Statistical Hydrology Project: Advanced Statistical Hydrology Analysis
VerifiedAdded on 2022/10/08
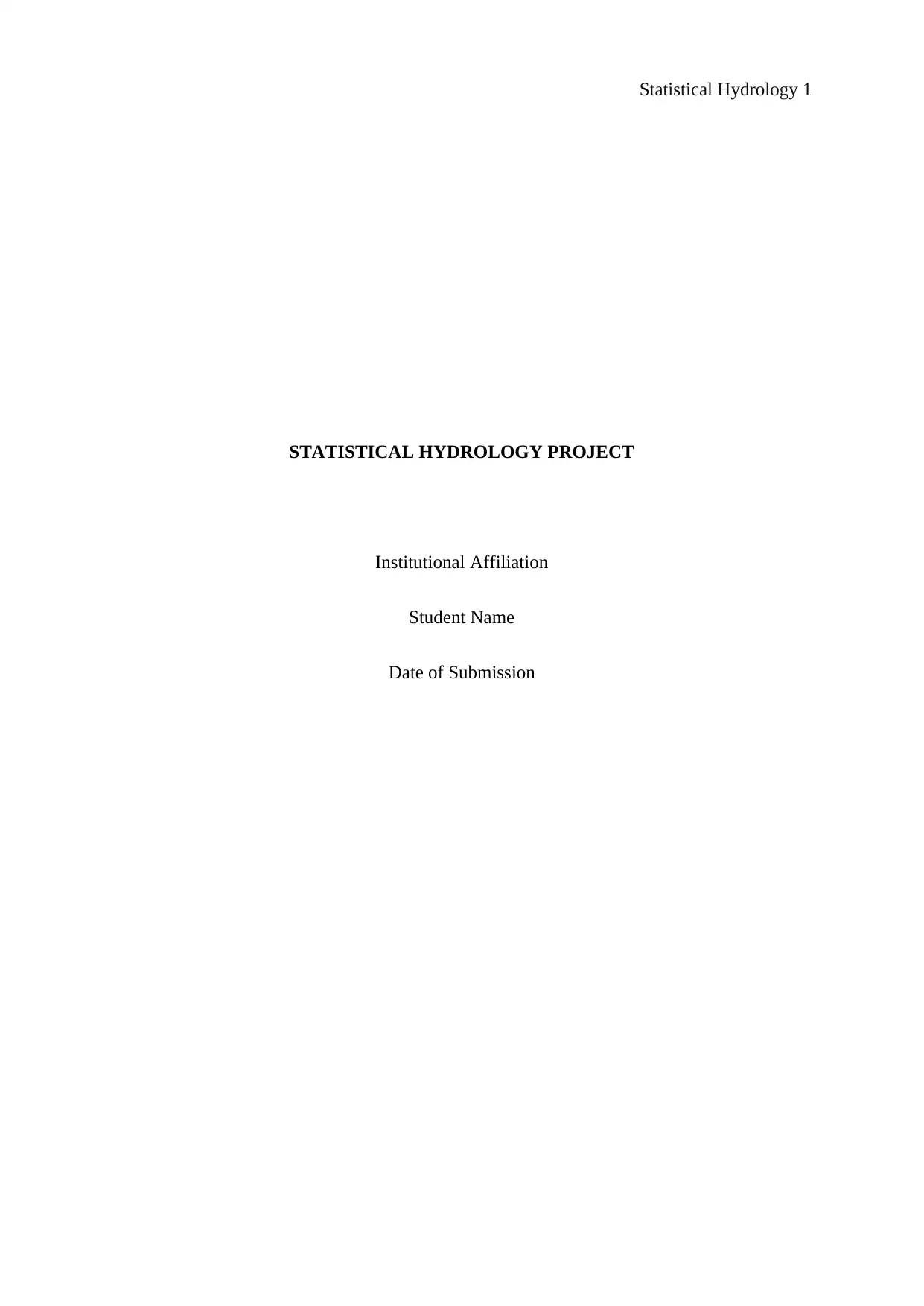
STATISTICAL HYDROLOGY PROJECT
Institutional Affiliation
Student Name
Date of Submission
Paraphrase This Document
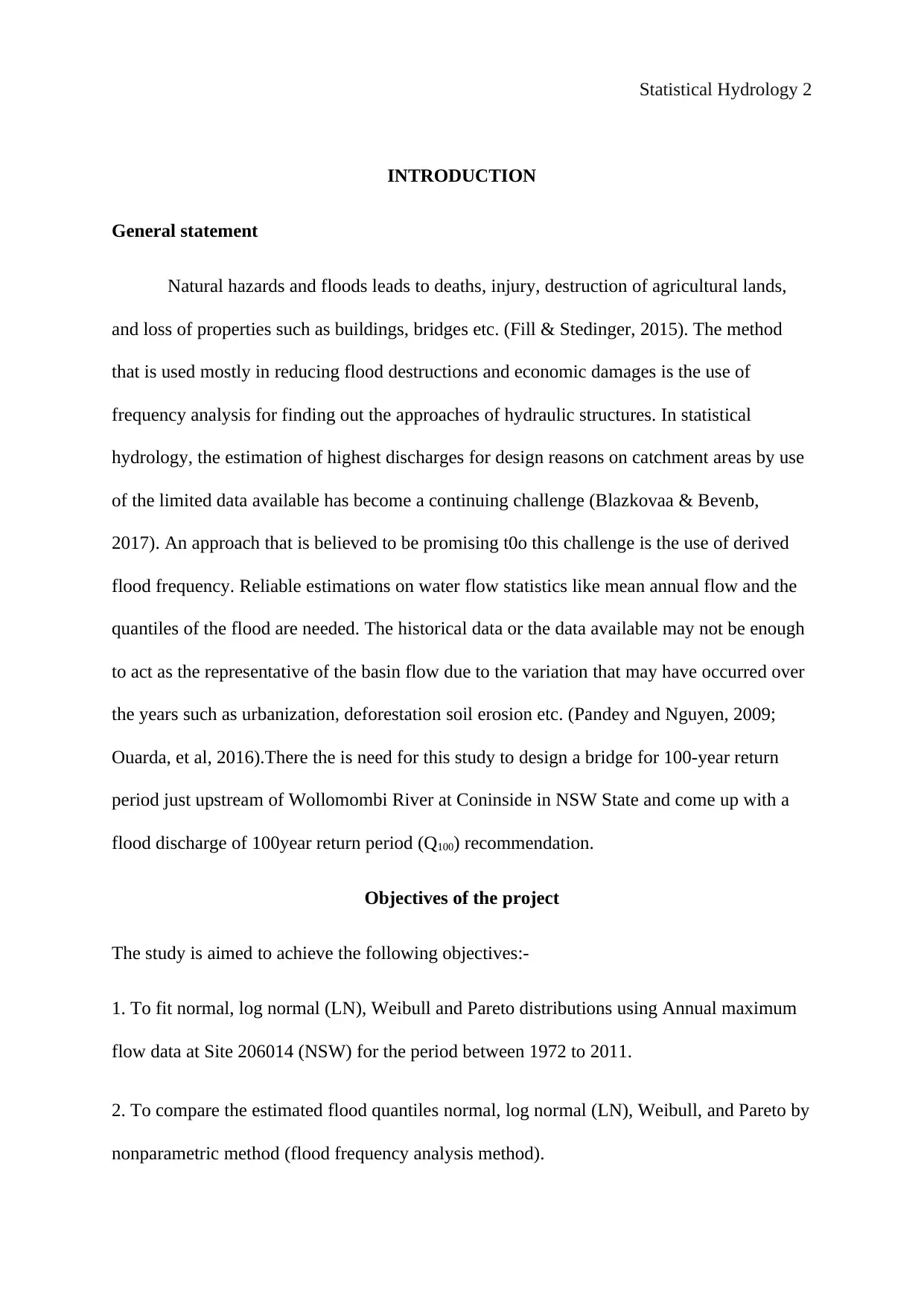
INTRODUCTION
General statement
Natural hazards and floods leads to deaths, injury, destruction of agricultural lands,
and loss of properties such as buildings, bridges etc. (Fill & Stedinger, 2015). The method
that is used mostly in reducing flood destructions and economic damages is the use of
frequency analysis for finding out the approaches of hydraulic structures. In statistical
hydrology, the estimation of highest discharges for design reasons on catchment areas by use
of the limited data available has become a continuing challenge (Blazkovaa & Bevenb,
2017). An approach that is believed to be promising t0o this challenge is the use of derived
flood frequency. Reliable estimations on water flow statistics like mean annual flow and the
quantiles of the flood are needed. The historical data or the data available may not be enough
to act as the representative of the basin flow due to the variation that may have occurred over
the years such as urbanization, deforestation soil erosion etc. (Pandey and Nguyen, 2009;
Ouarda, et al, 2016).There the is need for this study to design a bridge for 100-year return
period just upstream of Wollomombi River at Coninside in NSW State and come up with a
flood discharge of 100year return period (Q100) recommendation.
Objectives of the project
The study is aimed to achieve the following objectives:-
1. To fit normal, log normal (LN), Weibull and Pareto distributions using Annual maximum
flow data at Site 206014 (NSW) for the period between 1972 to 2011.
2. To compare the estimated flood quantiles normal, log normal (LN), Weibull, and Pareto by
nonparametric method (flood frequency analysis method).
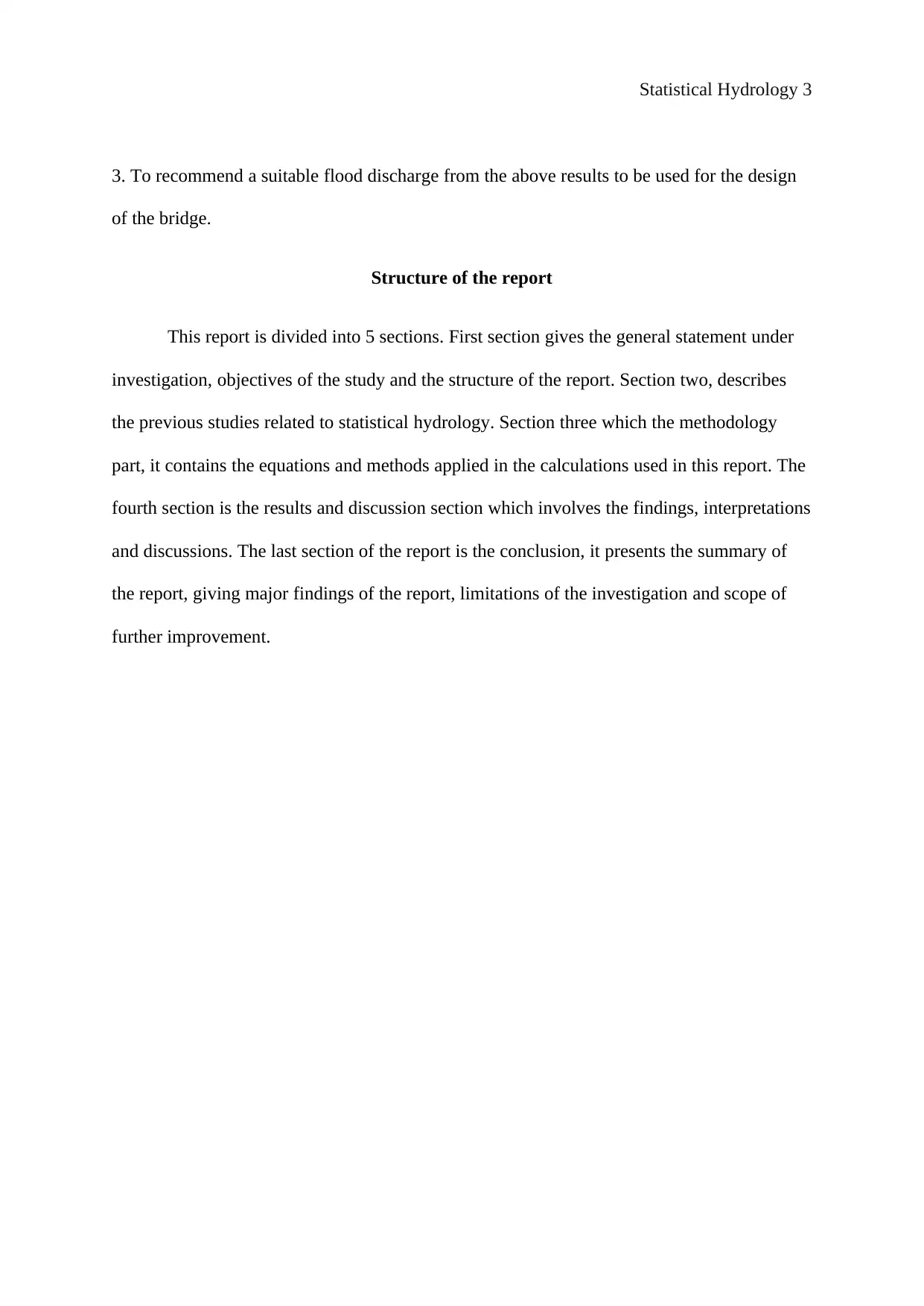
3. To recommend a suitable flood discharge from the above results to be used for the design
of the bridge.
