Project: Analyzing Politics & Social Cohesion on Twitter using Data
VerifiedAdded on 2023/06/11
|24
|4872
|426
Project
AI Summary
This project explores the role of politics and political parties in a society's cohesion by analyzing Twitter data. It covers general concepts of Australian politics, the state of polarization, textual data mining, basic data analysis and visualization, network analysis, and the effects of politics on day-to-...
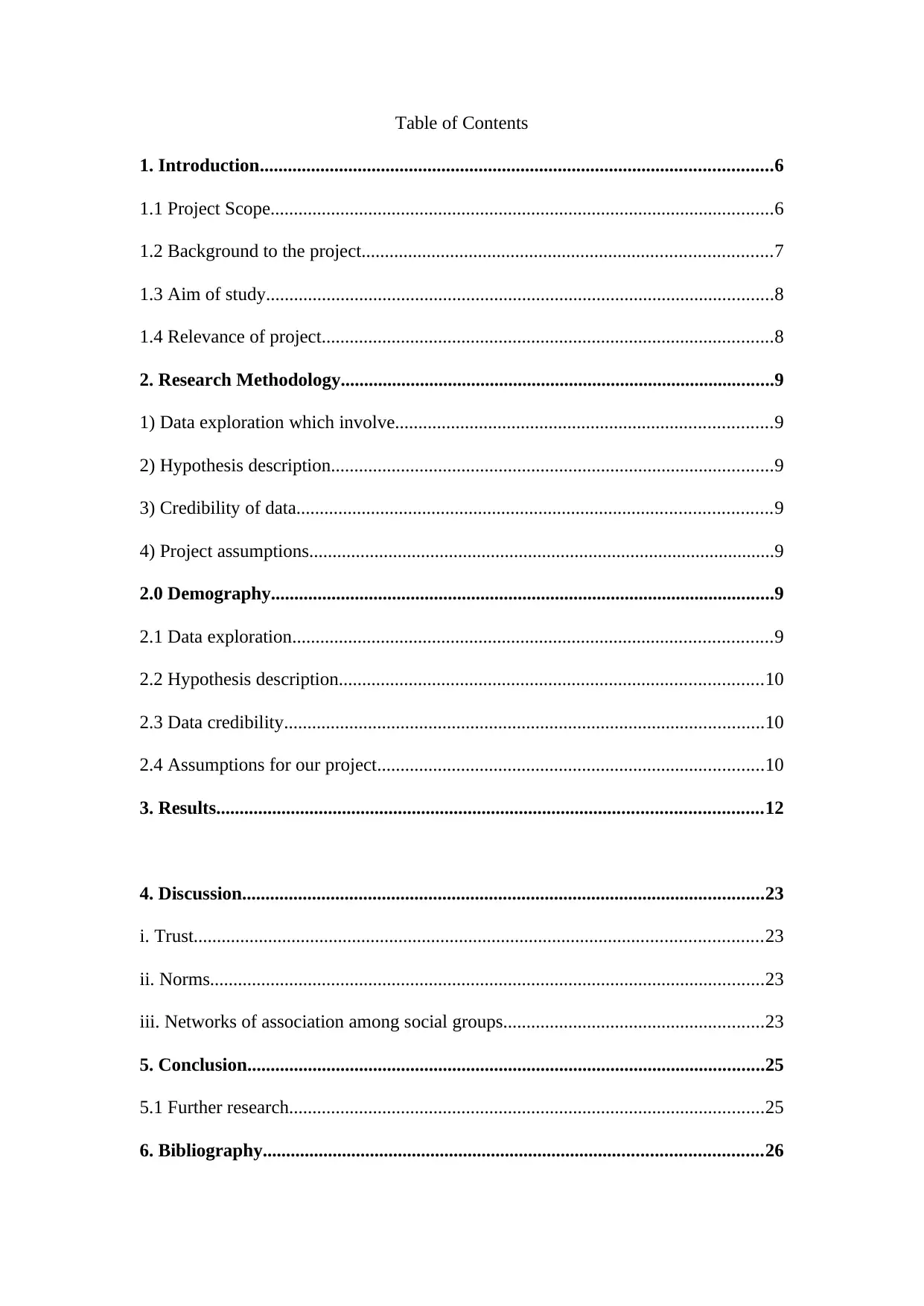
Table of Contents
1. Introduction..............................................................................................................6
1.1 Project Scope............................................................................................................6
1.2 Background to the project........................................................................................7
1.3 Aim of study.............................................................................................................8
1.4 Relevance of project.................................................................................................8
2. Research Methodology.............................................................................................9
1) Data exploration which involve.................................................................................9
2) Hypothesis description...............................................................................................9
3) Credibility of data......................................................................................................9
4) Project assumptions....................................................................................................9
2.0 Demography............................................................................................................9
2.1 Data exploration.......................................................................................................9
2.2 Hypothesis description...........................................................................................10
2.3 Data credibility.......................................................................................................10
2.4 Assumptions for our project...................................................................................10
3. Results.....................................................................................................................12
4. Discussion................................................................................................................23
i. Trust..........................................................................................................................23
ii. Norms.......................................................................................................................23
iii. Networks of association among social groups........................................................23
5. Conclusion...............................................................................................................25
5.1 Further research......................................................................................................25
6. Bibliography...........................................................................................................26
1. Introduction..............................................................................................................6
1.1 Project Scope............................................................................................................6
1.2 Background to the project........................................................................................7
1.3 Aim of study.............................................................................................................8
1.4 Relevance of project.................................................................................................8
2. Research Methodology.............................................................................................9
1) Data exploration which involve.................................................................................9
2) Hypothesis description...............................................................................................9
3) Credibility of data......................................................................................................9
4) Project assumptions....................................................................................................9
2.0 Demography............................................................................................................9
2.1 Data exploration.......................................................................................................9
2.2 Hypothesis description...........................................................................................10
2.3 Data credibility.......................................................................................................10
2.4 Assumptions for our project...................................................................................10
3. Results.....................................................................................................................12
4. Discussion................................................................................................................23
i. Trust..........................................................................................................................23
ii. Norms.......................................................................................................................23
iii. Networks of association among social groups........................................................23
5. Conclusion...............................................................................................................25
5.1 Further research......................................................................................................25
6. Bibliography...........................................................................................................26
Paraphrase This Document
Need a fresh take? Get an instant paraphrase of this document with our AI Paraphraser
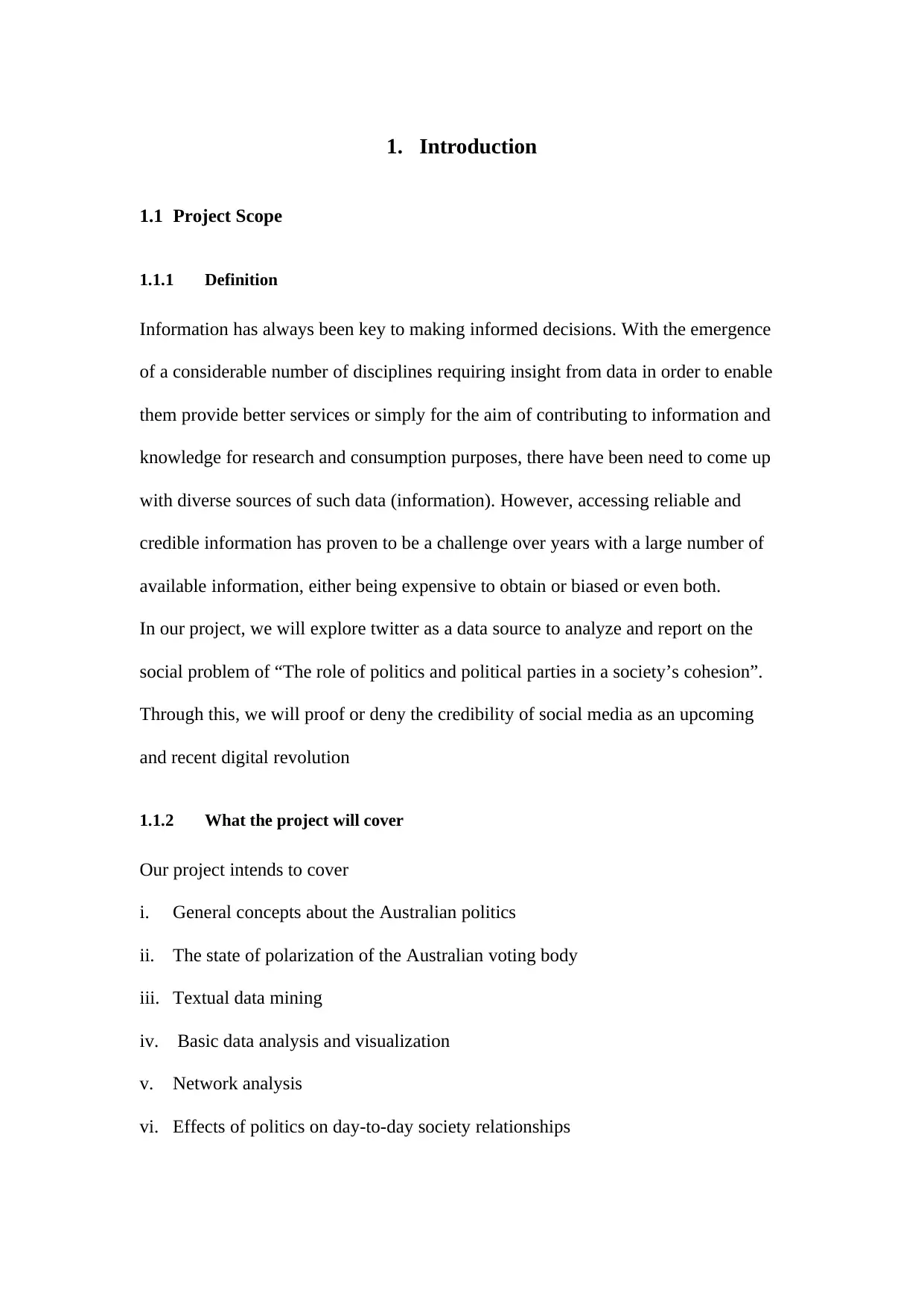
1. Introduction
1.1 Project Scope
1.1.1 Definition
Information has always been key to making informed decisions. With the emergence
of a considerable number of disciplines requiring insight from data in order to enable
them provide better services or simply for the aim of contributing to information and
knowledge for research and consumption purposes, there have been need to come up
with diverse sources of such data (information). However, accessing reliable and
credible information has proven to be a challenge over years with a large number of
available information, either being expensive to obtain or biased or even both.
In our project, we will explore twitter as a data source to analyze and report on the
social problem of “The role of politics and political parties in a society’s cohesion”.
Through this, we will proof or deny the credibility of social media as an upcoming
and recent digital revolution
1.1.2 What the project will cover
Our project intends to cover
i. General concepts about the Australian politics
ii. The state of polarization of the Australian voting body
iii. Textual data mining
iv. Basic data analysis and visualization
v. Network analysis
vi. Effects of politics on day-to-day society relationships
1.1 Project Scope
1.1.1 Definition
Information has always been key to making informed decisions. With the emergence
of a considerable number of disciplines requiring insight from data in order to enable
them provide better services or simply for the aim of contributing to information and
knowledge for research and consumption purposes, there have been need to come up
with diverse sources of such data (information). However, accessing reliable and
credible information has proven to be a challenge over years with a large number of
available information, either being expensive to obtain or biased or even both.
In our project, we will explore twitter as a data source to analyze and report on the
social problem of “The role of politics and political parties in a society’s cohesion”.
Through this, we will proof or deny the credibility of social media as an upcoming
and recent digital revolution
1.1.2 What the project will cover
Our project intends to cover
i. General concepts about the Australian politics
ii. The state of polarization of the Australian voting body
iii. Textual data mining
iv. Basic data analysis and visualization
v. Network analysis
vi. Effects of politics on day-to-day society relationships
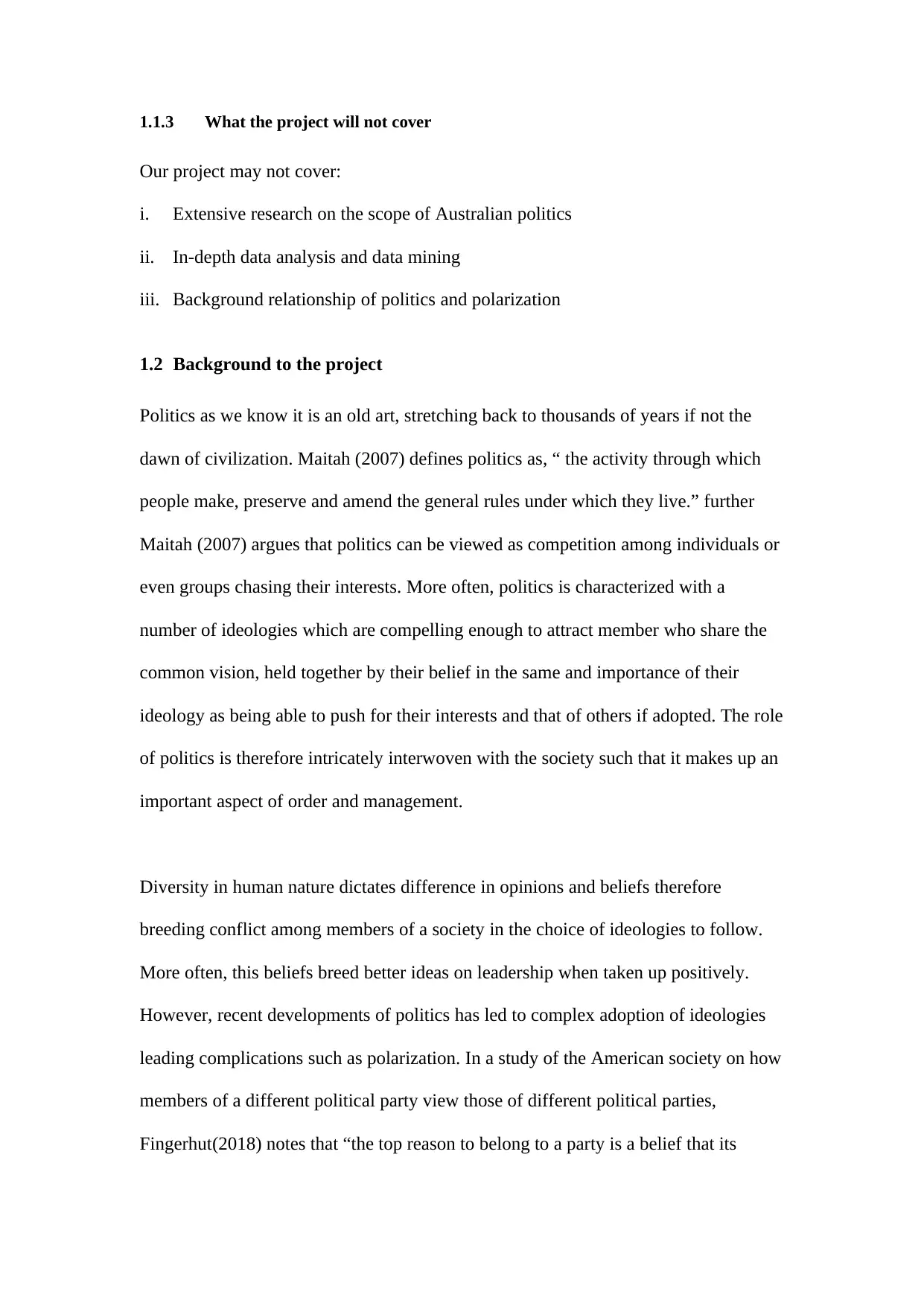
1.1.3 What the project will not cover
Our project may not cover:
i. Extensive research on the scope of Australian politics
ii. In-depth data analysis and data mining
iii. Background relationship of politics and polarization
1.2 Background to the project
Politics as we know it is an old art, stretching back to thousands of years if not the
dawn of civilization. Maitah (2007) defines politics as, “ the activity through which
people make, preserve and amend the general rules under which they live.” further
Maitah (2007) argues that politics can be viewed as competition among individuals or
even groups chasing their interests. More often, politics is characterized with a
number of ideologies which are compelling enough to attract member who share the
common vision, held together by their belief in the same and importance of their
ideology as being able to push for their interests and that of others if adopted. The role
of politics is therefore intricately interwoven with the society such that it makes up an
important aspect of order and management.
Diversity in human nature dictates difference in opinions and beliefs therefore
breeding conflict among members of a society in the choice of ideologies to follow.
More often, this beliefs breed better ideas on leadership when taken up positively.
However, recent developments of politics has led to complex adoption of ideologies
leading complications such as polarization. In a study of the American society on how
members of a different political party view those of different political parties,
Fingerhut(2018) notes that “the top reason to belong to a party is a belief that its
Our project may not cover:
i. Extensive research on the scope of Australian politics
ii. In-depth data analysis and data mining
iii. Background relationship of politics and polarization
1.2 Background to the project
Politics as we know it is an old art, stretching back to thousands of years if not the
dawn of civilization. Maitah (2007) defines politics as, “ the activity through which
people make, preserve and amend the general rules under which they live.” further
Maitah (2007) argues that politics can be viewed as competition among individuals or
even groups chasing their interests. More often, politics is characterized with a
number of ideologies which are compelling enough to attract member who share the
common vision, held together by their belief in the same and importance of their
ideology as being able to push for their interests and that of others if adopted. The role
of politics is therefore intricately interwoven with the society such that it makes up an
important aspect of order and management.
Diversity in human nature dictates difference in opinions and beliefs therefore
breeding conflict among members of a society in the choice of ideologies to follow.
More often, this beliefs breed better ideas on leadership when taken up positively.
However, recent developments of politics has led to complex adoption of ideologies
leading complications such as polarization. In a study of the American society on how
members of a different political party view those of different political parties,
Fingerhut(2018) notes that “the top reason to belong to a party is a belief that its
You're viewing a preview
Unlock full access by subscribing today!
