Statistics for Management: Data Analysis, Methods and Planning
VerifiedAdded on 2020/10/22
|12
|3134
|422
Report
AI Summary
This report provides a detailed exploration of data analysis techniques and statistical methods relevant to business management and planning. It begins with an introduction to qualitative and quantitative research methods, differentiating between their approaches and applications. The report delves i...
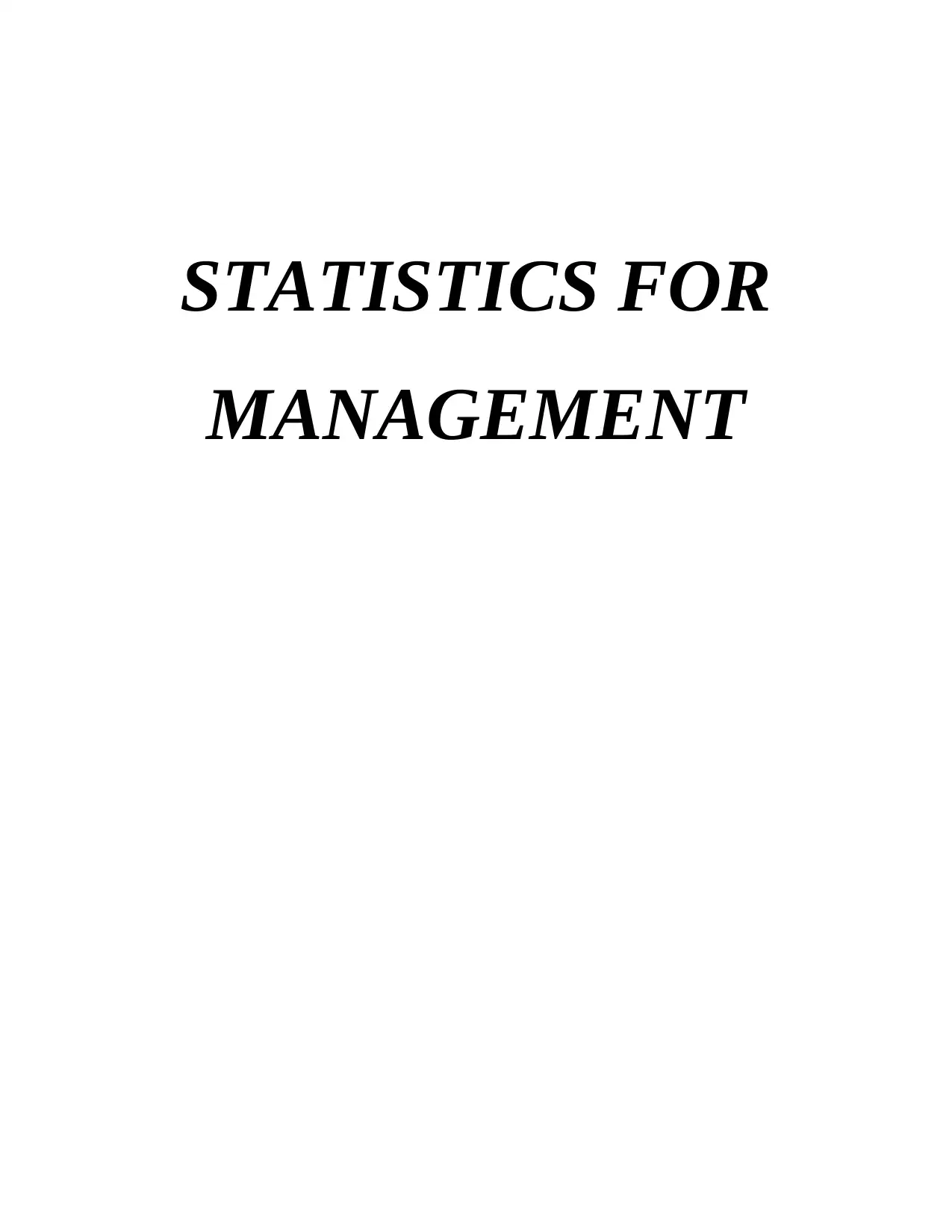
STATISTICS FOR
MANAGEMENT
MANAGEMENT
Paraphrase This Document
Need a fresh take? Get an instant paraphrase of this document with our AI Paraphraser
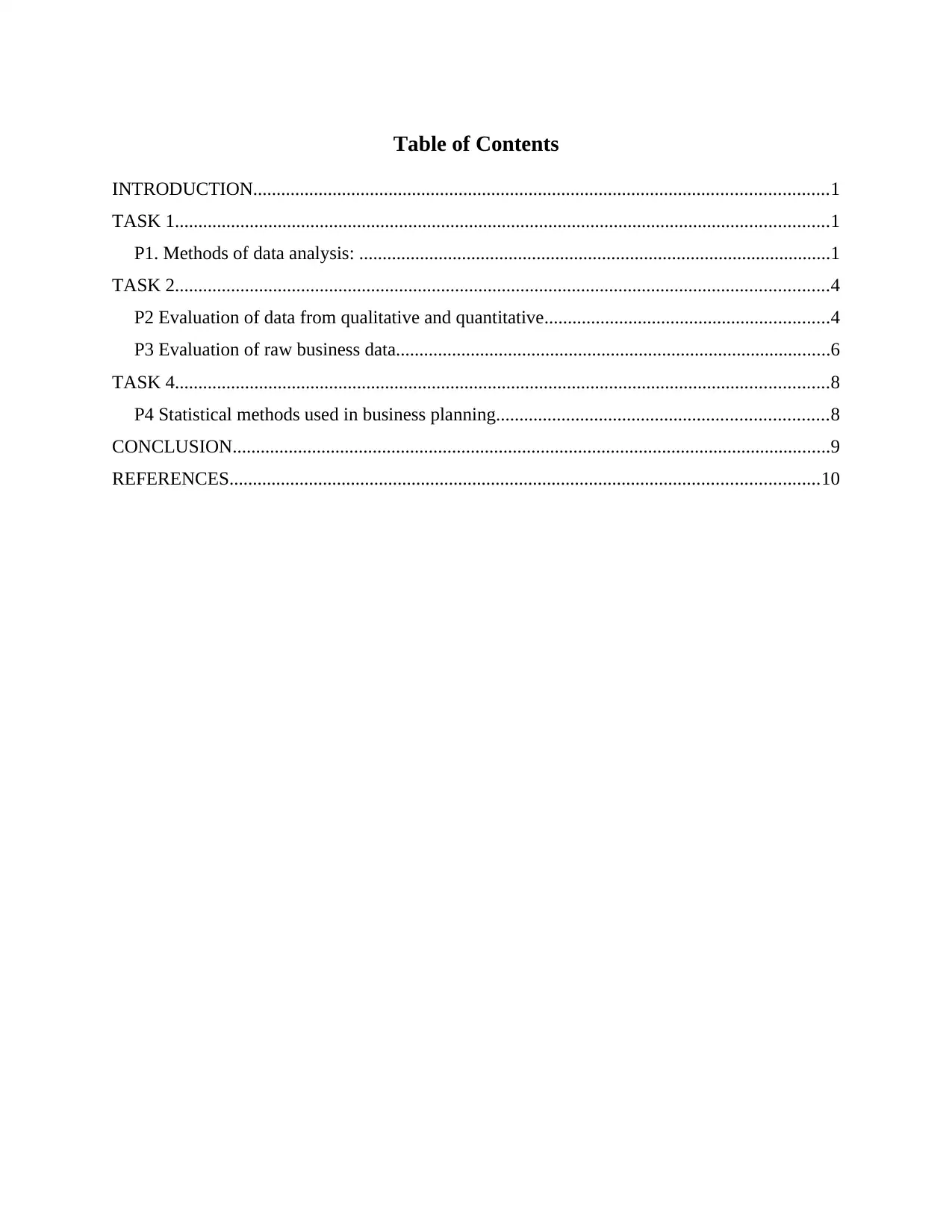
Table of Contents
INTRODUCTION...........................................................................................................................1
TASK 1............................................................................................................................................1
P1. Methods of data analysis: .....................................................................................................1
TASK 2............................................................................................................................................4
P2 Evaluation of data from qualitative and quantitative.............................................................4
P3 Evaluation of raw business data.............................................................................................6
TASK 4............................................................................................................................................8
P4 Statistical methods used in business planning.......................................................................8
CONCLUSION................................................................................................................................9
REFERENCES..............................................................................................................................10
INTRODUCTION...........................................................................................................................1
TASK 1............................................................................................................................................1
P1. Methods of data analysis: .....................................................................................................1
TASK 2............................................................................................................................................4
P2 Evaluation of data from qualitative and quantitative.............................................................4
P3 Evaluation of raw business data.............................................................................................6
TASK 4............................................................................................................................................8
P4 Statistical methods used in business planning.......................................................................8
CONCLUSION................................................................................................................................9
REFERENCES..............................................................................................................................10
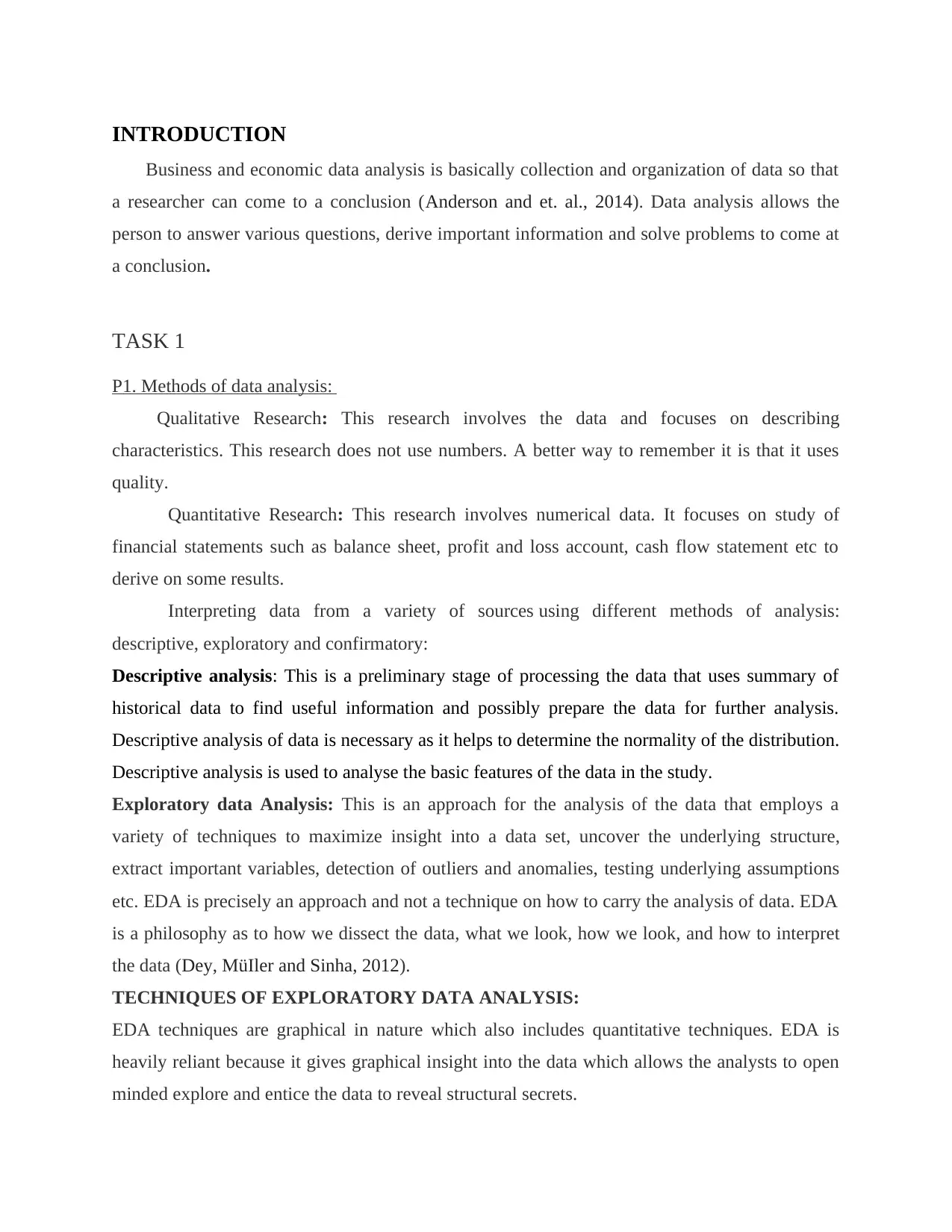
INTRODUCTION
Business and economic data analysis is basically collection and organization of data so that
a researcher can come to a conclusion (Anderson and et. al., 2014). Data analysis allows the
person to answer various questions, derive important information and solve problems to come at
a conclusion.
TASK 1
P1. Methods of data analysis:
Qualitative Research: This research involves the data and focuses on describing
characteristics. This research does not use numbers. A better way to remember it is that it uses
quality.