Structure of the report
This report is divided into 5 sections. First section gives the general statement under
investigation, objectives of the study and the structure of the report. Section two, describes
the previous studies related to statistical hydrology. Section three which the methodology
part, it contains the equations and methods applied in the calculations used in this report. The
fourth section is the results and discussion section which involves the findings, interpretations
and discussions. The last section of the report is the conclusion, it presents the summary of
the report, giving major findings of the report, limitations of the investigation and scope of
further improvement.
⊘ This is a preview!⊘
Do you want full access?
Subscribe today to unlock all pages.

Trusted by 1+ million students worldwide
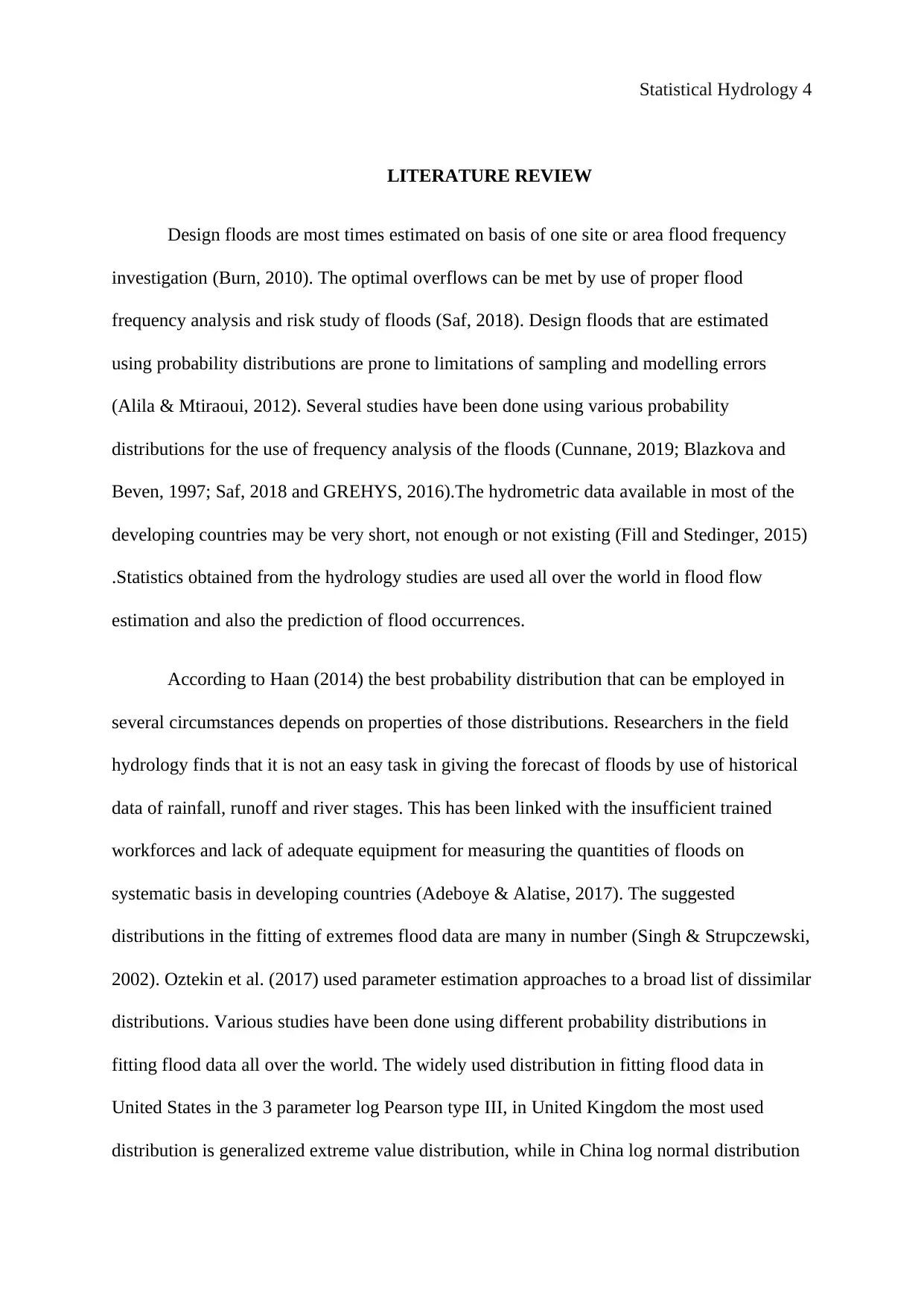
LITERATURE REVIEW
Design floods are most times estimated on basis of one site or area flood frequency
investigation (Burn, 2010). The optimal overflows can be met by use of proper flood
frequency analysis and risk study of floods (Saf, 2018). Design floods that are estimated
using probability distributions are prone to limitations of sampling and modelling errors
(Alila & Mtiraoui, 2012). Several studies have been done using various probability
distributions for the use of frequency analysis of the floods (Cunnane, 2019; Blazkova and
Beven, 1997; Saf, 2018 and GREHYS, 2016).The hydrometric data available in most of the
developing countries may be very short, not enough or not existing (Fill and Stedinger, 2015)
.Statistics obtained from the hydrology studies are used all over the world in flood flow
estimation and also the prediction of flood occurrences.
According to Haan (2014) the best probability distribution that can be employed in
several circumstances depends on properties of those distributions. Researchers in the field
hydrology finds that it is not an easy task in giving the forecast of floods by use of historical
data of rainfall, runoff and river stages. This has been linked with the insufficient trained
workforces and lack of adequate equipment for measuring the quantities of floods on
systematic basis in developing countries (Adeboye & Alatise, 2017). The suggested
distributions in the fitting of extremes flood data are many in number (Singh & Strupczewski,
2002). Oztekin et al. (2017) used parameter estimation approaches to a broad list of dissimilar
distributions. Various studies have been done using different probability distributions in
fitting flood data all over the world. The widely used distribution in fitting flood data in
United States in the 3 parameter log Pearson type III, in United Kingdom the most used
distribution is generalized extreme value distribution, while in China log normal distribution
Paraphrase This Document
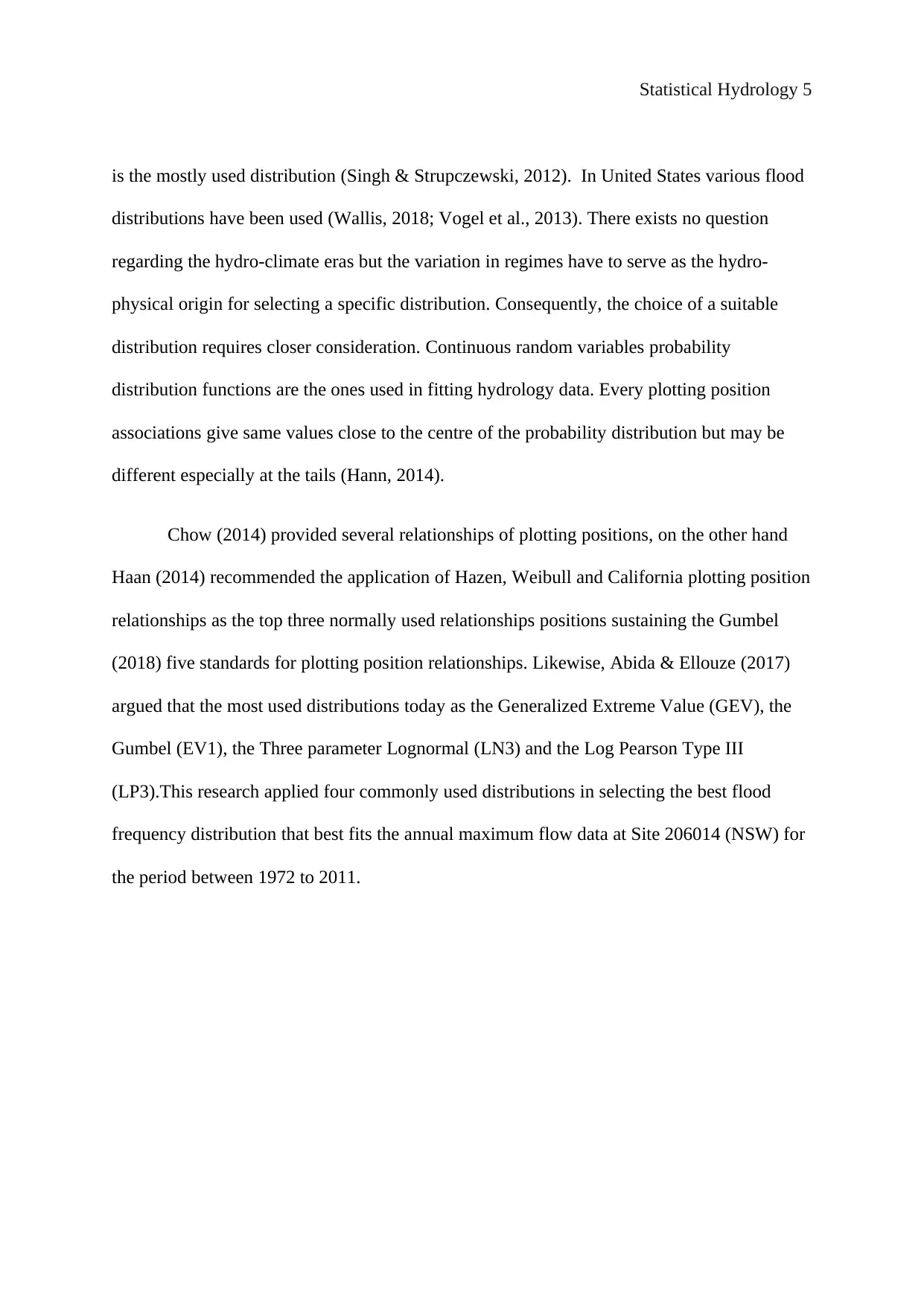
is the mostly used distribution (Singh & Strupczewski, 2012). In United States various flood
distributions have been used (Wallis, 2018; Vogel et al., 2013). There exists no question
regarding the hydro-climate eras but the variation in regimes have to serve as the hydro-
physical origin for selecting a specific distribution. Consequently, the choice of a suitable
distribution requires closer consideration. Continuous random variables probability
distribution functions are the ones used in fitting hydrology data. Every plotting position
associations give same values close to the centre of the probability distribution but may be
different especially at the tails (Hann, 2014).
