it would be overseen for empowering the assessment. The fourth value X4 is
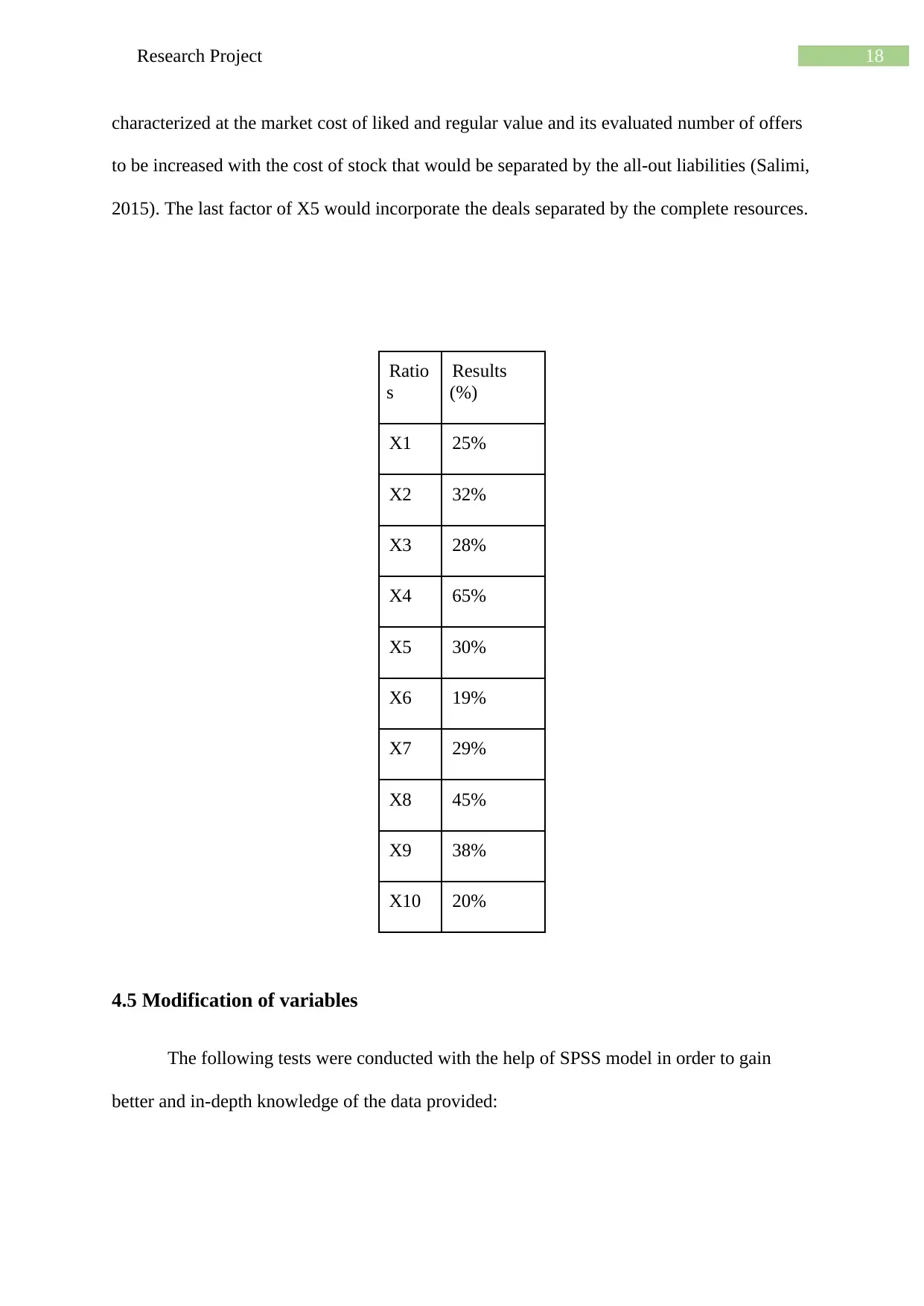
characterized at the market cost of liked and regular value and its evaluated number of offers
to be increased with the cost of stock that would be separated by the all-out liabilities (Salimi,
2015). The last factor of X5 would incorporate the deals separated by the complete resources.
Ratio
s
Results
(%)
X1 25%
X2 32%
X3 28%
X4 65%
X5 30%
X6 19%
X7 29%
X8 45%
X9 38%
X10 20%
4.5 Modification of variables
The following tests were conducted with the help of SPSS model in order to gain
better and in-depth knowledge of the data provided:
⊘ This is a preview!⊘
Do you want full access?
Subscribe today to unlock all pages.

Trusted by 1+ million students worldwide
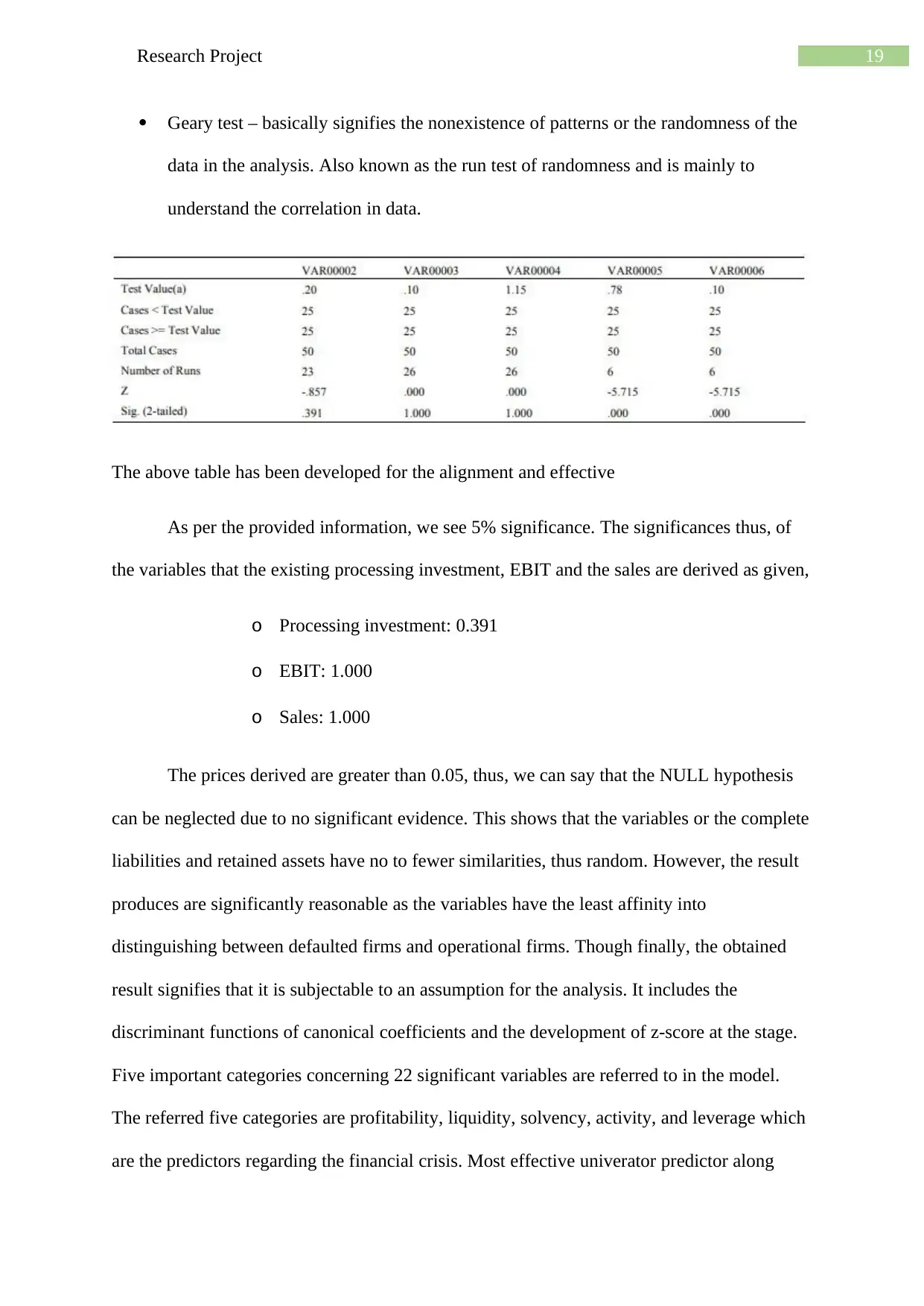
Geary test – basically signifies the nonexistence of patterns or the randomness of the
data in the analysis. Also known as the run test of randomness and is mainly to
understand the correlation in data.
