Security and Privacy Issues in Analytics - Dumnonia Corporation
VerifiedAdded on 2023/06/04
|13
|3421
|360
AI Summary
This report discusses security and privacy issues in analytics faced by Dumnonia Corporation, a leading insurance organization in Australia. It analyzes the implementation of k-anonymity as a model to protect privacy and assesses the technology solutions used by Dumnonia. The report also provides a guide for k-anonymity implementation.
Contribute Materials
Your contribution can guide someone’s learning journey. Share your
documents today.
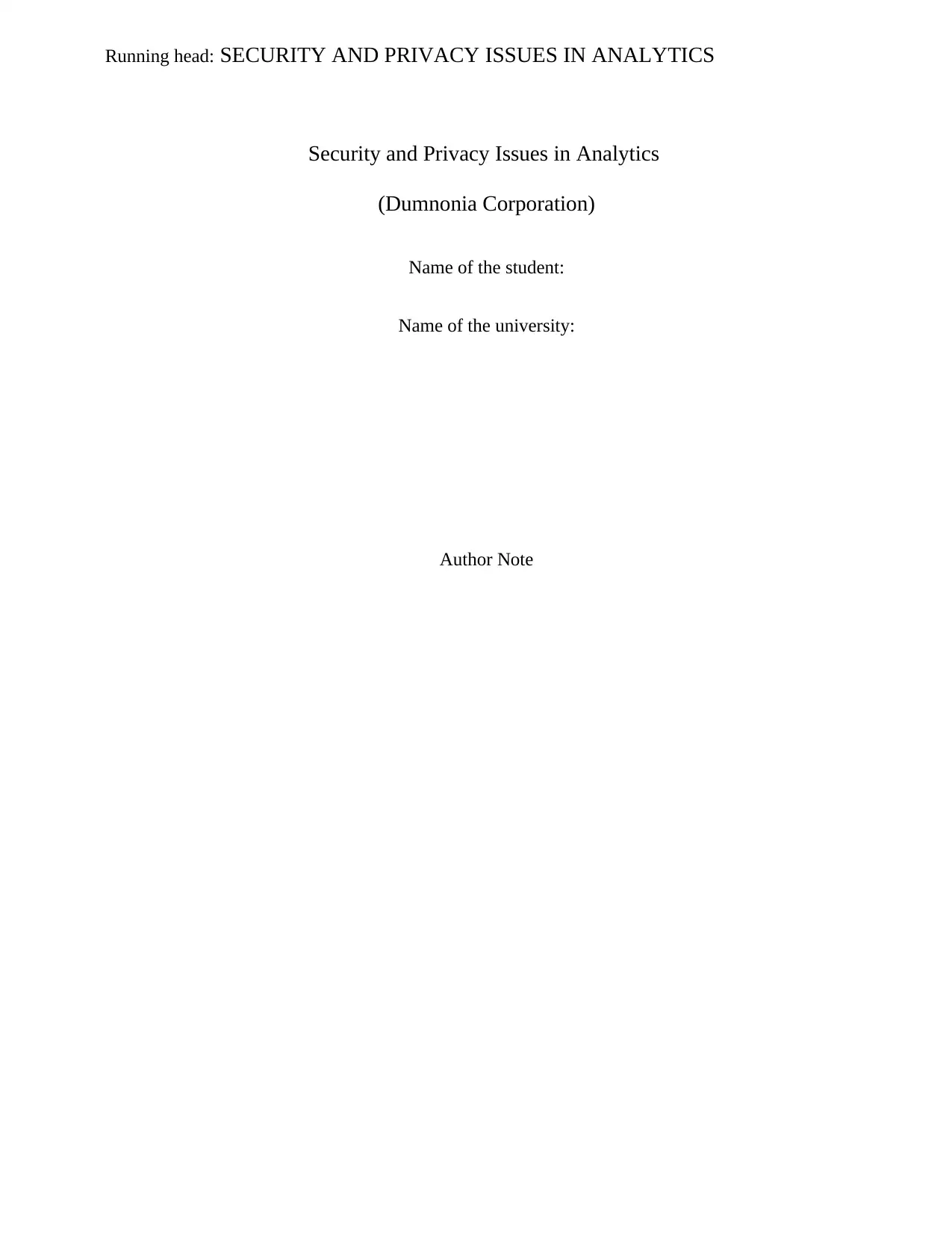
Running head: SECURITY AND PRIVACY ISSUES IN ANALYTICS
Security and Privacy Issues in Analytics
(Dumnonia Corporation)
Name of the student:
Name of the university:
Author Note
Security and Privacy Issues in Analytics
(Dumnonia Corporation)
Name of the student:
Name of the university:
Author Note
Secure Best Marks with AI Grader
Need help grading? Try our AI Grader for instant feedback on your assignments.
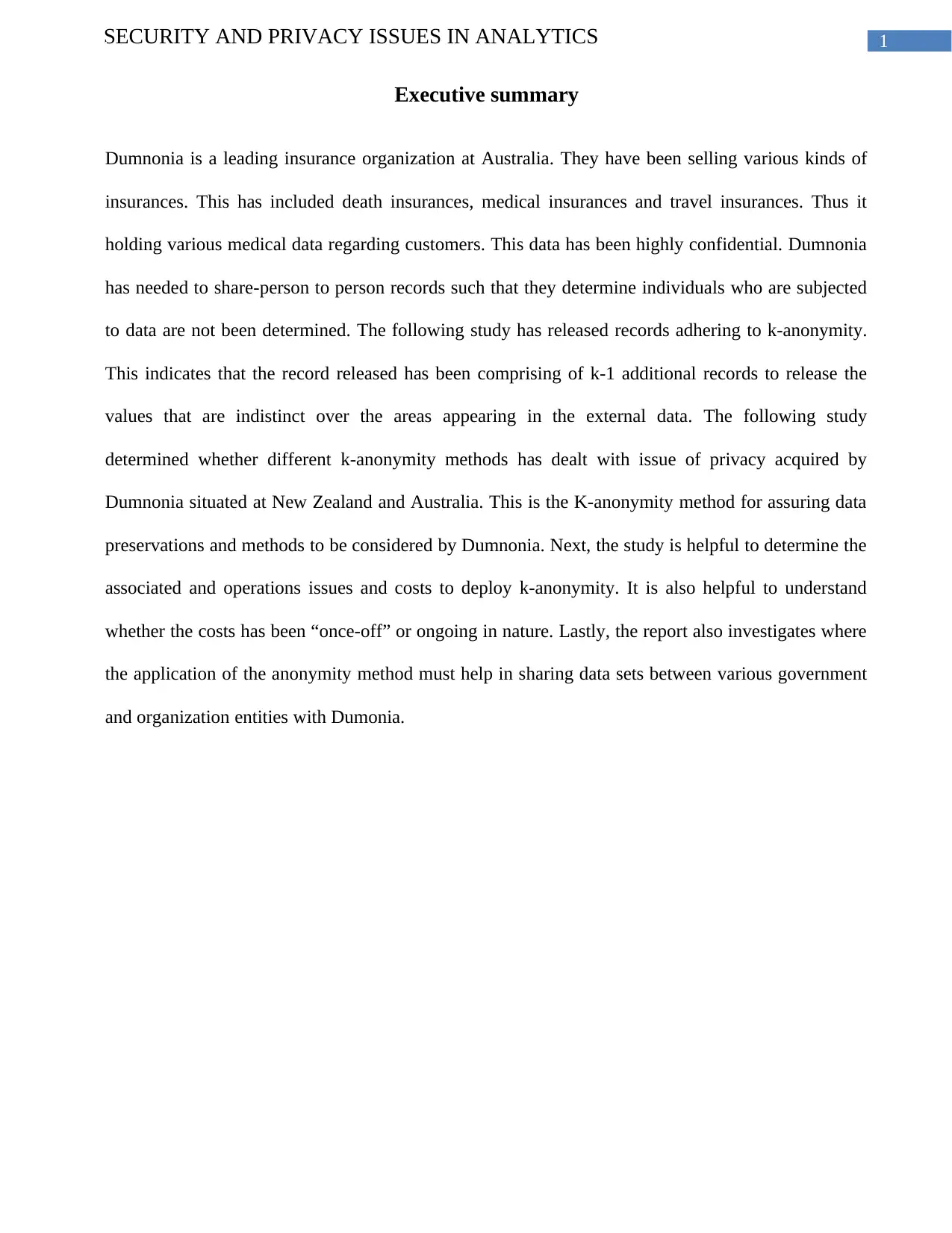
1SECURITY AND PRIVACY ISSUES IN ANALYTICS
Executive summary
Dumnonia is a leading insurance organization at Australia. They have been selling various kinds of
insurances. This has included death insurances, medical insurances and travel insurances. Thus it
holding various medical data regarding customers. This data has been highly confidential. Dumnonia
has needed to share-person to person records such that they determine individuals who are subjected
to data are not been determined. The following study has released records adhering to k-anonymity.
This indicates that the record released has been comprising of k-1 additional records to release the
values that are indistinct over the areas appearing in the external data. The following study
determined whether different k-anonymity methods has dealt with issue of privacy acquired by
Dumnonia situated at New Zealand and Australia. This is the K-anonymity method for assuring data
preservations and methods to be considered by Dumnonia. Next, the study is helpful to determine the
associated and operations issues and costs to deploy k-anonymity. It is also helpful to understand
whether the costs has been “once-off” or ongoing in nature. Lastly, the report also investigates where
the application of the anonymity method must help in sharing data sets between various government
and organization entities with Dumonia.
Executive summary
Dumnonia is a leading insurance organization at Australia. They have been selling various kinds of
insurances. This has included death insurances, medical insurances and travel insurances. Thus it
holding various medical data regarding customers. This data has been highly confidential. Dumnonia
has needed to share-person to person records such that they determine individuals who are subjected
to data are not been determined. The following study has released records adhering to k-anonymity.
This indicates that the record released has been comprising of k-1 additional records to release the
values that are indistinct over the areas appearing in the external data. The following study
determined whether different k-anonymity methods has dealt with issue of privacy acquired by
Dumnonia situated at New Zealand and Australia. This is the K-anonymity method for assuring data
preservations and methods to be considered by Dumnonia. Next, the study is helpful to determine the
associated and operations issues and costs to deploy k-anonymity. It is also helpful to understand
whether the costs has been “once-off” or ongoing in nature. Lastly, the report also investigates where
the application of the anonymity method must help in sharing data sets between various government
and organization entities with Dumonia.
