Statistical Analysis of Furniture Order Values and Mobile Phone Sales
VerifiedAdded on 2024/06/03
|11
|1548
|465
AI Summary
This report delves into the analysis of furniture order values and mobile phone sales data using various statistical tools and techniques. It explores measures of central tendency, ANOVA, regression analysis, and other relevant concepts to understand the relationships and patterns within the data. The report aims to provide insights into the factors influencing furniture order values and mobile phone sales, highlighting key findings and potential implications for business decision-making.
Contribute Materials
Your contribution can guide someone’s learning journey. Share your
documents today.
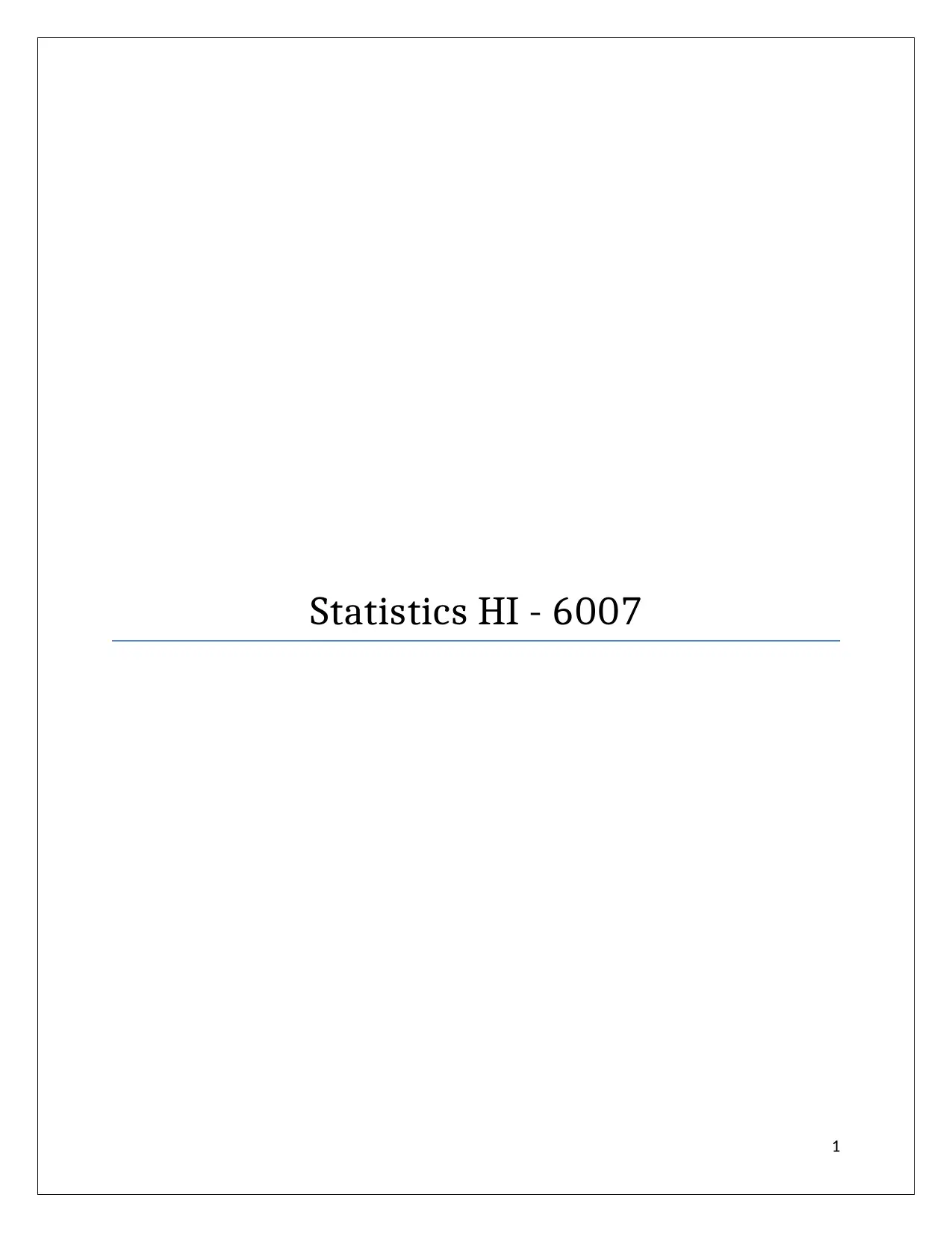
Statistics HI - 6007
1
1
Secure Best Marks with AI Grader
Need help grading? Try our AI Grader for instant feedback on your assignments.
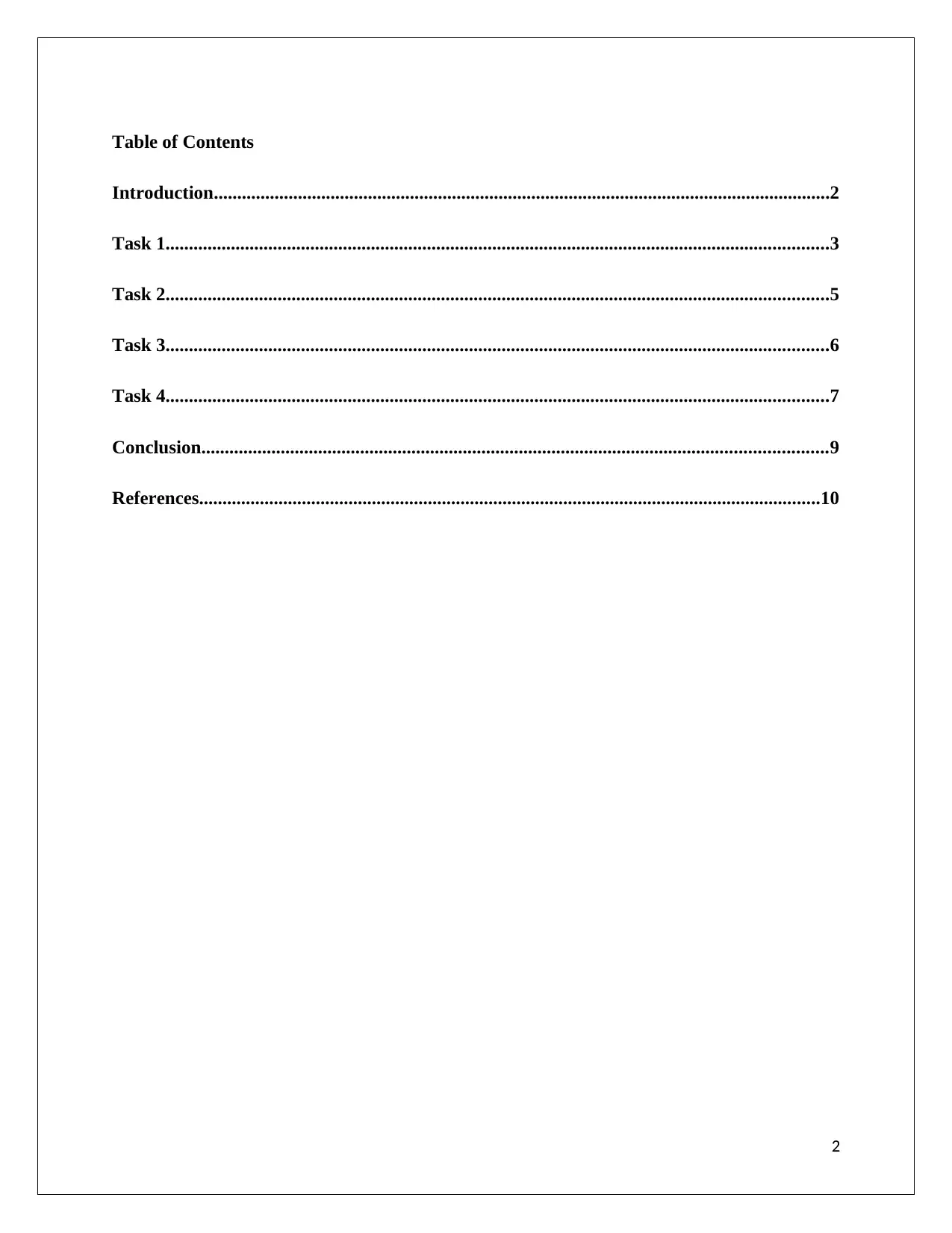
Table of Contents
Introduction....................................................................................................................................2
Task 1..............................................................................................................................................3
Task 2..............................................................................................................................................5
Task 3..............................................................................................................................................6
Task 4..............................................................................................................................................7
Conclusion......................................................................................................................................9
References.....................................................................................................................................10
2
Introduction....................................................................................................................................2
Task 1..............................................................................................................................................3
Task 2..............................................................................................................................................5
Task 3..............................................................................................................................................6