The above table has been developed for the alignment and effective
As per the provided information, we see 5% significance. The significances thus, of
the variables that the existing processing investment, EBIT and the sales are derived as given,
o Processing investment: 0.391
o EBIT: 1.000
o Sales: 1.000
The prices derived are greater than 0.05, thus, we can say that the NULL hypothesis
can be neglected due to no significant evidence. This shows that the variables or the complete
liabilities and retained assets have no to fewer similarities, thus random. However, the result
produces are significantly reasonable as the variables have the least affinity into
distinguishing between defaulted firms and operational firms. Though finally, the obtained
result signifies that it is subjectable to an assumption for the analysis. It includes the
discriminant functions of canonical coefficients and the development of z-score at the stage.
Five important categories concerning 22 significant variables are referred to in the model.
The referred five categories are profitability, liquidity, solvency, activity, and leverage which
are the predictors regarding the financial crisis. Most effective univerator predictor along
Paraphrase This Document
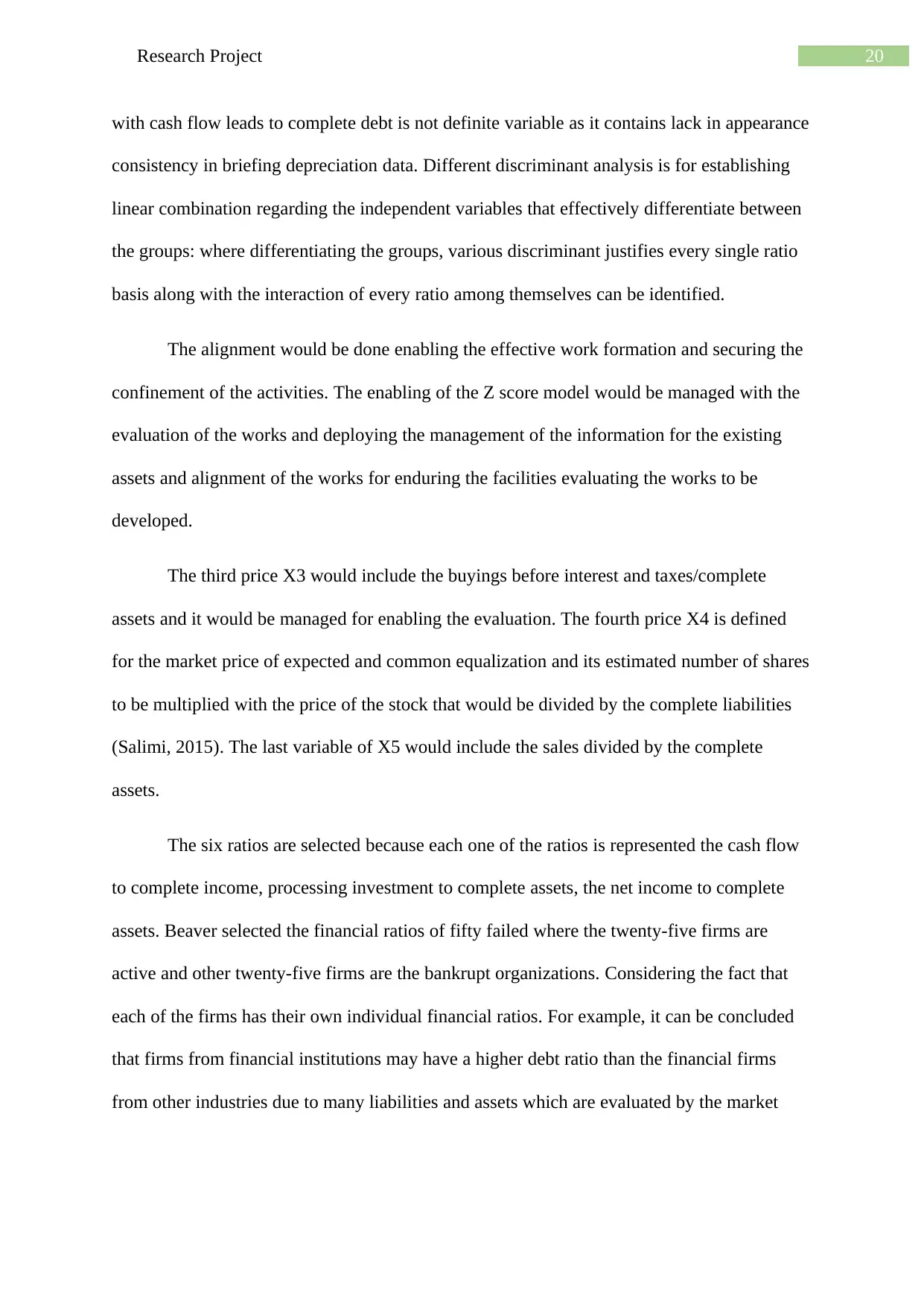
with cash flow leads to complete debt is not definite variable as it contains lack in appearance
consistency in briefing depreciation data. Different discriminant analysis is for establishing
linear combination regarding the independent variables that effectively differentiate between
the groups: where differentiating the groups, various discriminant justifies every single ratio
basis along with the interaction of every ratio among themselves can be identified.
The alignment would be done enabling the effective work formation and securing the
confinement of the activities. The enabling of the Z score model would be managed with the
evaluation of the works and deploying the management of the information for the existing
assets and alignment of the works for enduring the facilities evaluating the works to be
developed.
The third price X3 would include the buyings before interest and taxes/complete
assets and it would be managed for enabling the evaluation. The fourth price X4 is defined
for the market price of expected and common equalization and its estimated number of shares
to be multiplied with the price of the stock that would be divided by the complete liabilities
(Salimi, 2015). The last variable of X5 would include the sales divided by the complete
assets.
The six ratios are selected because each one of the ratios is represented the cash flow
to complete income, processing investment to complete assets, the net income to complete
assets. Beaver selected the financial ratios of fifty failed where the twenty-five firms are
active and other twenty-five firms are the bankrupt organizations. Considering the fact that
each of the firms has their own individual financial ratios. For example, it can be concluded
that firms from financial institutions may have a higher debt ratio than the financial firms
from other industries due to many liabilities and assets which are evaluated by the market
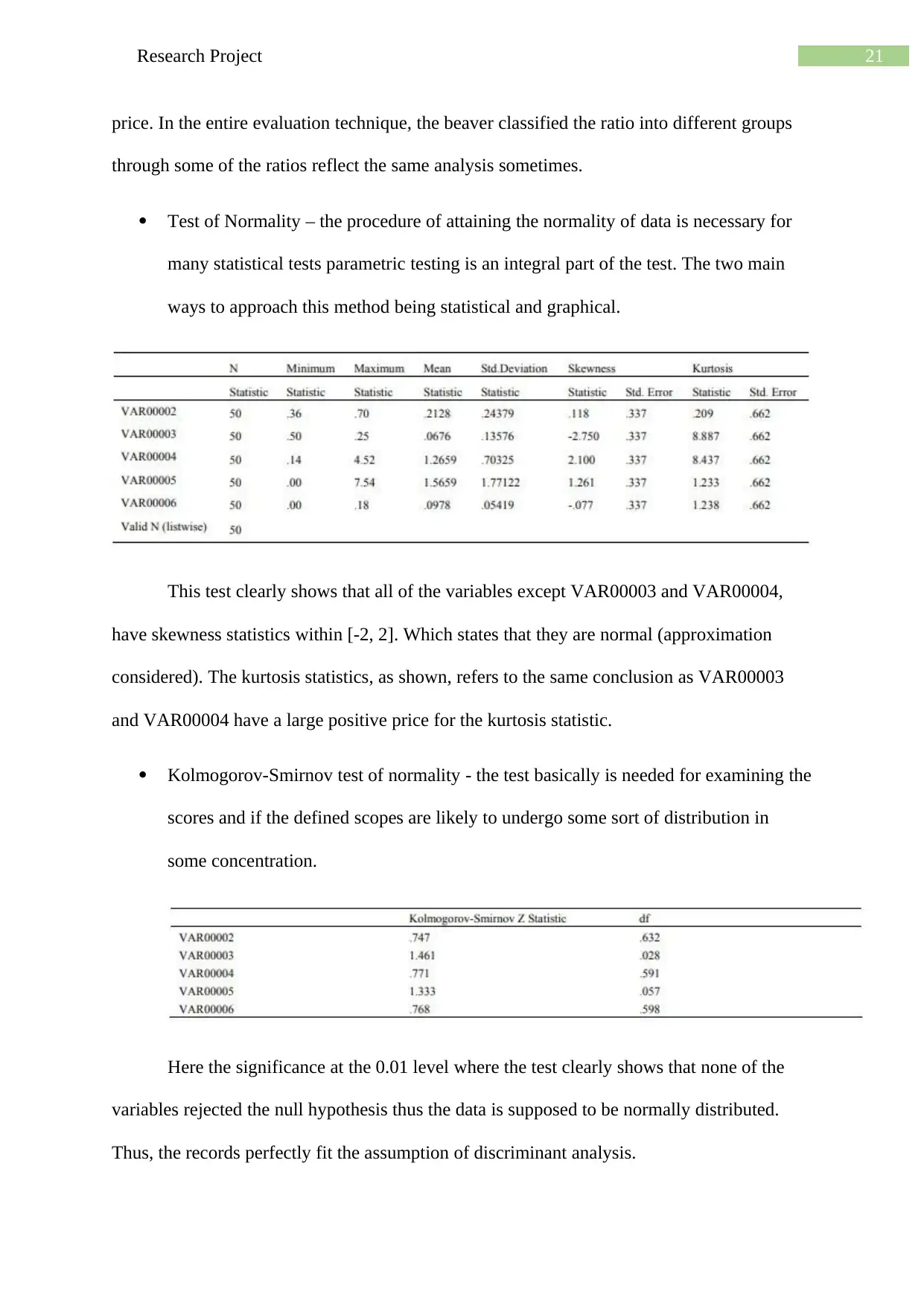
price. In the entire evaluation technique, the beaver classified the ratio into different groups
through some of the ratios reflect the same analysis sometimes.
