Reservoir Yield Assessment and Modelling for Water Supply Management
VerifiedAdded on 2023/06/15
|12
|3701
|122
AI Summary
This report discusses the development of a model for reservoir management and evaluation of maximum historical yield. It also analyzes the implications of future increase in demand and climate change on the reservoir performance. The report suggests preventive measures to reduce the vulnerability of water resource system to climate changes.
Contribute Materials
Your contribution can guide someone’s learning journey. Share your
documents today.
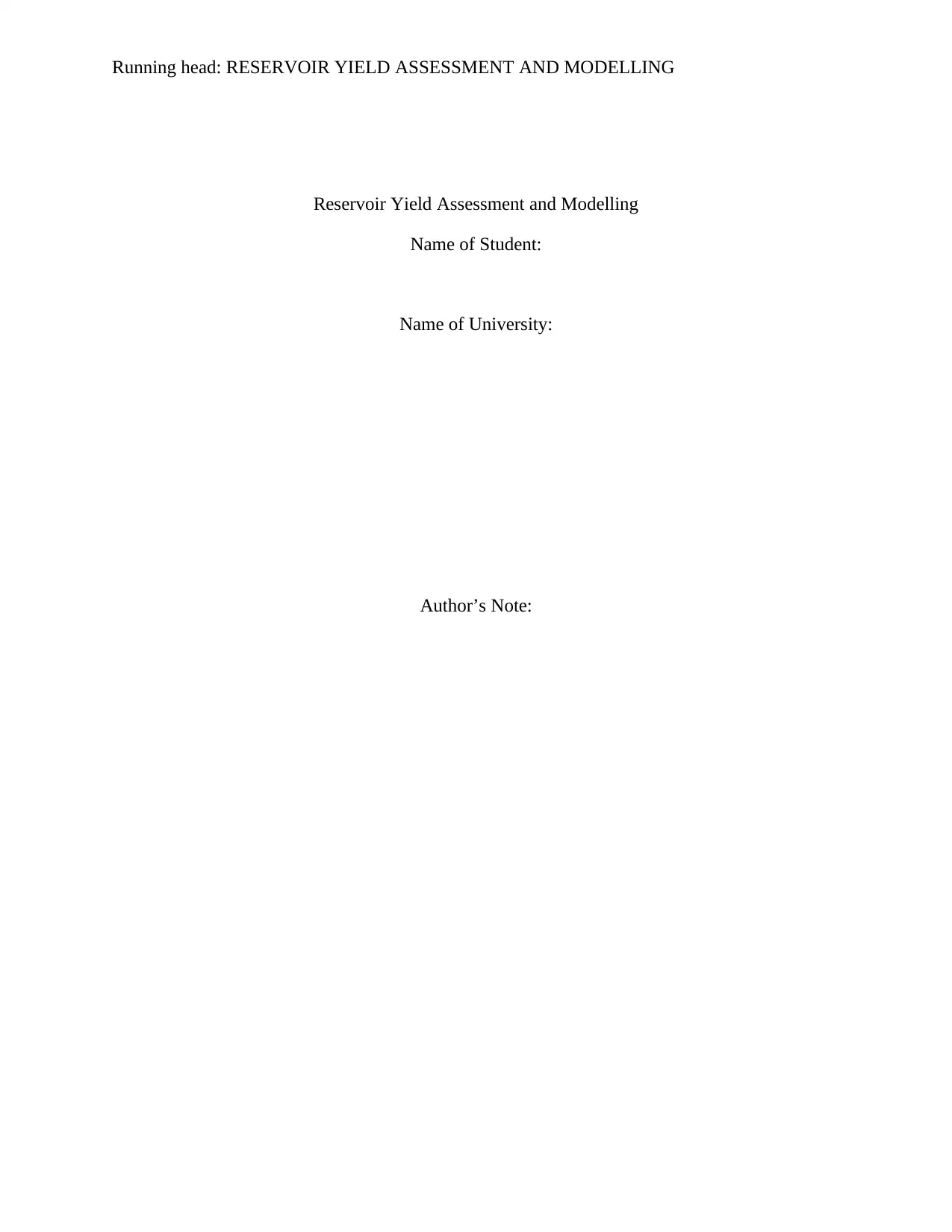
Running head: RESERVOIR YIELD ASSESSMENT AND MODELLING
Reservoir Yield Assessment and Modelling
Name of Student:
Name of University:
Author’s Note:
Reservoir Yield Assessment and Modelling
Name of Student:
Name of University:
Author’s Note:
Secure Best Marks with AI Grader
Need help grading? Try our AI Grader for instant feedback on your assignments.
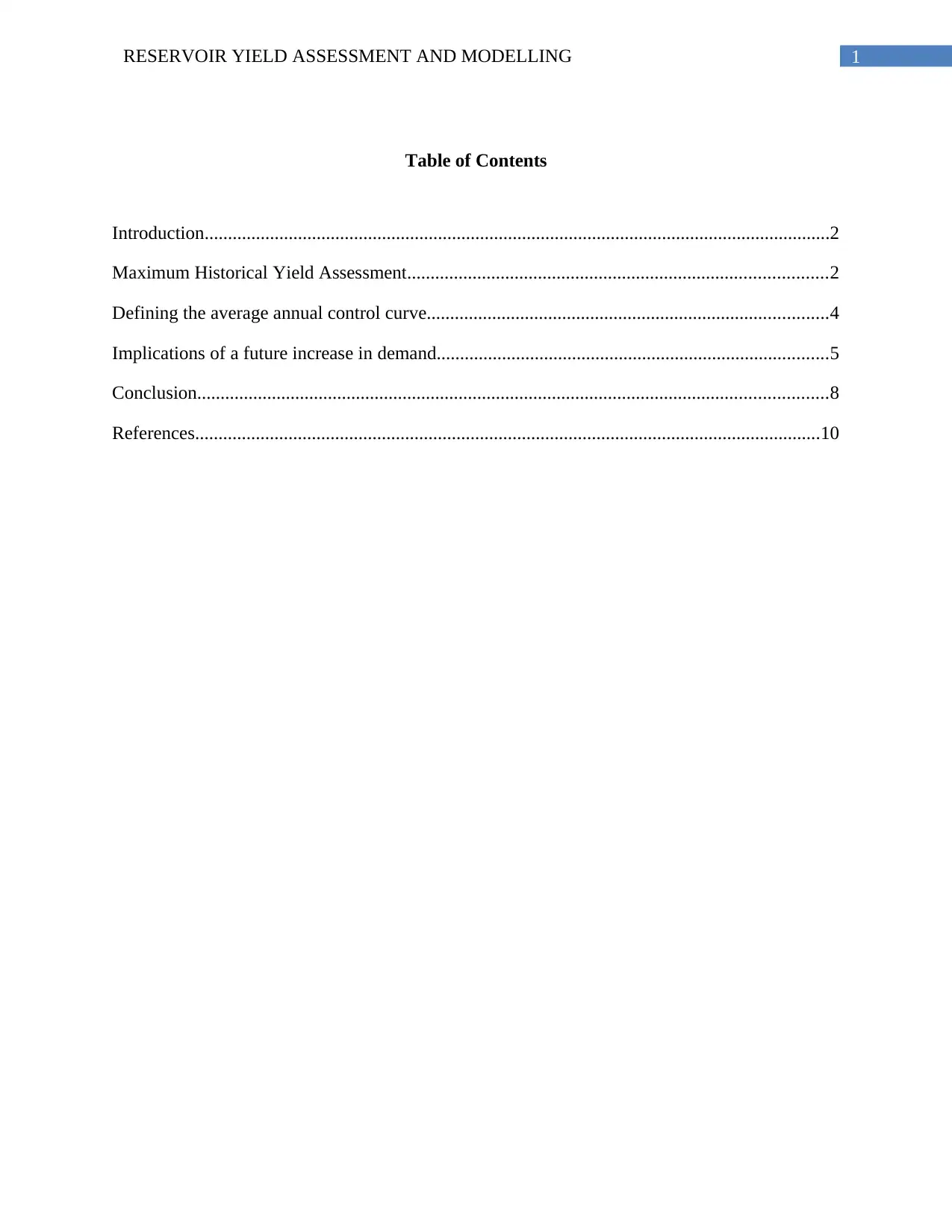
1RESERVOIR YIELD ASSESSMENT AND MODELLING
Table of Contents
Introduction......................................................................................................................................2
Maximum Historical Yield Assessment..........................................................................................2
Defining the average annual control curve......................................................................................4
Implications of a future increase in demand....................................................................................5
Conclusion.......................................................................................................................................8
References......................................................................................................................................10
Table of Contents
Introduction......................................................................................................................................2
Maximum Historical Yield Assessment..........................................................................................2
Defining the average annual control curve......................................................................................4
Implications of a future increase in demand....................................................................................5
Conclusion.......................................................................................................................................8
References......................................................................................................................................10
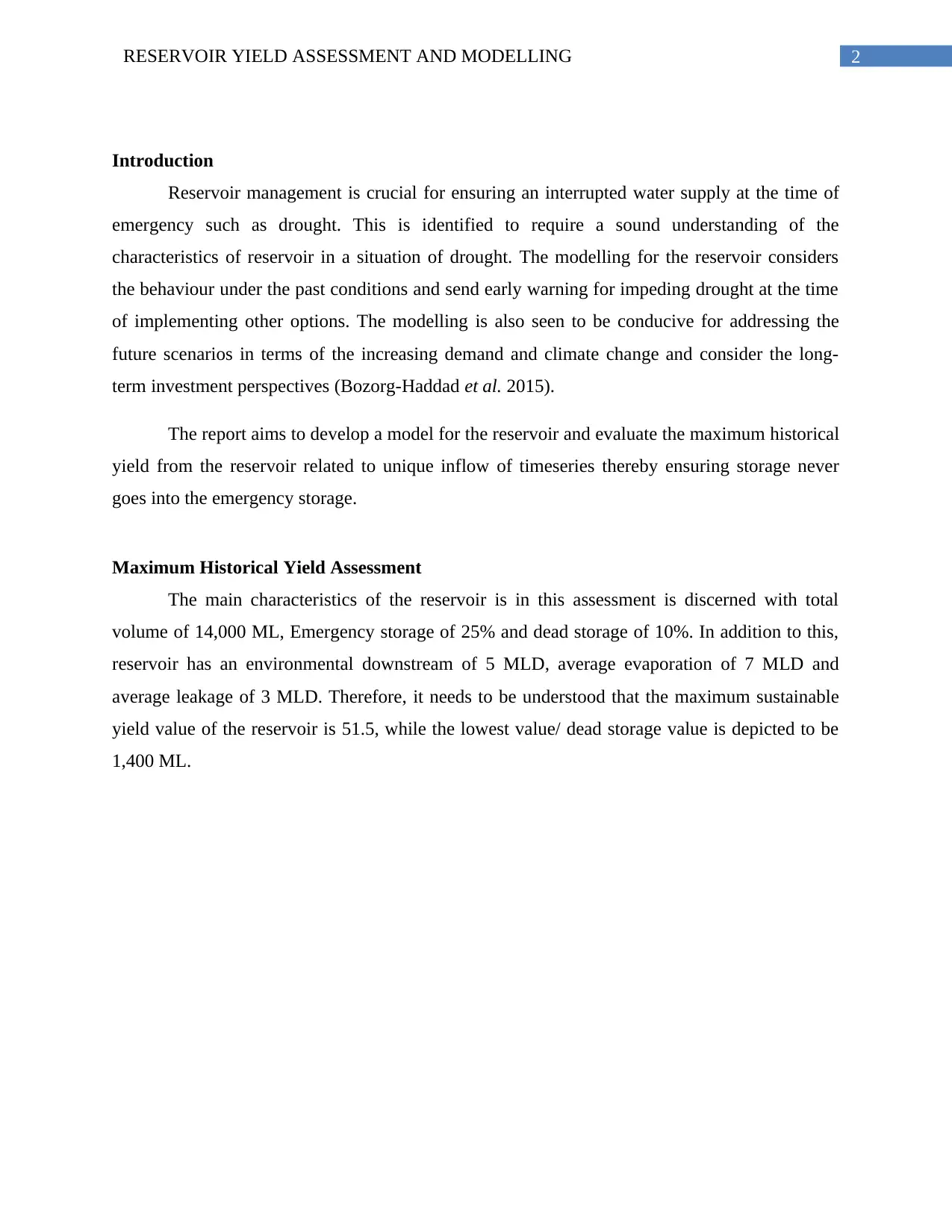
2RESERVOIR YIELD ASSESSMENT AND MODELLING
Introduction
Reservoir management is crucial for ensuring an interrupted water supply at the time of
emergency such as drought. This is identified to require a sound understanding of the
characteristics of reservoir in a situation of drought. The modelling for the reservoir considers
the behaviour under the past conditions and send early warning for impeding drought at the time
of implementing other options. The modelling is also seen to be conducive for addressing the
future scenarios in terms of the increasing demand and climate change and consider the long-
term investment perspectives (Bozorg-Haddad et al. 2015).