Task 4..............................................................................................................................................7
Conclusion......................................................................................................................................9
References.....................................................................................................................................10
2
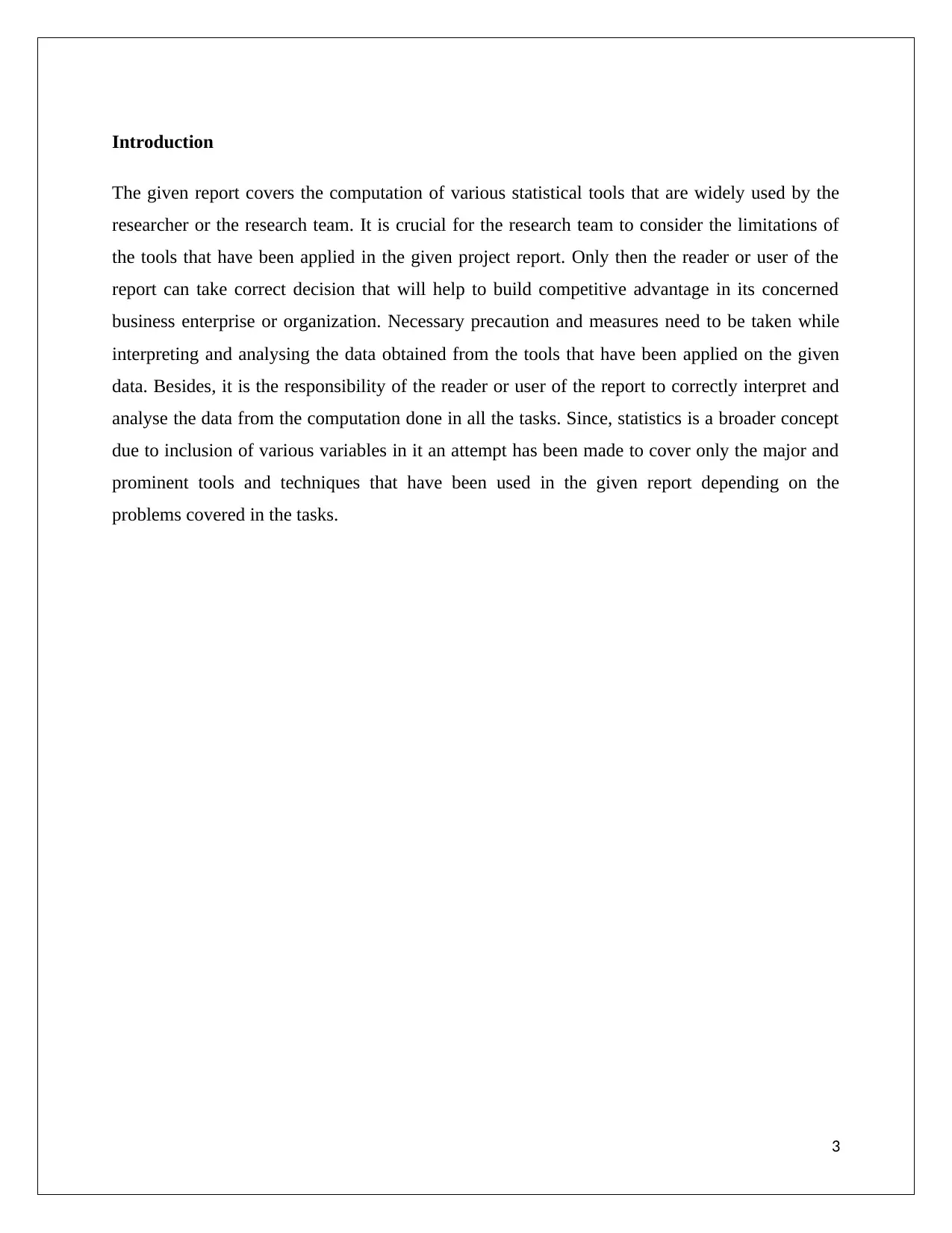
Introduction
The given report covers the computation of various statistical tools that are widely used by the
researcher or the research team. It is crucial for the research team to consider the limitations of
the tools that have been applied in the given project report. Only then the reader or user of the
report can take correct decision that will help to build competitive advantage in its concerned
business enterprise or organization. Necessary precaution and measures need to be taken while
interpreting and analysing the data obtained from the tools that have been applied on the given
data. Besides, it is the responsibility of the reader or user of the report to correctly interpret and
analyse the data from the computation done in all the tasks. Since, statistics is a broader concept
due to inclusion of various variables in it an attempt has been made to cover only the major and
prominent tools and techniques that have been used in the given report depending on the
problems covered in the tasks.
3
The given report covers the computation of various statistical tools that are widely used by the
researcher or the research team. It is crucial for the research team to consider the limitations of
the tools that have been applied in the given project report. Only then the reader or user of the
report can take correct decision that will help to build competitive advantage in its concerned
business enterprise or organization. Necessary precaution and measures need to be taken while
interpreting and analysing the data obtained from the tools that have been applied on the given
data. Besides, it is the responsibility of the reader or user of the report to correctly interpret and
analyse the data from the computation done in all the tasks. Since, statistics is a broader concept
due to inclusion of various variables in it an attempt has been made to cover only the major and
prominent tools and techniques that have been used in the given report depending on the
problems covered in the tasks.