Test of Normality – the procedure of attaining the normality of data is necessary for
many statistical tests parametric testing is an integral part of the test. The two main
ways to approach this method being statistical and graphical.
This test clearly shows that all of the variables except VAR00003 and VAR00004,
have skewness statistics within [-2, 2]. Which states that they are normal (approximation
considered). The kurtosis statistics, as shown, refers to the same conclusion as VAR00003
and VAR00004 have a large positive price for the kurtosis statistic.
Kolmogorov-Smirnov test of normality - the test basically is needed for examining the
scores and if the defined scopes are likely to undergo some sort of distribution in
some concentration.
Here the significance at the 0.01 level where the test clearly shows that none of the
variables rejected the null hypothesis thus the data is supposed to be normally distributed.
Thus, the records perfectly fit the assumption of discriminant analysis.
⊘ This is a preview!⊘
Do you want full access?
Subscribe today to unlock all pages.

Trusted by 1+ million students worldwide
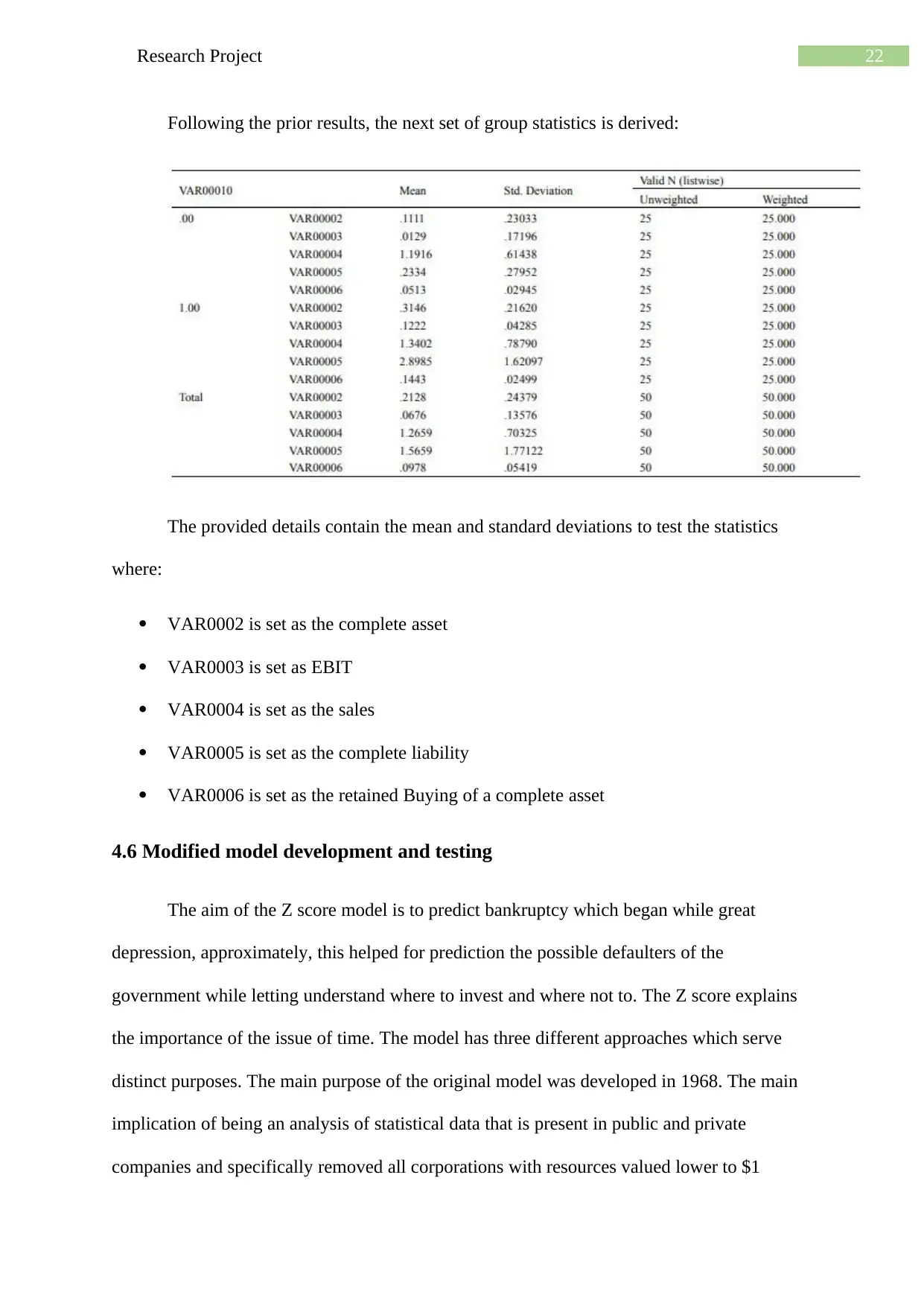
Following the prior results, the next set of group statistics is derived:
The provided details contain the mean and standard deviations to test the statistics
where:
VAR0002 is set as the complete asset
VAR0003 is set as EBIT
VAR0004 is set as the sales
VAR0005 is set as the complete liability
VAR0006 is set as the retained Buying of a complete asset
4.6 Modified model development and testing
The aim of the Z score model is to predict bankruptcy which began while great
depression, approximately, this helped for prediction the possible defaulters of the
government while letting understand where to invest and where not to. The Z score explains
the importance of the issue of time. The model has three different approaches which serve
distinct purposes. The main purpose of the original model was developed in 1968. The main
implication of being an analysis of statistical data that is present in public and private
companies and specifically removed all corporations with resources valued lower to $1
Paraphrase This Document
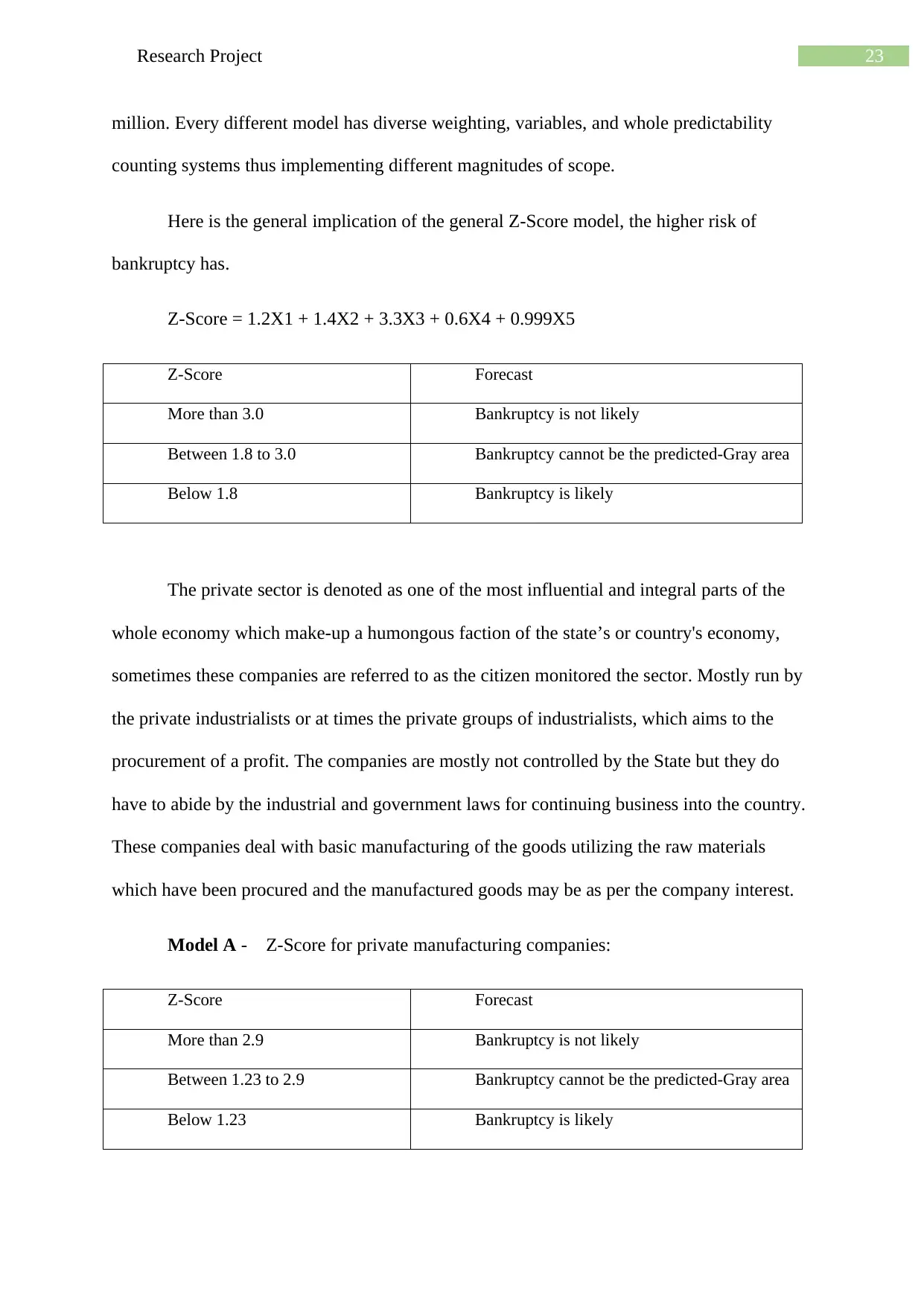
million. Every different model has diverse weighting, variables, and whole predictability
counting systems thus implementing different magnitudes of scope.