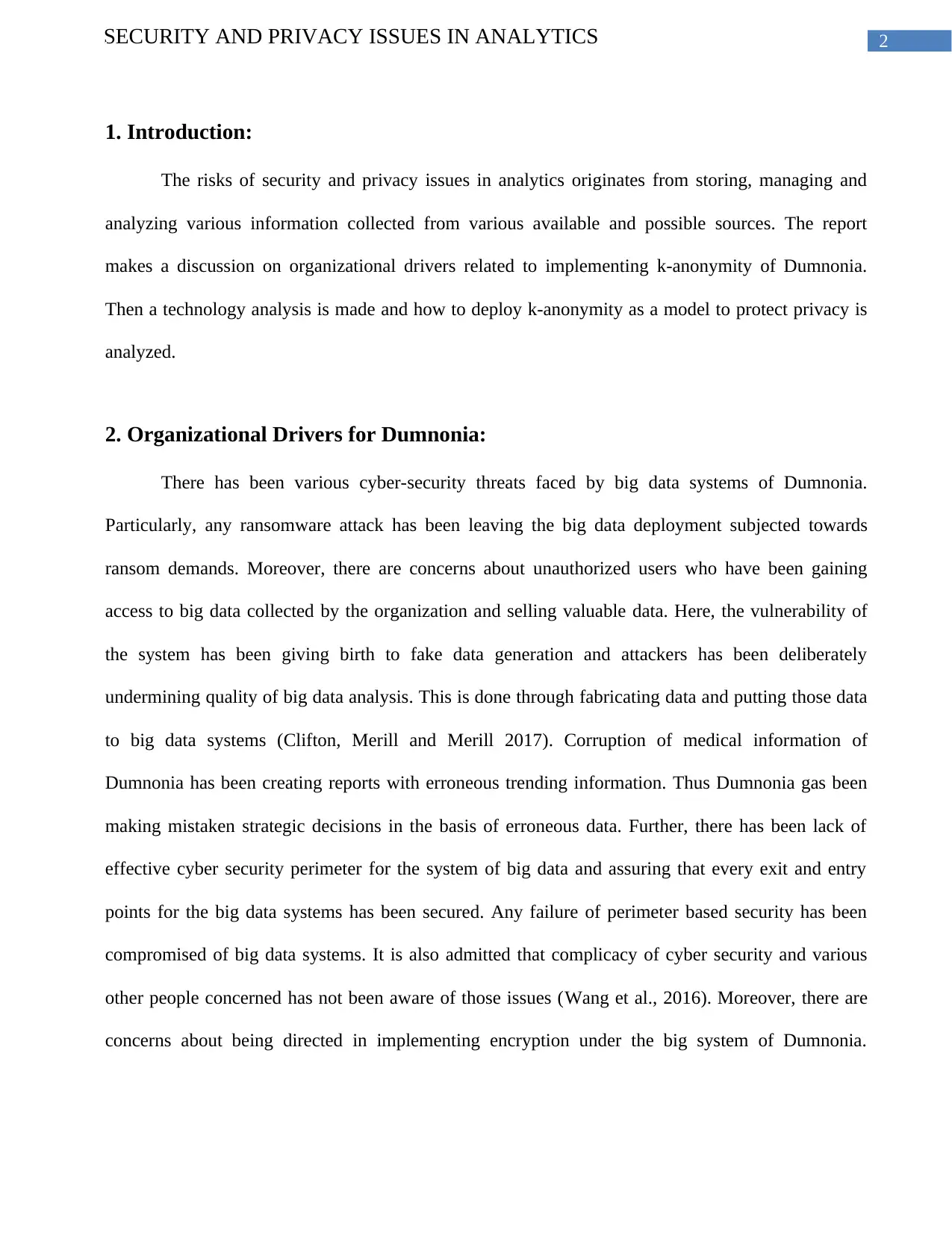
2SECURITY AND PRIVACY ISSUES IN ANALYTICS
1. Introduction:
The risks of security and privacy issues in analytics originates from storing, managing and
analyzing various information collected from various available and possible sources. The report
makes a discussion on organizational drivers related to implementing k-anonymity of Dumnonia.
Then a technology analysis is made and how to deploy k-anonymity as a model to protect privacy is
analyzed.
2. Organizational Drivers for Dumnonia:
There has been various cyber-security threats faced by big data systems of Dumnonia.
Particularly, any ransomware attack has been leaving the big data deployment subjected towards
ransom demands. Moreover, there are concerns about unauthorized users who have been gaining
access to big data collected by the organization and selling valuable data. Here, the vulnerability of
the system has been giving birth to fake data generation and attackers has been deliberately
undermining quality of big data analysis. This is done through fabricating data and putting those data
to big data systems (Clifton, Merill and Merill 2017). Corruption of medical information of
Dumnonia has been creating reports with erroneous trending information. Thus Dumnonia gas been
making mistaken strategic decisions in the basis of erroneous data. Further, there has been lack of
effective cyber security perimeter for the system of big data and assuring that every exit and entry
points for the big data systems has been secured. Any failure of perimeter based security has been
compromised of big data systems. It is also admitted that complicacy of cyber security and various
other people concerned has not been aware of those issues (Wang et al., 2016). Moreover, there are
concerns about being directed in implementing encryption under the big system of Dumnonia.
1. Introduction:
The risks of security and privacy issues in analytics originates from storing, managing and
analyzing various information collected from various available and possible sources. The report
makes a discussion on organizational drivers related to implementing k-anonymity of Dumnonia.
Then a technology analysis is made and how to deploy k-anonymity as a model to protect privacy is
analyzed.
2. Organizational Drivers for Dumnonia:
There has been various cyber-security threats faced by big data systems of Dumnonia.
Particularly, any ransomware attack has been leaving the big data deployment subjected towards
ransom demands. Moreover, there are concerns about unauthorized users who have been gaining
access to big data collected by the organization and selling valuable data. Here, the vulnerability of
the system has been giving birth to fake data generation and attackers has been deliberately
undermining quality of big data analysis. This is done through fabricating data and putting those data
to big data systems (Clifton, Merill and Merill 2017). Corruption of medical information of
Dumnonia has been creating reports with erroneous trending information. Thus Dumnonia gas been
making mistaken strategic decisions in the basis of erroneous data. Further, there has been lack of
effective cyber security perimeter for the system of big data and assuring that every exit and entry
points for the big data systems has been secured. Any failure of perimeter based security has been
compromised of big data systems. It is also admitted that complicacy of cyber security and various
other people concerned has not been aware of those issues (Wang et al., 2016). Moreover, there are
concerns about being directed in implementing encryption under the big system of Dumnonia.
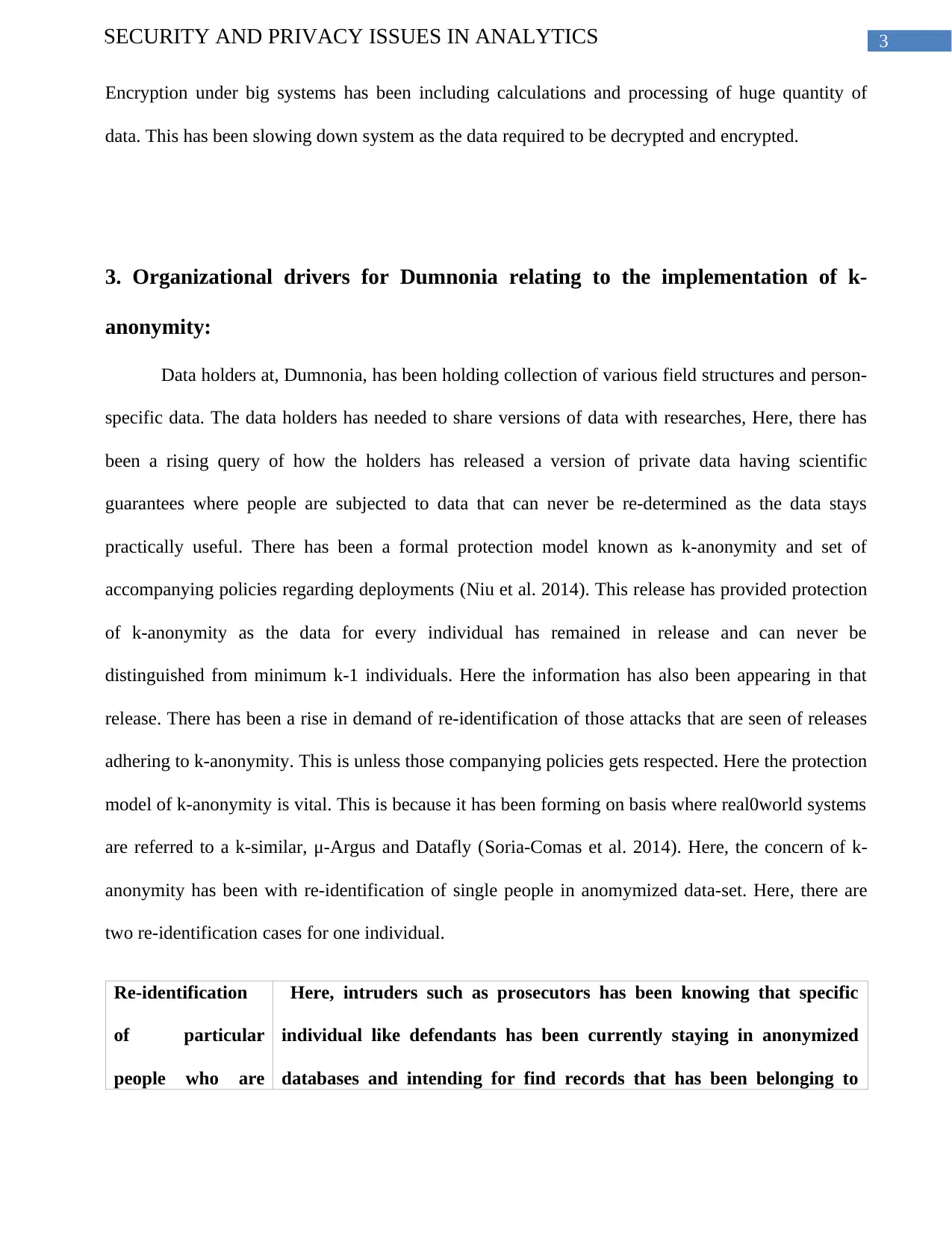
3SECURITY AND PRIVACY ISSUES IN ANALYTICS
Encryption under big systems has been including calculations and processing of huge quantity of
data. This has been slowing down system as the data required to be decrypted and encrypted.