3
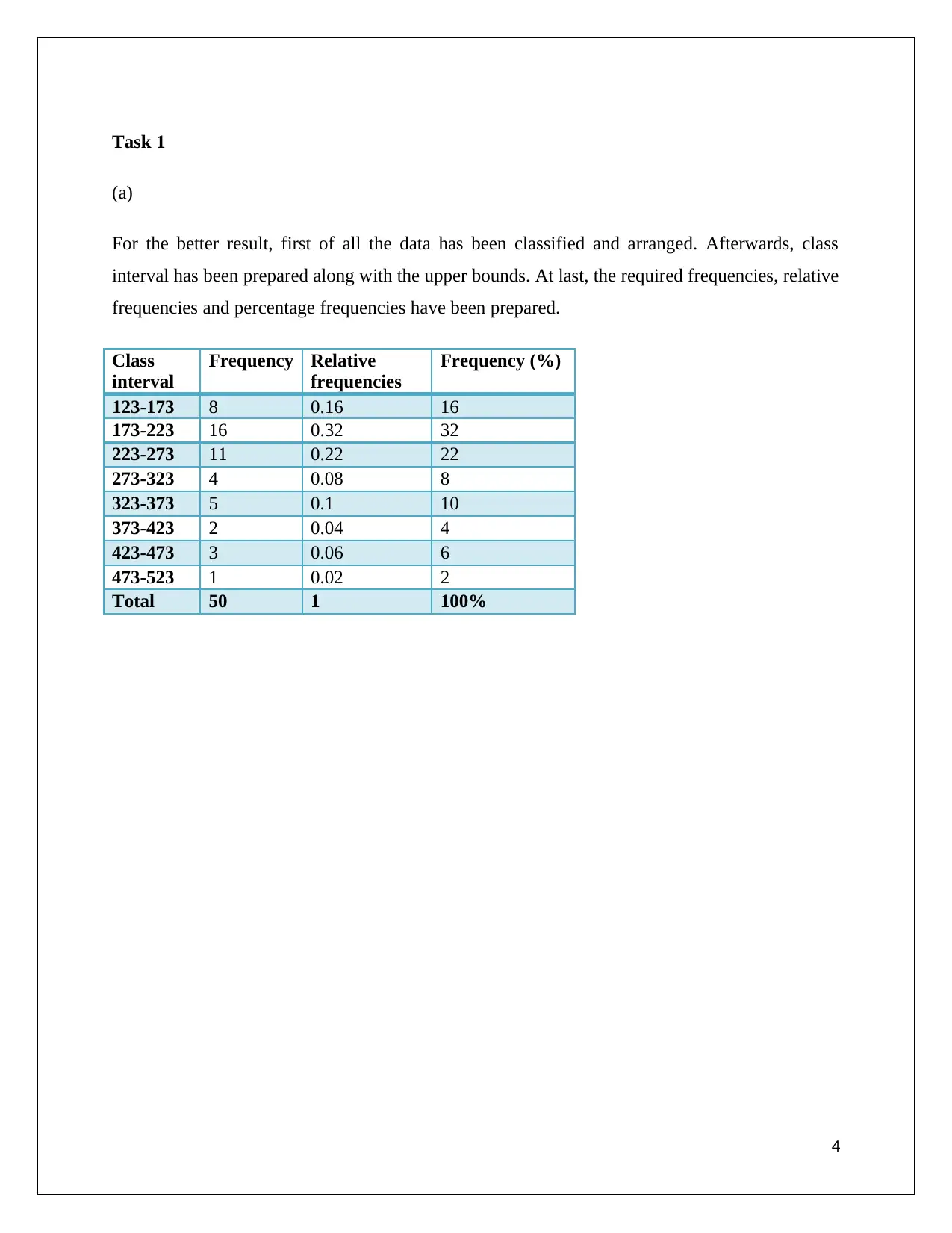
Task 1
(a)
For the better result, first of all the data has been classified and arranged. Afterwards, class
interval has been prepared along with the upper bounds. At last, the required frequencies, relative
frequencies and percentage frequencies have been prepared.
Class
interval
Frequency Relative
frequencies
Frequency (%)
123-173 8 0.16 16
173-223 16 0.32 32
223-273 11 0.22 22
273-323 4 0.08 8
323-373 5 0.1 10
373-423 2 0.04 4
423-473 3 0.06 6
473-523 1 0.02 2
Total 50 1 100%
4
(a)
For the better result, first of all the data has been classified and arranged. Afterwards, class
interval has been prepared along with the upper bounds. At last, the required frequencies, relative
frequencies and percentage frequencies have been prepared.
Class
interval
Frequency Relative
frequencies
Frequency (%)
123-173 8 0.16 16
173-223 16 0.32 32
223-273 11 0.22 22
273-323 4 0.08 8
323-373 5 0.1 10
373-423 2 0.04 4
423-473 3 0.06 6
473-523 1 0.02 2
Total 50 1 100%
4
Paraphrase This Document
Need a fresh take? Get an instant paraphrase of this document with our AI Paraphraser
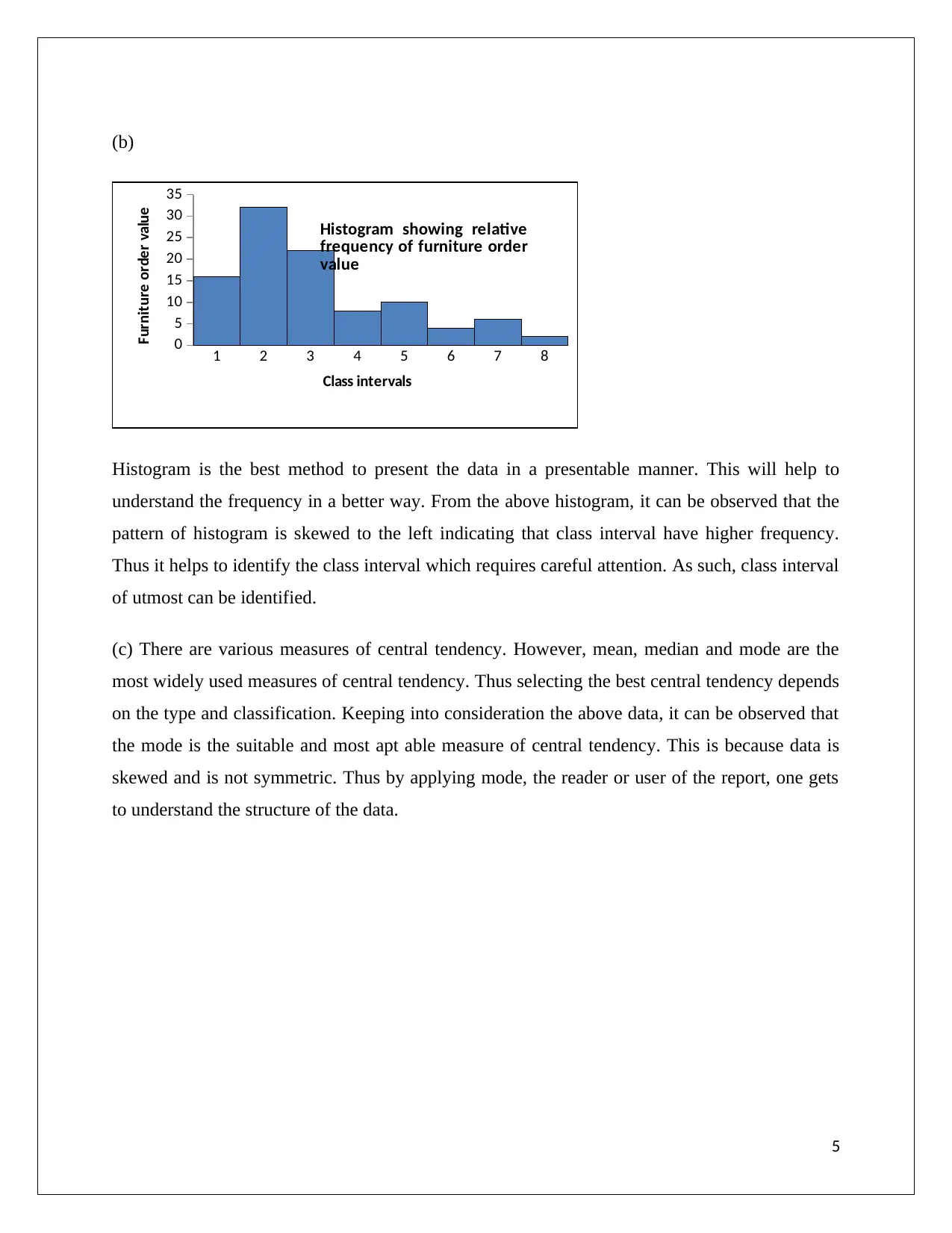
(b)
1 2 3 4 5 6 7 8
0
5
10
15
20
25
30
35
Class intervals
Furniture order value
Histogram showing relative
frequency of furniture order
value
Histogram is the best method to present the data in a presentable manner. This will help to
understand the frequency in a better way. From the above histogram, it can be observed that the
pattern of histogram is skewed to the left indicating that class interval have higher frequency.
