Stock Market Linkages: Evidence From the US, China and India During the Subprime Crisis
VerifiedAdded on 2023/04/21
|26
|12693
|268
AI Summary
This study analyzes the stock market linkages between the US, India and China during the subprime crisis. It examines the return and volatility spillovers and the causality between the markets. The findings suggest a unidirectional causality from the US market to the Indian and Chinese markets, as well as a volatility spillover from the US to the Indian market and from the Indian to the Chinese market. The study highlights the importance of considering these linkages for portfolio diversification strategies.
Contribute Materials
Your contribution can guide someone’s learning journey. Share your
documents today.
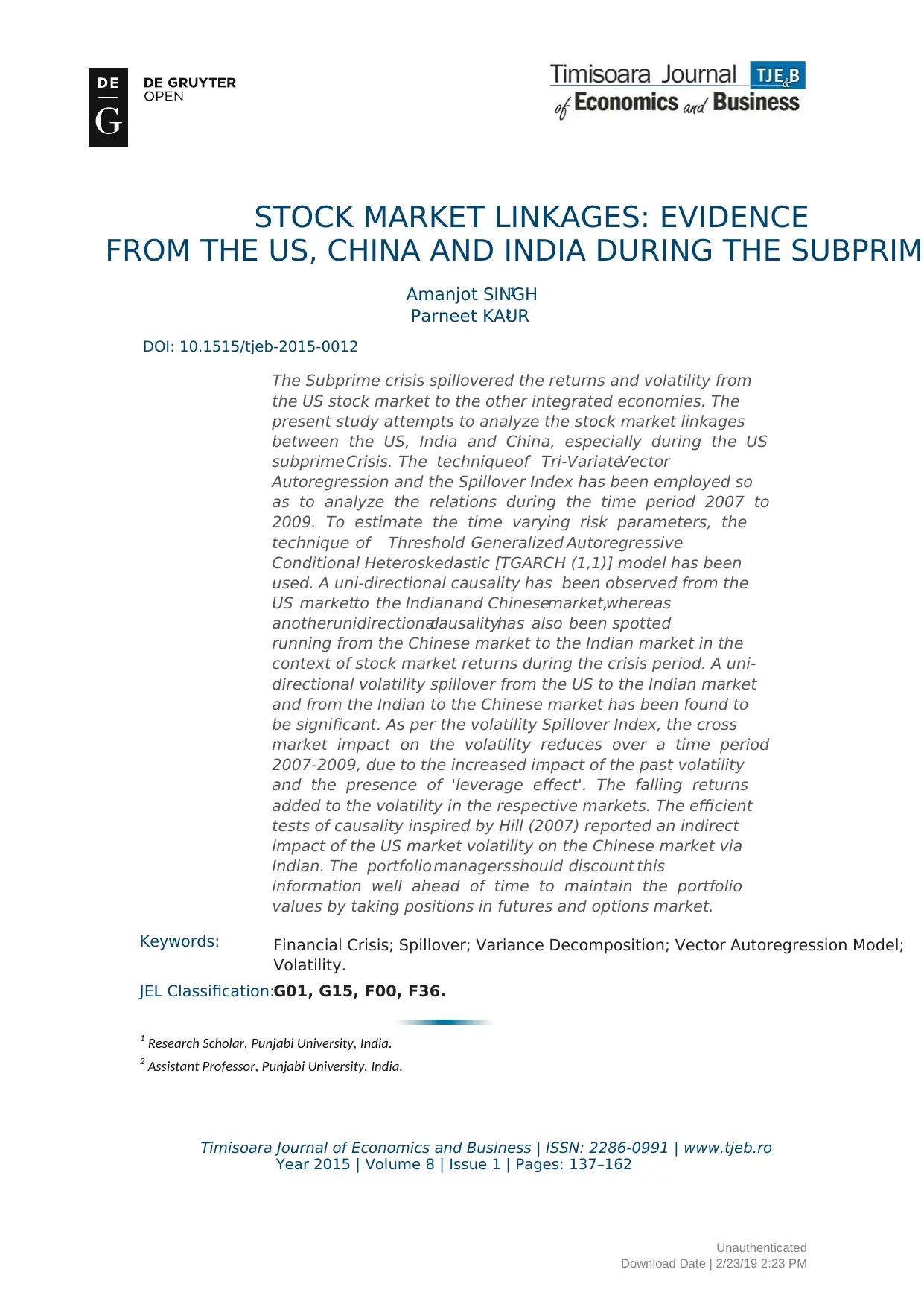
Timisoara Journal of Economics and Business | ISSN: 2286-0991 | www.tjeb.ro
Year 2015 | Volume 8 | Issue 1 | Pages: 137–162
STOCK MARKET LINKAGES: EVIDENCE
FROM THE US, CHINA AND INDIA DURING THE SUBPRIME
Amanjot SINGH1
Parneet KAUR2
DOI: 10.1515/tjeb-2015-0012
The Subprime crisis spillovered the returns and volatility from
the US stock market to the other integrated economies. The
present study attempts to analyze the stock market linkages
between the US, India and China, especially during the US
subprime Crisis. The techniqueof Tri-VariateVector
Autoregression and the Spillover Index has been employed so
as to analyze the relations during the time period 2007 to
2009. To estimate the time varying risk parameters, the
technique of Threshold Generalized Autoregressive
Conditional Heteroskedastic [TGARCH (1,1)] model has been
used. A uni-directional causality has been observed from the
US marketto the Indian and Chinesemarket,whereas
anotherunidirectionalcausalityhas also been spotted
running from the Chinese market to the Indian market in the
context of stock market returns during the crisis period. A uni-
directional volatility spillover from the US to the Indian market
and from the Indian to the Chinese market has been found to
be significant. As per the volatility Spillover Index, the cross
market impact on the volatility reduces over a time period
2007-2009, due to the increased impact of the past volatility
and the presence of 'leverage effect'. The falling returns
added to the volatility in the respective markets. The efficient
tests of causality inspired by Hill (2007) reported an indirect
impact of the US market volatility on the Chinese market via
Indian. The portfolio managersshould discount this
information well ahead of time to maintain the portfolio
values by taking positions in futures and options market.
Keywords: Financial Crisis; Spillover; Variance Decomposition; Vector Autoregression Model;
Volatility.
JEL Classification:G01, G15, F00, F36.
1 Research Scholar, Punjabi University, India.
2 Assistant Professor, Punjabi University, India.
Unauthenticated
Download Date | 2/23/19 2:23 PM
Year 2015 | Volume 8 | Issue 1 | Pages: 137–162
STOCK MARKET LINKAGES: EVIDENCE
FROM THE US, CHINA AND INDIA DURING THE SUBPRIME
Amanjot SINGH1
Parneet KAUR2
DOI: 10.1515/tjeb-2015-0012
The Subprime crisis spillovered the returns and volatility from
the US stock market to the other integrated economies. The
present study attempts to analyze the stock market linkages
between the US, India and China, especially during the US
subprime Crisis. The techniqueof Tri-VariateVector
Autoregression and the Spillover Index has been employed so
as to analyze the relations during the time period 2007 to
2009. To estimate the time varying risk parameters, the
technique of Threshold Generalized Autoregressive
Conditional Heteroskedastic [TGARCH (1,1)] model has been
used. A uni-directional causality has been observed from the
US marketto the Indian and Chinesemarket,whereas
anotherunidirectionalcausalityhas also been spotted
running from the Chinese market to the Indian market in the
context of stock market returns during the crisis period. A uni-
directional volatility spillover from the US to the Indian market
and from the Indian to the Chinese market has been found to
be significant. As per the volatility Spillover Index, the cross
market impact on the volatility reduces over a time period
2007-2009, due to the increased impact of the past volatility
and the presence of 'leverage effect'. The falling returns
added to the volatility in the respective markets. The efficient
tests of causality inspired by Hill (2007) reported an indirect
impact of the US market volatility on the Chinese market via
Indian. The portfolio managersshould discount this
information well ahead of time to maintain the portfolio
values by taking positions in futures and options market.
Keywords: Financial Crisis; Spillover; Variance Decomposition; Vector Autoregression Model;
Volatility.
JEL Classification:G01, G15, F00, F36.
1 Research Scholar, Punjabi University, India.
2 Assistant Professor, Punjabi University, India.
Unauthenticated
Download Date | 2/23/19 2:23 PM
Secure Best Marks with AI Grader
Need help grading? Try our AI Grader for instant feedback on your assignments.
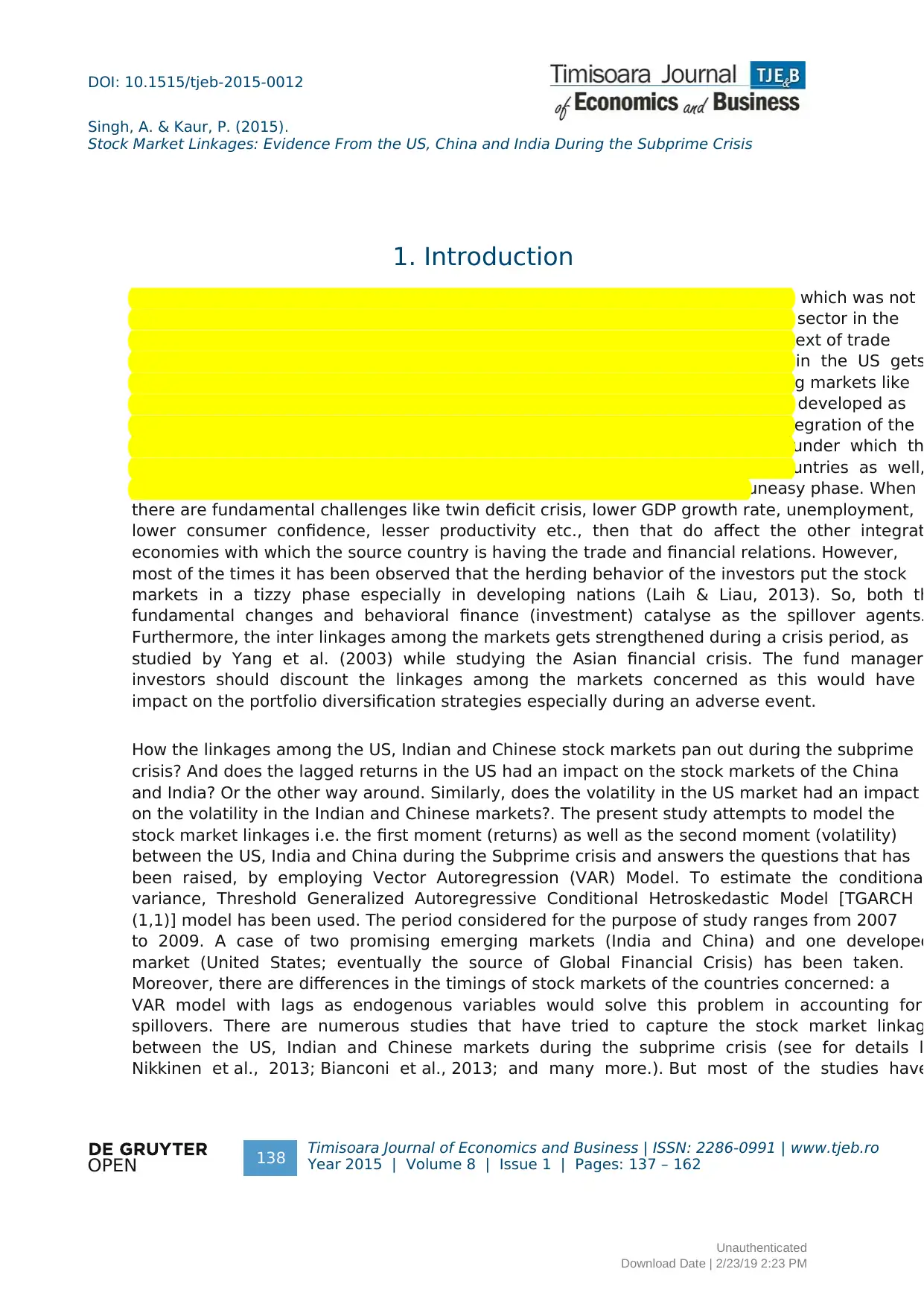
OPEN
DOI: 10.1515/tjeb-2015-0012
Singh, A. & Kaur, P. (2015).
Stock Market Linkages: Evidence From the US, China and India During the Subprime Crisis
Timisoara Journal of Economics and Business | ISSN: 2286-0991 | www.tjeb.ro
Year 2015 | Volume 8 | Issue 1 | Pages: 137 – 162138
1. Introduction
The bursting of the housing sector bubble triggered the subprime crisis in the US, which was not
limited only to the banking sector, but also had an impact on the overall financial sector in the
US. Moreover, being the dominant economy and increased integration in the context of trade
and financial channels with the other different countries, the crisis started in the US gets
transferred to the other countries as well, (Angkinand et al., 2009). Even emerging markets like
the BRIC nations felt the heat of the crisis due to their increasing exposure to the developed as
well as other nations (Beirne et al., 2009). The globalization and the increased integration of the
financial system have made the world economies into 'one single market' under which th
macroeconomic challenges in one country have an impact on the other countries as well,
making way for the stock markets in the recipient economies to witness an uneasy phase. When
there are fundamental challenges like twin deficit crisis, lower GDP growth rate, unemployment,
lower consumer confidence, lesser productivity etc., then that do affect the other integrat
economies with which the source country is having the trade and financial relations. However,
most of the times it has been observed that the herding behavior of the investors put the stock
markets in a tizzy phase especially in developing nations (Laih & Liau, 2013). So, both th
fundamental changes and behavioral finance (investment) catalyse as the spillover agents.
Furthermore, the inter linkages among the markets gets strengthened during a crisis period, as
studied by Yang et al. (2003) while studying the Asian financial crisis. The fund managers
investors should discount the linkages among the markets concerned as this would have
impact on the portfolio diversification strategies especially during an adverse event.
How the linkages among the US, Indian and Chinese stock markets pan out during the subprime
crisis? And does the lagged returns in the US had an impact on the stock markets of the China
and India? Or the other way around. Similarly, does the volatility in the US market had an impact
on the volatility in the Indian and Chinese markets?. The present study attempts to model the
stock market linkages i.e. the first moment (returns) as well as the second moment (volatility)
between the US, India and China during the Subprime crisis and answers the questions that has
been raised, by employing Vector Autoregression (VAR) Model. To estimate the conditional
variance, Threshold Generalized Autoregressive Conditional Hetroskedastic Model [TGARCH
(1,1)] model has been used. The period considered for the purpose of study ranges from 2007
to 2009. A case of two promising emerging markets (India and China) and one developed
market (United States; eventually the source of Global Financial Crisis) has been taken.
Moreover, there are differences in the timings of stock markets of the countries concerned: a
VAR model with lags as endogenous variables would solve this problem in accounting for
spillovers. There are numerous studies that have tried to capture the stock market linkag
between the US, Indian and Chinese markets during the subprime crisis (see for details l
Nikkinen et al., 2013; Bianconi et al., 2013; and many more.). But most of the studies have
Unauthenticated
Download Date | 2/23/19 2:23 PM
DOI: 10.1515/tjeb-2015-0012
Singh, A. & Kaur, P. (2015).
Stock Market Linkages: Evidence From the US, China and India During the Subprime Crisis
Timisoara Journal of Economics and Business | ISSN: 2286-0991 | www.tjeb.ro
Year 2015 | Volume 8 | Issue 1 | Pages: 137 – 162138
1. Introduction
The bursting of the housing sector bubble triggered the subprime crisis in the US, which was not
limited only to the banking sector, but also had an impact on the overall financial sector in the
US. Moreover, being the dominant economy and increased integration in the context of trade
and financial channels with the other different countries, the crisis started in the US gets
transferred to the other countries as well, (Angkinand et al., 2009). Even emerging markets like
the BRIC nations felt the heat of the crisis due to their increasing exposure to the developed as
well as other nations (Beirne et al., 2009). The globalization and the increased integration of the
financial system have made the world economies into 'one single market' under which th
macroeconomic challenges in one country have an impact on the other countries as well,
making way for the stock markets in the recipient economies to witness an uneasy phase. When
there are fundamental challenges like twin deficit crisis, lower GDP growth rate, unemployment,
lower consumer confidence, lesser productivity etc., then that do affect the other integrat
economies with which the source country is having the trade and financial relations. However,
most of the times it has been observed that the herding behavior of the investors put the stock
markets in a tizzy phase especially in developing nations (Laih & Liau, 2013). So, both th
fundamental changes and behavioral finance (investment) catalyse as the spillover agents.
Furthermore, the inter linkages among the markets gets strengthened during a crisis period, as
studied by Yang et al. (2003) while studying the Asian financial crisis. The fund managers
investors should discount the linkages among the markets concerned as this would have
impact on the portfolio diversification strategies especially during an adverse event.
How the linkages among the US, Indian and Chinese stock markets pan out during the subprime
crisis? And does the lagged returns in the US had an impact on the stock markets of the China
and India? Or the other way around. Similarly, does the volatility in the US market had an impact
on the volatility in the Indian and Chinese markets?. The present study attempts to model the
stock market linkages i.e. the first moment (returns) as well as the second moment (volatility)
between the US, India and China during the Subprime crisis and answers the questions that has
been raised, by employing Vector Autoregression (VAR) Model. To estimate the conditional
variance, Threshold Generalized Autoregressive Conditional Hetroskedastic Model [TGARCH
(1,1)] model has been used. The period considered for the purpose of study ranges from 2007
to 2009. A case of two promising emerging markets (India and China) and one developed
market (United States; eventually the source of Global Financial Crisis) has been taken.
Moreover, there are differences in the timings of stock markets of the countries concerned: a
VAR model with lags as endogenous variables would solve this problem in accounting for
spillovers. There are numerous studies that have tried to capture the stock market linkag
between the US, Indian and Chinese markets during the subprime crisis (see for details l
Nikkinen et al., 2013; Bianconi et al., 2013; and many more.). But most of the studies have
Unauthenticated
Download Date | 2/23/19 2:23 PM
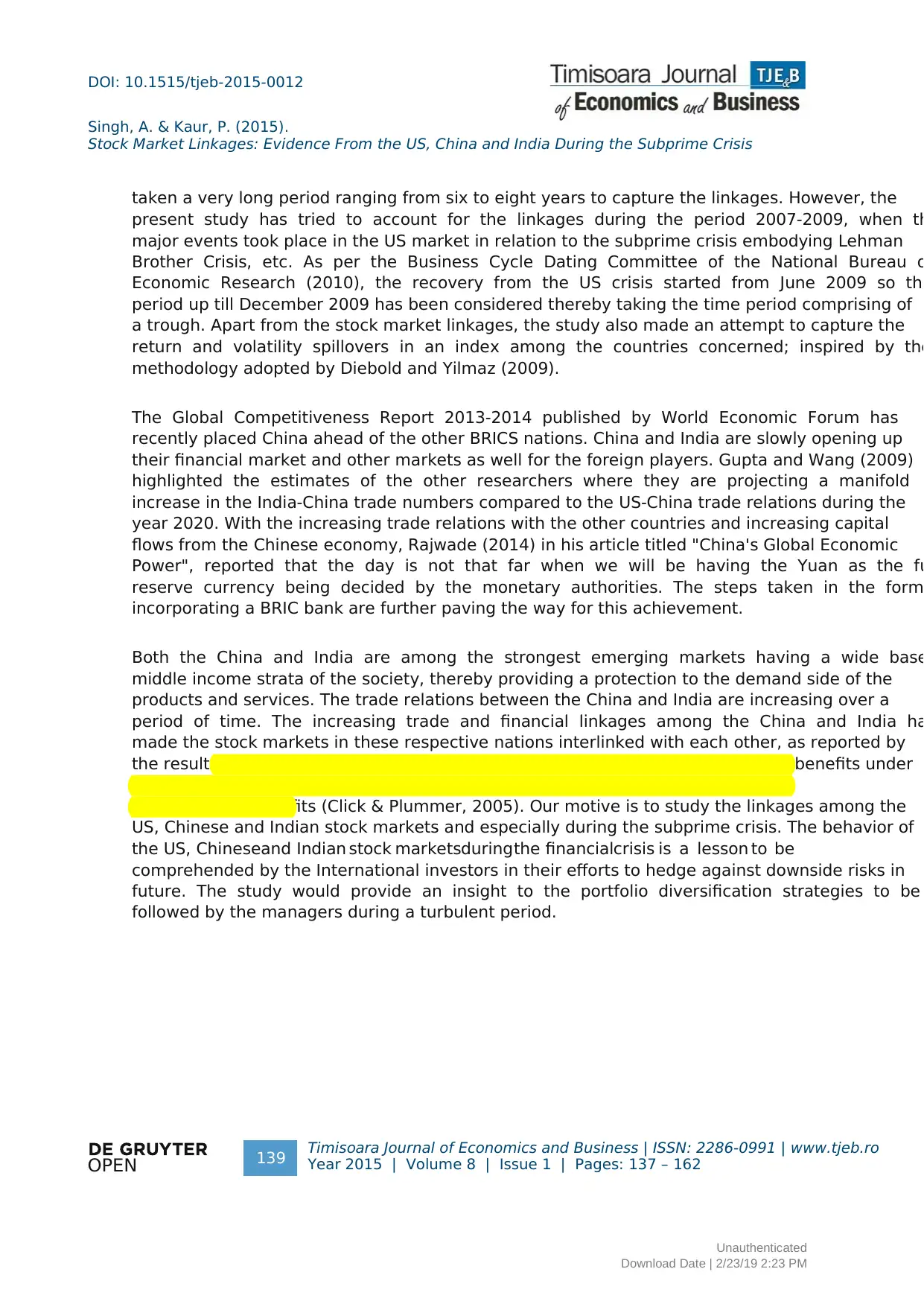
OPEN
DOI: 10.1515/tjeb-2015-0012
Singh, A. & Kaur, P. (2015).
Stock Market Linkages: Evidence From the US, China and India During the Subprime Crisis
Timisoara Journal of Economics and Business | ISSN: 2286-0991 | www.tjeb.ro
Year 2015 | Volume 8 | Issue 1 | Pages: 137 – 162139
taken a very long period ranging from six to eight years to capture the linkages. However, the
present study has tried to account for the linkages during the period 2007-2009, when th
major events took place in the US market in relation to the subprime crisis embodying Lehman
Brother Crisis, etc. As per the Business Cycle Dating Committee of the National Bureau o
Economic Research (2010), the recovery from the US crisis started from June 2009 so the
period up till December 2009 has been considered thereby taking the time period comprising of
a trough. Apart from the stock market linkages, the study also made an attempt to capture the
return and volatility spillovers in an index among the countries concerned; inspired by the
methodology adopted by Diebold and Yilmaz (2009).
The Global Competitiveness Report 2013-2014 published by World Economic Forum has
recently placed China ahead of the other BRICS nations. China and India are slowly opening up
their financial market and other markets as well for the foreign players. Gupta and Wang (2009)
highlighted the estimates of the other researchers where they are projecting a manifold
increase in the India-China trade numbers compared to the US-China trade relations during the
year 2020. With the increasing trade relations with the other countries and increasing capital
flows from the Chinese economy, Rajwade (2014) in his article titled "China's Global Economic
Power", reported that the day is not that far when we will be having the Yuan as the fu
reserve currency being decided by the monetary authorities. The steps taken in the form
incorporating a BRIC bank are further paving the way for this achievement.
Both the China and India are among the strongest emerging markets having a wide base
middle income strata of the society, thereby providing a protection to the demand side of the
products and services. The trade relations between the China and India are increasing over a
period of time. The increasing trade and financial linkages among the China and India ha
made the stock markets in these respective nations interlinked with each other, as reported by
the results. These linkages further have an impact on the portfolio diversification benefits under
which the correlationbetweenthe asset classes should be lower in order to enjoy the
diversification benefits (Click & Plummer, 2005). Our motive is to study the linkages among the
US, Chinese and Indian stock markets and especially during the subprime crisis. The behavior of
the US, Chineseand Indian stock marketsduring the financialcrisis is a lesson to be
comprehended by the International investors in their efforts to hedge against downside risks in
future. The study would provide an insight to the portfolio diversification strategies to be
followed by the managers during a turbulent period.
Unauthenticated
Download Date | 2/23/19 2:23 PM
DOI: 10.1515/tjeb-2015-0012
Singh, A. & Kaur, P. (2015).
Stock Market Linkages: Evidence From the US, China and India During the Subprime Crisis
Timisoara Journal of Economics and Business | ISSN: 2286-0991 | www.tjeb.ro
Year 2015 | Volume 8 | Issue 1 | Pages: 137 – 162139
taken a very long period ranging from six to eight years to capture the linkages. However, the
present study has tried to account for the linkages during the period 2007-2009, when th
major events took place in the US market in relation to the subprime crisis embodying Lehman
Brother Crisis, etc. As per the Business Cycle Dating Committee of the National Bureau o
Economic Research (2010), the recovery from the US crisis started from June 2009 so the
period up till December 2009 has been considered thereby taking the time period comprising of
a trough. Apart from the stock market linkages, the study also made an attempt to capture the
return and volatility spillovers in an index among the countries concerned; inspired by the
methodology adopted by Diebold and Yilmaz (2009).
The Global Competitiveness Report 2013-2014 published by World Economic Forum has
recently placed China ahead of the other BRICS nations. China and India are slowly opening up
their financial market and other markets as well for the foreign players. Gupta and Wang (2009)
highlighted the estimates of the other researchers where they are projecting a manifold
increase in the India-China trade numbers compared to the US-China trade relations during the
year 2020. With the increasing trade relations with the other countries and increasing capital
flows from the Chinese economy, Rajwade (2014) in his article titled "China's Global Economic
Power", reported that the day is not that far when we will be having the Yuan as the fu
reserve currency being decided by the monetary authorities. The steps taken in the form
incorporating a BRIC bank are further paving the way for this achievement.
Both the China and India are among the strongest emerging markets having a wide base
middle income strata of the society, thereby providing a protection to the demand side of the
products and services. The trade relations between the China and India are increasing over a
period of time. The increasing trade and financial linkages among the China and India ha
made the stock markets in these respective nations interlinked with each other, as reported by
the results. These linkages further have an impact on the portfolio diversification benefits under
which the correlationbetweenthe asset classes should be lower in order to enjoy the
diversification benefits (Click & Plummer, 2005). Our motive is to study the linkages among the
US, Chinese and Indian stock markets and especially during the subprime crisis. The behavior of
the US, Chineseand Indian stock marketsduring the financialcrisis is a lesson to be
comprehended by the International investors in their efforts to hedge against downside risks in
future. The study would provide an insight to the portfolio diversification strategies to be
followed by the managers during a turbulent period.