Quantitative Research: This research involves numerical data. It focuses on study of
financial statements such as balance sheet, profit and loss account, cash flow statement etc to
derive on some results.
Interpreting data from a variety of sources using different methods of analysis:
descriptive, exploratory and confirmatory:
Descriptive analysis: This is a preliminary stage of processing the data that uses summary of
historical data to find useful information and possibly prepare the data for further analysis.
Descriptive analysis of data is necessary as it helps to determine the normality of the distribution.
Descriptive analysis is used to analyse the basic features of the data in the study.
Exploratory data Analysis: This is an approach for the analysis of the data that employs a
variety of techniques to maximize insight into a data set, uncover the underlying structure,
extract important variables, detection of outliers and anomalies, testing underlying assumptions
etc. EDA is precisely an approach and not a technique on how to carry the analysis of data. EDA
is a philosophy as to how we dissect the data, what we look, how we look, and how to interpret
the data (Dey, MüIler and Sinha, 2012).
TECHNIQUES OF EXPLORATORY DATA ANALYSIS:
EDA techniques are graphical in nature which also includes quantitative techniques. EDA is
heavily reliant because it gives graphical insight into the data which allows the analysts to open
minded explore and entice the data to reveal structural secrets.
Business and economic data analysis is basically collection and organization of data so that
a researcher can come to a conclusion (Anderson and et. al., 2014). Data analysis allows the
person to answer various questions, derive important information and solve problems to come at
a conclusion.
TASK 1
P1. Methods of data analysis:
Qualitative Research: This research involves the data and focuses on describing
characteristics. This research does not use numbers. A better way to remember it is that it uses
quality.
Quantitative Research: This research involves numerical data. It focuses on study of
financial statements such as balance sheet, profit and loss account, cash flow statement etc to
derive on some results.
Interpreting data from a variety of sources using different methods of analysis:
descriptive, exploratory and confirmatory:
Descriptive analysis: This is a preliminary stage of processing the data that uses summary of
historical data to find useful information and possibly prepare the data for further analysis.
Descriptive analysis of data is necessary as it helps to determine the normality of the distribution.
Descriptive analysis is used to analyse the basic features of the data in the study.
Exploratory data Analysis: This is an approach for the analysis of the data that employs a
variety of techniques to maximize insight into a data set, uncover the underlying structure,
extract important variables, detection of outliers and anomalies, testing underlying assumptions
etc. EDA is precisely an approach and not a technique on how to carry the analysis of data. EDA
is a philosophy as to how we dissect the data, what we look, how we look, and how to interpret
the data (Dey, MüIler and Sinha, 2012).
TECHNIQUES OF EXPLORATORY DATA ANALYSIS:
EDA techniques are graphical in nature which also includes quantitative techniques. EDA is
heavily reliant because it gives graphical insight into the data which allows the analysts to open
minded explore and entice the data to reveal structural secrets.
⊘ This is a preview!⊘
Do you want full access?
Subscribe today to unlock all pages.

Trusted by 1+ million students worldwide
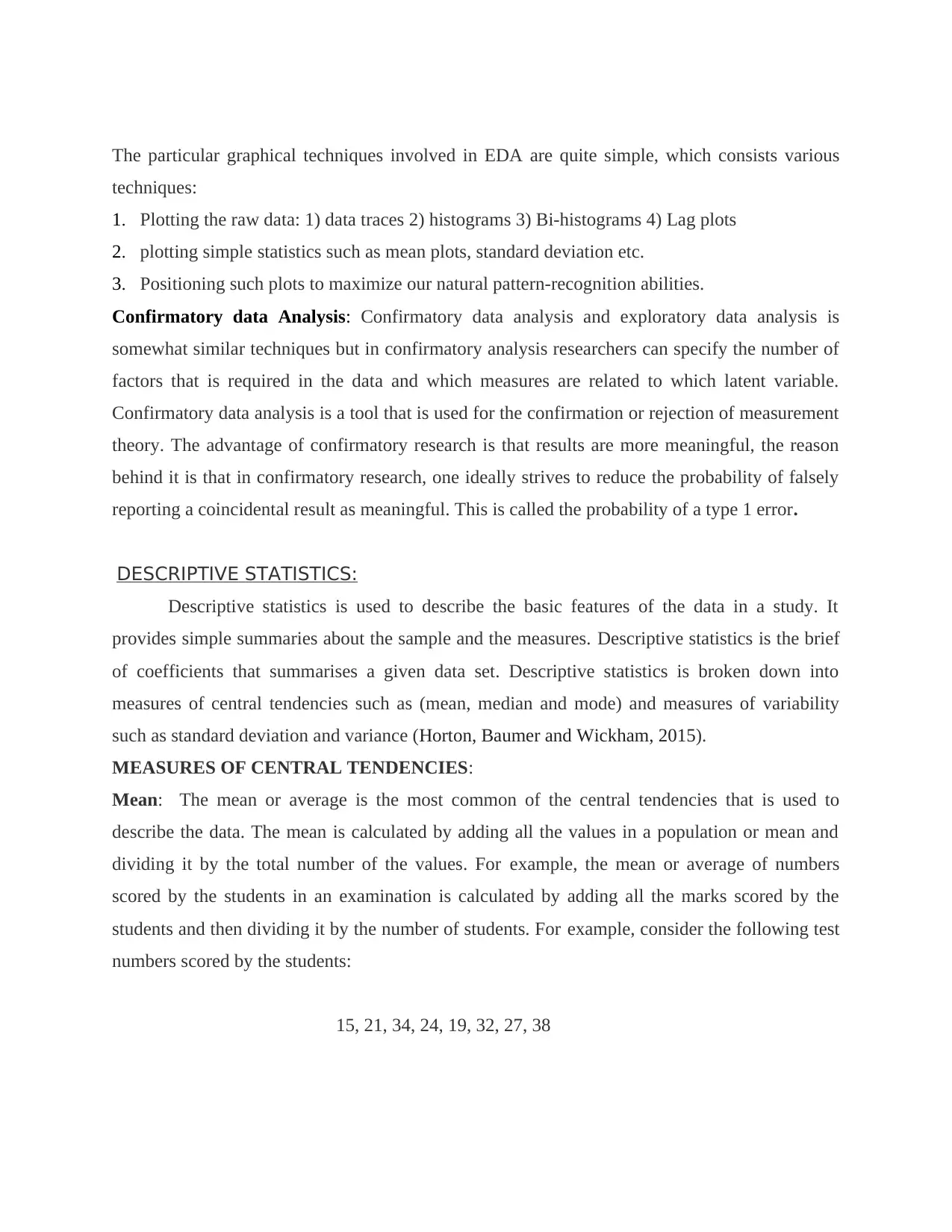
The particular graphical techniques involved in EDA are quite simple, which consists various
techniques:
1. Plotting the raw data: 1) data traces 2) histograms 3) Bi-histograms 4) Lag plots
2. plotting simple statistics such as mean plots, standard deviation etc.