Thus it helps to identify the class interval which requires careful attention. As such, class interval
of utmost can be identified.
(c) There are various measures of central tendency. However, mean, median and mode are the
most widely used measures of central tendency. Thus selecting the best central tendency depends
on the type and classification. Keeping into consideration the above data, it can be observed that
the mode is the suitable and most apt able measure of central tendency. This is because data is
skewed and is not symmetric. Thus by applying mode, the reader or user of the report, one gets
to understand the structure of the data.
5
1 2 3 4 5 6 7 8
0
5
10
15
20
25
30
35
Class intervals
Furniture order value
Histogram showing relative
frequency of furniture order
value
Histogram is the best method to present the data in a presentable manner. This will help to
understand the frequency in a better way. From the above histogram, it can be observed that the
pattern of histogram is skewed to the left indicating that class interval have higher frequency.
Thus it helps to identify the class interval which requires careful attention. As such, class interval
of utmost can be identified.
(c) There are various measures of central tendency. However, mean, median and mode are the
most widely used measures of central tendency. Thus selecting the best central tendency depends
on the type and classification. Keeping into consideration the above data, it can be observed that
the mode is the suitable and most apt able measure of central tendency. This is because data is
skewed and is not symmetric. Thus by applying mode, the reader or user of the report, one gets
to understand the structure of the data.
5
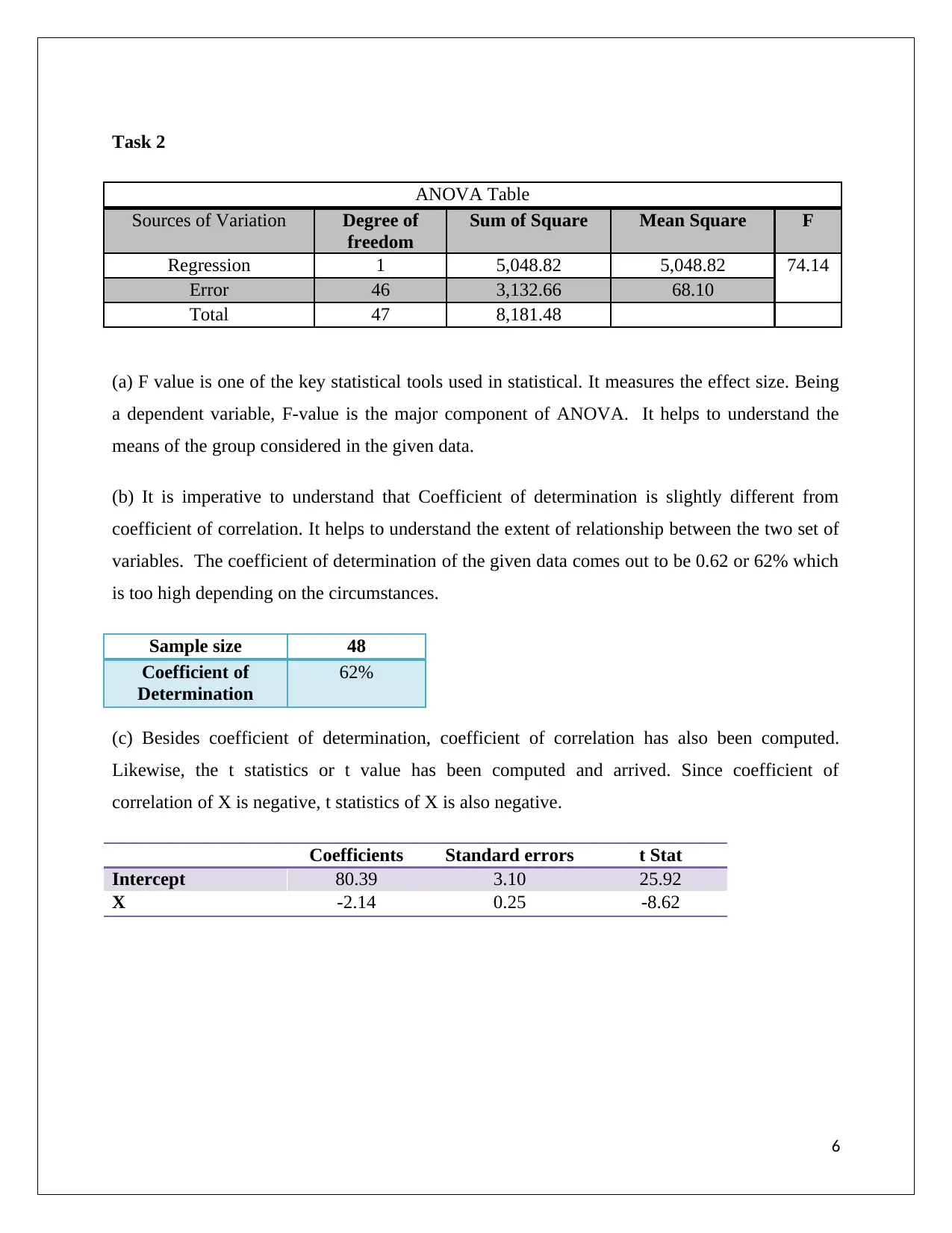
Task 2
ANOVA Table
Sources of Variation Degree of
freedom
Sum of Square Mean Square F
Regression 1 5,048.82 5,048.82 74.14
Error 46 3,132.66 68.10
Total 47 8,181.48
(a) F value is one of the key statistical tools used in statistical. It measures the effect size. Being
a dependent variable, F-value is the major component of ANOVA. It helps to understand the
means of the group considered in the given data.
(b) It is imperative to understand that Coefficient of determination is slightly different from
coefficient of correlation. It helps to understand the extent of relationship between the two set of
variables. The coefficient of determination of the given data comes out to be 0.62 or 62% which
is too high depending on the circumstances.
Sample size 48
Coefficient of
Determination
62%
(c) Besides coefficient of determination, coefficient of correlation has also been computed.