Chow (2014) provided several relationships of plotting positions, on the other hand
Haan (2014) recommended the application of Hazen, Weibull and California plotting position
relationships as the top three normally used relationships positions sustaining the Gumbel
(2018) five standards for plotting position relationships. Likewise, Abida & Ellouze (2017)
argued that the most used distributions today as the Generalized Extreme Value (GEV), the
Gumbel (EV1), the Three parameter Lognormal (LN3) and the Log Pearson Type III
(LP3).This research applied four commonly used distributions in selecting the best flood
frequency distribution that best fits the annual maximum flow data at Site 206014 (NSW) for
the period between 1972 to 2011.
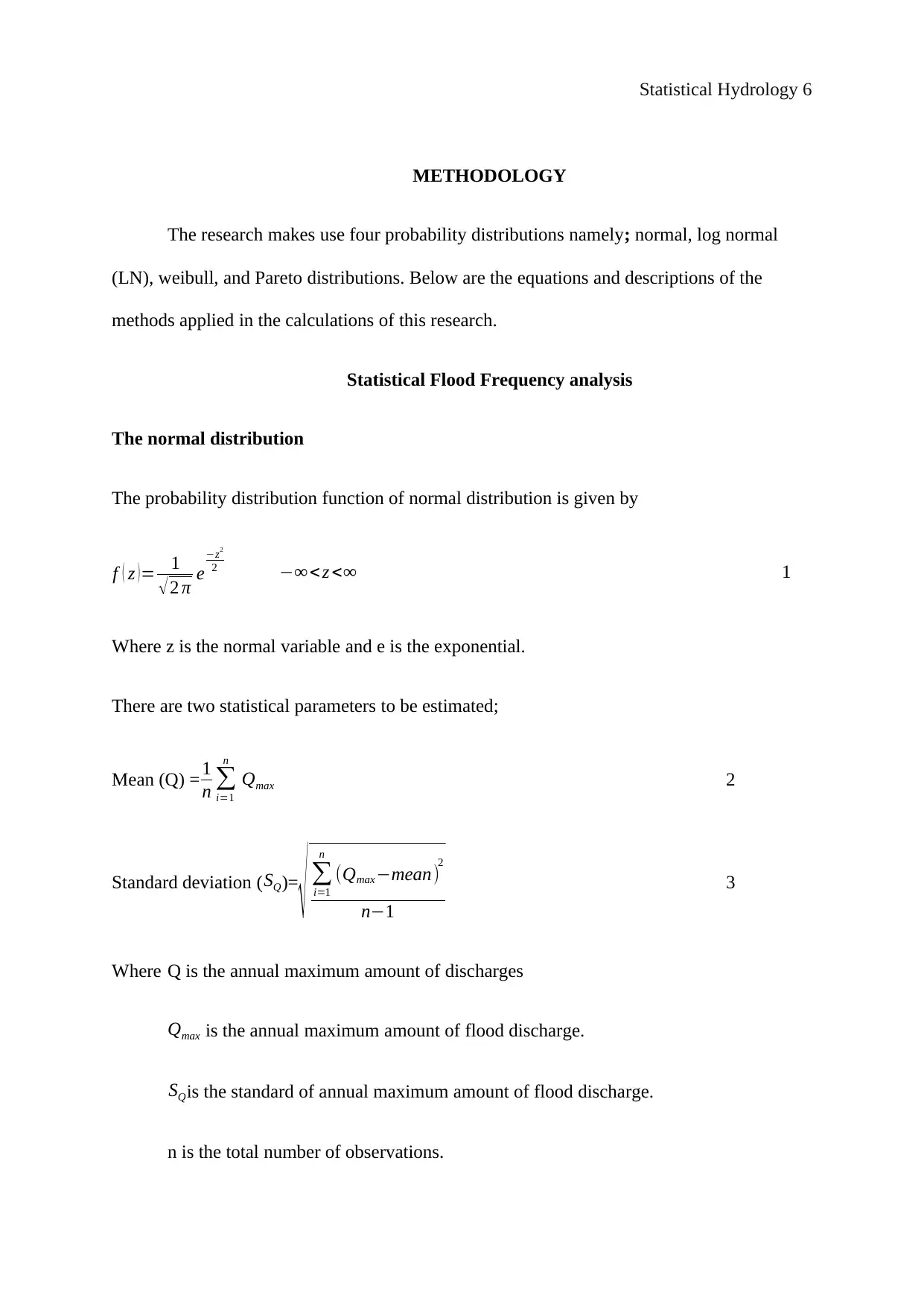
METHODOLOGY
The research makes use four probability distributions namely; normal, log normal
(LN), weibull, and Pareto distributions. Below are the equations and descriptions of the
methods applied in the calculations of this research.
Statistical Flood Frequency analysis
The normal distribution
The probability distribution function of normal distribution is given by
f ( z ) = 1
√ 2 π e
−z2
2 −∞< z <∞ 1
Where z is the normal variable and e is the exponential.
There are two statistical parameters to be estimated;
Mean (Q) = 1
n ∑
i=1
n
Qmax 2
Standard deviation ( SQ)=
√ ∑
i=1
n
(Qmax −mean)2
n−1
3
Where Q is the annual maximum amount of discharges
Qmax is the annual maximum amount of flood discharge.
SQis the standard of annual maximum amount of flood discharge.
n is the total number of observations.
⊘ This is a preview!⊘
Do you want full access?
Subscribe today to unlock all pages.

Trusted by 1+ million students worldwide
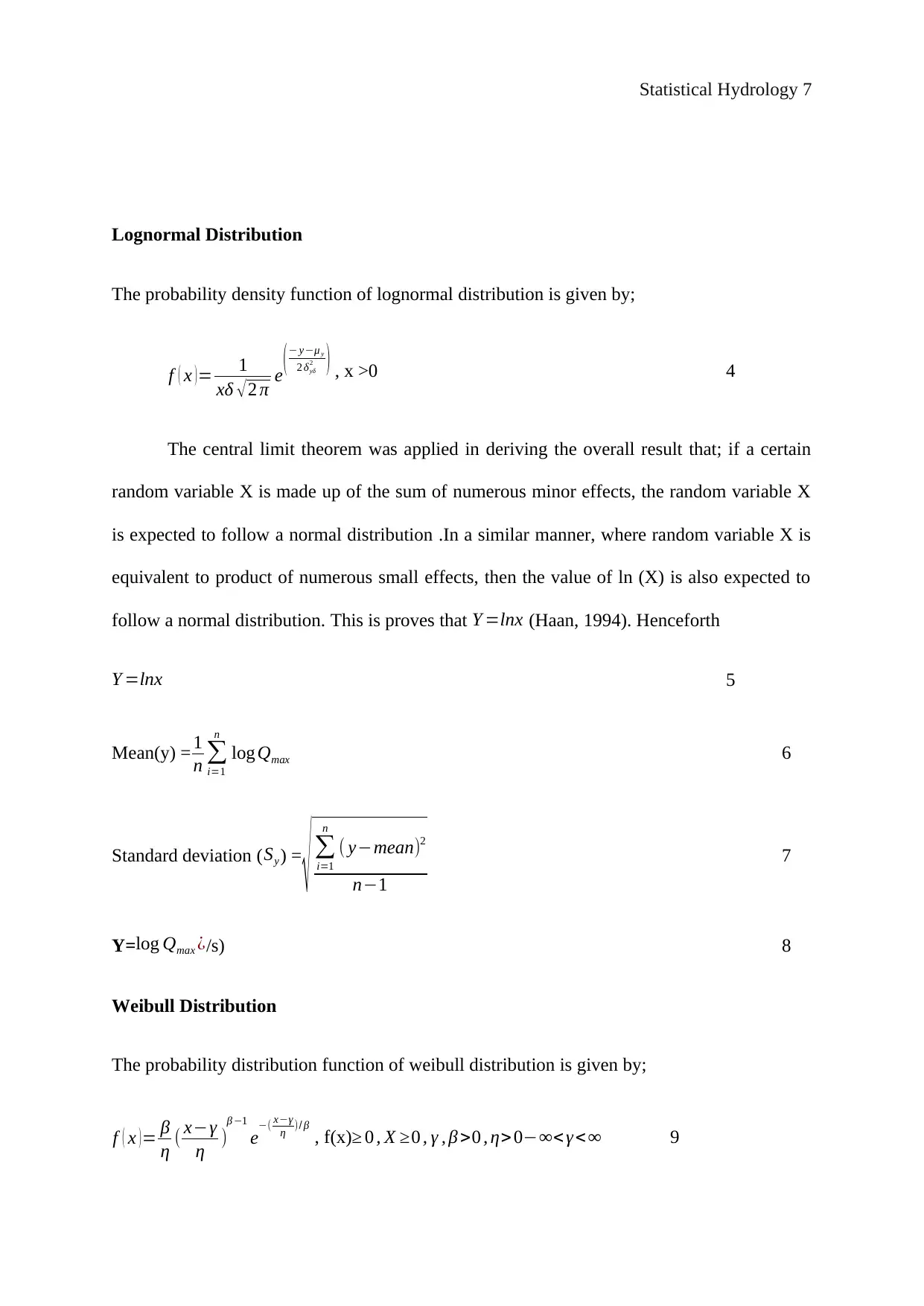
Lognormal Distribution
The probability density function of lognormal distribution is given by;
f ( x ) = 1
xδ √ 2 π e
( − y−μ y
2 δyδ
2 ) , x >0 4
The central limit theorem was applied in deriving the overall result that; if a certain
random variable X is made up of the sum of numerous minor effects, the random variable X
is expected to follow a normal distribution .In a similar manner, where random variable X is
equivalent to product of numerous small effects, then the value of ln (X) is also expected to
follow a normal distribution. This is proves that Y =lnx (Haan, 1994). Henceforth
Y =lnx 5
Mean(y) = 1
n ∑
i=1
n
log Qmax 6
Standard deviation ( Sy ) =
√ ∑
i=1
n
( y−mean)2
n−1
7
Y=log Qmax ¿/s) 8
Weibull Distribution
The probability distribution function of weibull distribution is given by;
f ( x )= β
η ( x−γ
η )
β −1
e−( x−γ
η )/ β
, f(x)≥ 0 , X ≥0 , γ , β >0 , η>0−∞< γ <∞ 9
Paraphrase This Document
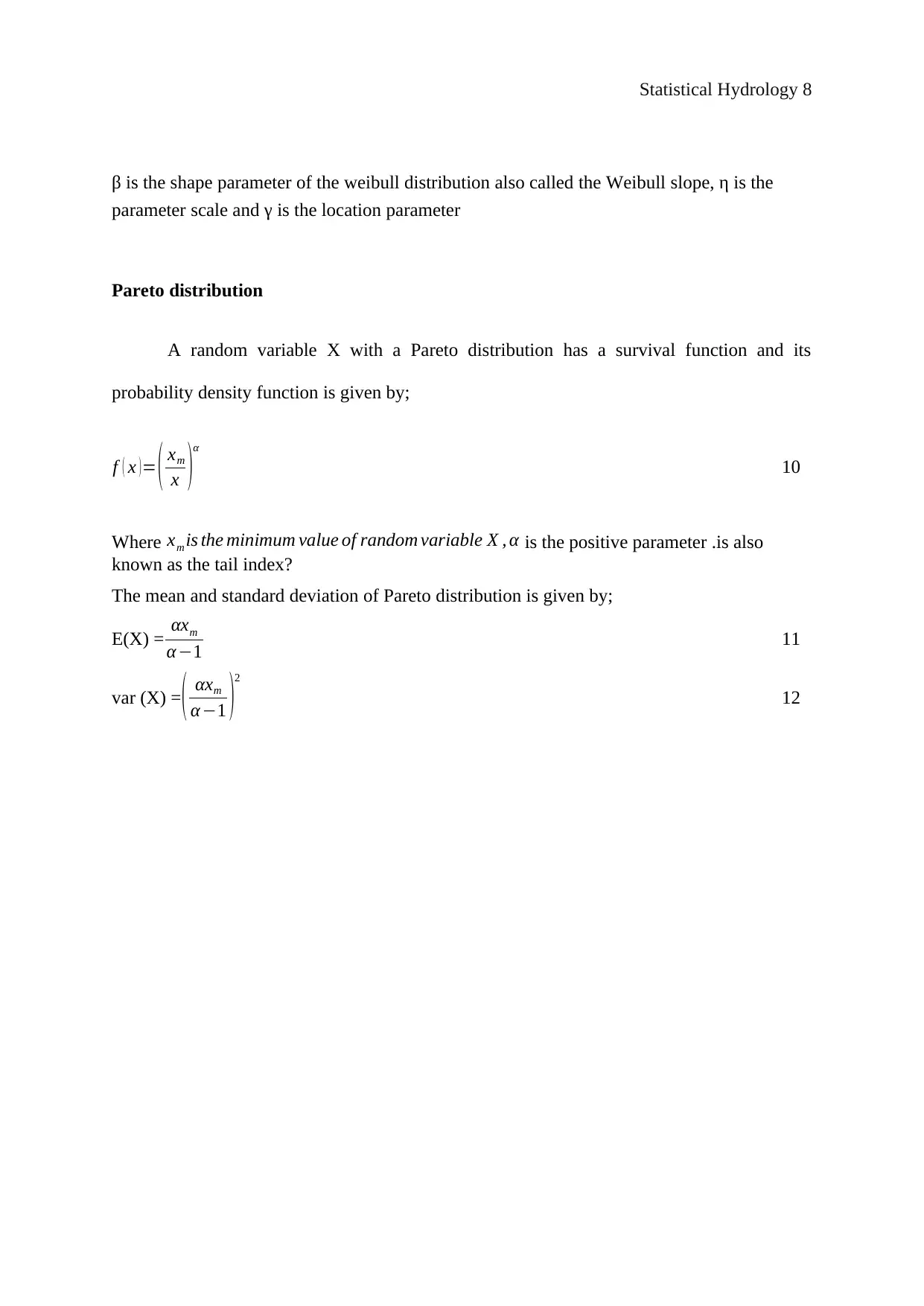
β is the shape parameter of the weibull distribution also called the Weibull slope, η is the
parameter scale and γ is the location parameter
Pareto distribution
A random variable X with a Pareto distribution has a survival function and its
probability density function is given by;
f ( x ) = ( xm
x )
α
10
Where xm is the minimum value of random variable X , α is the positive parameter .is also
known as the tail index?