The report aims to develop a model for the reservoir and evaluate the maximum historical
yield from the reservoir related to unique inflow of timeseries thereby ensuring storage never
goes into the emergency storage.
Maximum Historical Yield Assessment
The main characteristics of the reservoir is in this assessment is discerned with total
volume of 14,000 ML, Emergency storage of 25% and dead storage of 10%. In addition to this,
reservoir has an environmental downstream of 5 MLD, average evaporation of 7 MLD and
average leakage of 3 MLD. Therefore, it needs to be understood that the maximum sustainable
yield value of the reservoir is 51.5, while the lowest value/ dead storage value is depicted to be
1,400 ML.
Introduction
Reservoir management is crucial for ensuring an interrupted water supply at the time of
emergency such as drought. This is identified to require a sound understanding of the
characteristics of reservoir in a situation of drought. The modelling for the reservoir considers
the behaviour under the past conditions and send early warning for impeding drought at the time
of implementing other options. The modelling is also seen to be conducive for addressing the
future scenarios in terms of the increasing demand and climate change and consider the long-
term investment perspectives (Bozorg-Haddad et al. 2015).
The report aims to develop a model for the reservoir and evaluate the maximum historical
yield from the reservoir related to unique inflow of timeseries thereby ensuring storage never
goes into the emergency storage.
Maximum Historical Yield Assessment
The main characteristics of the reservoir is in this assessment is discerned with total
volume of 14,000 ML, Emergency storage of 25% and dead storage of 10%. In addition to this,
reservoir has an environmental downstream of 5 MLD, average evaporation of 7 MLD and
average leakage of 3 MLD. Therefore, it needs to be understood that the maximum sustainable
yield value of the reservoir is 51.5, while the lowest value/ dead storage value is depicted to be
1,400 ML.
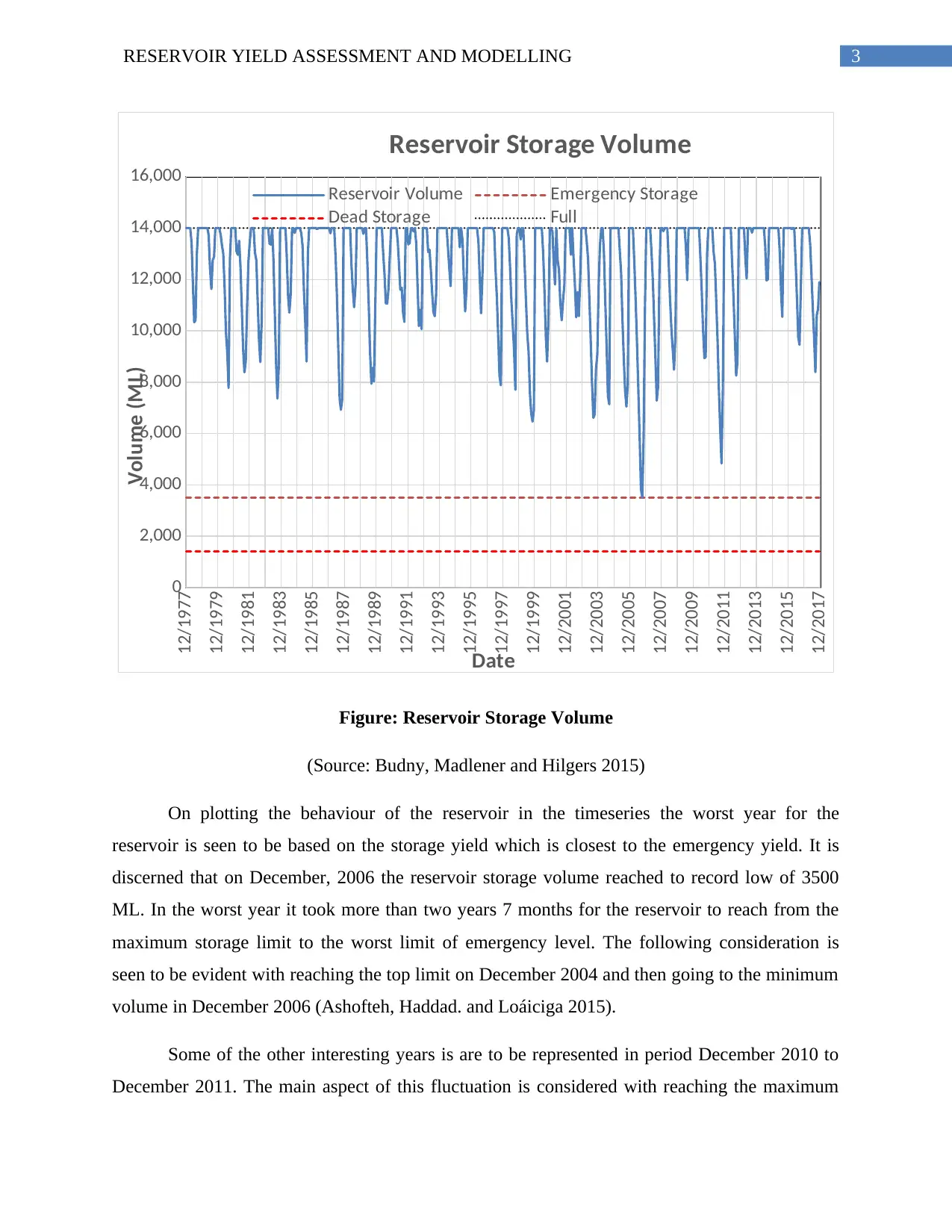
3RESERVOIR YIELD ASSESSMENT AND MODELLING
12/1977
12/1979
12/1981
12/1983
12/1985
12/1987
12/1989
12/1991
12/1993
12/1995
12/1997
12/1999
12/2001
12/2003
12/2005
12/2007
12/2009
12/2011
12/2013
12/2015
12/20170
2,000
4,000
6,000
8,000
10,000
12,000
14,000
16,000
Reservoir Storage Volume
Reservoir Volume Emergency Storage
Dead Storage Full
Date
Volume (ML)
Figure: Reservoir Storage Volume
(Source: Budny, Madlener and Hilgers 2015)
On plotting the behaviour of the reservoir in the timeseries the worst year for the
reservoir is seen to be based on the storage yield which is closest to the emergency yield. It is
discerned that on December, 2006 the reservoir storage volume reached to record low of 3500
ML. In the worst year it took more than two years 7 months for the reservoir to reach from the
maximum storage limit to the worst limit of emergency level. The following consideration is
seen to be evident with reaching the top limit on December 2004 and then going to the minimum
volume in December 2006 (Ashofteh, Haddad. and Loáiciga 2015).
Some of the other interesting years is are to be represented in period December 2010 to
December 2011. The main aspect of this fluctuation is considered with reaching the maximum
12/1977
12/1979
12/1981
12/1983
12/1985
12/1987
12/1989
12/1991
12/1993
12/1995
12/1997
12/1999
12/2001
12/2003
12/2005
12/2007
12/2009
12/2011
12/2013
12/2015
12/20170
2,000
4,000
6,000
8,000
10,000
12,000
14,000
16,000
Reservoir Storage Volume
Reservoir Volume Emergency Storage
Dead Storage Full
Date
Volume (ML)
Figure: Reservoir Storage Volume
(Source: Budny, Madlener and Hilgers 2015)
On plotting the behaviour of the reservoir in the timeseries the worst year for the
reservoir is seen to be based on the storage yield which is closest to the emergency yield. It is
discerned that on December, 2006 the reservoir storage volume reached to record low of 3500
ML. In the worst year it took more than two years 7 months for the reservoir to reach from the
maximum storage limit to the worst limit of emergency level. The following consideration is
seen to be evident with reaching the top limit on December 2004 and then going to the minimum
volume in December 2006 (Ashofteh, Haddad. and Loáiciga 2015).