3. Positioning such plots to maximize our natural pattern-recognition abilities.
Confirmatory data Analysis: Confirmatory data analysis and exploratory data analysis is
somewhat similar techniques but in confirmatory analysis researchers can specify the number of
factors that is required in the data and which measures are related to which latent variable.
Confirmatory data analysis is a tool that is used for the confirmation or rejection of measurement
theory. The advantage of confirmatory research is that results are more meaningful, the reason
behind it is that in confirmatory research, one ideally strives to reduce the probability of falsely
reporting a coincidental result as meaningful. This is called the probability of a type 1 error.
DESCRIPTIVE STATISTICS:
Descriptive statistics is used to describe the basic features of the data in a study. It
provides simple summaries about the sample and the measures. Descriptive statistics is the brief
of coefficients that summarises a given data set. Descriptive statistics is broken down into
measures of central tendencies such as (mean, median and mode) and measures of variability
such as standard deviation and variance (Horton, Baumer and Wickham, 2015).
MEASURES OF CENTRAL TENDENCIES:
Mean: The mean or average is the most common of the central tendencies that is used to
describe the data. The mean is calculated by adding all the values in a population or mean and
dividing it by the total number of the values. For example, the mean or average of numbers
scored by the students in an examination is calculated by adding all the marks scored by the
students and then dividing it by the number of students. For example, consider the following test
numbers scored by the students:
15, 21, 34, 24, 19, 32, 27, 38
techniques:
1. Plotting the raw data: 1) data traces 2) histograms 3) Bi-histograms 4) Lag plots
2. plotting simple statistics such as mean plots, standard deviation etc.
3. Positioning such plots to maximize our natural pattern-recognition abilities.
Confirmatory data Analysis: Confirmatory data analysis and exploratory data analysis is
somewhat similar techniques but in confirmatory analysis researchers can specify the number of
factors that is required in the data and which measures are related to which latent variable.
Confirmatory data analysis is a tool that is used for the confirmation or rejection of measurement
theory. The advantage of confirmatory research is that results are more meaningful, the reason
behind it is that in confirmatory research, one ideally strives to reduce the probability of falsely
reporting a coincidental result as meaningful. This is called the probability of a type 1 error.
DESCRIPTIVE STATISTICS:
Descriptive statistics is used to describe the basic features of the data in a study. It
provides simple summaries about the sample and the measures. Descriptive statistics is the brief
of coefficients that summarises a given data set. Descriptive statistics is broken down into
measures of central tendencies such as (mean, median and mode) and measures of variability
such as standard deviation and variance (Horton, Baumer and Wickham, 2015).
MEASURES OF CENTRAL TENDENCIES:
Mean: The mean or average is the most common of the central tendencies that is used to
describe the data. The mean is calculated by adding all the values in a population or mean and
dividing it by the total number of the values. For example, the mean or average of numbers
scored by the students in an examination is calculated by adding all the marks scored by the
students and then dividing it by the number of students. For example, consider the following test
numbers scored by the students:
15, 21, 34, 24, 19, 32, 27, 38
Paraphrase This Document
Need a fresh take? Get an instant paraphrase of this document with our AI Paraphraser
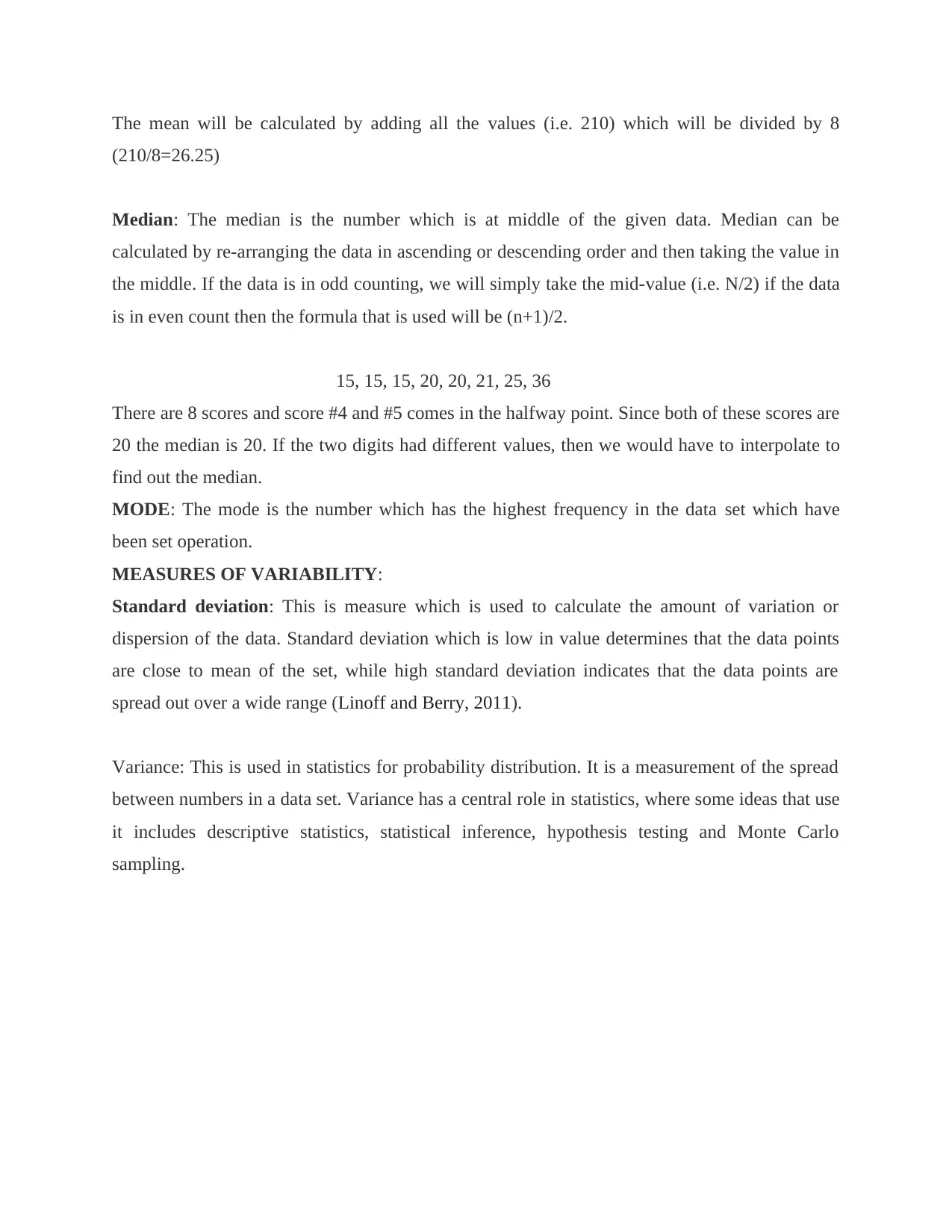
The mean will be calculated by adding all the values (i.e. 210) which will be divided by 8
(210/8=26.25)
Median: The median is the number which is at middle of the given data. Median can be
calculated by re-arranging the data in ascending or descending order and then taking the value in
the middle. If the data is in odd counting, we will simply take the mid-value (i.e. N/2) if the data
is in even count then the formula that is used will be (n+1)/2.
15, 15, 15, 20, 20, 21, 25, 36
There are 8 scores and score #4 and #5 comes in the halfway point. Since both of these scores are
20 the median is 20. If the two digits had different values, then we would have to interpolate to
find out the median.
MODE: The mode is the number which has the highest frequency in the data set which have
been set operation.