Unauthenticated
Download Date | 2/23/19 2:23 PM
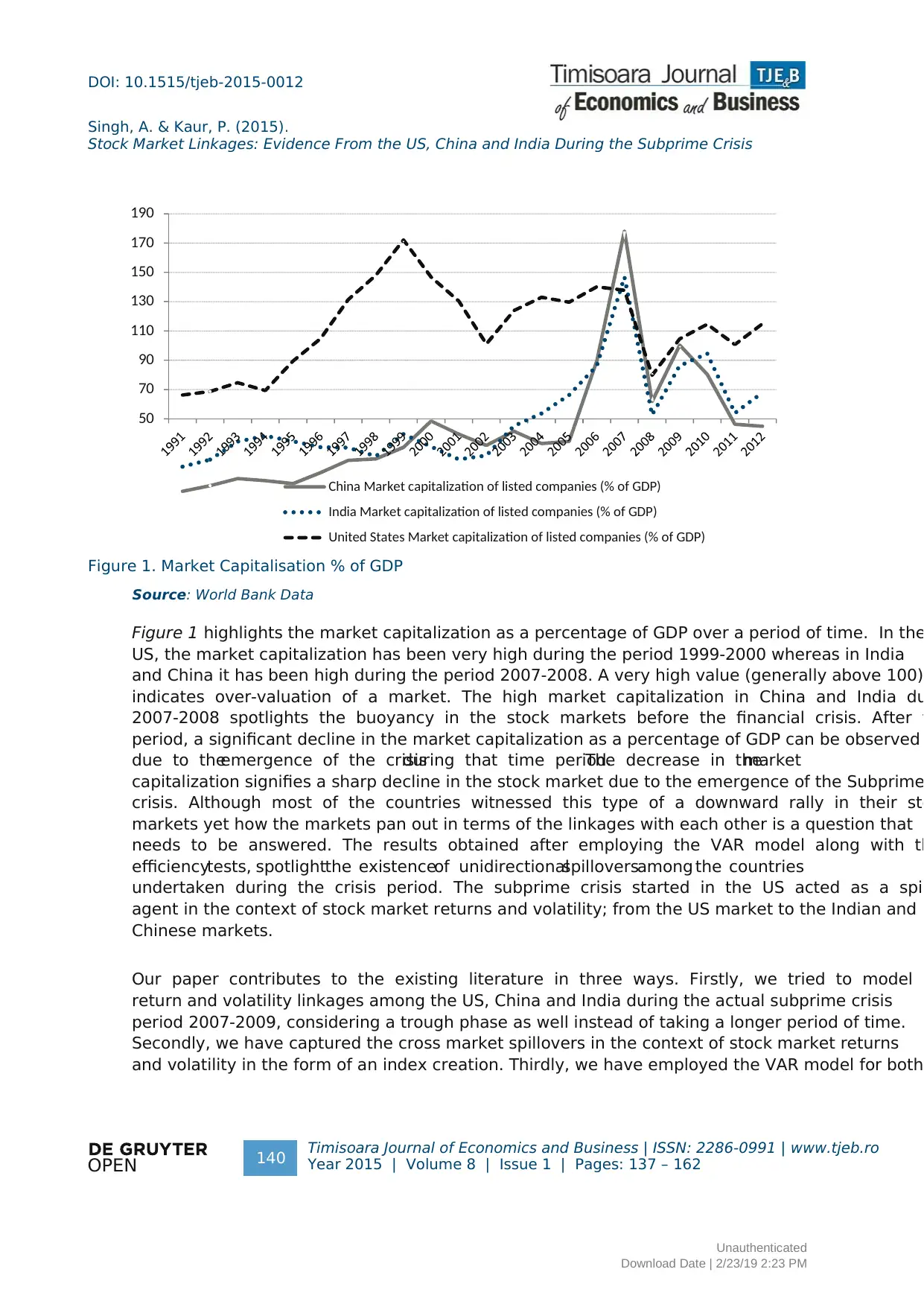
OPEN
DOI: 10.1515/tjeb-2015-0012
Singh, A. & Kaur, P. (2015).
Stock Market Linkages: Evidence From the US, China and India During the Subprime Crisis
Timisoara Journal of Economics and Business | ISSN: 2286-0991 | www.tjeb.ro
Year 2015 | Volume 8 | Issue 1 | Pages: 137 – 162140
Figure 1. Market Capitalisation % of GDP
Source: World Bank Data
Figure 1 highlights the market capitalization as a percentage of GDP over a period of time. In the
US, the market capitalization has been very high during the period 1999-2000 whereas in India
and China it has been high during the period 2007-2008. A very high value (generally above 100)
indicates over-valuation of a market. The high market capitalization in China and India du
2007-2008 spotlights the buoyancy in the stock markets before the financial crisis. After t
period, a significant decline in the market capitalization as a percentage of GDP can be observed
due to theemergence of the crisisduring that time period.The decrease in themarket
capitalization signifies a sharp decline in the stock market due to the emergence of the Subprime
crisis. Although most of the countries witnessed this type of a downward rally in their sto
markets yet how the markets pan out in terms of the linkages with each other is a question that
needs to be answered. The results obtained after employing the VAR model along with th
efficiencytests, spotlightthe existenceof unidirectionalspilloversamong the countries
undertaken during the crisis period. The subprime crisis started in the US acted as a spil
agent in the context of stock market returns and volatility; from the US market to the Indian and
Chinese markets.
Our paper contributes to the existing literature in three ways. Firstly, we tried to model
return and volatility linkages among the US, China and India during the actual subprime crisis
period 2007-2009, considering a trough phase as well instead of taking a longer period of time.
Secondly, we have captured the cross market spillovers in the context of stock market returns
and volatility in the form of an index creation. Thirdly, we have employed the VAR model for both
50
70
90
110
130
150
170
190
China Market capitalization of listed companies (% of GDP)
India Market capitalization of listed companies (% of GDP)
United States Market capitalization of listed companies (% of GDP)
Unauthenticated
Download Date | 2/23/19 2:23 PM
DOI: 10.1515/tjeb-2015-0012
Singh, A. & Kaur, P. (2015).
Stock Market Linkages: Evidence From the US, China and India During the Subprime Crisis
Timisoara Journal of Economics and Business | ISSN: 2286-0991 | www.tjeb.ro
Year 2015 | Volume 8 | Issue 1 | Pages: 137 – 162140
Figure 1. Market Capitalisation % of GDP
Source: World Bank Data
Figure 1 highlights the market capitalization as a percentage of GDP over a period of time. In the
US, the market capitalization has been very high during the period 1999-2000 whereas in India
and China it has been high during the period 2007-2008. A very high value (generally above 100)
indicates over-valuation of a market. The high market capitalization in China and India du
2007-2008 spotlights the buoyancy in the stock markets before the financial crisis. After t
period, a significant decline in the market capitalization as a percentage of GDP can be observed
due to theemergence of the crisisduring that time period.The decrease in themarket
capitalization signifies a sharp decline in the stock market due to the emergence of the Subprime
crisis. Although most of the countries witnessed this type of a downward rally in their sto
markets yet how the markets pan out in terms of the linkages with each other is a question that
needs to be answered. The results obtained after employing the VAR model along with th
efficiencytests, spotlightthe existenceof unidirectionalspilloversamong the countries
undertaken during the crisis period. The subprime crisis started in the US acted as a spil
agent in the context of stock market returns and volatility; from the US market to the Indian and
Chinese markets.
Our paper contributes to the existing literature in three ways. Firstly, we tried to model
return and volatility linkages among the US, China and India during the actual subprime crisis
period 2007-2009, considering a trough phase as well instead of taking a longer period of time.
Secondly, we have captured the cross market spillovers in the context of stock market returns
and volatility in the form of an index creation. Thirdly, we have employed the VAR model for both
50
70
90
110
130
150
170
190
China Market capitalization of listed companies (% of GDP)
India Market capitalization of listed companies (% of GDP)
United States Market capitalization of listed companies (% of GDP)
Unauthenticated
Download Date | 2/23/19 2:23 PM
Paraphrase This Document
Need a fresh take? Get an instant paraphrase of this document with our AI Paraphraser
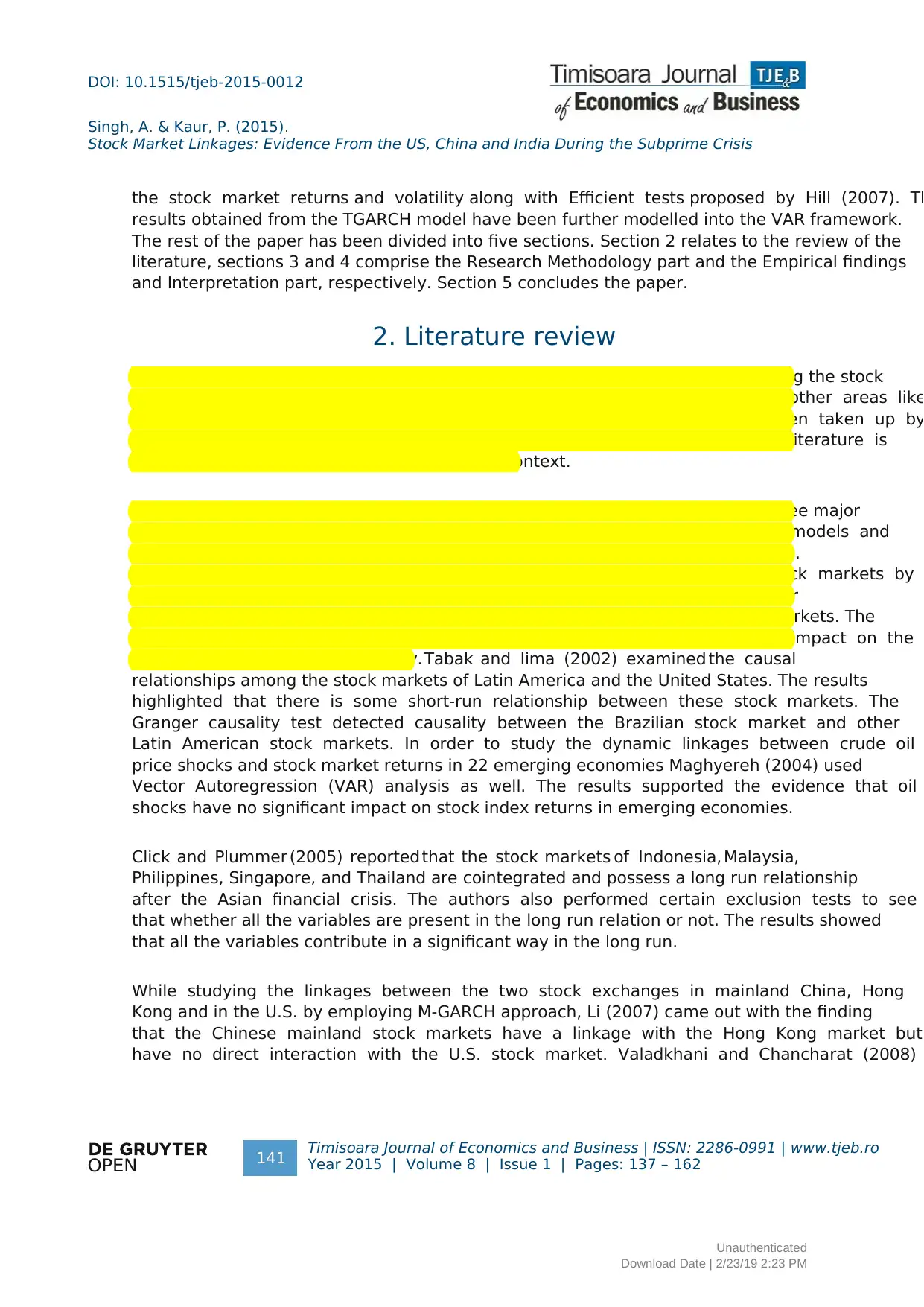
OPEN
DOI: 10.1515/tjeb-2015-0012
Singh, A. & Kaur, P. (2015).
Stock Market Linkages: Evidence From the US, China and India During the Subprime Crisis
Timisoara Journal of Economics and Business | ISSN: 2286-0991 | www.tjeb.ro
Year 2015 | Volume 8 | Issue 1 | Pages: 137 – 162141
the stock market returns and volatility along with Efficient tests proposed by Hill (2007). Th
results obtained from the TGARCH model have been further modelled into the VAR framework.
The rest of the paper has been divided into five sections. Section 2 relates to the review of the
literature, sections 3 and 4 comprise the Research Methodology part and the Empirical findings
and Interpretation part, respectively. Section 5 concludes the paper.
2. Literature review
Over a period of time, many researchers have tried to capture the linkages among the stock
markets. The studies are not limited to the stock markets alone, yet the other areas like
commodity markets, currency markets and the debt markets have also been taken up by
the researchers in order to account for the linkages among them. A rich literature is
available on the stock market linkages in this context.
Hamao et al. (1990) examined return spillovers and volatility spillovers in the three major
stock markets (New York, Tokyo, and London) by using univariate GARCH models and
reported significant volatility and return spillovers among the concerned countries.
Friedman and Shachmurove (1997) examined the European Community stock markets by
employinga Vector Autoregression(VAR) model. The results showed that the smaller
markets, such as Belgium, Denmark and Italy do not have an impact on other markets. The
impulse responses reported that Britain is a leading market that have an impact on the
France, Netherlands, and Germany. Tabak and lima (2002) examined the causal
relationships among the stock markets of Latin America and the United States. The results
highlighted that there is some short-run relationship between these stock markets. The
Granger causality test detected causality between the Brazilian stock market and other
Latin American stock markets. In order to study the dynamic linkages between crude oil
price shocks and stock market returns in 22 emerging economies Maghyereh (2004) used
Vector Autoregression (VAR) analysis as well. The results supported the evidence that oil
shocks have no significant impact on stock index returns in emerging economies.
Click and Plummer (2005) reported that the stock markets of Indonesia, Malaysia,
Philippines, Singapore, and Thailand are cointegrated and possess a long run relationship
after the Asian financial crisis. The authors also performed certain exclusion tests to see
that whether all the variables are present in the long run relation or not. The results showed
that all the variables contribute in a significant way in the long run.
While studying the linkages between the two stock exchanges in mainland China, Hong
Kong and in the U.S. by employing M-GARCH approach, Li (2007) came out with the finding
that the Chinese mainland stock markets have a linkage with the Hong Kong market but
have no direct interaction with the U.S. stock market. Valadkhani and Chancharat (2008)
Unauthenticated
Download Date | 2/23/19 2:23 PM
DOI: 10.1515/tjeb-2015-0012
Singh, A. & Kaur, P. (2015).
Stock Market Linkages: Evidence From the US, China and India During the Subprime Crisis
Timisoara Journal of Economics and Business | ISSN: 2286-0991 | www.tjeb.ro
Year 2015 | Volume 8 | Issue 1 | Pages: 137 – 162141
the stock market returns and volatility along with Efficient tests proposed by Hill (2007). Th
results obtained from the TGARCH model have been further modelled into the VAR framework.
The rest of the paper has been divided into five sections. Section 2 relates to the review of the
literature, sections 3 and 4 comprise the Research Methodology part and the Empirical findings
and Interpretation part, respectively. Section 5 concludes the paper.
2. Literature review
Over a period of time, many researchers have tried to capture the linkages among the stock
markets. The studies are not limited to the stock markets alone, yet the other areas like
commodity markets, currency markets and the debt markets have also been taken up by
the researchers in order to account for the linkages among them. A rich literature is
available on the stock market linkages in this context.
Hamao et al. (1990) examined return spillovers and volatility spillovers in the three major
stock markets (New York, Tokyo, and London) by using univariate GARCH models and
reported significant volatility and return spillovers among the concerned countries.
Friedman and Shachmurove (1997) examined the European Community stock markets by
employinga Vector Autoregression(VAR) model. The results showed that the smaller
markets, such as Belgium, Denmark and Italy do not have an impact on other markets. The
impulse responses reported that Britain is a leading market that have an impact on the
France, Netherlands, and Germany. Tabak and lima (2002) examined the causal
relationships among the stock markets of Latin America and the United States. The results
highlighted that there is some short-run relationship between these stock markets. The
Granger causality test detected causality between the Brazilian stock market and other
Latin American stock markets. In order to study the dynamic linkages between crude oil
price shocks and stock market returns in 22 emerging economies Maghyereh (2004) used
Vector Autoregression (VAR) analysis as well. The results supported the evidence that oil
shocks have no significant impact on stock index returns in emerging economies.
Click and Plummer (2005) reported that the stock markets of Indonesia, Malaysia,
Philippines, Singapore, and Thailand are cointegrated and possess a long run relationship
after the Asian financial crisis. The authors also performed certain exclusion tests to see
that whether all the variables are present in the long run relation or not. The results showed
that all the variables contribute in a significant way in the long run.
While studying the linkages between the two stock exchanges in mainland China, Hong
Kong and in the U.S. by employing M-GARCH approach, Li (2007) came out with the finding
that the Chinese mainland stock markets have a linkage with the Hong Kong market but
have no direct interaction with the U.S. stock market. Valadkhani and Chancharat (2008)
Unauthenticated
Download Date | 2/23/19 2:23 PM
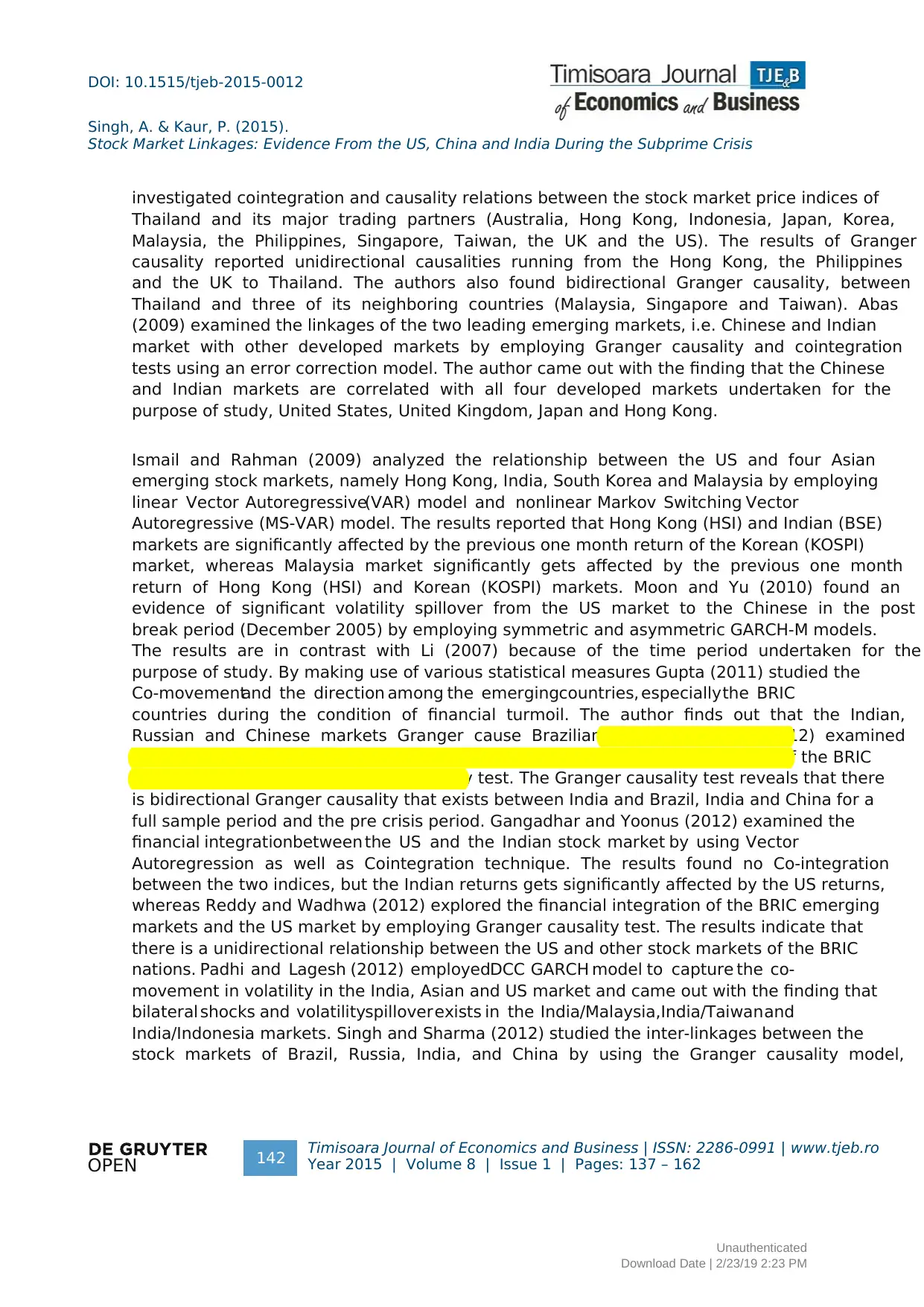
OPEN
DOI: 10.1515/tjeb-2015-0012
Singh, A. & Kaur, P. (2015).
Stock Market Linkages: Evidence From the US, China and India During the Subprime Crisis
Timisoara Journal of Economics and Business | ISSN: 2286-0991 | www.tjeb.ro
Year 2015 | Volume 8 | Issue 1 | Pages: 137 – 162142
investigated cointegration and causality relations between the stock market price indices of
Thailand and its major trading partners (Australia, Hong Kong, Indonesia, Japan, Korea,
Malaysia, the Philippines, Singapore, Taiwan, the UK and the US). The results of Granger
causality reported unidirectional causalities running from the Hong Kong, the Philippines
and the UK to Thailand. The authors also found bidirectional Granger causality, between
Thailand and three of its neighboring countries (Malaysia, Singapore and Taiwan). Abas
(2009) examined the linkages of the two leading emerging markets, i.e. Chinese and Indian
market with other developed markets by employing Granger causality and cointegration
tests using an error correction model. The author came out with the finding that the Chinese
and Indian markets are correlated with all four developed markets undertaken for the
purpose of study, United States, United Kingdom, Japan and Hong Kong.
Ismail and Rahman (2009) analyzed the relationship between the US and four Asian
emerging stock markets, namely Hong Kong, India, South Korea and Malaysia by employing
linear Vector Autoregressive(VAR) model and nonlinear Markov Switching Vector
Autoregressive (MS-VAR) model. The results reported that Hong Kong (HSI) and Indian (BSE)
markets are significantly affected by the previous one month return of the Korean (KOSPI)
market, whereas Malaysia market significantly gets affected by the previous one month
return of Hong Kong (HSI) and Korean (KOSPI) markets. Moon and Yu (2010) found an
evidence of significant volatility spillover from the US market to the Chinese in the post
break period (December 2005) by employing symmetric and asymmetric GARCH-M models.
The results are in contrast with Li (2007) because of the time period undertaken for the
purpose of study. By making use of various statistical measures Gupta (2011) studied the
Co-movementand the direction among the emergingcountries, especially the BRIC
countries during the condition of financial turmoil. The author finds out that the Indian,
Russian and Chinese markets Granger cause Brazilian market. Jeyanthi (2012) examined
both the long - run as well as short - run relationships between the stock prices of the BRIC
countries by employing Granger Causality test. The Granger causality test reveals that there
is bidirectional Granger causality that exists between India and Brazil, India and China for a
full sample period and the pre crisis period. Gangadhar and Yoonus (2012) examined the
financial integrationbetween the US and the Indian stock market by using Vector
Autoregression as well as Cointegration technique. The results found no Co-integration
between the two indices, but the Indian returns gets significantly affected by the US returns,
whereas Reddy and Wadhwa (2012) explored the financial integration of the BRIC emerging
markets and the US market by employing Granger causality test. The results indicate that
there is a unidirectional relationship between the US and other stock markets of the BRIC
nations. Padhi and Lagesh (2012) employedDCC GARCH model to capture the co-
movement in volatility in the India, Asian and US market and came out with the finding that
bilateral shocks and volatilityspillover exists in the India/Malaysia,India/Taiwan and
India/Indonesia markets. Singh and Sharma (2012) studied the inter-linkages between the
stock markets of Brazil, Russia, India, and China by using the Granger causality model,
Unauthenticated
Download Date | 2/23/19 2:23 PM
DOI: 10.1515/tjeb-2015-0012
Singh, A. & Kaur, P. (2015).
Stock Market Linkages: Evidence From the US, China and India During the Subprime Crisis
Timisoara Journal of Economics and Business | ISSN: 2286-0991 | www.tjeb.ro
Year 2015 | Volume 8 | Issue 1 | Pages: 137 – 162142
investigated cointegration and causality relations between the stock market price indices of
Thailand and its major trading partners (Australia, Hong Kong, Indonesia, Japan, Korea,
Malaysia, the Philippines, Singapore, Taiwan, the UK and the US). The results of Granger
causality reported unidirectional causalities running from the Hong Kong, the Philippines
and the UK to Thailand. The authors also found bidirectional Granger causality, between
Thailand and three of its neighboring countries (Malaysia, Singapore and Taiwan). Abas
(2009) examined the linkages of the two leading emerging markets, i.e. Chinese and Indian
market with other developed markets by employing Granger causality and cointegration
tests using an error correction model. The author came out with the finding that the Chinese
and Indian markets are correlated with all four developed markets undertaken for the
purpose of study, United States, United Kingdom, Japan and Hong Kong.
Ismail and Rahman (2009) analyzed the relationship between the US and four Asian
emerging stock markets, namely Hong Kong, India, South Korea and Malaysia by employing
linear Vector Autoregressive(VAR) model and nonlinear Markov Switching Vector
Autoregressive (MS-VAR) model. The results reported that Hong Kong (HSI) and Indian (BSE)
markets are significantly affected by the previous one month return of the Korean (KOSPI)
market, whereas Malaysia market significantly gets affected by the previous one month
return of Hong Kong (HSI) and Korean (KOSPI) markets. Moon and Yu (2010) found an
evidence of significant volatility spillover from the US market to the Chinese in the post
break period (December 2005) by employing symmetric and asymmetric GARCH-M models.
The results are in contrast with Li (2007) because of the time period undertaken for the
purpose of study. By making use of various statistical measures Gupta (2011) studied the
Co-movementand the direction among the emergingcountries, especially the BRIC
countries during the condition of financial turmoil. The author finds out that the Indian,
Russian and Chinese markets Granger cause Brazilian market. Jeyanthi (2012) examined
both the long - run as well as short - run relationships between the stock prices of the BRIC
countries by employing Granger Causality test. The Granger causality test reveals that there
is bidirectional Granger causality that exists between India and Brazil, India and China for a
full sample period and the pre crisis period. Gangadhar and Yoonus (2012) examined the
financial integrationbetween the US and the Indian stock market by using Vector
Autoregression as well as Cointegration technique. The results found no Co-integration
between the two indices, but the Indian returns gets significantly affected by the US returns,
whereas Reddy and Wadhwa (2012) explored the financial integration of the BRIC emerging
markets and the US market by employing Granger causality test. The results indicate that
there is a unidirectional relationship between the US and other stock markets of the BRIC
nations. Padhi and Lagesh (2012) employedDCC GARCH model to capture the co-
movement in volatility in the India, Asian and US market and came out with the finding that
bilateral shocks and volatilityspillover exists in the India/Malaysia,India/Taiwan and
India/Indonesia markets. Singh and Sharma (2012) studied the inter-linkages between the
stock markets of Brazil, Russia, India, and China by using the Granger causality model,
Unauthenticated
Download Date | 2/23/19 2:23 PM
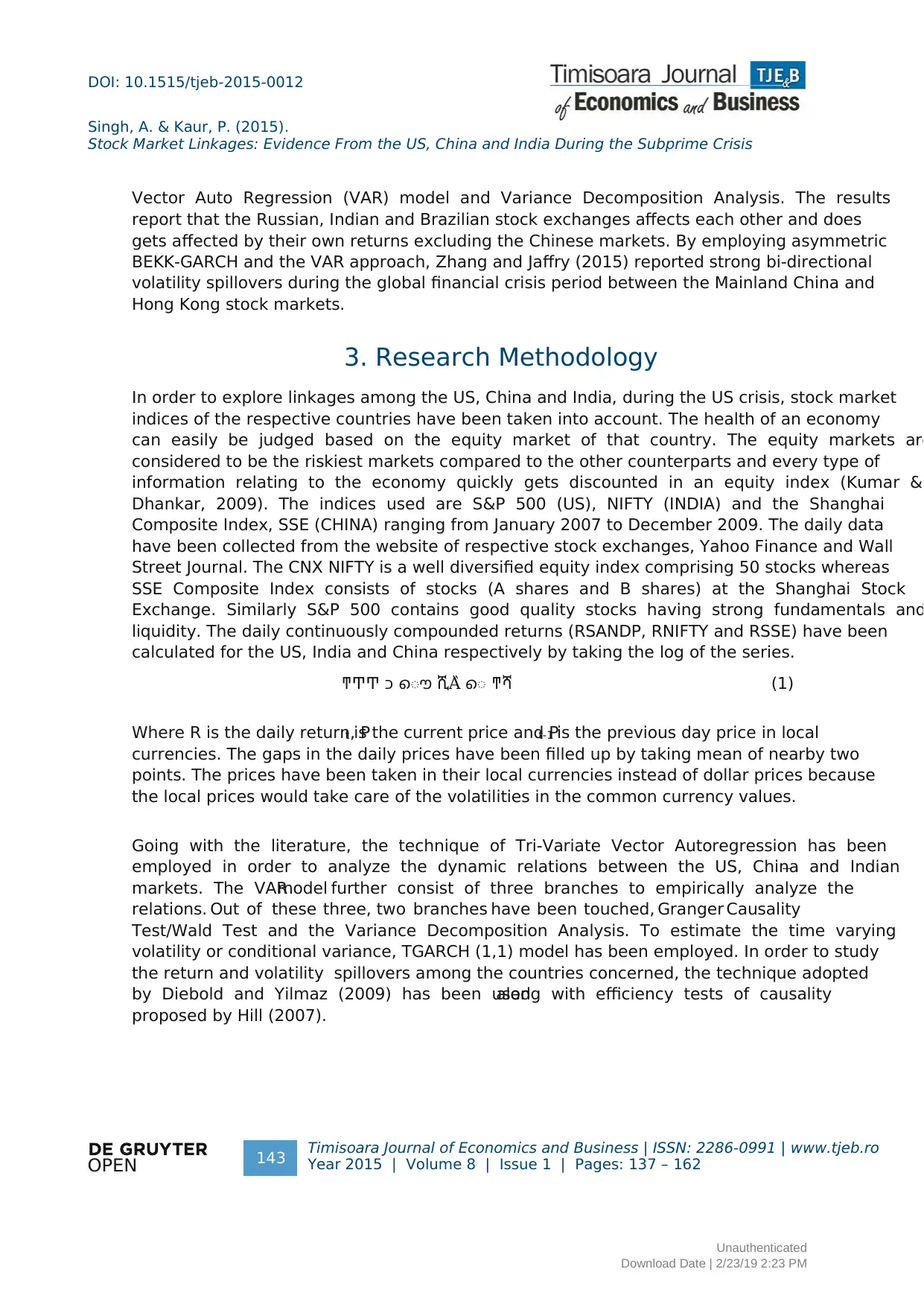
OPEN
DOI: 10.1515/tjeb-2015-0012
Singh, A. & Kaur, P. (2015).