3. Organizational drivers for Dumnonia relating to the implementation of k-
anonymity:
Data holders at, Dumnonia, has been holding collection of various field structures and person-
specific data. The data holders has needed to share versions of data with researches, Here, there has
been a rising query of how the holders has released a version of private data having scientific
guarantees where people are subjected to data that can never be re-determined as the data stays
practically useful. There has been a formal protection model known as k-anonymity and set of
accompanying policies regarding deployments (Niu et al. 2014). This release has provided protection
of k-anonymity as the data for every individual has remained in release and can never be
distinguished from minimum k-1 individuals. Here the information has also been appearing in that
release. There has been a rise in demand of re-identification of those attacks that are seen of releases
adhering to k-anonymity. This is unless those companying policies gets respected. Here the protection
model of k-anonymity is vital. This is because it has been forming on basis where real0world systems
are referred to a k-similar, μ-Argus and Datafly (Soria-Comas et al. 2014). Here, the concern of k-
anonymity has been with re-identification of single people in anomymized data-set. Here, there are
two re-identification cases for one individual.
Re-identification
of particular
people who are
Here, intruders such as prosecutors has been knowing that specific
individual like defendants has been currently staying in anonymized
databases and intending for find records that has been belonging to
Encryption under big systems has been including calculations and processing of huge quantity of
data. This has been slowing down system as the data required to be decrypted and encrypted.
3. Organizational drivers for Dumnonia relating to the implementation of k-
anonymity:
Data holders at, Dumnonia, has been holding collection of various field structures and person-
specific data. The data holders has needed to share versions of data with researches, Here, there has
been a rising query of how the holders has released a version of private data having scientific
guarantees where people are subjected to data that can never be re-determined as the data stays
practically useful. There has been a formal protection model known as k-anonymity and set of
accompanying policies regarding deployments (Niu et al. 2014). This release has provided protection
of k-anonymity as the data for every individual has remained in release and can never be
distinguished from minimum k-1 individuals. Here the information has also been appearing in that
release. There has been a rise in demand of re-identification of those attacks that are seen of releases
adhering to k-anonymity. This is unless those companying policies gets respected. Here the protection
model of k-anonymity is vital. This is because it has been forming on basis where real0world systems
are referred to a k-similar, μ-Argus and Datafly (Soria-Comas et al. 2014). Here, the concern of k-
anonymity has been with re-identification of single people in anomymized data-set. Here, there are
two re-identification cases for one individual.
Re-identification
of particular
people who are
Here, intruders such as prosecutors has been knowing that specific
individual like defendants has been currently staying in anonymized
databases and intending for find records that has been belonging to
Secure Best Marks with AI Grader
Need help grading? Try our AI Grader for instant feedback on your assignments.
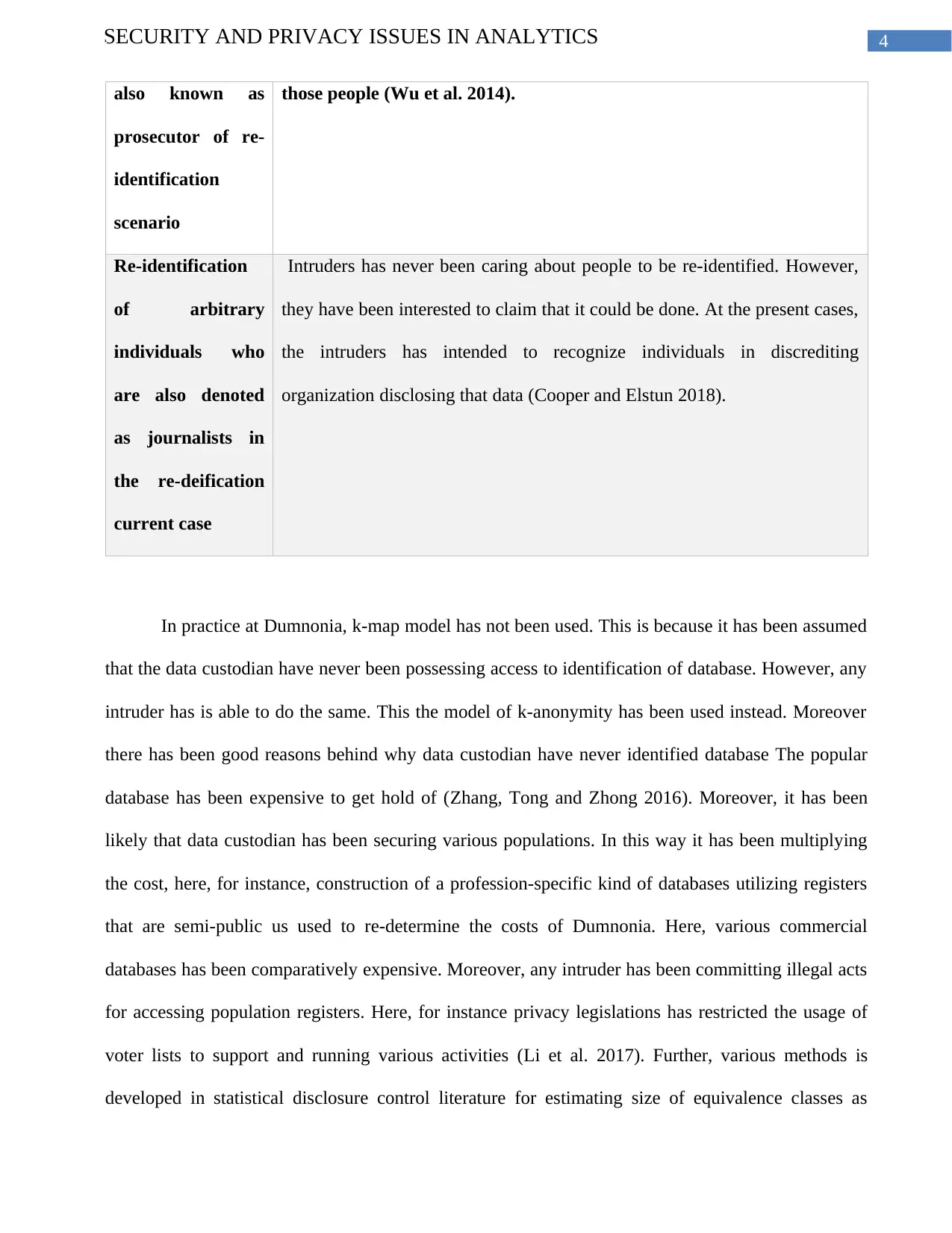
4SECURITY AND PRIVACY ISSUES IN ANALYTICS
also known as
prosecutor of re-
identification
scenario
those people (Wu et al. 2014).
Re-identification
of arbitrary
individuals who
are also denoted
as journalists in
the re-deification
current case
Intruders has never been caring about people to be re-identified. However,
they have been interested to claim that it could be done. At the present cases,
the intruders has intended to recognize individuals in discrediting
organization disclosing that data (Cooper and Elstun 2018).
In practice at Dumnonia, k-map model has not been used. This is because it has been assumed
that the data custodian have never been possessing access to identification of database. However, any
intruder has is able to do the same. This the model of k-anonymity has been used instead. Moreover
there has been good reasons behind why data custodian have never identified database The popular
database has been expensive to get hold of (Zhang, Tong and Zhong 2016). Moreover, it has been
likely that data custodian has been securing various populations. In this way it has been multiplying
the cost, here, for instance, construction of a profession-specific kind of databases utilizing registers
that are semi-public us used to re-determine the costs of Dumnonia. Here, various commercial
databases has been comparatively expensive. Moreover, any intruder has been committing illegal acts
for accessing population registers. Here, for instance privacy legislations has restricted the usage of
voter lists to support and running various activities (Li et al. 2017). Further, various methods is
developed in statistical disclosure control literature for estimating size of equivalence classes as
also known as
prosecutor of re-
identification
scenario
those people (Wu et al. 2014).
Re-identification
of arbitrary
individuals who
are also denoted
as journalists in
the re-deification
current case
Intruders has never been caring about people to be re-identified. However,
they have been interested to claim that it could be done. At the present cases,
the intruders has intended to recognize individuals in discrediting
organization disclosing that data (Cooper and Elstun 2018).