Here is the general implication of the general Z-Score model, the higher risk of
bankruptcy has.
Z-Score = 1.2X1 + 1.4X2 + 3.3X3 + 0.6X4 + 0.999X5
Z-Score Forecast
More than 3.0 Bankruptcy is not likely
Between 1.8 to 3.0 Bankruptcy cannot be the predicted-Gray area
Below 1.8 Bankruptcy is likely
The private sector is denoted as one of the most influential and integral parts of the
whole economy which make-up a humongous faction of the state’s or country's economy,
sometimes these companies are referred to as the citizen monitored the sector. Mostly run by
the private industrialists or at times the private groups of industrialists, which aims to the
procurement of a profit. The companies are mostly not controlled by the State but they do
have to abide by the industrial and government laws for continuing business into the country.
These companies deal with basic manufacturing of the goods utilizing the raw materials
which have been procured and the manufactured goods may be as per the company interest.
Model A - Z-Score for private manufacturing companies:
Z-Score Forecast
More than 2.9 Bankruptcy is not likely
Between 1.23 to 2.9 Bankruptcy cannot be the predicted-Gray area
Below 1.23 Bankruptcy is likely
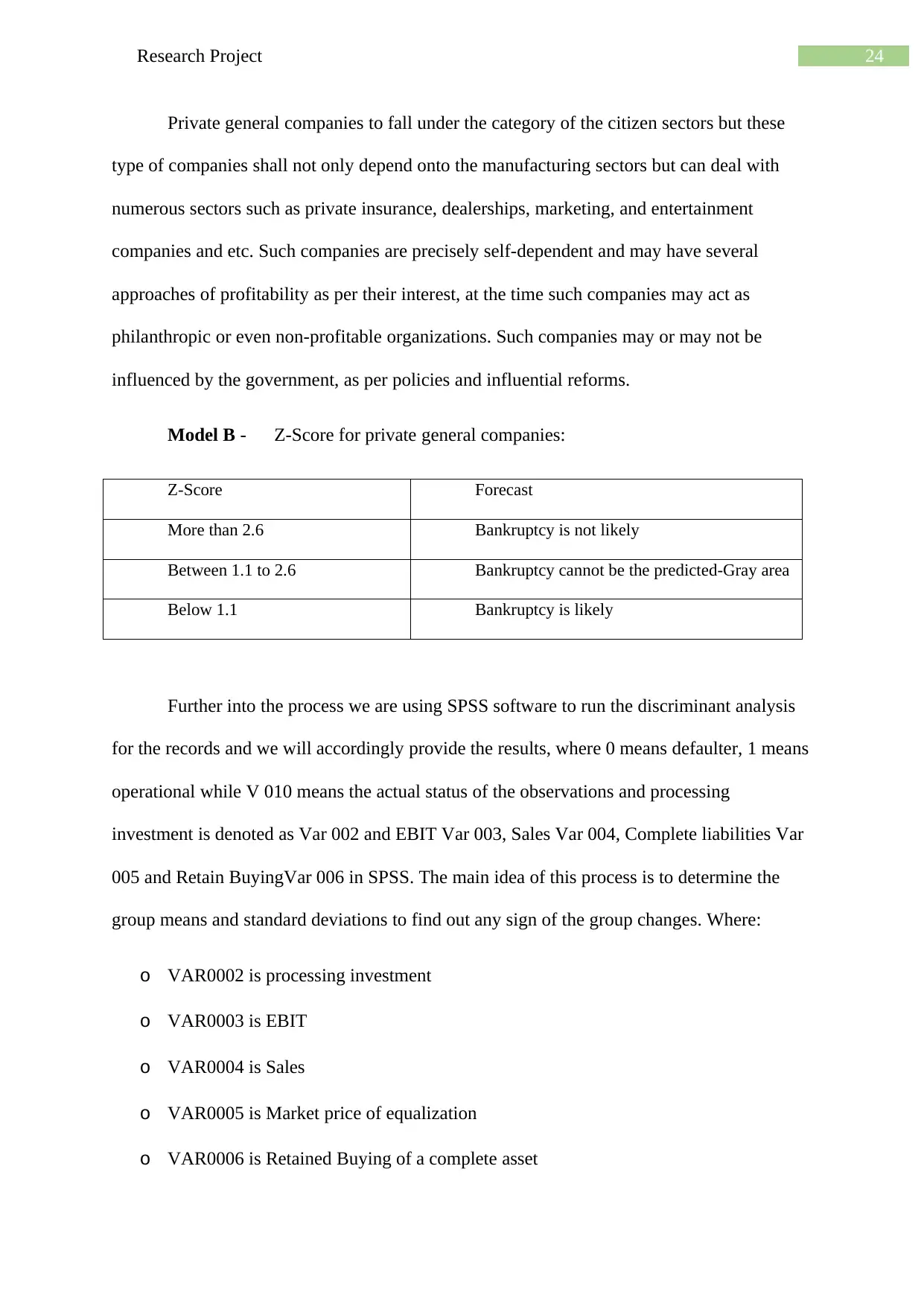
Private general companies to fall under the category of the citizen sectors but these
type of companies shall not only depend onto the manufacturing sectors but can deal with
numerous sectors such as private insurance, dealerships, marketing, and entertainment
companies and etc. Such companies are precisely self-dependent and may have several
approaches of profitability as per their interest, at the time such companies may act as
philanthropic or even non-profitable organizations. Such companies may or may not be
influenced by the government, as per policies and influential reforms.
Model B - Z-Score for private general companies:
Z-Score Forecast
More than 2.6 Bankruptcy is not likely
Between 1.1 to 2.6 Bankruptcy cannot be the predicted-Gray area
Below 1.1 Bankruptcy is likely
Further into the process we are using SPSS software to run the discriminant analysis
for the records and we will accordingly provide the results, where 0 means defaulter, 1 means
operational while V 010 means the actual status of the observations and processing
investment is denoted as Var 002 and EBIT Var 003, Sales Var 004, Complete liabilities Var
005 and Retain BuyingVar 006 in SPSS. The main idea of this process is to determine the
group means and standard deviations to find out any sign of the group changes. Where:
o VAR0002 is processing investment
o VAR0003 is EBIT
o VAR0004 is Sales
o VAR0005 is Market price of equalization
o VAR0006 is Retained Buying of a complete asset
⊘ This is a preview!⊘
Do you want full access?
Subscribe today to unlock all pages.