Stock Market Linkages: Evidence From the US, China and India During the Subprime Crisis
Timisoara Journal of Economics and Business | ISSN: 2286-0991 | www.tjeb.ro
Year 2015 | Volume 8 | Issue 1 | Pages: 137 – 162143
Vector Auto Regression (VAR) model and Variance Decomposition Analysis. The results
report that the Russian, Indian and Brazilian stock exchanges affects each other and does
gets affected by their own returns excluding the Chinese markets. By employing asymmetric
BEKK-GARCH and the VAR approach, Zhang and Jaffry (2015) reported strong bi-directional
volatility spillovers during the global financial crisis period between the Mainland China and
Hong Kong stock markets.
3. Research Methodology
In order to explore linkages among the US, China and India, during the US crisis, stock market
indices of the respective countries have been taken into account. The health of an economy
can easily be judged based on the equity market of that country. The equity markets are
considered to be the riskiest markets compared to the other counterparts and every type of
information relating to the economy quickly gets discounted in an equity index (Kumar &
Dhankar, 2009). The indices used are S&P 500 (US), NIFTY (INDIA) and the Shanghai
Composite Index, SSE (CHINA) ranging from January 2007 to December 2009. The daily data
have been collected from the website of respective stock exchanges, Yahoo Finance and Wall
Street Journal. The CNX NIFTY is a well diversified equity index comprising 50 stocks whereas
SSE Composite Index consists of stocks (A shares and B shares) at the Shanghai Stock
Exchange. Similarly S&P 500 contains good quality stocks having strong fundamentals and
liquidity. The daily continuously compounded returns (RSANDP, RNIFTY and RSSE) have been
calculated for the US, India and China respectively by taking the log of the series.
ൌ ሺȀ ൌ ͳሻכͳͲͲ (1)
Where R is the daily return, Pt is the current price and Pt-1 is the previous day price in local
currencies. The gaps in the daily prices have been filled up by taking mean of nearby two
points. The prices have been taken in their local currencies instead of dollar prices because
the local prices would take care of the volatilities in the common currency values.
Going with the literature, the technique of Tri-Variate Vector Autoregression has been
employed in order to analyze the dynamic relations between the US, China and Indian
markets. The VARmodel further consist of three branches to empirically analyze the
relations. Out of these three, two branches have been touched, Granger Causality
Test/Wald Test and the Variance Decomposition Analysis. To estimate the time varying
volatility or conditional variance, TGARCH (1,1) model has been employed. In order to study
the return and volatility spillovers among the countries concerned, the technique adopted
by Diebold and Yilmaz (2009) has been usedalong with efficiency tests of causality
proposed by Hill (2007).
Unauthenticated
Download Date | 2/23/19 2:23 PM
DOI: 10.1515/tjeb-2015-0012
Singh, A. & Kaur, P. (2015).
Stock Market Linkages: Evidence From the US, China and India During the Subprime Crisis
Timisoara Journal of Economics and Business | ISSN: 2286-0991 | www.tjeb.ro
Year 2015 | Volume 8 | Issue 1 | Pages: 137 – 162143
Vector Auto Regression (VAR) model and Variance Decomposition Analysis. The results
report that the Russian, Indian and Brazilian stock exchanges affects each other and does
gets affected by their own returns excluding the Chinese markets. By employing asymmetric
BEKK-GARCH and the VAR approach, Zhang and Jaffry (2015) reported strong bi-directional
volatility spillovers during the global financial crisis period between the Mainland China and
Hong Kong stock markets.
3. Research Methodology
In order to explore linkages among the US, China and India, during the US crisis, stock market
indices of the respective countries have been taken into account. The health of an economy
can easily be judged based on the equity market of that country. The equity markets are
considered to be the riskiest markets compared to the other counterparts and every type of
information relating to the economy quickly gets discounted in an equity index (Kumar &
Dhankar, 2009). The indices used are S&P 500 (US), NIFTY (INDIA) and the Shanghai
Composite Index, SSE (CHINA) ranging from January 2007 to December 2009. The daily data
have been collected from the website of respective stock exchanges, Yahoo Finance and Wall
Street Journal. The CNX NIFTY is a well diversified equity index comprising 50 stocks whereas
SSE Composite Index consists of stocks (A shares and B shares) at the Shanghai Stock
Exchange. Similarly S&P 500 contains good quality stocks having strong fundamentals and
liquidity. The daily continuously compounded returns (RSANDP, RNIFTY and RSSE) have been
calculated for the US, India and China respectively by taking the log of the series.
ൌ ሺȀ ൌ ͳሻכͳͲͲ (1)
Where R is the daily return, Pt is the current price and Pt-1 is the previous day price in local
currencies. The gaps in the daily prices have been filled up by taking mean of nearby two
points. The prices have been taken in their local currencies instead of dollar prices because
the local prices would take care of the volatilities in the common currency values.
Going with the literature, the technique of Tri-Variate Vector Autoregression has been
employed in order to analyze the dynamic relations between the US, China and Indian
markets. The VARmodel further consist of three branches to empirically analyze the
relations. Out of these three, two branches have been touched, Granger Causality
Test/Wald Test and the Variance Decomposition Analysis. To estimate the time varying
volatility or conditional variance, TGARCH (1,1) model has been employed. In order to study
the return and volatility spillovers among the countries concerned, the technique adopted
by Diebold and Yilmaz (2009) has been usedalong with efficiency tests of causality
proposed by Hill (2007).
Unauthenticated
Download Date | 2/23/19 2:23 PM
Secure Best Marks with AI Grader
Need help grading? Try our AI Grader for instant feedback on your assignments.
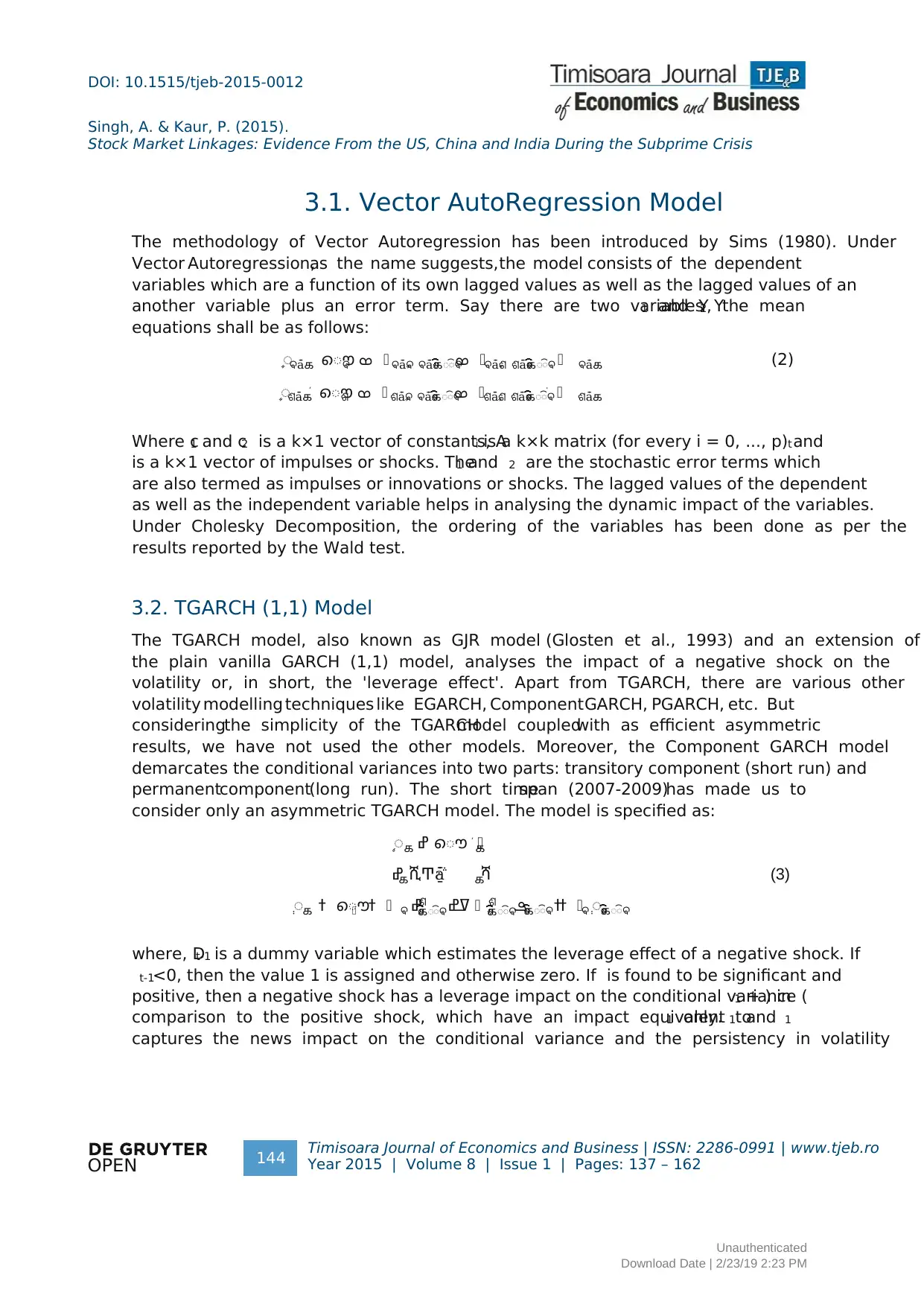
OPEN
DOI: 10.1515/tjeb-2015-0012
Singh, A. & Kaur, P. (2015).
Stock Market Linkages: Evidence From the US, China and India During the Subprime Crisis
Timisoara Journal of Economics and Business | ISSN: 2286-0991 | www.tjeb.ro
Year 2015 | Volume 8 | Issue 1 | Pages: 137 – 162144
3.1. Vector AutoRegression Model
The methodology of Vector Autoregression has been introduced by Sims (1980). Under
Vector Autoregression,as the name suggests, the model consists of the dependent
variables which are a function of its own lagged values as well as the lagged values of an
another variable plus an error term. Say there are two variables Y1 and Y2, the mean
equations shall be as follows:ܻ
ଵǡ௧ ൌଵ ܣ ଵǡଵܻ ଵǡ௧ିି ଵିିିିିିିିିିିିି ܣ ଵǡଶܻ ଶǡ௧ିି ଵିିିିିିିିିିିିି ଵǡ௧ (2)ܻ
ଶǡ௧ ൌܿ ଶ ܣ ଶǡଵܻ ଵǡ௧ିି ଵିିିିିିିିିିିିି ܣ ଶǡଶܻ ଶǡ௧ିି ଵିିିିିିିିିିିିି ݁ ଶǡ௧
Where c1 and c2 is a k×1 vector of constants, Ai is a k×k matrix (for every i = 0, ..., p) andt
is a k×1 vector of impulses or shocks. The1 and 2 are the stochastic error terms which
are also termed as impulses or innovations or shocks. The lagged values of the dependent
as well as the independent variable helps in analysing the dynamic impact of the variables.
Under Cholesky Decomposition, the ordering of the variables has been done as per the
results reported by the Wald test.
3.2. TGARCH (1,1) Model
The TGARCH model, also known as GJR model (Glosten et al., 1993) and an extension of
the plain vanilla GARCH (1,1) model, analyses the impact of a negative shock on the
volatility or, in short, the 'leverage effect'. Apart from TGARCH, there are various other
volatility modelling techniques like EGARCH, Component GARCH, PGARCH, etc. But
consideringthe simplicity of the TGARCHmodel coupledwith as efficient asymmetric
results, we have not used the other models. Moreover, the Component GARCH model
demarcates the conditional variances into two parts: transitory component (short run) and
permanentcomponent(long run). The short timespan (2007-2009)has made us to
consider only an asymmetric TGARCH model. The model is specified as:ܴ
௧ ൌ ܿߝ ௧
ߝ௧̱݅݅݀ ሺ ǡͲ ௧ሻ (3)݄
௧ ൌߙ ߙ ଵ ߝ௧ିି ଵିିିିିିିିିିିିି
ଶ ߜߝ ௧ିି ଵିିିିିିିିିିିିି
ଶ ܦ௧ିି ଵିିିିିିିିିିିିି ߚ ଵ݄ ௧ିି ଵିିିିିିିିିିିିି
where, Dt-1 is a dummy variable which estimates the leverage effect of a negative shock. If
t-1<0, then the value 1 is assigned and otherwise zero. If is found to be significant and
positive, then a negative shock has a leverage impact on the conditional variance (1 + ) in
comparison to the positive shock, which have an impact equivalent to1 only. 1 and 1
captures the news impact on the conditional variance and the persistency in volatility
Unauthenticated
Download Date | 2/23/19 2:23 PM
DOI: 10.1515/tjeb-2015-0012
Singh, A. & Kaur, P. (2015).
Stock Market Linkages: Evidence From the US, China and India During the Subprime Crisis
Timisoara Journal of Economics and Business | ISSN: 2286-0991 | www.tjeb.ro
Year 2015 | Volume 8 | Issue 1 | Pages: 137 – 162144
3.1. Vector AutoRegression Model
The methodology of Vector Autoregression has been introduced by Sims (1980). Under
Vector Autoregression,as the name suggests, the model consists of the dependent
variables which are a function of its own lagged values as well as the lagged values of an
another variable plus an error term. Say there are two variables Y1 and Y2, the mean
equations shall be as follows:ܻ
ଵǡ௧ ൌଵ ܣ ଵǡଵܻ ଵǡ௧ିି ଵିିିିିିିିିିିିି ܣ ଵǡଶܻ ଶǡ௧ିି ଵିିିିିିିିିିିିି ଵǡ௧ (2)ܻ
ଶǡ௧ ൌܿ ଶ ܣ ଶǡଵܻ ଵǡ௧ିି ଵିିିିିିିିିିିିି ܣ ଶǡଶܻ ଶǡ௧ିି ଵିିିିିିିିିିିିି ݁ ଶǡ௧
Where c1 and c2 is a k×1 vector of constants, Ai is a k×k matrix (for every i = 0, ..., p) andt
is a k×1 vector of impulses or shocks. The1 and 2 are the stochastic error terms which
are also termed as impulses or innovations or shocks. The lagged values of the dependent
as well as the independent variable helps in analysing the dynamic impact of the variables.
Under Cholesky Decomposition, the ordering of the variables has been done as per the
results reported by the Wald test.
3.2. TGARCH (1,1) Model
The TGARCH model, also known as GJR model (Glosten et al., 1993) and an extension of
the plain vanilla GARCH (1,1) model, analyses the impact of a negative shock on the
volatility or, in short, the 'leverage effect'. Apart from TGARCH, there are various other
volatility modelling techniques like EGARCH, Component GARCH, PGARCH, etc. But
consideringthe simplicity of the TGARCHmodel coupledwith as efficient asymmetric
results, we have not used the other models. Moreover, the Component GARCH model
demarcates the conditional variances into two parts: transitory component (short run) and
permanentcomponent(long run). The short timespan (2007-2009)has made us to
consider only an asymmetric TGARCH model. The model is specified as:ܴ
௧ ൌ ܿߝ ௧
ߝ௧̱݅݅݀ ሺ ǡͲ ௧ሻ (3)݄
௧ ൌߙ ߙ ଵ ߝ௧ିି ଵିିିିିିିିିିିିି
ଶ ߜߝ ௧ିି ଵିିିିିିିିିିିିି
ଶ ܦ௧ିି ଵିିିିିିିିିିିିି ߚ ଵ݄ ௧ିି ଵିିିିିିିିିିିିି
where, Dt-1 is a dummy variable which estimates the leverage effect of a negative shock. If
t-1<0, then the value 1 is assigned and otherwise zero. If is found to be significant and
positive, then a negative shock has a leverage impact on the conditional variance (1 + ) in
comparison to the positive shock, which have an impact equivalent to1 only. 1 and 1
captures the news impact on the conditional variance and the persistency in volatility
Unauthenticated
Download Date | 2/23/19 2:23 PM
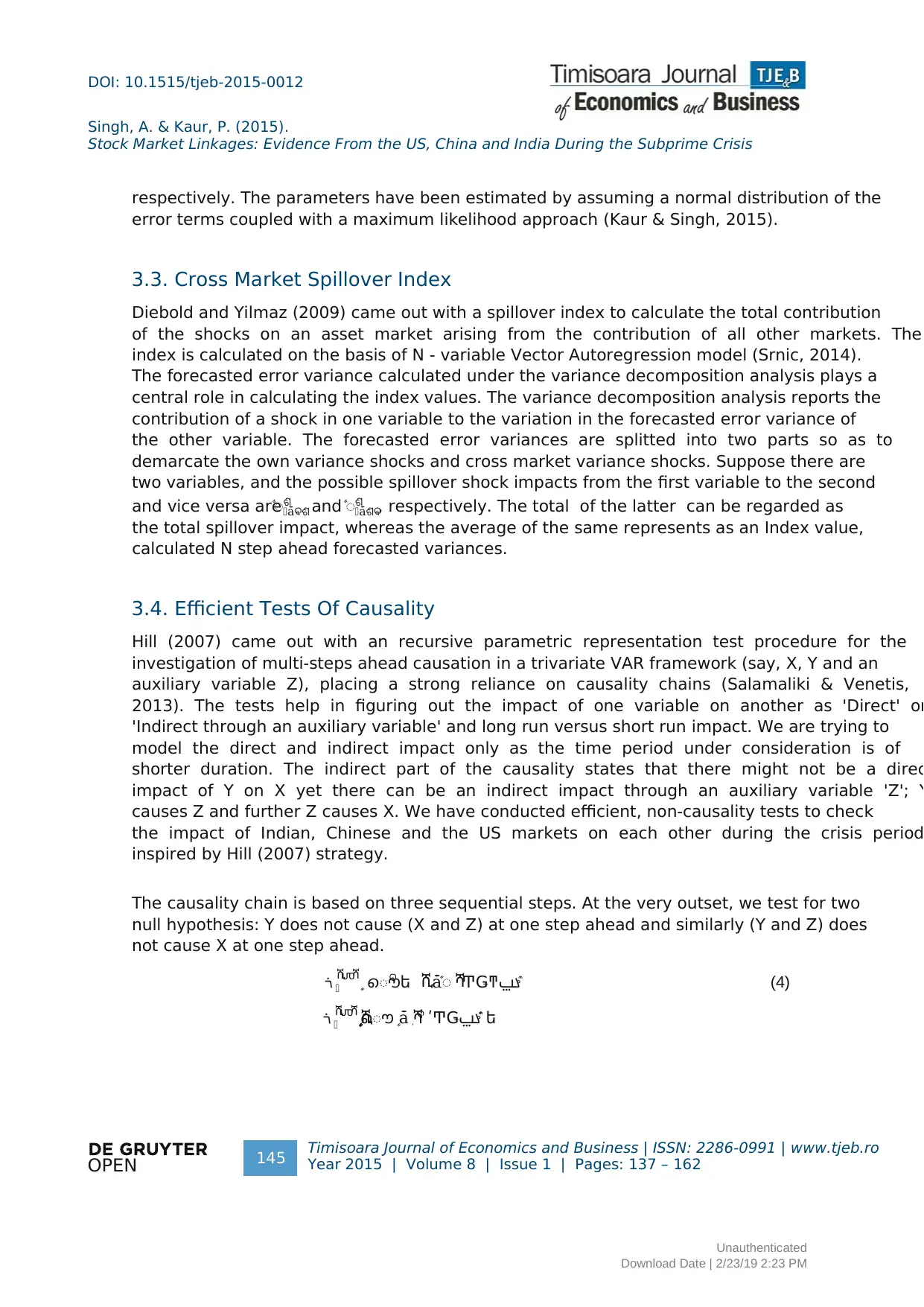
OPEN
DOI: 10.1515/tjeb-2015-0012
Singh, A. & Kaur, P. (2015).
Stock Market Linkages: Evidence From the US, China and India During the Subprime Crisis
Timisoara Journal of Economics and Business | ISSN: 2286-0991 | www.tjeb.ro
Year 2015 | Volume 8 | Issue 1 | Pages: 137 – 162145
respectively. The parameters have been estimated by assuming a normal distribution of the
error terms coupled with a maximum likelihood approach (Kaur & Singh, 2015).
3.3. Cross Market Spillover Index
Diebold and Yilmaz (2009) came out with a spillover index to calculate the total contribution
of the shocks on an asset market arising from the contribution of all other markets. The
index is calculated on the basis of N - variable Vector Autoregression model (Srnic, 2014).
The forecasted error variance calculated under the variance decomposition analysis plays a
central role in calculating the index values. The variance decomposition analysis reports the
contribution of a shock in one variable to the variation in the forecasted error variance of
the other variable. The forecasted error variances are splitted into two parts so as to
demarcate the own variance shocks and cross market variance shocks. Suppose there are
two variables, and the possible spillover shock impacts from the first variable to the second
and vice versa areܽ ǡଵଶ
ଶ andܽ ǡଶଵ
ଶ , respectively. The total of the latter can be regarded as
the total spillover impact, whereas the average of the same represents as an Index value,
calculated N step ahead forecasted variances.
3.4. Efficient Tests Of Causality
Hill (2007) came out with an recursive parametric representation test procedure for the
investigation of multi-steps ahead causation in a trivariate VAR framework (say, X, Y and an
auxiliary variable Z), placing a strong reliance on causality chains (Salamaliki & Venetis,
2013). The tests help in figuring out the impact of one variable on another as 'Direct' or
'Indirect through an auxiliary variable' and long run versus short run impact. We are trying to
model the direct and indirect impact only as the time period under consideration is of
shorter duration. The indirect part of the causality states that there might not be a direc
impact of Y on X yet there can be an indirect impact through an auxiliary variable 'Z'; Y
causes Z and further Z causes X. We have conducted efficient, non-causality tests to check
the impact of Indian, Chinese and the US markets on each other during the crisis period
inspired by Hill (2007) strategy.
The causality chain is based on three sequential steps. At the very outset, we test for two
null hypothesis: Y does not cause (X and Z) at one step ahead and similarly (Y and Z) does
not cause X at one step ahead.
ܪ
ሺஶሻ ൌܻଵ ե ሺ ǡ ሻ ܶ݁ݏݐͲǤͳ (4)
ܪ
ሺஶሻ ൌሺܻܻܻܻܻܻܻܻܻܻܻܻܻ ǡܼ ሻଵ եݐܶ݁ݏͲǤʹ
Unauthenticated
Download Date | 2/23/19 2:23 PM
DOI: 10.1515/tjeb-2015-0012
Singh, A. & Kaur, P. (2015).
Stock Market Linkages: Evidence From the US, China and India During the Subprime Crisis
Timisoara Journal of Economics and Business | ISSN: 2286-0991 | www.tjeb.ro
Year 2015 | Volume 8 | Issue 1 | Pages: 137 – 162145
respectively. The parameters have been estimated by assuming a normal distribution of the
error terms coupled with a maximum likelihood approach (Kaur & Singh, 2015).
3.3. Cross Market Spillover Index
Diebold and Yilmaz (2009) came out with a spillover index to calculate the total contribution
of the shocks on an asset market arising from the contribution of all other markets. The
index is calculated on the basis of N - variable Vector Autoregression model (Srnic, 2014).
The forecasted error variance calculated under the variance decomposition analysis plays a
central role in calculating the index values. The variance decomposition analysis reports the
contribution of a shock in one variable to the variation in the forecasted error variance of
the other variable. The forecasted error variances are splitted into two parts so as to
demarcate the own variance shocks and cross market variance shocks. Suppose there are
two variables, and the possible spillover shock impacts from the first variable to the second
and vice versa areܽ ǡଵଶ
ଶ andܽ ǡଶଵ
ଶ , respectively. The total of the latter can be regarded as
the total spillover impact, whereas the average of the same represents as an Index value,
calculated N step ahead forecasted variances.
3.4. Efficient Tests Of Causality
Hill (2007) came out with an recursive parametric representation test procedure for the
investigation of multi-steps ahead causation in a trivariate VAR framework (say, X, Y and an
auxiliary variable Z), placing a strong reliance on causality chains (Salamaliki & Venetis,
2013). The tests help in figuring out the impact of one variable on another as 'Direct' or
'Indirect through an auxiliary variable' and long run versus short run impact. We are trying to
model the direct and indirect impact only as the time period under consideration is of
shorter duration. The indirect part of the causality states that there might not be a direc
impact of Y on X yet there can be an indirect impact through an auxiliary variable 'Z'; Y
causes Z and further Z causes X. We have conducted efficient, non-causality tests to check
the impact of Indian, Chinese and the US markets on each other during the crisis period
inspired by Hill (2007) strategy.
The causality chain is based on three sequential steps. At the very outset, we test for two
null hypothesis: Y does not cause (X and Z) at one step ahead and similarly (Y and Z) does
not cause X at one step ahead.