Likewise, the t statistics or t value has been computed and arrived. Since coefficient of
correlation of X is negative, t statistics of X is also negative.
Coefficients Standard errors t Stat
Intercept 80.39 3.10 25.92
X -2.14 0.25 -8.62
6
ANOVA Table
Sources of Variation Degree of
freedom
Sum of Square Mean Square F
Regression 1 5,048.82 5,048.82 74.14
Error 46 3,132.66 68.10
Total 47 8,181.48
(a) F value is one of the key statistical tools used in statistical. It measures the effect size. Being
a dependent variable, F-value is the major component of ANOVA. It helps to understand the
means of the group considered in the given data.
(b) It is imperative to understand that Coefficient of determination is slightly different from
coefficient of correlation. It helps to understand the extent of relationship between the two set of
variables. The coefficient of determination of the given data comes out to be 0.62 or 62% which
is too high depending on the circumstances.
Sample size 48
Coefficient of
Determination
62%
(c) Besides coefficient of determination, coefficient of correlation has also been computed.
Likewise, the t statistics or t value has been computed and arrived. Since coefficient of
correlation of X is negative, t statistics of X is also negative.
Coefficients Standard errors t Stat
Intercept 80.39 3.10 25.92
X -2.14 0.25 -8.62
6
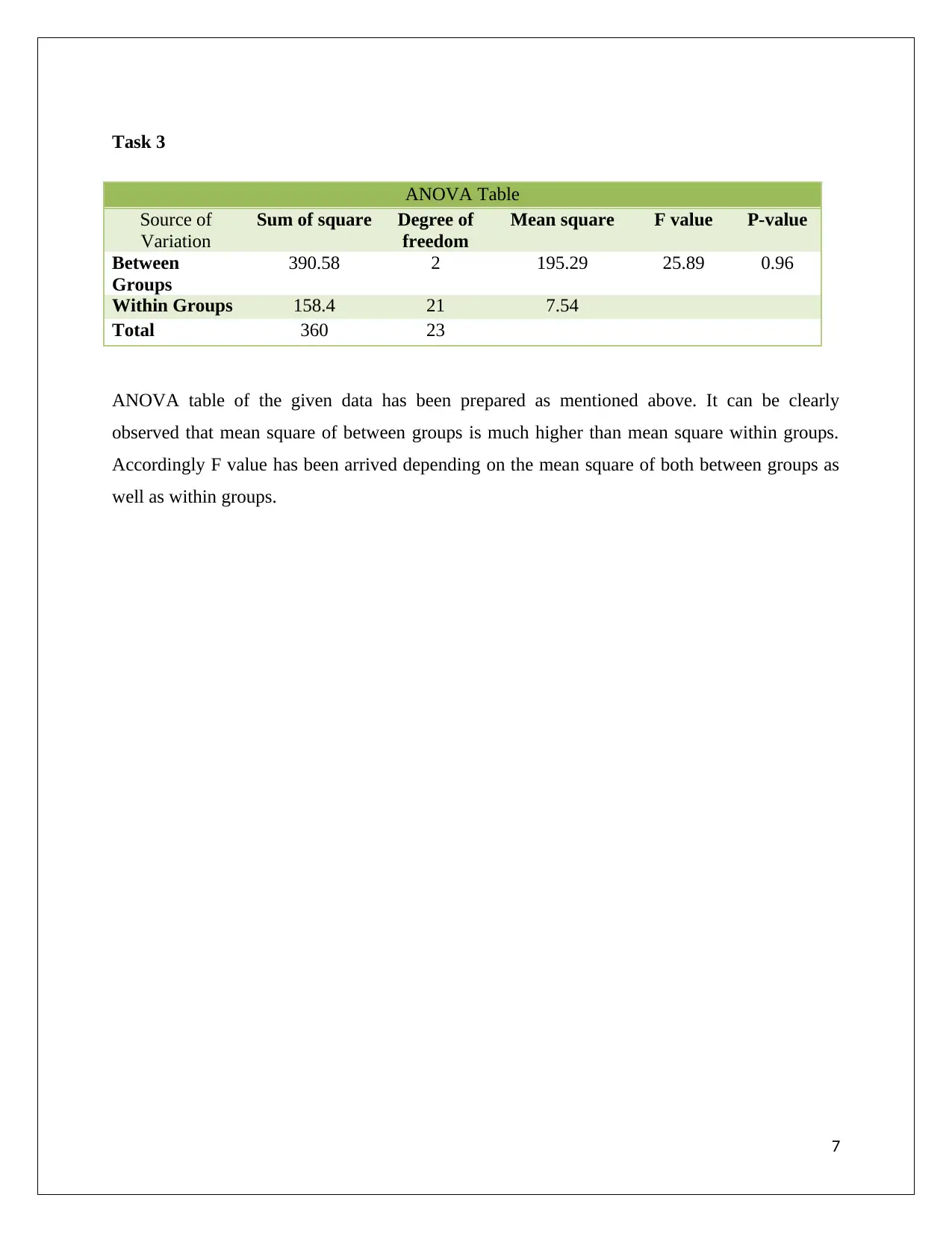
Task 3
ANOVA Table
Source of
Variation
Sum of square Degree of
freedom
Mean square F value P-value
Between
Groups
390.58 2 195.29 25.89 0.96
Within Groups 158.4 21 7.54
Total 360 23
ANOVA table of the given data has been prepared as mentioned above. It can be clearly
observed that mean square of between groups is much higher than mean square within groups.
Accordingly F value has been arrived depending on the mean square of both between groups as
well as within groups.
7
ANOVA Table
Source of
Variation
Sum of square Degree of
freedom
Mean square F value P-value
Between
Groups
390.58 2 195.29 25.89 0.96
Within Groups 158.4 21 7.54
Total 360 23
ANOVA table of the given data has been prepared as mentioned above. It can be clearly
observed that mean square of between groups is much higher than mean square within groups.
Accordingly F value has been arrived depending on the mean square of both between groups as
well as within groups.
7
Secure Best Marks with AI Grader
Need help grading? Try our AI Grader for instant feedback on your assignments.