MEASURES OF VARIABILITY:
Standard deviation: This is measure which is used to calculate the amount of variation or
dispersion of the data. Standard deviation which is low in value determines that the data points
are close to mean of the set, while high standard deviation indicates that the data points are
spread out over a wide range (Linoff and Berry, 2011).
Variance: This is used in statistics for probability distribution. It is a measurement of the spread
between numbers in a data set. Variance has a central role in statistics, where some ideas that use
it includes descriptive statistics, statistical inference, hypothesis testing and Monte Carlo
sampling.
(210/8=26.25)
Median: The median is the number which is at middle of the given data. Median can be
calculated by re-arranging the data in ascending or descending order and then taking the value in
the middle. If the data is in odd counting, we will simply take the mid-value (i.e. N/2) if the data
is in even count then the formula that is used will be (n+1)/2.
15, 15, 15, 20, 20, 21, 25, 36
There are 8 scores and score #4 and #5 comes in the halfway point. Since both of these scores are
20 the median is 20. If the two digits had different values, then we would have to interpolate to
find out the median.
MODE: The mode is the number which has the highest frequency in the data set which have
been set operation.
MEASURES OF VARIABILITY:
Standard deviation: This is measure which is used to calculate the amount of variation or
dispersion of the data. Standard deviation which is low in value determines that the data points
are close to mean of the set, while high standard deviation indicates that the data points are
spread out over a wide range (Linoff and Berry, 2011).
Variance: This is used in statistics for probability distribution. It is a measurement of the spread
between numbers in a data set. Variance has a central role in statistics, where some ideas that use
it includes descriptive statistics, statistical inference, hypothesis testing and Monte Carlo
sampling.
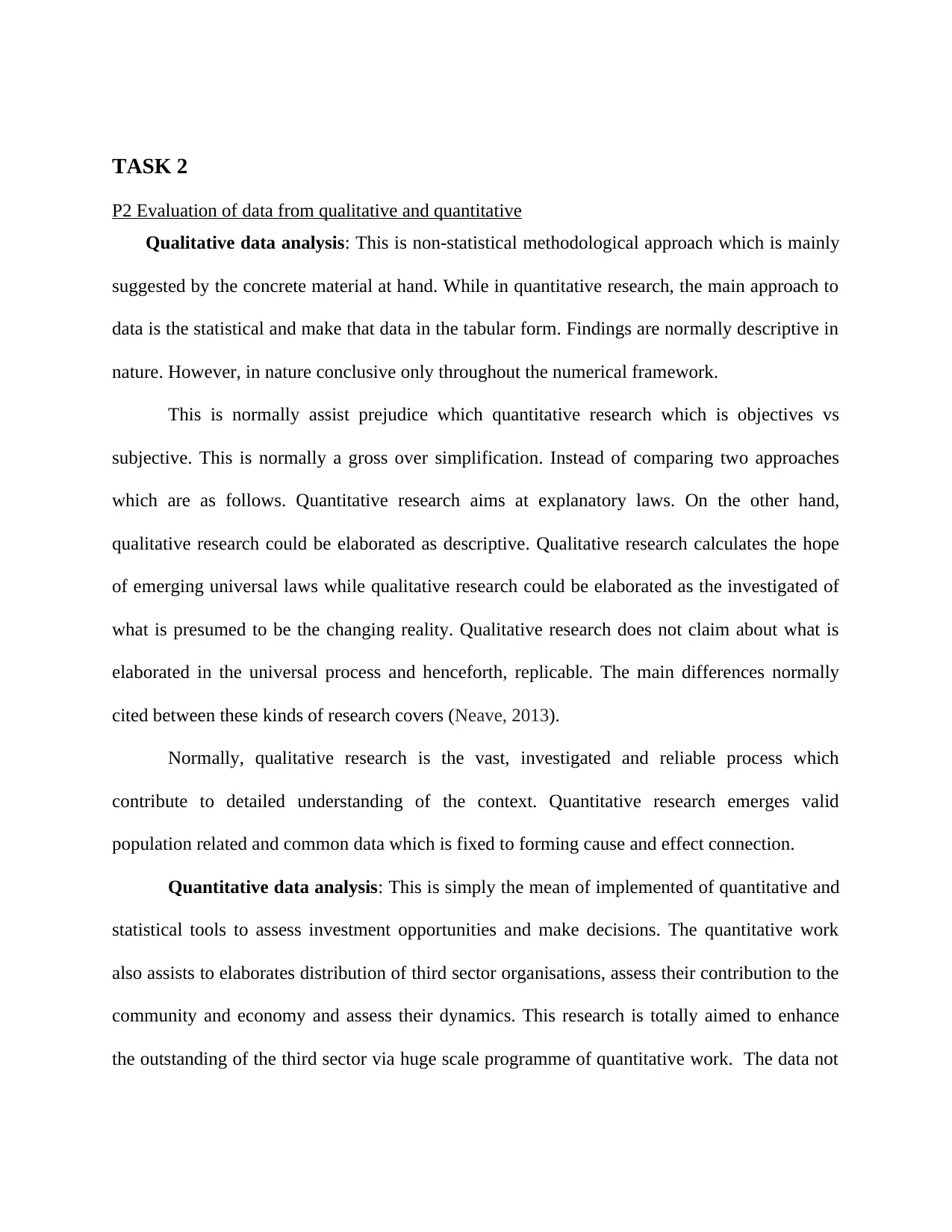
TASK 2
P2 Evaluation of data from qualitative and quantitative
Qualitative data analysis: This is non-statistical methodological approach which is mainly
suggested by the concrete material at hand. While in quantitative research, the main approach to
data is the statistical and make that data in the tabular form. Findings are normally descriptive in
nature. However, in nature conclusive only throughout the numerical framework.
This is normally assist prejudice which quantitative research which is objectives vs
subjective. This is normally a gross over simplification. Instead of comparing two approaches
which are as follows. Quantitative research aims at explanatory laws. On the other hand,
qualitative research could be elaborated as descriptive. Qualitative research calculates the hope
of emerging universal laws while qualitative research could be elaborated as the investigated of
what is presumed to be the changing reality. Qualitative research does not claim about what is
elaborated in the universal process and henceforth, replicable. The main differences normally
cited between these kinds of research covers (Neave, 2013).
Normally, qualitative research is the vast, investigated and reliable process which
contribute to detailed understanding of the context. Quantitative research emerges valid
population related and common data which is fixed to forming cause and effect connection.
Quantitative data analysis: This is simply the mean of implemented of quantitative and
statistical tools to assess investment opportunities and make decisions. The quantitative work
also assists to elaborates distribution of third sector organisations, assess their contribution to the
community and economy and assess their dynamics. This research is totally aimed to enhance
the outstanding of the third sector via huge scale programme of quantitative work. The data not
P2 Evaluation of data from qualitative and quantitative
Qualitative data analysis: This is non-statistical methodological approach which is mainly
suggested by the concrete material at hand. While in quantitative research, the main approach to
data is the statistical and make that data in the tabular form. Findings are normally descriptive in
nature. However, in nature conclusive only throughout the numerical framework.
This is normally assist prejudice which quantitative research which is objectives vs
subjective. This is normally a gross over simplification. Instead of comparing two approaches
which are as follows. Quantitative research aims at explanatory laws. On the other hand,
qualitative research could be elaborated as descriptive. Qualitative research calculates the hope
of emerging universal laws while qualitative research could be elaborated as the investigated of
what is presumed to be the changing reality. Qualitative research does not claim about what is
elaborated in the universal process and henceforth, replicable. The main differences normally
cited between these kinds of research covers (Neave, 2013).
Normally, qualitative research is the vast, investigated and reliable process which
contribute to detailed understanding of the context. Quantitative research emerges valid
population related and common data which is fixed to forming cause and effect connection.
Quantitative data analysis: This is simply the mean of implemented of quantitative and
statistical tools to assess investment opportunities and make decisions. The quantitative work
also assists to elaborates distribution of third sector organisations, assess their contribution to the
community and economy and assess their dynamics. This research is totally aimed to enhance
the outstanding of the third sector via huge scale programme of quantitative work. The data not
⊘ This is a preview!⊘
Do you want full access?
Subscribe today to unlock all pages.