ܪ
ሺஶሻ ൌܻଵ ե ሺ ǡ ሻ ܶ݁ݏݐͲǤͳ (4)
ܪ
ሺஶሻ ൌሺܻܻܻܻܻܻܻܻܻܻܻܻܻ ǡܼ ሻଵ եݐܶ݁ݏͲǤʹ
Unauthenticated
Download Date | 2/23/19 2:23 PM
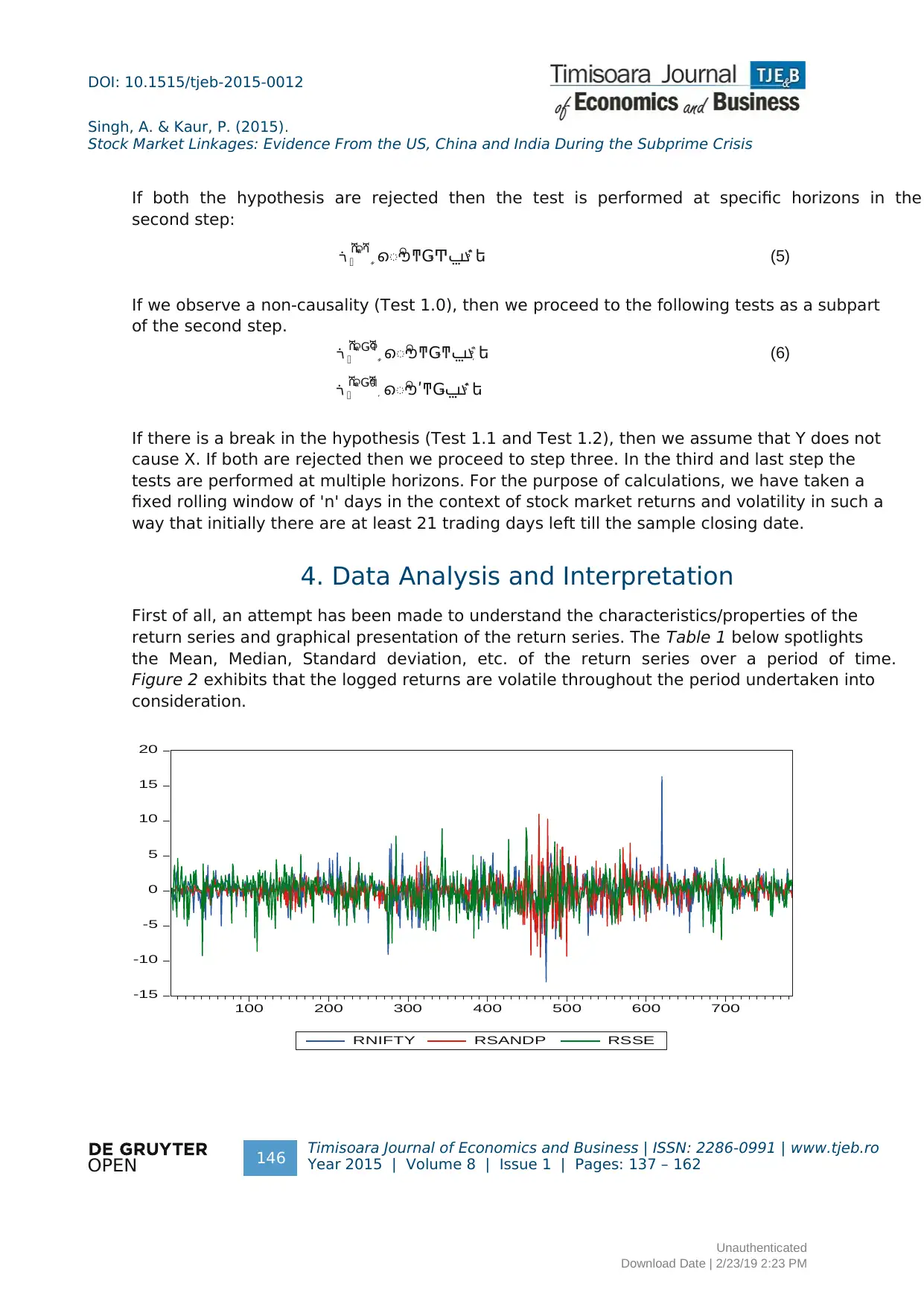
OPEN
DOI: 10.1515/tjeb-2015-0012
Singh, A. & Kaur, P. (2015).
Stock Market Linkages: Evidence From the US, China and India During the Subprime Crisis
Timisoara Journal of Economics and Business | ISSN: 2286-0991 | www.tjeb.ro
Year 2015 | Volume 8 | Issue 1 | Pages: 137 – 162146
If both the hypothesis are rejected then the test is performed at specific horizons in the
second step:
ܪ
ሺଵሻ ൌܻଵ եݐܶ݁ݏͳǤͲ (5)
If we observe a non-causality (Test 1.0), then we proceed to the following tests as a subpart
of the second step.
ܪ
ሺଵǤଵሻ ൌܻଵ եݐܼܶ݁ݏͳǤͳ (6)
ܪ
ሺଵǤଶሻ ൌܼଵ եݐܶ݁ݏͳǤʹ
If there is a break in the hypothesis (Test 1.1 and Test 1.2), then we assume that Y does not
cause X. If both are rejected then we proceed to step three. In the third and last step the
tests are performed at multiple horizons. For the purpose of calculations, we have taken a
fixed rolling window of 'n' days in the context of stock market returns and volatility in such a
way that initially there are at least 21 trading days left till the sample closing date.
4. Data Analysis and Interpretation
First of all, an attempt has been made to understand the characteristics/properties of the
return series and graphical presentation of the return series. The Table 1 below spotlights
the Mean, Median, Standard deviation, etc. of the return series over a period of time.
Figure 2 exhibits that the logged returns are volatile throughout the period undertaken into
consideration.
-15
-10
-5
0
5
10
15
20
100 200 300 400 500 600 700
RNIFTY RSANDP RSSE
Unauthenticated
Download Date | 2/23/19 2:23 PM
DOI: 10.1515/tjeb-2015-0012
Singh, A. & Kaur, P. (2015).
Stock Market Linkages: Evidence From the US, China and India During the Subprime Crisis
Timisoara Journal of Economics and Business | ISSN: 2286-0991 | www.tjeb.ro
Year 2015 | Volume 8 | Issue 1 | Pages: 137 – 162146
If both the hypothesis are rejected then the test is performed at specific horizons in the
second step:
ܪ
ሺଵሻ ൌܻଵ եݐܶ݁ݏͳǤͲ (5)
If we observe a non-causality (Test 1.0), then we proceed to the following tests as a subpart
of the second step.
ܪ
ሺଵǤଵሻ ൌܻଵ եݐܼܶ݁ݏͳǤͳ (6)
ܪ
ሺଵǤଶሻ ൌܼଵ եݐܶ݁ݏͳǤʹ
If there is a break in the hypothesis (Test 1.1 and Test 1.2), then we assume that Y does not
cause X. If both are rejected then we proceed to step three. In the third and last step the
tests are performed at multiple horizons. For the purpose of calculations, we have taken a
fixed rolling window of 'n' days in the context of stock market returns and volatility in such a
way that initially there are at least 21 trading days left till the sample closing date.
4. Data Analysis and Interpretation
First of all, an attempt has been made to understand the characteristics/properties of the
return series and graphical presentation of the return series. The Table 1 below spotlights
the Mean, Median, Standard deviation, etc. of the return series over a period of time.
Figure 2 exhibits that the logged returns are volatile throughout the period undertaken into
consideration.
-15
-10
-5
0
5
10
15
20
100 200 300 400 500 600 700
RNIFTY RSANDP RSSE
Unauthenticated
Download Date | 2/23/19 2:23 PM
Paraphrase This Document
Need a fresh take? Get an instant paraphrase of this document with our AI Paraphraser
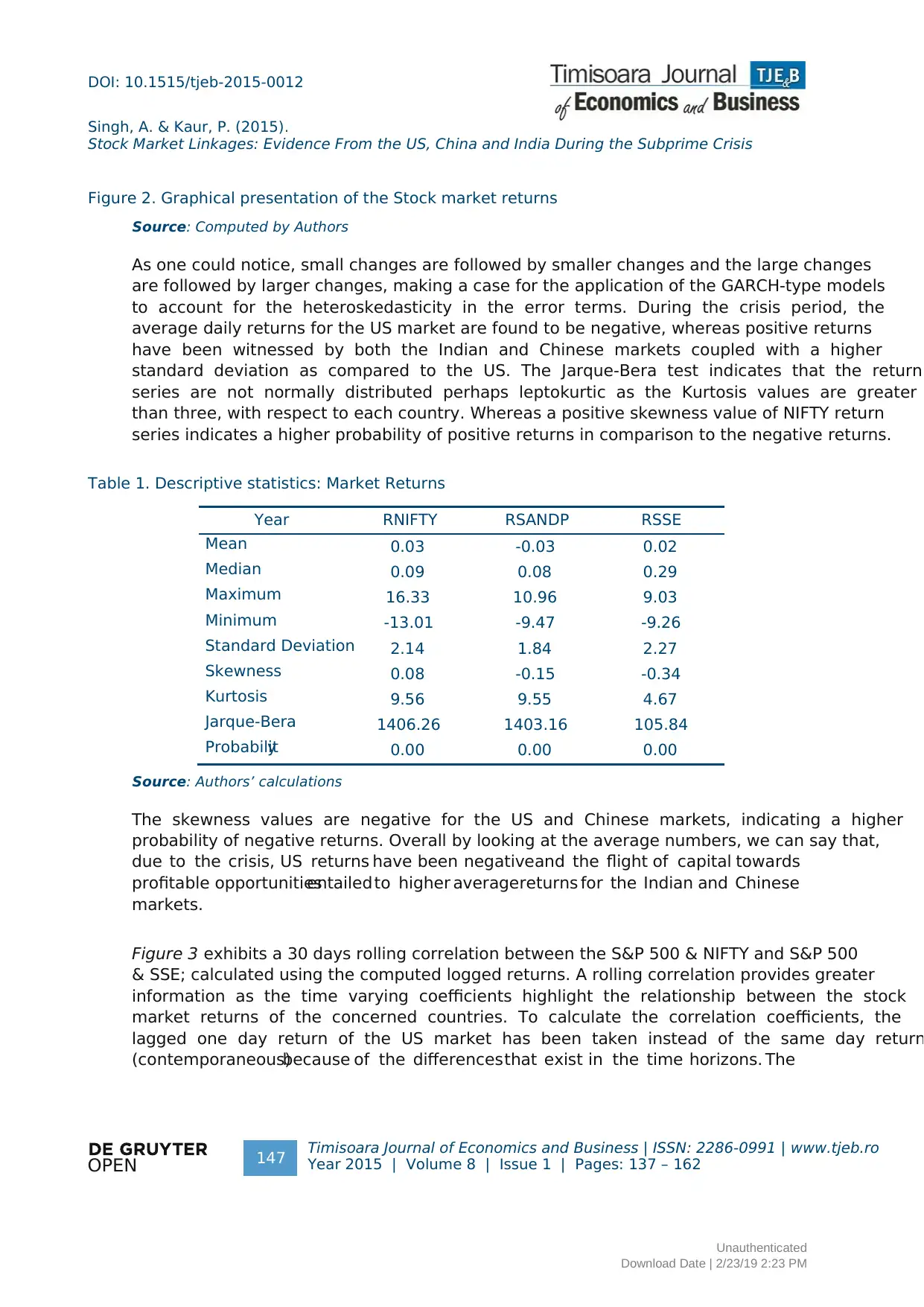
OPEN
DOI: 10.1515/tjeb-2015-0012
Singh, A. & Kaur, P. (2015).
Stock Market Linkages: Evidence From the US, China and India During the Subprime Crisis
Timisoara Journal of Economics and Business | ISSN: 2286-0991 | www.tjeb.ro
Year 2015 | Volume 8 | Issue 1 | Pages: 137 – 162147
Figure 2. Graphical presentation of the Stock market returns
Source: Computed by Authors
As one could notice, small changes are followed by smaller changes and the large changes
are followed by larger changes, making a case for the application of the GARCH-type models
to account for the heteroskedasticity in the error terms. During the crisis period, the
average daily returns for the US market are found to be negative, whereas positive returns
have been witnessed by both the Indian and Chinese markets coupled with a higher
standard deviation as compared to the US. The Jarque-Bera test indicates that the return
series are not normally distributed perhaps leptokurtic as the Kurtosis values are greater
than three, with respect to each country. Whereas a positive skewness value of NIFTY return
series indicates a higher probability of positive returns in comparison to the negative returns.
Table 1. Descriptive statistics: Market Returns
Year RNIFTY RSANDP RSSE
Mean 0.03 -0.03 0.02
Median 0.09 0.08 0.29
Maximum 16.33 10.96 9.03
Minimum -13.01 -9.47 -9.26
Standard Deviation 2.14 1.84 2.27
Skewness 0.08 -0.15 -0.34
Kurtosis 9.56 9.55 4.67
Jarque-Bera 1406.26 1403.16 105.84
Probability 0.00 0.00 0.00
Source: Authors’ calculations
The skewness values are negative for the US and Chinese markets, indicating a higher
probability of negative returns. Overall by looking at the average numbers, we can say that,
due to the crisis, US returns have been negativeand the flight of capital towards
profitable opportunitiesentailed to higher averagereturns for the Indian and Chinese
markets.
Figure 3 exhibits a 30 days rolling correlation between the S&P 500 & NIFTY and S&P 500
& SSE; calculated using the computed logged returns. A rolling correlation provides greater
information as the time varying coefficients highlight the relationship between the stock
market returns of the concerned countries. To calculate the correlation coefficients, the
lagged one day return of the US market has been taken instead of the same day return
(contemporaneous)because of the differences that exist in the time horizons. The
Unauthenticated
Download Date | 2/23/19 2:23 PM
DOI: 10.1515/tjeb-2015-0012
Singh, A. & Kaur, P. (2015).
Stock Market Linkages: Evidence From the US, China and India During the Subprime Crisis
Timisoara Journal of Economics and Business | ISSN: 2286-0991 | www.tjeb.ro
Year 2015 | Volume 8 | Issue 1 | Pages: 137 – 162147
Figure 2. Graphical presentation of the Stock market returns
Source: Computed by Authors
As one could notice, small changes are followed by smaller changes and the large changes
are followed by larger changes, making a case for the application of the GARCH-type models
to account for the heteroskedasticity in the error terms. During the crisis period, the
average daily returns for the US market are found to be negative, whereas positive returns
have been witnessed by both the Indian and Chinese markets coupled with a higher
standard deviation as compared to the US. The Jarque-Bera test indicates that the return
series are not normally distributed perhaps leptokurtic as the Kurtosis values are greater
than three, with respect to each country. Whereas a positive skewness value of NIFTY return
series indicates a higher probability of positive returns in comparison to the negative returns.
Table 1. Descriptive statistics: Market Returns
Year RNIFTY RSANDP RSSE
Mean 0.03 -0.03 0.02
Median 0.09 0.08 0.29
Maximum 16.33 10.96 9.03
Minimum -13.01 -9.47 -9.26
Standard Deviation 2.14 1.84 2.27
Skewness 0.08 -0.15 -0.34
Kurtosis 9.56 9.55 4.67
Jarque-Bera 1406.26 1403.16 105.84
Probability 0.00 0.00 0.00
Source: Authors’ calculations
The skewness values are negative for the US and Chinese markets, indicating a higher
probability of negative returns. Overall by looking at the average numbers, we can say that,
due to the crisis, US returns have been negativeand the flight of capital towards
profitable opportunitiesentailed to higher averagereturns for the Indian and Chinese
markets.
Figure 3 exhibits a 30 days rolling correlation between the S&P 500 & NIFTY and S&P 500
& SSE; calculated using the computed logged returns. A rolling correlation provides greater
information as the time varying coefficients highlight the relationship between the stock
market returns of the concerned countries. To calculate the correlation coefficients, the
lagged one day return of the US market has been taken instead of the same day return
(contemporaneous)because of the differences that exist in the time horizons. The
Unauthenticated
Download Date | 2/23/19 2:23 PM
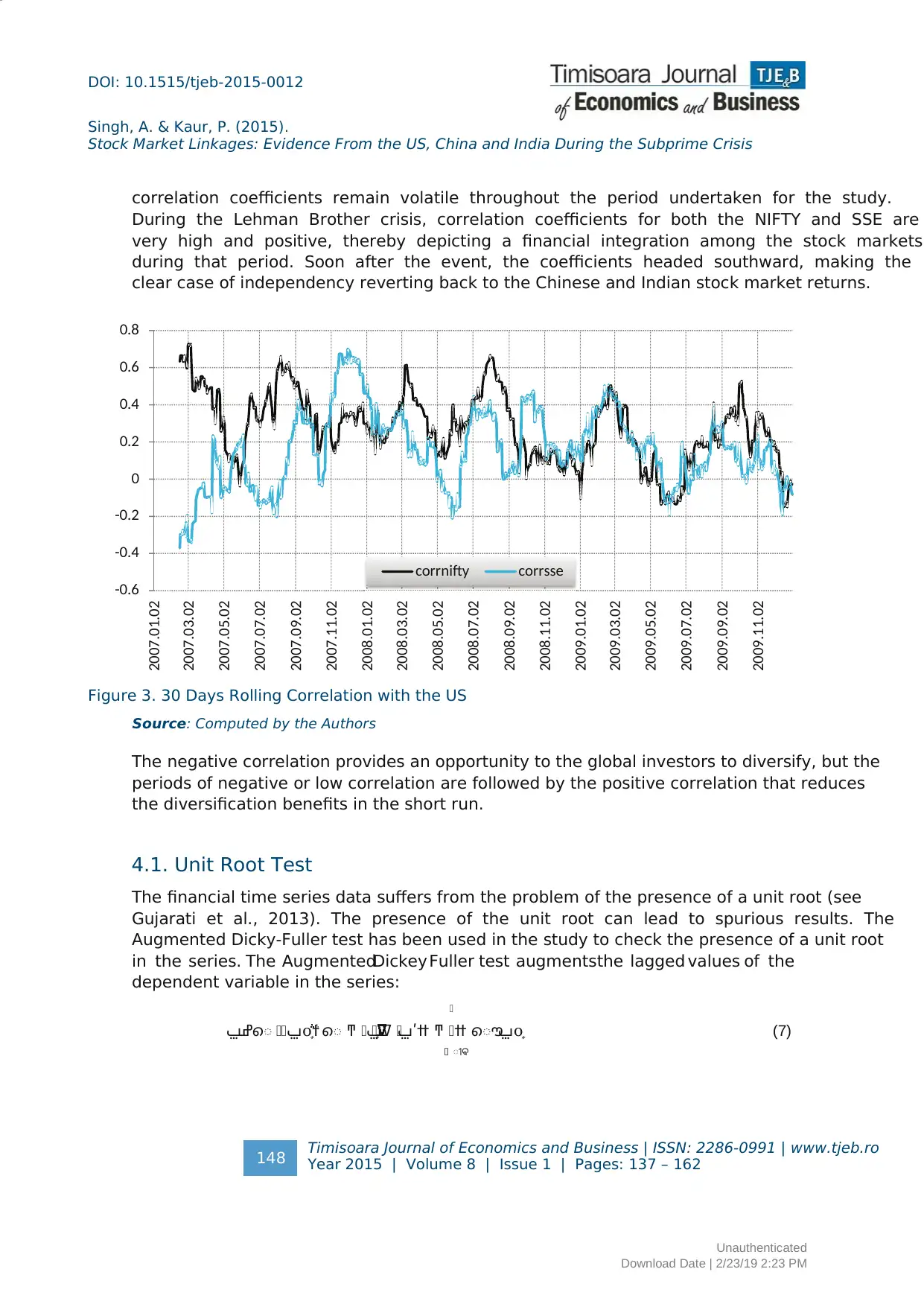
N
DOI: 10.1515/tjeb-2015-0012
Singh, A. & Kaur, P. (2015).
Stock Market Linkages: Evidence From the US, China and India During the Subprime Crisis
Timisoara Journal of Economics and Business | ISSN: 2286-0991 | www.tjeb.ro
Year 2015 | Volume 8 | Issue 1 | Pages: 137 – 162148
correlation coefficients remain volatile throughout the period undertaken for the study.
During the Lehman Brother crisis, correlation coefficients for both the NIFTY and SSE are
very high and positive, thereby depicting a financial integration among the stock markets
during that period. Soon after the event, the coefficients headed southward, making the
clear case of independency reverting back to the Chinese and Indian stock market returns.
Figure 3. 30 Days Rolling Correlation with the US
Source: Computed by the Authors
The negative correlation provides an opportunity to the global investors to diversify, but the
periods of negative or low correlation are followed by the positive correlation that reduces
the diversification benefits in the short run.
4.1. Unit Root Test
The financial time series data suffers from the problem of the presence of a unit root (see
Gujarati et al., 2013). The presence of the unit root can lead to spurious results. The
Augmented Dicky-Fuller test has been used in the study to check the presence of a unit root
in the series. The AugmentedDickey Fuller test augmentsthe lagged values of the
dependent variable in the series:
οܻݐൌߚͳݐߚʹݐܻߜߜߜߜߜߜߜߜߜߜߜߜߜൌ ͳ ߙ݅݅݅݅݅݅݅݅݅݅݅݅݅݅οܻݐൌݐߝ
ି ଵ
(7)
-0.6
-0.4
-0.2
0
0.2
0.4
0.6
0.8
2007.01.02
2007.03.02
2007.05.02
2007.07.02
2007.09.02
2007.11.02
2008.01.02
2008.03.02
2008.05.02
2008.07.02
2008.09.02
2008.11.02
2009.01.02
2009.03.02
2009.05.02
2009.07.02
2009.09.02
2009.11.02
corrnifty corrsse
Unauthenticated
Download Date | 2/23/19 2:23 PM
DOI: 10.1515/tjeb-2015-0012
Singh, A. & Kaur, P. (2015).
Stock Market Linkages: Evidence From the US, China and India During the Subprime Crisis
Timisoara Journal of Economics and Business | ISSN: 2286-0991 | www.tjeb.ro
Year 2015 | Volume 8 | Issue 1 | Pages: 137 – 162148
correlation coefficients remain volatile throughout the period undertaken for the study.
During the Lehman Brother crisis, correlation coefficients for both the NIFTY and SSE are
very high and positive, thereby depicting a financial integration among the stock markets
during that period. Soon after the event, the coefficients headed southward, making the
clear case of independency reverting back to the Chinese and Indian stock market returns.
Figure 3. 30 Days Rolling Correlation with the US
Source: Computed by the Authors
The negative correlation provides an opportunity to the global investors to diversify, but the
periods of negative or low correlation are followed by the positive correlation that reduces
the diversification benefits in the short run.
4.1. Unit Root Test
The financial time series data suffers from the problem of the presence of a unit root (see
Gujarati et al., 2013). The presence of the unit root can lead to spurious results. The
Augmented Dicky-Fuller test has been used in the study to check the presence of a unit root
in the series. The AugmentedDickey Fuller test augmentsthe lagged values of the
dependent variable in the series:
οܻݐൌߚͳݐߚʹݐܻߜߜߜߜߜߜߜߜߜߜߜߜߜൌ ͳ ߙ݅݅݅݅݅݅݅݅݅݅݅݅݅݅οܻݐൌݐߝ
ି ଵ
(7)
-0.6
-0.4
-0.2
0
0.2
0.4
0.6
0.8
2007.01.02
2007.03.02
2007.05.02
2007.07.02
2007.09.02
2007.11.02
2008.01.02
2008.03.02
2008.05.02
2008.07.02
2008.09.02
2008.11.02
2009.01.02
2009.03.02
2009.05.02
2009.07.02
2009.09.02
2009.11.02
corrnifty corrsse
Unauthenticated
Download Date | 2/23/19 2:23 PM
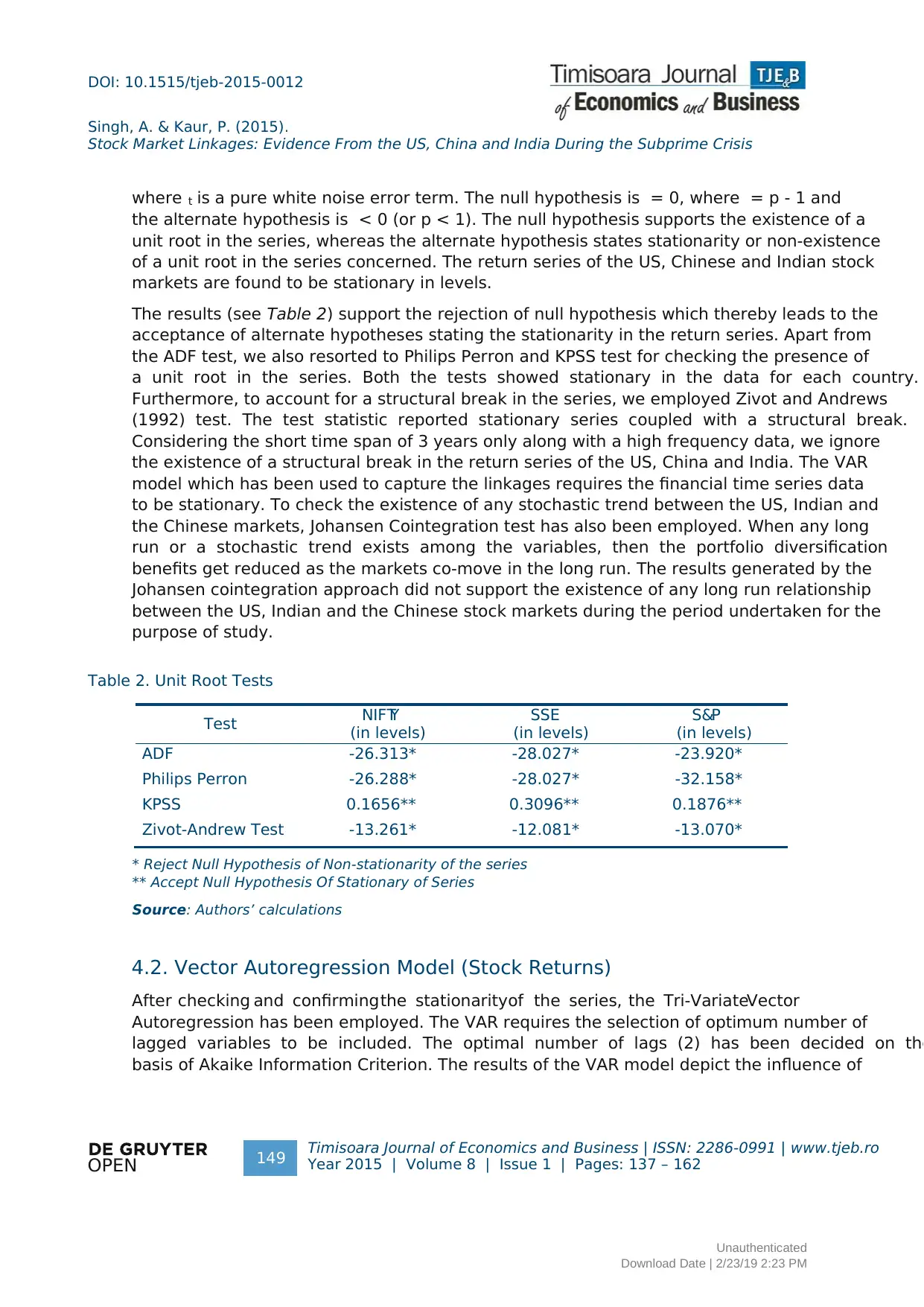
OPEN
DOI: 10.1515/tjeb-2015-0012
Singh, A. & Kaur, P. (2015).
Stock Market Linkages: Evidence From the US, China and India During the Subprime Crisis
Timisoara Journal of Economics and Business | ISSN: 2286-0991 | www.tjeb.ro
Year 2015 | Volume 8 | Issue 1 | Pages: 137 – 162149
where t is a pure white noise error term. The null hypothesis is = 0, where = p - 1 and
the alternate hypothesis is < 0 (or p < 1). The null hypothesis supports the existence of a
unit root in the series, whereas the alternate hypothesis states stationarity or non-existence
of a unit root in the series concerned. The return series of the US, Chinese and Indian stock
markets are found to be stationary in levels.
The results (see Table 2) support the rejection of null hypothesis which thereby leads to the
acceptance of alternate hypotheses stating the stationarity in the return series. Apart from
the ADF test, we also resorted to Philips Perron and KPSS test for checking the presence of
a unit root in the series. Both the tests showed stationary in the data for each country.
Furthermore, to account for a structural break in the series, we employed Zivot and Andrews
(1992) test. The test statistic reported stationary series coupled with a structural break.
Considering the short time span of 3 years only along with a high frequency data, we ignore
the existence of a structural break in the return series of the US, China and India. The VAR
model which has been used to capture the linkages requires the financial time series data
to be stationary. To check the existence of any stochastic trend between the US, Indian and
the Chinese markets, Johansen Cointegration test has also been employed. When any long
run or a stochastic trend exists among the variables, then the portfolio diversification
benefits get reduced as the markets co-move in the long run. The results generated by the
Johansen cointegration approach did not support the existence of any long run relationship
between the US, Indian and the Chinese stock markets during the period undertaken for the
purpose of study.
Table 2. Unit Root Tests
Test NIFTY
(in levels)
SSE
(in levels)
S&P
(in levels)
ADF -26.313* -28.027* -23.920*
Philips Perron -26.288* -28.027* -32.158*
KPSS 0.1656** 0.3096** 0.1876**
Zivot-Andrew Test -13.261* -12.081* -13.070*
* Reject Null Hypothesis of Non-stationarity of the series
** Accept Null Hypothesis Of Stationary of Series
Source: Authors’ calculations
4.2. Vector Autoregression Model (Stock Returns)
After checking and confirming the stationarityof the series, the Tri-VariateVector
Autoregression has been employed. The VAR requires the selection of optimum number of
lagged variables to be included. The optimal number of lags (2) has been decided on the
basis of Akaike Information Criterion. The results of the VAR model depict the influence of
Unauthenticated
Download Date | 2/23/19 2:23 PM
DOI: 10.1515/tjeb-2015-0012
Singh, A. & Kaur, P. (2015).