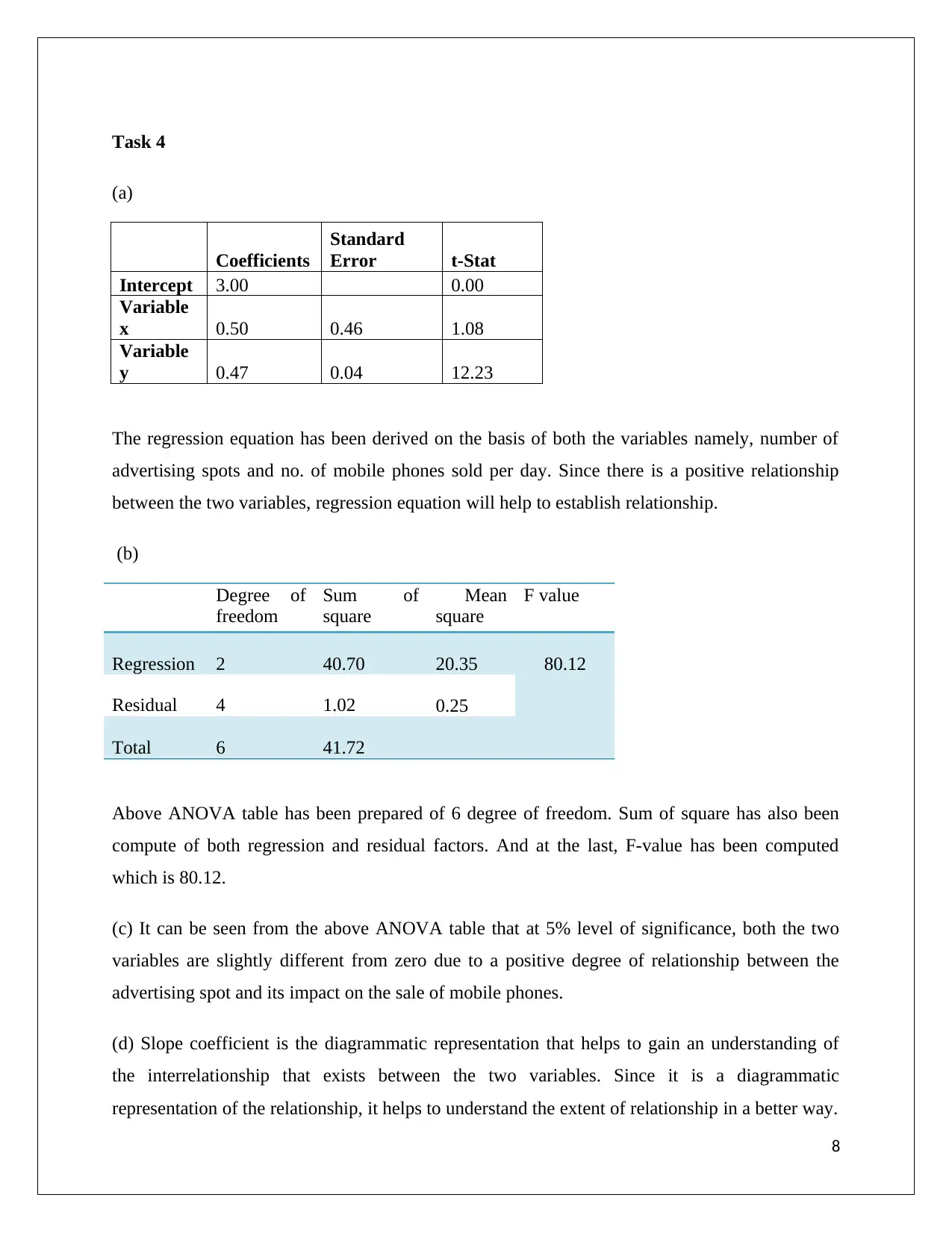
Task 4
(a)
Coefficients
Standard
Error t-Stat
Intercept 3.00 0.00
Variable
x 0.50 0.46 1.08
Variable
y 0.47 0.04 12.23
The regression equation has been derived on the basis of both the variables namely, number of
advertising spots and no. of mobile phones sold per day. Since there is a positive relationship
between the two variables, regression equation will help to establish relationship.
(b)
Degree of
freedom
Sum of
square
Mean
square
F value
Regression 2 40.70 20.35 80.12
Residual 4 1.02 0.25
Total 6 41.72
Above ANOVA table has been prepared of 6 degree of freedom. Sum of square has also been
compute of both regression and residual factors. And at the last, F-value has been computed
which is 80.12.
(c) It can be seen from the above ANOVA table that at 5% level of significance, both the two
variables are slightly different from zero due to a positive degree of relationship between the
advertising spot and its impact on the sale of mobile phones.
(d) Slope coefficient is the diagrammatic representation that helps to gain an understanding of
the interrelationship that exists between the two variables. Since it is a diagrammatic
representation of the relationship, it helps to understand the extent of relationship in a better way.
8
(a)
Coefficients
Standard
Error t-Stat
Intercept 3.00 0.00
Variable
x 0.50 0.46 1.08
Variable
y 0.47 0.04 12.23
The regression equation has been derived on the basis of both the variables namely, number of
advertising spots and no. of mobile phones sold per day. Since there is a positive relationship
between the two variables, regression equation will help to establish relationship.
(b)
Degree of
freedom
Sum of
square
Mean
square
F value
Regression 2 40.70 20.35 80.12
Residual 4 1.02 0.25
Total 6 41.72
Above ANOVA table has been prepared of 6 degree of freedom. Sum of square has also been
compute of both regression and residual factors. And at the last, F-value has been computed
which is 80.12.
(c) It can be seen from the above ANOVA table that at 5% level of significance, both the two
variables are slightly different from zero due to a positive degree of relationship between the
advertising spot and its impact on the sale of mobile phones.
(d) Slope coefficient is the diagrammatic representation that helps to gain an understanding of
the interrelationship that exists between the two variables. Since it is a diagrammatic
representation of the relationship, it helps to understand the extent of relationship in a better way.
8
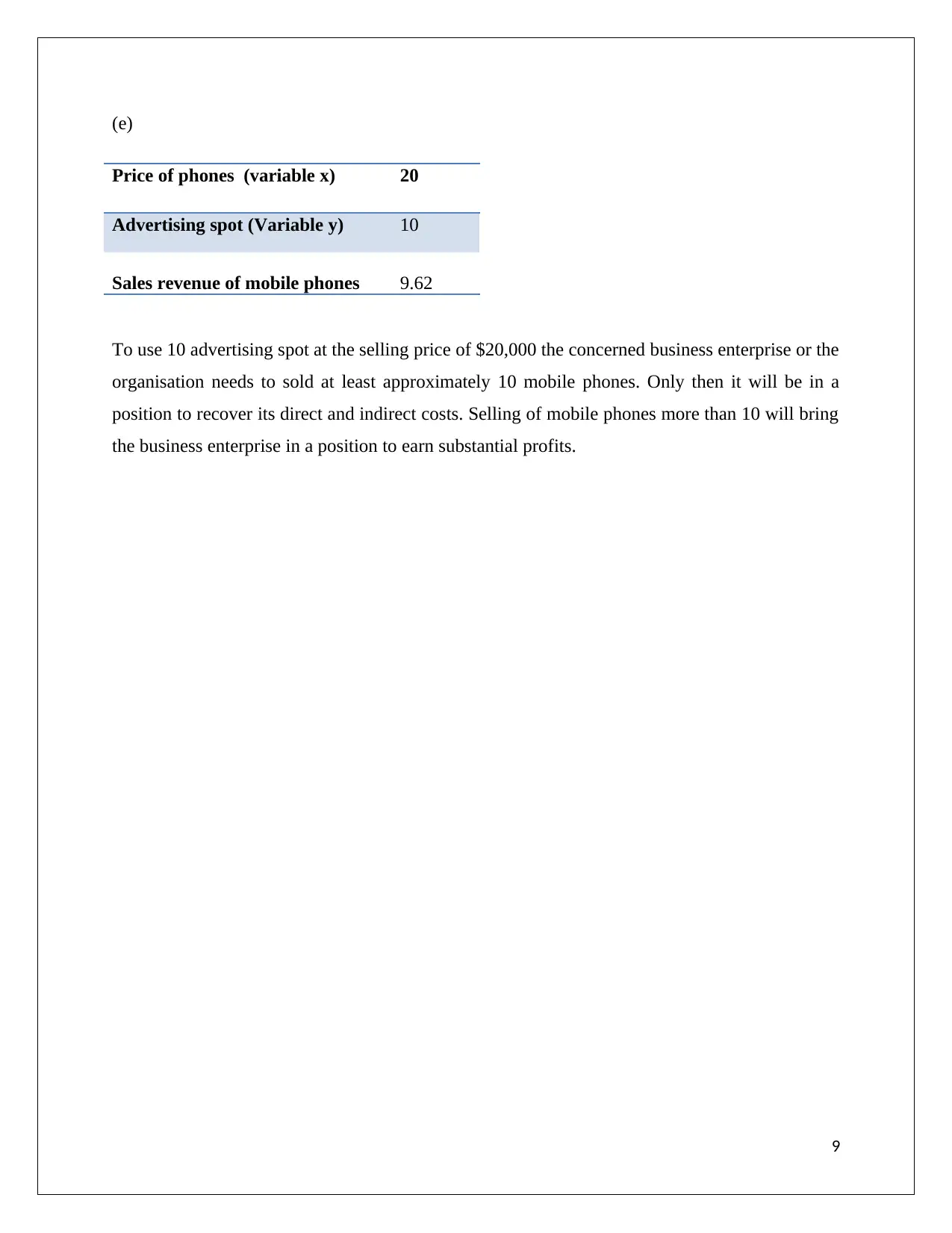
(e)
Price of phones (variable x) 20
Advertising spot (Variable y) 10
Sales revenue of mobile phones 9.62
To use 10 advertising spot at the selling price of $20,000 the concerned business enterprise or the
organisation needs to sold at least approximately 10 mobile phones. Only then it will be in a
position to recover its direct and indirect costs. Selling of mobile phones more than 10 will bring
the business enterprise in a position to earn substantial profits.