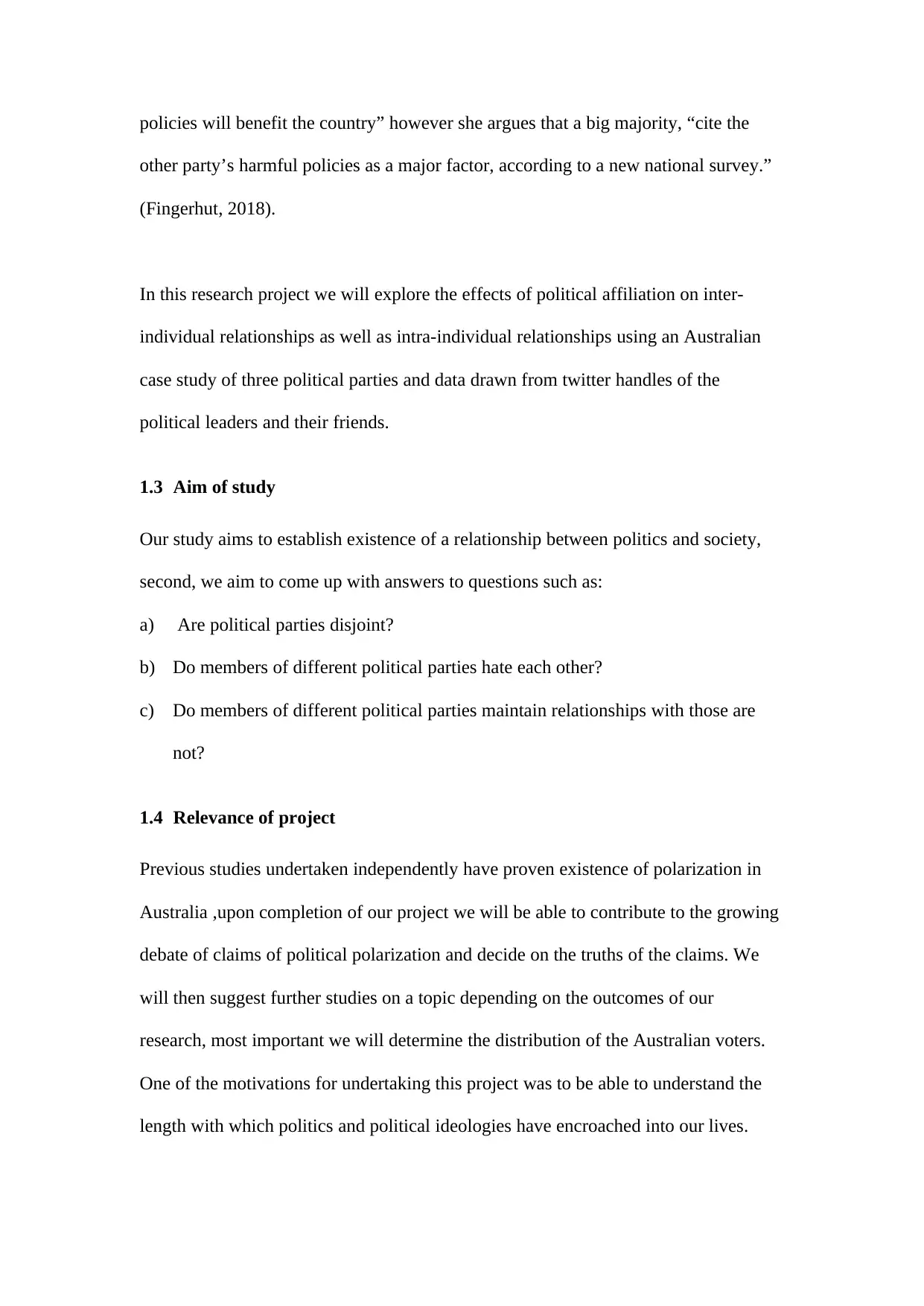
policies will benefit the country” however she argues that a big majority, “cite the
other party’s harmful policies as a major factor, according to a new national survey.”
(Fingerhut, 2018).
In this research project we will explore the effects of political affiliation on inter-
individual relationships as well as intra-individual relationships using an Australian
case study of three political parties and data drawn from twitter handles of the
political leaders and their friends.
1.3 Aim of study
Our study aims to establish existence of a relationship between politics and society,
second, we aim to come up with answers to questions such as:
a) Are political parties disjoint?
b) Do members of different political parties hate each other?
c) Do members of different political parties maintain relationships with those are
not?
1.4 Relevance of project
Previous studies undertaken independently have proven existence of polarization in
Australia ,upon completion of our project we will be able to contribute to the growing
debate of claims of political polarization and decide on the truths of the claims. We
will then suggest further studies on a topic depending on the outcomes of our
research, most important we will determine the distribution of the Australian voters.
One of the motivations for undertaking this project was to be able to understand the
length with which politics and political ideologies have encroached into our lives.
other party’s harmful policies as a major factor, according to a new national survey.”
(Fingerhut, 2018).
In this research project we will explore the effects of political affiliation on inter-
individual relationships as well as intra-individual relationships using an Australian
case study of three political parties and data drawn from twitter handles of the
political leaders and their friends.
1.3 Aim of study
Our study aims to establish existence of a relationship between politics and society,
second, we aim to come up with answers to questions such as:
a) Are political parties disjoint?
b) Do members of different political parties hate each other?
c) Do members of different political parties maintain relationships with those are
not?
1.4 Relevance of project
Previous studies undertaken independently have proven existence of polarization in
Australia ,upon completion of our project we will be able to contribute to the growing
debate of claims of political polarization and decide on the truths of the claims. We
will then suggest further studies on a topic depending on the outcomes of our
research, most important we will determine the distribution of the Australian voters.
One of the motivations for undertaking this project was to be able to understand the
length with which politics and political ideologies have encroached into our lives.
Paraphrase This Document
Need a fresh take? Get an instant paraphrase of this document with our AI Paraphraser
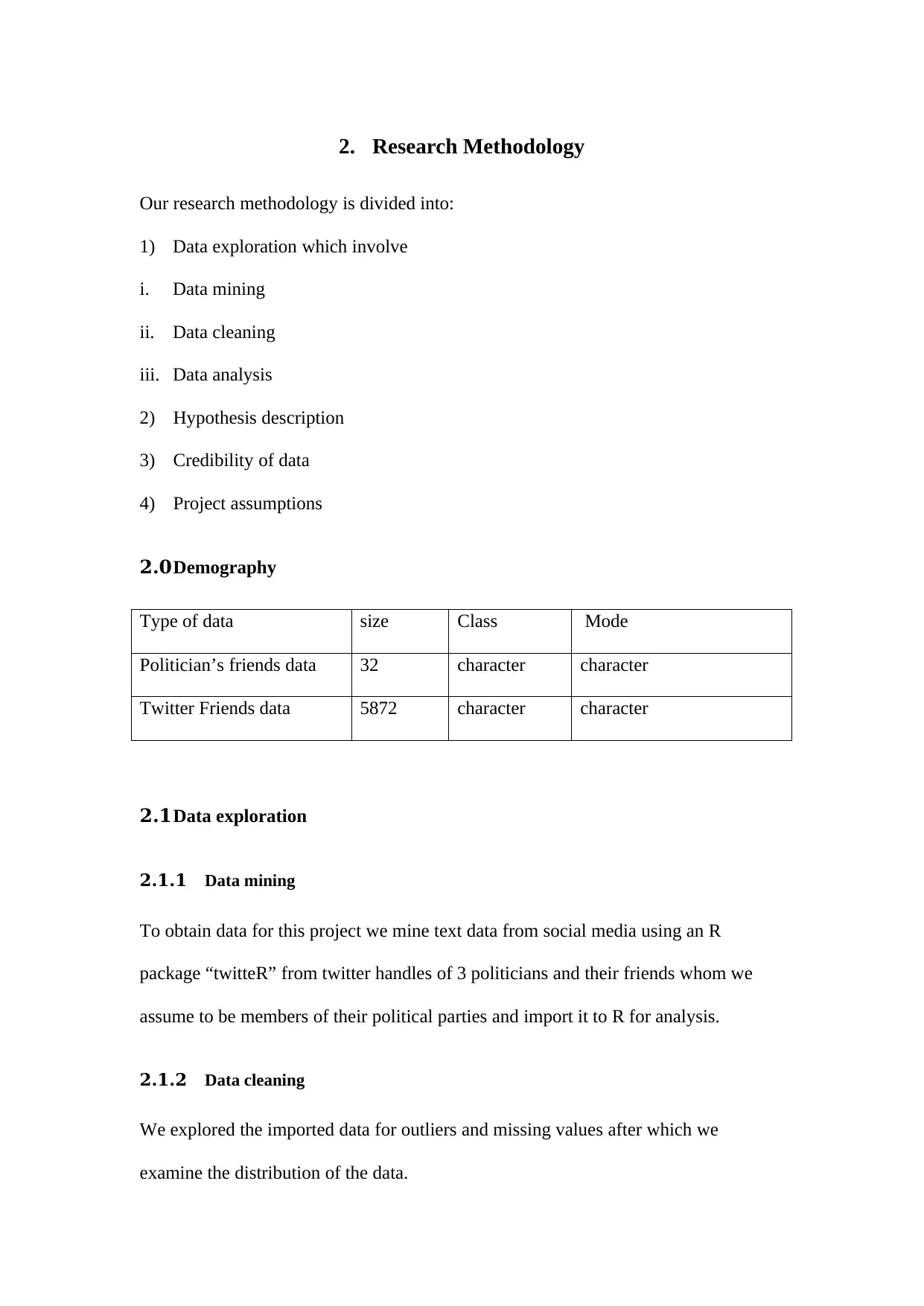
2. Research Methodology
Our research methodology is divided into:
1) Data exploration which involve
i. Data mining
ii. Data cleaning
iii. Data analysis
2) Hypothesis description
3) Credibility of data
4) Project assumptions
2.0Demography
Type of data size Class Mode
Politician’s friends data 32 character character
Twitter Friends data 5872 character character
2.1Data exploration
2.1.1 Data mining
To obtain data for this project we mine text data from social media using an R
package “twitteR” from twitter handles of 3 politicians and their friends whom we
assume to be members of their political parties and import it to R for analysis.
2.1.2 Data cleaning
We explored the imported data for outliers and missing values after which we
examine the distribution of the data.
Our research methodology is divided into:
1) Data exploration which involve
i. Data mining
ii. Data cleaning
iii. Data analysis
2) Hypothesis description
3) Credibility of data
4) Project assumptions
2.0Demography
Type of data size Class Mode
Politician’s friends data 32 character character
Twitter Friends data 5872 character character
2.1Data exploration
2.1.1 Data mining
To obtain data for this project we mine text data from social media using an R
package “twitteR” from twitter handles of 3 politicians and their friends whom we
assume to be members of their political parties and import it to R for analysis.
2.1.2 Data cleaning
We explored the imported data for outliers and missing values after which we
examine the distribution of the data.
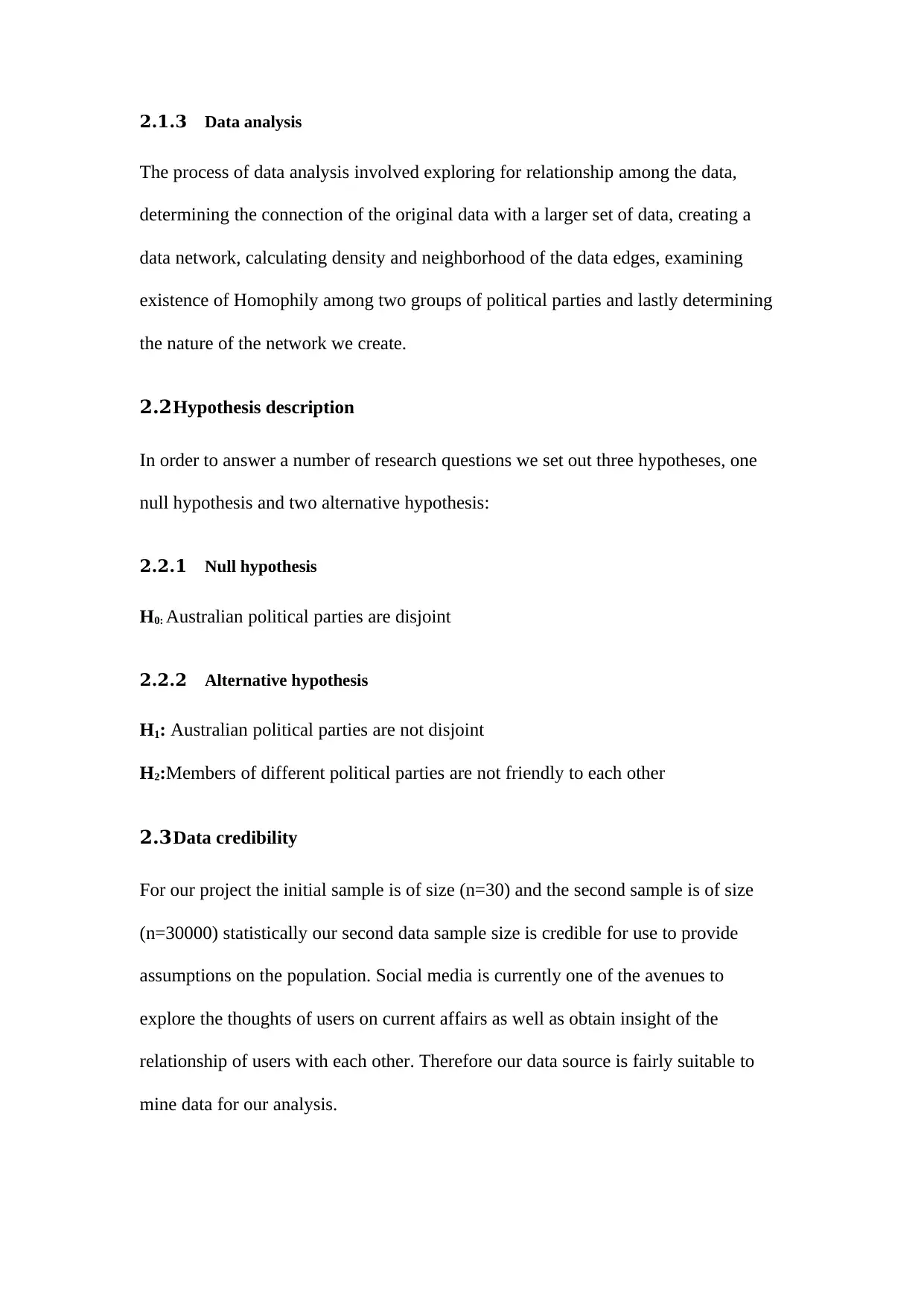
2.1.3 Data analysis
The process of data analysis involved exploring for relationship among the data,
determining the connection of the original data with a larger set of data, creating a
data network, calculating density and neighborhood of the data edges, examining
existence of Homophily among two groups of political parties and lastly determining
the nature of the network we create.
2.2Hypothesis description
In order to answer a number of research questions we set out three hypotheses, one
null hypothesis and two alternative hypothesis:
2.2.1 Null hypothesis
H0: Australian political parties are disjoint
2.2.2 Alternative hypothesis
H1: Australian political parties are not disjoint
H2:Members of different political parties are not friendly to each other
2.3Data credibility
For our project the initial sample is of size (n=30) and the second sample is of size
(n=30000) statistically our second data sample size is credible for use to provide
assumptions on the population. Social media is currently one of the avenues to
explore the thoughts of users on current affairs as well as obtain insight of the
relationship of users with each other. Therefore our data source is fairly suitable to
mine data for our analysis.
The process of data analysis involved exploring for relationship among the data,
determining the connection of the original data with a larger set of data, creating a
data network, calculating density and neighborhood of the data edges, examining
existence of Homophily among two groups of political parties and lastly determining
the nature of the network we create.
2.2Hypothesis description
In order to answer a number of research questions we set out three hypotheses, one
null hypothesis and two alternative hypothesis:
2.2.1 Null hypothesis
H0: Australian political parties are disjoint
2.2.2 Alternative hypothesis
H1: Australian political parties are not disjoint
H2:Members of different political parties are not friendly to each other
2.3Data credibility
For our project the initial sample is of size (n=30) and the second sample is of size
(n=30000) statistically our second data sample size is credible for use to provide
assumptions on the population. Social media is currently one of the avenues to
explore the thoughts of users on current affairs as well as obtain insight of the
relationship of users with each other. Therefore our data source is fairly suitable to
mine data for our analysis.
You're viewing a preview
Unlock full access by subscribing today!