In practice at Dumnonia, k-map model has not been used. This is because it has been assumed
that the data custodian have never been possessing access to identification of database. However, any
intruder has is able to do the same. This the model of k-anonymity has been used instead. Moreover
there has been good reasons behind why data custodian have never identified database The popular
database has been expensive to get hold of (Zhang, Tong and Zhong 2016). Moreover, it has been
likely that data custodian has been securing various populations. In this way it has been multiplying
the cost, here, for instance, construction of a profession-specific kind of databases utilizing registers
that are semi-public us used to re-determine the costs of Dumnonia. Here, various commercial
databases has been comparatively expensive. Moreover, any intruder has been committing illegal acts
for accessing population registers. Here, for instance privacy legislations has restricted the usage of
voter lists to support and running various activities (Li et al. 2017). Further, various methods is
developed in statistical disclosure control literature for estimating size of equivalence classes as
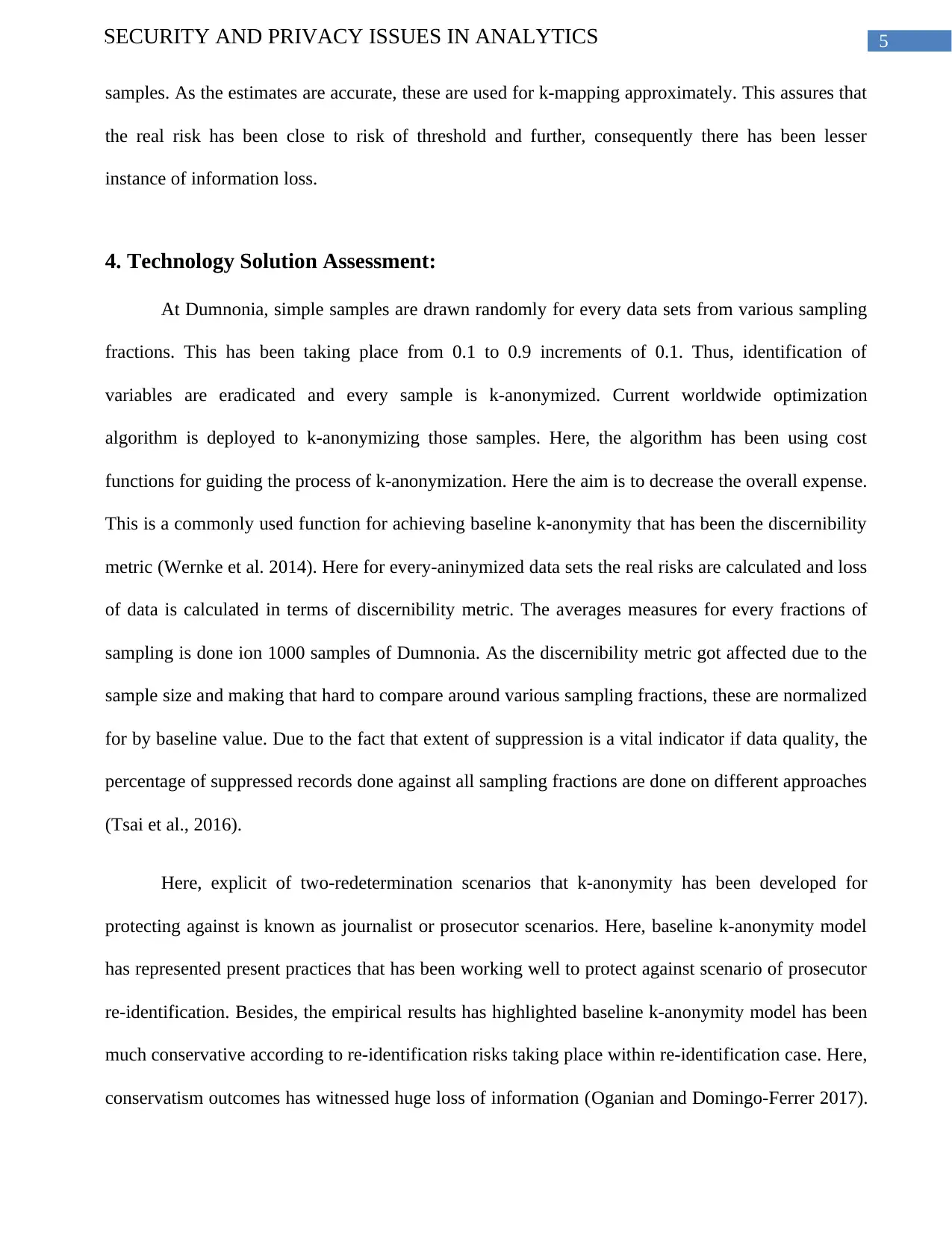
5SECURITY AND PRIVACY ISSUES IN ANALYTICS
samples. As the estimates are accurate, these are used for k-mapping approximately. This assures that
the real risk has been close to risk of threshold and further, consequently there has been lesser
instance of information loss.
4. Technology Solution Assessment:
At Dumnonia, simple samples are drawn randomly for every data sets from various sampling
fractions. This has been taking place from 0.1 to 0.9 increments of 0.1. Thus, identification of
variables are eradicated and every sample is k-anonymized. Current worldwide optimization
algorithm is deployed to k-anonymizing those samples. Here, the algorithm has been using cost
functions for guiding the process of k-anonymization. Here the aim is to decrease the overall expense.
This is a commonly used function for achieving baseline k-anonymity that has been the discernibility
metric (Wernke et al. 2014). Here for every-aninymized data sets the real risks are calculated and loss
of data is calculated in terms of discernibility metric. The averages measures for every fractions of
sampling is done ion 1000 samples of Dumnonia. As the discernibility metric got affected due to the
sample size and making that hard to compare around various sampling fractions, these are normalized
for by baseline value. Due to the fact that extent of suppression is a vital indicator if data quality, the
percentage of suppressed records done against all sampling fractions are done on different approaches
(Tsai et al., 2016).
Here, explicit of two-redetermination scenarios that k-anonymity has been developed for
protecting against is known as journalist or prosecutor scenarios. Here, baseline k-anonymity model
has represented present practices that has been working well to protect against scenario of prosecutor
re-identification. Besides, the empirical results has highlighted baseline k-anonymity model has been
much conservative according to re-identification risks taking place within re-identification case. Here,
conservatism outcomes has witnessed huge loss of information (Oganian and Domingo-Ferrer 2017).
samples. As the estimates are accurate, these are used for k-mapping approximately. This assures that
the real risk has been close to risk of threshold and further, consequently there has been lesser
instance of information loss.
4. Technology Solution Assessment:
At Dumnonia, simple samples are drawn randomly for every data sets from various sampling
fractions. This has been taking place from 0.1 to 0.9 increments of 0.1. Thus, identification of
variables are eradicated and every sample is k-anonymized. Current worldwide optimization
algorithm is deployed to k-anonymizing those samples. Here, the algorithm has been using cost
functions for guiding the process of k-anonymization. Here the aim is to decrease the overall expense.
This is a commonly used function for achieving baseline k-anonymity that has been the discernibility
metric (Wernke et al. 2014). Here for every-aninymized data sets the real risks are calculated and loss
of data is calculated in terms of discernibility metric. The averages measures for every fractions of
sampling is done ion 1000 samples of Dumnonia. As the discernibility metric got affected due to the
sample size and making that hard to compare around various sampling fractions, these are normalized
for by baseline value. Due to the fact that extent of suppression is a vital indicator if data quality, the
percentage of suppressed records done against all sampling fractions are done on different approaches
(Tsai et al., 2016).
Here, explicit of two-redetermination scenarios that k-anonymity has been developed for
protecting against is known as journalist or prosecutor scenarios. Here, baseline k-anonymity model
has represented present practices that has been working well to protect against scenario of prosecutor
re-identification. Besides, the empirical results has highlighted baseline k-anonymity model has been
much conservative according to re-identification risks taking place within re-identification case. Here,
conservatism outcomes has witnessed huge loss of information (Oganian and Domingo-Ferrer 2017).
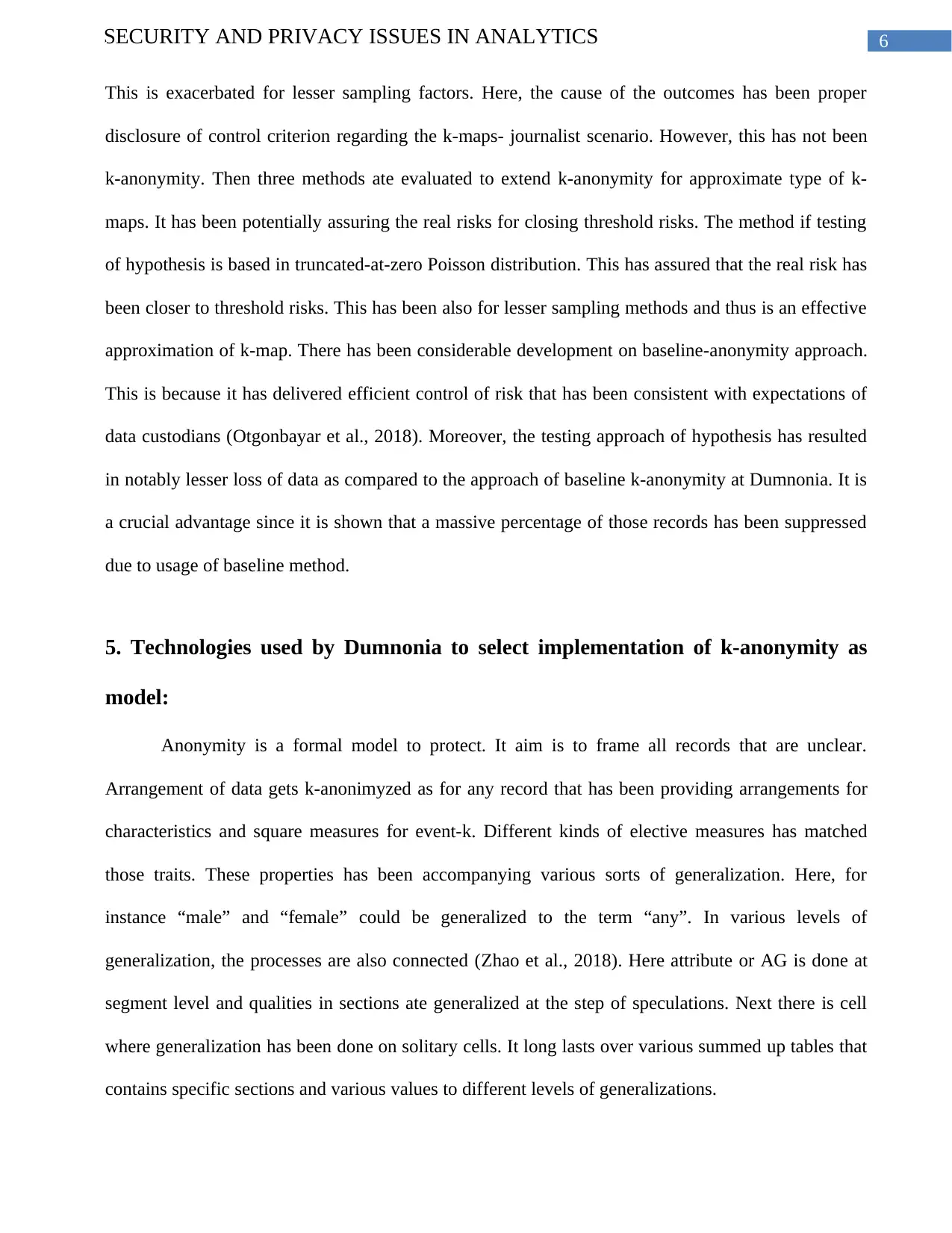
6SECURITY AND PRIVACY ISSUES IN ANALYTICS
This is exacerbated for lesser sampling factors. Here, the cause of the outcomes has been proper
disclosure of control criterion regarding the k-maps- journalist scenario. However, this has not been
k-anonymity. Then three methods ate evaluated to extend k-anonymity for approximate type of k-
maps. It has been potentially assuring the real risks for closing threshold risks. The method if testing
of hypothesis is based in truncated-at-zero Poisson distribution. This has assured that the real risk has
been closer to threshold risks. This has been also for lesser sampling methods and thus is an effective
approximation of k-map. There has been considerable development on baseline-anonymity approach.