Stock Market Linkages: Evidence From the US, China and India During the Subprime Crisis
Timisoara Journal of Economics and Business | ISSN: 2286-0991 | www.tjeb.ro
Year 2015 | Volume 8 | Issue 1 | Pages: 137 – 162149
where t is a pure white noise error term. The null hypothesis is = 0, where = p - 1 and
the alternate hypothesis is < 0 (or p < 1). The null hypothesis supports the existence of a
unit root in the series, whereas the alternate hypothesis states stationarity or non-existence
of a unit root in the series concerned. The return series of the US, Chinese and Indian stock
markets are found to be stationary in levels.
The results (see Table 2) support the rejection of null hypothesis which thereby leads to the
acceptance of alternate hypotheses stating the stationarity in the return series. Apart from
the ADF test, we also resorted to Philips Perron and KPSS test for checking the presence of
a unit root in the series. Both the tests showed stationary in the data for each country.
Furthermore, to account for a structural break in the series, we employed Zivot and Andrews
(1992) test. The test statistic reported stationary series coupled with a structural break.
Considering the short time span of 3 years only along with a high frequency data, we ignore
the existence of a structural break in the return series of the US, China and India. The VAR
model which has been used to capture the linkages requires the financial time series data
to be stationary. To check the existence of any stochastic trend between the US, Indian and
the Chinese markets, Johansen Cointegration test has also been employed. When any long
run or a stochastic trend exists among the variables, then the portfolio diversification
benefits get reduced as the markets co-move in the long run. The results generated by the
Johansen cointegration approach did not support the existence of any long run relationship
between the US, Indian and the Chinese stock markets during the period undertaken for the
purpose of study.
Table 2. Unit Root Tests
Test NIFTY
(in levels)
SSE
(in levels)
S&P
(in levels)
ADF -26.313* -28.027* -23.920*
Philips Perron -26.288* -28.027* -32.158*
KPSS 0.1656** 0.3096** 0.1876**
Zivot-Andrew Test -13.261* -12.081* -13.070*
* Reject Null Hypothesis of Non-stationarity of the series
** Accept Null Hypothesis Of Stationary of Series
Source: Authors’ calculations
4.2. Vector Autoregression Model (Stock Returns)
After checking and confirming the stationarityof the series, the Tri-VariateVector
Autoregression has been employed. The VAR requires the selection of optimum number of
lagged variables to be included. The optimal number of lags (2) has been decided on the
basis of Akaike Information Criterion. The results of the VAR model depict the influence of
Unauthenticated
Download Date | 2/23/19 2:23 PM
Secure Best Marks with AI Grader
Need help grading? Try our AI Grader for instant feedback on your assignments.
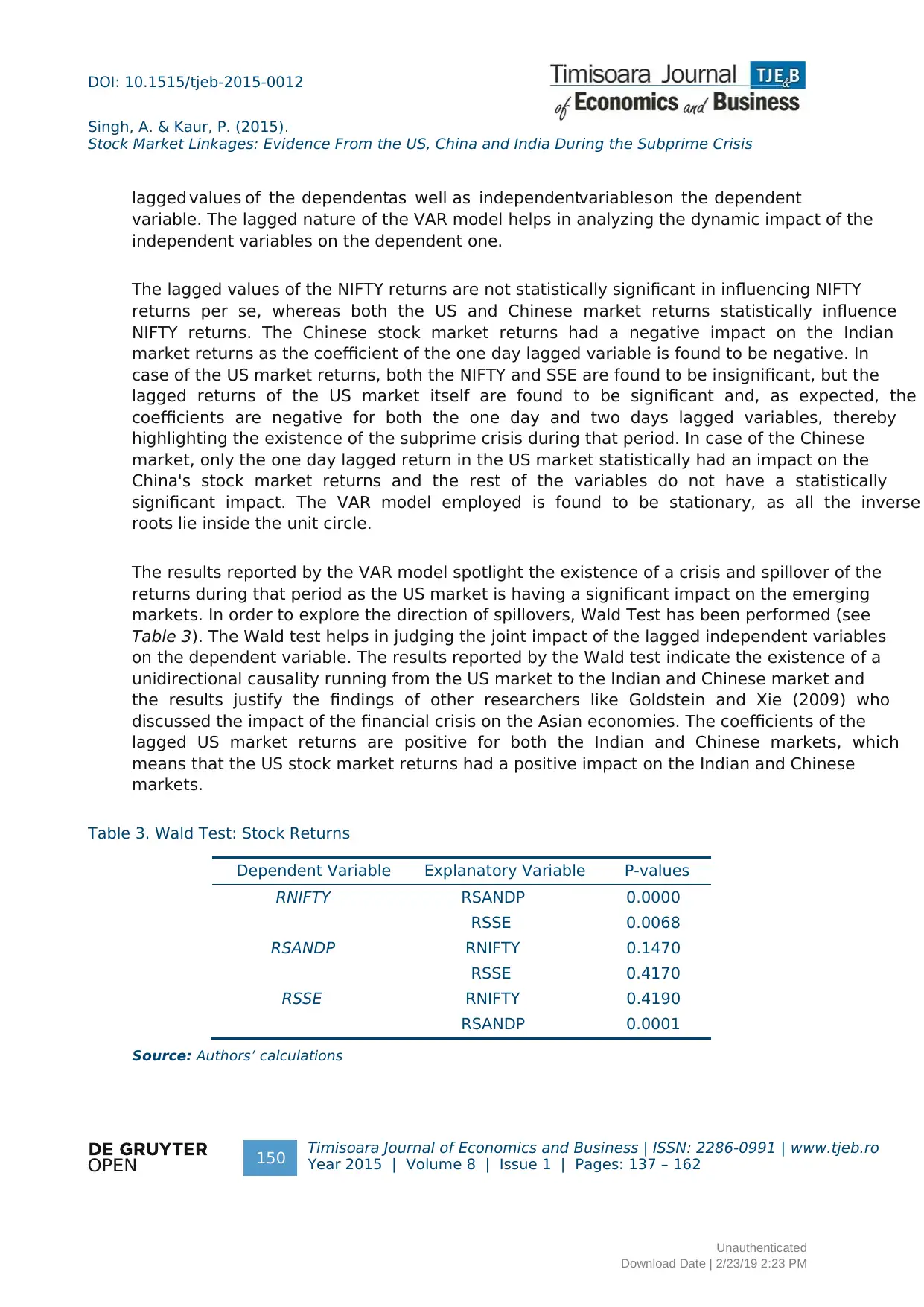
OPEN
DOI: 10.1515/tjeb-2015-0012
Singh, A. & Kaur, P. (2015).
Stock Market Linkages: Evidence From the US, China and India During the Subprime Crisis
Timisoara Journal of Economics and Business | ISSN: 2286-0991 | www.tjeb.ro
Year 2015 | Volume 8 | Issue 1 | Pages: 137 – 162150
lagged values of the dependentas well as independentvariables on the dependent
variable. The lagged nature of the VAR model helps in analyzing the dynamic impact of the
independent variables on the dependent one.
The lagged values of the NIFTY returns are not statistically significant in influencing NIFTY
returns per se, whereas both the US and Chinese market returns statistically influence
NIFTY returns. The Chinese stock market returns had a negative impact on the Indian
market returns as the coefficient of the one day lagged variable is found to be negative. In
case of the US market returns, both the NIFTY and SSE are found to be insignificant, but the
lagged returns of the US market itself are found to be significant and, as expected, the
coefficients are negative for both the one day and two days lagged variables, thereby
highlighting the existence of the subprime crisis during that period. In case of the Chinese
market, only the one day lagged return in the US market statistically had an impact on the
China's stock market returns and the rest of the variables do not have a statistically
significant impact. The VAR model employed is found to be stationary, as all the inverse
roots lie inside the unit circle.
The results reported by the VAR model spotlight the existence of a crisis and spillover of the
returns during that period as the US market is having a significant impact on the emerging
markets. In order to explore the direction of spillovers, Wald Test has been performed (see
Table 3). The Wald test helps in judging the joint impact of the lagged independent variables
on the dependent variable. The results reported by the Wald test indicate the existence of a
unidirectional causality running from the US market to the Indian and Chinese market and
the results justify the findings of other researchers like Goldstein and Xie (2009) who
discussed the impact of the financial crisis on the Asian economies. The coefficients of the
lagged US market returns are positive for both the Indian and Chinese markets, which
means that the US stock market returns had a positive impact on the Indian and Chinese
markets.
Table 3. Wald Test: Stock Returns
Dependent Variable Explanatory Variable P-values
RNIFTY RSANDP 0.0000
RSSE 0.0068
RSANDP RNIFTY 0.1470
RSSE 0.4170
RSSE RNIFTY 0.4190
RSANDP 0.0001
Source: Authors’ calculations
Unauthenticated
Download Date | 2/23/19 2:23 PM
DOI: 10.1515/tjeb-2015-0012
Singh, A. & Kaur, P. (2015).
Stock Market Linkages: Evidence From the US, China and India During the Subprime Crisis
Timisoara Journal of Economics and Business | ISSN: 2286-0991 | www.tjeb.ro
Year 2015 | Volume 8 | Issue 1 | Pages: 137 – 162150
lagged values of the dependentas well as independentvariables on the dependent
variable. The lagged nature of the VAR model helps in analyzing the dynamic impact of the
independent variables on the dependent one.
The lagged values of the NIFTY returns are not statistically significant in influencing NIFTY
returns per se, whereas both the US and Chinese market returns statistically influence
NIFTY returns. The Chinese stock market returns had a negative impact on the Indian
market returns as the coefficient of the one day lagged variable is found to be negative. In
case of the US market returns, both the NIFTY and SSE are found to be insignificant, but the
lagged returns of the US market itself are found to be significant and, as expected, the
coefficients are negative for both the one day and two days lagged variables, thereby
highlighting the existence of the subprime crisis during that period. In case of the Chinese
market, only the one day lagged return in the US market statistically had an impact on the
China's stock market returns and the rest of the variables do not have a statistically
significant impact. The VAR model employed is found to be stationary, as all the inverse
roots lie inside the unit circle.
The results reported by the VAR model spotlight the existence of a crisis and spillover of the
returns during that period as the US market is having a significant impact on the emerging
markets. In order to explore the direction of spillovers, Wald Test has been performed (see
Table 3). The Wald test helps in judging the joint impact of the lagged independent variables
on the dependent variable. The results reported by the Wald test indicate the existence of a
unidirectional causality running from the US market to the Indian and Chinese market and
the results justify the findings of other researchers like Goldstein and Xie (2009) who
discussed the impact of the financial crisis on the Asian economies. The coefficients of the
lagged US market returns are positive for both the Indian and Chinese markets, which
means that the US stock market returns had a positive impact on the Indian and Chinese
markets.
Table 3. Wald Test: Stock Returns
Dependent Variable Explanatory Variable P-values
RNIFTY RSANDP 0.0000
RSSE 0.0068
RSANDP RNIFTY 0.1470
RSSE 0.4170
RSSE RNIFTY 0.4190
RSANDP 0.0001
Source: Authors’ calculations
Unauthenticated
Download Date | 2/23/19 2:23 PM
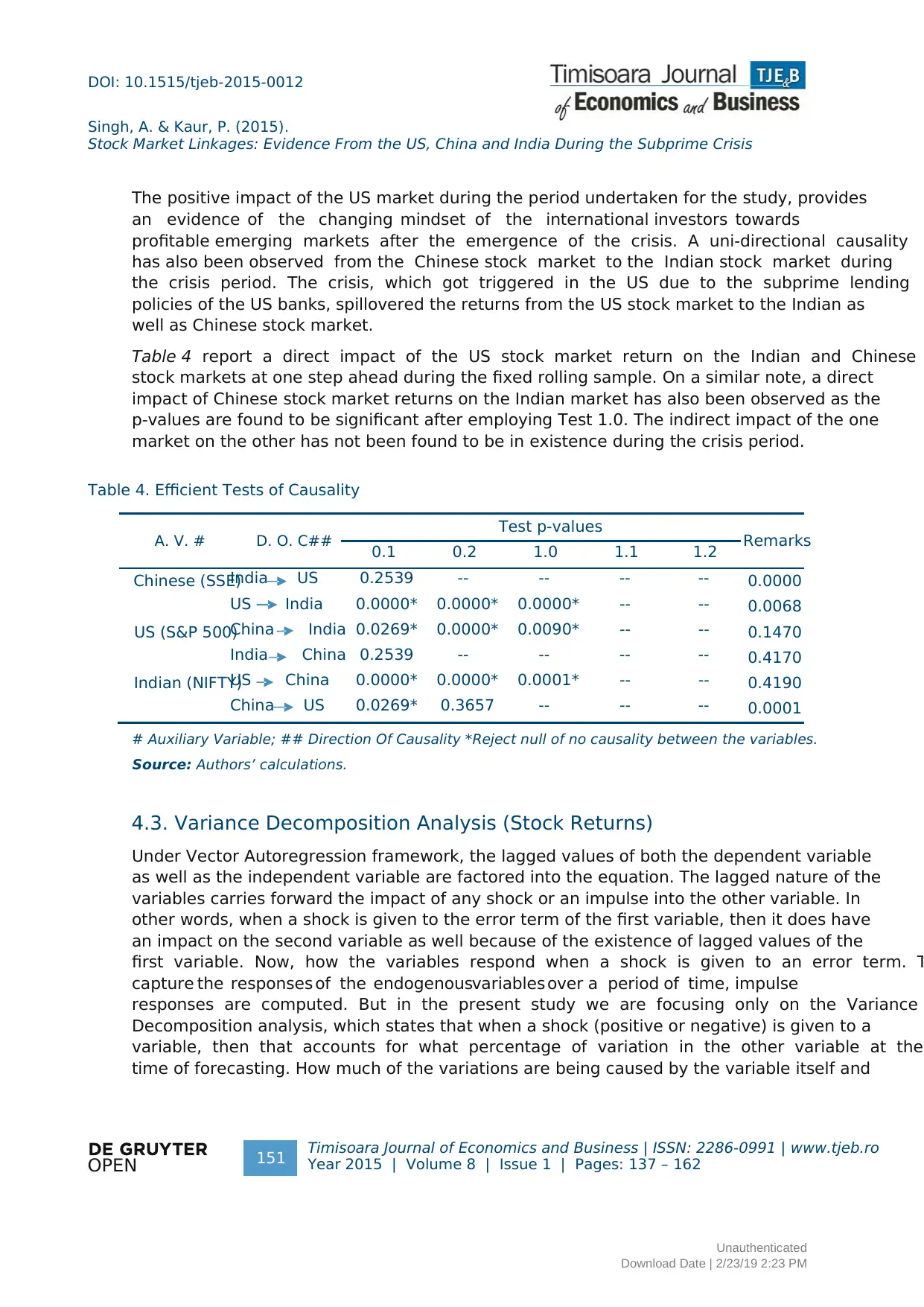
OPEN
DOI: 10.1515/tjeb-2015-0012
Singh, A. & Kaur, P. (2015).
Stock Market Linkages: Evidence From the US, China and India During the Subprime Crisis
Timisoara Journal of Economics and Business | ISSN: 2286-0991 | www.tjeb.ro
Year 2015 | Volume 8 | Issue 1 | Pages: 137 – 162151
The positive impact of the US market during the period undertaken for the study, provides
an evidence of the changing mindset of the international investors towards
profitable emerging markets after the emergence of the crisis. A uni-directional causality
has also been observed from the Chinese stock market to the Indian stock market during
the crisis period. The crisis, which got triggered in the US due to the subprime lending
policies of the US banks, spillovered the returns from the US stock market to the Indian as
well as Chinese stock market.
Table 4 report a direct impact of the US stock market return on the Indian and Chinese
stock markets at one step ahead during the fixed rolling sample. On a similar note, a direct
impact of Chinese stock market returns on the Indian market has also been observed as the
p-values are found to be significant after employing Test 1.0. The indirect impact of the one
market on the other has not been found to be in existence during the crisis period.
Table 4. Efficient Tests of Causality
A. V. # D. O. C## Test p-values Remarks
0.1 0.2 1.0 1.1 1.2
Chinese (SSE)India US 0.2539 -- -- -- -- 0.0000
US India 0.0000* 0.0000* 0.0000* -- -- 0.0068
US (S&P 500)China India 0.0269* 0.0000* 0.0090* -- -- 0.1470
India China 0.2539 -- -- -- -- 0.4170
Indian (NIFTY)US China 0.0000* 0.0000* 0.0001* -- -- 0.4190
China US 0.0269* 0.3657 -- -- -- 0.0001
# Auxiliary Variable; ## Direction Of Causality *Reject null of no causality between the variables.
Source: Authors’ calculations.
4.3. Variance Decomposition Analysis (Stock Returns)
Under Vector Autoregression framework, the lagged values of both the dependent variable
as well as the independent variable are factored into the equation. The lagged nature of the
variables carries forward the impact of any shock or an impulse into the other variable. In
other words, when a shock is given to the error term of the first variable, then it does have
an impact on the second variable as well because of the existence of lagged values of the
first variable. Now, how the variables respond when a shock is given to an error term. T
capture the responses of the endogenousvariables over a period of time, impulse
responses are computed. But in the present study we are focusing only on the Variance
Decomposition analysis, which states that when a shock (positive or negative) is given to a
variable, then that accounts for what percentage of variation in the other variable at the
time of forecasting. How much of the variations are being caused by the variable itself and
Unauthenticated
Download Date | 2/23/19 2:23 PM
DOI: 10.1515/tjeb-2015-0012
Singh, A. & Kaur, P. (2015).
Stock Market Linkages: Evidence From the US, China and India During the Subprime Crisis
Timisoara Journal of Economics and Business | ISSN: 2286-0991 | www.tjeb.ro
Year 2015 | Volume 8 | Issue 1 | Pages: 137 – 162151
The positive impact of the US market during the period undertaken for the study, provides
an evidence of the changing mindset of the international investors towards
profitable emerging markets after the emergence of the crisis. A uni-directional causality
has also been observed from the Chinese stock market to the Indian stock market during
the crisis period. The crisis, which got triggered in the US due to the subprime lending
policies of the US banks, spillovered the returns from the US stock market to the Indian as
well as Chinese stock market.
Table 4 report a direct impact of the US stock market return on the Indian and Chinese
stock markets at one step ahead during the fixed rolling sample. On a similar note, a direct
impact of Chinese stock market returns on the Indian market has also been observed as the
p-values are found to be significant after employing Test 1.0. The indirect impact of the one
market on the other has not been found to be in existence during the crisis period.
Table 4. Efficient Tests of Causality
A. V. # D. O. C## Test p-values Remarks
0.1 0.2 1.0 1.1 1.2
Chinese (SSE)India US 0.2539 -- -- -- -- 0.0000
US India 0.0000* 0.0000* 0.0000* -- -- 0.0068
US (S&P 500)China India 0.0269* 0.0000* 0.0090* -- -- 0.1470
India China 0.2539 -- -- -- -- 0.4170
Indian (NIFTY)US China 0.0000* 0.0000* 0.0001* -- -- 0.4190
China US 0.0269* 0.3657 -- -- -- 0.0001
# Auxiliary Variable; ## Direction Of Causality *Reject null of no causality between the variables.
Source: Authors’ calculations.
4.3. Variance Decomposition Analysis (Stock Returns)
Under Vector Autoregression framework, the lagged values of both the dependent variable
as well as the independent variable are factored into the equation. The lagged nature of the
variables carries forward the impact of any shock or an impulse into the other variable. In
other words, when a shock is given to the error term of the first variable, then it does have
an impact on the second variable as well because of the existence of lagged values of the
first variable. Now, how the variables respond when a shock is given to an error term. T
capture the responses of the endogenousvariables over a period of time, impulse
responses are computed. But in the present study we are focusing only on the Variance
Decomposition analysis, which states that when a shock (positive or negative) is given to a
variable, then that accounts for what percentage of variation in the other variable at the
time of forecasting. How much of the variations are being caused by the variable itself and
Unauthenticated
Download Date | 2/23/19 2:23 PM
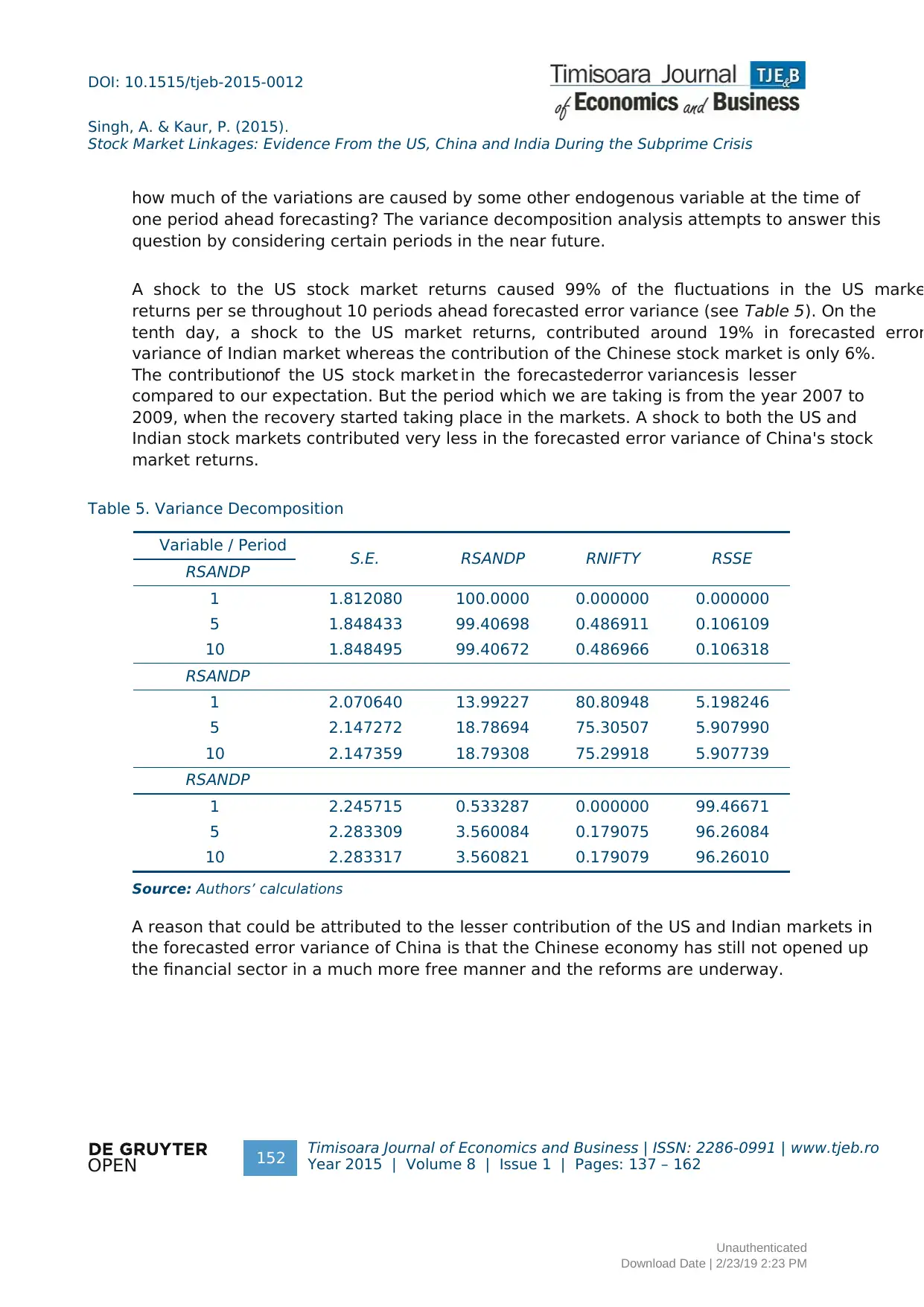
OPEN
DOI: 10.1515/tjeb-2015-0012
Singh, A. & Kaur, P. (2015).
Stock Market Linkages: Evidence From the US, China and India During the Subprime Crisis
Timisoara Journal of Economics and Business | ISSN: 2286-0991 | www.tjeb.ro
Year 2015 | Volume 8 | Issue 1 | Pages: 137 – 162152
how much of the variations are caused by some other endogenous variable at the time of
one period ahead forecasting? The variance decomposition analysis attempts to answer this
question by considering certain periods in the near future.
A shock to the US stock market returns caused 99% of the fluctuations in the US marke
returns per se throughout 10 periods ahead forecasted error variance (see Table 5). On the
tenth day, a shock to the US market returns, contributed around 19% in forecasted error
variance of Indian market whereas the contribution of the Chinese stock market is only 6%.
The contributionof the US stock market in the forecastederror variances is lesser
compared to our expectation. But the period which we are taking is from the year 2007 to
2009, when the recovery started taking place in the markets. A shock to both the US and
Indian stock markets contributed very less in the forecasted error variance of China's stock
market returns.
Table 5. Variance Decomposition
Variable / Period S.E. RSANDP RNIFTY RSSE
RSANDP
1 1.812080 100.0000 0.000000 0.000000
5 1.848433 99.40698 0.486911 0.106109
10 1.848495 99.40672 0.486966 0.106318
RSANDP
1 2.070640 13.99227 80.80948 5.198246
5 2.147272 18.78694 75.30507 5.907990
10 2.147359 18.79308 75.29918 5.907739
RSANDP
1 2.245715 0.533287 0.000000 99.46671
5 2.283309 3.560084 0.179075 96.26084
10 2.283317 3.560821 0.179079 96.26010
Source: Authors’ calculations
A reason that could be attributed to the lesser contribution of the US and Indian markets in
the forecasted error variance of China is that the Chinese economy has still not opened up
the financial sector in a much more free manner and the reforms are underway.
Unauthenticated
Download Date | 2/23/19 2:23 PM
DOI: 10.1515/tjeb-2015-0012
Singh, A. & Kaur, P. (2015).
Stock Market Linkages: Evidence From the US, China and India During the Subprime Crisis
Timisoara Journal of Economics and Business | ISSN: 2286-0991 | www.tjeb.ro
Year 2015 | Volume 8 | Issue 1 | Pages: 137 – 162152
how much of the variations are caused by some other endogenous variable at the time of
one period ahead forecasting? The variance decomposition analysis attempts to answer this
question by considering certain periods in the near future.
A shock to the US stock market returns caused 99% of the fluctuations in the US marke
returns per se throughout 10 periods ahead forecasted error variance (see Table 5). On the
tenth day, a shock to the US market returns, contributed around 19% in forecasted error
variance of Indian market whereas the contribution of the Chinese stock market is only 6%.
The contributionof the US stock market in the forecastederror variances is lesser
compared to our expectation. But the period which we are taking is from the year 2007 to
2009, when the recovery started taking place in the markets. A shock to both the US and
Indian stock markets contributed very less in the forecasted error variance of China's stock
market returns.
Table 5. Variance Decomposition
Variable / Period S.E. RSANDP RNIFTY RSSE
RSANDP
1 1.812080 100.0000 0.000000 0.000000
5 1.848433 99.40698 0.486911 0.106109
10 1.848495 99.40672 0.486966 0.106318
RSANDP
1 2.070640 13.99227 80.80948 5.198246
5 2.147272 18.78694 75.30507 5.907990
10 2.147359 18.79308 75.29918 5.907739
RSANDP
1 2.245715 0.533287 0.000000 99.46671
5 2.283309 3.560084 0.179075 96.26084
10 2.283317 3.560821 0.179079 96.26010
Source: Authors’ calculations
A reason that could be attributed to the lesser contribution of the US and Indian markets in
the forecasted error variance of China is that the Chinese economy has still not opened up
the financial sector in a much more free manner and the reforms are underway.