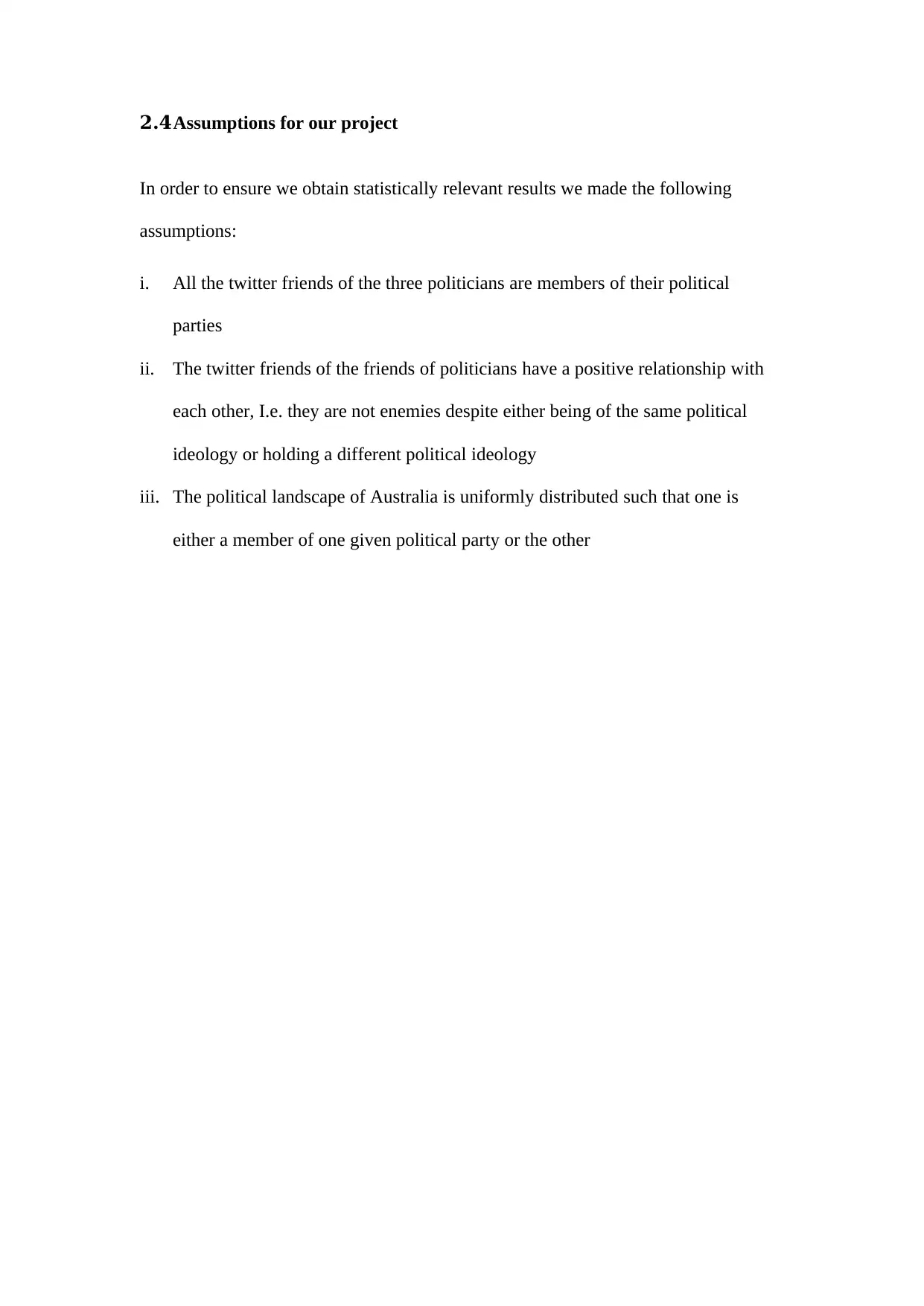
2.4Assumptions for our project
In order to ensure we obtain statistically relevant results we made the following
assumptions:
i. All the twitter friends of the three politicians are members of their political
parties
ii. The twitter friends of the friends of politicians have a positive relationship with
each other, I.e. they are not enemies despite either being of the same political
ideology or holding a different political ideology
iii. The political landscape of Australia is uniformly distributed such that one is
either a member of one given political party or the other
In order to ensure we obtain statistically relevant results we made the following
assumptions:
i. All the twitter friends of the three politicians are members of their political
parties
ii. The twitter friends of the friends of politicians have a positive relationship with
each other, I.e. they are not enemies despite either being of the same political
ideology or holding a different political ideology
iii. The political landscape of Australia is uniformly distributed such that one is
either a member of one given political party or the other
Paraphrase This Document
Need a fresh take? Get an instant paraphrase of this document with our AI Paraphraser
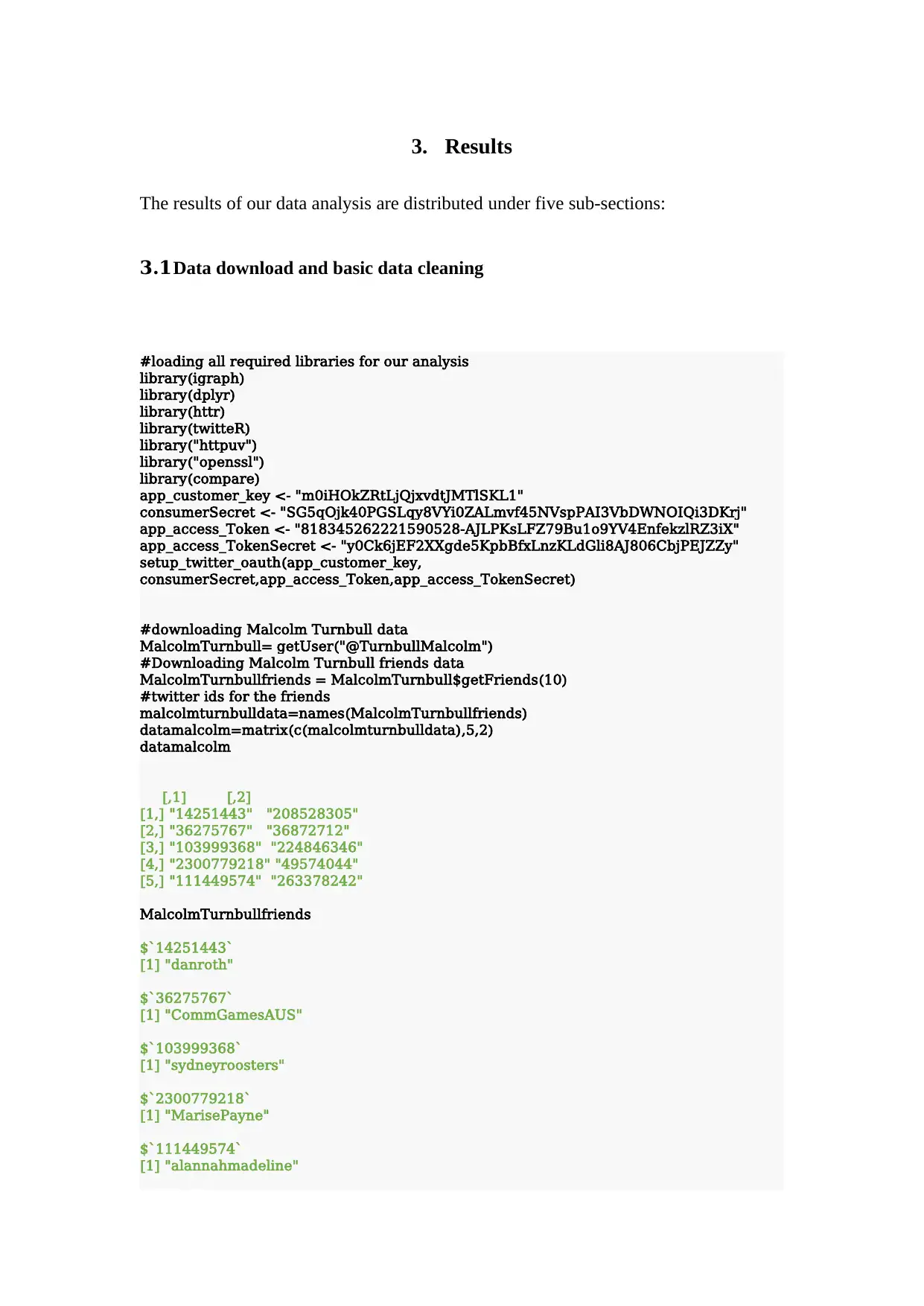
3. Results
The results of our data analysis are distributed under five sub-sections:
3.1Data download and basic data cleaning
#loading all required libraries for our analysis
library(igraph)
library(dplyr)
library(httr)
library(twitteR)
library("httpuv")
library("openssl")
library(compare)
app_customer_key <- "m0iHOkZRtLjQjxvdtJMTlSKL1"
consumerSecret <- "SG5qOjk40PGSLqy8VYi0ZALmvf45NVspPAI3VbDWNOIQi3DKrj"
app_access_Token <- "818345262221590528-AJLPKsLFZ79Bu1o9YV4EnfekzlRZ3iX"
app_access_TokenSecret <- "y0Ck6jEF2XXgde5KpbBfxLnzKLdGli8AJ806CbjPEJZZy"
setup_twitter_oauth(app_customer_key,
consumerSecret,app_access_Token,app_access_TokenSecret)
#downloading Malcolm Turnbull data
MalcolmTurnbull= getUser("@TurnbullMalcolm")
#Downloading Malcolm Turnbull friends data
MalcolmTurnbullfriends = MalcolmTurnbull$getFriends(10)
#twitter ids for the friends
malcolmturnbulldata=names(MalcolmTurnbullfriends)
datamalcolm=matrix(c(malcolmturnbulldata),5,2)
datamalcolm
[,1] [,2]
[1,] "14251443" "208528305"
[2,] "36275767" "36872712"
[3,] "103999368" "224846346"
[4,] "2300779218" "49574044"
[5,] "111449574" "263378242"
MalcolmTurnbullfriends
$`14251443`
[1] "danroth"
$`36275767`
[1] "CommGamesAUS"
$`103999368`
[1] "sydneyroosters"
$`2300779218`
[1] "MarisePayne"
$`111449574`
[1] "alannahmadeline"
The results of our data analysis are distributed under five sub-sections:
3.1Data download and basic data cleaning
#loading all required libraries for our analysis
library(igraph)
library(dplyr)
library(httr)
library(twitteR)
library("httpuv")
library("openssl")
library(compare)
app_customer_key <- "m0iHOkZRtLjQjxvdtJMTlSKL1"
consumerSecret <- "SG5qOjk40PGSLqy8VYi0ZALmvf45NVspPAI3VbDWNOIQi3DKrj"
app_access_Token <- "818345262221590528-AJLPKsLFZ79Bu1o9YV4EnfekzlRZ3iX"
app_access_TokenSecret <- "y0Ck6jEF2XXgde5KpbBfxLnzKLdGli8AJ806CbjPEJZZy"
setup_twitter_oauth(app_customer_key,
consumerSecret,app_access_Token,app_access_TokenSecret)
#downloading Malcolm Turnbull data
MalcolmTurnbull= getUser("@TurnbullMalcolm")
#Downloading Malcolm Turnbull friends data
MalcolmTurnbullfriends = MalcolmTurnbull$getFriends(10)
#twitter ids for the friends
malcolmturnbulldata=names(MalcolmTurnbullfriends)
datamalcolm=matrix(c(malcolmturnbulldata),5,2)
datamalcolm
[,1] [,2]
[1,] "14251443" "208528305"
[2,] "36275767" "36872712"
[3,] "103999368" "224846346"
[4,] "2300779218" "49574044"
[5,] "111449574" "263378242"
MalcolmTurnbullfriends
$`14251443`
[1] "danroth"
$`36275767`
[1] "CommGamesAUS"
$`103999368`
[1] "sydneyroosters"
$`2300779218`
[1] "MarisePayne"
$`111449574`
[1] "alannahmadeline"
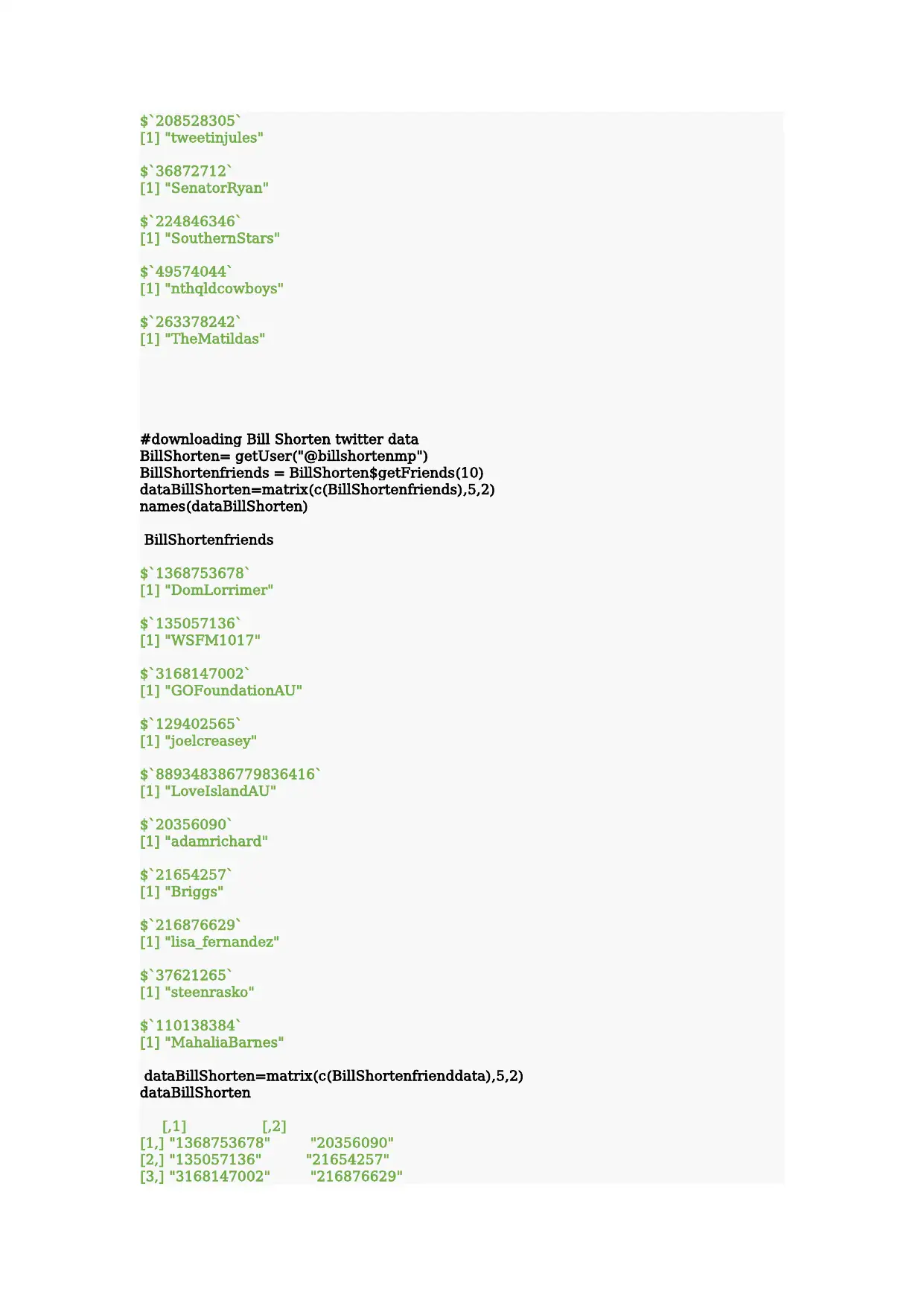
$`208528305`
[1] "tweetinjules"
$`36872712`
[1] "SenatorRyan"
$`224846346`
[1] "SouthernStars"
$`49574044`
[1] "nthqldcowboys"
$`263378242`
[1] "TheMatildas"
#downloading Bill Shorten twitter data
BillShorten= getUser("@billshortenmp")
BillShortenfriends = BillShorten$getFriends(10)
dataBillShorten=matrix(c(BillShortenfriends),5,2)
names(dataBillShorten)
BillShortenfriends
$`1368753678`
[1] "DomLorrimer"
$`135057136`
[1] "WSFM1017"
$`3168147002`
[1] "GOFoundationAU"
$`129402565`
[1] "joelcreasey"
$`889348386779836416`
[1] "LoveIslandAU"
$`20356090`
[1] "adamrichard"
$`21654257`
[1] "Briggs"
$`216876629`
[1] "lisa_fernandez"
$`37621265`
[1] "steenrasko"
$`110138384`
[1] "MahaliaBarnes"
dataBillShorten=matrix(c(BillShortenfrienddata),5,2)
dataBillShorten
[,1] [,2]
[1,] "1368753678" "20356090"
[2,] "135057136" "21654257"
[3,] "3168147002" "216876629"
[1] "tweetinjules"
$`36872712`
[1] "SenatorRyan"
$`224846346`
[1] "SouthernStars"
$`49574044`
[1] "nthqldcowboys"
$`263378242`
[1] "TheMatildas"
#downloading Bill Shorten twitter data
BillShorten= getUser("@billshortenmp")
BillShortenfriends = BillShorten$getFriends(10)
dataBillShorten=matrix(c(BillShortenfriends),5,2)
names(dataBillShorten)
BillShortenfriends
$`1368753678`
[1] "DomLorrimer"
$`135057136`
[1] "WSFM1017"
$`3168147002`
[1] "GOFoundationAU"
$`129402565`
[1] "joelcreasey"
$`889348386779836416`
[1] "LoveIslandAU"
$`20356090`
[1] "adamrichard"
$`21654257`
[1] "Briggs"
$`216876629`
[1] "lisa_fernandez"
$`37621265`
[1] "steenrasko"
$`110138384`
[1] "MahaliaBarnes"
dataBillShorten=matrix(c(BillShortenfrienddata),5,2)
dataBillShorten
[,1] [,2]
[1,] "1368753678" "20356090"
[2,] "135057136" "21654257"
[3,] "3168147002" "216876629"
You're viewing a preview
Unlock full access by subscribing today!