The mean and standard deviation of Pareto distribution is given by;
E(X) = αxm
α −1 11
var (X) =( αxm
α −1 )
2
12
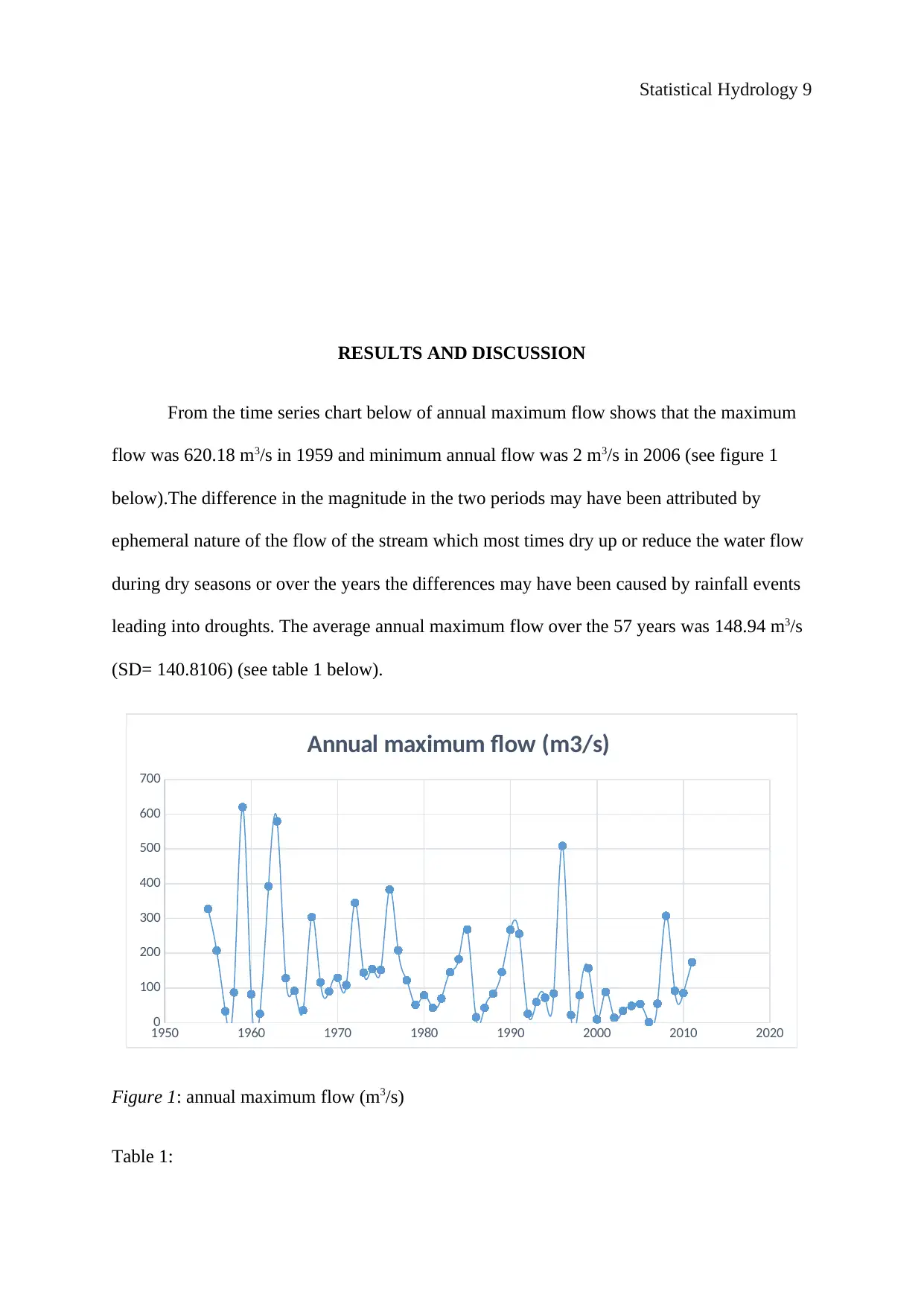
RESULTS AND DISCUSSION
From the time series chart below of annual maximum flow shows that the maximum
flow was 620.18 m3/s in 1959 and minimum annual flow was 2 m3/s in 2006 (see figure 1
below).The difference in the magnitude in the two periods may have been attributed by
ephemeral nature of the flow of the stream which most times dry up or reduce the water flow
during dry seasons or over the years the differences may have been caused by rainfall events
leading into droughts. The average annual maximum flow over the 57 years was 148.94 m3/s
(SD= 140.8106) (see table 1 below).
1950 1960 1970 1980 1990 2000 2010 2020
0
100
200
300
400
500
600
700
Annual maximum flow (m3/s)
Figure 1: annual maximum flow (m3/s)
Table 1:
⊘ This is a preview!⊘
Do you want full access?
Subscribe today to unlock all pages.

Trusted by 1+ million students worldwide
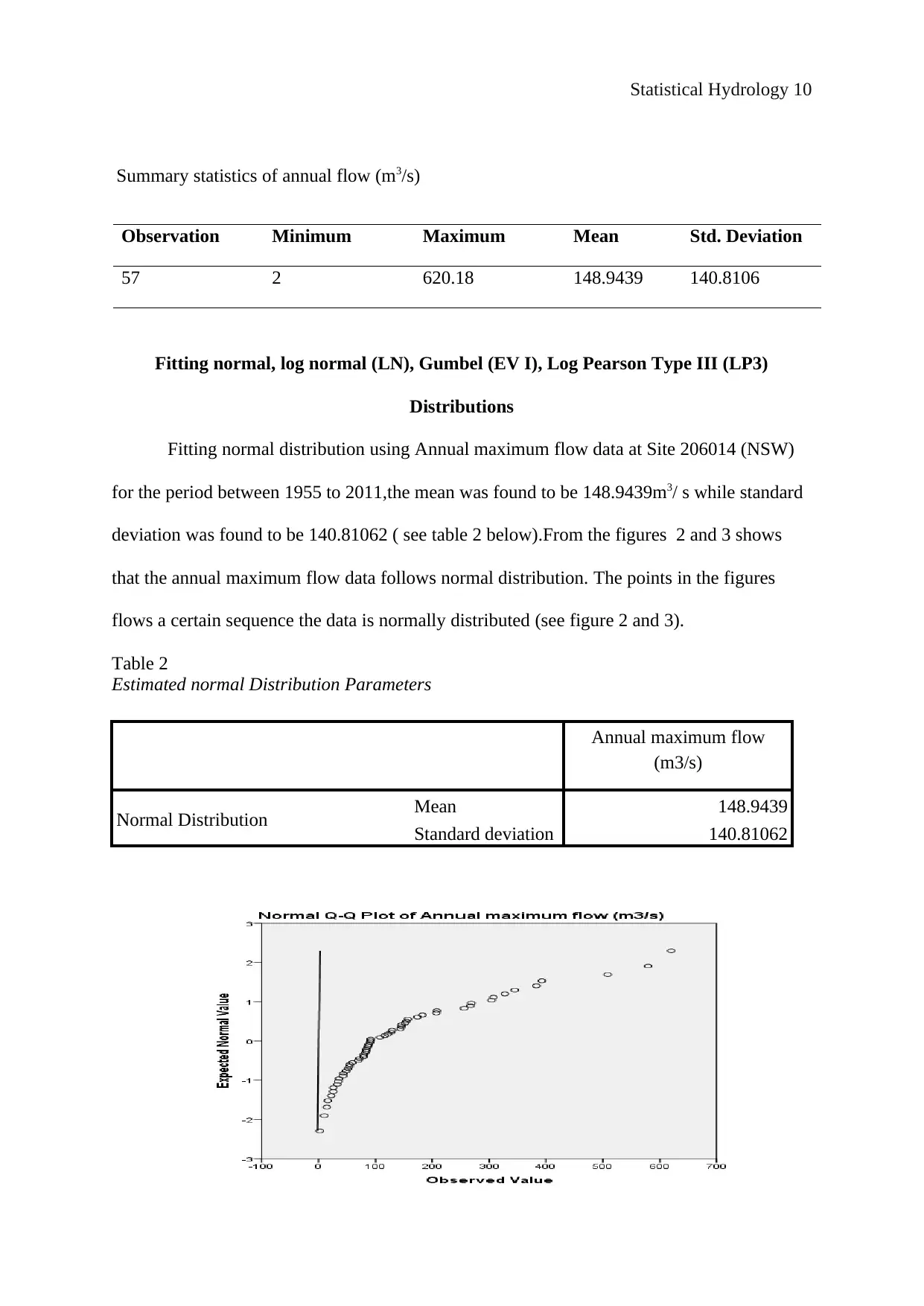
Summary statistics of annual flow (m3/s)
Observation Minimum Maximum Mean Std. Deviation
57 2 620.18 148.9439 140.8106
Fitting normal, log normal (LN), Gumbel (EV I), Log Pearson Type III (LP3)
Distributions
Fitting normal distribution using Annual maximum flow data at Site 206014 (NSW)
for the period between 1955 to 2011,the mean was found to be 148.9439m3/ s while standard
deviation was found to be 140.81062 ( see table 2 below).From the figures 2 and 3 shows
that the annual maximum flow data follows normal distribution. The points in the figures
flows a certain sequence the data is normally distributed (see figure 2 and 3).