This is because it has delivered efficient control of risk that has been consistent with expectations of
data custodians (Otgonbayar et al., 2018). Moreover, the testing approach of hypothesis has resulted
in notably lesser loss of data as compared to the approach of baseline k-anonymity at Dumnonia. It is
a crucial advantage since it is shown that a massive percentage of those records has been suppressed
due to usage of baseline method.
5. Technologies used by Dumnonia to select implementation of k-anonymity as
model:
Anonymity is a formal model to protect. It aim is to frame all records that are unclear.
Arrangement of data gets k-anonimyzed as for any record that has been providing arrangements for
characteristics and square measures for event-k. Different kinds of elective measures has matched
those traits. These properties has been accompanying various sorts of generalization. Here, for
instance “male” and “female” could be generalized to the term “any”. In various levels of
generalization, the processes are also connected (Zhao et al., 2018). Here attribute or AG is done at
segment level and qualities in sections ate generalized at the step of speculations. Next there is cell
where generalization has been done on solitary cells. It long lasts over various summed up tables that
contains specific sections and various values to different levels of generalizations.
This is exacerbated for lesser sampling factors. Here, the cause of the outcomes has been proper
disclosure of control criterion regarding the k-maps- journalist scenario. However, this has not been
k-anonymity. Then three methods ate evaluated to extend k-anonymity for approximate type of k-
maps. It has been potentially assuring the real risks for closing threshold risks. The method if testing
of hypothesis is based in truncated-at-zero Poisson distribution. This has assured that the real risk has
been closer to threshold risks. This has been also for lesser sampling methods and thus is an effective
approximation of k-map. There has been considerable development on baseline-anonymity approach.
This is because it has delivered efficient control of risk that has been consistent with expectations of
data custodians (Otgonbayar et al., 2018). Moreover, the testing approach of hypothesis has resulted
in notably lesser loss of data as compared to the approach of baseline k-anonymity at Dumnonia. It is
a crucial advantage since it is shown that a massive percentage of those records has been suppressed
due to usage of baseline method.
5. Technologies used by Dumnonia to select implementation of k-anonymity as
model:
Anonymity is a formal model to protect. It aim is to frame all records that are unclear.
Arrangement of data gets k-anonimyzed as for any record that has been providing arrangements for
characteristics and square measures for event-k. Different kinds of elective measures has matched
those traits. These properties has been accompanying various sorts of generalization. Here, for
instance “male” and “female” could be generalized to the term “any”. In various levels of
generalization, the processes are also connected (Zhao et al., 2018). Here attribute or AG is done at
segment level and qualities in sections ate generalized at the step of speculations. Next there is cell
where generalization has been done on solitary cells. It long lasts over various summed up tables that
contains specific sections and various values to different levels of generalizations.
Paraphrase This Document
Need a fresh take? Get an instant paraphrase of this document with our AI Paraphraser
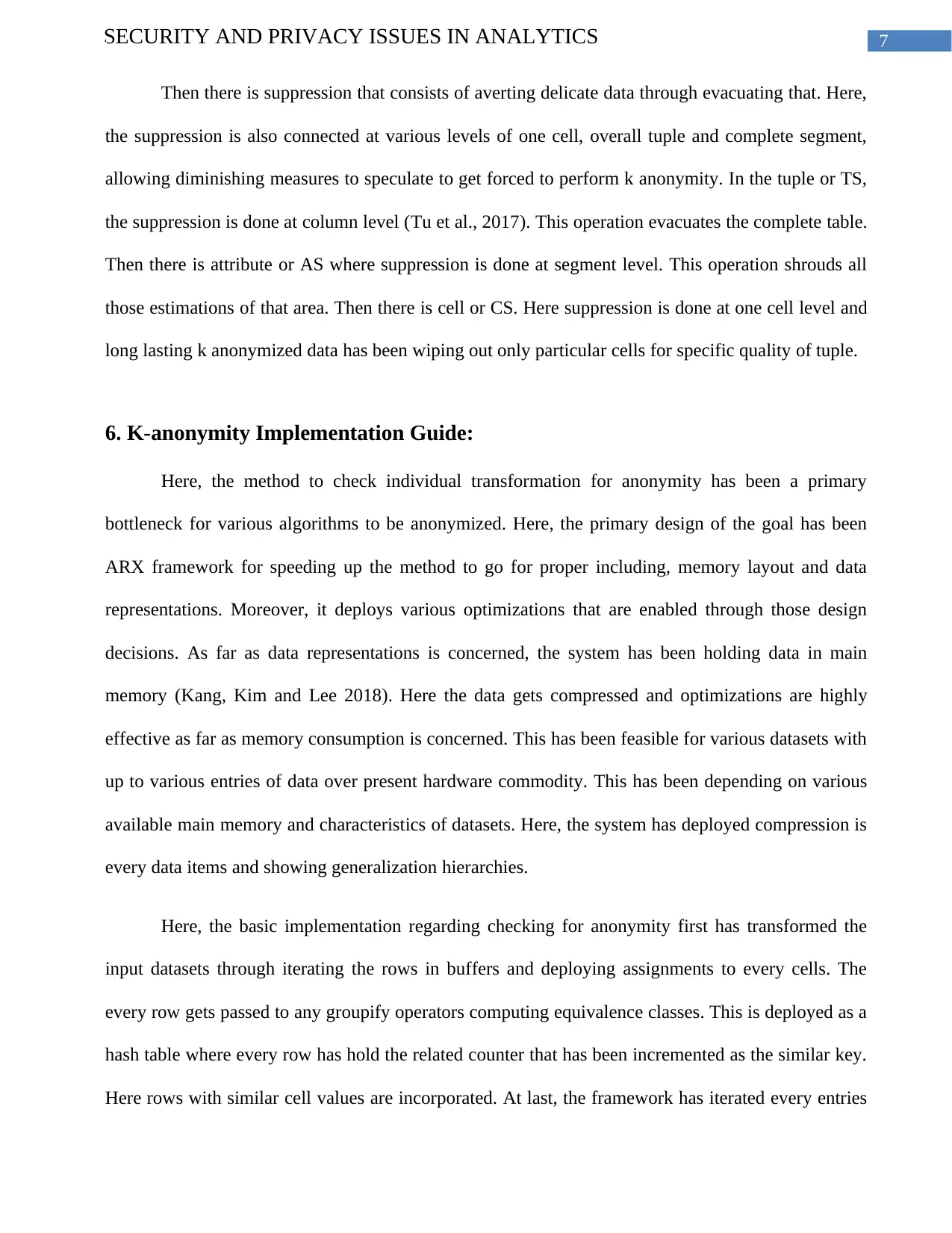
7SECURITY AND PRIVACY ISSUES IN ANALYTICS
Then there is suppression that consists of averting delicate data through evacuating that. Here,
the suppression is also connected at various levels of one cell, overall tuple and complete segment,
allowing diminishing measures to speculate to get forced to perform k anonymity. In the tuple or TS,
the suppression is done at column level (Tu et al., 2017). This operation evacuates the complete table.
Then there is attribute or AS where suppression is done at segment level. This operation shrouds all
those estimations of that area. Then there is cell or CS. Here suppression is done at one cell level and
long lasting k anonymized data has been wiping out only particular cells for specific quality of tuple.
6. K-anonymity Implementation Guide:
Here, the method to check individual transformation for anonymity has been a primary
bottleneck for various algorithms to be anonymized. Here, the primary design of the goal has been
ARX framework for speeding up the method to go for proper including, memory layout and data
representations. Moreover, it deploys various optimizations that are enabled through those design
decisions. As far as data representations is concerned, the system has been holding data in main
memory (Kang, Kim and Lee 2018). Here the data gets compressed and optimizations are highly
effective as far as memory consumption is concerned. This has been feasible for various datasets with
up to various entries of data over present hardware commodity. This has been depending on various
available main memory and characteristics of datasets. Here, the system has deployed compression is
every data items and showing generalization hierarchies.
Here, the basic implementation regarding checking for anonymity first has transformed the
input datasets through iterating the rows in buffers and deploying assignments to every cells. The
every row gets passed to any groupify operators computing equivalence classes. This is deployed as a
hash table where every row has hold the related counter that has been incremented as the similar key.
Here rows with similar cell values are incorporated. At last, the framework has iterated every entries
Then there is suppression that consists of averting delicate data through evacuating that. Here,
the suppression is also connected at various levels of one cell, overall tuple and complete segment,
allowing diminishing measures to speculate to get forced to perform k anonymity. In the tuple or TS,
the suppression is done at column level (Tu et al., 2017). This operation evacuates the complete table.
Then there is attribute or AS where suppression is done at segment level. This operation shrouds all
those estimations of that area. Then there is cell or CS. Here suppression is done at one cell level and
long lasting k anonymized data has been wiping out only particular cells for specific quality of tuple.
6. K-anonymity Implementation Guide:
Here, the method to check individual transformation for anonymity has been a primary
bottleneck for various algorithms to be anonymized. Here, the primary design of the goal has been
ARX framework for speeding up the method to go for proper including, memory layout and data
representations. Moreover, it deploys various optimizations that are enabled through those design
decisions. As far as data representations is concerned, the system has been holding data in main
memory (Kang, Kim and Lee 2018). Here the data gets compressed and optimizations are highly
effective as far as memory consumption is concerned. This has been feasible for various datasets with
up to various entries of data over present hardware commodity. This has been depending on various
available main memory and characteristics of datasets. Here, the system has deployed compression is
every data items and showing generalization hierarchies.
Here, the basic implementation regarding checking for anonymity first has transformed the
input datasets through iterating the rows in buffers and deploying assignments to every cells. The
every row gets passed to any groupify operators computing equivalence classes. This is deployed as a
hash table where every row has hold the related counter that has been incremented as the similar key.