Unauthenticated
Download Date | 2/23/19 2:23 PM
Paraphrase This Document
Need a fresh take? Get an instant paraphrase of this document with our AI Paraphraser
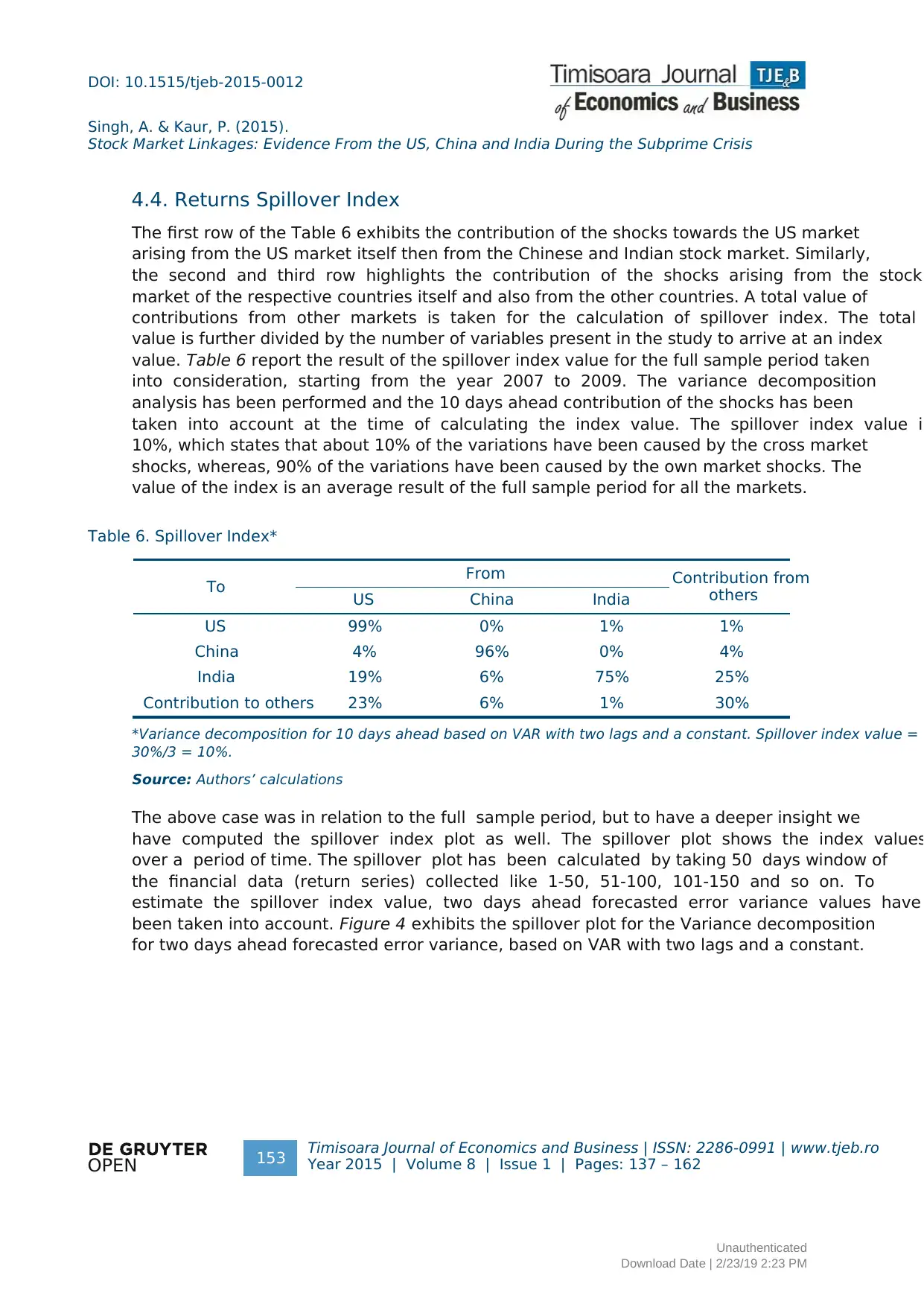
OPEN
DOI: 10.1515/tjeb-2015-0012
Singh, A. & Kaur, P. (2015).
Stock Market Linkages: Evidence From the US, China and India During the Subprime Crisis
Timisoara Journal of Economics and Business | ISSN: 2286-0991 | www.tjeb.ro
Year 2015 | Volume 8 | Issue 1 | Pages: 137 – 162153
4.4. Returns Spillover Index
The first row of the Table 6 exhibits the contribution of the shocks towards the US market
arising from the US market itself then from the Chinese and Indian stock market. Similarly,
the second and third row highlights the contribution of the shocks arising from the stock
market of the respective countries itself and also from the other countries. A total value of
contributions from other markets is taken for the calculation of spillover index. The total
value is further divided by the number of variables present in the study to arrive at an index
value. Table 6 report the result of the spillover index value for the full sample period taken
into consideration, starting from the year 2007 to 2009. The variance decomposition
analysis has been performed and the 10 days ahead contribution of the shocks has been
taken into account at the time of calculating the index value. The spillover index value is
10%, which states that about 10% of the variations have been caused by the cross market
shocks, whereas, 90% of the variations have been caused by the own market shocks. The
value of the index is an average result of the full sample period for all the markets.
Table 6. Spillover Index*
To From Contribution from
othersUS China India
US 99% 0% 1% 1%
China 4% 96% 0% 4%
India 19% 6% 75% 25%
Contribution to others 23% 6% 1% 30%
*Variance decomposition for 10 days ahead based on VAR with two lags and a constant. Spillover index value =
30%/3 = 10%.
Source: Authors’ calculations
The above case was in relation to the full sample period, but to have a deeper insight we
have computed the spillover index plot as well. The spillover plot shows the index values
over a period of time. The spillover plot has been calculated by taking 50 days window of
the financial data (return series) collected like 1-50, 51-100, 101-150 and so on. To
estimate the spillover index value, two days ahead forecasted error variance values have
been taken into account. Figure 4 exhibits the spillover plot for the Variance decomposition
for two days ahead forecasted error variance, based on VAR with two lags and a constant.
Unauthenticated
Download Date | 2/23/19 2:23 PM
DOI: 10.1515/tjeb-2015-0012
Singh, A. & Kaur, P. (2015).
Stock Market Linkages: Evidence From the US, China and India During the Subprime Crisis
Timisoara Journal of Economics and Business | ISSN: 2286-0991 | www.tjeb.ro
Year 2015 | Volume 8 | Issue 1 | Pages: 137 – 162153
4.4. Returns Spillover Index
The first row of the Table 6 exhibits the contribution of the shocks towards the US market
arising from the US market itself then from the Chinese and Indian stock market. Similarly,
the second and third row highlights the contribution of the shocks arising from the stock
market of the respective countries itself and also from the other countries. A total value of
contributions from other markets is taken for the calculation of spillover index. The total
value is further divided by the number of variables present in the study to arrive at an index
value. Table 6 report the result of the spillover index value for the full sample period taken
into consideration, starting from the year 2007 to 2009. The variance decomposition
analysis has been performed and the 10 days ahead contribution of the shocks has been
taken into account at the time of calculating the index value. The spillover index value is
10%, which states that about 10% of the variations have been caused by the cross market
shocks, whereas, 90% of the variations have been caused by the own market shocks. The
value of the index is an average result of the full sample period for all the markets.
Table 6. Spillover Index*
To From Contribution from
othersUS China India
US 99% 0% 1% 1%
China 4% 96% 0% 4%
India 19% 6% 75% 25%
Contribution to others 23% 6% 1% 30%
*Variance decomposition for 10 days ahead based on VAR with two lags and a constant. Spillover index value =
30%/3 = 10%.
Source: Authors’ calculations
The above case was in relation to the full sample period, but to have a deeper insight we
have computed the spillover index plot as well. The spillover plot shows the index values
over a period of time. The spillover plot has been calculated by taking 50 days window of
the financial data (return series) collected like 1-50, 51-100, 101-150 and so on. To
estimate the spillover index value, two days ahead forecasted error variance values have
been taken into account. Figure 4 exhibits the spillover plot for the Variance decomposition
for two days ahead forecasted error variance, based on VAR with two lags and a constant.
Unauthenticated
Download Date | 2/23/19 2:23 PM
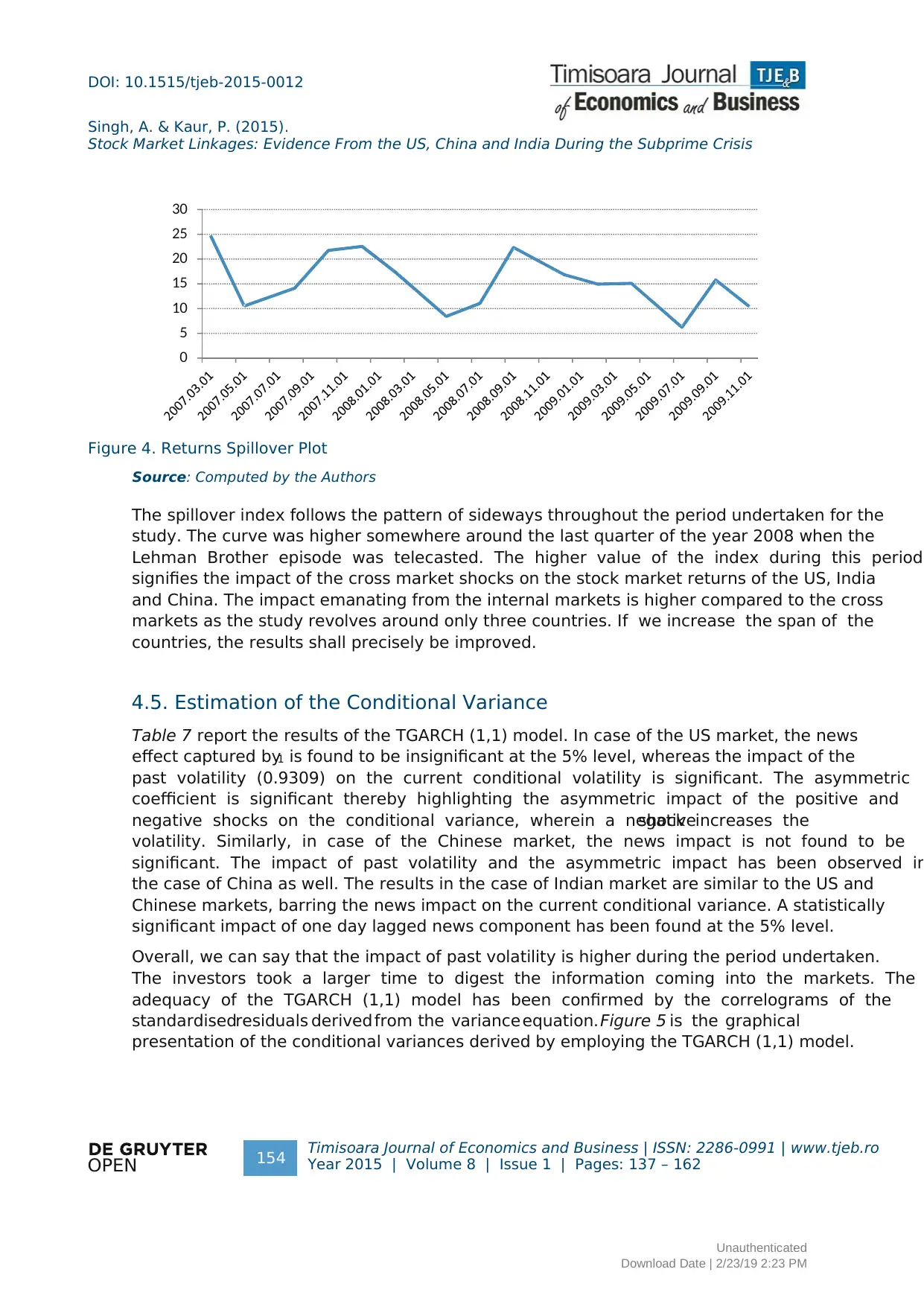
OPEN
DOI: 10.1515/tjeb-2015-0012
Singh, A. & Kaur, P. (2015).
Stock Market Linkages: Evidence From the US, China and India During the Subprime Crisis
Timisoara Journal of Economics and Business | ISSN: 2286-0991 | www.tjeb.ro
Year 2015 | Volume 8 | Issue 1 | Pages: 137 – 162154
Figure 4. Returns Spillover Plot
Source: Computed by the Authors
The spillover index follows the pattern of sideways throughout the period undertaken for the
study. The curve was higher somewhere around the last quarter of the year 2008 when the
Lehman Brother episode was telecasted. The higher value of the index during this period
signifies the impact of the cross market shocks on the stock market returns of the US, India
and China. The impact emanating from the internal markets is higher compared to the cross
markets as the study revolves around only three countries. If we increase the span of the
countries, the results shall precisely be improved.
4.5. Estimation of the Conditional Variance
Table 7 report the results of the TGARCH (1,1) model. In case of the US market, the news
effect captured by1 is found to be insignificant at the 5% level, whereas the impact of the
past volatility (0.9309) on the current conditional volatility is significant. The asymmetric
coefficient is significant thereby highlighting the asymmetric impact of the positive and
negative shocks on the conditional variance, wherein a negativeshock increases the
volatility. Similarly, in case of the Chinese market, the news impact is not found to be
significant. The impact of past volatility and the asymmetric impact has been observed in
the case of China as well. The results in the case of Indian market are similar to the US and
Chinese markets, barring the news impact on the current conditional variance. A statistically
significant impact of one day lagged news component has been found at the 5% level.
Overall, we can say that the impact of past volatility is higher during the period undertaken.
The investors took a larger time to digest the information coming into the markets. The
adequacy of the TGARCH (1,1) model has been confirmed by the correlograms of the
standardisedresiduals derived from the variance equation. Figure 5 is the graphical
presentation of the conditional variances derived by employing the TGARCH (1,1) model.
0
5
10
15
20
25
30
Unauthenticated
Download Date | 2/23/19 2:23 PM
DOI: 10.1515/tjeb-2015-0012
Singh, A. & Kaur, P. (2015).
Stock Market Linkages: Evidence From the US, China and India During the Subprime Crisis
Timisoara Journal of Economics and Business | ISSN: 2286-0991 | www.tjeb.ro
Year 2015 | Volume 8 | Issue 1 | Pages: 137 – 162154
Figure 4. Returns Spillover Plot
Source: Computed by the Authors
The spillover index follows the pattern of sideways throughout the period undertaken for the
study. The curve was higher somewhere around the last quarter of the year 2008 when the
Lehman Brother episode was telecasted. The higher value of the index during this period
signifies the impact of the cross market shocks on the stock market returns of the US, India
and China. The impact emanating from the internal markets is higher compared to the cross
markets as the study revolves around only three countries. If we increase the span of the
countries, the results shall precisely be improved.
4.5. Estimation of the Conditional Variance
Table 7 report the results of the TGARCH (1,1) model. In case of the US market, the news
effect captured by1 is found to be insignificant at the 5% level, whereas the impact of the
past volatility (0.9309) on the current conditional volatility is significant. The asymmetric
coefficient is significant thereby highlighting the asymmetric impact of the positive and
negative shocks on the conditional variance, wherein a negativeshock increases the
volatility. Similarly, in case of the Chinese market, the news impact is not found to be
significant. The impact of past volatility and the asymmetric impact has been observed in
the case of China as well. The results in the case of Indian market are similar to the US and
Chinese markets, barring the news impact on the current conditional variance. A statistically
significant impact of one day lagged news component has been found at the 5% level.
Overall, we can say that the impact of past volatility is higher during the period undertaken.
The investors took a larger time to digest the information coming into the markets. The
adequacy of the TGARCH (1,1) model has been confirmed by the correlograms of the
standardisedresiduals derived from the variance equation. Figure 5 is the graphical
presentation of the conditional variances derived by employing the TGARCH (1,1) model.
0
5
10
15
20
25
30
Unauthenticated
Download Date | 2/23/19 2:23 PM
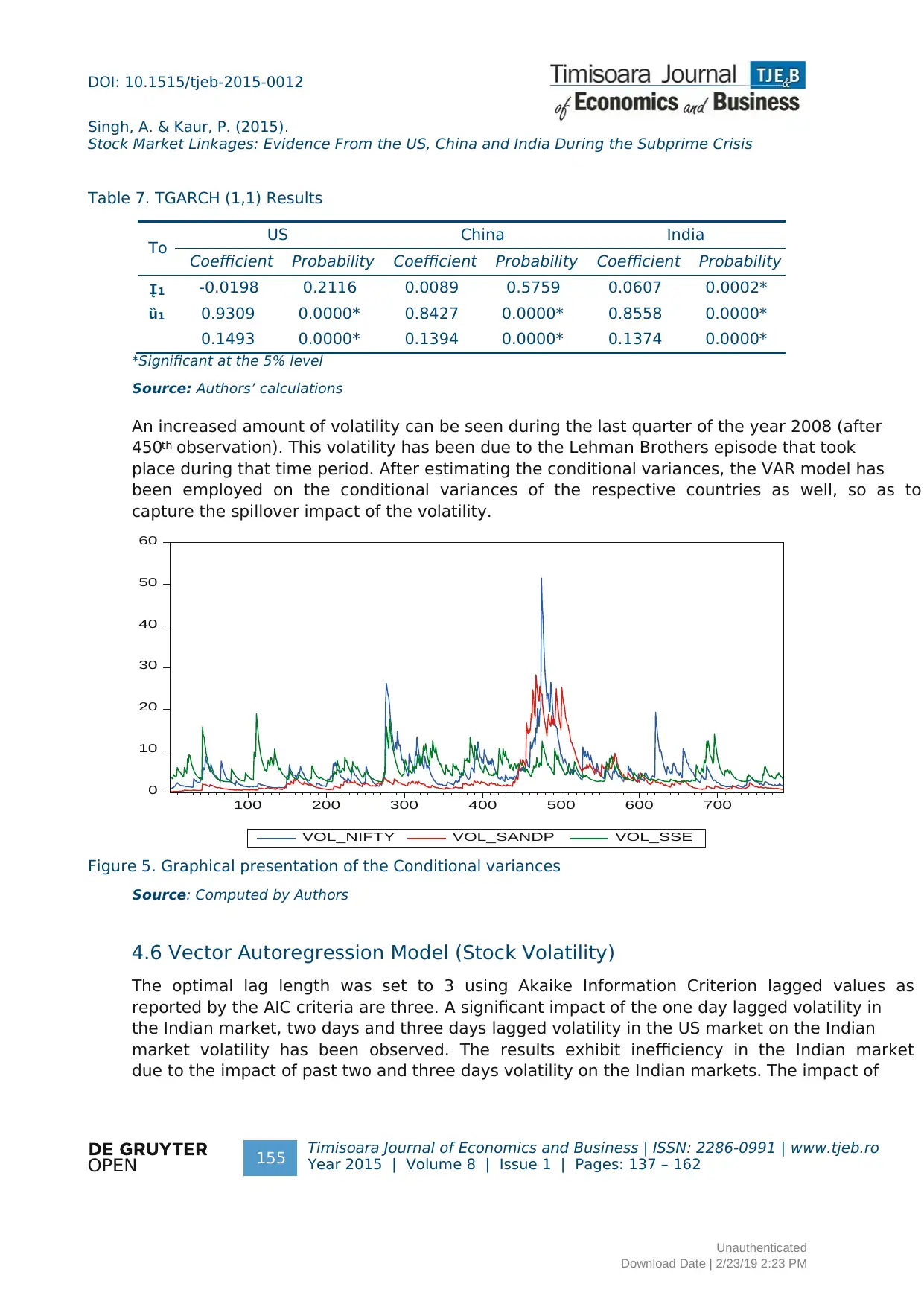
OPEN
DOI: 10.1515/tjeb-2015-0012
Singh, A. & Kaur, P. (2015).
Stock Market Linkages: Evidence From the US, China and India During the Subprime Crisis
Timisoara Journal of Economics and Business | ISSN: 2286-0991 | www.tjeb.ro
Year 2015 | Volume 8 | Issue 1 | Pages: 137 – 162155
Table 7. TGARCH (1,1) Results
To US China India
Coefficient Probability Coefficient Probability Coefficient Probability
Į1 -0.0198 0.2116 0.0089 0.5759 0.0607 0.0002*
ȕ1 0.9309 0.0000* 0.8427 0.0000* 0.8558 0.0000*
0.1493 0.0000* 0.1394 0.0000* 0.1374 0.0000*
*Significant at the 5% level
Source: Authors’ calculations
An increased amount of volatility can be seen during the last quarter of the year 2008 (after
450th observation). This volatility has been due to the Lehman Brothers episode that took
place during that time period. After estimating the conditional variances, the VAR model has
been employed on the conditional variances of the respective countries as well, so as to
capture the spillover impact of the volatility.
Figure 5. Graphical presentation of the Conditional variances
Source: Computed by Authors
4.6 Vector Autoregression Model (Stock Volatility)
The optimal lag length was set to 3 using Akaike Information Criterion lagged values as
reported by the AIC criteria are three. A significant impact of the one day lagged volatility in
the Indian market, two days and three days lagged volatility in the US market on the Indian
market volatility has been observed. The results exhibit inefficiency in the Indian market
due to the impact of past two and three days volatility on the Indian markets. The impact of
0
10
20
30
40
50
60
100 200 300 400 500 600 700
VOL_NIFTY VOL_SANDP VOL_SSE
Unauthenticated
Download Date | 2/23/19 2:23 PM
DOI: 10.1515/tjeb-2015-0012
Singh, A. & Kaur, P. (2015).
Stock Market Linkages: Evidence From the US, China and India During the Subprime Crisis
Timisoara Journal of Economics and Business | ISSN: 2286-0991 | www.tjeb.ro
Year 2015 | Volume 8 | Issue 1 | Pages: 137 – 162155
Table 7. TGARCH (1,1) Results
To US China India
Coefficient Probability Coefficient Probability Coefficient Probability
Į1 -0.0198 0.2116 0.0089 0.5759 0.0607 0.0002*
ȕ1 0.9309 0.0000* 0.8427 0.0000* 0.8558 0.0000*
0.1493 0.0000* 0.1394 0.0000* 0.1374 0.0000*
*Significant at the 5% level
Source: Authors’ calculations
An increased amount of volatility can be seen during the last quarter of the year 2008 (after
450th observation). This volatility has been due to the Lehman Brothers episode that took
place during that time period. After estimating the conditional variances, the VAR model has
been employed on the conditional variances of the respective countries as well, so as to
capture the spillover impact of the volatility.
Figure 5. Graphical presentation of the Conditional variances
Source: Computed by Authors
4.6 Vector Autoregression Model (Stock Volatility)
The optimal lag length was set to 3 using Akaike Information Criterion lagged values as
reported by the AIC criteria are three. A significant impact of the one day lagged volatility in
the Indian market, two days and three days lagged volatility in the US market on the Indian
market volatility has been observed. The results exhibit inefficiency in the Indian market
due to the impact of past two and three days volatility on the Indian markets. The impact of
0
10
20
30
40
50
60
100 200 300 400 500 600 700
VOL_NIFTY VOL_SANDP VOL_SSE
Unauthenticated
Download Date | 2/23/19 2:23 PM
Secure Best Marks with AI Grader
Need help grading? Try our AI Grader for instant feedback on your assignments.
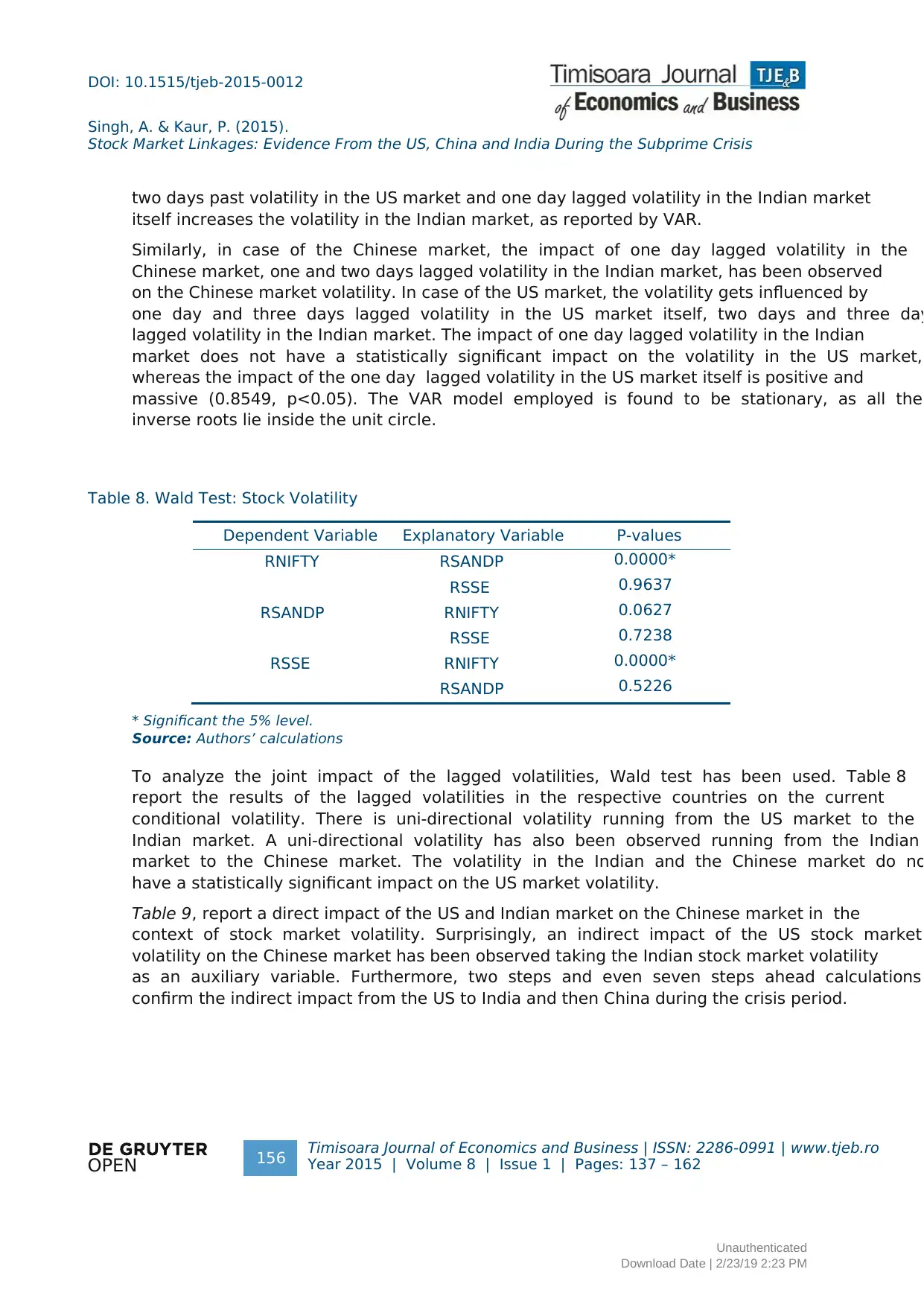
OPEN
DOI: 10.1515/tjeb-2015-0012
Singh, A. & Kaur, P. (2015).
Stock Market Linkages: Evidence From the US, China and India During the Subprime Crisis
Timisoara Journal of Economics and Business | ISSN: 2286-0991 | www.tjeb.ro
Year 2015 | Volume 8 | Issue 1 | Pages: 137 – 162156
two days past volatility in the US market and one day lagged volatility in the Indian market
itself increases the volatility in the Indian market, as reported by VAR.
Similarly, in case of the Chinese market, the impact of one day lagged volatility in the
Chinese market, one and two days lagged volatility in the Indian market, has been observed
on the Chinese market volatility. In case of the US market, the volatility gets influenced by
one day and three days lagged volatility in the US market itself, two days and three day
lagged volatility in the Indian market. The impact of one day lagged volatility in the Indian
market does not have a statistically significant impact on the volatility in the US market,
whereas the impact of the one day lagged volatility in the US market itself is positive and
massive (0.8549, p<0.05). The VAR model employed is found to be stationary, as all the
inverse roots lie inside the unit circle.
Table 8. Wald Test: Stock Volatility
Dependent Variable Explanatory Variable P-values
RNIFTY RSANDP 0.0000*
RSSE 0.9637
RSANDP RNIFTY 0.0627
RSSE 0.7238
RSSE RNIFTY 0.0000*
RSANDP 0.5226
* Significant the 5% level.
Source: Authors’ calculations
To analyze the joint impact of the lagged volatilities, Wald test has been used. Table 8
report the results of the lagged volatilities in the respective countries on the current
conditional volatility. There is uni-directional volatility running from the US market to the
Indian market. A uni-directional volatility has also been observed running from the Indian
market to the Chinese market. The volatility in the Indian and the Chinese market do no
have a statistically significant impact on the US market volatility.