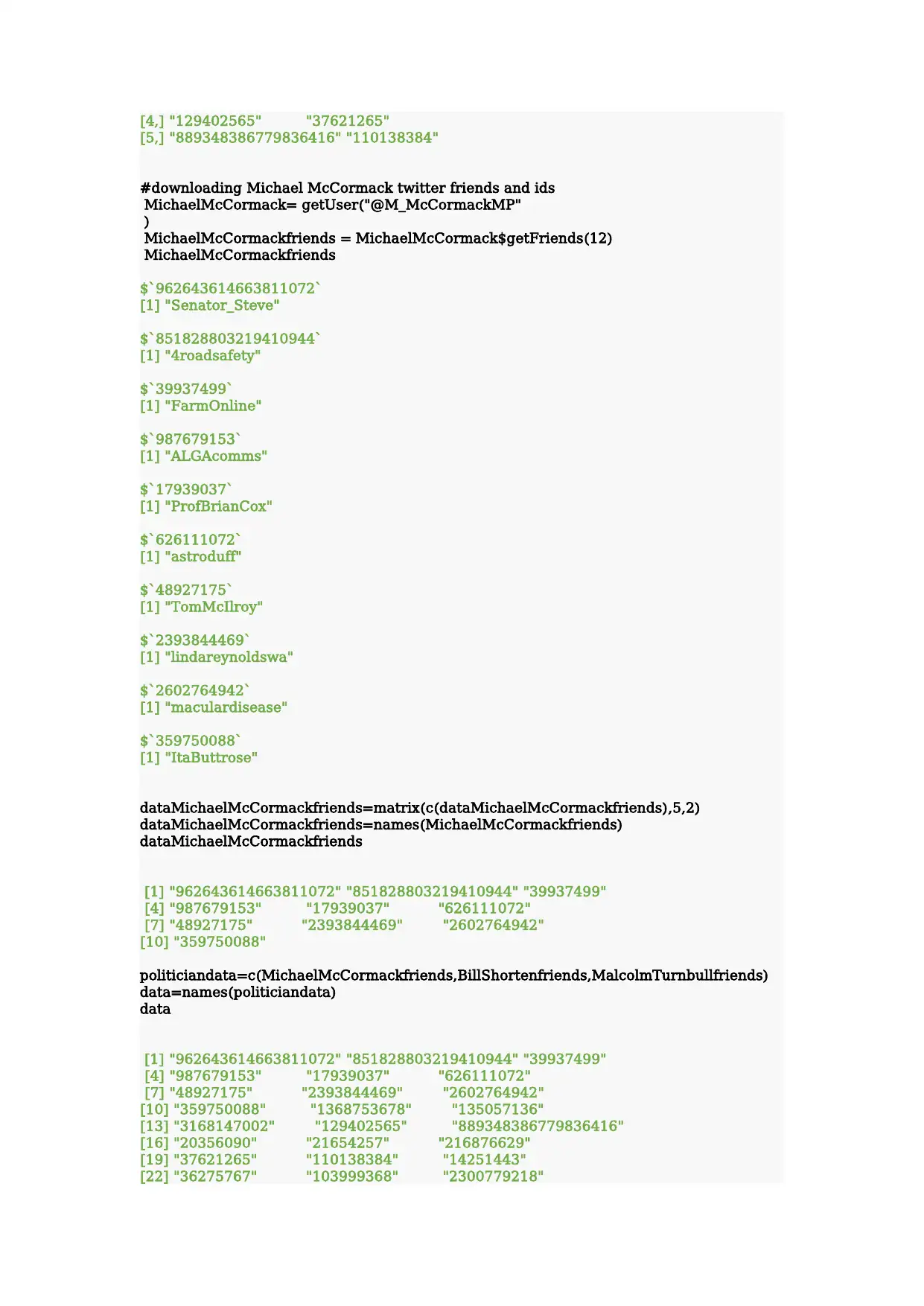
[4,] "129402565" "37621265"
[5,] "889348386779836416" "110138384"
#downloading Michael McCormack twitter friends and ids
MichaelMcCormack= getUser("@M_McCormackMP"
)
MichaelMcCormackfriends = MichaelMcCormack$getFriends(12)
MichaelMcCormackfriends
$`962643614663811072`
[1] "Senator_Steve"
$`851828803219410944`
[1] "4roadsafety"
$`39937499`
[1] "FarmOnline"
$`987679153`
[1] "ALGAcomms"
$`17939037`
[1] "ProfBrianCox"
$`626111072`
[1] "astroduff"
$`48927175`
[1] "TomMcIlroy"
$`2393844469`
[1] "lindareynoldswa"
$`2602764942`
[1] "maculardisease"
$`359750088`
[1] "ItaButtrose"
dataMichaelMcCormackfriends=matrix(c(dataMichaelMcCormackfriends),5,2)
dataMichaelMcCormackfriends=names(MichaelMcCormackfriends)
dataMichaelMcCormackfriends
[1] "962643614663811072" "851828803219410944" "39937499"
[4] "987679153" "17939037" "626111072"
[7] "48927175" "2393844469" "2602764942"
[10] "359750088"
politiciandata=c(MichaelMcCormackfriends,BillShortenfriends,MalcolmTurnbullfriends)
data=names(politiciandata)
data
[1] "962643614663811072" "851828803219410944" "39937499"
[4] "987679153" "17939037" "626111072"
[7] "48927175" "2393844469" "2602764942"
[10] "359750088" "1368753678" "135057136"
[13] "3168147002" "129402565" "889348386779836416"
[16] "20356090" "21654257" "216876629"
[19] "37621265" "110138384" "14251443"
[22] "36275767" "103999368" "2300779218"
[5,] "889348386779836416" "110138384"
#downloading Michael McCormack twitter friends and ids
MichaelMcCormack= getUser("@M_McCormackMP"
)
MichaelMcCormackfriends = MichaelMcCormack$getFriends(12)
MichaelMcCormackfriends
$`962643614663811072`
[1] "Senator_Steve"
$`851828803219410944`
[1] "4roadsafety"
$`39937499`
[1] "FarmOnline"
$`987679153`
[1] "ALGAcomms"
$`17939037`
[1] "ProfBrianCox"
$`626111072`
[1] "astroduff"
$`48927175`
[1] "TomMcIlroy"
$`2393844469`
[1] "lindareynoldswa"
$`2602764942`
[1] "maculardisease"
$`359750088`
[1] "ItaButtrose"
dataMichaelMcCormackfriends=matrix(c(dataMichaelMcCormackfriends),5,2)
dataMichaelMcCormackfriends=names(MichaelMcCormackfriends)
dataMichaelMcCormackfriends
[1] "962643614663811072" "851828803219410944" "39937499"
[4] "987679153" "17939037" "626111072"
[7] "48927175" "2393844469" "2602764942"
[10] "359750088"
politiciandata=c(MichaelMcCormackfriends,BillShortenfriends,MalcolmTurnbullfriends)
data=names(politiciandata)
data
[1] "962643614663811072" "851828803219410944" "39937499"
[4] "987679153" "17939037" "626111072"
[7] "48927175" "2393844469" "2602764942"
[10] "359750088" "1368753678" "135057136"
[13] "3168147002" "129402565" "889348386779836416"
[16] "20356090" "21654257" "216876629"
[19] "37621265" "110138384" "14251443"
[22] "36275767" "103999368" "2300779218"
Paraphrase This Document
Need a fresh take? Get an instant paraphrase of this document with our AI Paraphraser
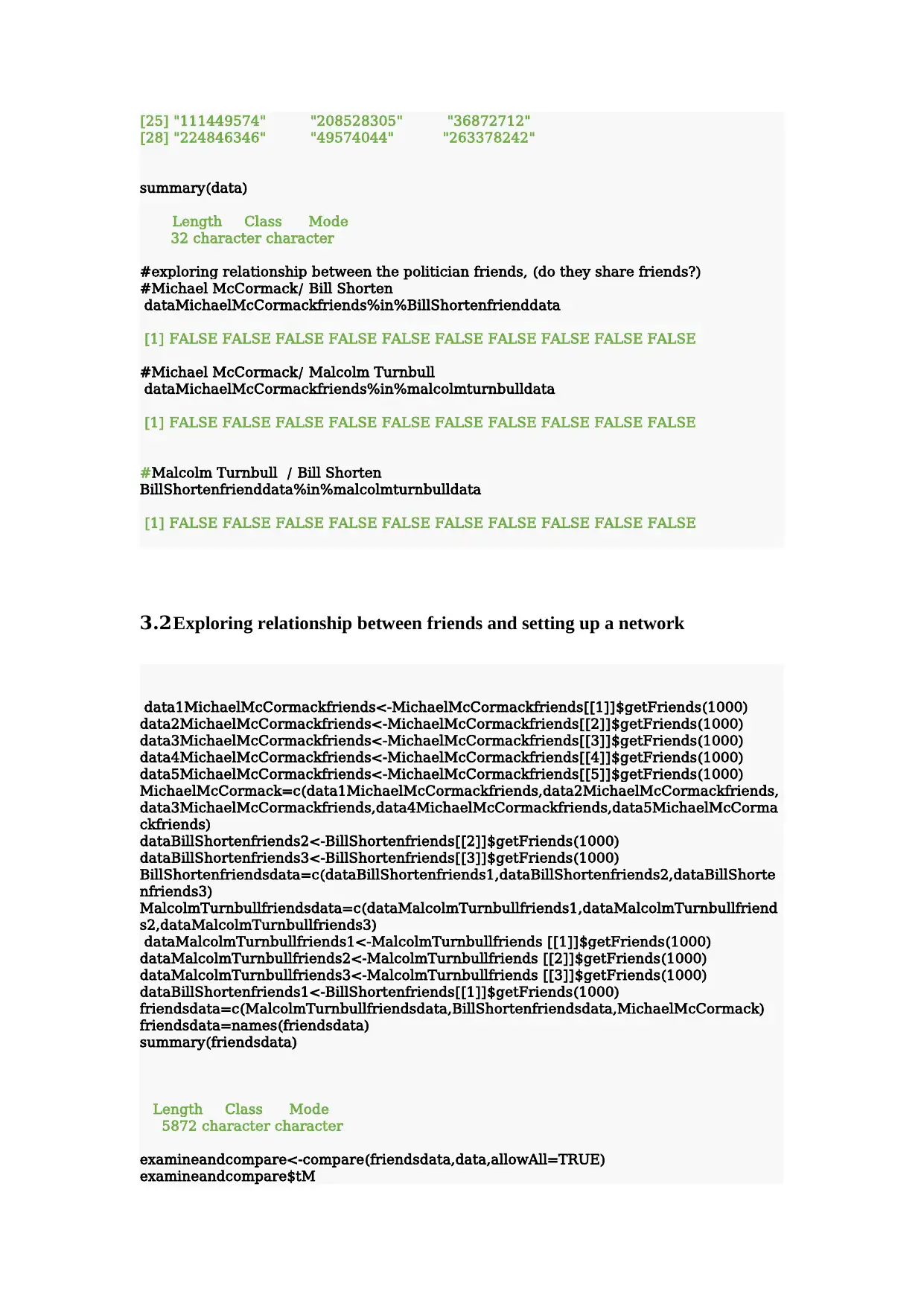
[25] "111449574" "208528305" "36872712"
[28] "224846346" "49574044" "263378242"
summary(data)
Length Class Mode
32 character character
#exploring relationship between the politician friends, (do they share friends?)
#Michael McCormack/ Bill Shorten
dataMichaelMcCormackfriends%in%BillShortenfrienddata
[1] FALSE FALSE FALSE FALSE FALSE FALSE FALSE FALSE FALSE FALSE
#Michael McCormack/ Malcolm Turnbull
dataMichaelMcCormackfriends%in%malcolmturnbulldata
[1] FALSE FALSE FALSE FALSE FALSE FALSE FALSE FALSE FALSE FALSE
#Malcolm Turnbull / Bill Shorten
BillShortenfrienddata%in%malcolmturnbulldata
[1] FALSE FALSE FALSE FALSE FALSE FALSE FALSE FALSE FALSE FALSE
3.2Exploring relationship between friends and setting up a network
data1MichaelMcCormackfriends<-MichaelMcCormackfriends[[1]]$getFriends(1000)
data2MichaelMcCormackfriends<-MichaelMcCormackfriends[[2]]$getFriends(1000)
data3MichaelMcCormackfriends<-MichaelMcCormackfriends[[3]]$getFriends(1000)
data4MichaelMcCormackfriends<-MichaelMcCormackfriends[[4]]$getFriends(1000)
data5MichaelMcCormackfriends<-MichaelMcCormackfriends[[5]]$getFriends(1000)
MichaelMcCormack=c(data1MichaelMcCormackfriends,data2MichaelMcCormackfriends,
data3MichaelMcCormackfriends,data4MichaelMcCormackfriends,data5MichaelMcCorma
ckfriends)
dataBillShortenfriends2<-BillShortenfriends[[2]]$getFriends(1000)
dataBillShortenfriends3<-BillShortenfriends[[3]]$getFriends(1000)
BillShortenfriendsdata=c(dataBillShortenfriends1,dataBillShortenfriends2,dataBillShorte
nfriends3)
MalcolmTurnbullfriendsdata=c(dataMalcolmTurnbullfriends1,dataMalcolmTurnbullfriend
s2,dataMalcolmTurnbullfriends3)
dataMalcolmTurnbullfriends1<-MalcolmTurnbullfriends [[1]]$getFriends(1000)
dataMalcolmTurnbullfriends2<-MalcolmTurnbullfriends [[2]]$getFriends(1000)
dataMalcolmTurnbullfriends3<-MalcolmTurnbullfriends [[3]]$getFriends(1000)
dataBillShortenfriends1<-BillShortenfriends[[1]]$getFriends(1000)
friendsdata=c(MalcolmTurnbullfriendsdata,BillShortenfriendsdata,MichaelMcCormack)
friendsdata=names(friendsdata)
summary(friendsdata)
Length Class Mode
5872 character character
examineandcompare<-compare(friendsdata,data,allowAll=TRUE)
examineandcompare$tM
[28] "224846346" "49574044" "263378242"
summary(data)
Length Class Mode
32 character character
#exploring relationship between the politician friends, (do they share friends?)
#Michael McCormack/ Bill Shorten
dataMichaelMcCormackfriends%in%BillShortenfrienddata
[1] FALSE FALSE FALSE FALSE FALSE FALSE FALSE FALSE FALSE FALSE
#Michael McCormack/ Malcolm Turnbull
dataMichaelMcCormackfriends%in%malcolmturnbulldata
[1] FALSE FALSE FALSE FALSE FALSE FALSE FALSE FALSE FALSE FALSE
#Malcolm Turnbull / Bill Shorten
BillShortenfrienddata%in%malcolmturnbulldata
[1] FALSE FALSE FALSE FALSE FALSE FALSE FALSE FALSE FALSE FALSE
3.2Exploring relationship between friends and setting up a network
data1MichaelMcCormackfriends<-MichaelMcCormackfriends[[1]]$getFriends(1000)
data2MichaelMcCormackfriends<-MichaelMcCormackfriends[[2]]$getFriends(1000)
data3MichaelMcCormackfriends<-MichaelMcCormackfriends[[3]]$getFriends(1000)
data4MichaelMcCormackfriends<-MichaelMcCormackfriends[[4]]$getFriends(1000)
data5MichaelMcCormackfriends<-MichaelMcCormackfriends[[5]]$getFriends(1000)
MichaelMcCormack=c(data1MichaelMcCormackfriends,data2MichaelMcCormackfriends,
data3MichaelMcCormackfriends,data4MichaelMcCormackfriends,data5MichaelMcCorma
ckfriends)
dataBillShortenfriends2<-BillShortenfriends[[2]]$getFriends(1000)
dataBillShortenfriends3<-BillShortenfriends[[3]]$getFriends(1000)
BillShortenfriendsdata=c(dataBillShortenfriends1,dataBillShortenfriends2,dataBillShorte
nfriends3)
MalcolmTurnbullfriendsdata=c(dataMalcolmTurnbullfriends1,dataMalcolmTurnbullfriend
s2,dataMalcolmTurnbullfriends3)
dataMalcolmTurnbullfriends1<-MalcolmTurnbullfriends [[1]]$getFriends(1000)
dataMalcolmTurnbullfriends2<-MalcolmTurnbullfriends [[2]]$getFriends(1000)
dataMalcolmTurnbullfriends3<-MalcolmTurnbullfriends [[3]]$getFriends(1000)
dataBillShortenfriends1<-BillShortenfriends[[1]]$getFriends(1000)
friendsdata=c(MalcolmTurnbullfriendsdata,BillShortenfriendsdata,MichaelMcCormack)
friendsdata=names(friendsdata)
summary(friendsdata)
Length Class Mode
5872 character character
examineandcompare<-compare(friendsdata,data,allowAll=TRUE)
examineandcompare$tM
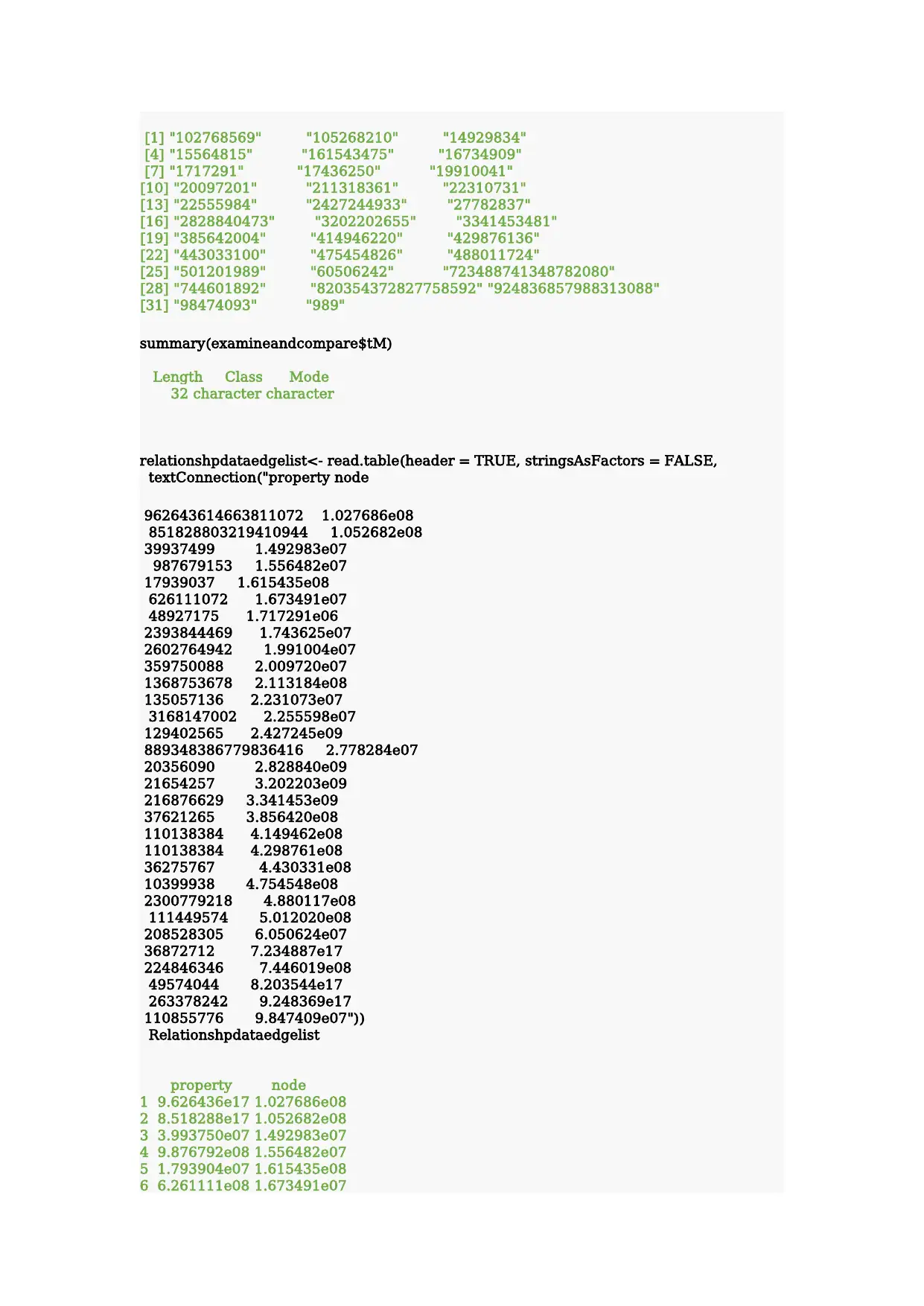
[1] "102768569" "105268210" "14929834"
[4] "15564815" "161543475" "16734909"
[7] "1717291" "17436250" "19910041"
[10] "20097201" "211318361" "22310731"
[13] "22555984" "2427244933" "27782837"
[16] "2828840473" "3202202655" "3341453481"
[19] "385642004" "414946220" "429876136"
[22] "443033100" "475454826" "488011724"
[25] "501201989" "60506242" "723488741348782080"
[28] "744601892" "820354372827758592" "924836857988313088"
[31] "98474093" "989"
summary(examineandcompare$tM)
Length Class Mode
32 character character
relationshpdataedgelist<- read.table(header = TRUE, stringsAsFactors = FALSE,
textConnection("property node
962643614663811072 1.027686e08
851828803219410944 1.052682e08
39937499 1.492983e07
987679153 1.556482e07
17939037 1.615435e08
626111072 1.673491e07
48927175 1.717291e06
2393844469 1.743625e07
2602764942 1.991004e07
359750088 2.009720e07
1368753678 2.113184e08
135057136 2.231073e07
3168147002 2.255598e07
129402565 2.427245e09
889348386779836416 2.778284e07
20356090 2.828840e09
21654257 3.202203e09
216876629 3.341453e09
37621265 3.856420e08
110138384 4.149462e08
110138384 4.298761e08
36275767 4.430331e08
10399938 4.754548e08
2300779218 4.880117e08
111449574 5.012020e08
208528305 6.050624e07
36872712 7.234887e17
224846346 7.446019e08
49574044 8.203544e17
263378242 9.248369e17
110855776 9.847409e07"))
Relationshpdataedgelist
property node
1 9.626436e17 1.027686e08
2 8.518288e17 1.052682e08
3 3.993750e07 1.492983e07
4 9.876792e08 1.556482e07
5 1.793904e07 1.615435e08
6 6.261111e08 1.673491e07
[4] "15564815" "161543475" "16734909"
[7] "1717291" "17436250" "19910041"
[10] "20097201" "211318361" "22310731"
[13] "22555984" "2427244933" "27782837"
[16] "2828840473" "3202202655" "3341453481"
[19] "385642004" "414946220" "429876136"
[22] "443033100" "475454826" "488011724"
[25] "501201989" "60506242" "723488741348782080"
[28] "744601892" "820354372827758592" "924836857988313088"
[31] "98474093" "989"
summary(examineandcompare$tM)
Length Class Mode
32 character character
relationshpdataedgelist<- read.table(header = TRUE, stringsAsFactors = FALSE,
textConnection("property node
962643614663811072 1.027686e08
851828803219410944 1.052682e08
39937499 1.492983e07
987679153 1.556482e07
17939037 1.615435e08
626111072 1.673491e07
48927175 1.717291e06
2393844469 1.743625e07
2602764942 1.991004e07
359750088 2.009720e07
1368753678 2.113184e08
135057136 2.231073e07
3168147002 2.255598e07
129402565 2.427245e09
889348386779836416 2.778284e07
20356090 2.828840e09
21654257 3.202203e09
216876629 3.341453e09
37621265 3.856420e08
110138384 4.149462e08
110138384 4.298761e08
36275767 4.430331e08
10399938 4.754548e08
2300779218 4.880117e08
111449574 5.012020e08
208528305 6.050624e07
36872712 7.234887e17
224846346 7.446019e08
49574044 8.203544e17
263378242 9.248369e17
110855776 9.847409e07"))
Relationshpdataedgelist
property node
1 9.626436e17 1.027686e08
2 8.518288e17 1.052682e08
3 3.993750e07 1.492983e07
4 9.876792e08 1.556482e07
5 1.793904e07 1.615435e08
6 6.261111e08 1.673491e07
You're viewing a preview
Unlock full access by subscribing today!