Trusted by 1+ million students worldwide
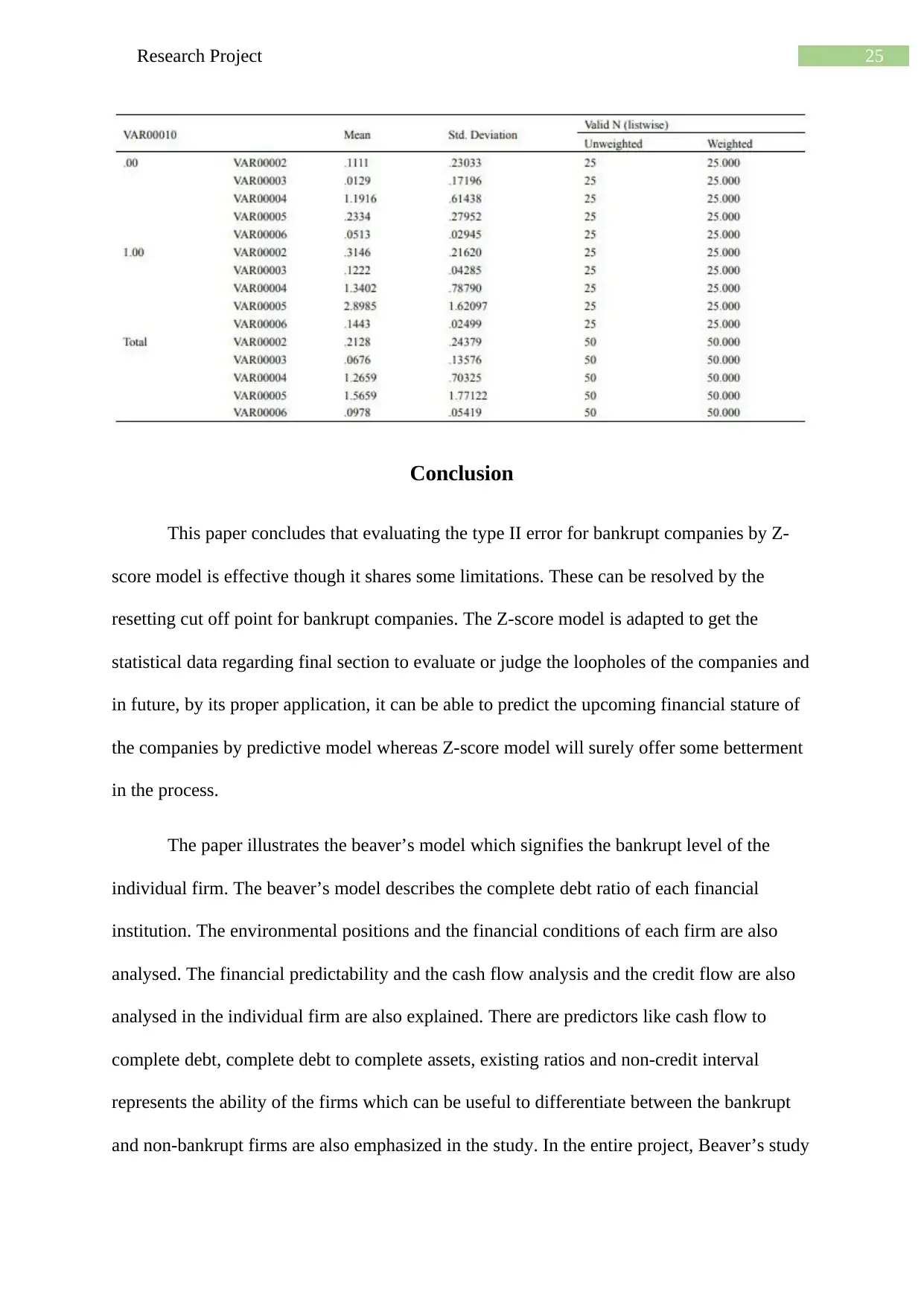
Conclusion
This paper concludes that evaluating the type II error for bankrupt companies by Z-
score model is effective though it shares some limitations. These can be resolved by the
resetting cut off point for bankrupt companies. The Z-score model is adapted to get the
statistical data regarding final section to evaluate or judge the loopholes of the companies and
in future, by its proper application, it can be able to predict the upcoming financial stature of
the companies by predictive model whereas Z-score model will surely offer some betterment
in the process.
The paper illustrates the beaver’s model which signifies the bankrupt level of the
individual firm. The beaver’s model describes the complete debt ratio of each financial
institution. The environmental positions and the financial conditions of each firm are also
analysed. The financial predictability and the cash flow analysis and the credit flow are also
analysed in the individual firm are also explained. There are predictors like cash flow to
complete debt, complete debt to complete assets, existing ratios and non-credit interval
represents the ability of the firms which can be useful to differentiate between the bankrupt
and non-bankrupt firms are also emphasized in the study. In the entire project, Beaver’s study
Paraphrase This Document
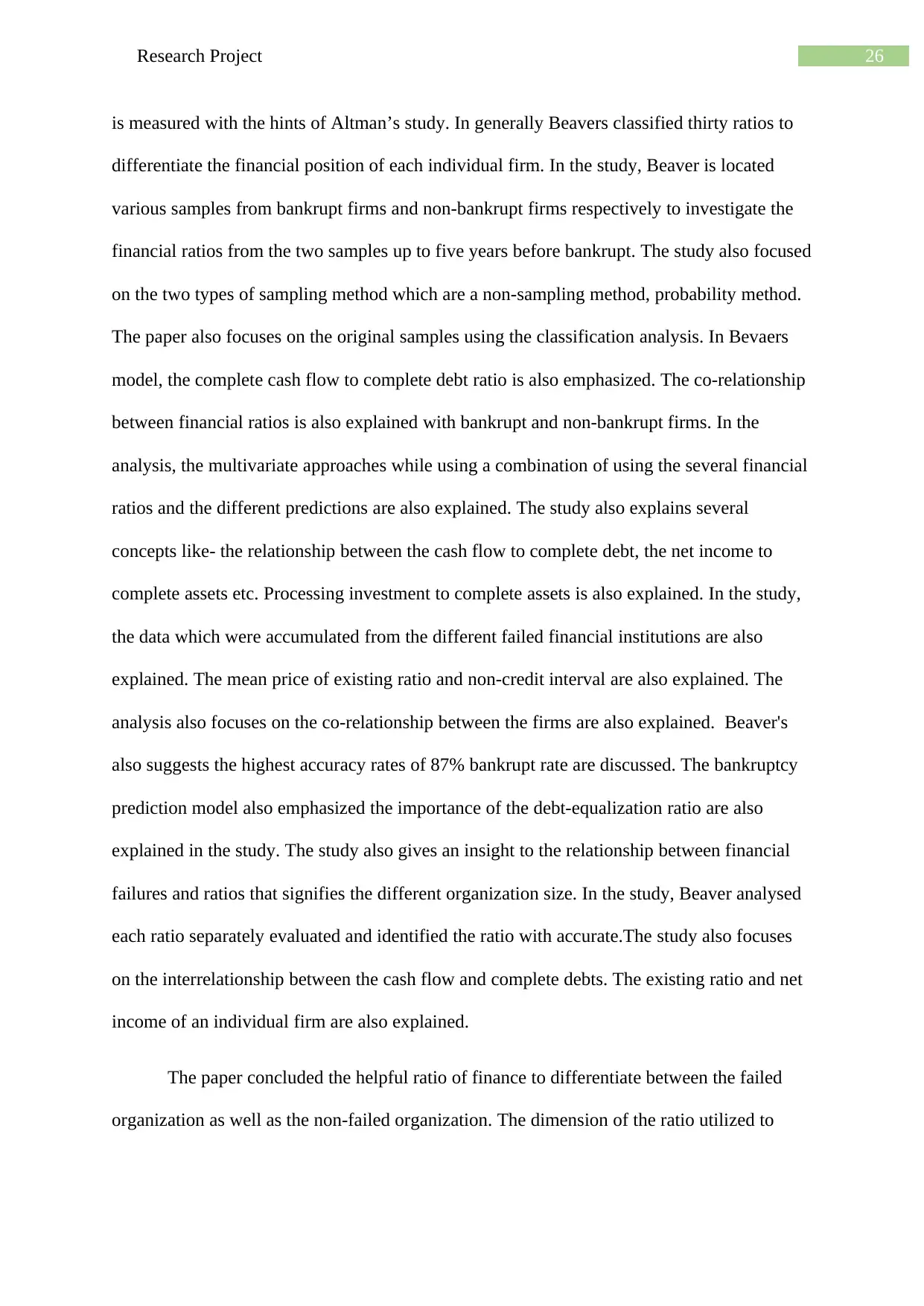
is measured with the hints of Altman’s study. In generally Beavers classified thirty ratios to
differentiate the financial position of each individual firm. In the study, Beaver is located
various samples from bankrupt firms and non-bankrupt firms respectively to investigate the
financial ratios from the two samples up to five years before bankrupt. The study also focused
on the two types of sampling method which are a non-sampling method, probability method.
The paper also focuses on the original samples using the classification analysis. In Bevaers
model, the complete cash flow to complete debt ratio is also emphasized. The co-relationship
between financial ratios is also explained with bankrupt and non-bankrupt firms. In the
analysis, the multivariate approaches while using a combination of using the several financial
ratios and the different predictions are also explained. The study also explains several
concepts like- the relationship between the cash flow to complete debt, the net income to
complete assets etc. Processing investment to complete assets is also explained. In the study,
the data which were accumulated from the different failed financial institutions are also
explained. The mean price of existing ratio and non-credit interval are also explained. The
analysis also focuses on the co-relationship between the firms are also explained. Beaver's
also suggests the highest accuracy rates of 87% bankrupt rate are discussed. The bankruptcy
prediction model also emphasized the importance of the debt-equalization ratio are also
explained in the study. The study also gives an insight to the relationship between financial
failures and ratios that signifies the different organization size. In the study, Beaver analysed
each ratio separately evaluated and identified the ratio with accurate.The study also focuses
on the interrelationship between the cash flow and complete debts. The existing ratio and net
income of an individual firm are also explained.