Trusted by 1+ million students worldwide
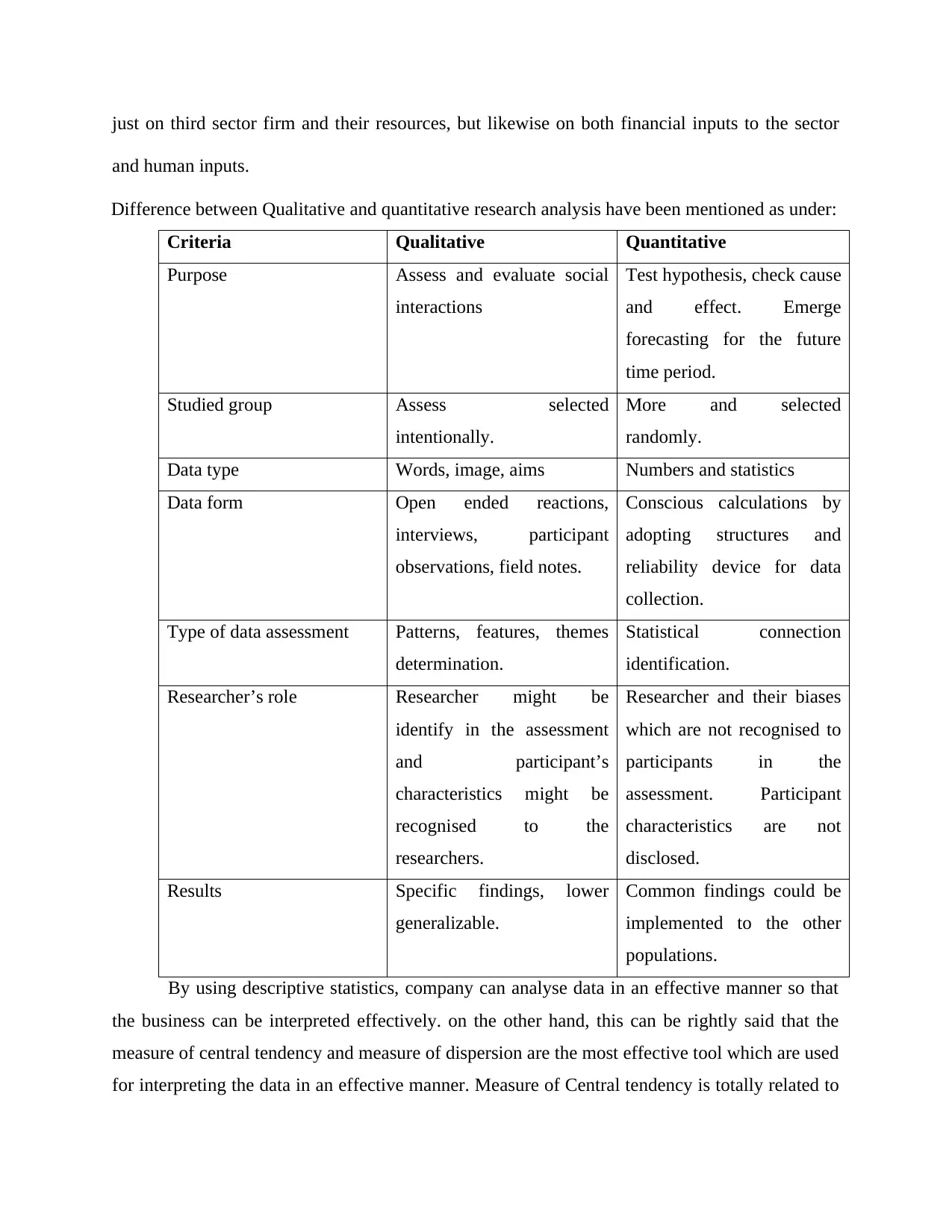
just on third sector firm and their resources, but likewise on both financial inputs to the sector
and human inputs.
Difference between Qualitative and quantitative research analysis have been mentioned as under:
Criteria Qualitative Quantitative
Purpose Assess and evaluate social
interactions
Test hypothesis, check cause
and effect. Emerge
forecasting for the future
time period.
Studied group Assess selected
intentionally.
More and selected
randomly.
Data type Words, image, aims Numbers and statistics
Data form Open ended reactions,
interviews, participant
observations, field notes.
Conscious calculations by
adopting structures and
reliability device for data
collection.
Type of data assessment Patterns, features, themes
determination.
Statistical connection
identification.
Researcher’s role Researcher might be
identify in the assessment
and participant’s
characteristics might be
recognised to the
researchers.
Researcher and their biases
which are not recognised to
participants in the
assessment. Participant
characteristics are not
disclosed.
Results Specific findings, lower
generalizable.
Common findings could be
implemented to the other
populations.
By using descriptive statistics, company can analyse data in an effective manner so that
the business can be interpreted effectively. on the other hand, this can be rightly said that the
measure of central tendency and measure of dispersion are the most effective tool which are used
for interpreting the data in an effective manner. Measure of Central tendency is totally related to
and human inputs.
Difference between Qualitative and quantitative research analysis have been mentioned as under:
Criteria Qualitative Quantitative
Purpose Assess and evaluate social
interactions
Test hypothesis, check cause
and effect. Emerge
forecasting for the future
time period.
Studied group Assess selected
intentionally.
More and selected
randomly.
Data type Words, image, aims Numbers and statistics
Data form Open ended reactions,
interviews, participant
observations, field notes.
Conscious calculations by
adopting structures and
reliability device for data
collection.
Type of data assessment Patterns, features, themes
determination.
Statistical connection
identification.
Researcher’s role Researcher might be
identify in the assessment
and participant’s
characteristics might be
recognised to the
researchers.
Researcher and their biases
which are not recognised to
participants in the
assessment. Participant
characteristics are not
disclosed.
Results Specific findings, lower
generalizable.
Common findings could be
implemented to the other
populations.
By using descriptive statistics, company can analyse data in an effective manner so that
the business can be interpreted effectively. on the other hand, this can be rightly said that the
measure of central tendency and measure of dispersion are the most effective tool which are used
for interpreting the data in an effective manner. Measure of Central tendency is totally related to
Paraphrase This Document
Need a fresh take? Get an instant paraphrase of this document with our AI Paraphraser
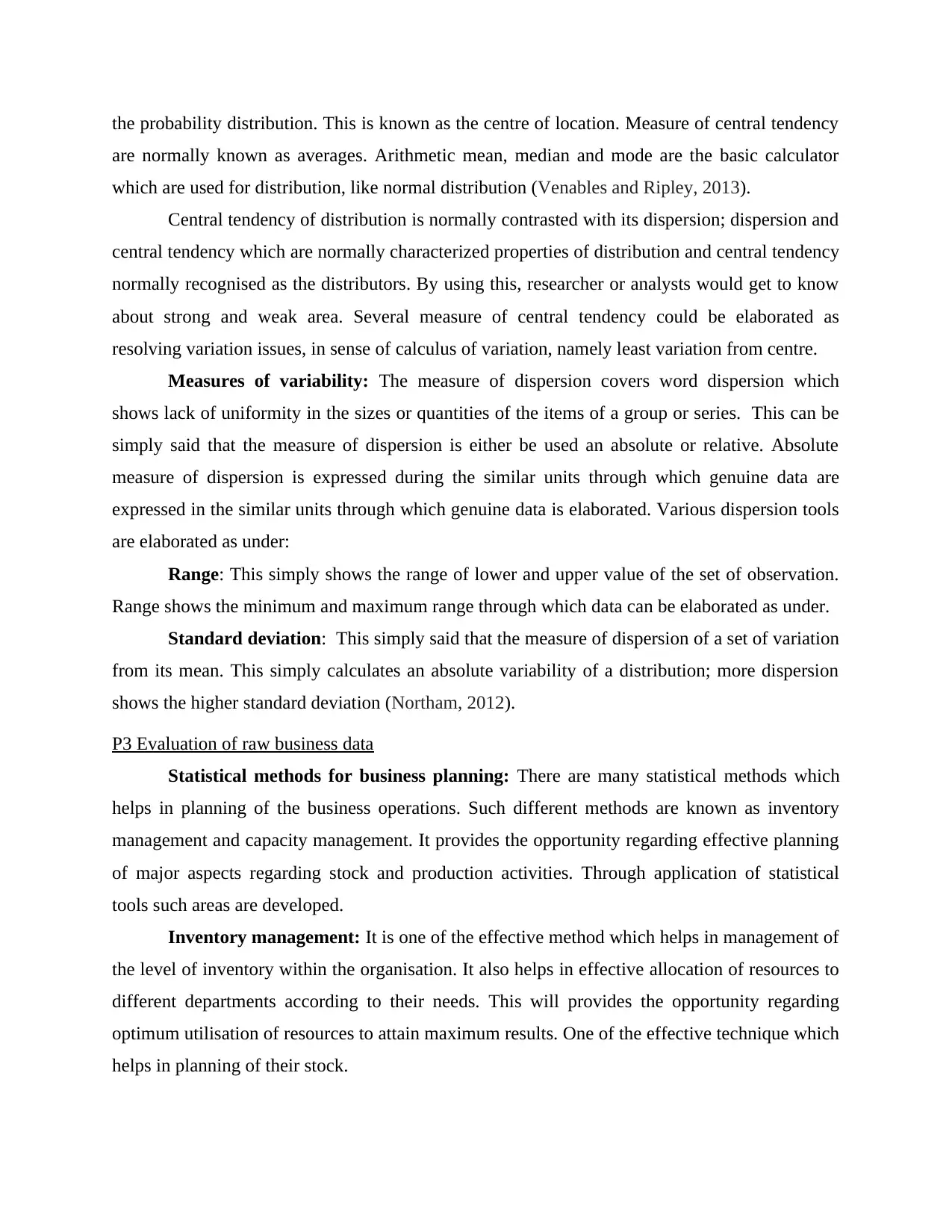
the probability distribution. This is known as the centre of location. Measure of central tendency
are normally known as averages. Arithmetic mean, median and mode are the basic calculator
which are used for distribution, like normal distribution (Venables and Ripley, 2013).