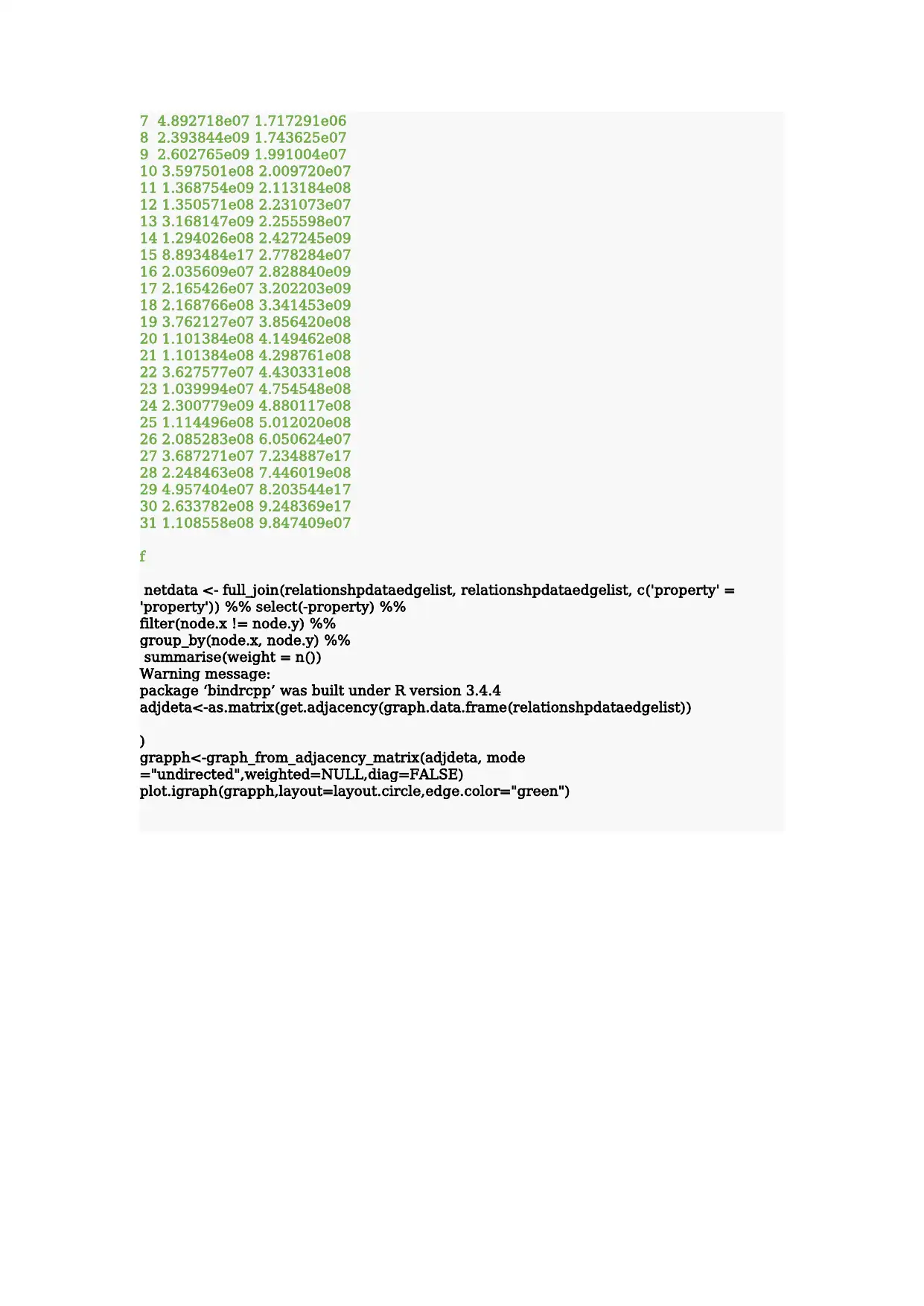
7 4.892718e07 1.717291e06
8 2.393844e09 1.743625e07
9 2.602765e09 1.991004e07
10 3.597501e08 2.009720e07
11 1.368754e09 2.113184e08
12 1.350571e08 2.231073e07
13 3.168147e09 2.255598e07
14 1.294026e08 2.427245e09
15 8.893484e17 2.778284e07
16 2.035609e07 2.828840e09
17 2.165426e07 3.202203e09
18 2.168766e08 3.341453e09
19 3.762127e07 3.856420e08
20 1.101384e08 4.149462e08
21 1.101384e08 4.298761e08
22 3.627577e07 4.430331e08
23 1.039994e07 4.754548e08
24 2.300779e09 4.880117e08
25 1.114496e08 5.012020e08
26 2.085283e08 6.050624e07
27 3.687271e07 7.234887e17
28 2.248463e08 7.446019e08
29 4.957404e07 8.203544e17
30 2.633782e08 9.248369e17
31 1.108558e08 9.847409e07
f
netdata <- full_join(relationshpdataedgelist, relationshpdataedgelist, c('property' =
'property')) %% select(-property) %%
filter(node.x != node.y) %%
group_by(node.x, node.y) %%
summarise(weight = n())
Warning message:
package ‘bindrcpp’ was built under R version 3.4.4
adjdeta<-as.matrix(get.adjacency(graph.data.frame(relationshpdataedgelist))
)
grapph<-graph_from_adjacency_matrix(adjdeta, mode
="undirected",weighted=NULL,diag=FALSE)
plot.igraph(grapph,layout=layout.circle,edge.color="green")
8 2.393844e09 1.743625e07
9 2.602765e09 1.991004e07
10 3.597501e08 2.009720e07
11 1.368754e09 2.113184e08
12 1.350571e08 2.231073e07
13 3.168147e09 2.255598e07
14 1.294026e08 2.427245e09
15 8.893484e17 2.778284e07
16 2.035609e07 2.828840e09
17 2.165426e07 3.202203e09
18 2.168766e08 3.341453e09
19 3.762127e07 3.856420e08
20 1.101384e08 4.149462e08
21 1.101384e08 4.298761e08
22 3.627577e07 4.430331e08
23 1.039994e07 4.754548e08
24 2.300779e09 4.880117e08
25 1.114496e08 5.012020e08
26 2.085283e08 6.050624e07
27 3.687271e07 7.234887e17
28 2.248463e08 7.446019e08
29 4.957404e07 8.203544e17
30 2.633782e08 9.248369e17
31 1.108558e08 9.847409e07
f
netdata <- full_join(relationshpdataedgelist, relationshpdataedgelist, c('property' =
'property')) %% select(-property) %%
filter(node.x != node.y) %%
group_by(node.x, node.y) %%
summarise(weight = n())
Warning message:
package ‘bindrcpp’ was built under R version 3.4.4
adjdeta<-as.matrix(get.adjacency(graph.data.frame(relationshpdataedgelist))
)
grapph<-graph_from_adjacency_matrix(adjdeta, mode
="undirected",weighted=NULL,diag=FALSE)
plot.igraph(grapph,layout=layout.circle,edge.color="green")
Paraphrase This Document
Need a fresh take? Get an instant paraphrase of this document with our AI Paraphraser
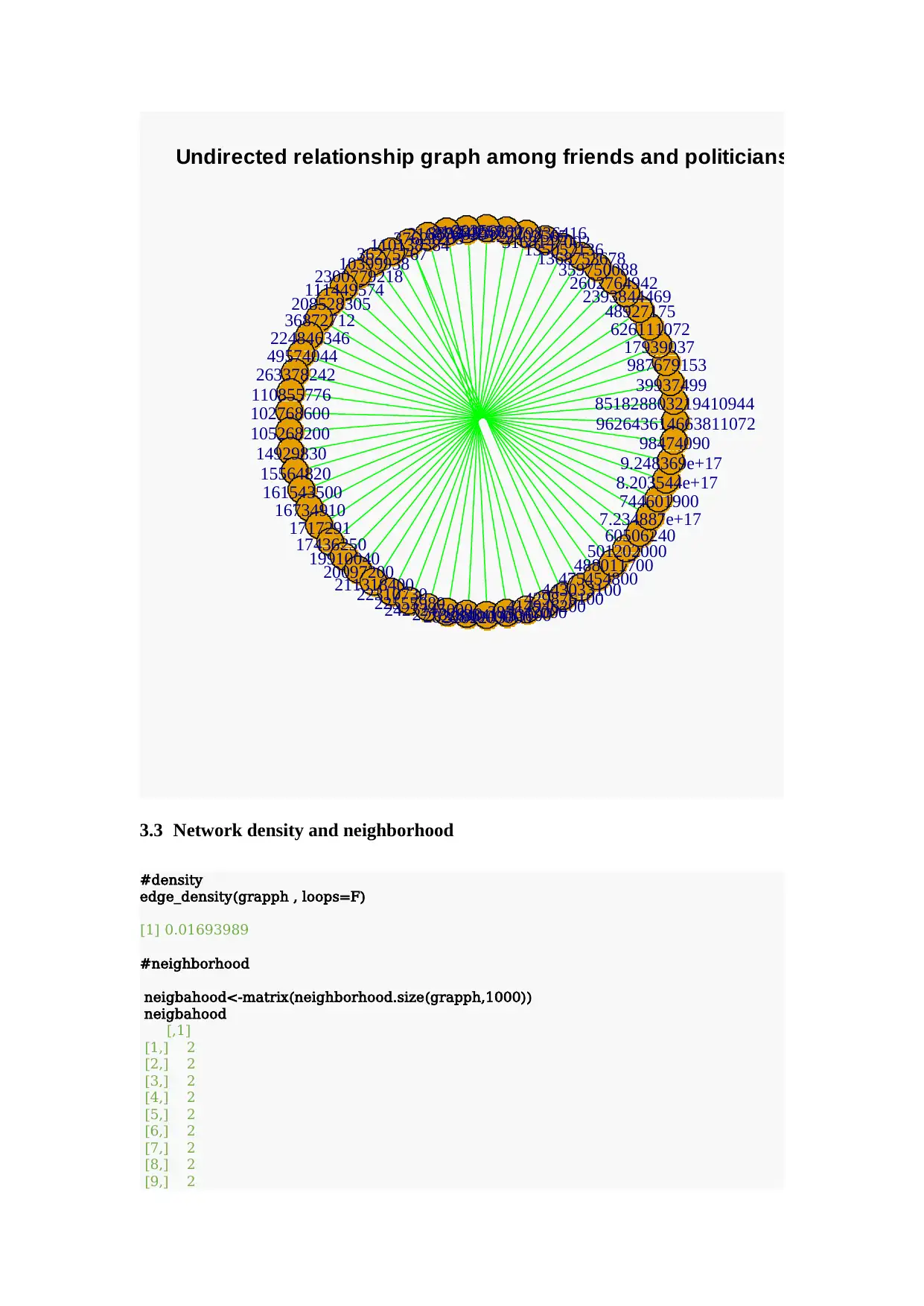
Undirected relationship graph among friends and politicians
962643614663811072
851828803219410944
39937499
987679153
17939037
626111072
48927175
2393844469
2602764942
359750088
1368753678135057136316814700212940256588934838677983641620356090216542572168766293762126511013838436275767
10399938
2300779218
111449574
208528305
36872712
224846346
49574044
263378242
110855776
102768600
105268200
14929830
15564820
161543500
16734910
1717291
17436250
19910040
20097200
211318400
2231073022555980242724500027782840282884000032022030003341453000385642000414946200429876100443033100
475454800
488011700
501202000
60506240
7.234887e+17
744601900
8.203544e+17
9.248369e+17
98474090
3.3 Network density and neighborhood
#density
edge_density(grapph , loops=F)
[1] 0.01693989
#neighborhood
neigbahood<-matrix(neighborhood.size(grapph,1000))
neigbahood
[,1]
[1,] 2
[2,] 2
[3,] 2
[4,] 2
[5,] 2
[6,] 2
[7,] 2
[8,] 2
[9,] 2
962643614663811072
851828803219410944
39937499
987679153
17939037
626111072
48927175
2393844469
2602764942
359750088
1368753678135057136316814700212940256588934838677983641620356090216542572168766293762126511013838436275767
10399938
2300779218
111449574
208528305
36872712
224846346
49574044
263378242
110855776
102768600
105268200
14929830
15564820
161543500
16734910
1717291
17436250
19910040
20097200
211318400
2231073022555980242724500027782840282884000032022030003341453000385642000414946200429876100443033100
475454800
488011700
501202000
60506240
7.234887e+17
744601900
8.203544e+17
9.248369e+17
98474090
3.3 Network density and neighborhood
#density
edge_density(grapph , loops=F)
[1] 0.01693989
#neighborhood
neigbahood<-matrix(neighborhood.size(grapph,1000))
neigbahood
[,1]
[1,] 2
[2,] 2
[3,] 2
[4,] 2
[5,] 2
[6,] 2
[7,] 2
[8,] 2
[9,] 2
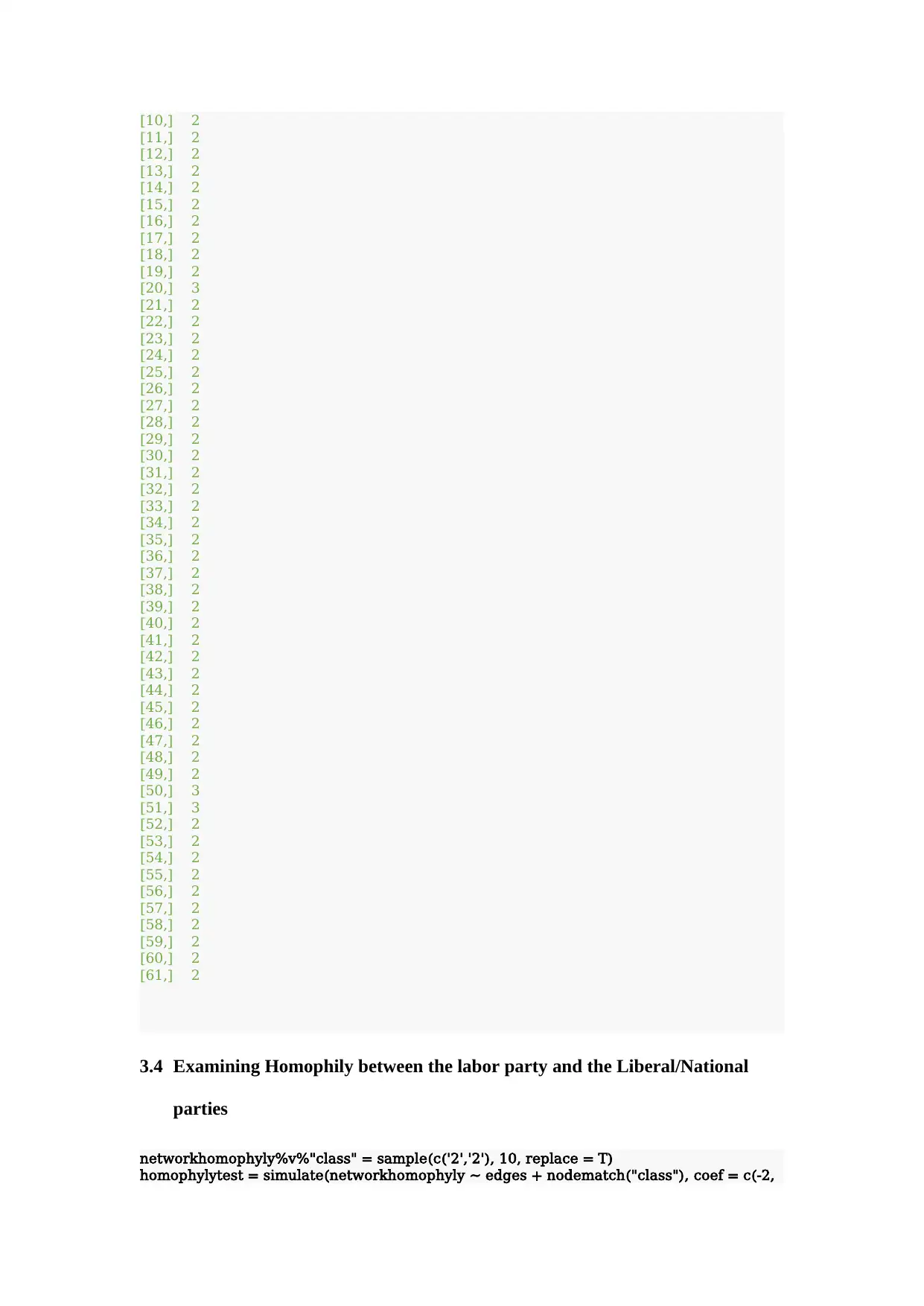
[10,] 2
[11,] 2
[12,] 2
[13,] 2
[14,] 2
[15,] 2
[16,] 2
[17,] 2