Some of the other interesting years is are to be represented in period December 2010 to
December 2011. The main aspect of this fluctuation is considered with reaching the maximum
Secure Best Marks with AI Grader
Need help grading? Try our AI Grader for instant feedback on your assignments.
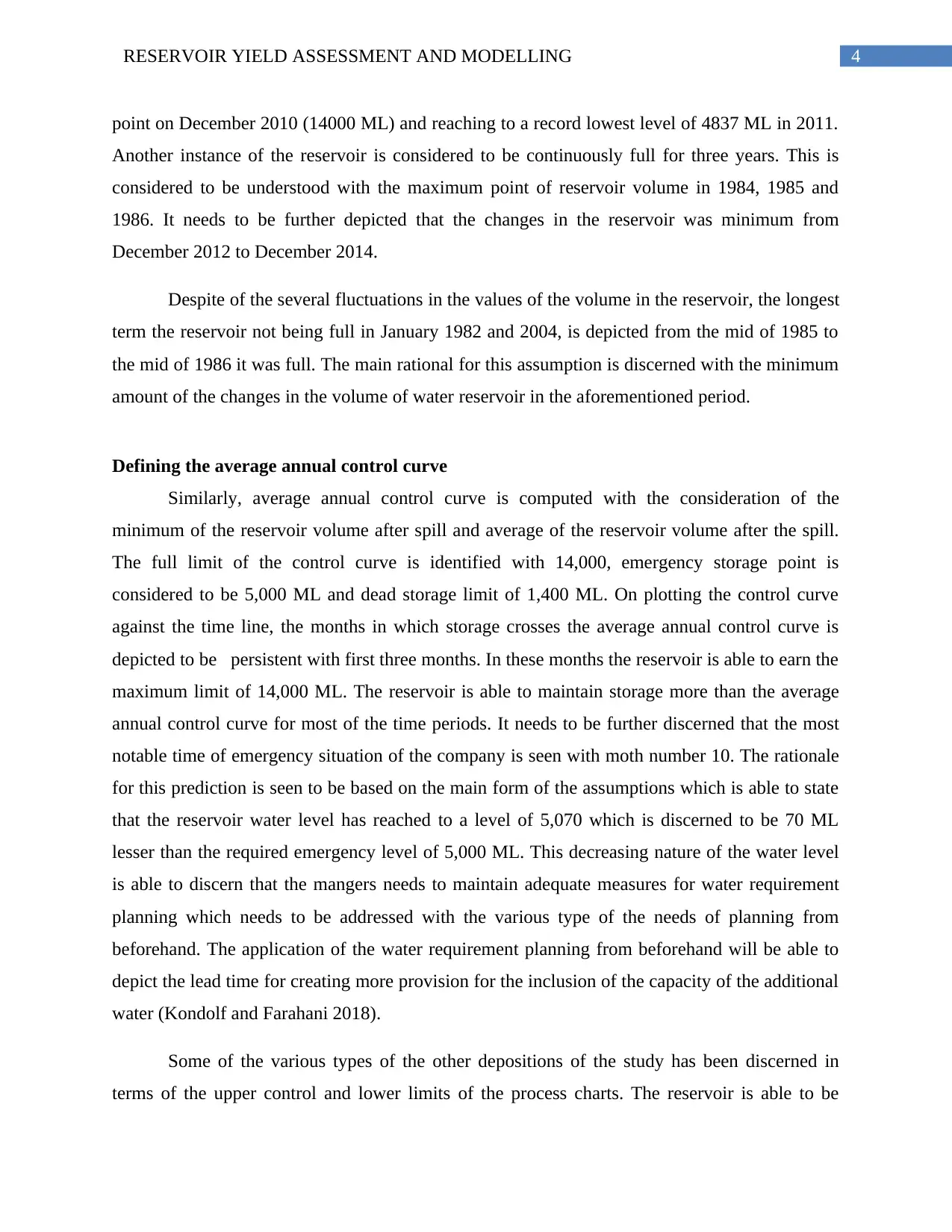
4RESERVOIR YIELD ASSESSMENT AND MODELLING
point on December 2010 (14000 ML) and reaching to a record lowest level of 4837 ML in 2011.
Another instance of the reservoir is considered to be continuously full for three years. This is
considered to be understood with the maximum point of reservoir volume in 1984, 1985 and
1986. It needs to be further depicted that the changes in the reservoir was minimum from
December 2012 to December 2014.
Despite of the several fluctuations in the values of the volume in the reservoir, the longest
term the reservoir not being full in January 1982 and 2004, is depicted from the mid of 1985 to
the mid of 1986 it was full. The main rational for this assumption is discerned with the minimum
amount of the changes in the volume of water reservoir in the aforementioned period.
Defining the average annual control curve
Similarly, average annual control curve is computed with the consideration of the
minimum of the reservoir volume after spill and average of the reservoir volume after the spill.
The full limit of the control curve is identified with 14,000, emergency storage point is
considered to be 5,000 ML and dead storage limit of 1,400 ML. On plotting the control curve
against the time line, the months in which storage crosses the average annual control curve is
depicted to be persistent with first three months. In these months the reservoir is able to earn the
maximum limit of 14,000 ML. The reservoir is able to maintain storage more than the average
annual control curve for most of the time periods. It needs to be further discerned that the most
notable time of emergency situation of the company is seen with moth number 10. The rationale
for this prediction is seen to be based on the main form of the assumptions which is able to state
that the reservoir water level has reached to a level of 5,070 which is discerned to be 70 ML
lesser than the required emergency level of 5,000 ML. This decreasing nature of the water level
is able to discern that the mangers needs to maintain adequate measures for water requirement
planning which needs to be addressed with the various type of the needs of planning from
beforehand. The application of the water requirement planning from beforehand will be able to
depict the lead time for creating more provision for the inclusion of the capacity of the additional
water (Kondolf and Farahani 2018).
Some of the various types of the other depositions of the study has been discerned in
terms of the upper control and lower limits of the process charts. The reservoir is able to be
point on December 2010 (14000 ML) and reaching to a record lowest level of 4837 ML in 2011.
Another instance of the reservoir is considered to be continuously full for three years. This is
considered to be understood with the maximum point of reservoir volume in 1984, 1985 and
1986. It needs to be further depicted that the changes in the reservoir was minimum from
December 2012 to December 2014.
Despite of the several fluctuations in the values of the volume in the reservoir, the longest
term the reservoir not being full in January 1982 and 2004, is depicted from the mid of 1985 to
the mid of 1986 it was full. The main rational for this assumption is discerned with the minimum
amount of the changes in the volume of water reservoir in the aforementioned period.
Defining the average annual control curve
Similarly, average annual control curve is computed with the consideration of the
minimum of the reservoir volume after spill and average of the reservoir volume after the spill.
The full limit of the control curve is identified with 14,000, emergency storage point is
considered to be 5,000 ML and dead storage limit of 1,400 ML. On plotting the control curve
against the time line, the months in which storage crosses the average annual control curve is
depicted to be persistent with first three months. In these months the reservoir is able to earn the
maximum limit of 14,000 ML. The reservoir is able to maintain storage more than the average
annual control curve for most of the time periods. It needs to be further discerned that the most
notable time of emergency situation of the company is seen with moth number 10. The rationale
for this prediction is seen to be based on the main form of the assumptions which is able to state
that the reservoir water level has reached to a level of 5,070 which is discerned to be 70 ML
lesser than the required emergency level of 5,000 ML. This decreasing nature of the water level
is able to discern that the mangers needs to maintain adequate measures for water requirement
planning which needs to be addressed with the various type of the needs of planning from
beforehand. The application of the water requirement planning from beforehand will be able to
depict the lead time for creating more provision for the inclusion of the capacity of the additional
water (Kondolf and Farahani 2018).