Table 2
Estimated normal Distribution Parameters
Annual maximum flow
(m3/s)
Normal Distribution Mean 148.9439
Standard deviation 140.81062
Paraphrase This Document
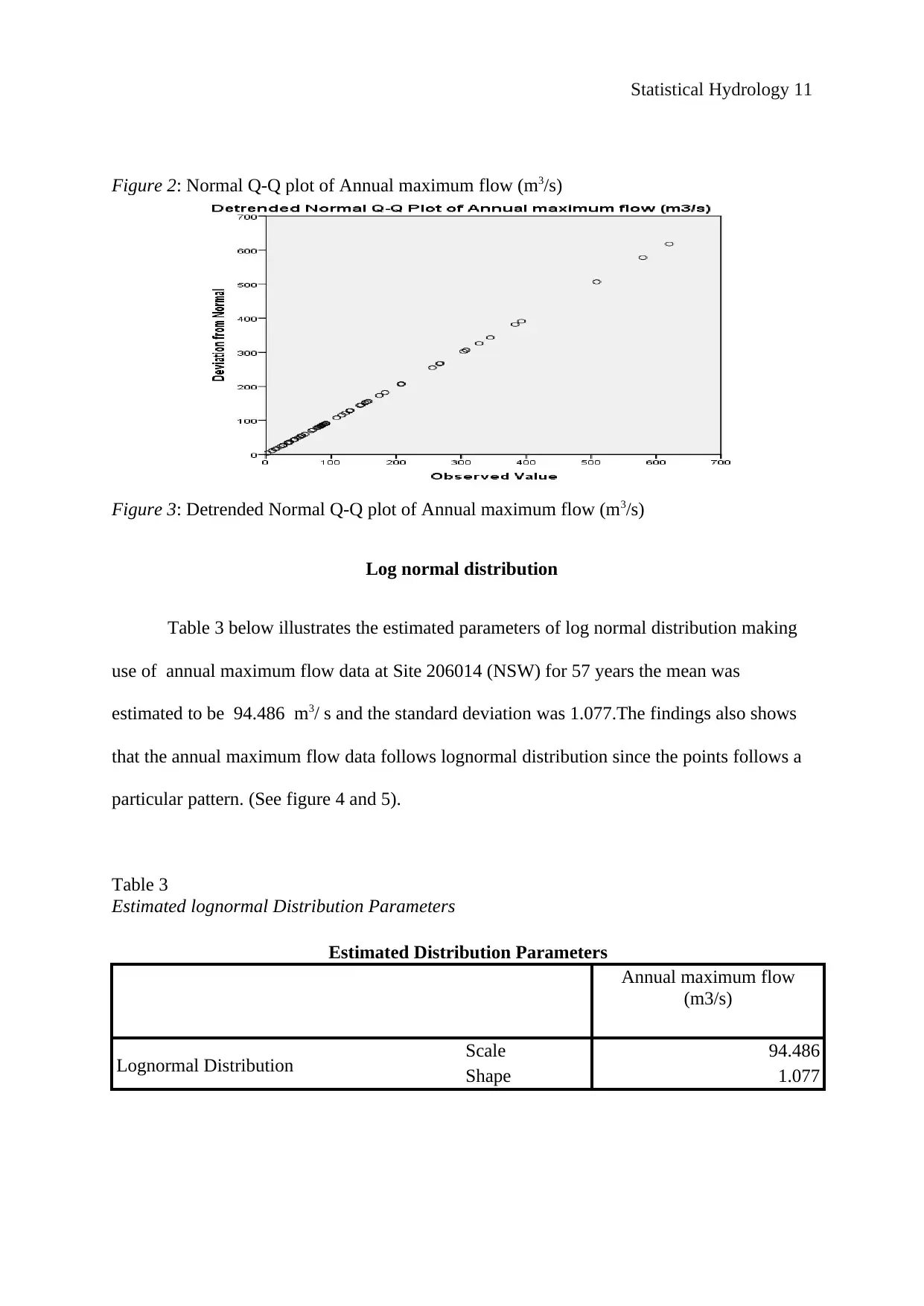
Figure 2: Normal Q-Q plot of Annual maximum flow (m3/s)
Figure 3: Detrended Normal Q-Q plot of Annual maximum flow (m3/s)
Log normal distribution
Table 3 below illustrates the estimated parameters of log normal distribution making
use of annual maximum flow data at Site 206014 (NSW) for 57 years the mean was
estimated to be 94.486 m3/ s and the standard deviation was 1.077.The findings also shows
that the annual maximum flow data follows lognormal distribution since the points follows a
particular pattern. (See figure 4 and 5).
Table 3
Estimated lognormal Distribution Parameters
Estimated Distribution Parameters
Annual maximum flow
(m3/s)
Lognormal Distribution Scale 94.486
Shape 1.077
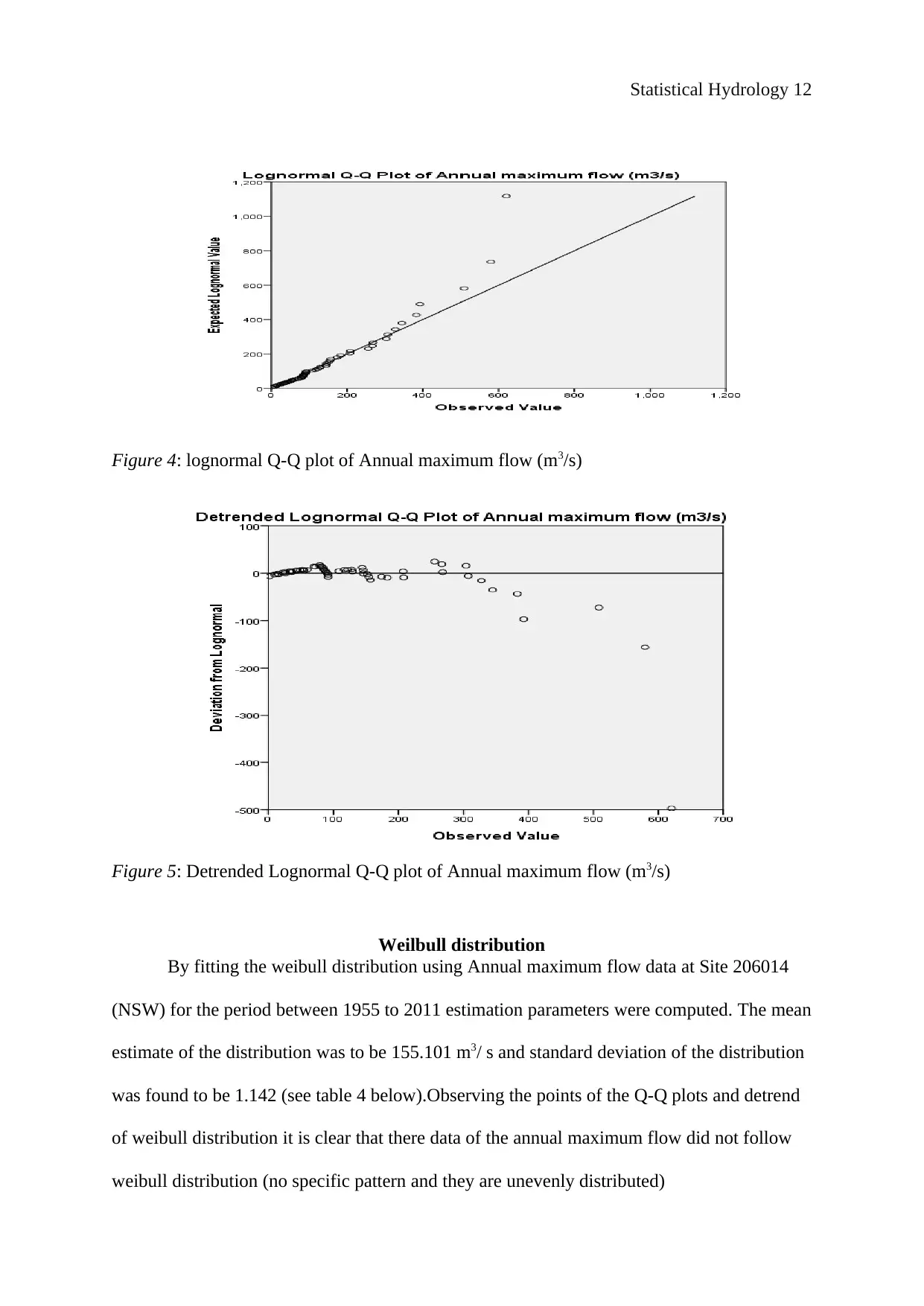
Figure 4: lognormal Q-Q plot of Annual maximum flow (m3/s)
Figure 5: Detrended Lognormal Q-Q plot of Annual maximum flow (m3/s)
Weilbull distribution
By fitting the weibull distribution using Annual maximum flow data at Site 206014
(NSW) for the period between 1955 to 2011 estimation parameters were computed. The mean
estimate of the distribution was to be 155.101 m3/ s and standard deviation of the distribution
was found to be 1.142 (see table 4 below).Observing the points of the Q-Q plots and detrend
of weibull distribution it is clear that there data of the annual maximum flow did not follow
weibull distribution (no specific pattern and they are unevenly distributed)
⊘ This is a preview!⊘
Do you want full access?
Subscribe today to unlock all pages.