Central tendency of distribution is normally contrasted with its dispersion; dispersion and
central tendency which are normally characterized properties of distribution and central tendency
normally recognised as the distributors. By using this, researcher or analysts would get to know
about strong and weak area. Several measure of central tendency could be elaborated as
resolving variation issues, in sense of calculus of variation, namely least variation from centre.
Measures of variability: The measure of dispersion covers word dispersion which
shows lack of uniformity in the sizes or quantities of the items of a group or series. This can be
simply said that the measure of dispersion is either be used an absolute or relative. Absolute
measure of dispersion is expressed during the similar units through which genuine data are
expressed in the similar units through which genuine data is elaborated. Various dispersion tools
are elaborated as under:
Range: This simply shows the range of lower and upper value of the set of observation.
Range shows the minimum and maximum range through which data can be elaborated as under.
Standard deviation: This simply said that the measure of dispersion of a set of variation
from its mean. This simply calculates an absolute variability of a distribution; more dispersion
shows the higher standard deviation (Northam, 2012).
P3 Evaluation of raw business data
Statistical methods for business planning: There are many statistical methods which
helps in planning of the business operations. Such different methods are known as inventory
management and capacity management. It provides the opportunity regarding effective planning
of major aspects regarding stock and production activities. Through application of statistical
tools such areas are developed.
Inventory management: It is one of the effective method which helps in management of
the level of inventory within the organisation. It also helps in effective allocation of resources to
different departments according to their needs. This will provides the opportunity regarding
optimum utilisation of resources to attain maximum results. One of the effective technique which
helps in planning of their stock.
are normally known as averages. Arithmetic mean, median and mode are the basic calculator
which are used for distribution, like normal distribution (Venables and Ripley, 2013).
Central tendency of distribution is normally contrasted with its dispersion; dispersion and
central tendency which are normally characterized properties of distribution and central tendency
normally recognised as the distributors. By using this, researcher or analysts would get to know
about strong and weak area. Several measure of central tendency could be elaborated as
resolving variation issues, in sense of calculus of variation, namely least variation from centre.
Measures of variability: The measure of dispersion covers word dispersion which
shows lack of uniformity in the sizes or quantities of the items of a group or series. This can be
simply said that the measure of dispersion is either be used an absolute or relative. Absolute
measure of dispersion is expressed during the similar units through which genuine data are
expressed in the similar units through which genuine data is elaborated. Various dispersion tools
are elaborated as under:
Range: This simply shows the range of lower and upper value of the set of observation.
Range shows the minimum and maximum range through which data can be elaborated as under.
Standard deviation: This simply said that the measure of dispersion of a set of variation
from its mean. This simply calculates an absolute variability of a distribution; more dispersion
shows the higher standard deviation (Northam, 2012).
P3 Evaluation of raw business data
Statistical methods for business planning: There are many statistical methods which
helps in planning of the business operations. Such different methods are known as inventory
management and capacity management. It provides the opportunity regarding effective planning
of major aspects regarding stock and production activities. Through application of statistical
tools such areas are developed.
Inventory management: It is one of the effective method which helps in management of
the level of inventory within the organisation. It also helps in effective allocation of resources to
different departments according to their needs. This will provides the opportunity regarding
optimum utilisation of resources to attain maximum results. One of the effective technique which
helps in planning of their stock.
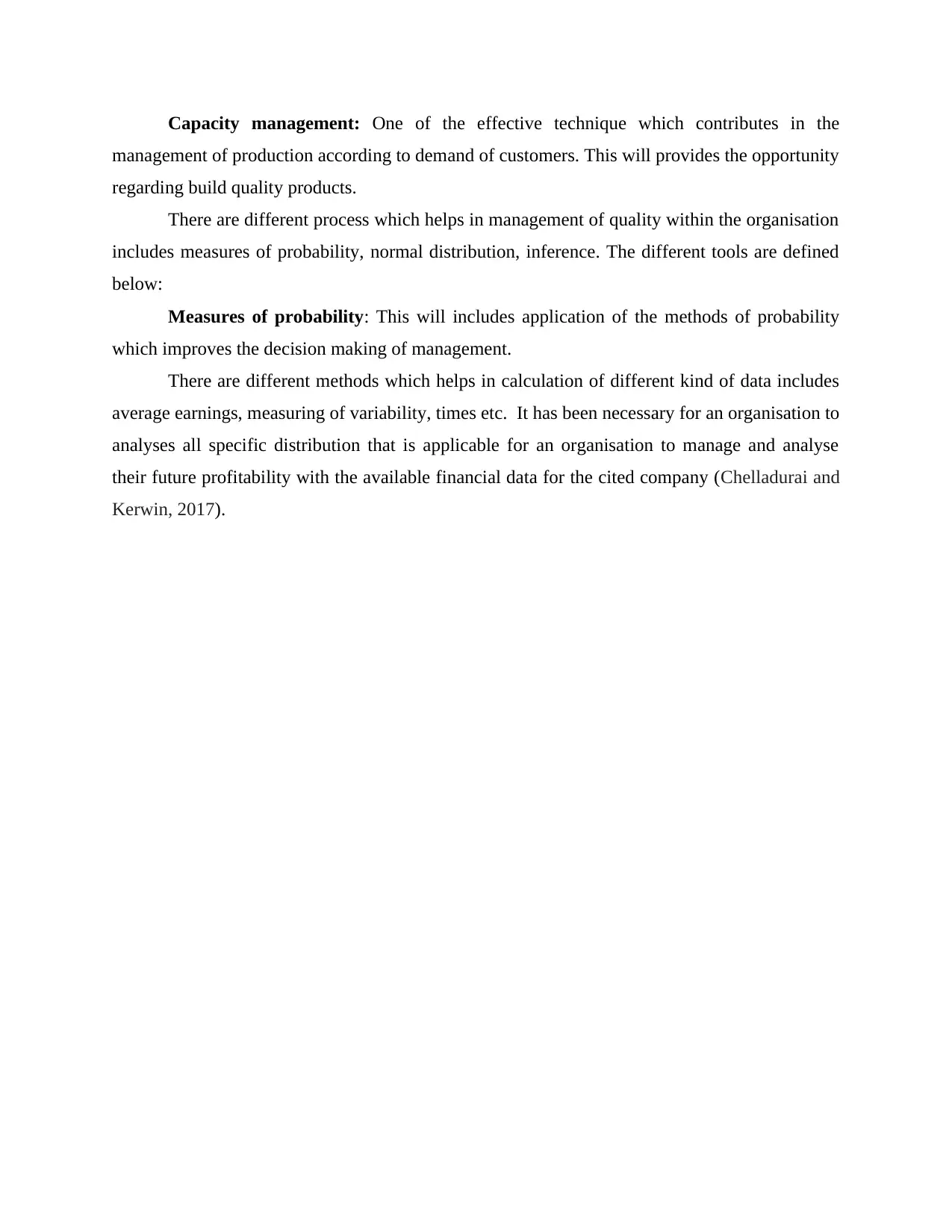
Capacity management: One of the effective technique which contributes in the
management of production according to demand of customers. This will provides the opportunity
regarding build quality products.
There are different process which helps in management of quality within the organisation
includes measures of probability, normal distribution, inference. The different tools are defined
below:
Measures of probability: This will includes application of the methods of probability
which improves the decision making of management.
There are different methods which helps in calculation of different kind of data includes
average earnings, measuring of variability, times etc. It has been necessary for an organisation to
analyses all specific distribution that is applicable for an organisation to manage and analyse
their future profitability with the available financial data for the cited company (Chelladurai and
Kerwin, 2017).
management of production according to demand of customers. This will provides the opportunity
regarding build quality products.
There are different process which helps in management of quality within the organisation
includes measures of probability, normal distribution, inference. The different tools are defined
below:
Measures of probability: This will includes application of the methods of probability
which improves the decision making of management.
There are different methods which helps in calculation of different kind of data includes
average earnings, measuring of variability, times etc. It has been necessary for an organisation to
analyses all specific distribution that is applicable for an organisation to manage and analyse
their future profitability with the available financial data for the cited company (Chelladurai and
Kerwin, 2017).
⊘ This is a preview!⊘
Do you want full access?
Subscribe today to unlock all pages.