Some of the various types of the other depositions of the study has been discerned in
terms of the upper control and lower limits of the process charts. The reservoir is able to be
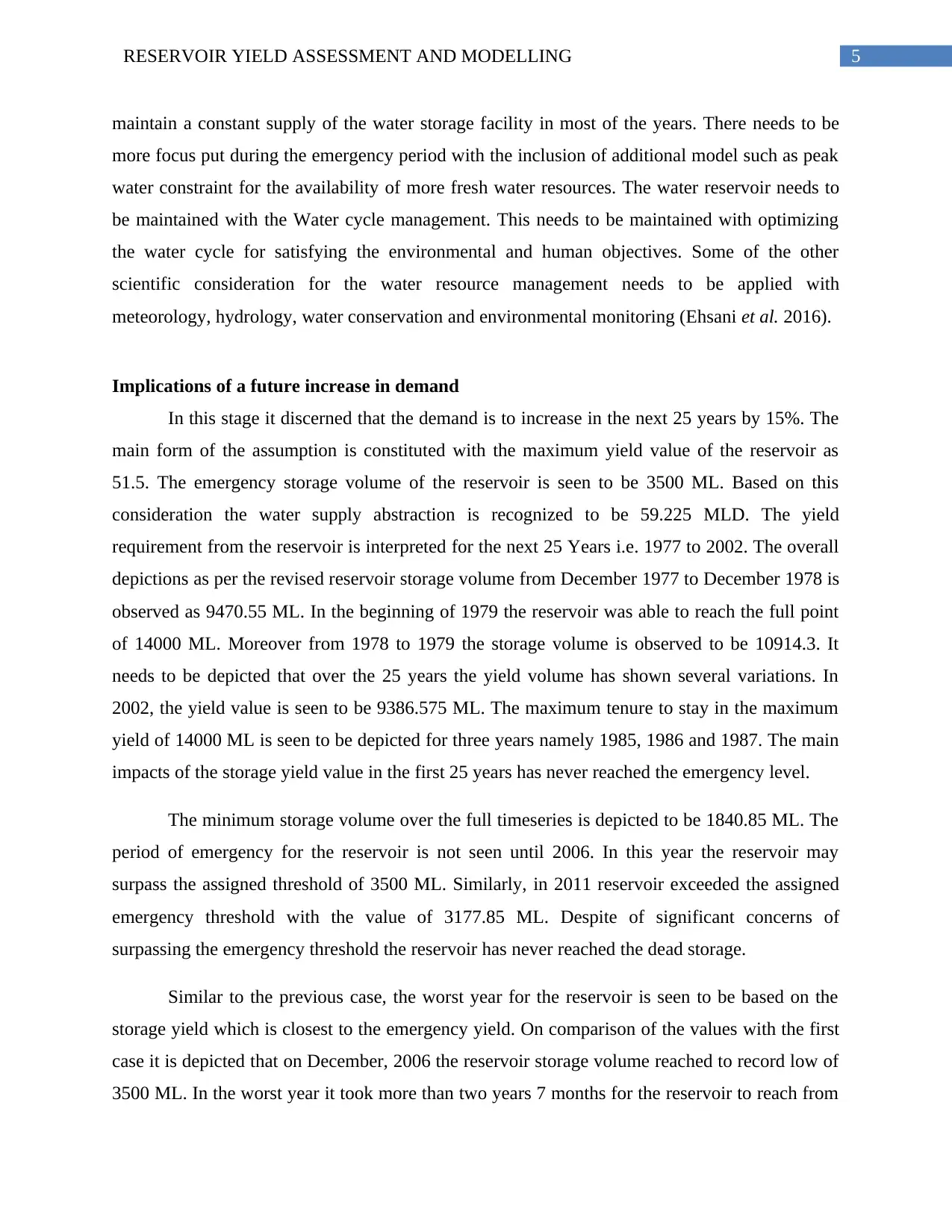
5RESERVOIR YIELD ASSESSMENT AND MODELLING
maintain a constant supply of the water storage facility in most of the years. There needs to be
more focus put during the emergency period with the inclusion of additional model such as peak
water constraint for the availability of more fresh water resources. The water reservoir needs to
be maintained with the Water cycle management. This needs to be maintained with optimizing
the water cycle for satisfying the environmental and human objectives. Some of the other
scientific consideration for the water resource management needs to be applied with
meteorology, hydrology, water conservation and environmental monitoring (Ehsani et al. 2016).
Implications of a future increase in demand
In this stage it discerned that the demand is to increase in the next 25 years by 15%. The
main form of the assumption is constituted with the maximum yield value of the reservoir as
51.5. The emergency storage volume of the reservoir is seen to be 3500 ML. Based on this
consideration the water supply abstraction is recognized to be 59.225 MLD. The yield
requirement from the reservoir is interpreted for the next 25 Years i.e. 1977 to 2002. The overall
depictions as per the revised reservoir storage volume from December 1977 to December 1978 is
observed as 9470.55 ML. In the beginning of 1979 the reservoir was able to reach the full point
of 14000 ML. Moreover from 1978 to 1979 the storage volume is observed to be 10914.3. It
needs to be depicted that over the 25 years the yield volume has shown several variations. In
2002, the yield value is seen to be 9386.575 ML. The maximum tenure to stay in the maximum
yield of 14000 ML is seen to be depicted for three years namely 1985, 1986 and 1987. The main
impacts of the storage yield value in the first 25 years has never reached the emergency level.
The minimum storage volume over the full timeseries is depicted to be 1840.85 ML. The
period of emergency for the reservoir is not seen until 2006. In this year the reservoir may
surpass the assigned threshold of 3500 ML. Similarly, in 2011 reservoir exceeded the assigned
emergency threshold with the value of 3177.85 ML. Despite of significant concerns of
surpassing the emergency threshold the reservoir has never reached the dead storage.
Similar to the previous case, the worst year for the reservoir is seen to be based on the
storage yield which is closest to the emergency yield. On comparison of the values with the first
case it is depicted that on December, 2006 the reservoir storage volume reached to record low of
3500 ML. In the worst year it took more than two years 7 months for the reservoir to reach from
maintain a constant supply of the water storage facility in most of the years. There needs to be
more focus put during the emergency period with the inclusion of additional model such as peak
water constraint for the availability of more fresh water resources. The water reservoir needs to
be maintained with the Water cycle management. This needs to be maintained with optimizing
the water cycle for satisfying the environmental and human objectives. Some of the other
scientific consideration for the water resource management needs to be applied with
meteorology, hydrology, water conservation and environmental monitoring (Ehsani et al. 2016).
Implications of a future increase in demand
In this stage it discerned that the demand is to increase in the next 25 years by 15%. The
main form of the assumption is constituted with the maximum yield value of the reservoir as
51.5. The emergency storage volume of the reservoir is seen to be 3500 ML. Based on this
consideration the water supply abstraction is recognized to be 59.225 MLD. The yield
requirement from the reservoir is interpreted for the next 25 Years i.e. 1977 to 2002. The overall
depictions as per the revised reservoir storage volume from December 1977 to December 1978 is
observed as 9470.55 ML. In the beginning of 1979 the reservoir was able to reach the full point
of 14000 ML. Moreover from 1978 to 1979 the storage volume is observed to be 10914.3. It
needs to be depicted that over the 25 years the yield volume has shown several variations. In
2002, the yield value is seen to be 9386.575 ML. The maximum tenure to stay in the maximum
yield of 14000 ML is seen to be depicted for three years namely 1985, 1986 and 1987. The main
impacts of the storage yield value in the first 25 years has never reached the emergency level.
The minimum storage volume over the full timeseries is depicted to be 1840.85 ML. The
period of emergency for the reservoir is not seen until 2006. In this year the reservoir may
surpass the assigned threshold of 3500 ML. Similarly, in 2011 reservoir exceeded the assigned
emergency threshold with the value of 3177.85 ML. Despite of significant concerns of
surpassing the emergency threshold the reservoir has never reached the dead storage.
Similar to the previous case, the worst year for the reservoir is seen to be based on the
storage yield which is closest to the emergency yield. On comparison of the values with the first
case it is depicted that on December, 2006 the reservoir storage volume reached to record low of
3500 ML. In the worst year it took more than two years 7 months for the reservoir to reach from
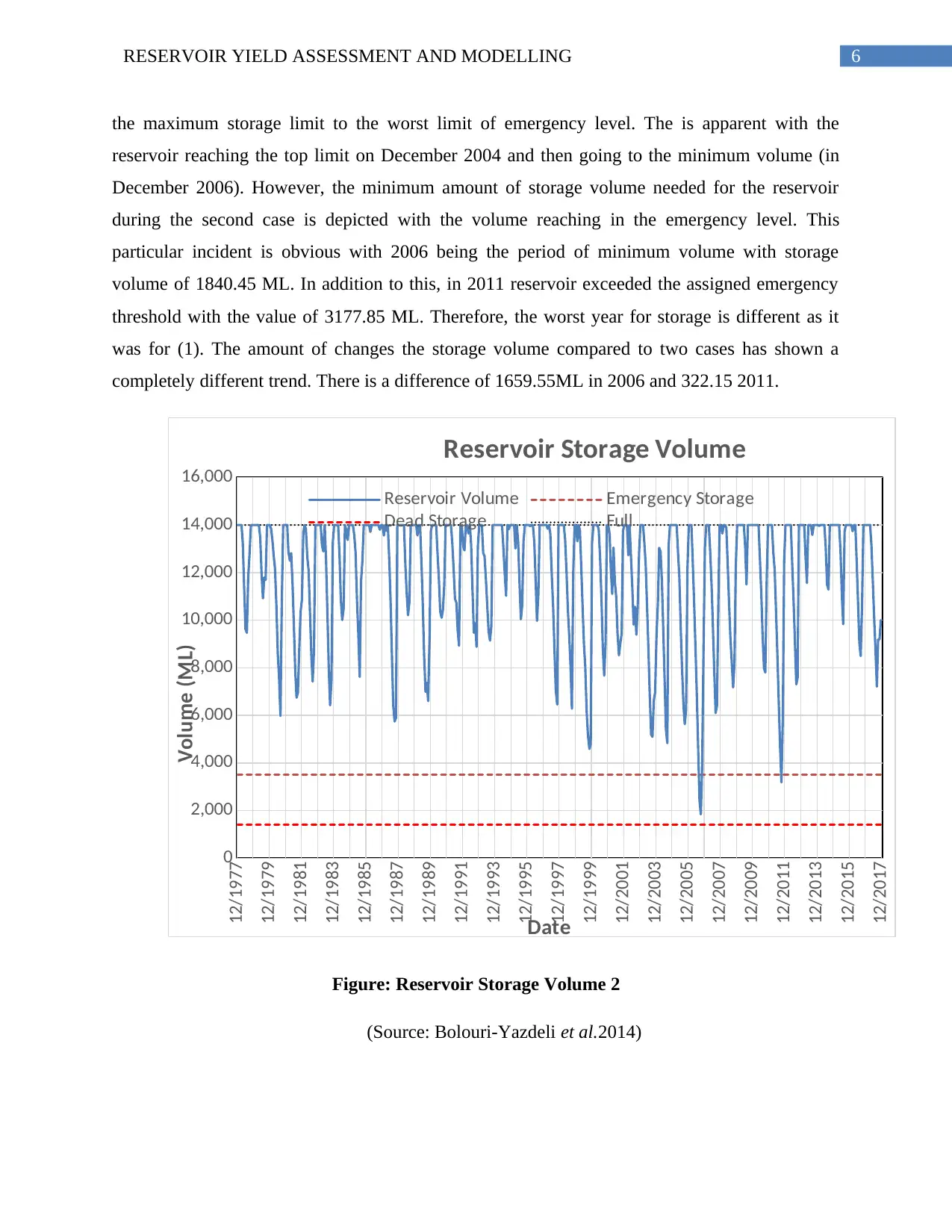
6RESERVOIR YIELD ASSESSMENT AND MODELLING
the maximum storage limit to the worst limit of emergency level. The is apparent with the
reservoir reaching the top limit on December 2004 and then going to the minimum volume (in
December 2006). However, the minimum amount of storage volume needed for the reservoir
during the second case is depicted with the volume reaching in the emergency level. This
particular incident is obvious with 2006 being the period of minimum volume with storage
volume of 1840.45 ML. In addition to this, in 2011 reservoir exceeded the assigned emergency
threshold with the value of 3177.85 ML. Therefore, the worst year for storage is different as it
was for (1). The amount of changes the storage volume compared to two cases has shown a
completely different trend. There is a difference of 1659.55ML in 2006 and 322.15 2011.