Trusted by 1+ million students worldwide
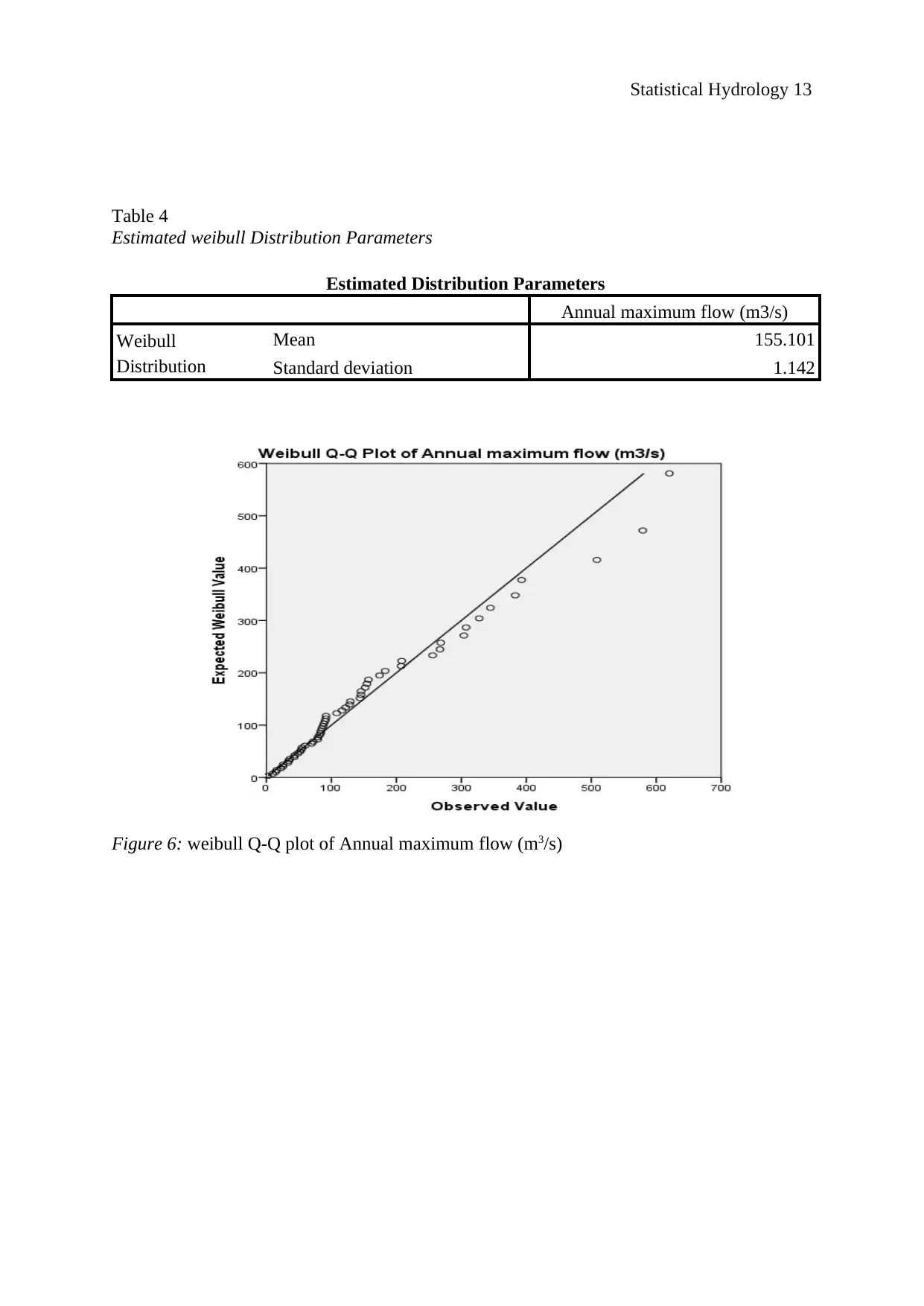
Table 4
Estimated weibull Distribution Parameters
Estimated Distribution Parameters
Annual maximum flow (m3/s)
Weibull
Distribution
Mean 155.101
Standard deviation 1.142
Figure 6: weibull Q-Q plot of Annual maximum flow (m3/s)
Paraphrase This Document
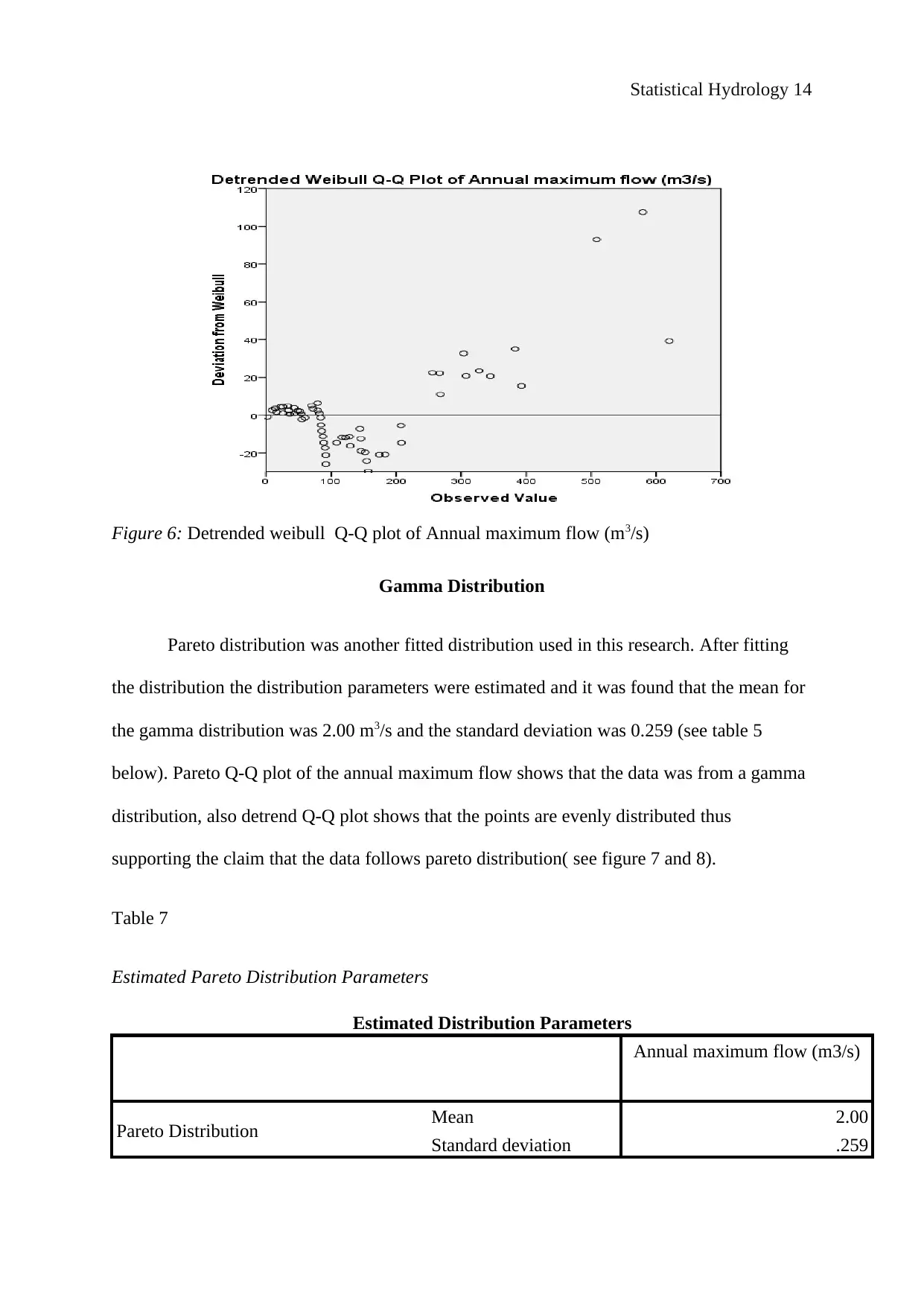
Figure 6: Detrended weibull Q-Q plot of Annual maximum flow (m3/s)
Gamma Distribution
Pareto distribution was another fitted distribution used in this research. After fitting
the distribution the distribution parameters were estimated and it was found that the mean for
the gamma distribution was 2.00 m3/s and the standard deviation was 0.259 (see table 5
below). Pareto Q-Q plot of the annual maximum flow shows that the data was from a gamma
distribution, also detrend Q-Q plot shows that the points are evenly distributed thus
supporting the claim that the data follows pareto distribution( see figure 7 and 8).
Table 7
Estimated Pareto Distribution Parameters
Estimated Distribution Parameters
Annual maximum flow (m3/s)
Pareto Distribution Mean 2.00
Standard deviation .259
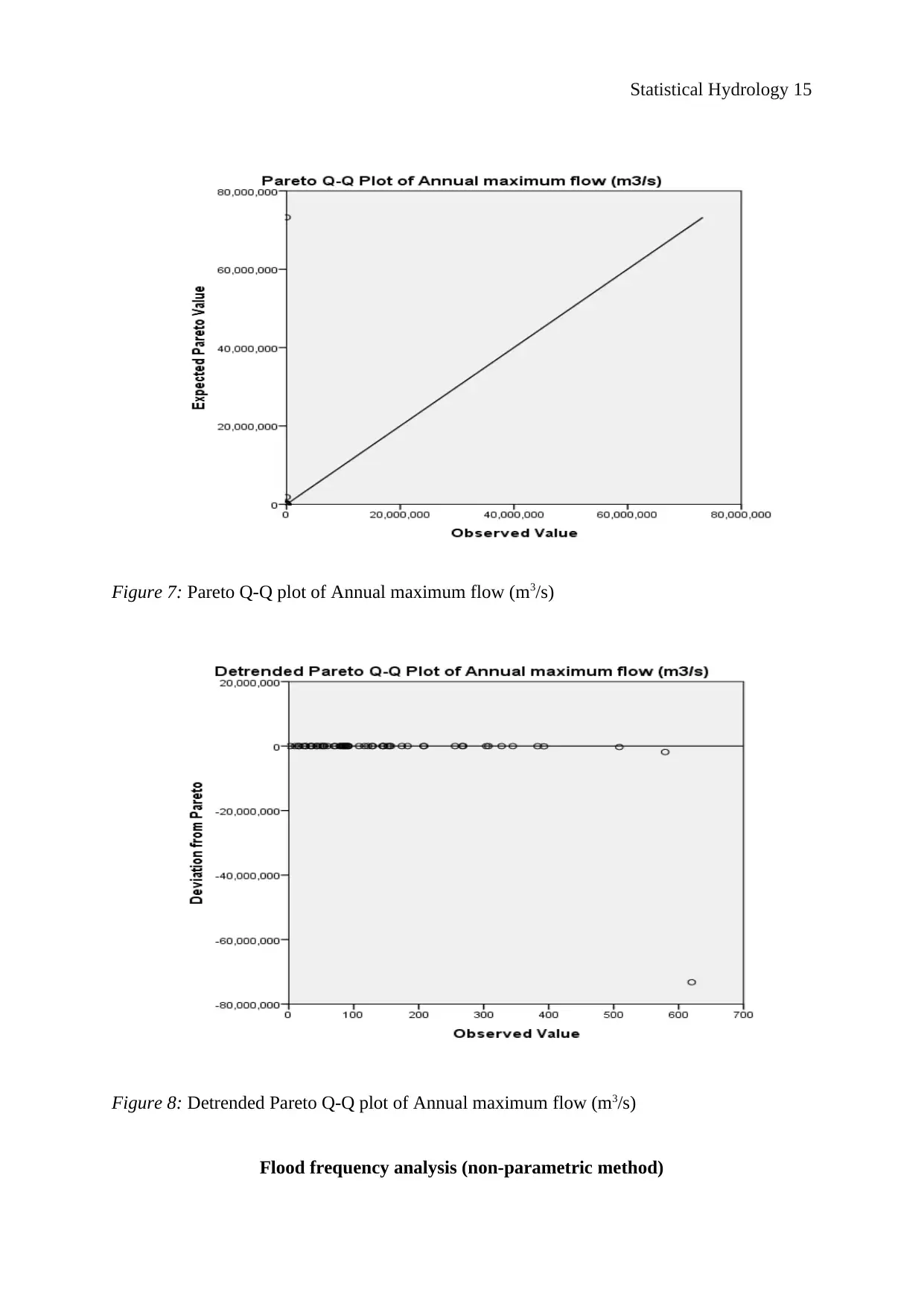
Figure 7: Pareto Q-Q plot of Annual maximum flow (m3/s)
Figure 8: Detrended Pareto Q-Q plot of Annual maximum flow (m3/s)
Flood frequency analysis (non-parametric method)
⊘ This is a preview!⊘
Do you want full access?
Subscribe today to unlock all pages.