Trusted by 1+ million students worldwide
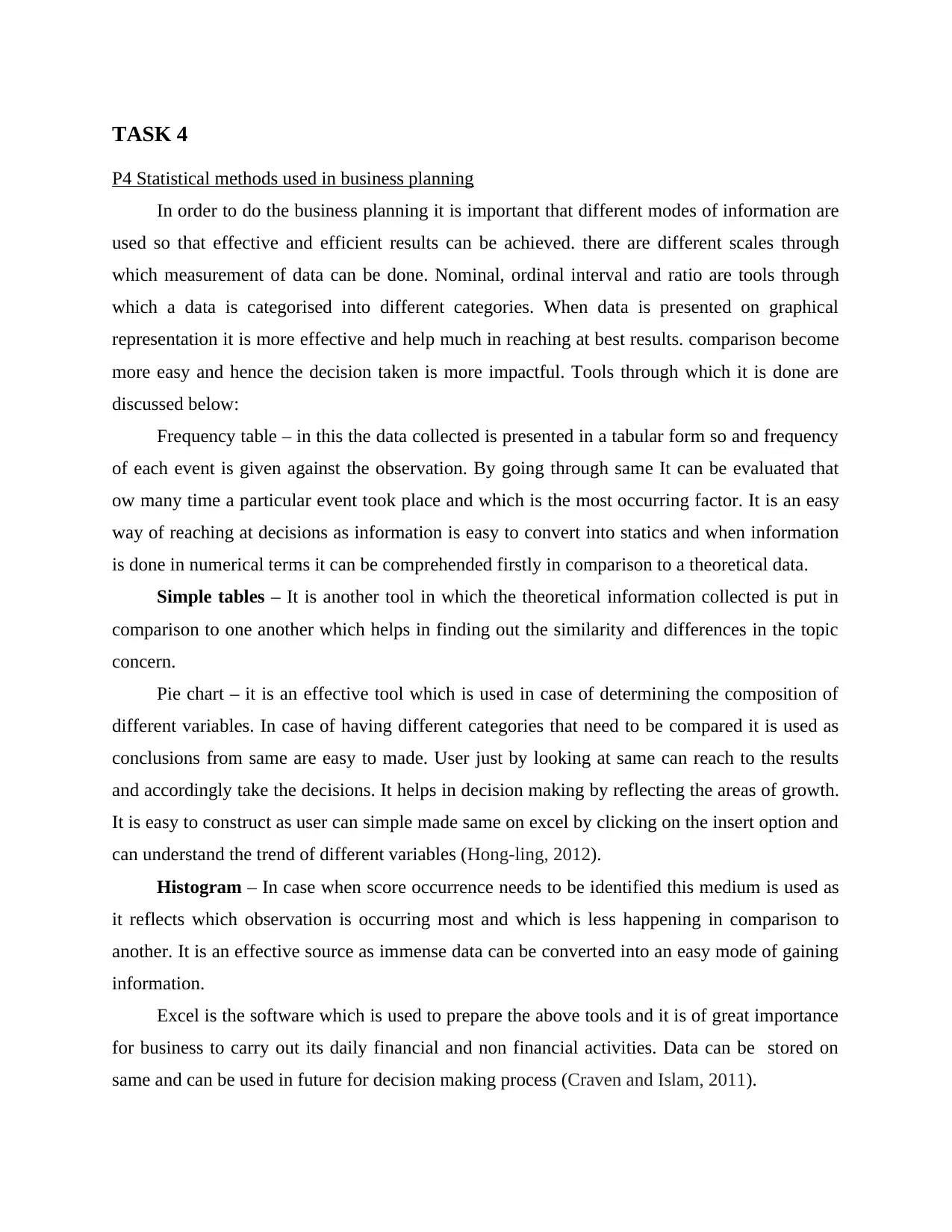
TASK 4
P4 Statistical methods used in business planning
In order to do the business planning it is important that different modes of information are
used so that effective and efficient results can be achieved. there are different scales through
which measurement of data can be done. Nominal, ordinal interval and ratio are tools through
which a data is categorised into different categories. When data is presented on graphical
representation it is more effective and help much in reaching at best results. comparison become
more easy and hence the decision taken is more impactful. Tools through which it is done are
discussed below:
Frequency table – in this the data collected is presented in a tabular form so and frequency
of each event is given against the observation. By going through same It can be evaluated that
ow many time a particular event took place and which is the most occurring factor. It is an easy
way of reaching at decisions as information is easy to convert into statics and when information
is done in numerical terms it can be comprehended firstly in comparison to a theoretical data.
Simple tables – It is another tool in which the theoretical information collected is put in
comparison to one another which helps in finding out the similarity and differences in the topic
concern.
Pie chart – it is an effective tool which is used in case of determining the composition of
different variables. In case of having different categories that need to be compared it is used as
conclusions from same are easy to made. User just by looking at same can reach to the results
and accordingly take the decisions. It helps in decision making by reflecting the areas of growth.
It is easy to construct as user can simple made same on excel by clicking on the insert option and
can understand the trend of different variables (Hong-ling, 2012).
Histogram – In case when score occurrence needs to be identified this medium is used as
it reflects which observation is occurring most and which is less happening in comparison to
another. It is an effective source as immense data can be converted into an easy mode of gaining
information.
Excel is the software which is used to prepare the above tools and it is of great importance
for business to carry out its daily financial and non financial activities. Data can be stored on
same and can be used in future for decision making process (Craven and Islam, 2011).
P4 Statistical methods used in business planning
In order to do the business planning it is important that different modes of information are
used so that effective and efficient results can be achieved. there are different scales through
which measurement of data can be done. Nominal, ordinal interval and ratio are tools through
which a data is categorised into different categories. When data is presented on graphical
representation it is more effective and help much in reaching at best results. comparison become
more easy and hence the decision taken is more impactful. Tools through which it is done are
discussed below:
Frequency table – in this the data collected is presented in a tabular form so and frequency
of each event is given against the observation. By going through same It can be evaluated that
ow many time a particular event took place and which is the most occurring factor. It is an easy
way of reaching at decisions as information is easy to convert into statics and when information
is done in numerical terms it can be comprehended firstly in comparison to a theoretical data.
Simple tables – It is another tool in which the theoretical information collected is put in
comparison to one another which helps in finding out the similarity and differences in the topic
concern.
Pie chart – it is an effective tool which is used in case of determining the composition of
different variables. In case of having different categories that need to be compared it is used as
conclusions from same are easy to made. User just by looking at same can reach to the results
and accordingly take the decisions. It helps in decision making by reflecting the areas of growth.
It is easy to construct as user can simple made same on excel by clicking on the insert option and
can understand the trend of different variables (Hong-ling, 2012).
Histogram – In case when score occurrence needs to be identified this medium is used as
it reflects which observation is occurring most and which is less happening in comparison to
another. It is an effective source as immense data can be converted into an easy mode of gaining
information.
Excel is the software which is used to prepare the above tools and it is of great importance
for business to carry out its daily financial and non financial activities. Data can be stored on
same and can be used in future for decision making process (Craven and Islam, 2011).
Paraphrase This Document
Need a fresh take? Get an instant paraphrase of this document with our AI Paraphraser
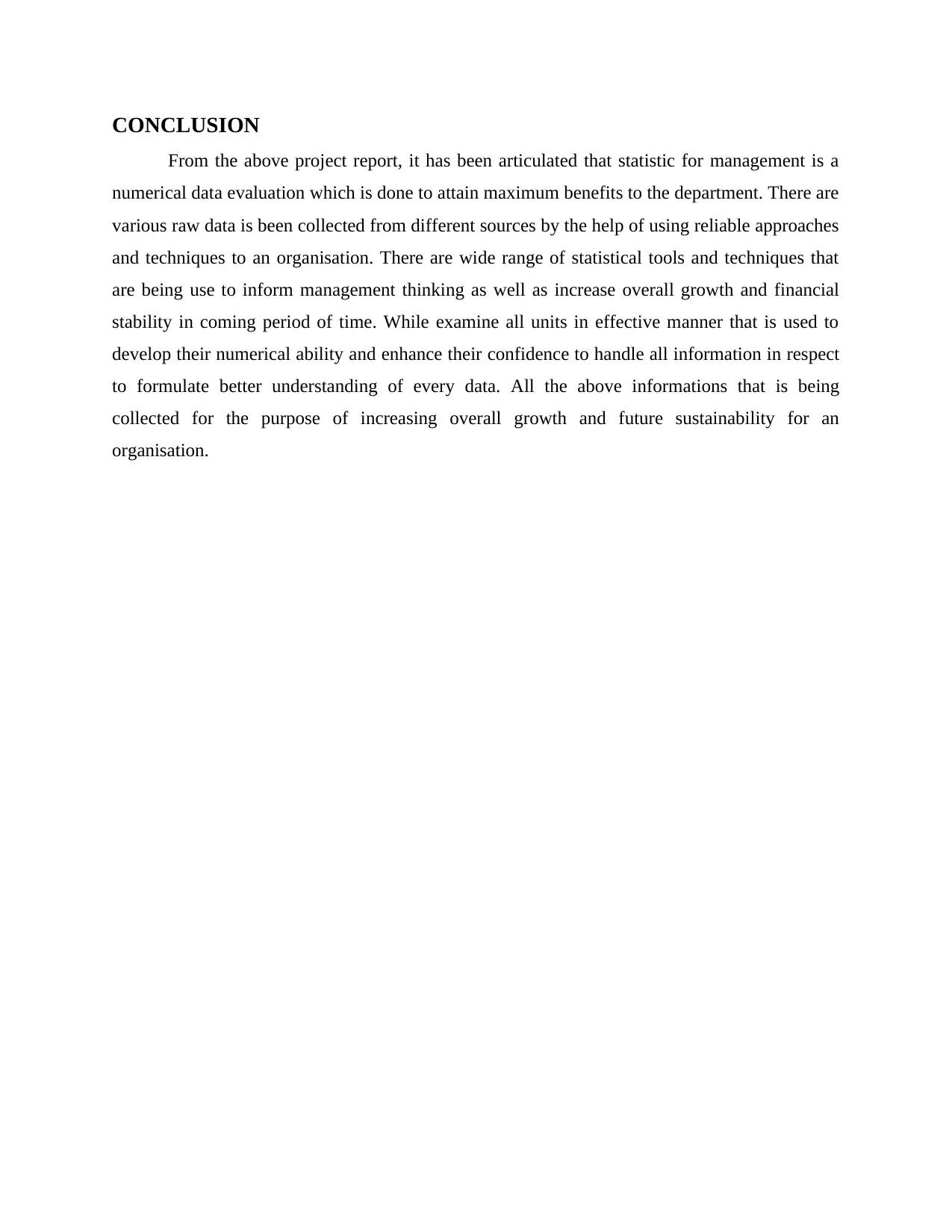
CONCLUSION
From the above project report, it has been articulated that statistic for management is a
numerical data evaluation which is done to attain maximum benefits to the department. There are
various raw data is been collected from different sources by the help of using reliable approaches
and techniques to an organisation. There are wide range of statistical tools and techniques that
are being use to inform management thinking as well as increase overall growth and financial
stability in coming period of time. While examine all units in effective manner that is used to
develop their numerical ability and enhance their confidence to handle all information in respect
to formulate better understanding of every data. All the above informations that is being
collected for the purpose of increasing overall growth and future sustainability for an
organisation.