12/1977
12/1979
12/1981
12/1983
12/1985
12/1987
12/1989
12/1991
12/1993
12/1995
12/1997
12/1999
12/2001
12/2003
12/2005
12/2007
12/2009
12/2011
12/2013
12/2015
12/20170
2,000
4,000
6,000
8,000
10,000
12,000
14,000
16,000
Reservoir Storage Volume
Reservoir Volume Emergency Storage
Dead Storage Full
Date
Volume (ML)
Figure: Reservoir Storage Volume 2
(Source: Bolouri-Yazdeli et al.2014)
the maximum storage limit to the worst limit of emergency level. The is apparent with the
reservoir reaching the top limit on December 2004 and then going to the minimum volume (in
December 2006). However, the minimum amount of storage volume needed for the reservoir
during the second case is depicted with the volume reaching in the emergency level. This
particular incident is obvious with 2006 being the period of minimum volume with storage
volume of 1840.45 ML. In addition to this, in 2011 reservoir exceeded the assigned emergency
threshold with the value of 3177.85 ML. Therefore, the worst year for storage is different as it
was for (1). The amount of changes the storage volume compared to two cases has shown a
completely different trend. There is a difference of 1659.55ML in 2006 and 322.15 2011.
12/1977
12/1979
12/1981
12/1983
12/1985
12/1987
12/1989
12/1991
12/1993
12/1995
12/1997
12/1999
12/2001
12/2003
12/2005
12/2007
12/2009
12/2011
12/2013
12/2015
12/20170
2,000
4,000
6,000
8,000
10,000
12,000
14,000
16,000
Reservoir Storage Volume
Reservoir Volume Emergency Storage
Dead Storage Full
Date
Volume (ML)
Figure: Reservoir Storage Volume 2
(Source: Bolouri-Yazdeli et al.2014)
Paraphrase This Document
Need a fresh take? Get an instant paraphrase of this document with our AI Paraphraser
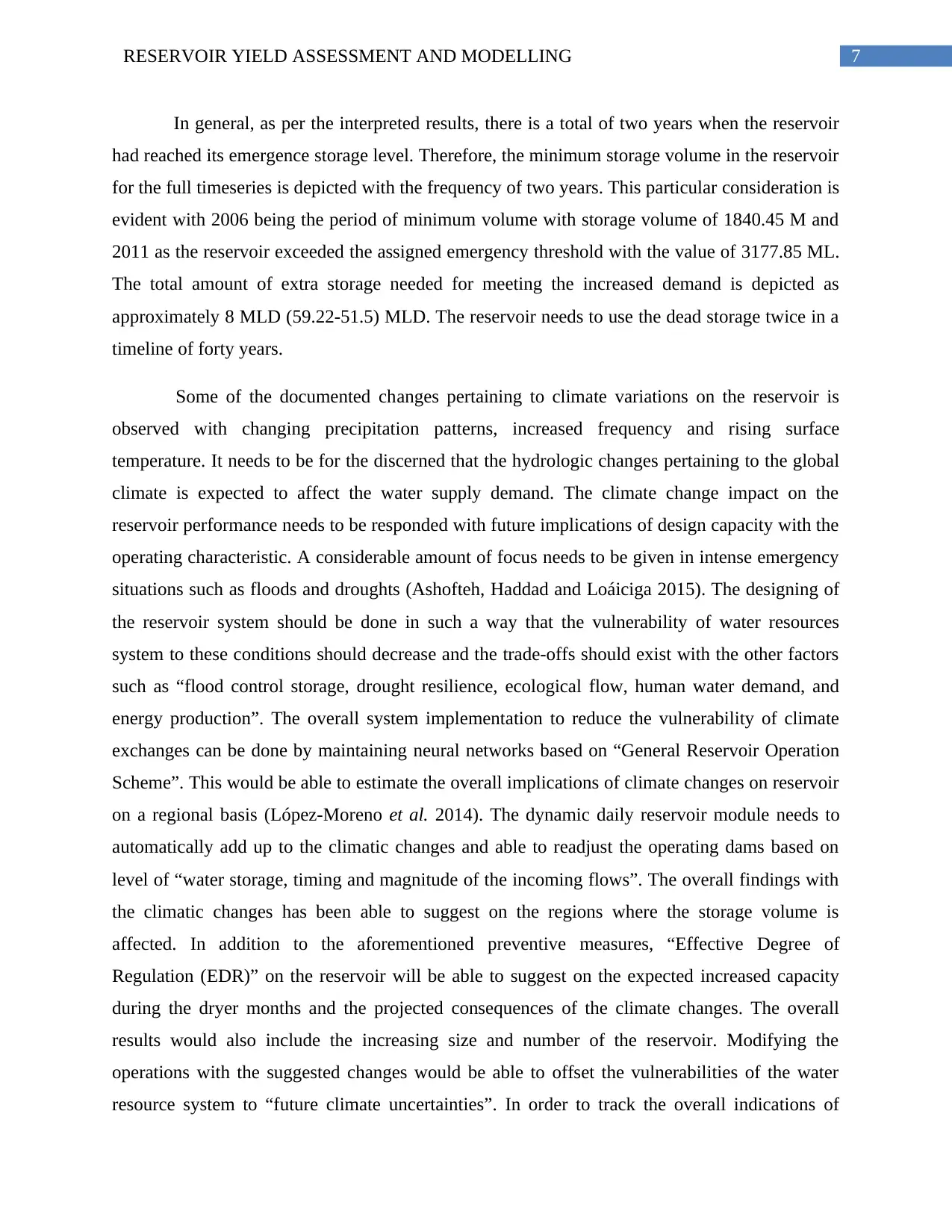
7RESERVOIR YIELD ASSESSMENT AND MODELLING
In general, as per the interpreted results, there is a total of two years when the reservoir
had reached its emergence storage level. Therefore, the minimum storage volume in the reservoir
for the full timeseries is depicted with the frequency of two years. This particular consideration is
evident with 2006 being the period of minimum volume with storage volume of 1840.45 M and
2011 as the reservoir exceeded the assigned emergency threshold with the value of 3177.85 ML.
The total amount of extra storage needed for meeting the increased demand is depicted as
approximately 8 MLD (59.22-51.5) MLD. The reservoir needs to use the dead storage twice in a
timeline of forty years.
Some of the documented changes pertaining to climate variations on the reservoir is
observed with changing precipitation patterns, increased frequency and rising surface
temperature. It needs to be for the discerned that the hydrologic changes pertaining to the global
climate is expected to affect the water supply demand. The climate change impact on the
reservoir performance needs to be responded with future implications of design capacity with the
operating characteristic. A considerable amount of focus needs to be given in intense emergency
situations such as floods and droughts (Ashofteh, Haddad and Loáiciga 2015). The designing of
the reservoir system should be done in such a way that the vulnerability of water resources
system to these conditions should decrease and the trade-offs should exist with the other factors
such as “flood control storage, drought resilience, ecological flow, human water demand, and
energy production”. The overall system implementation to reduce the vulnerability of climate
exchanges can be done by maintaining neural networks based on “General Reservoir Operation
Scheme”. This would be able to estimate the overall implications of climate changes on reservoir
on a regional basis (López-Moreno et al. 2014). The dynamic daily reservoir module needs to
automatically add up to the climatic changes and able to readjust the operating dams based on
level of “water storage, timing and magnitude of the incoming flows”. The overall findings with
the climatic changes has been able to suggest on the regions where the storage volume is
affected. In addition to the aforementioned preventive measures, “Effective Degree of
Regulation (EDR)” on the reservoir will be able to suggest on the expected increased capacity
during the dryer months and the projected consequences of the climate changes. The overall
results would also include the increasing size and number of the reservoir. Modifying the
operations with the suggested changes would be able to offset the vulnerabilities of the water
resource system to “future climate uncertainties”. In order to track the overall indications of
In general, as per the interpreted results, there is a total of two years when the reservoir
had reached its emergence storage level. Therefore, the minimum storage volume in the reservoir
for the full timeseries is depicted with the frequency of two years. This particular consideration is
evident with 2006 being the period of minimum volume with storage volume of 1840.45 M and
2011 as the reservoir exceeded the assigned emergency threshold with the value of 3177.85 ML.
The total amount of extra storage needed for meeting the increased demand is depicted as
approximately 8 MLD (59.22-51.5) MLD. The reservoir needs to use the dead storage twice in a
timeline of forty years.