[18,] 2
[19,] 2
[20,] 3
[21,] 2
[22,] 2
[23,] 2
[24,] 2
[25,] 2
[26,] 2
[27,] 2
[28,] 2
[29,] 2
[30,] 2
[31,] 2
[32,] 2
[33,] 2
[34,] 2
[35,] 2
[36,] 2
[37,] 2
[38,] 2
[39,] 2
[40,] 2
[41,] 2
[42,] 2
[43,] 2
[44,] 2
[45,] 2
[46,] 2
[47,] 2
[48,] 2
[49,] 2
[50,] 3
[51,] 3
[52,] 2
[53,] 2
[54,] 2
[55,] 2
[56,] 2
[57,] 2
[58,] 2
[59,] 2
[60,] 2
[61,] 2
3.4 Examining Homophily between the labor party and the Liberal/National
parties
networkhomophyly%v%"class" = sample(c('2','2'), 10, replace = T)
homophylytest = simulate(networkhomophyly ~ edges + nodematch("class"), coef = c(-2,
[11,] 2
[12,] 2
[13,] 2
[14,] 2
[15,] 2
[16,] 2
[17,] 2
[18,] 2
[19,] 2
[20,] 3
[21,] 2
[22,] 2
[23,] 2
[24,] 2
[25,] 2
[26,] 2
[27,] 2
[28,] 2
[29,] 2
[30,] 2
[31,] 2
[32,] 2
[33,] 2
[34,] 2
[35,] 2
[36,] 2
[37,] 2
[38,] 2
[39,] 2
[40,] 2
[41,] 2
[42,] 2
[43,] 2
[44,] 2
[45,] 2
[46,] 2
[47,] 2
[48,] 2
[49,] 2
[50,] 3
[51,] 3
[52,] 2
[53,] 2
[54,] 2
[55,] 2
[56,] 2
[57,] 2
[58,] 2
[59,] 2
[60,] 2
[61,] 2
3.4 Examining Homophily between the labor party and the Liberal/National
parties
networkhomophyly%v%"class" = sample(c('2','2'), 10, replace = T)
homophylytest = simulate(networkhomophyly ~ edges + nodematch("class"), coef = c(-2,
You're viewing a preview
Unlock full access by subscribing today!
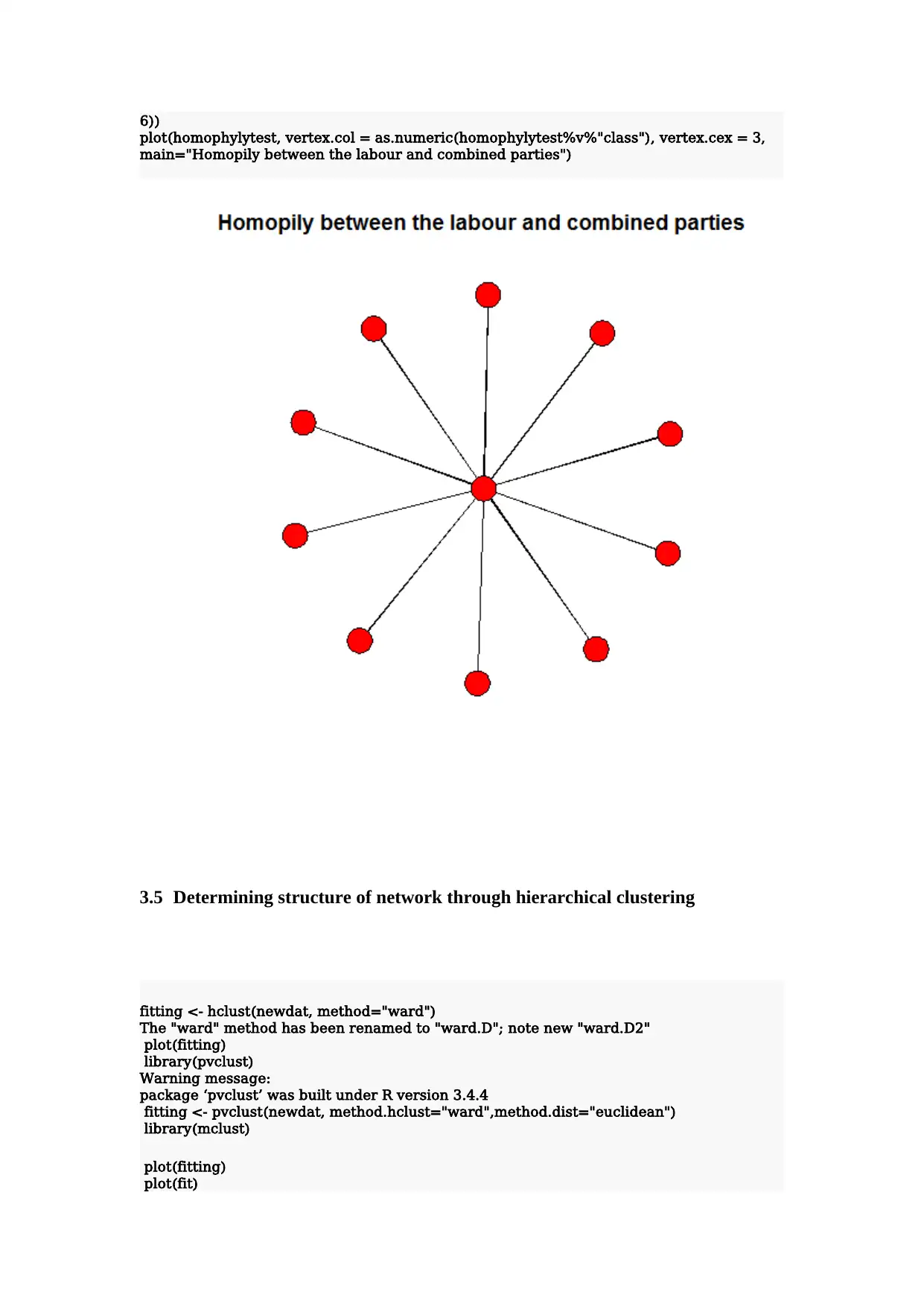
6))
plot(homophylytest, vertex.col = as.numeric(homophylytest%v%"class"), vertex.cex = 3,
main="Homopily between the labour and combined parties")
3.5 Determining structure of network through hierarchical clustering
fitting <- hclust(newdat, method="ward")
The "ward" method has been renamed to "ward.D"; note new "ward.D2"
plot(fitting)
library(pvclust)
Warning message:
package ‘pvclust’ was built under R version 3.4.4
fitting <- pvclust(newdat, method.hclust="ward",method.dist="euclidean")
library(mclust)
plot(fitting)
plot(fit)
plot(homophylytest, vertex.col = as.numeric(homophylytest%v%"class"), vertex.cex = 3,
main="Homopily between the labour and combined parties")
3.5 Determining structure of network through hierarchical clustering
fitting <- hclust(newdat, method="ward")
The "ward" method has been renamed to "ward.D"; note new "ward.D2"
plot(fitting)
library(pvclust)
Warning message:
package ‘pvclust’ was built under R version 3.4.4
fitting <- pvclust(newdat, method.hclust="ward",method.dist="euclidean")
library(mclust)
plot(fitting)
plot(fit)
Paraphrase This Document
Need a fresh take? Get an instant paraphrase of this document with our AI Paraphraser
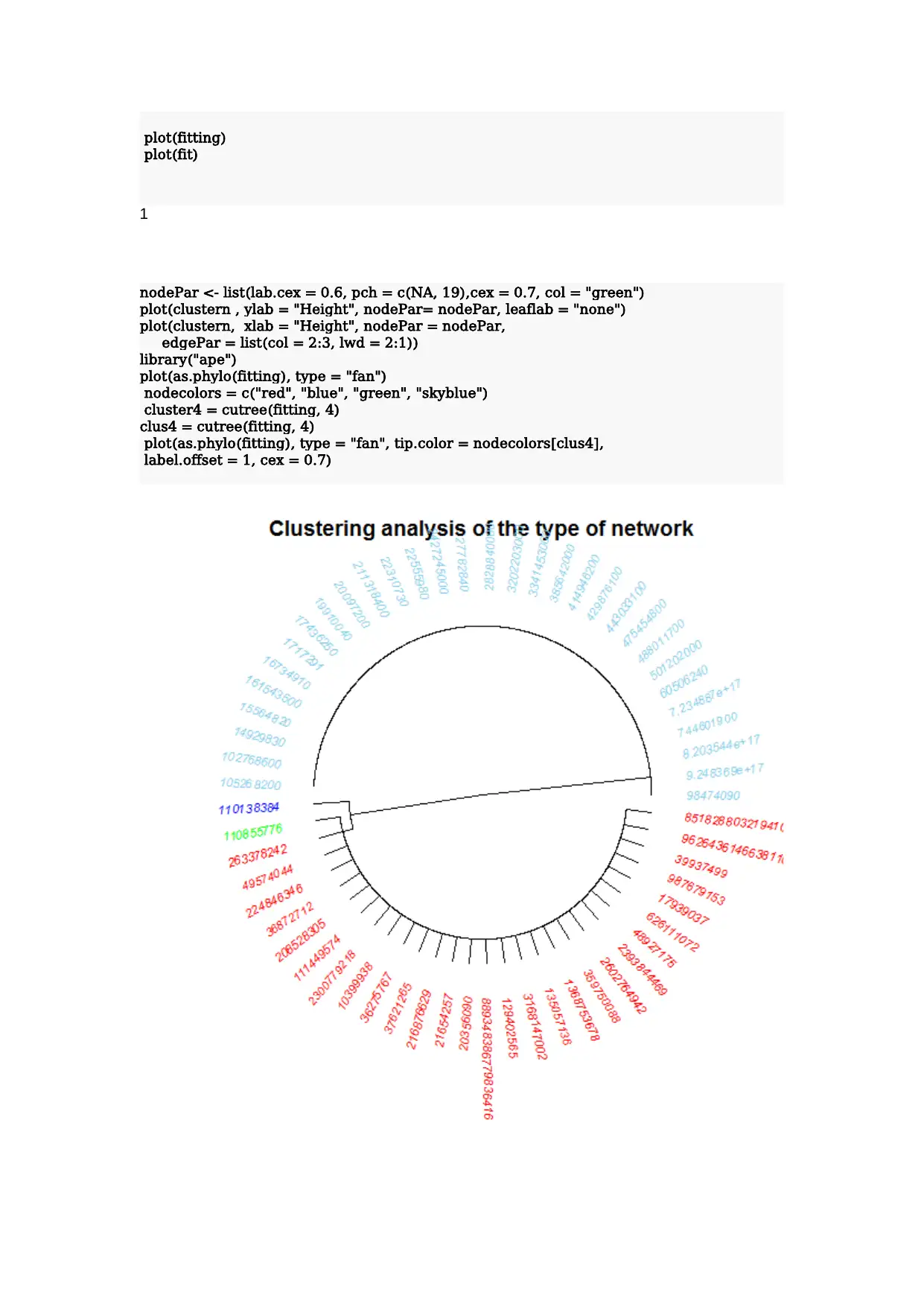
plot(fitting)
plot(fit)
1
nodePar <- list(lab.cex = 0.6, pch = c(NA, 19),cex = 0.7, col = "green")
plot(clustern , ylab = "Height", nodePar= nodePar, leaflab = "none")
plot(clustern, xlab = "Height", nodePar = nodePar,
edgePar = list(col = 2:3, lwd = 2:1))
library("ape")
plot(as.phylo(fitting), type = "fan")
nodecolors = c("red", "blue", "green", "skyblue")
cluster4 = cutree(fitting, 4)
clus4 = cutree(fitting, 4)
plot(as.phylo(fitting), type = "fan", tip.color = nodecolors[clus4],
label.offset = 1, cex = 0.7)
plot(fit)
1
nodePar <- list(lab.cex = 0.6, pch = c(NA, 19),cex = 0.7, col = "green")
plot(clustern , ylab = "Height", nodePar= nodePar, leaflab = "none")
plot(clustern, xlab = "Height", nodePar = nodePar,
edgePar = list(col = 2:3, lwd = 2:1))
library("ape")
plot(as.phylo(fitting), type = "fan")
nodecolors = c("red", "blue", "green", "skyblue")
cluster4 = cutree(fitting, 4)
clus4 = cutree(fitting, 4)
plot(as.phylo(fitting), type = "fan", tip.color = nodecolors[clus4],
label.offset = 1, cex = 0.7)
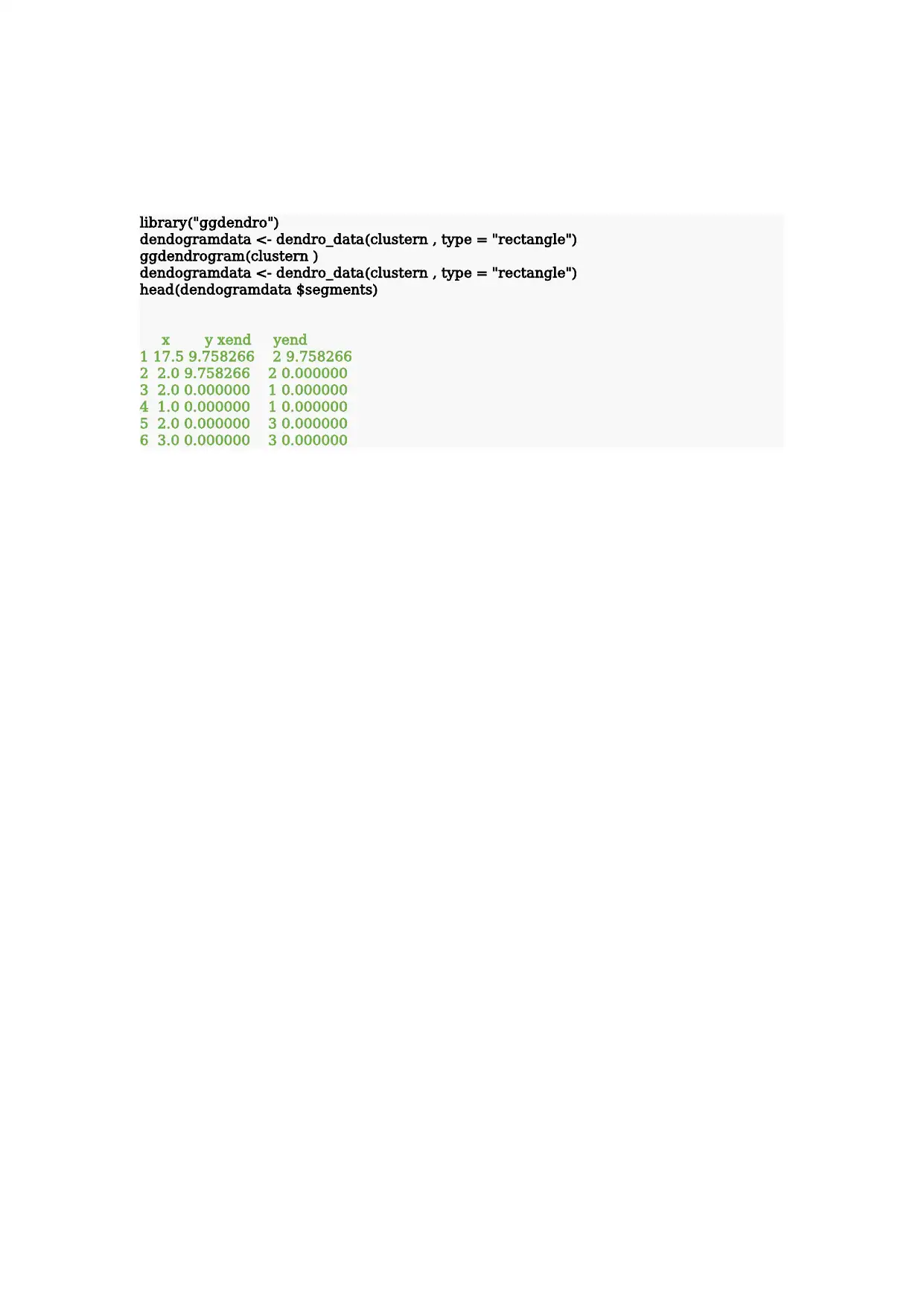
library("ggdendro")
dendogramdata <- dendro_data(clustern , type = "rectangle")
ggdendrogram(clustern )
dendogramdata <- dendro_data(clustern , type = "rectangle")
head(dendogramdata $segments)
x y xend yend
1 17.5 9.758266 2 9.758266
2 2.0 9.758266 2 0.000000
3 2.0 0.000000 1 0.000000
4 1.0 0.000000 1 0.000000
5 2.0 0.000000 3 0.000000
6 3.0 0.000000 3 0.000000
dendogramdata <- dendro_data(clustern , type = "rectangle")
ggdendrogram(clustern )
dendogramdata <- dendro_data(clustern , type = "rectangle")
head(dendogramdata $segments)
x y xend yend
1 17.5 9.758266 2 9.758266
2 2.0 9.758266 2 0.000000
3 2.0 0.000000 1 0.000000
4 1.0 0.000000 1 0.000000
5 2.0 0.000000 3 0.000000
6 3.0 0.000000 3 0.000000
You're viewing a preview
Unlock full access by subscribing today!