From the above project report, it has been articulated that statistic for management is a
numerical data evaluation which is done to attain maximum benefits to the department. There are
various raw data is been collected from different sources by the help of using reliable approaches
and techniques to an organisation. There are wide range of statistical tools and techniques that
are being use to inform management thinking as well as increase overall growth and financial
stability in coming period of time. While examine all units in effective manner that is used to
develop their numerical ability and enhance their confidence to handle all information in respect
to formulate better understanding of every data. All the above informations that is being
collected for the purpose of increasing overall growth and future sustainability for an
organisation.
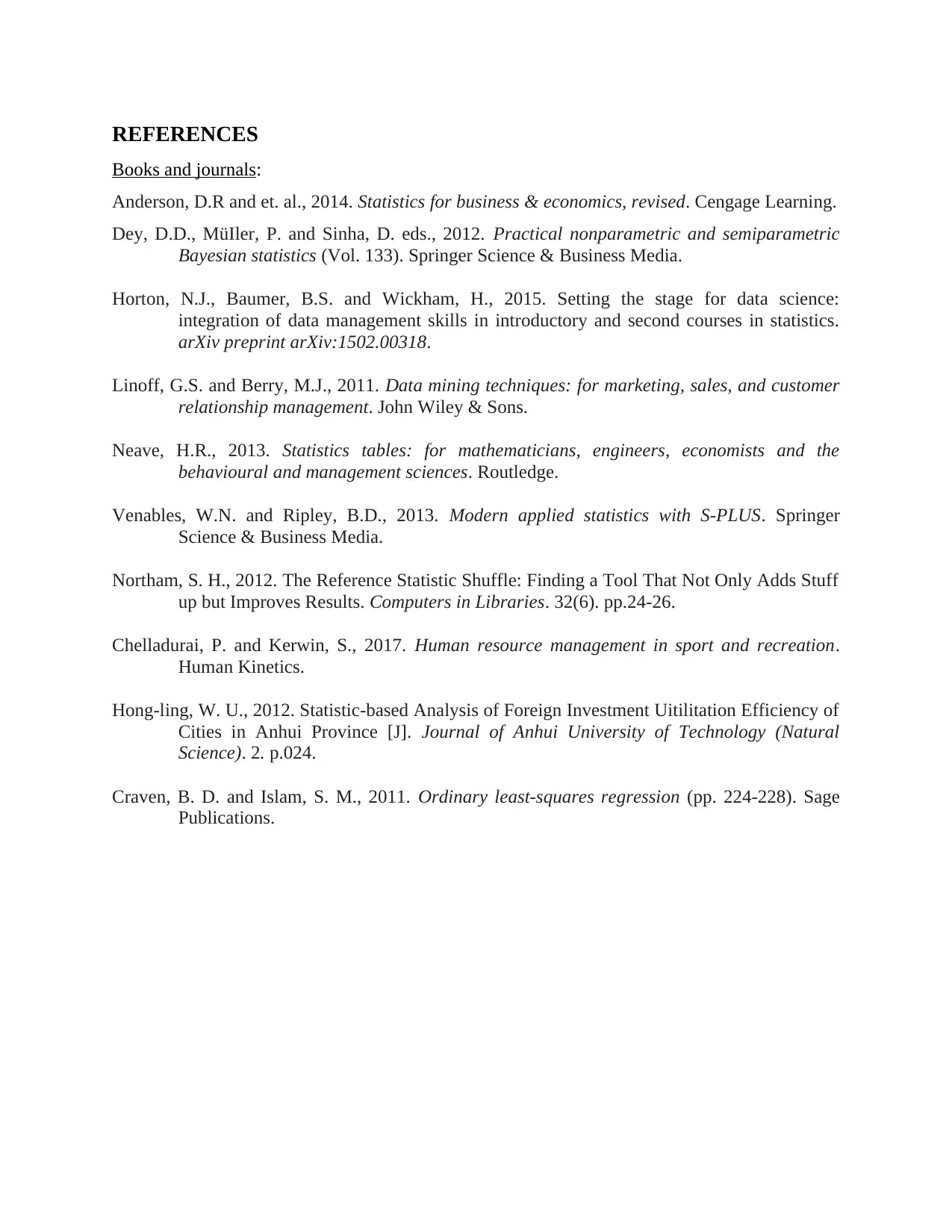
REFERENCES
Books and journals:
Anderson, D.R and et. al., 2014. Statistics for business & economics, revised. Cengage Learning.
Dey, D.D., MüIler, P. and Sinha, D. eds., 2012. Practical nonparametric and semiparametric
Bayesian statistics (Vol. 133). Springer Science & Business Media.
Horton, N.J., Baumer, B.S. and Wickham, H., 2015. Setting the stage for data science:
integration of data management skills in introductory and second courses in statistics.
arXiv preprint arXiv:1502.00318.
Linoff, G.S. and Berry, M.J., 2011. Data mining techniques: for marketing, sales, and customer
relationship management. John Wiley & Sons.
Neave, H.R., 2013. Statistics tables: for mathematicians, engineers, economists and the
behavioural and management sciences. Routledge.
Venables, W.N. and Ripley, B.D., 2013. Modern applied statistics with S-PLUS. Springer
Science & Business Media.
Northam, S. H., 2012. The Reference Statistic Shuffle: Finding a Tool That Not Only Adds Stuff
up but Improves Results. Computers in Libraries. 32(6). pp.24-26.
Chelladurai, P. and Kerwin, S., 2017. Human resource management in sport and recreation.
Human Kinetics.
Hong-ling, W. U., 2012. Statistic-based Analysis of Foreign Investment Uitilitation Efficiency of
Cities in Anhui Province [J]. Journal of Anhui University of Technology (Natural
Science). 2. p.024.
Craven, B. D. and Islam, S. M., 2011. Ordinary least-squares regression (pp. 224-228). Sage
Publications.
Books and journals:
Anderson, D.R and et. al., 2014. Statistics for business & economics, revised. Cengage Learning.
Dey, D.D., MüIler, P. and Sinha, D. eds., 2012. Practical nonparametric and semiparametric
Bayesian statistics (Vol. 133). Springer Science & Business Media.
Horton, N.J., Baumer, B.S. and Wickham, H., 2015. Setting the stage for data science:
integration of data management skills in introductory and second courses in statistics.
arXiv preprint arXiv:1502.00318.
Linoff, G.S. and Berry, M.J., 2011. Data mining techniques: for marketing, sales, and customer
relationship management. John Wiley & Sons.
Neave, H.R., 2013. Statistics tables: for mathematicians, engineers, economists and the
behavioural and management sciences. Routledge.
Venables, W.N. and Ripley, B.D., 2013. Modern applied statistics with S-PLUS. Springer
Science & Business Media.
Northam, S. H., 2012. The Reference Statistic Shuffle: Finding a Tool That Not Only Adds Stuff
up but Improves Results. Computers in Libraries. 32(6). pp.24-26.
Chelladurai, P. and Kerwin, S., 2017. Human resource management in sport and recreation.
Human Kinetics.
Hong-ling, W. U., 2012. Statistic-based Analysis of Foreign Investment Uitilitation Efficiency of
Cities in Anhui Province [J]. Journal of Anhui University of Technology (Natural
Science). 2. p.024.
Craven, B. D. and Islam, S. M., 2011. Ordinary least-squares regression (pp. 224-228). Sage
Publications.
⊘ This is a preview!⊘
Do you want full access?
Subscribe today to unlock all pages.

Trusted by 1+ million students worldwide
1 out of 12
Related Documents

Your All-in-One AI-Powered Toolkit for Academic Success.
+13062052269
info@desklib.com
Available 24*7 on WhatsApp / Email
Unlock your academic potential
© 2024 | Zucol Services PVT LTD | All rights reserved.