9
Price of phones (variable x) 20
Advertising spot (Variable y) 10
Sales revenue of mobile phones 9.62
To use 10 advertising spot at the selling price of $20,000 the concerned business enterprise or the
organisation needs to sold at least approximately 10 mobile phones. Only then it will be in a
position to recover its direct and indirect costs. Selling of mobile phones more than 10 will bring
the business enterprise in a position to earn substantial profits.
9
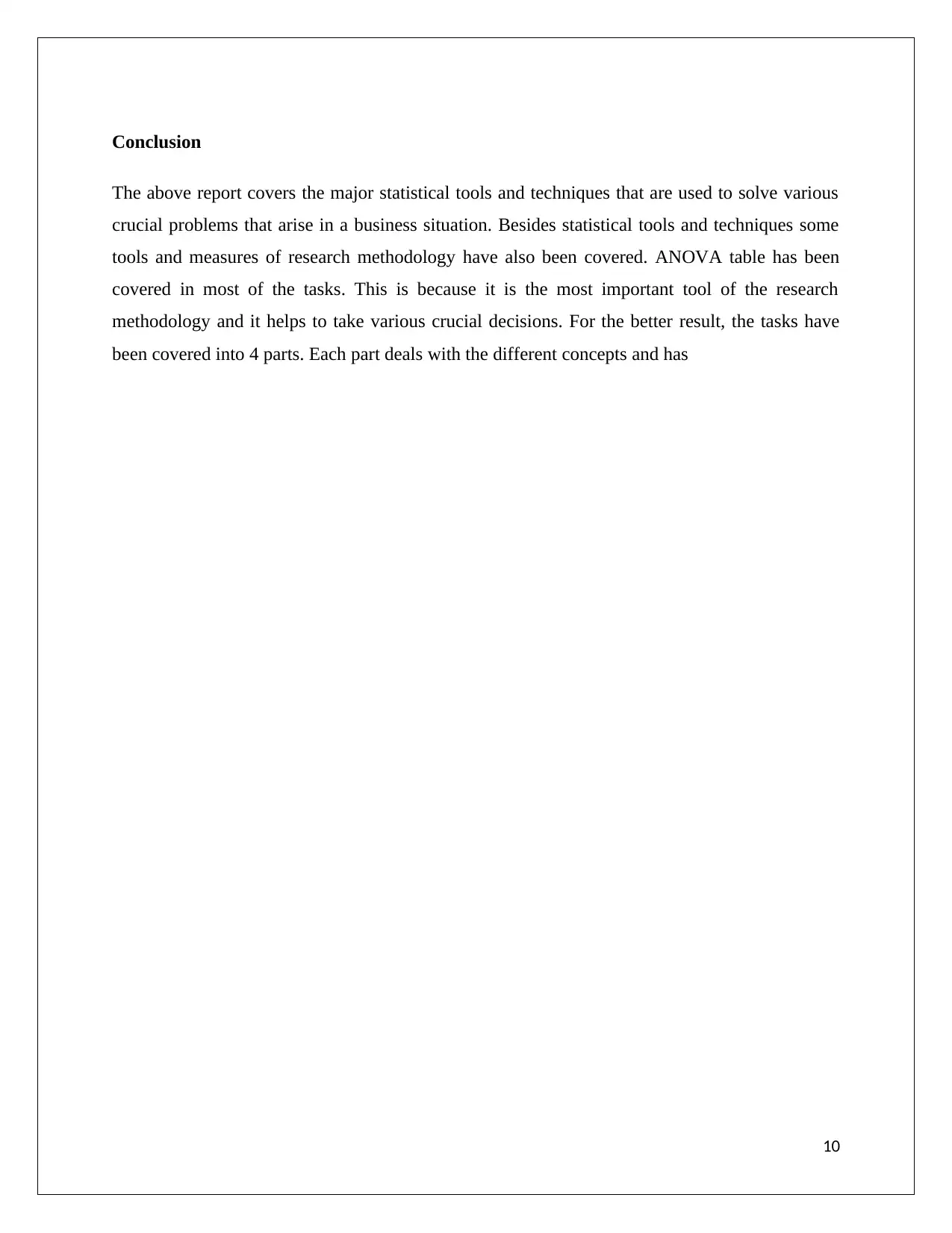
Conclusion
The above report covers the major statistical tools and techniques that are used to solve various
crucial problems that arise in a business situation. Besides statistical tools and techniques some
tools and measures of research methodology have also been covered. ANOVA table has been
covered in most of the tasks. This is because it is the most important tool of the research
methodology and it helps to take various crucial decisions. For the better result, the tasks have
been covered into 4 parts. Each part deals with the different concepts and has
10
The above report covers the major statistical tools and techniques that are used to solve various
crucial problems that arise in a business situation. Besides statistical tools and techniques some
tools and measures of research methodology have also been covered. ANOVA table has been
covered in most of the tasks. This is because it is the most important tool of the research
methodology and it helps to take various crucial decisions. For the better result, the tasks have
been covered into 4 parts. Each part deals with the different concepts and has
10
Paraphrase This Document
Need a fresh take? Get an instant paraphrase of this document with our AI Paraphraser
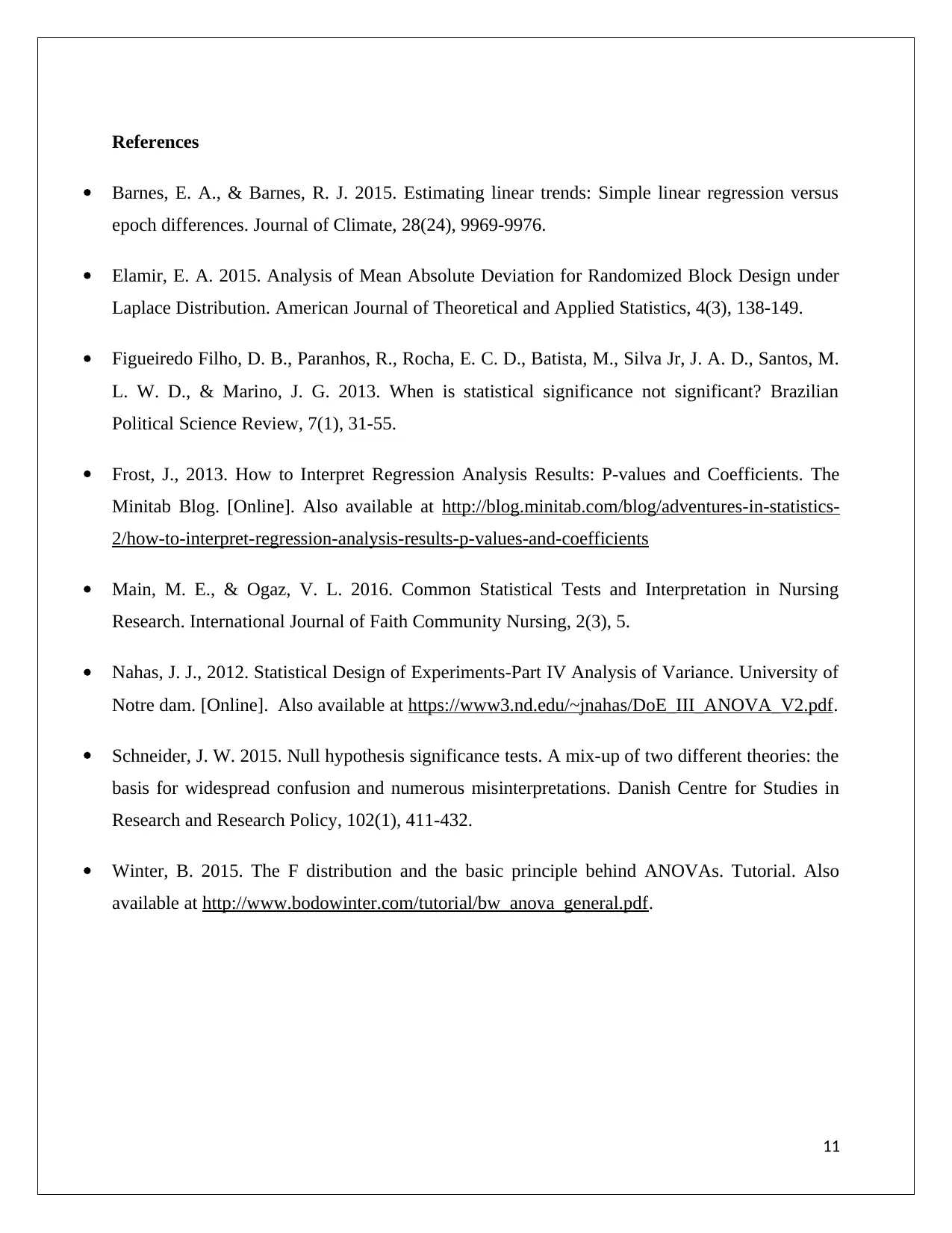
References
Barnes, E. A., & Barnes, R. J. 2015. Estimating linear trends: Simple linear regression versus
epoch differences. Journal of Climate, 28(24), 9969-9976.
Elamir, E. A. 2015. Analysis of Mean Absolute Deviation for Randomized Block Design under
Laplace Distribution. American Journal of Theoretical and Applied Statistics, 4(3), 138-149.
Figueiredo Filho, D. B., Paranhos, R., Rocha, E. C. D., Batista, M., Silva Jr, J. A. D., Santos, M.
L. W. D., & Marino, J. G. 2013. When is statistical significance not significant? Brazilian
Political Science Review, 7(1), 31-55.
Frost, J., 2013. How to Interpret Regression Analysis Results: P-values and Coefficients. The
Minitab Blog. [Online]. Also available at http://blog.minitab.com/blog/adventures-in-statistics-
2/how-to-interpret-regression-analysis-results-p-values-and-coefficients