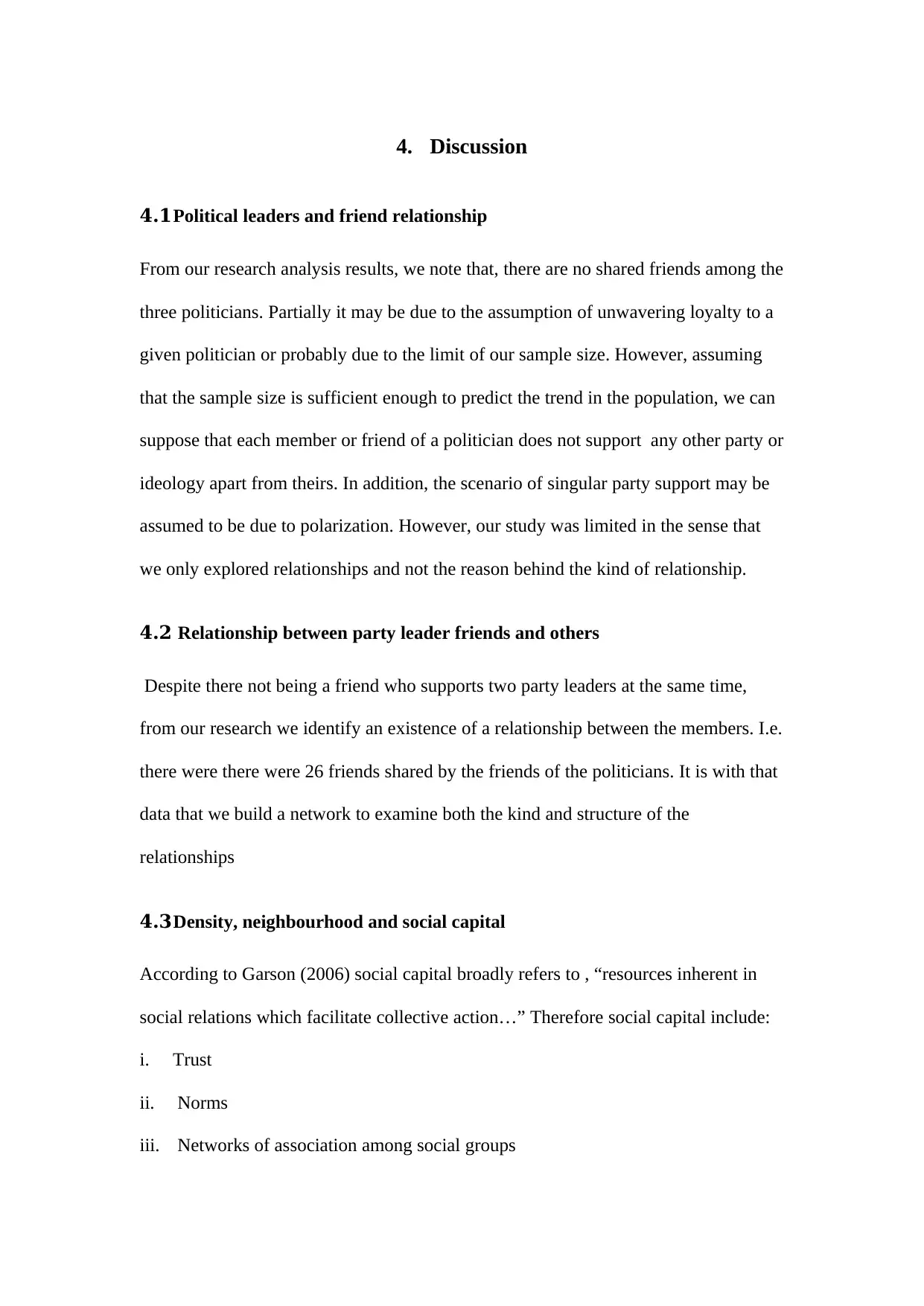
4. Discussion
4.1Political leaders and friend relationship
From our research analysis results, we note that, there are no shared friends among the
three politicians. Partially it may be due to the assumption of unwavering loyalty to a
given politician or probably due to the limit of our sample size. However, assuming
that the sample size is sufficient enough to predict the trend in the population, we can
suppose that each member or friend of a politician does not support any other party or
ideology apart from theirs. In addition, the scenario of singular party support may be
assumed to be due to polarization. However, our study was limited in the sense that
we only explored relationships and not the reason behind the kind of relationship.
4.2 Relationship between party leader friends and others
Despite there not being a friend who supports two party leaders at the same time,
from our research we identify an existence of a relationship between the members. I.e.
there were there were 26 friends shared by the friends of the politicians. It is with that
data that we build a network to examine both the kind and structure of the
relationships
4.3Density, neighbourhood and social capital
According to Garson (2006) social capital broadly refers to , “resources inherent in
social relations which facilitate collective action…” Therefore social capital include:
i. Trust
ii. Norms
iii. Networks of association among social groups
4.1Political leaders and friend relationship
From our research analysis results, we note that, there are no shared friends among the
three politicians. Partially it may be due to the assumption of unwavering loyalty to a
given politician or probably due to the limit of our sample size. However, assuming
that the sample size is sufficient enough to predict the trend in the population, we can
suppose that each member or friend of a politician does not support any other party or
ideology apart from theirs. In addition, the scenario of singular party support may be
assumed to be due to polarization. However, our study was limited in the sense that
we only explored relationships and not the reason behind the kind of relationship.
4.2 Relationship between party leader friends and others
Despite there not being a friend who supports two party leaders at the same time,
from our research we identify an existence of a relationship between the members. I.e.
there were there were 26 friends shared by the friends of the politicians. It is with that
data that we build a network to examine both the kind and structure of the
relationships
4.3Density, neighbourhood and social capital
According to Garson (2006) social capital broadly refers to , “resources inherent in
social relations which facilitate collective action…” Therefore social capital include:
i. Trust
ii. Norms
iii. Networks of association among social groups
Paraphrase This Document
Need a fresh take? Get an instant paraphrase of this document with our AI Paraphraser
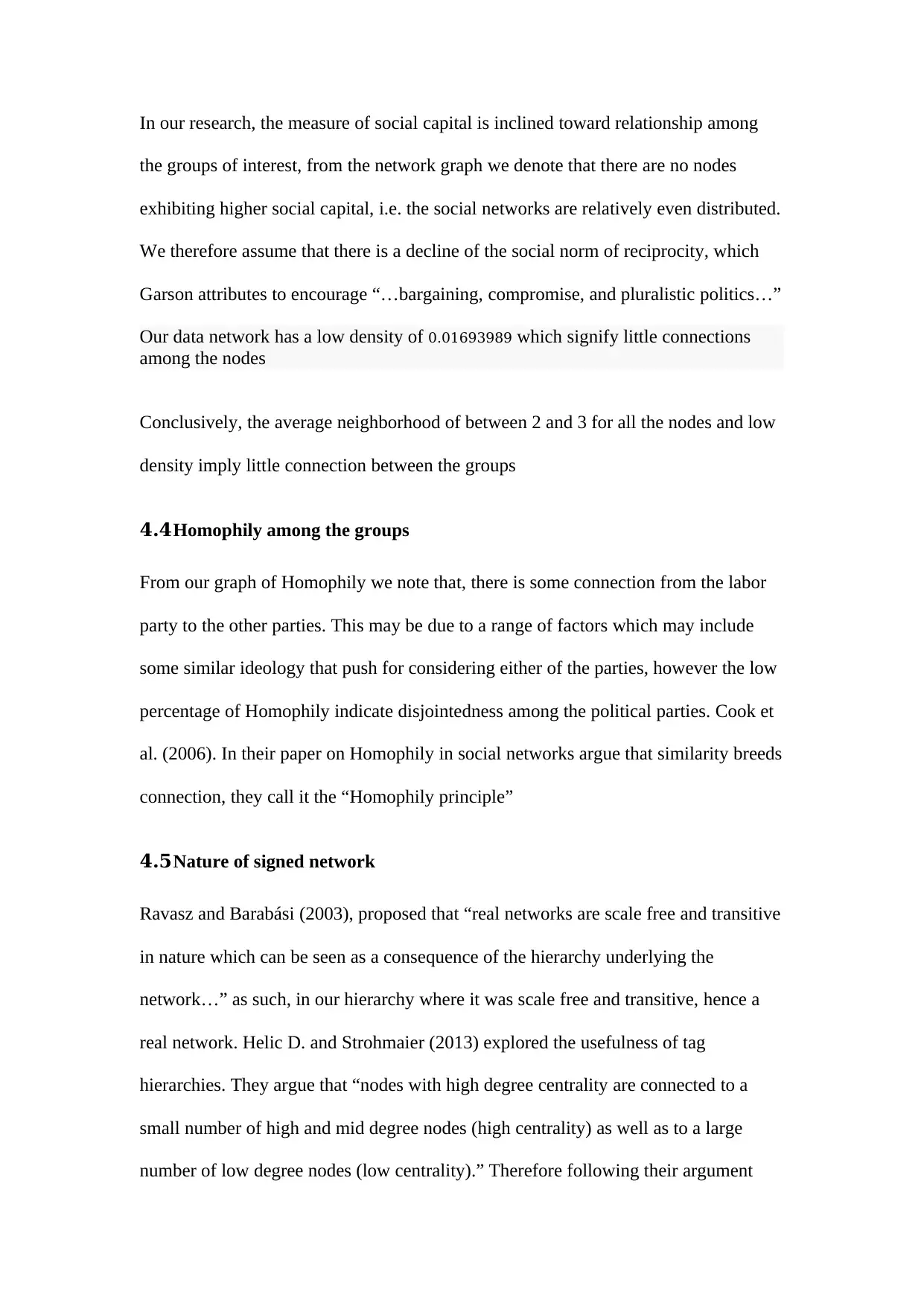
In our research, the measure of social capital is inclined toward relationship among
the groups of interest, from the network graph we denote that there are no nodes
exhibiting higher social capital, i.e. the social networks are relatively even distributed.
We therefore assume that there is a decline of the social norm of reciprocity, which
Garson attributes to encourage “…bargaining, compromise, and pluralistic politics…”
Our data network has a low density of 0.01693989 which signify little connections
among the nodes
Conclusively, the average neighborhood of between 2 and 3 for all the nodes and low
density imply little connection between the groups
4.4Homophily among the groups
From our graph of Homophily we note that, there is some connection from the labor
party to the other parties. This may be due to a range of factors which may include
some similar ideology that push for considering either of the parties, however the low
percentage of Homophily indicate disjointedness among the political parties. Cook et
al. (2006). In their paper on Homophily in social networks argue that similarity breeds
connection, they call it the “Homophily principle”
4.5Nature of signed network
Ravasz and Barabási (2003), proposed that “real networks are scale free and transitive
in nature which can be seen as a consequence of the hierarchy underlying the
network…” as such, in our hierarchy where it was scale free and transitive, hence a
real network. Helic D. and Strohmaier (2013) explored the usefulness of tag
hierarchies. They argue that “nodes with high degree centrality are connected to a
small number of high and mid degree nodes (high centrality) as well as to a large
number of low degree nodes (low centrality).” Therefore following their argument
the groups of interest, from the network graph we denote that there are no nodes
exhibiting higher social capital, i.e. the social networks are relatively even distributed.
We therefore assume that there is a decline of the social norm of reciprocity, which
Garson attributes to encourage “…bargaining, compromise, and pluralistic politics…”
Our data network has a low density of 0.01693989 which signify little connections
among the nodes
Conclusively, the average neighborhood of between 2 and 3 for all the nodes and low
density imply little connection between the groups
4.4Homophily among the groups
From our graph of Homophily we note that, there is some connection from the labor
party to the other parties. This may be due to a range of factors which may include
some similar ideology that push for considering either of the parties, however the low
percentage of Homophily indicate disjointedness among the political parties. Cook et
al. (2006). In their paper on Homophily in social networks argue that similarity breeds
connection, they call it the “Homophily principle”
4.5Nature of signed network
Ravasz and Barabási (2003), proposed that “real networks are scale free and transitive
in nature which can be seen as a consequence of the hierarchy underlying the
network…” as such, in our hierarchy where it was scale free and transitive, hence a
real network. Helic D. and Strohmaier (2013) explored the usefulness of tag
hierarchies. They argue that “nodes with high degree centrality are connected to a
small number of high and mid degree nodes (high centrality) as well as to a large
number of low degree nodes (low centrality).” Therefore following their argument
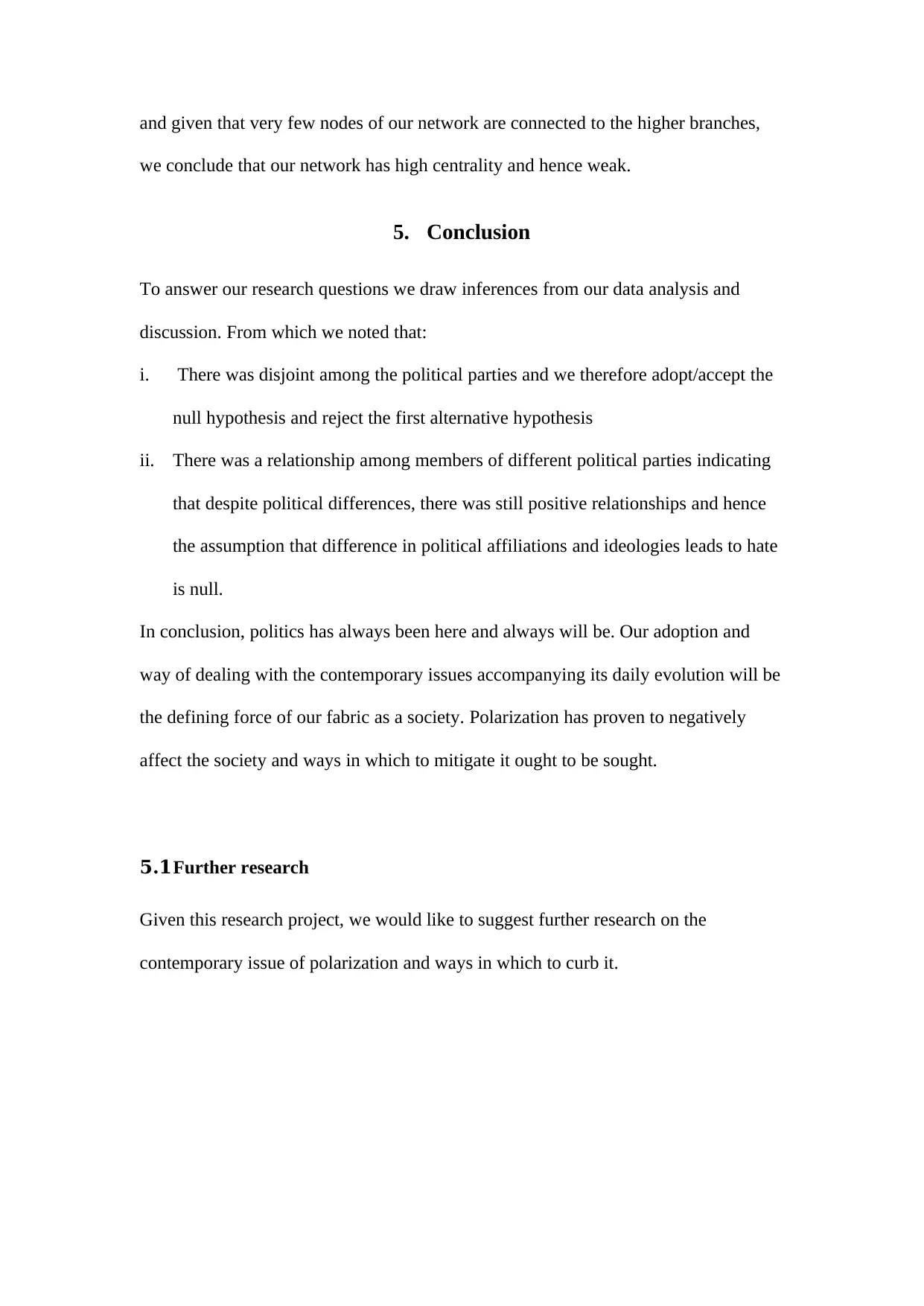
and given that very few nodes of our network are connected to the higher branches,
we conclude that our network has high centrality and hence weak.