Some of the documented changes pertaining to climate variations on the reservoir is
observed with changing precipitation patterns, increased frequency and rising surface
temperature. It needs to be for the discerned that the hydrologic changes pertaining to the global
climate is expected to affect the water supply demand. The climate change impact on the
reservoir performance needs to be responded with future implications of design capacity with the
operating characteristic. A considerable amount of focus needs to be given in intense emergency
situations such as floods and droughts (Ashofteh, Haddad and Loáiciga 2015). The designing of
the reservoir system should be done in such a way that the vulnerability of water resources
system to these conditions should decrease and the trade-offs should exist with the other factors
such as “flood control storage, drought resilience, ecological flow, human water demand, and
energy production”. The overall system implementation to reduce the vulnerability of climate
exchanges can be done by maintaining neural networks based on “General Reservoir Operation
Scheme”. This would be able to estimate the overall implications of climate changes on reservoir
on a regional basis (López-Moreno et al. 2014). The dynamic daily reservoir module needs to
automatically add up to the climatic changes and able to readjust the operating dams based on
level of “water storage, timing and magnitude of the incoming flows”. The overall findings with
the climatic changes has been able to suggest on the regions where the storage volume is
affected. In addition to the aforementioned preventive measures, “Effective Degree of
Regulation (EDR)” on the reservoir will be able to suggest on the expected increased capacity
during the dryer months and the projected consequences of the climate changes. The overall
results would also include the increasing size and number of the reservoir. Modifying the
operations with the suggested changes would be able to offset the vulnerabilities of the water
resource system to “future climate uncertainties”. In order to track the overall indications of
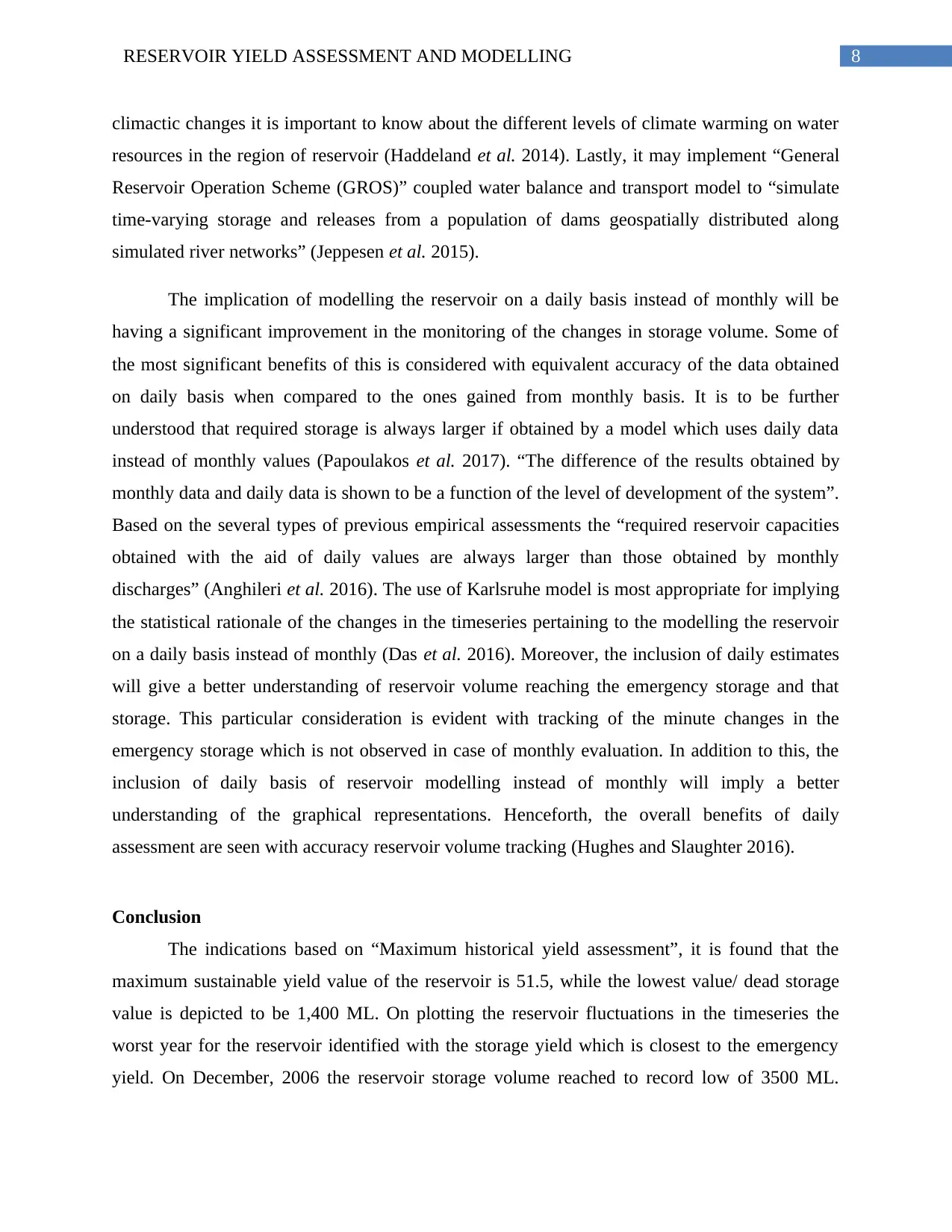
8RESERVOIR YIELD ASSESSMENT AND MODELLING
climactic changes it is important to know about the different levels of climate warming on water
resources in the region of reservoir (Haddeland et al. 2014). Lastly, it may implement “General
Reservoir Operation Scheme (GROS)” coupled water balance and transport model to “simulate
time-varying storage and releases from a population of dams geospatially distributed along
simulated river networks” (Jeppesen et al. 2015).
The implication of modelling the reservoir on a daily basis instead of monthly will be
having a significant improvement in the monitoring of the changes in storage volume. Some of
the most significant benefits of this is considered with equivalent accuracy of the data obtained
on daily basis when compared to the ones gained from monthly basis. It is to be further
understood that required storage is always larger if obtained by a model which uses daily data
instead of monthly values (Papoulakos et al. 2017). “The difference of the results obtained by
monthly data and daily data is shown to be a function of the level of development of the system”.
Based on the several types of previous empirical assessments the “required reservoir capacities
obtained with the aid of daily values are always larger than those obtained by monthly
discharges” (Anghileri et al. 2016). The use of Karlsruhe model is most appropriate for implying
the statistical rationale of the changes in the timeseries pertaining to the modelling the reservoir
on a daily basis instead of monthly (Das et al. 2016). Moreover, the inclusion of daily estimates
will give a better understanding of reservoir volume reaching the emergency storage and that
storage. This particular consideration is evident with tracking of the minute changes in the
emergency storage which is not observed in case of monthly evaluation. In addition to this, the
inclusion of daily basis of reservoir modelling instead of monthly will imply a better
understanding of the graphical representations. Henceforth, the overall benefits of daily
assessment are seen with accuracy reservoir volume tracking (Hughes and Slaughter 2016).
Conclusion
The indications based on “Maximum historical yield assessment”, it is found that the
maximum sustainable yield value of the reservoir is 51.5, while the lowest value/ dead storage
value is depicted to be 1,400 ML. On plotting the reservoir fluctuations in the timeseries the
worst year for the reservoir identified with the storage yield which is closest to the emergency
yield. On December, 2006 the reservoir storage volume reached to record low of 3500 ML.
climactic changes it is important to know about the different levels of climate warming on water
resources in the region of reservoir (Haddeland et al. 2014). Lastly, it may implement “General
Reservoir Operation Scheme (GROS)” coupled water balance and transport model to “simulate
time-varying storage and releases from a population of dams geospatially distributed along
simulated river networks” (Jeppesen et al. 2015).
The implication of modelling the reservoir on a daily basis instead of monthly will be
having a significant improvement in the monitoring of the changes in storage volume. Some of
the most significant benefits of this is considered with equivalent accuracy of the data obtained
on daily basis when compared to the ones gained from monthly basis. It is to be further
understood that required storage is always larger if obtained by a model which uses daily data
instead of monthly values (Papoulakos et al. 2017). “The difference of the results obtained by
monthly data and daily data is shown to be a function of the level of development of the system”.
Based on the several types of previous empirical assessments the “required reservoir capacities
obtained with the aid of daily values are always larger than those obtained by monthly
discharges” (Anghileri et al. 2016). The use of Karlsruhe model is most appropriate for implying
the statistical rationale of the changes in the timeseries pertaining to the modelling the reservoir
on a daily basis instead of monthly (Das et al. 2016). Moreover, the inclusion of daily estimates
will give a better understanding of reservoir volume reaching the emergency storage and that
storage. This particular consideration is evident with tracking of the minute changes in the
emergency storage which is not observed in case of monthly evaluation. In addition to this, the
inclusion of daily basis of reservoir modelling instead of monthly will imply a better
understanding of the graphical representations. Henceforth, the overall benefits of daily
assessment are seen with accuracy reservoir volume tracking (Hughes and Slaughter 2016).
Conclusion
The indications based on “Maximum historical yield assessment”, it is found that the
maximum sustainable yield value of the reservoir is 51.5, while the lowest value/ dead storage
value is depicted to be 1,400 ML. On plotting the reservoir fluctuations in the timeseries the
worst year for the reservoir identified with the storage yield which is closest to the emergency
yield. On December, 2006 the reservoir storage volume reached to record low of 3500 ML.