Trusted by 1+ million students worldwide
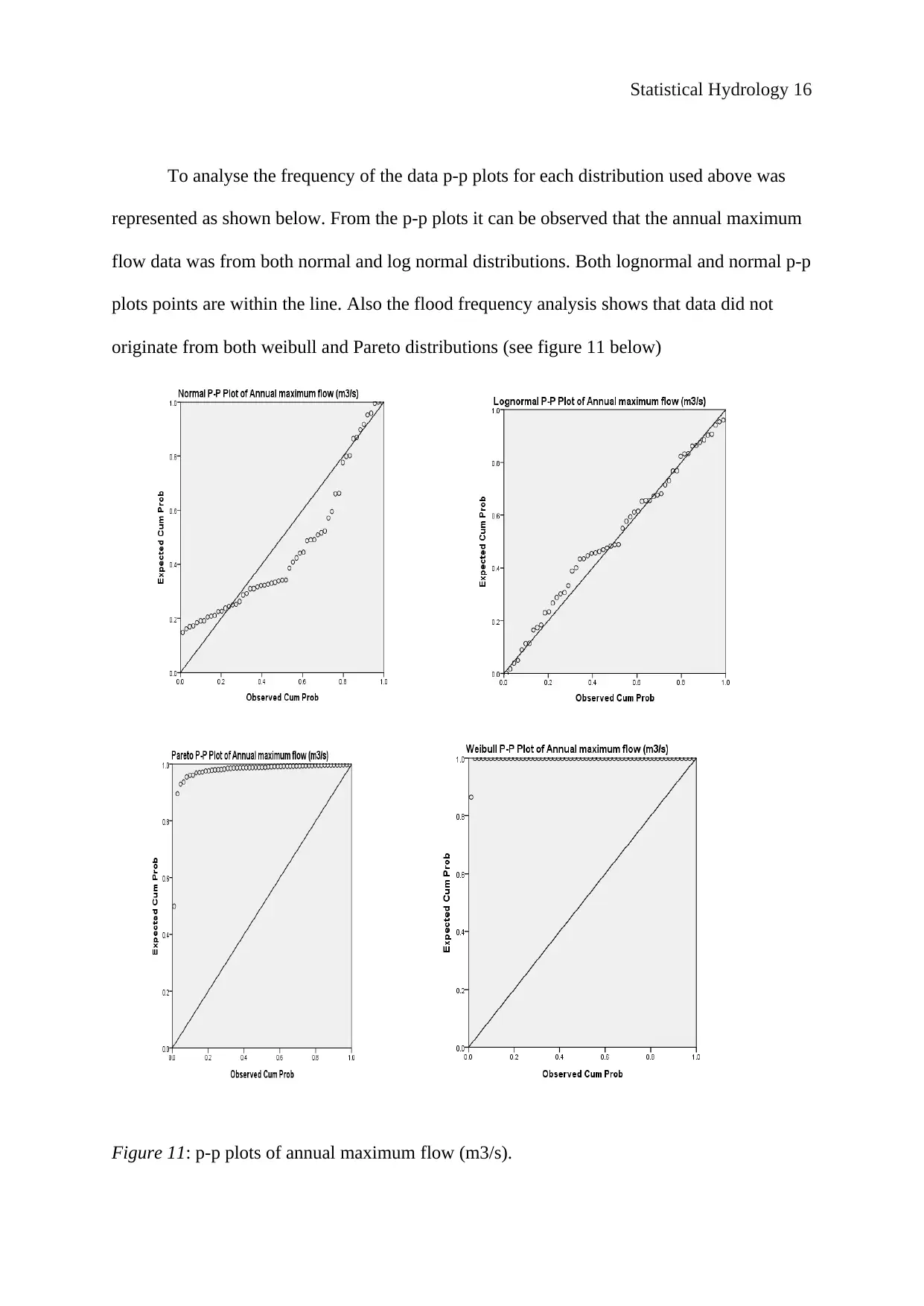
To analyse the frequency of the data p-p plots for each distribution used above was
represented as shown below. From the p-p plots it can be observed that the annual maximum
flow data was from both normal and log normal distributions. Both lognormal and normal p-p
plots points are within the line. Also the flood frequency analysis shows that data did not
originate from both weibull and Pareto distributions (see figure 11 below)
Figure 11: p-p plots of annual maximum flow (m3/s).
Paraphrase This Document
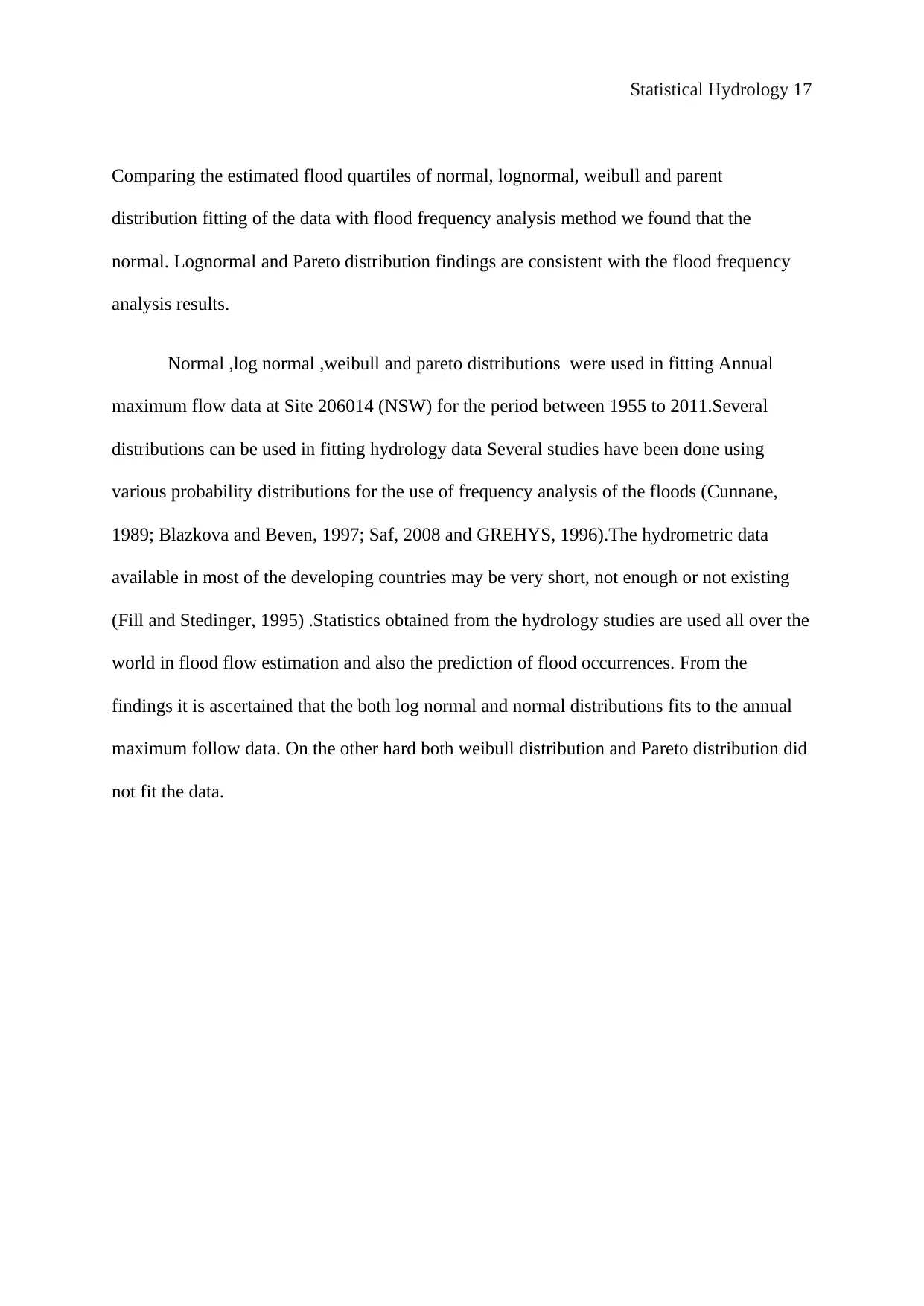
Comparing the estimated flood quartiles of normal, lognormal, weibull and parent
distribution fitting of the data with flood frequency analysis method we found that the
normal. Lognormal and Pareto distribution findings are consistent with the flood frequency
analysis results.
Normal ,log normal ,weibull and pareto distributions were used in fitting Annual
maximum flow data at Site 206014 (NSW) for the period between 1955 to 2011.Several
distributions can be used in fitting hydrology data Several studies have been done using
various probability distributions for the use of frequency analysis of the floods (Cunnane,
1989; Blazkova and Beven, 1997; Saf, 2008 and GREHYS, 1996).The hydrometric data
available in most of the developing countries may be very short, not enough or not existing
(Fill and Stedinger, 1995) .Statistics obtained from the hydrology studies are used all over the
world in flood flow estimation and also the prediction of flood occurrences. From the
findings it is ascertained that the both log normal and normal distributions fits to the annual
maximum follow data. On the other hard both weibull distribution and Pareto distribution did
not fit the data.
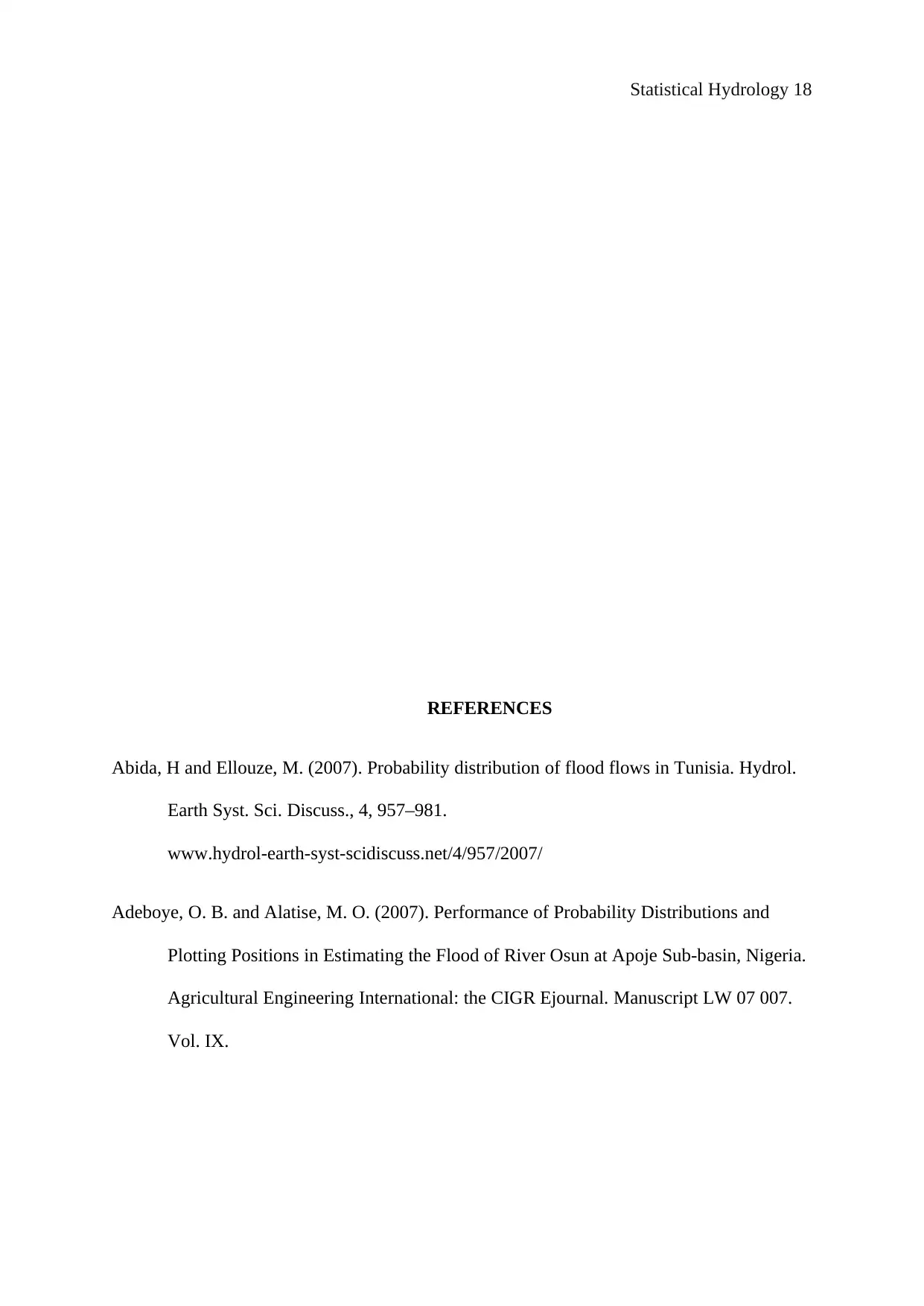
REFERENCES
Abida, H and Ellouze, M. (2007). Probability distribution of flood flows in Tunisia. Hydrol.