Here rows with similar cell values are incorporated. At last, the framework has iterated every entries
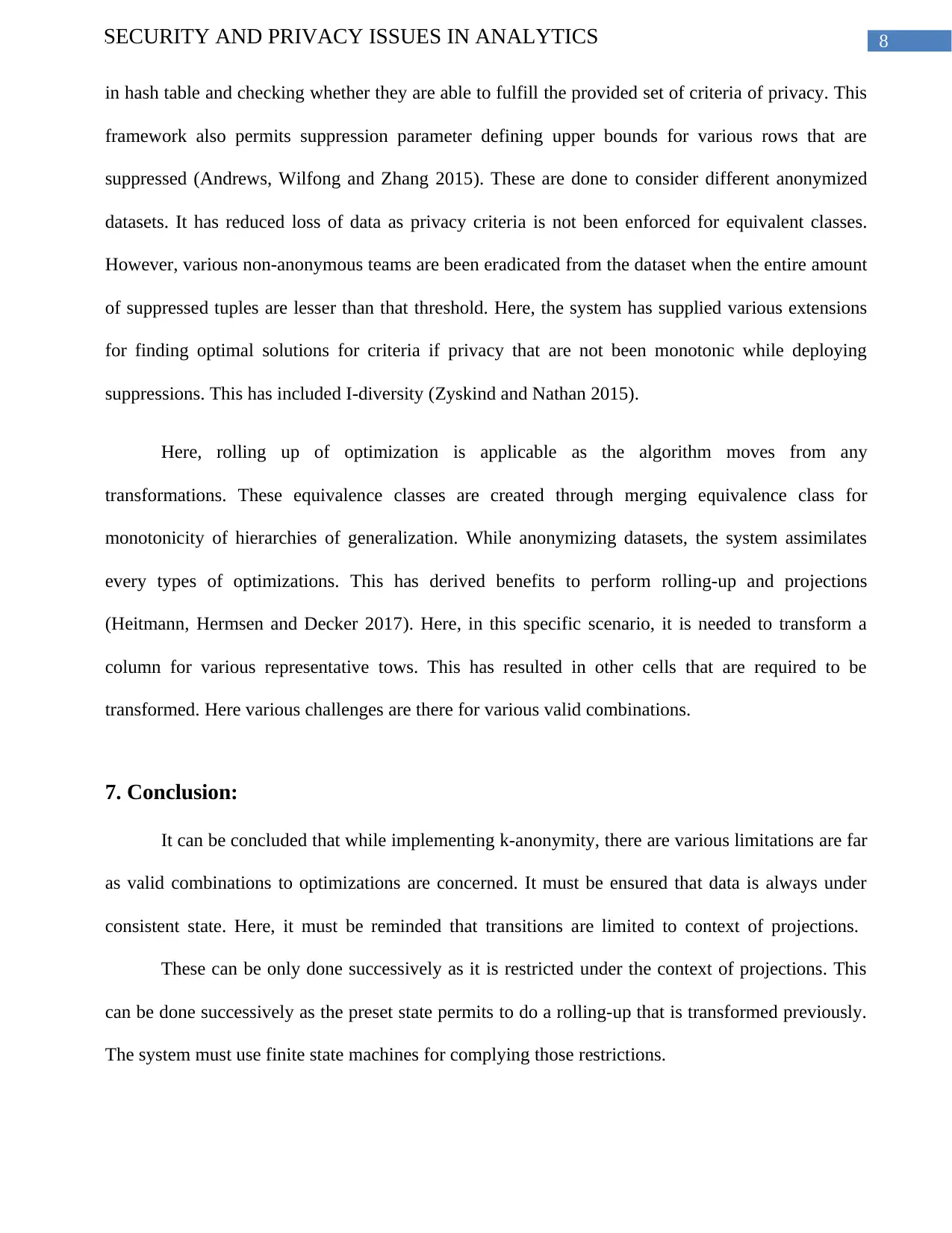
8SECURITY AND PRIVACY ISSUES IN ANALYTICS
in hash table and checking whether they are able to fulfill the provided set of criteria of privacy. This
framework also permits suppression parameter defining upper bounds for various rows that are
suppressed (Andrews, Wilfong and Zhang 2015). These are done to consider different anonymized
datasets. It has reduced loss of data as privacy criteria is not been enforced for equivalent classes.
However, various non-anonymous teams are been eradicated from the dataset when the entire amount
of suppressed tuples are lesser than that threshold. Here, the system has supplied various extensions
for finding optimal solutions for criteria if privacy that are not been monotonic while deploying
suppressions. This has included I-diversity (Zyskind and Nathan 2015).
Here, rolling up of optimization is applicable as the algorithm moves from any
transformations. These equivalence classes are created through merging equivalence class for
monotonicity of hierarchies of generalization. While anonymizing datasets, the system assimilates
every types of optimizations. This has derived benefits to perform rolling-up and projections
(Heitmann, Hermsen and Decker 2017). Here, in this specific scenario, it is needed to transform a
column for various representative tows. This has resulted in other cells that are required to be
transformed. Here various challenges are there for various valid combinations.
7. Conclusion:
It can be concluded that while implementing k-anonymity, there are various limitations are far
as valid combinations to optimizations are concerned. It must be ensured that data is always under
consistent state. Here, it must be reminded that transitions are limited to context of projections.
These can be only done successively as it is restricted under the context of projections. This
can be done successively as the preset state permits to do a rolling-up that is transformed previously.
The system must use finite state machines for complying those restrictions.
in hash table and checking whether they are able to fulfill the provided set of criteria of privacy. This
framework also permits suppression parameter defining upper bounds for various rows that are
suppressed (Andrews, Wilfong and Zhang 2015). These are done to consider different anonymized
datasets. It has reduced loss of data as privacy criteria is not been enforced for equivalent classes.
However, various non-anonymous teams are been eradicated from the dataset when the entire amount
of suppressed tuples are lesser than that threshold. Here, the system has supplied various extensions
for finding optimal solutions for criteria if privacy that are not been monotonic while deploying
suppressions. This has included I-diversity (Zyskind and Nathan 2015).
Here, rolling up of optimization is applicable as the algorithm moves from any
transformations. These equivalence classes are created through merging equivalence class for
monotonicity of hierarchies of generalization. While anonymizing datasets, the system assimilates
every types of optimizations. This has derived benefits to perform rolling-up and projections
(Heitmann, Hermsen and Decker 2017). Here, in this specific scenario, it is needed to transform a
column for various representative tows. This has resulted in other cells that are required to be
transformed. Here various challenges are there for various valid combinations.
7. Conclusion:
It can be concluded that while implementing k-anonymity, there are various limitations are far
as valid combinations to optimizations are concerned. It must be ensured that data is always under
consistent state. Here, it must be reminded that transitions are limited to context of projections.
These can be only done successively as it is restricted under the context of projections. This
can be done successively as the preset state permits to do a rolling-up that is transformed previously.
The system must use finite state machines for complying those restrictions.
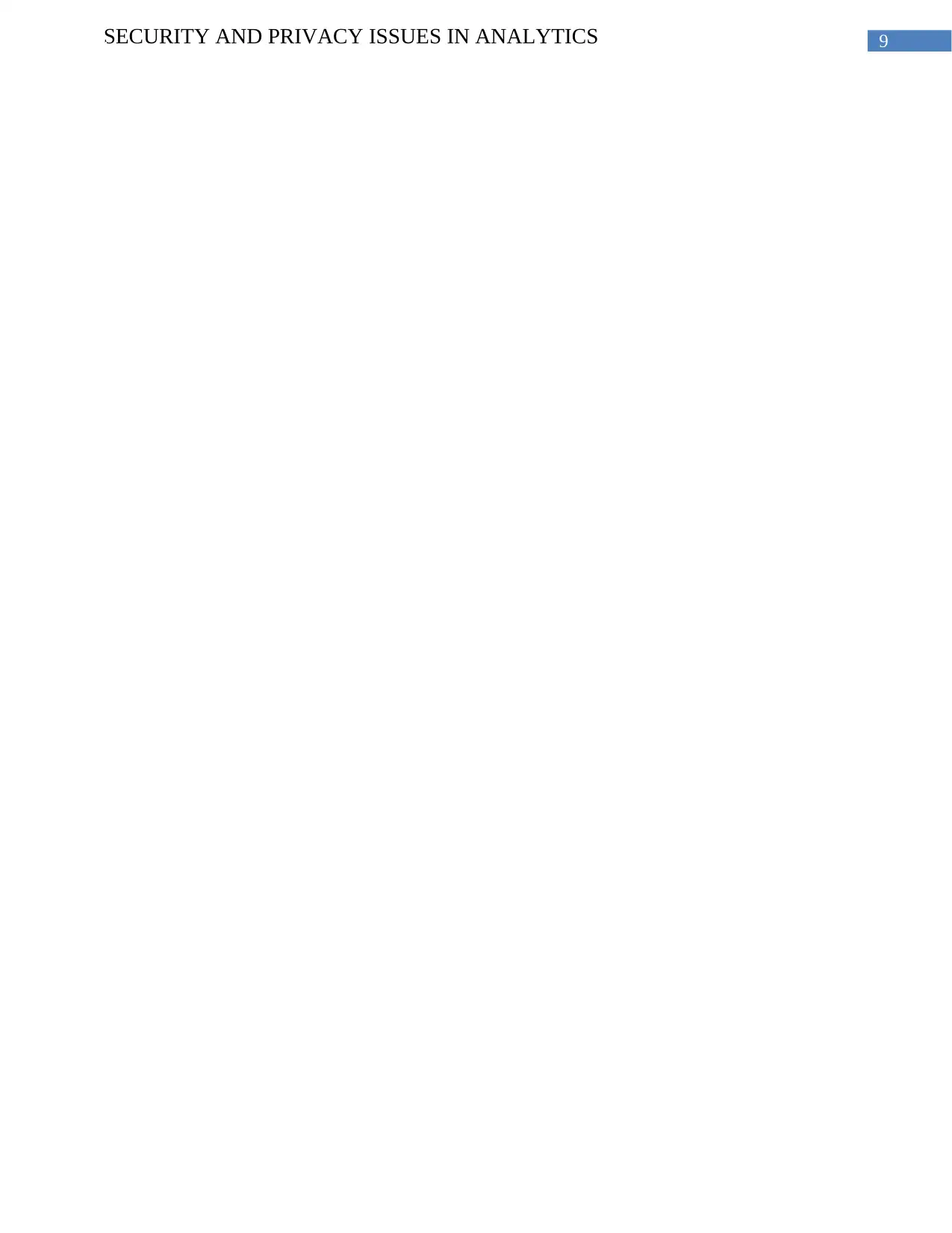
9SECURITY AND PRIVACY ISSUES IN ANALYTICS
Secure Best Marks with AI Grader
Need help grading? Try our AI Grader for instant feedback on your assignments.