The paper concluded the helpful ratio of finance to differentiate between the failed
organization as well as the non-failed organization. The dimension of the ratio utilized to
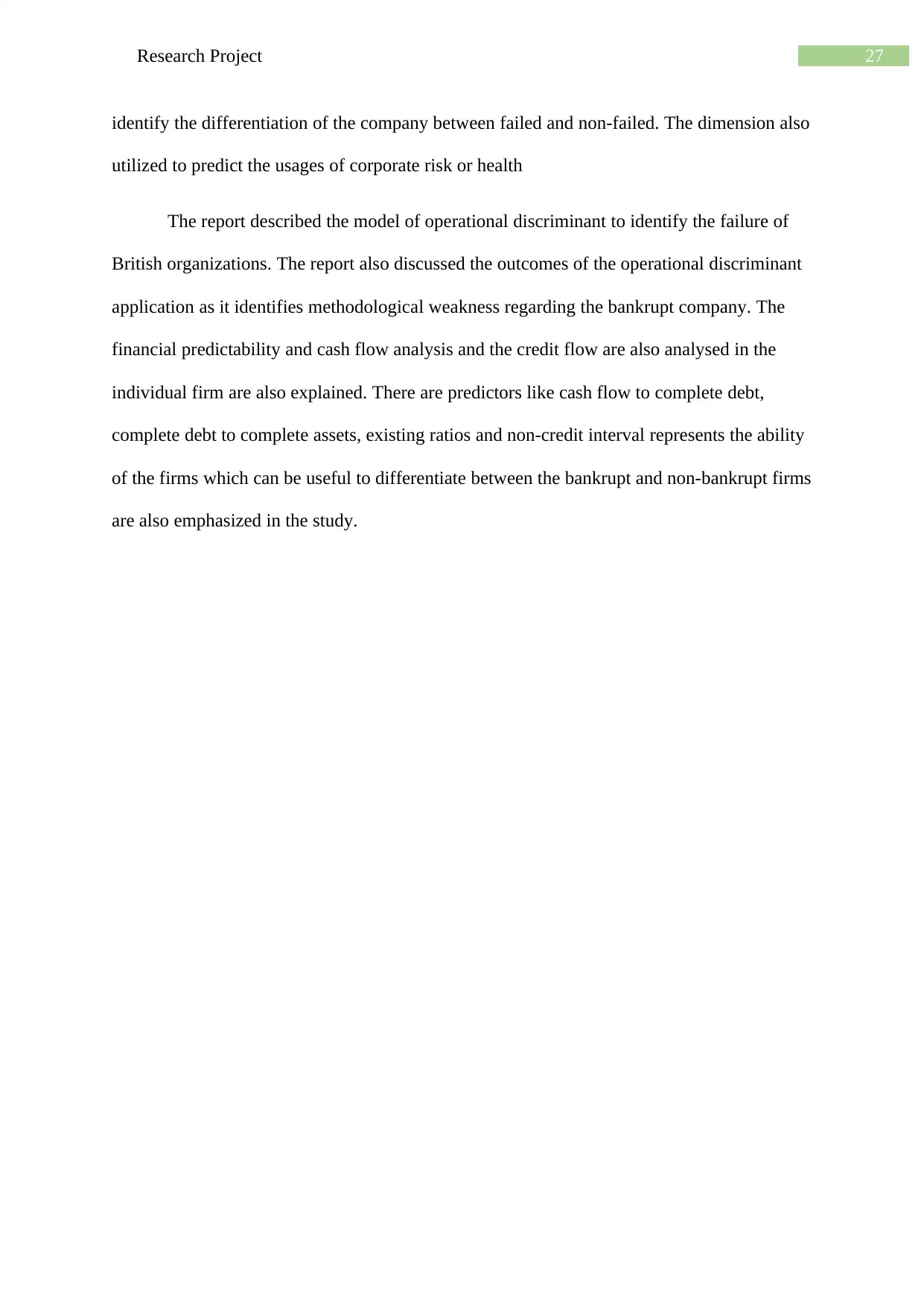
identify the differentiation of the company between failed and non-failed. The dimension also
utilized to predict the usages of corporate risk or health
The report described the model of operational discriminant to identify the failure of
British organizations. The report also discussed the outcomes of the operational discriminant
application as it identifies methodological weakness regarding the bankrupt company. The
financial predictability and cash flow analysis and the credit flow are also analysed in the
individual firm are also explained. There are predictors like cash flow to complete debt,
complete debt to complete assets, existing ratios and non-credit interval represents the ability
of the firms which can be useful to differentiate between the bankrupt and non-bankrupt firms
are also emphasized in the study.
⊘ This is a preview!⊘
Do you want full access?
Subscribe today to unlock all pages.

Trusted by 1+ million students worldwide
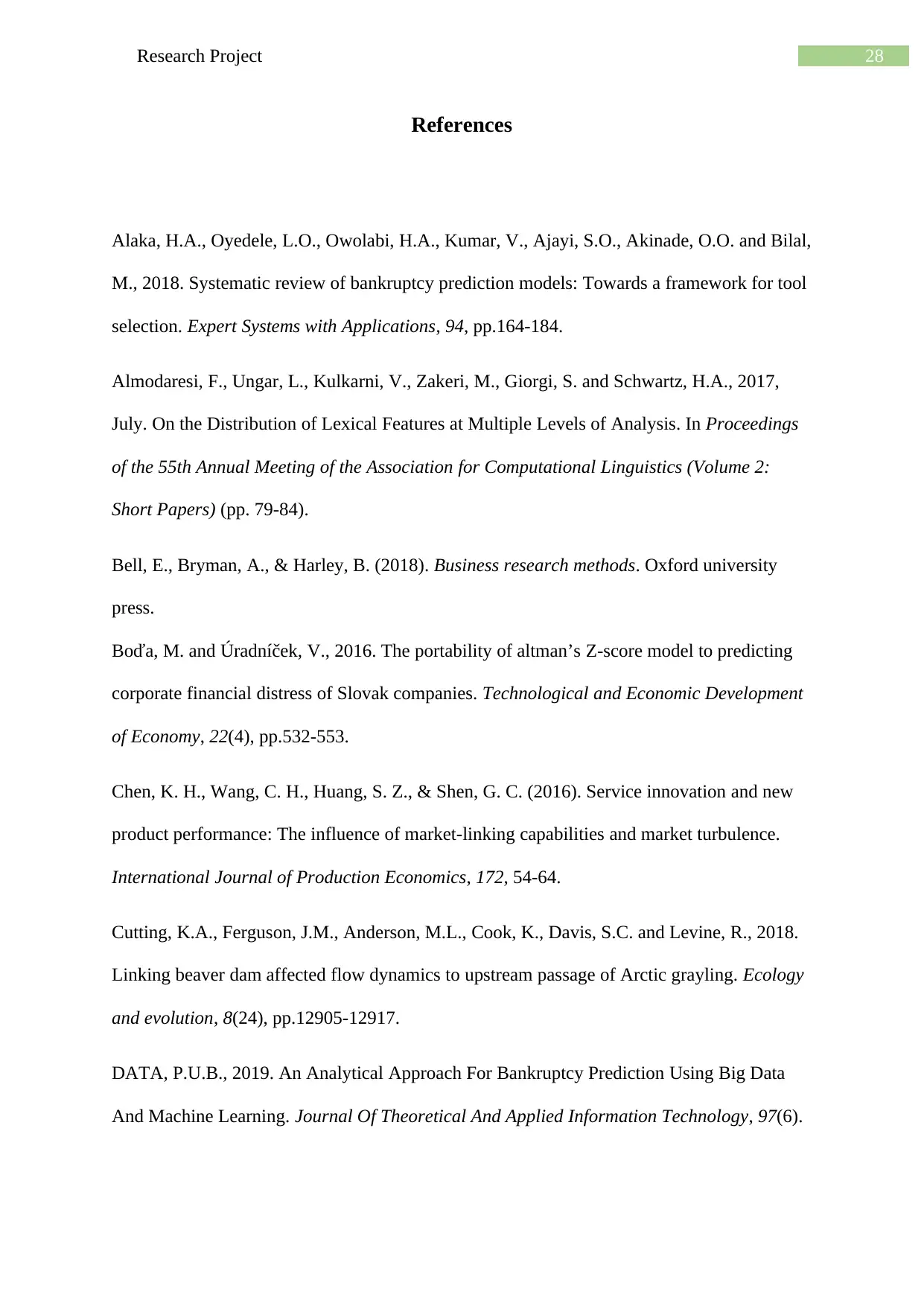
References
Alaka, H.A., Oyedele, L.O., Owolabi, H.A., Kumar, V., Ajayi, S.O., Akinade, O.O. and Bilal,
M., 2018. Systematic review of bankruptcy prediction models: Towards a framework for tool
selection. Expert Systems with Applications, 94, pp.164-184.