Earth Syst. Sci. Discuss., 4, 957–981.
www.hydrol-earth-syst-scidiscuss.net/4/957/2007/
Adeboye, O. B. and Alatise, M. O. (2007). Performance of Probability Distributions and
Plotting Positions in Estimating the Flood of River Osun at Apoje Sub-basin, Nigeria.
Agricultural Engineering International: the CIGR Ejournal. Manuscript LW 07 007.
Vol. IX.
⊘ This is a preview!⊘
Do you want full access?
Subscribe today to unlock all pages.

Trusted by 1+ million students worldwide
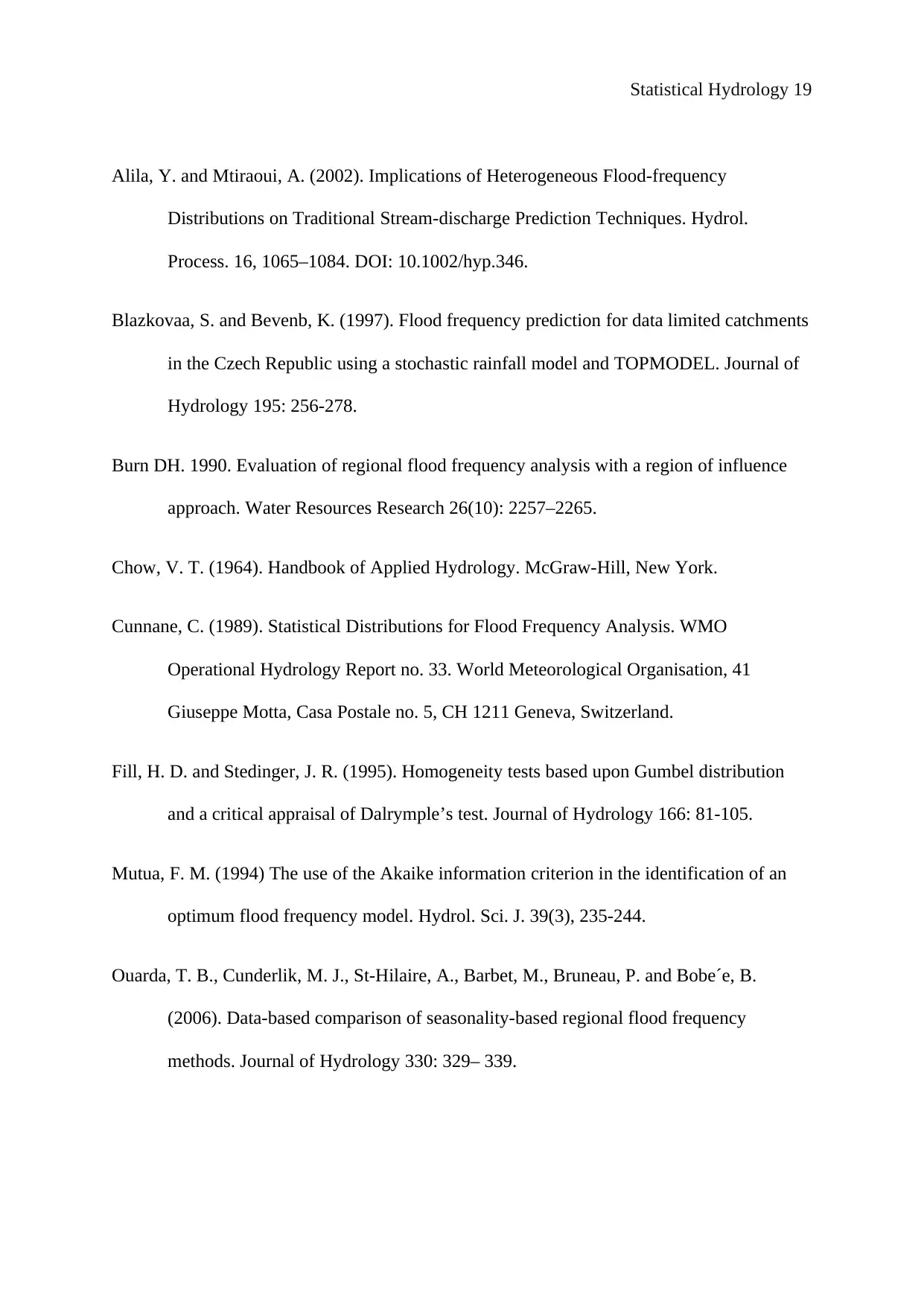
Alila, Y. and Mtiraoui, A. (2002). Implications of Heterogeneous Flood-frequency
Distributions on Traditional Stream-discharge Prediction Techniques. Hydrol.
Process. 16, 1065–1084. DOI: 10.1002/hyp.346.
Blazkovaa, S. and Bevenb, K. (1997). Flood frequency prediction for data limited catchments
in the Czech Republic using a stochastic rainfall model and TOPMODEL. Journal of
Hydrology 195: 256-278.
Burn DH. 1990. Evaluation of regional flood frequency analysis with a region of influence
approach. Water Resources Research 26(10): 2257–2265.
Chow, V. T. (1964). Handbook of Applied Hydrology. McGraw-Hill, New York.
Cunnane, C. (1989). Statistical Distributions for Flood Frequency Analysis. WMO
Operational Hydrology Report no. 33. World Meteorological Organisation, 41
Giuseppe Motta, Casa Postale no. 5, CH 1211 Geneva, Switzerland.
Fill, H. D. and Stedinger, J. R. (1995). Homogeneity tests based upon Gumbel distribution
and a critical appraisal of Dalrymple’s test. Journal of Hydrology 166: 81-105.
Mutua, F. M. (1994) The use of the Akaike information criterion in the identification of an
optimum flood frequency model. Hydrol. Sci. J. 39(3), 235-244.
Ouarda, T. B., Cunderlik, M. J., St-Hilaire, A., Barbet, M., Bruneau, P. and Bobe´e, B.
(2006). Data-based comparison of seasonality-based regional flood frequency
methods. Journal of Hydrology 330: 329– 339.
Paraphrase This Document
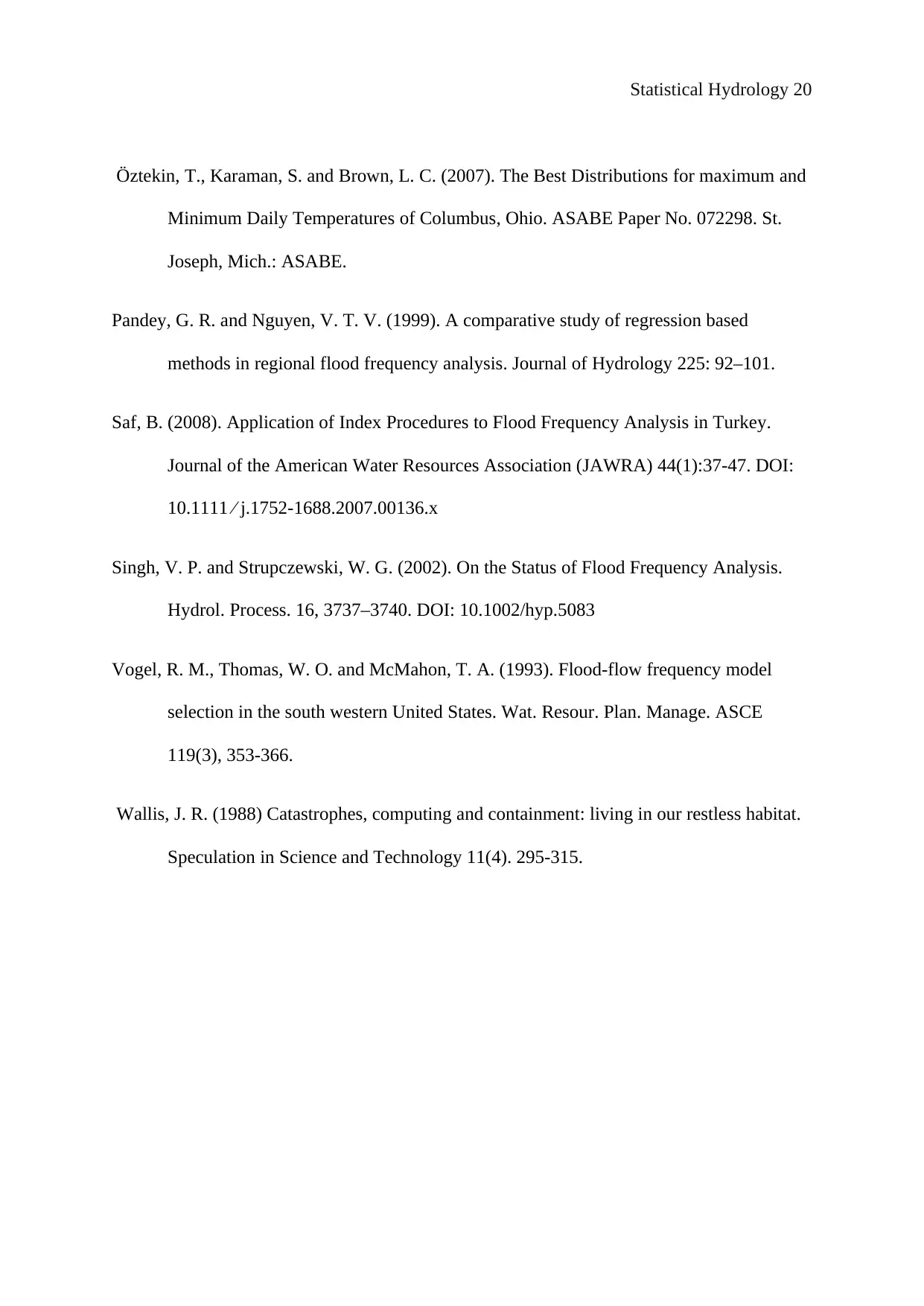
Öztekin, T., Karaman, S. and Brown, L. C. (2007). The Best Distributions for maximum and
Minimum Daily Temperatures of Columbus, Ohio. ASABE Paper No. 072298. St.
Joseph, Mich.: ASABE.
Pandey, G. R. and Nguyen, V. T. V. (1999). A comparative study of regression based
methods in regional flood frequency analysis. Journal of Hydrology 225: 92–101.
Saf, B. (2008). Application of Index Procedures to Flood Frequency Analysis in Turkey.
Journal of the American Water Resources Association (JAWRA) 44(1):37-47. DOI:
10.1111 ⁄ j.1752-1688.2007.00136.x
Singh, V. P. and Strupczewski, W. G. (2002). On the Status of Flood Frequency Analysis.
Hydrol. Process. 16, 3737–3740. DOI: 10.1002/hyp.5083
Vogel, R. M., Thomas, W. O. and McMahon, T. A. (1993). Flood-flow frequency model
selection in the south western United States. Wat. Resour. Plan. Manage. ASCE
119(3), 353-366.
Wallis, J. R. (1988) Catastrophes, computing and containment: living in our restless habitat.
Speculation in Science and Technology 11(4). 295-315.
Related Documents

Your All-in-One AI-Powered Toolkit for Academic Success.
+13062052269
info@desklib.com
Available 24*7 on WhatsApp / Email
© 2024 | Zucol Services PVT LTD | All rights reserved.