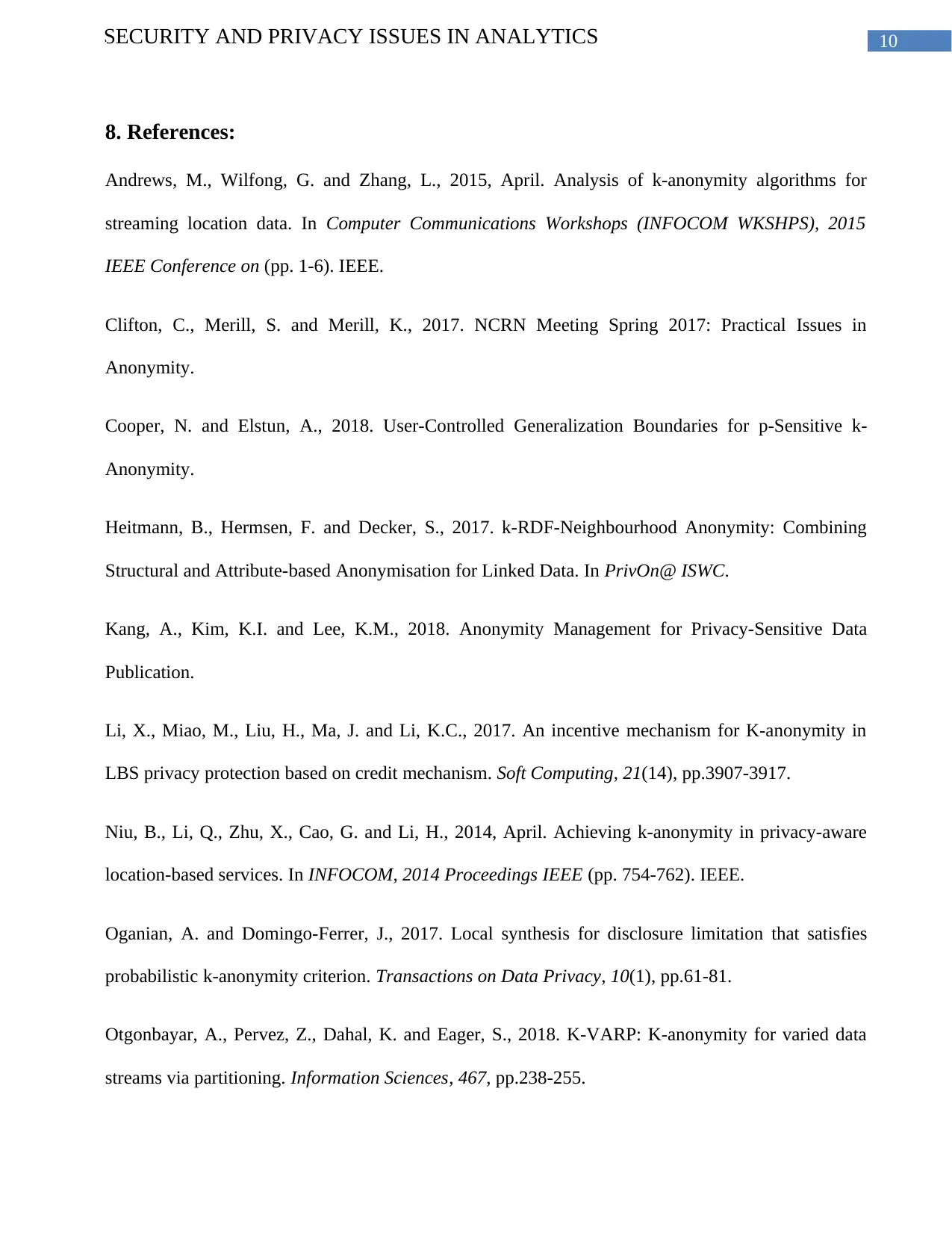
10SECURITY AND PRIVACY ISSUES IN ANALYTICS
8. References:
Andrews, M., Wilfong, G. and Zhang, L., 2015, April. Analysis of k-anonymity algorithms for
streaming location data. In Computer Communications Workshops (INFOCOM WKSHPS), 2015
IEEE Conference on (pp. 1-6). IEEE.
Clifton, C., Merill, S. and Merill, K., 2017. NCRN Meeting Spring 2017: Practical Issues in
Anonymity.
Cooper, N. and Elstun, A., 2018. User-Controlled Generalization Boundaries for p-Sensitive k-
Anonymity.
Heitmann, B., Hermsen, F. and Decker, S., 2017. k-RDF-Neighbourhood Anonymity: Combining
Structural and Attribute-based Anonymisation for Linked Data. In PrivOn@ ISWC.
Kang, A., Kim, K.I. and Lee, K.M., 2018. Anonymity Management for Privacy-Sensitive Data
Publication.
Li, X., Miao, M., Liu, H., Ma, J. and Li, K.C., 2017. An incentive mechanism for K-anonymity in
LBS privacy protection based on credit mechanism. Soft Computing, 21(14), pp.3907-3917.
Niu, B., Li, Q., Zhu, X., Cao, G. and Li, H., 2014, April. Achieving k-anonymity in privacy-aware
location-based services. In INFOCOM, 2014 Proceedings IEEE (pp. 754-762). IEEE.
Oganian, A. and Domingo-Ferrer, J., 2017. Local synthesis for disclosure limitation that satisfies
probabilistic k-anonymity criterion. Transactions on Data Privacy, 10(1), pp.61-81.
Otgonbayar, A., Pervez, Z., Dahal, K. and Eager, S., 2018. K-VARP: K-anonymity for varied data
streams via partitioning. Information Sciences, 467, pp.238-255.
8. References:
Andrews, M., Wilfong, G. and Zhang, L., 2015, April. Analysis of k-anonymity algorithms for
streaming location data. In Computer Communications Workshops (INFOCOM WKSHPS), 2015
IEEE Conference on (pp. 1-6). IEEE.
Clifton, C., Merill, S. and Merill, K., 2017. NCRN Meeting Spring 2017: Practical Issues in
Anonymity.
Cooper, N. and Elstun, A., 2018. User-Controlled Generalization Boundaries for p-Sensitive k-
Anonymity.
Heitmann, B., Hermsen, F. and Decker, S., 2017. k-RDF-Neighbourhood Anonymity: Combining
Structural and Attribute-based Anonymisation for Linked Data. In PrivOn@ ISWC.
Kang, A., Kim, K.I. and Lee, K.M., 2018. Anonymity Management for Privacy-Sensitive Data
Publication.
Li, X., Miao, M., Liu, H., Ma, J. and Li, K.C., 2017. An incentive mechanism for K-anonymity in
LBS privacy protection based on credit mechanism. Soft Computing, 21(14), pp.3907-3917.
Niu, B., Li, Q., Zhu, X., Cao, G. and Li, H., 2014, April. Achieving k-anonymity in privacy-aware
location-based services. In INFOCOM, 2014 Proceedings IEEE (pp. 754-762). IEEE.
Oganian, A. and Domingo-Ferrer, J., 2017. Local synthesis for disclosure limitation that satisfies
probabilistic k-anonymity criterion. Transactions on Data Privacy, 10(1), pp.61-81.
Otgonbayar, A., Pervez, Z., Dahal, K. and Eager, S., 2018. K-VARP: K-anonymity for varied data
streams via partitioning. Information Sciences, 467, pp.238-255.
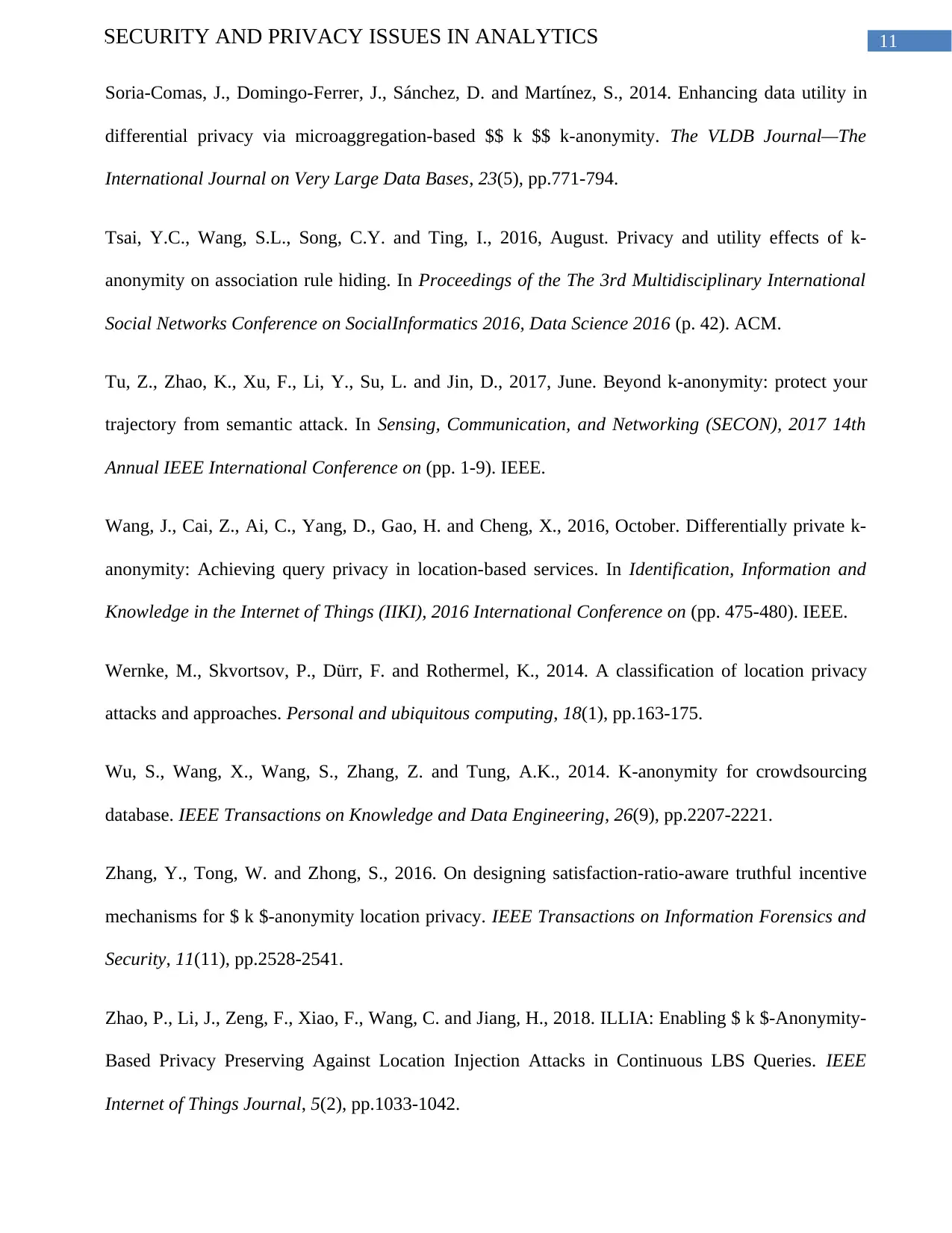
11SECURITY AND PRIVACY ISSUES IN ANALYTICS
Soria-Comas, J., Domingo-Ferrer, J., Sánchez, D. and Martínez, S., 2014. Enhancing data utility in
differential privacy via microaggregation-based $$ k $$ k-anonymity. The VLDB Journal—The
International Journal on Very Large Data Bases, 23(5), pp.771-794.