Table 9, report a direct impact of the US and Indian market on the Chinese market in the
context of stock market volatility. Surprisingly, an indirect impact of the US stock market
volatility on the Chinese market has been observed taking the Indian stock market volatility
as an auxiliary variable. Furthermore, two steps and even seven steps ahead calculations
confirm the indirect impact from the US to India and then China during the crisis period.
Unauthenticated
Download Date | 2/23/19 2:23 PM
DOI: 10.1515/tjeb-2015-0012
Singh, A. & Kaur, P. (2015).
Stock Market Linkages: Evidence From the US, China and India During the Subprime Crisis
Timisoara Journal of Economics and Business | ISSN: 2286-0991 | www.tjeb.ro
Year 2015 | Volume 8 | Issue 1 | Pages: 137 – 162156
two days past volatility in the US market and one day lagged volatility in the Indian market
itself increases the volatility in the Indian market, as reported by VAR.
Similarly, in case of the Chinese market, the impact of one day lagged volatility in the
Chinese market, one and two days lagged volatility in the Indian market, has been observed
on the Chinese market volatility. In case of the US market, the volatility gets influenced by
one day and three days lagged volatility in the US market itself, two days and three day
lagged volatility in the Indian market. The impact of one day lagged volatility in the Indian
market does not have a statistically significant impact on the volatility in the US market,
whereas the impact of the one day lagged volatility in the US market itself is positive and
massive (0.8549, p<0.05). The VAR model employed is found to be stationary, as all the
inverse roots lie inside the unit circle.
Table 8. Wald Test: Stock Volatility
Dependent Variable Explanatory Variable P-values
RNIFTY RSANDP 0.0000*
RSSE 0.9637
RSANDP RNIFTY 0.0627
RSSE 0.7238
RSSE RNIFTY 0.0000*
RSANDP 0.5226
* Significant the 5% level.
Source: Authors’ calculations
To analyze the joint impact of the lagged volatilities, Wald test has been used. Table 8
report the results of the lagged volatilities in the respective countries on the current
conditional volatility. There is uni-directional volatility running from the US market to the
Indian market. A uni-directional volatility has also been observed running from the Indian
market to the Chinese market. The volatility in the Indian and the Chinese market do no
have a statistically significant impact on the US market volatility.
Table 9, report a direct impact of the US and Indian market on the Chinese market in the
context of stock market volatility. Surprisingly, an indirect impact of the US stock market
volatility on the Chinese market has been observed taking the Indian stock market volatility
as an auxiliary variable. Furthermore, two steps and even seven steps ahead calculations
confirm the indirect impact from the US to India and then China during the crisis period.
Unauthenticated
Download Date | 2/23/19 2:23 PM
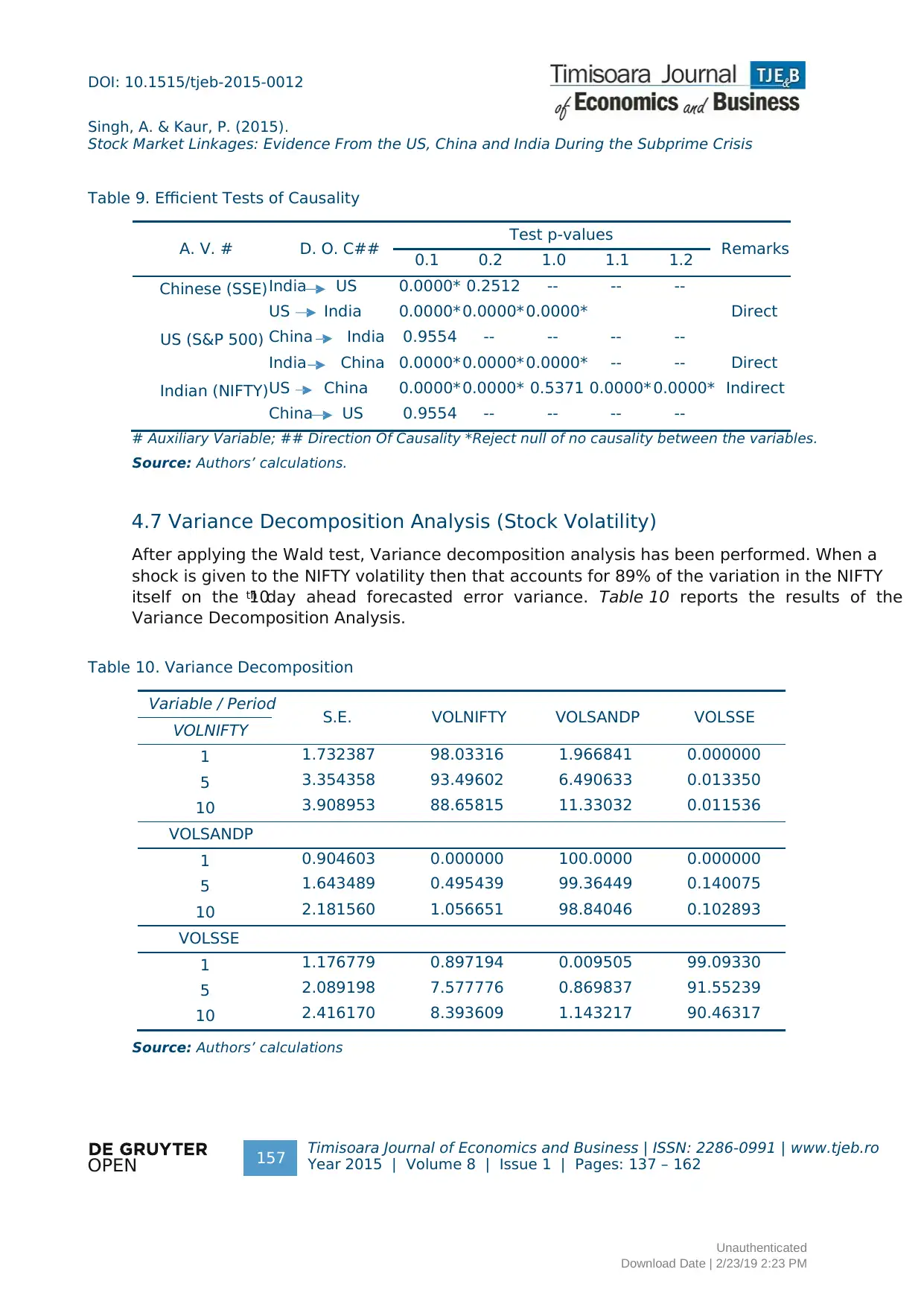
OPEN
DOI: 10.1515/tjeb-2015-0012
Singh, A. & Kaur, P. (2015).
Stock Market Linkages: Evidence From the US, China and India During the Subprime Crisis
Timisoara Journal of Economics and Business | ISSN: 2286-0991 | www.tjeb.ro
Year 2015 | Volume 8 | Issue 1 | Pages: 137 – 162157
Table 9. Efficient Tests of Causality
A. V. # D. O. C## Test p-values Remarks
0.1 0.2 1.0 1.1 1.2
Chinese (SSE) India US 0.0000* 0.2512 -- -- --
US India 0.0000*0.0000* 0.0000* Direct
US (S&P 500) China India 0.9554 -- -- -- --
India China 0.0000*0.0000* 0.0000* -- -- Direct
Indian (NIFTY)US China 0.0000*0.0000* 0.5371 0.0000* 0.0000* Indirect
China US 0.9554 -- -- -- --
# Auxiliary Variable; ## Direction Of Causality *Reject null of no causality between the variables.
Source: Authors’ calculations.
4.7 Variance Decomposition Analysis (Stock Volatility)
After applying the Wald test, Variance decomposition analysis has been performed. When a
shock is given to the NIFTY volatility then that accounts for 89% of the variation in the NIFTY
itself on the 10th day ahead forecasted error variance. Table 10 reports the results of the
Variance Decomposition Analysis.
Table 10. Variance Decomposition
Variable / Period S.E. VOLNIFTY VOLSANDP VOLSSE
VOLNIFTY
1 1.732387 98.03316 1.966841 0.000000
5 3.354358 93.49602 6.490633 0.013350
10 3.908953 88.65815 11.33032 0.011536
VOLSANDP
1 0.904603 0.000000 100.0000 0.000000
5 1.643489 0.495439 99.36449 0.140075
10 2.181560 1.056651 98.84046 0.102893
VOLSSE
1 1.176779 0.897194 0.009505 99.09330
5 2.089198 7.577776 0.869837 91.55239
10 2.416170 8.393609 1.143217 90.46317
Source: Authors’ calculations
Unauthenticated
Download Date | 2/23/19 2:23 PM
DOI: 10.1515/tjeb-2015-0012
Singh, A. & Kaur, P. (2015).
Stock Market Linkages: Evidence From the US, China and India During the Subprime Crisis
Timisoara Journal of Economics and Business | ISSN: 2286-0991 | www.tjeb.ro
Year 2015 | Volume 8 | Issue 1 | Pages: 137 – 162157
Table 9. Efficient Tests of Causality
A. V. # D. O. C## Test p-values Remarks
0.1 0.2 1.0 1.1 1.2
Chinese (SSE) India US 0.0000* 0.2512 -- -- --
US India 0.0000*0.0000* 0.0000* Direct
US (S&P 500) China India 0.9554 -- -- -- --
India China 0.0000*0.0000* 0.0000* -- -- Direct
Indian (NIFTY)US China 0.0000*0.0000* 0.5371 0.0000* 0.0000* Indirect
China US 0.9554 -- -- -- --
# Auxiliary Variable; ## Direction Of Causality *Reject null of no causality between the variables.
Source: Authors’ calculations.
4.7 Variance Decomposition Analysis (Stock Volatility)
After applying the Wald test, Variance decomposition analysis has been performed. When a
shock is given to the NIFTY volatility then that accounts for 89% of the variation in the NIFTY
itself on the 10th day ahead forecasted error variance. Table 10 reports the results of the
Variance Decomposition Analysis.
Table 10. Variance Decomposition
Variable / Period S.E. VOLNIFTY VOLSANDP VOLSSE
VOLNIFTY
1 1.732387 98.03316 1.966841 0.000000
5 3.354358 93.49602 6.490633 0.013350
10 3.908953 88.65815 11.33032 0.011536
VOLSANDP
1 0.904603 0.000000 100.0000 0.000000
5 1.643489 0.495439 99.36449 0.140075
10 2.181560 1.056651 98.84046 0.102893
VOLSSE
1 1.176779 0.897194 0.009505 99.09330
5 2.089198 7.577776 0.869837 91.55239
10 2.416170 8.393609 1.143217 90.46317
Source: Authors’ calculations
Unauthenticated
Download Date | 2/23/19 2:23 PM
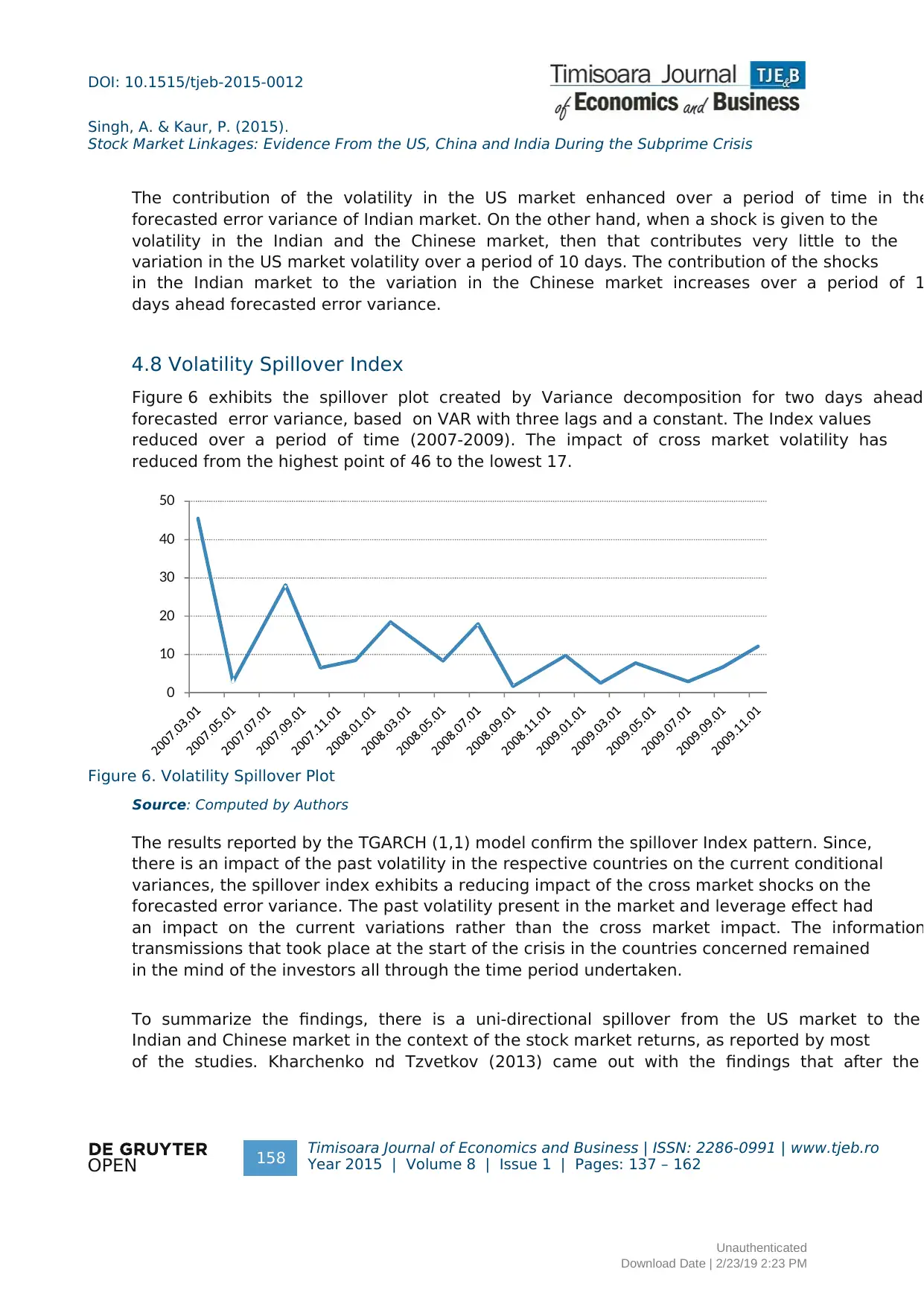
OPEN
DOI: 10.1515/tjeb-2015-0012
Singh, A. & Kaur, P. (2015).
Stock Market Linkages: Evidence From the US, China and India During the Subprime Crisis
Timisoara Journal of Economics and Business | ISSN: 2286-0991 | www.tjeb.ro
Year 2015 | Volume 8 | Issue 1 | Pages: 137 – 162158
The contribution of the volatility in the US market enhanced over a period of time in the
forecasted error variance of Indian market. On the other hand, when a shock is given to the
volatility in the Indian and the Chinese market, then that contributes very little to the
variation in the US market volatility over a period of 10 days. The contribution of the shocks
in the Indian market to the variation in the Chinese market increases over a period of 1
days ahead forecasted error variance.
4.8 Volatility Spillover Index
Figure 6 exhibits the spillover plot created by Variance decomposition for two days ahead
forecasted error variance, based on VAR with three lags and a constant. The Index values
reduced over a period of time (2007-2009). The impact of cross market volatility has
reduced from the highest point of 46 to the lowest 17.
Figure 6. Volatility Spillover Plot
Source: Computed by Authors
The results reported by the TGARCH (1,1) model confirm the spillover Index pattern. Since,
there is an impact of the past volatility in the respective countries on the current conditional
variances, the spillover index exhibits a reducing impact of the cross market shocks on the
forecasted error variance. The past volatility present in the market and leverage effect had
an impact on the current variations rather than the cross market impact. The information
transmissions that took place at the start of the crisis in the countries concerned remained
in the mind of the investors all through the time period undertaken.
To summarize the findings, there is a uni-directional spillover from the US market to the
Indian and Chinese market in the context of the stock market returns, as reported by most
of the studies. Kharchenko nd Tzvetkov (2013) came out with the findings that after the
0
10
20
30
40
50
Unauthenticated
Download Date | 2/23/19 2:23 PM
DOI: 10.1515/tjeb-2015-0012
Singh, A. & Kaur, P. (2015).
Stock Market Linkages: Evidence From the US, China and India During the Subprime Crisis
Timisoara Journal of Economics and Business | ISSN: 2286-0991 | www.tjeb.ro
Year 2015 | Volume 8 | Issue 1 | Pages: 137 – 162158
The contribution of the volatility in the US market enhanced over a period of time in the
forecasted error variance of Indian market. On the other hand, when a shock is given to the
volatility in the Indian and the Chinese market, then that contributes very little to the
variation in the US market volatility over a period of 10 days. The contribution of the shocks
in the Indian market to the variation in the Chinese market increases over a period of 1
days ahead forecasted error variance.
4.8 Volatility Spillover Index
Figure 6 exhibits the spillover plot created by Variance decomposition for two days ahead
forecasted error variance, based on VAR with three lags and a constant. The Index values
reduced over a period of time (2007-2009). The impact of cross market volatility has
reduced from the highest point of 46 to the lowest 17.
Figure 6. Volatility Spillover Plot
Source: Computed by Authors
The results reported by the TGARCH (1,1) model confirm the spillover Index pattern. Since,
there is an impact of the past volatility in the respective countries on the current conditional
variances, the spillover index exhibits a reducing impact of the cross market shocks on the
forecasted error variance. The past volatility present in the market and leverage effect had
an impact on the current variations rather than the cross market impact. The information
transmissions that took place at the start of the crisis in the countries concerned remained
in the mind of the investors all through the time period undertaken.
To summarize the findings, there is a uni-directional spillover from the US market to the
Indian and Chinese market in the context of the stock market returns, as reported by most
of the studies. Kharchenko nd Tzvetkov (2013) came out with the findings that after the
0
10
20
30
40
50
Unauthenticated
Download Date | 2/23/19 2:23 PM
Paraphrase This Document
Need a fresh take? Get an instant paraphrase of this document with our AI Paraphraser
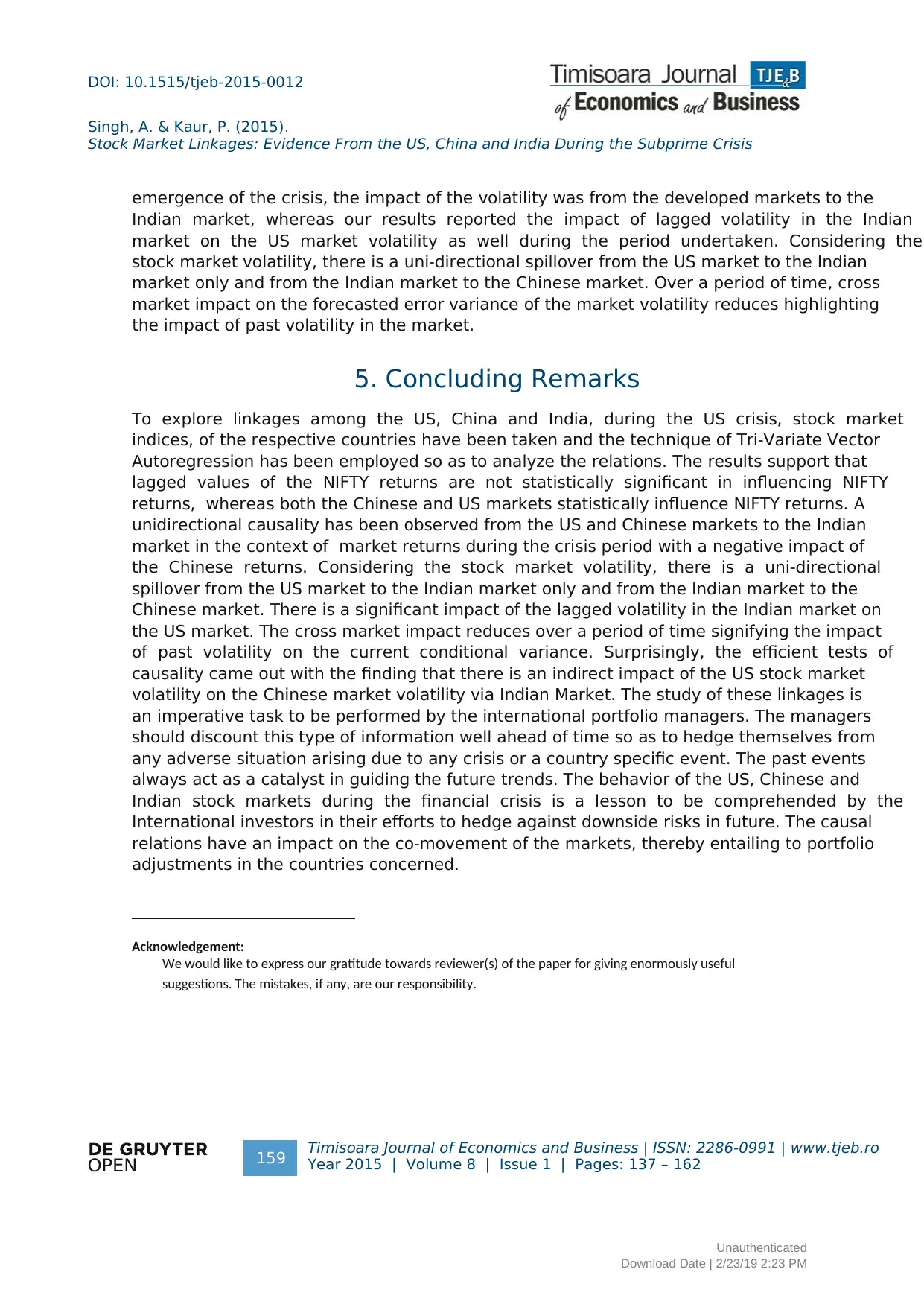
OPEN
DOI: 10.1515/tjeb-2015-0012
Singh, A. & Kaur, P. (2015).
Stock Market Linkages: Evidence From the US, China and India During the Subprime Crisis
Timisoara Journal of Economics and Business | ISSN: 2286-0991 | www.tjeb.ro
Year 2015 | Volume 8 | Issue 1 | Pages: 137 – 162159
emergence of the crisis, the impact of the volatility was from the developed markets to the
Indian market, whereas our results reported the impact of lagged volatility in the Indian
market on the US market volatility as well during the period undertaken. Considering the
stock market volatility, there is a uni-directional spillover from the US market to the Indian
market only and from the Indian market to the Chinese market. Over a period of time, cross
market impact on the forecasted error variance of the market volatility reduces highlighting
the impact of past volatility in the market.
5. Concluding Remarks
To explore linkages among the US, China and India, during the US crisis, stock market
indices, of the respective countries have been taken and the technique of Tri-Variate Vector
Autoregression has been employed so as to analyze the relations. The results support that
lagged values of the NIFTY returns are not statistically significant in influencing NIFTY
returns, whereas both the Chinese and US markets statistically influence NIFTY returns. A
unidirectional causality has been observed from the US and Chinese markets to the Indian
market in the context of market returns during the crisis period with a negative impact of
the Chinese returns. Considering the stock market volatility, there is a uni-directional
spillover from the US market to the Indian market only and from the Indian market to the
Chinese market. There is a significant impact of the lagged volatility in the Indian market on
the US market. The cross market impact reduces over a period of time signifying the impact
of past volatility on the current conditional variance. Surprisingly, the efficient tests of
causality came out with the finding that there is an indirect impact of the US stock market
volatility on the Chinese market volatility via Indian Market. The study of these linkages is
an imperative task to be performed by the international portfolio managers. The managers
should discount this type of information well ahead of time so as to hedge themselves from
any adverse situation arising due to any crisis or a country specific event. The past events
always act as a catalyst in guiding the future trends. The behavior of the US, Chinese and
Indian stock markets during the financial crisis is a lesson to be comprehended by the
International investors in their efforts to hedge against downside risks in future. The causal
relations have an impact on the co-movement of the markets, thereby entailing to portfolio
adjustments in the countries concerned.
Acknowledgement:
We would like to express our gratitude towards reviewer(s) of the paper for giving enormously useful
suggestions. The mistakes, if any, are our responsibility.
Unauthenticated
Download Date | 2/23/19 2:23 PM
DOI: 10.1515/tjeb-2015-0012
Singh, A. & Kaur, P. (2015).
Stock Market Linkages: Evidence From the US, China and India During the Subprime Crisis
Timisoara Journal of Economics and Business | ISSN: 2286-0991 | www.tjeb.ro
Year 2015 | Volume 8 | Issue 1 | Pages: 137 – 162159
emergence of the crisis, the impact of the volatility was from the developed markets to the
Indian market, whereas our results reported the impact of lagged volatility in the Indian
market on the US market volatility as well during the period undertaken. Considering the
stock market volatility, there is a uni-directional spillover from the US market to the Indian
market only and from the Indian market to the Chinese market. Over a period of time, cross
market impact on the forecasted error variance of the market volatility reduces highlighting
the impact of past volatility in the market.
5. Concluding Remarks
To explore linkages among the US, China and India, during the US crisis, stock market
indices, of the respective countries have been taken and the technique of Tri-Variate Vector
Autoregression has been employed so as to analyze the relations. The results support that
lagged values of the NIFTY returns are not statistically significant in influencing NIFTY
returns, whereas both the Chinese and US markets statistically influence NIFTY returns. A
unidirectional causality has been observed from the US and Chinese markets to the Indian
market in the context of market returns during the crisis period with a negative impact of
the Chinese returns. Considering the stock market volatility, there is a uni-directional
spillover from the US market to the Indian market only and from the Indian market to the
Chinese market. There is a significant impact of the lagged volatility in the Indian market on
the US market. The cross market impact reduces over a period of time signifying the impact
of past volatility on the current conditional variance. Surprisingly, the efficient tests of
causality came out with the finding that there is an indirect impact of the US stock market
volatility on the Chinese market volatility via Indian Market. The study of these linkages is
an imperative task to be performed by the international portfolio managers. The managers
should discount this type of information well ahead of time so as to hedge themselves from
any adverse situation arising due to any crisis or a country specific event. The past events
always act as a catalyst in guiding the future trends. The behavior of the US, Chinese and
Indian stock markets during the financial crisis is a lesson to be comprehended by the
International investors in their efforts to hedge against downside risks in future. The causal
relations have an impact on the co-movement of the markets, thereby entailing to portfolio
adjustments in the countries concerned.
Acknowledgement:
We would like to express our gratitude towards reviewer(s) of the paper for giving enormously useful
suggestions. The mistakes, if any, are our responsibility.