5. Conclusion
To answer our research questions we draw inferences from our data analysis and
discussion. From which we noted that:
i. There was disjoint among the political parties and we therefore adopt/accept the
null hypothesis and reject the first alternative hypothesis
ii. There was a relationship among members of different political parties indicating
that despite political differences, there was still positive relationships and hence
the assumption that difference in political affiliations and ideologies leads to hate
is null.
In conclusion, politics has always been here and always will be. Our adoption and
way of dealing with the contemporary issues accompanying its daily evolution will be
the defining force of our fabric as a society. Polarization has proven to negatively
affect the society and ways in which to mitigate it ought to be sought.
5.1Further research
Given this research project, we would like to suggest further research on the
contemporary issue of polarization and ways in which to curb it.
we conclude that our network has high centrality and hence weak.
5. Conclusion
To answer our research questions we draw inferences from our data analysis and
discussion. From which we noted that:
i. There was disjoint among the political parties and we therefore adopt/accept the
null hypothesis and reject the first alternative hypothesis
ii. There was a relationship among members of different political parties indicating
that despite political differences, there was still positive relationships and hence
the assumption that difference in political affiliations and ideologies leads to hate
is null.
In conclusion, politics has always been here and always will be. Our adoption and
way of dealing with the contemporary issues accompanying its daily evolution will be
the defining force of our fabric as a society. Polarization has proven to negatively
affect the society and ways in which to mitigate it ought to be sought.
5.1Further research
Given this research project, we would like to suggest further research on the
contemporary issue of polarization and ways in which to curb it.
You're viewing a preview
Unlock full access by subscribing today!
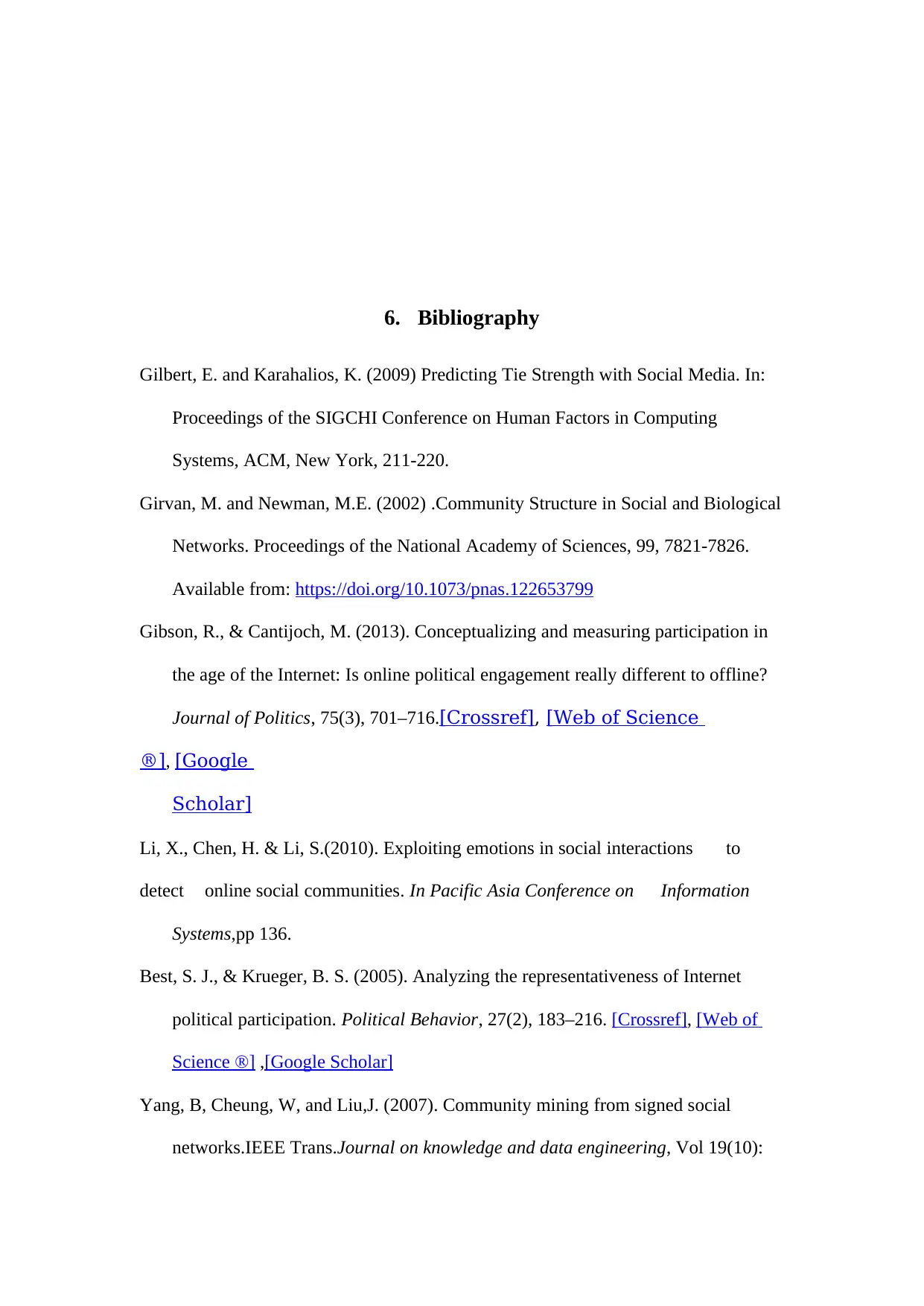
6. Bibliography
Gilbert, E. and Karahalios, K. (2009) Predicting Tie Strength with Social Media. In:
Proceedings of the SIGCHI Conference on Human Factors in Computing
Systems, ACM, New York, 211-220.
Girvan, M. and Newman, M.E. (2002) .Community Structure in Social and Biological
Networks. Proceedings of the National Academy of Sciences, 99, 7821-7826.
Available from: https://doi.org/10.1073/pnas.122653799
Gibson, R., & Cantijoch, M. (2013). Conceptualizing and measuring participation in
the age of the Internet: Is online political engagement really different to offline?
Journal of Politics, 75(3), 701–716.[Crossref], [Web of Science
®], [Google
Scholar]
Li, X., Chen, H. & Li, S.(2010). Exploiting emotions in social interactions to
detect online social communities. In Pacific Asia Conference on Information
Systems,pp 136.
Best, S. J., & Krueger, B. S. (2005). Analyzing the representativeness of Internet
political participation. Political Behavior, 27(2), 183–216. [Crossref], [Web of
Science ®] ,[Google Scholar]
Yang, B, Cheung, W, and Liu,J. (2007). Community mining from signed social
networks.IEEE Trans.Journal on knowledge and data engineering, Vol 19(10):
Gilbert, E. and Karahalios, K. (2009) Predicting Tie Strength with Social Media. In:
Proceedings of the SIGCHI Conference on Human Factors in Computing
Systems, ACM, New York, 211-220.
Girvan, M. and Newman, M.E. (2002) .Community Structure in Social and Biological
Networks. Proceedings of the National Academy of Sciences, 99, 7821-7826.
Available from: https://doi.org/10.1073/pnas.122653799
Gibson, R., & Cantijoch, M. (2013). Conceptualizing and measuring participation in
the age of the Internet: Is online political engagement really different to offline?
Journal of Politics, 75(3), 701–716.[Crossref], [Web of Science
Scholar]
Li, X., Chen, H. & Li, S.(2010). Exploiting emotions in social interactions to
detect online social communities. In Pacific Asia Conference on Information
Systems,pp 136.
Best, S. J., & Krueger, B. S. (2005). Analyzing the representativeness of Internet
political participation. Political Behavior, 27(2), 183–216. [Crossref], [Web of
Science ®] ,[Google Scholar]
Yang, B, Cheung, W, and Liu,J. (2007). Community mining from signed social
networks.IEEE Trans.Journal on knowledge and data engineering, Vol 19(10):
Paraphrase This Document
Need a fresh take? Get an instant paraphrase of this document with our AI Paraphraser
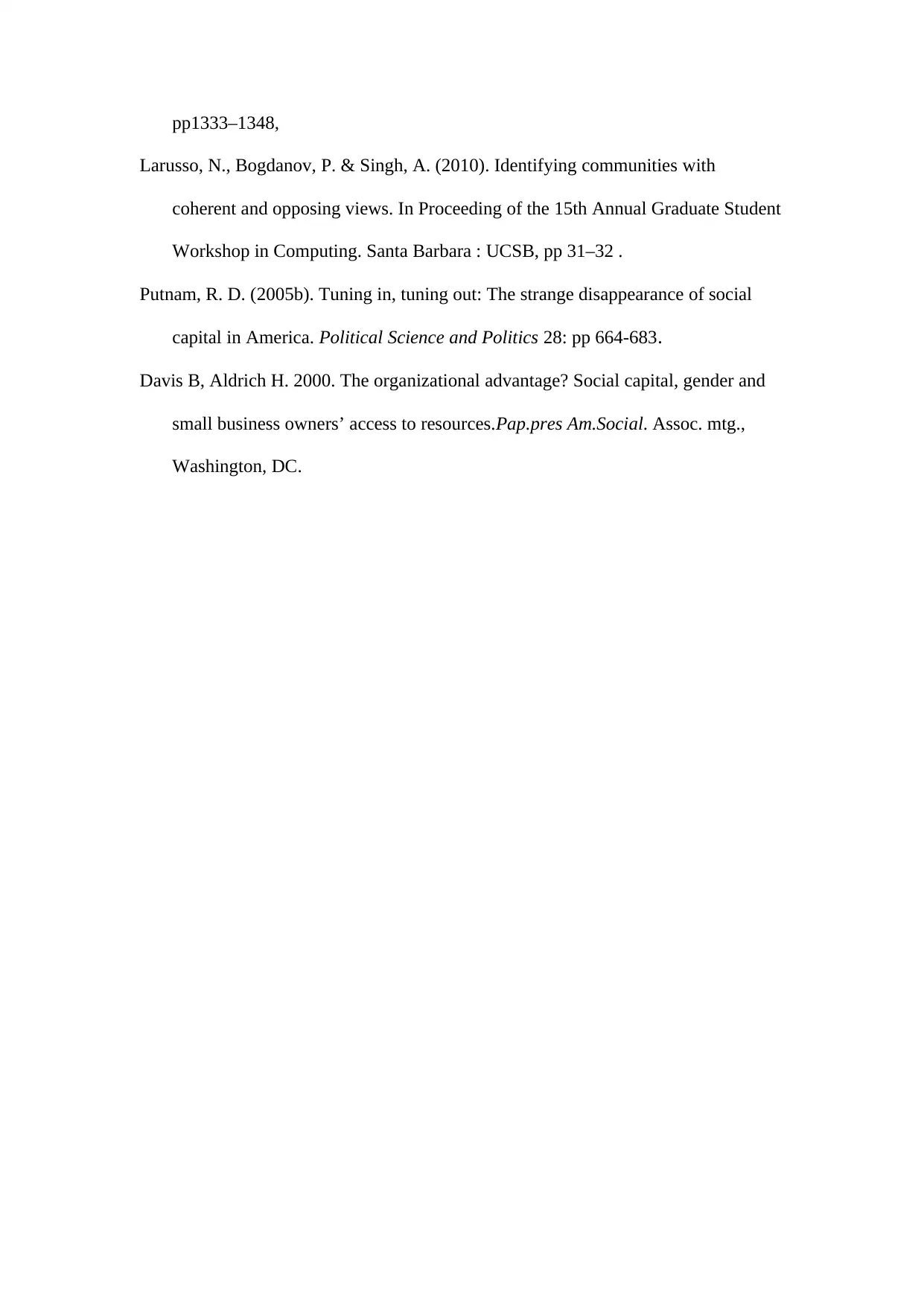
pp1333–1348,
Larusso, N., Bogdanov, P. & Singh, A. (2010). Identifying communities with
coherent and opposing views. In Proceeding of the 15th Annual Graduate Student
Workshop in Computing. Santa Barbara : UCSB, pp 31–32 .
Putnam, R. D. (2005b). Tuning in, tuning out: The strange disappearance of social
capital in America. Political Science and Politics 28: pp 664-683.
Davis B, Aldrich H. 2000. The organizational advantage? Social capital, gender and
small business owners’ access to resources.Pap.pres Am.Social. Assoc. mtg.,
Washington, DC.
Larusso, N., Bogdanov, P. & Singh, A. (2010). Identifying communities with
coherent and opposing views. In Proceeding of the 15th Annual Graduate Student
Workshop in Computing. Santa Barbara : UCSB, pp 31–32 .
Putnam, R. D. (2005b). Tuning in, tuning out: The strange disappearance of social
capital in America. Political Science and Politics 28: pp 664-683.
Davis B, Aldrich H. 2000. The organizational advantage? Social capital, gender and
small business owners’ access to resources.Pap.pres Am.Social. Assoc. mtg.,
Washington, DC.
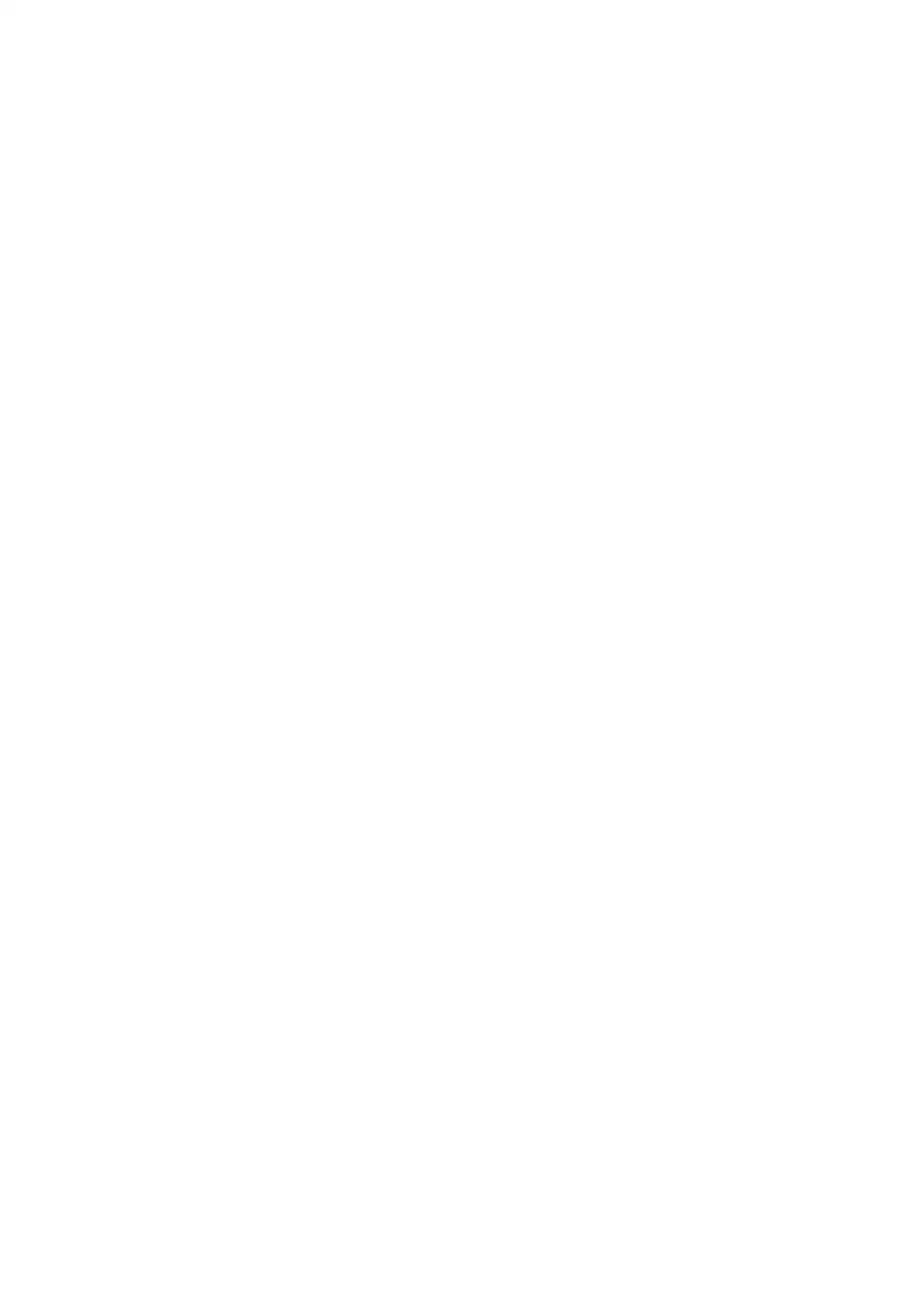
You're viewing a preview
Unlock full access by subscribing today!
1 out of 24
Related Documents

Your All-in-One AI-Powered Toolkit for Academic Success.
+13062052269
info@desklib.com
Available 24*7 on WhatsApp / Email
Unlock your academic potential
© 2024 | Zucol Services PVT LTD | All rights reserved.