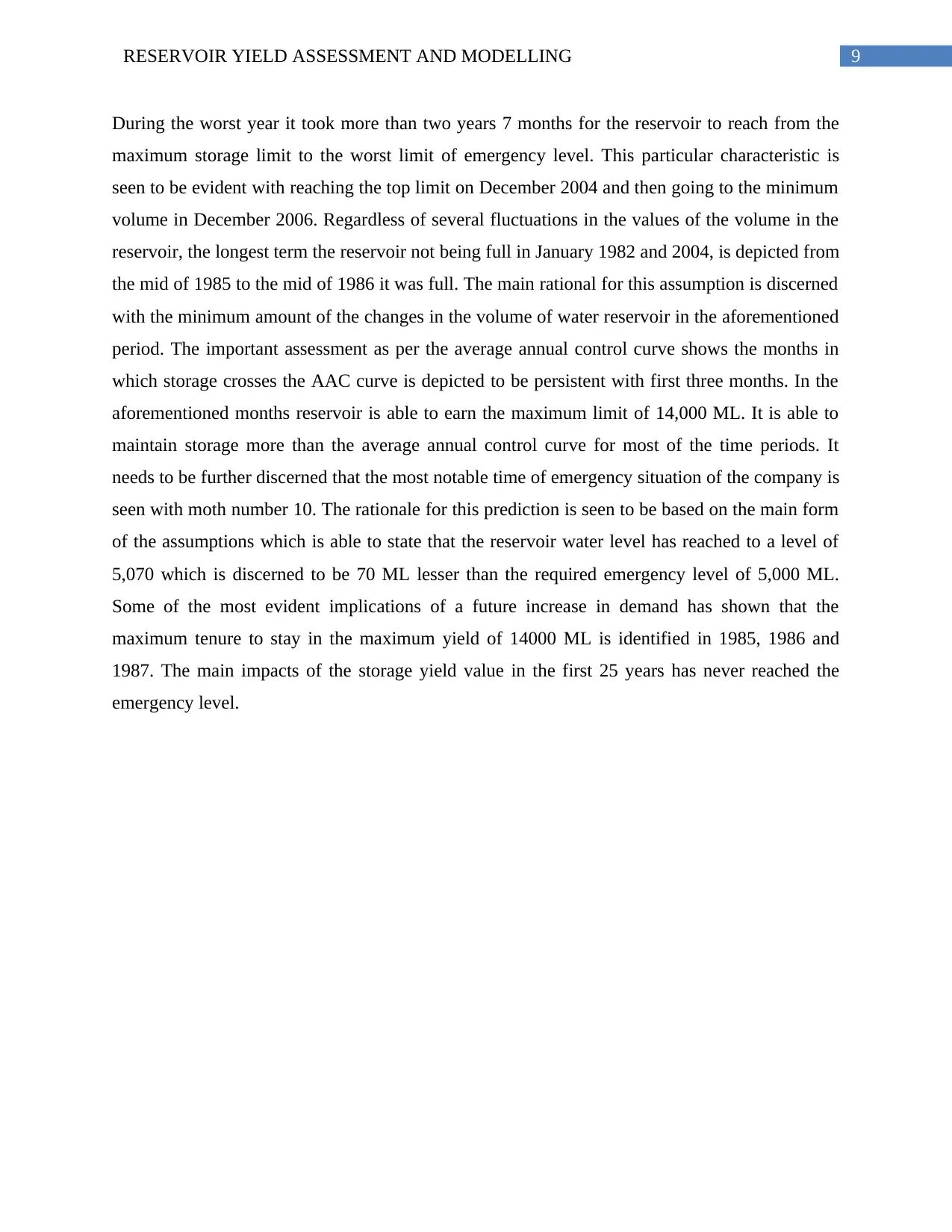
9RESERVOIR YIELD ASSESSMENT AND MODELLING
During the worst year it took more than two years 7 months for the reservoir to reach from the
maximum storage limit to the worst limit of emergency level. This particular characteristic is
seen to be evident with reaching the top limit on December 2004 and then going to the minimum
volume in December 2006. Regardless of several fluctuations in the values of the volume in the
reservoir, the longest term the reservoir not being full in January 1982 and 2004, is depicted from
the mid of 1985 to the mid of 1986 it was full. The main rational for this assumption is discerned
with the minimum amount of the changes in the volume of water reservoir in the aforementioned
period. The important assessment as per the average annual control curve shows the months in
which storage crosses the AAC curve is depicted to be persistent with first three months. In the
aforementioned months reservoir is able to earn the maximum limit of 14,000 ML. It is able to
maintain storage more than the average annual control curve for most of the time periods. It
needs to be further discerned that the most notable time of emergency situation of the company is
seen with moth number 10. The rationale for this prediction is seen to be based on the main form
of the assumptions which is able to state that the reservoir water level has reached to a level of
5,070 which is discerned to be 70 ML lesser than the required emergency level of 5,000 ML.
Some of the most evident implications of a future increase in demand has shown that the
maximum tenure to stay in the maximum yield of 14000 ML is identified in 1985, 1986 and
1987. The main impacts of the storage yield value in the first 25 years has never reached the
emergency level.
During the worst year it took more than two years 7 months for the reservoir to reach from the
maximum storage limit to the worst limit of emergency level. This particular characteristic is
seen to be evident with reaching the top limit on December 2004 and then going to the minimum
volume in December 2006. Regardless of several fluctuations in the values of the volume in the
reservoir, the longest term the reservoir not being full in January 1982 and 2004, is depicted from
the mid of 1985 to the mid of 1986 it was full. The main rational for this assumption is discerned
with the minimum amount of the changes in the volume of water reservoir in the aforementioned
period. The important assessment as per the average annual control curve shows the months in
which storage crosses the AAC curve is depicted to be persistent with first three months. In the
aforementioned months reservoir is able to earn the maximum limit of 14,000 ML. It is able to
maintain storage more than the average annual control curve for most of the time periods. It
needs to be further discerned that the most notable time of emergency situation of the company is
seen with moth number 10. The rationale for this prediction is seen to be based on the main form
of the assumptions which is able to state that the reservoir water level has reached to a level of
5,070 which is discerned to be 70 ML lesser than the required emergency level of 5,000 ML.
Some of the most evident implications of a future increase in demand has shown that the
maximum tenure to stay in the maximum yield of 14000 ML is identified in 1985, 1986 and
1987. The main impacts of the storage yield value in the first 25 years has never reached the
emergency level.
Secure Best Marks with AI Grader
Need help grading? Try our AI Grader for instant feedback on your assignments.
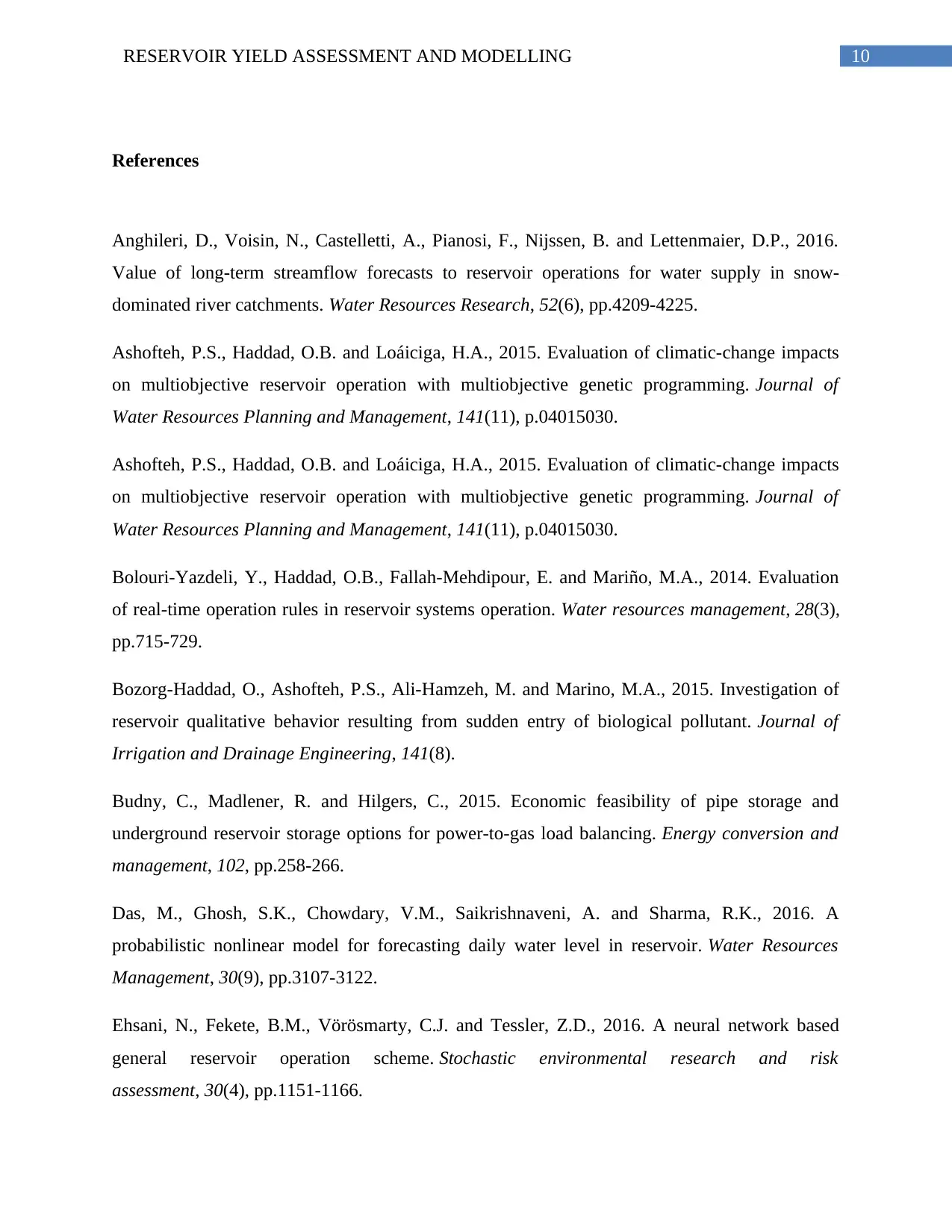
10RESERVOIR YIELD ASSESSMENT AND MODELLING
References
Anghileri, D., Voisin, N., Castelletti, A., Pianosi, F., Nijssen, B. and Lettenmaier, D.P., 2016.
Value of long‐term streamflow forecasts to reservoir operations for water supply in snow‐
dominated river catchments. Water Resources Research, 52(6), pp.4209-4225.
Ashofteh, P.S., Haddad, O.B. and Loáiciga, H.A., 2015. Evaluation of climatic-change impacts
on multiobjective reservoir operation with multiobjective genetic programming. Journal of
Water Resources Planning and Management, 141(11), p.04015030.
Ashofteh, P.S., Haddad, O.B. and Loáiciga, H.A., 2015. Evaluation of climatic-change impacts
on multiobjective reservoir operation with multiobjective genetic programming. Journal of
Water Resources Planning and Management, 141(11), p.04015030.
Bolouri-Yazdeli, Y., Haddad, O.B., Fallah-Mehdipour, E. and Mariño, M.A., 2014. Evaluation
of real-time operation rules in reservoir systems operation. Water resources management, 28(3),
pp.715-729.
Bozorg-Haddad, O., Ashofteh, P.S., Ali-Hamzeh, M. and Marino, M.A., 2015. Investigation of
reservoir qualitative behavior resulting from sudden entry of biological pollutant. Journal of
Irrigation and Drainage Engineering, 141(8).
Budny, C., Madlener, R. and Hilgers, C., 2015. Economic feasibility of pipe storage and
underground reservoir storage options for power-to-gas load balancing. Energy conversion and
management, 102, pp.258-266.
Das, M., Ghosh, S.K., Chowdary, V.M., Saikrishnaveni, A. and Sharma, R.K., 2016. A
probabilistic nonlinear model for forecasting daily water level in reservoir. Water Resources
Management, 30(9), pp.3107-3122.