Tsai, Y.C., Wang, S.L., Song, C.Y. and Ting, I., 2016, August. Privacy and utility effects of k-
anonymity on association rule hiding. In Proceedings of the The 3rd Multidisciplinary International
Social Networks Conference on SocialInformatics 2016, Data Science 2016 (p. 42). ACM.
Tu, Z., Zhao, K., Xu, F., Li, Y., Su, L. and Jin, D., 2017, June. Beyond k-anonymity: protect your
trajectory from semantic attack. In Sensing, Communication, and Networking (SECON), 2017 14th
Annual IEEE International Conference on (pp. 1-9). IEEE.
Wang, J., Cai, Z., Ai, C., Yang, D., Gao, H. and Cheng, X., 2016, October. Differentially private k-
anonymity: Achieving query privacy in location-based services. In Identification, Information and
Knowledge in the Internet of Things (IIKI), 2016 International Conference on (pp. 475-480). IEEE.
Wernke, M., Skvortsov, P., Dürr, F. and Rothermel, K., 2014. A classification of location privacy
attacks and approaches. Personal and ubiquitous computing, 18(1), pp.163-175.
Wu, S., Wang, X., Wang, S., Zhang, Z. and Tung, A.K., 2014. K-anonymity for crowdsourcing
database. IEEE Transactions on Knowledge and Data Engineering, 26(9), pp.2207-2221.
Zhang, Y., Tong, W. and Zhong, S., 2016. On designing satisfaction-ratio-aware truthful incentive
mechanisms for $ k $-anonymity location privacy. IEEE Transactions on Information Forensics and
Security, 11(11), pp.2528-2541.
Zhao, P., Li, J., Zeng, F., Xiao, F., Wang, C. and Jiang, H., 2018. ILLIA: Enabling $ k $-Anonymity-
Based Privacy Preserving Against Location Injection Attacks in Continuous LBS Queries. IEEE
Internet of Things Journal, 5(2), pp.1033-1042.
Soria-Comas, J., Domingo-Ferrer, J., Sánchez, D. and Martínez, S., 2014. Enhancing data utility in
differential privacy via microaggregation-based $$ k $$ k-anonymity. The VLDB Journal—The
International Journal on Very Large Data Bases, 23(5), pp.771-794.
Tsai, Y.C., Wang, S.L., Song, C.Y. and Ting, I., 2016, August. Privacy and utility effects of k-
anonymity on association rule hiding. In Proceedings of the The 3rd Multidisciplinary International
Social Networks Conference on SocialInformatics 2016, Data Science 2016 (p. 42). ACM.
Tu, Z., Zhao, K., Xu, F., Li, Y., Su, L. and Jin, D., 2017, June. Beyond k-anonymity: protect your
trajectory from semantic attack. In Sensing, Communication, and Networking (SECON), 2017 14th
Annual IEEE International Conference on (pp. 1-9). IEEE.
Wang, J., Cai, Z., Ai, C., Yang, D., Gao, H. and Cheng, X., 2016, October. Differentially private k-
anonymity: Achieving query privacy in location-based services. In Identification, Information and
Knowledge in the Internet of Things (IIKI), 2016 International Conference on (pp. 475-480). IEEE.
Wernke, M., Skvortsov, P., Dürr, F. and Rothermel, K., 2014. A classification of location privacy
attacks and approaches. Personal and ubiquitous computing, 18(1), pp.163-175.
Wu, S., Wang, X., Wang, S., Zhang, Z. and Tung, A.K., 2014. K-anonymity for crowdsourcing
database. IEEE Transactions on Knowledge and Data Engineering, 26(9), pp.2207-2221.
Zhang, Y., Tong, W. and Zhong, S., 2016. On designing satisfaction-ratio-aware truthful incentive
mechanisms for $ k $-anonymity location privacy. IEEE Transactions on Information Forensics and
Security, 11(11), pp.2528-2541.
Zhao, P., Li, J., Zeng, F., Xiao, F., Wang, C. and Jiang, H., 2018. ILLIA: Enabling $ k $-Anonymity-
Based Privacy Preserving Against Location Injection Attacks in Continuous LBS Queries. IEEE
Internet of Things Journal, 5(2), pp.1033-1042.
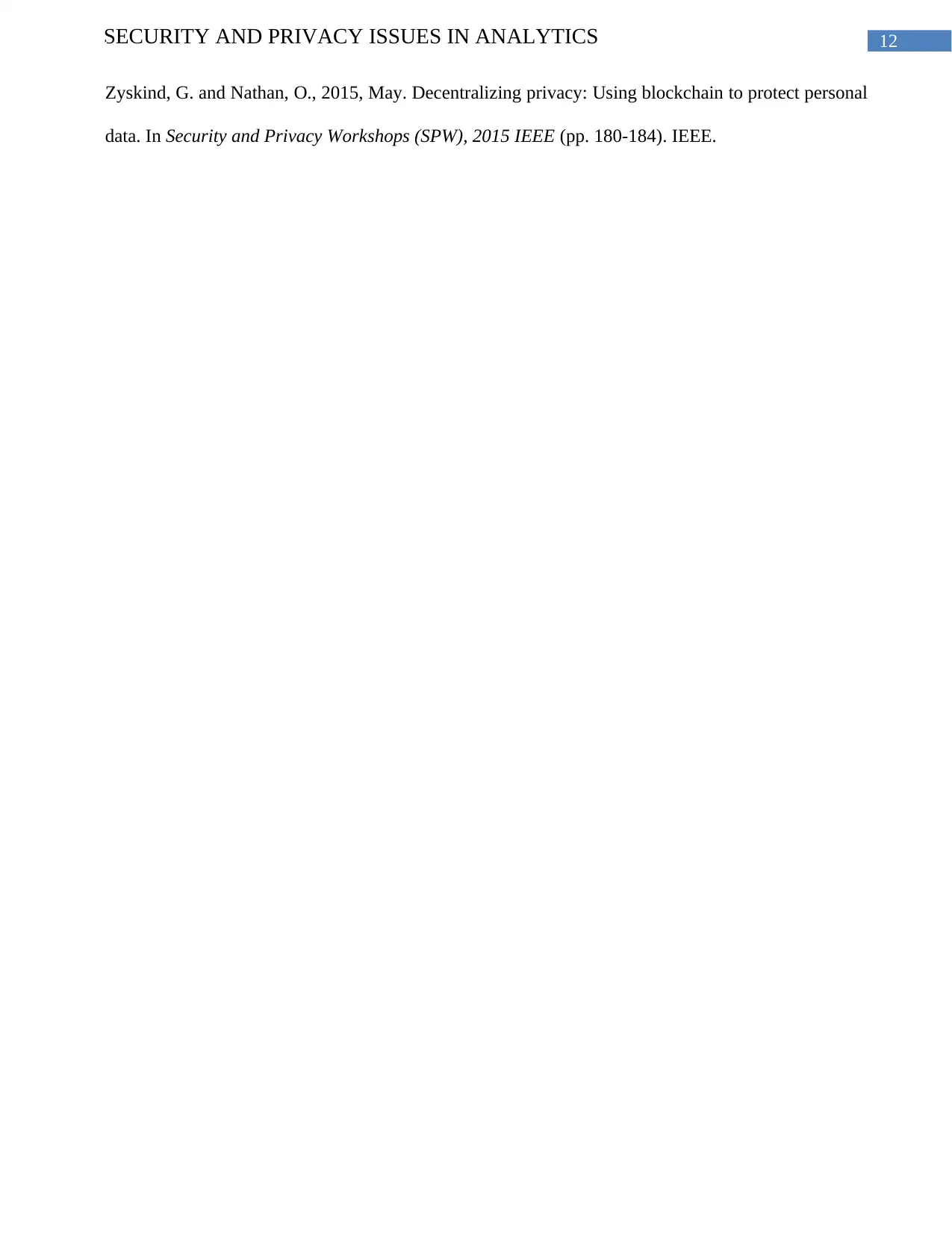
12SECURITY AND PRIVACY ISSUES IN ANALYTICS
Zyskind, G. and Nathan, O., 2015, May. Decentralizing privacy: Using blockchain to protect personal
data. In Security and Privacy Workshops (SPW), 2015 IEEE (pp. 180-184). IEEE.
Zyskind, G. and Nathan, O., 2015, May. Decentralizing privacy: Using blockchain to protect personal
data. In Security and Privacy Workshops (SPW), 2015 IEEE (pp. 180-184). IEEE.
1 out of 13
Related Documents
![[object Object]](/_next/image/?url=%2F_next%2Fstatic%2Fmedia%2Flogo.6d15ce61.png&w=640&q=75)
Your All-in-One AI-Powered Toolkit for Academic Success.
+13062052269
info@desklib.com
Available 24*7 on WhatsApp / Email
Unlock your academic potential
© 2024 | Zucol Services PVT LTD | All rights reserved.