Almodaresi, F., Ungar, L., Kulkarni, V., Zakeri, M., Giorgi, S. and Schwartz, H.A., 2017,
July. On the Distribution of Lexical Features at Multiple Levels of Analysis. In Proceedings
of the 55th Annual Meeting of the Association for Computational Linguistics (Volume 2:
Short Papers) (pp. 79-84).
Bell, E., Bryman, A., & Harley, B. (2018). Business research methods. Oxford university
press.
Boďa, M. and Úradníček, V., 2016. The portability of altman’s Z-score model to predicting
corporate financial distress of Slovak companies. Technological and Economic Development
of Economy, 22(4), pp.532-553.
Chen, K. H., Wang, C. H., Huang, S. Z., & Shen, G. C. (2016). Service innovation and new
product performance: The influence of market-linking capabilities and market turbulence.
International Journal of Production Economics, 172, 54-64.
Cutting, K.A., Ferguson, J.M., Anderson, M.L., Cook, K., Davis, S.C. and Levine, R., 2018.
Linking beaver dam affected flow dynamics to upstream passage of Arctic grayling. Ecology
and evolution, 8(24), pp.12905-12917.
DATA, P.U.B., 2019. An Analytical Approach For Bankruptcy Prediction Using Big Data
And Machine Learning. Journal Of Theoretical And Applied Information Technology, 97(6).
Paraphrase This Document
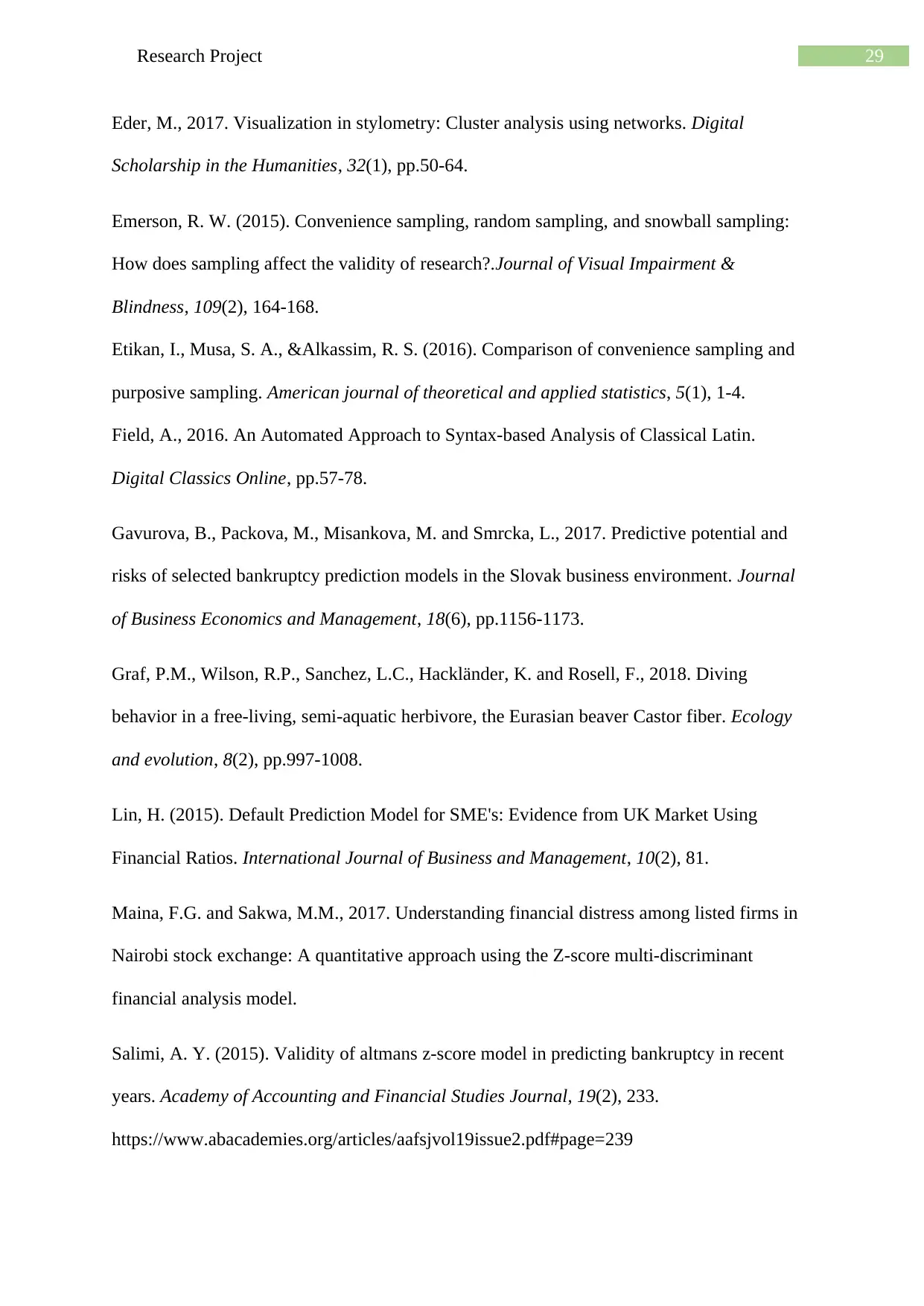
Eder, M., 2017. Visualization in stylometry: Cluster analysis using networks. Digital
Scholarship in the Humanities, 32(1), pp.50-64.
Emerson, R. W. (2015). Convenience sampling, random sampling, and snowball sampling:
How does sampling affect the validity of research?.Journal of Visual Impairment &
Blindness, 109(2), 164-168.
Etikan, I., Musa, S. A., &Alkassim, R. S. (2016). Comparison of convenience sampling and
purposive sampling. American journal of theoretical and applied statistics, 5(1), 1-4.
Field, A., 2016. An Automated Approach to Syntax-based Analysis of Classical Latin.
Digital Classics Online, pp.57-78.
Gavurova, B., Packova, M., Misankova, M. and Smrcka, L., 2017. Predictive potential and
risks of selected bankruptcy prediction models in the Slovak business environment. Journal
of Business Economics and Management, 18(6), pp.1156-1173.
Graf, P.M., Wilson, R.P., Sanchez, L.C., Hacklӓnder, K. and Rosell, F., 2018. Diving
behavior in a free‐living, semi‐aquatic herbivore, the Eurasian beaver Castor fiber. Ecology
and evolution, 8(2), pp.997-1008.
Lin, H. (2015). Default Prediction Model for SME's: Evidence from UK Market Using
Financial Ratios. International Journal of Business and Management, 10(2), 81.
Maina, F.G. and Sakwa, M.M., 2017. Understanding financial distress among listed firms in
Nairobi stock exchange: A quantitative approach using the Z-score multi-discriminant
financial analysis model.
Salimi, A. Y. (2015). Validity of altmans z-score model in predicting bankruptcy in recent
years. Academy of Accounting and Financial Studies Journal, 19(2), 233.