Main, M. E., & Ogaz, V. L. 2016. Common Statistical Tests and Interpretation in Nursing
Research. International Journal of Faith Community Nursing, 2(3), 5.
Nahas, J. J., 2012. Statistical Design of Experiments‐Part IV Analysis of Variance. University of
Notre dam. [Online]. Also available at https://www3.nd.edu/~jnahas/DoE_III_ANOVA_V2.pdf.
Schneider, J. W. 2015. Null hypothesis significance tests. A mix-up of two different theories: the
basis for widespread confusion and numerous misinterpretations. Danish Centre for Studies in
Research and Research Policy, 102(1), 411-432.
Winter, B. 2015. The F distribution and the basic principle behind ANOVAs. Tutorial. Also
available at http://www.bodowinter.com/tutorial/bw_anova_general.pdf.
11
Barnes, E. A., & Barnes, R. J. 2015. Estimating linear trends: Simple linear regression versus
epoch differences. Journal of Climate, 28(24), 9969-9976.
Elamir, E. A. 2015. Analysis of Mean Absolute Deviation for Randomized Block Design under
Laplace Distribution. American Journal of Theoretical and Applied Statistics, 4(3), 138-149.
Figueiredo Filho, D. B., Paranhos, R., Rocha, E. C. D., Batista, M., Silva Jr, J. A. D., Santos, M.
L. W. D., & Marino, J. G. 2013. When is statistical significance not significant? Brazilian
Political Science Review, 7(1), 31-55.
Frost, J., 2013. How to Interpret Regression Analysis Results: P-values and Coefficients. The
Minitab Blog. [Online]. Also available at http://blog.minitab.com/blog/adventures-in-statistics-
2/how-to-interpret-regression-analysis-results-p-values-and-coefficients
Main, M. E., & Ogaz, V. L. 2016. Common Statistical Tests and Interpretation in Nursing
Research. International Journal of Faith Community Nursing, 2(3), 5.
Nahas, J. J., 2012. Statistical Design of Experiments‐Part IV Analysis of Variance. University of
Notre dam. [Online]. Also available at https://www3.nd.edu/~jnahas/DoE_III_ANOVA_V2.pdf.
Schneider, J. W. 2015. Null hypothesis significance tests. A mix-up of two different theories: the
basis for widespread confusion and numerous misinterpretations. Danish Centre for Studies in
Research and Research Policy, 102(1), 411-432.
Winter, B. 2015. The F distribution and the basic principle behind ANOVAs. Tutorial. Also
available at http://www.bodowinter.com/tutorial/bw_anova_general.pdf.
11
1 out of 11
Related Documents
![[object Object]](/_next/image/?url=%2F_next%2Fstatic%2Fmedia%2Flogo.6d15ce61.png&w=640&q=75)
Your All-in-One AI-Powered Toolkit for Academic Success.
+13062052269
info@desklib.com
Available 24*7 on WhatsApp / Email
Unlock your academic potential
© 2024 | Zucol Services PVT LTD | All rights reserved.