Ehsani, N., Fekete, B.M., Vörösmarty, C.J. and Tessler, Z.D., 2016. A neural network based
general reservoir operation scheme. Stochastic environmental research and risk
assessment, 30(4), pp.1151-1166.
References
Anghileri, D., Voisin, N., Castelletti, A., Pianosi, F., Nijssen, B. and Lettenmaier, D.P., 2016.
Value of long‐term streamflow forecasts to reservoir operations for water supply in snow‐
dominated river catchments. Water Resources Research, 52(6), pp.4209-4225.
Ashofteh, P.S., Haddad, O.B. and Loáiciga, H.A., 2015. Evaluation of climatic-change impacts
on multiobjective reservoir operation with multiobjective genetic programming. Journal of
Water Resources Planning and Management, 141(11), p.04015030.
Ashofteh, P.S., Haddad, O.B. and Loáiciga, H.A., 2015. Evaluation of climatic-change impacts
on multiobjective reservoir operation with multiobjective genetic programming. Journal of
Water Resources Planning and Management, 141(11), p.04015030.
Bolouri-Yazdeli, Y., Haddad, O.B., Fallah-Mehdipour, E. and Mariño, M.A., 2014. Evaluation
of real-time operation rules in reservoir systems operation. Water resources management, 28(3),
pp.715-729.
Bozorg-Haddad, O., Ashofteh, P.S., Ali-Hamzeh, M. and Marino, M.A., 2015. Investigation of
reservoir qualitative behavior resulting from sudden entry of biological pollutant. Journal of
Irrigation and Drainage Engineering, 141(8).
Budny, C., Madlener, R. and Hilgers, C., 2015. Economic feasibility of pipe storage and
underground reservoir storage options for power-to-gas load balancing. Energy conversion and
management, 102, pp.258-266.
Das, M., Ghosh, S.K., Chowdary, V.M., Saikrishnaveni, A. and Sharma, R.K., 2016. A
probabilistic nonlinear model for forecasting daily water level in reservoir. Water Resources
Management, 30(9), pp.3107-3122.
Ehsani, N., Fekete, B.M., Vörösmarty, C.J. and Tessler, Z.D., 2016. A neural network based
general reservoir operation scheme. Stochastic environmental research and risk
assessment, 30(4), pp.1151-1166.
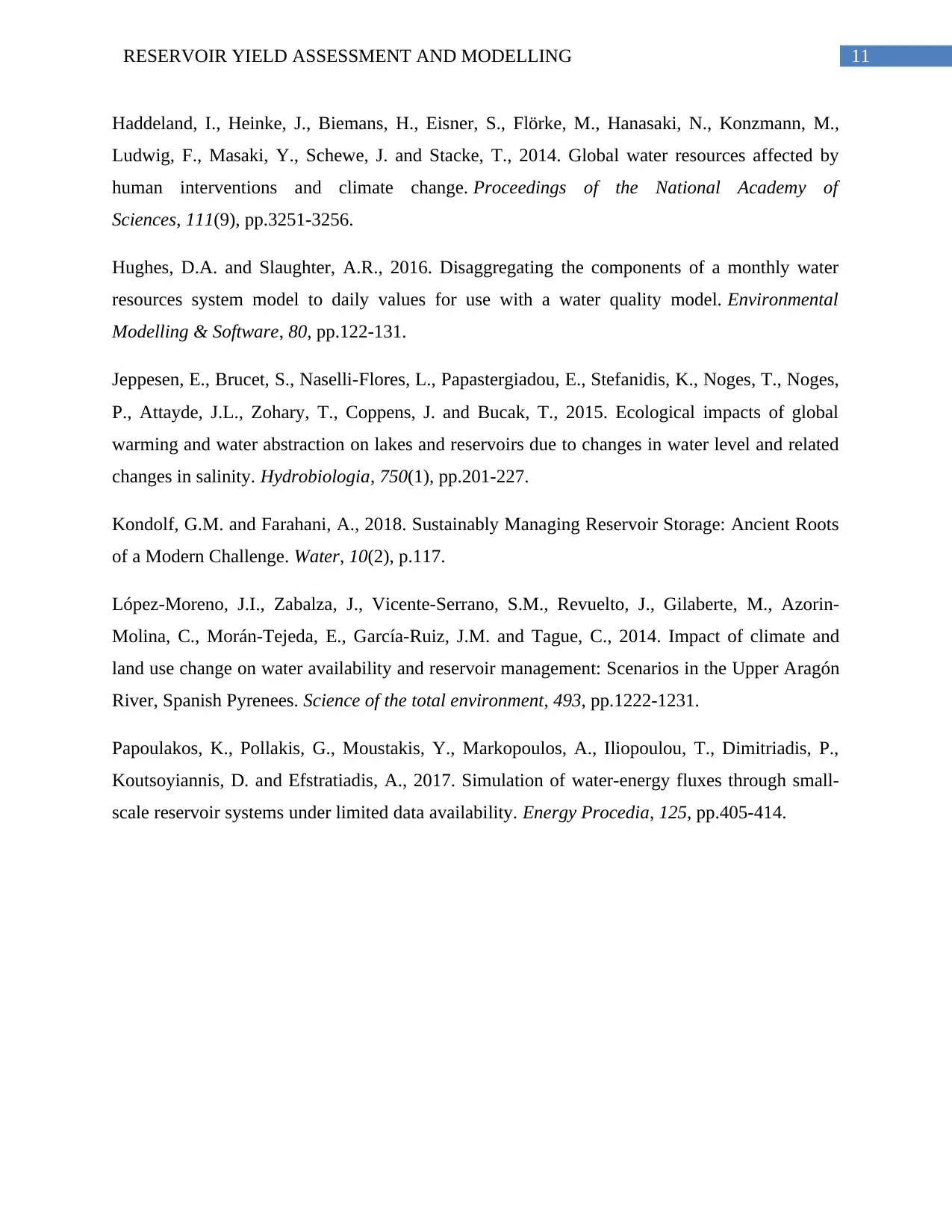
11RESERVOIR YIELD ASSESSMENT AND MODELLING
Haddeland, I., Heinke, J., Biemans, H., Eisner, S., Flörke, M., Hanasaki, N., Konzmann, M.,
Ludwig, F., Masaki, Y., Schewe, J. and Stacke, T., 2014. Global water resources affected by
human interventions and climate change. Proceedings of the National Academy of
Sciences, 111(9), pp.3251-3256.
Hughes, D.A. and Slaughter, A.R., 2016. Disaggregating the components of a monthly water
resources system model to daily values for use with a water quality model. Environmental
Modelling & Software, 80, pp.122-131.
Jeppesen, E., Brucet, S., Naselli-Flores, L., Papastergiadou, E., Stefanidis, K., Noges, T., Noges,
P., Attayde, J.L., Zohary, T., Coppens, J. and Bucak, T., 2015. Ecological impacts of global
warming and water abstraction on lakes and reservoirs due to changes in water level and related
changes in salinity. Hydrobiologia, 750(1), pp.201-227.
Kondolf, G.M. and Farahani, A., 2018. Sustainably Managing Reservoir Storage: Ancient Roots
of a Modern Challenge. Water, 10(2), p.117.
López-Moreno, J.I., Zabalza, J., Vicente-Serrano, S.M., Revuelto, J., Gilaberte, M., Azorin-
Molina, C., Morán-Tejeda, E., García-Ruiz, J.M. and Tague, C., 2014. Impact of climate and
land use change on water availability and reservoir management: Scenarios in the Upper Aragón
River, Spanish Pyrenees. Science of the total environment, 493, pp.1222-1231.
Papoulakos, K., Pollakis, G., Moustakis, Y., Markopoulos, A., Iliopoulou, T., Dimitriadis, P.,
Koutsoyiannis, D. and Efstratiadis, A., 2017. Simulation of water-energy fluxes through small-
scale reservoir systems under limited data availability. Energy Procedia, 125, pp.405-414.
Haddeland, I., Heinke, J., Biemans, H., Eisner, S., Flörke, M., Hanasaki, N., Konzmann, M.,
Ludwig, F., Masaki, Y., Schewe, J. and Stacke, T., 2014. Global water resources affected by
human interventions and climate change. Proceedings of the National Academy of
Sciences, 111(9), pp.3251-3256.
Hughes, D.A. and Slaughter, A.R., 2016. Disaggregating the components of a monthly water
resources system model to daily values for use with a water quality model. Environmental
Modelling & Software, 80, pp.122-131.
Jeppesen, E., Brucet, S., Naselli-Flores, L., Papastergiadou, E., Stefanidis, K., Noges, T., Noges,
P., Attayde, J.L., Zohary, T., Coppens, J. and Bucak, T., 2015. Ecological impacts of global
warming and water abstraction on lakes and reservoirs due to changes in water level and related
changes in salinity. Hydrobiologia, 750(1), pp.201-227.
Kondolf, G.M. and Farahani, A., 2018. Sustainably Managing Reservoir Storage: Ancient Roots
of a Modern Challenge. Water, 10(2), p.117.
López-Moreno, J.I., Zabalza, J., Vicente-Serrano, S.M., Revuelto, J., Gilaberte, M., Azorin-
Molina, C., Morán-Tejeda, E., García-Ruiz, J.M. and Tague, C., 2014. Impact of climate and
land use change on water availability and reservoir management: Scenarios in the Upper Aragón
River, Spanish Pyrenees. Science of the total environment, 493, pp.1222-1231.
Papoulakos, K., Pollakis, G., Moustakis, Y., Markopoulos, A., Iliopoulou, T., Dimitriadis, P.,
Koutsoyiannis, D. and Efstratiadis, A., 2017. Simulation of water-energy fluxes through small-
scale reservoir systems under limited data availability. Energy Procedia, 125, pp.405-414.
1 out of 12
![[object Object]](/_next/image/?url=%2F_next%2Fstatic%2Fmedia%2Flogo.6d15ce61.png&w=640&q=75)
Your All-in-One AI-Powered Toolkit for Academic Success.
+13062052269
info@desklib.com
Available 24*7 on WhatsApp / Email
Unlock your academic potential
© 2024 | Zucol Services PVT LTD | All rights reserved.