https://www.abacademies.org/articles/aafsjvol19issue2.pdf#page=239
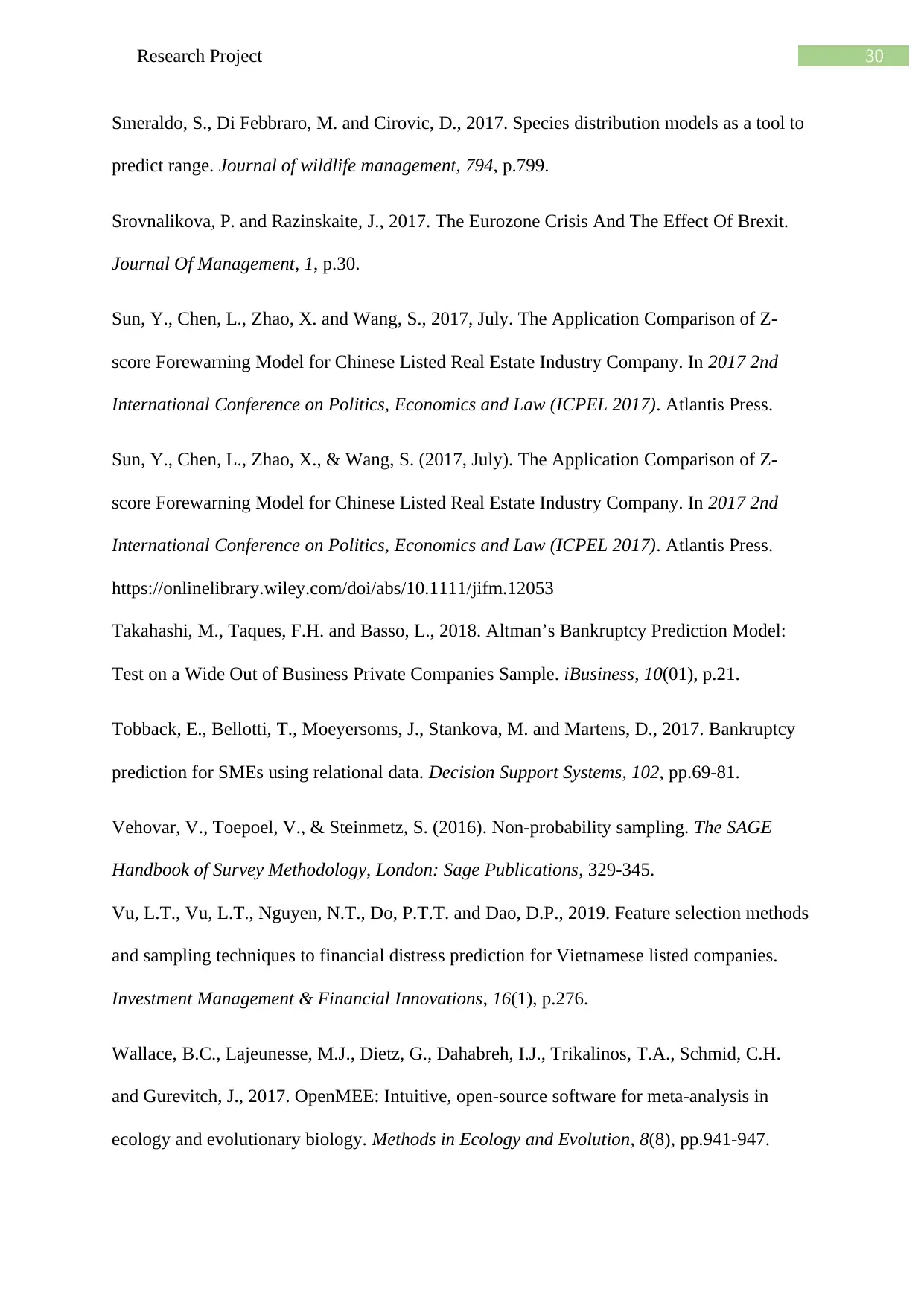
Smeraldo, S., Di Febbraro, M. and Cirovic, D., 2017. Species distribution models as a tool to
predict range. Journal of wildlife management, 794, p.799.
Srovnalikova, P. and Razinskaite, J., 2017. The Eurozone Crisis And The Effect Of Brexit.
Journal Of Management, 1, p.30.
Sun, Y., Chen, L., Zhao, X. and Wang, S., 2017, July. The Application Comparison of Z-
score Forewarning Model for Chinese Listed Real Estate Industry Company. In 2017 2nd
International Conference on Politics, Economics and Law (ICPEL 2017). Atlantis Press.
Sun, Y., Chen, L., Zhao, X., & Wang, S. (2017, July). The Application Comparison of Z-
score Forewarning Model for Chinese Listed Real Estate Industry Company. In 2017 2nd
International Conference on Politics, Economics and Law (ICPEL 2017). Atlantis Press.
https://onlinelibrary.wiley.com/doi/abs/10.1111/jifm.12053
Takahashi, M., Taques, F.H. and Basso, L., 2018. Altman’s Bankruptcy Prediction Model:
Test on a Wide Out of Business Private Companies Sample. iBusiness, 10(01), p.21.
Tobback, E., Bellotti, T., Moeyersoms, J., Stankova, M. and Martens, D., 2017. Bankruptcy
prediction for SMEs using relational data. Decision Support Systems, 102, pp.69-81.
Vehovar, V., Toepoel, V., & Steinmetz, S. (2016). Non-probability sampling. The SAGE
Handbook of Survey Methodology, London: Sage Publications, 329-345.
Vu, L.T., Vu, L.T., Nguyen, N.T., Do, P.T.T. and Dao, D.P., 2019. Feature selection methods
and sampling techniques to financial distress prediction for Vietnamese listed companies.
Investment Management & Financial Innovations, 16(1), p.276.
Wallace, B.C., Lajeunesse, M.J., Dietz, G., Dahabreh, I.J., Trikalinos, T.A., Schmid, C.H.
and Gurevitch, J., 2017. OpenMEE: Intuitive, open‐source software for meta‐analysis in
ecology and evolutionary biology. Methods in Ecology and Evolution, 8(8), pp.941-947.
⊘ This is a preview!⊘
Do you want full access?
Subscribe today to unlock all pages.

Trusted by 1+ million students worldwide
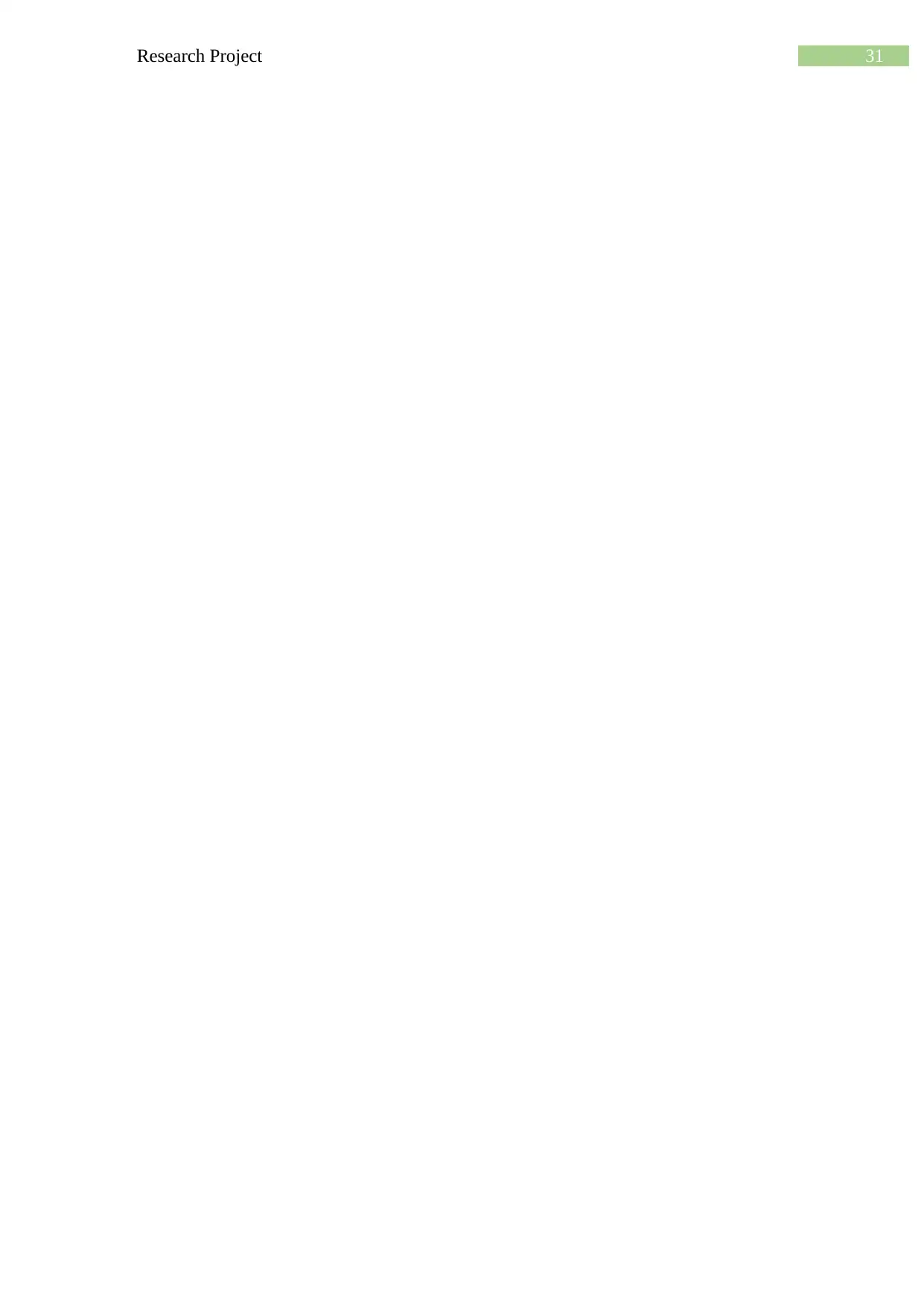
Related Documents

Your All-in-One AI-Powered Toolkit for Academic Success.
+13062052269
info@desklib.com
Available 24*7 on WhatsApp / Email
© 2024 | Zucol Services PVT LTD | All rights reserved.