Unauthenticated
Download Date | 2/23/19 2:23 PM
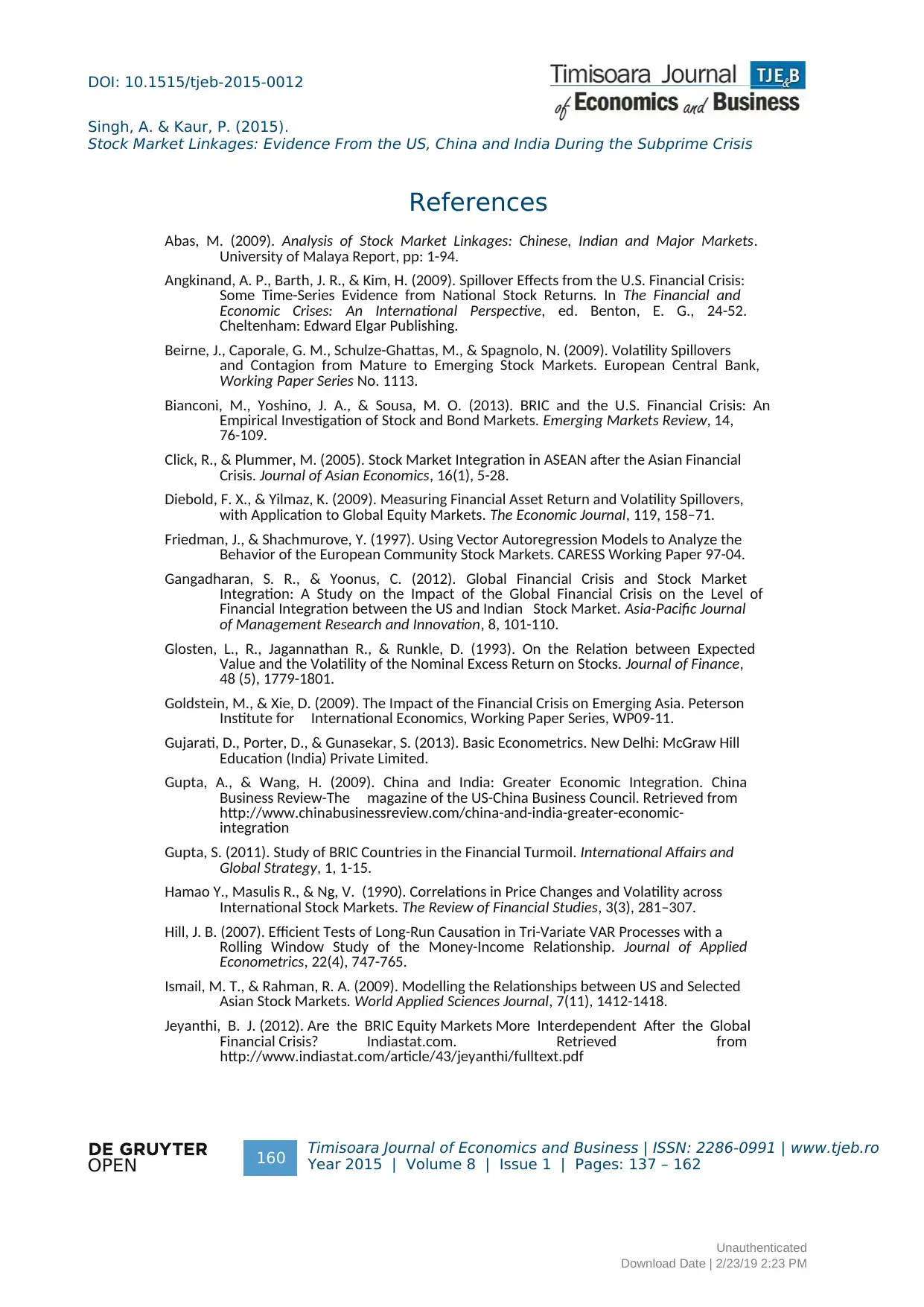
OPEN
DOI: 10.1515/tjeb-2015-0012
Singh, A. & Kaur, P. (2015).
Stock Market Linkages: Evidence From the US, China and India During the Subprime Crisis
Timisoara Journal of Economics and Business | ISSN: 2286-0991 | www.tjeb.ro
Year 2015 | Volume 8 | Issue 1 | Pages: 137 – 162160
References
Abas, M. (2009). Analysis of Stock Market Linkages: Chinese, Indian and Major Markets.
University of Malaya Report, pp: 1-94.
Angkinand, A. P., Barth, J. R., & Kim, H. (2009). Spillover Effects from the U.S. Financial Crisis:
Some Time-Series Evidence from National Stock Returns. In The Financial and
Economic Crises: An International Perspective, ed. Benton, E. G., 24-52.
Cheltenham: Edward Elgar Publishing.
Beirne, J., Caporale, G. M., Schulze-Ghattas, M., & Spagnolo, N. (2009). Volatility Spillovers
and Contagion from Mature to Emerging Stock Markets. European Central Bank,
Working Paper Series No. 1113.
Bianconi, M., Yoshino, J. A., & Sousa, M. O. (2013). BRIC and the U.S. Financial Crisis: An
Empirical Investigation of Stock and Bond Markets. Emerging Markets Review, 14,
76-109.
Click, R., & Plummer, M. (2005). Stock Market Integration in ASEAN after the Asian Financial
Crisis. Journal of Asian Economics, 16(1), 5-28.
Diebold, F. X., & Yilmaz, K. (2009). Measuring Financial Asset Return and Volatility Spillovers,
with Application to Global Equity Markets. The Economic Journal, 119, 158–71.
Friedman, J., & Shachmurove, Y. (1997). Using Vector Autoregression Models to Analyze the
Behavior of the European Community Stock Markets. CARESS Working Paper 97-04.
Gangadharan, S. R., & Yoonus, C. (2012). Global Financial Crisis and Stock Market
Integration: A Study on the Impact of the Global Financial Crisis on the Level of
Financial Integration between the US and Indian Stock Market. Asia-Pacific Journal
of Management Research and Innovation, 8, 101-110.
Glosten, L., R., Jagannathan R., & Runkle, D. (1993). On the Relation between Expected
Value and the Volatility of the Nominal Excess Return on Stocks. Journal of Finance,
48 (5), 1779-1801.
Goldstein, M., & Xie, D. (2009). The Impact of the Financial Crisis on Emerging Asia. Peterson
Institute for International Economics, Working Paper Series, WP09-11.
Gujarati, D., Porter, D., & Gunasekar, S. (2013). Basic Econometrics. New Delhi: McGraw Hill
Education (India) Private Limited.
Gupta, A., & Wang, H. (2009). China and India: Greater Economic Integration. China
Business Review-The magazine of the US-China Business Council. Retrieved from
http://www.chinabusinessreview.com/china-and-india-greater-economic-
integration
Gupta, S. (2011). Study of BRIC Countries in the Financial Turmoil. International Affairs and
Global Strategy, 1, 1-15.
Hamao Y., Masulis R., & Ng, V. (1990). Correlations in Price Changes and Volatility across
International Stock Markets. The Review of Financial Studies, 3(3), 281–307.
Hill, J. B. (2007). Efficient Tests of Long-Run Causation in Tri-Variate VAR Processes with a
Rolling Window Study of the Money-Income Relationship. Journal of Applied
Econometrics, 22(4), 747-765.
Ismail, M. T., & Rahman, R. A. (2009). Modelling the Relationships between US and Selected
Asian Stock Markets. World Applied Sciences Journal, 7(11), 1412-1418.
Jeyanthi, B. J. (2012). Are the BRIC Equity Markets More Interdependent After the Global
Financial Crisis? Indiastat.com. Retrieved from
http://www.indiastat.com/article/43/jeyanthi/fulltext.pdf
Unauthenticated
Download Date | 2/23/19 2:23 PM
DOI: 10.1515/tjeb-2015-0012
Singh, A. & Kaur, P. (2015).
Stock Market Linkages: Evidence From the US, China and India During the Subprime Crisis
Timisoara Journal of Economics and Business | ISSN: 2286-0991 | www.tjeb.ro
Year 2015 | Volume 8 | Issue 1 | Pages: 137 – 162160
References
Abas, M. (2009). Analysis of Stock Market Linkages: Chinese, Indian and Major Markets.
University of Malaya Report, pp: 1-94.
Angkinand, A. P., Barth, J. R., & Kim, H. (2009). Spillover Effects from the U.S. Financial Crisis:
Some Time-Series Evidence from National Stock Returns. In The Financial and
Economic Crises: An International Perspective, ed. Benton, E. G., 24-52.
Cheltenham: Edward Elgar Publishing.
Beirne, J., Caporale, G. M., Schulze-Ghattas, M., & Spagnolo, N. (2009). Volatility Spillovers
and Contagion from Mature to Emerging Stock Markets. European Central Bank,
Working Paper Series No. 1113.
Bianconi, M., Yoshino, J. A., & Sousa, M. O. (2013). BRIC and the U.S. Financial Crisis: An
Empirical Investigation of Stock and Bond Markets. Emerging Markets Review, 14,
76-109.
Click, R., & Plummer, M. (2005). Stock Market Integration in ASEAN after the Asian Financial
Crisis. Journal of Asian Economics, 16(1), 5-28.
Diebold, F. X., & Yilmaz, K. (2009). Measuring Financial Asset Return and Volatility Spillovers,
with Application to Global Equity Markets. The Economic Journal, 119, 158–71.
Friedman, J., & Shachmurove, Y. (1997). Using Vector Autoregression Models to Analyze the
Behavior of the European Community Stock Markets. CARESS Working Paper 97-04.
Gangadharan, S. R., & Yoonus, C. (2012). Global Financial Crisis and Stock Market
Integration: A Study on the Impact of the Global Financial Crisis on the Level of
Financial Integration between the US and Indian Stock Market. Asia-Pacific Journal
of Management Research and Innovation, 8, 101-110.
Glosten, L., R., Jagannathan R., & Runkle, D. (1993). On the Relation between Expected
Value and the Volatility of the Nominal Excess Return on Stocks. Journal of Finance,
48 (5), 1779-1801.
Goldstein, M., & Xie, D. (2009). The Impact of the Financial Crisis on Emerging Asia. Peterson
Institute for International Economics, Working Paper Series, WP09-11.
Gujarati, D., Porter, D., & Gunasekar, S. (2013). Basic Econometrics. New Delhi: McGraw Hill
Education (India) Private Limited.
Gupta, A., & Wang, H. (2009). China and India: Greater Economic Integration. China
Business Review-The magazine of the US-China Business Council. Retrieved from
http://www.chinabusinessreview.com/china-and-india-greater-economic-
integration
Gupta, S. (2011). Study of BRIC Countries in the Financial Turmoil. International Affairs and
Global Strategy, 1, 1-15.
Hamao Y., Masulis R., & Ng, V. (1990). Correlations in Price Changes and Volatility across
International Stock Markets. The Review of Financial Studies, 3(3), 281–307.
Hill, J. B. (2007). Efficient Tests of Long-Run Causation in Tri-Variate VAR Processes with a
Rolling Window Study of the Money-Income Relationship. Journal of Applied
Econometrics, 22(4), 747-765.
Ismail, M. T., & Rahman, R. A. (2009). Modelling the Relationships between US and Selected
Asian Stock Markets. World Applied Sciences Journal, 7(11), 1412-1418.
Jeyanthi, B. J. (2012). Are the BRIC Equity Markets More Interdependent After the Global
Financial Crisis? Indiastat.com. Retrieved from
http://www.indiastat.com/article/43/jeyanthi/fulltext.pdf
Unauthenticated
Download Date | 2/23/19 2:23 PM
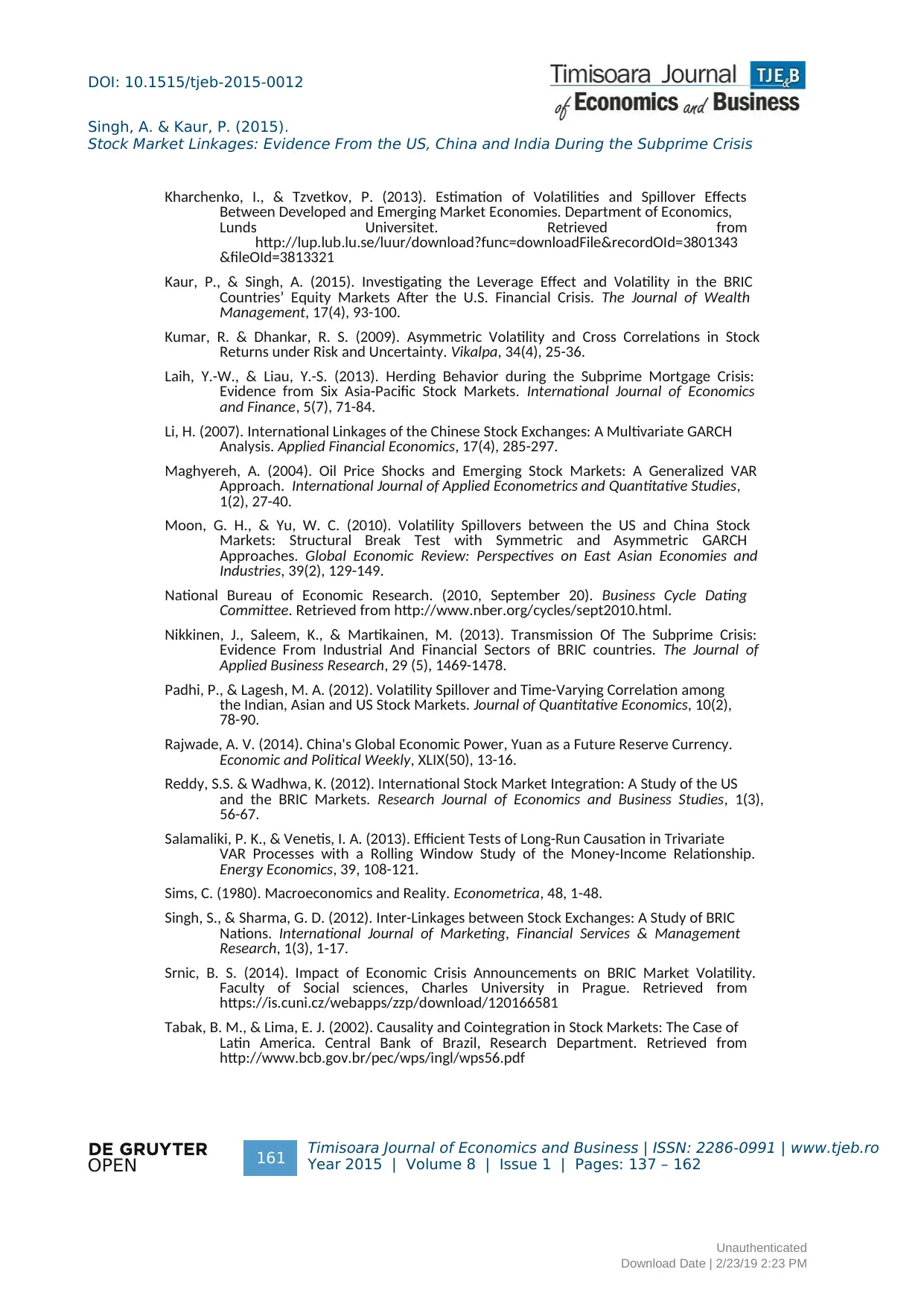
OPEN
DOI: 10.1515/tjeb-2015-0012
Singh, A. & Kaur, P. (2015).
Stock Market Linkages: Evidence From the US, China and India During the Subprime Crisis
Timisoara Journal of Economics and Business | ISSN: 2286-0991 | www.tjeb.ro
Year 2015 | Volume 8 | Issue 1 | Pages: 137 – 162161
Kharchenko, I., & Tzvetkov, P. (2013). Estimation of Volatilities and Spillover Effects
Between Developed and Emerging Market Economies. Department of Economics,
Lunds Universitet. Retrieved from
http://lup.lub.lu.se/luur/download?func=downloadFile&recordOId=3801343
&fileOId=3813321
Kaur, P., & Singh, A. (2015). Investigating the Leverage Effect and Volatility in the BRIC
Countries’ Equity Markets After the U.S. Financial Crisis. The Journal of Wealth
Management, 17(4), 93-100.
Kumar, R. & Dhankar, R. S. (2009). Asymmetric Volatility and Cross Correlations in Stock
Returns under Risk and Uncertainty. Vikalpa, 34(4), 25-36.
Laih, Y.-W., & Liau, Y.-S. (2013). Herding Behavior during the Subprime Mortgage Crisis:
Evidence from Six Asia-Pacific Stock Markets. International Journal of Economics
and Finance, 5(7), 71-84.
Li, H. (2007). International Linkages of the Chinese Stock Exchanges: A Multivariate GARCH
Analysis. Applied Financial Economics, 17(4), 285-297.
Maghyereh, A. (2004). Oil Price Shocks and Emerging Stock Markets: A Generalized VAR
Approach. International Journal of Applied Econometrics and Quantitative Studies,
1(2), 27-40.
Moon, G. H., & Yu, W. C. (2010). Volatility Spillovers between the US and China Stock
Markets: Structural Break Test with Symmetric and Asymmetric GARCH
Approaches. Global Economic Review: Perspectives on East Asian Economies and
Industries, 39(2), 129-149.
National Bureau of Economic Research. (2010, September 20). Business Cycle Dating
Committee. Retrieved from http://www.nber.org/cycles/sept2010.html.
Nikkinen, J., Saleem, K., & Martikainen, M. (2013). Transmission Of The Subprime Crisis:
Evidence From Industrial And Financial Sectors of BRIC countries. The Journal of
Applied Business Research, 29 (5), 1469-1478.
Padhi, P., & Lagesh, M. A. (2012). Volatility Spillover and Time-Varying Correlation among
the Indian, Asian and US Stock Markets. Journal of Quantitative Economics, 10(2),
78-90.
Rajwade, A. V. (2014). China's Global Economic Power, Yuan as a Future Reserve Currency.
Economic and Political Weekly, XLIX(50), 13-16.
Reddy, S.S. & Wadhwa, K. (2012). International Stock Market Integration: A Study of the US
and the BRIC Markets. Research Journal of Economics and Business Studies, 1(3),
56-67.
Salamaliki, P. K., & Venetis, I. A. (2013). Efficient Tests of Long-Run Causation in Trivariate
VAR Processes with a Rolling Window Study of the Money-Income Relationship.
Energy Economics, 39, 108-121.
Sims, C. (1980). Macroeconomics and Reality. Econometrica, 48, 1-48.
Singh, S., & Sharma, G. D. (2012). Inter-Linkages between Stock Exchanges: A Study of BRIC
Nations. International Journal of Marketing, Financial Services & Management
Research, 1(3), 1-17.
Srnic, B. S. (2014). Impact of Economic Crisis Announcements on BRIC Market Volatility.
Faculty of Social sciences, Charles University in Prague. Retrieved from
https://is.cuni.cz/webapps/zzp/download/120166581
Tabak, B. M., & Lima, E. J. (2002). Causality and Cointegration in Stock Markets: The Case of
Latin America. Central Bank of Brazil, Research Department. Retrieved from
http://www.bcb.gov.br/pec/wps/ingl/wps56.pdf
Unauthenticated
Download Date | 2/23/19 2:23 PM
DOI: 10.1515/tjeb-2015-0012
Singh, A. & Kaur, P. (2015).
Stock Market Linkages: Evidence From the US, China and India During the Subprime Crisis
Timisoara Journal of Economics and Business | ISSN: 2286-0991 | www.tjeb.ro
Year 2015 | Volume 8 | Issue 1 | Pages: 137 – 162161
Kharchenko, I., & Tzvetkov, P. (2013). Estimation of Volatilities and Spillover Effects
Between Developed and Emerging Market Economies. Department of Economics,
Lunds Universitet. Retrieved from
http://lup.lub.lu.se/luur/download?func=downloadFile&recordOId=3801343
&fileOId=3813321
Kaur, P., & Singh, A. (2015). Investigating the Leverage Effect and Volatility in the BRIC
Countries’ Equity Markets After the U.S. Financial Crisis. The Journal of Wealth
Management, 17(4), 93-100.
Kumar, R. & Dhankar, R. S. (2009). Asymmetric Volatility and Cross Correlations in Stock
Returns under Risk and Uncertainty. Vikalpa, 34(4), 25-36.
Laih, Y.-W., & Liau, Y.-S. (2013). Herding Behavior during the Subprime Mortgage Crisis:
Evidence from Six Asia-Pacific Stock Markets. International Journal of Economics
and Finance, 5(7), 71-84.
Li, H. (2007). International Linkages of the Chinese Stock Exchanges: A Multivariate GARCH
Analysis. Applied Financial Economics, 17(4), 285-297.
Maghyereh, A. (2004). Oil Price Shocks and Emerging Stock Markets: A Generalized VAR
Approach. International Journal of Applied Econometrics and Quantitative Studies,
1(2), 27-40.
Moon, G. H., & Yu, W. C. (2010). Volatility Spillovers between the US and China Stock
Markets: Structural Break Test with Symmetric and Asymmetric GARCH
Approaches. Global Economic Review: Perspectives on East Asian Economies and
Industries, 39(2), 129-149.
National Bureau of Economic Research. (2010, September 20). Business Cycle Dating
Committee. Retrieved from http://www.nber.org/cycles/sept2010.html.
Nikkinen, J., Saleem, K., & Martikainen, M. (2013). Transmission Of The Subprime Crisis:
Evidence From Industrial And Financial Sectors of BRIC countries. The Journal of
Applied Business Research, 29 (5), 1469-1478.
Padhi, P., & Lagesh, M. A. (2012). Volatility Spillover and Time-Varying Correlation among
the Indian, Asian and US Stock Markets. Journal of Quantitative Economics, 10(2),
78-90.
Rajwade, A. V. (2014). China's Global Economic Power, Yuan as a Future Reserve Currency.
Economic and Political Weekly, XLIX(50), 13-16.
Reddy, S.S. & Wadhwa, K. (2012). International Stock Market Integration: A Study of the US
and the BRIC Markets. Research Journal of Economics and Business Studies, 1(3),
56-67.
Salamaliki, P. K., & Venetis, I. A. (2013). Efficient Tests of Long-Run Causation in Trivariate
VAR Processes with a Rolling Window Study of the Money-Income Relationship.
Energy Economics, 39, 108-121.
Sims, C. (1980). Macroeconomics and Reality. Econometrica, 48, 1-48.
Singh, S., & Sharma, G. D. (2012). Inter-Linkages between Stock Exchanges: A Study of BRIC
Nations. International Journal of Marketing, Financial Services & Management
Research, 1(3), 1-17.
Srnic, B. S. (2014). Impact of Economic Crisis Announcements on BRIC Market Volatility.
Faculty of Social sciences, Charles University in Prague. Retrieved from
https://is.cuni.cz/webapps/zzp/download/120166581
Tabak, B. M., & Lima, E. J. (2002). Causality and Cointegration in Stock Markets: The Case of
Latin America. Central Bank of Brazil, Research Department. Retrieved from
http://www.bcb.gov.br/pec/wps/ingl/wps56.pdf
Unauthenticated
Download Date | 2/23/19 2:23 PM
Secure Best Marks with AI Grader
Need help grading? Try our AI Grader for instant feedback on your assignments.
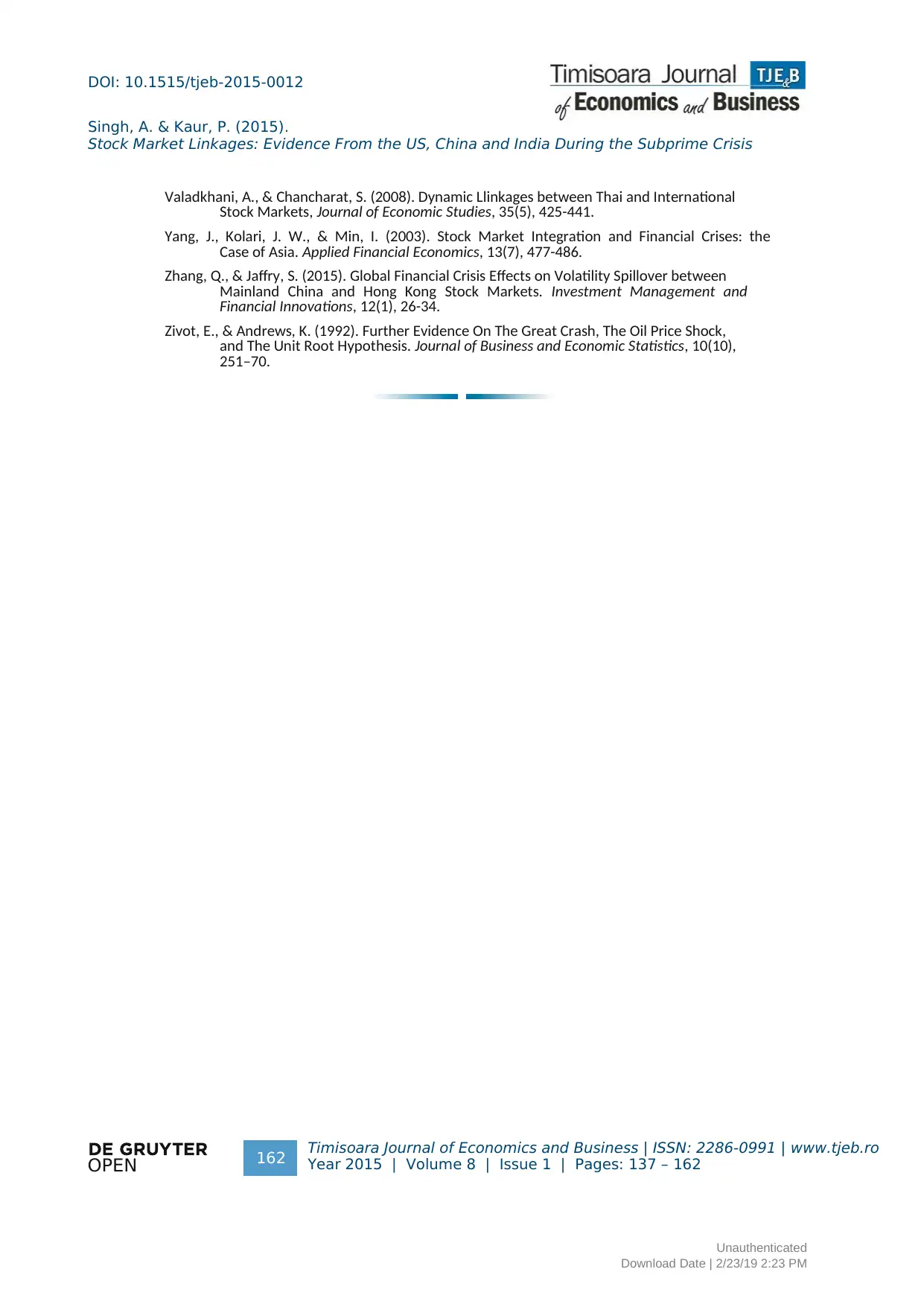
OPEN
DOI: 10.1515/tjeb-2015-0012
Singh, A. & Kaur, P. (2015).
Stock Market Linkages: Evidence From the US, China and India During the Subprime Crisis
Timisoara Journal of Economics and Business | ISSN: 2286-0991 | www.tjeb.ro
Year 2015 | Volume 8 | Issue 1 | Pages: 137 – 162162
Valadkhani, A., & Chancharat, S. (2008). Dynamic Llinkages between Thai and International
Stock Markets, Journal of Economic Studies, 35(5), 425-441.
Yang, J., Kolari, J. W., & Min, I. (2003). Stock Market Integration and Financial Crises: the
Case of Asia. Applied Financial Economics, 13(7), 477-486.
Zhang, Q., & Jaffry, S. (2015). Global Financial Crisis Effects on Volatility Spillover between
Mainland China and Hong Kong Stock Markets. Investment Management and
Financial Innovations, 12(1), 26-34.
Zivot, E., & Andrews, K. (1992). Further Evidence On The Great Crash, The Oil Price Shock,
and The Unit Root Hypothesis. Journal of Business and Economic Statistics, 10(10),
251–70.
Unauthenticated
Download Date | 2/23/19 2:23 PM
DOI: 10.1515/tjeb-2015-0012
Singh, A. & Kaur, P. (2015).
Stock Market Linkages: Evidence From the US, China and India During the Subprime Crisis
Timisoara Journal of Economics and Business | ISSN: 2286-0991 | www.tjeb.ro
Year 2015 | Volume 8 | Issue 1 | Pages: 137 – 162162
Valadkhani, A., & Chancharat, S. (2008). Dynamic Llinkages between Thai and International
Stock Markets, Journal of Economic Studies, 35(5), 425-441.
Yang, J., Kolari, J. W., & Min, I. (2003). Stock Market Integration and Financial Crises: the
Case of Asia. Applied Financial Economics, 13(7), 477-486.
Zhang, Q., & Jaffry, S. (2015). Global Financial Crisis Effects on Volatility Spillover between
Mainland China and Hong Kong Stock Markets. Investment Management and
Financial Innovations, 12(1), 26-34.
Zivot, E., & Andrews, K. (1992). Further Evidence On The Great Crash, The Oil Price Shock,
and The Unit Root Hypothesis. Journal of Business and Economic Statistics, 10(10),
251–70.
Unauthenticated
Download Date | 2/23/19 2:23 PM
1 out of 26
Related Documents
![[object Object]](/_next/image/?url=%2F_next%2Fstatic%2Fmedia%2Flogo.6d15ce61.png&w=640&q=75)
Your All-in-One AI-Powered Toolkit for Academic Success.
+13062052269
info@desklib.com
Available 24*7 on WhatsApp / Email
Unlock your academic potential
© 2024 | Zucol Services PVT LTD | All rights reserved.