Assessment of FluffyGroCo's Briefing Note on Data Science Concepts
VerifiedAdded on 2022/11/10
|14
|4970
|202
AI Summary
This report assesses FluffyGroCo's briefing note on data science concepts and identifies limitations in the application of statistical methods and algorithms. The report provides an overview of the investigation, analysis, and results, and discusses ethical and security considerations in data science. The dataset used is quantitative in nature and can be analyzed using both descriptive and inferential statistics. The report recommends the adoption of a participatory approach to address ethical issues in data science.
Contribute Materials
Your contribution can guide someone’s learning journey. Share your
documents today.
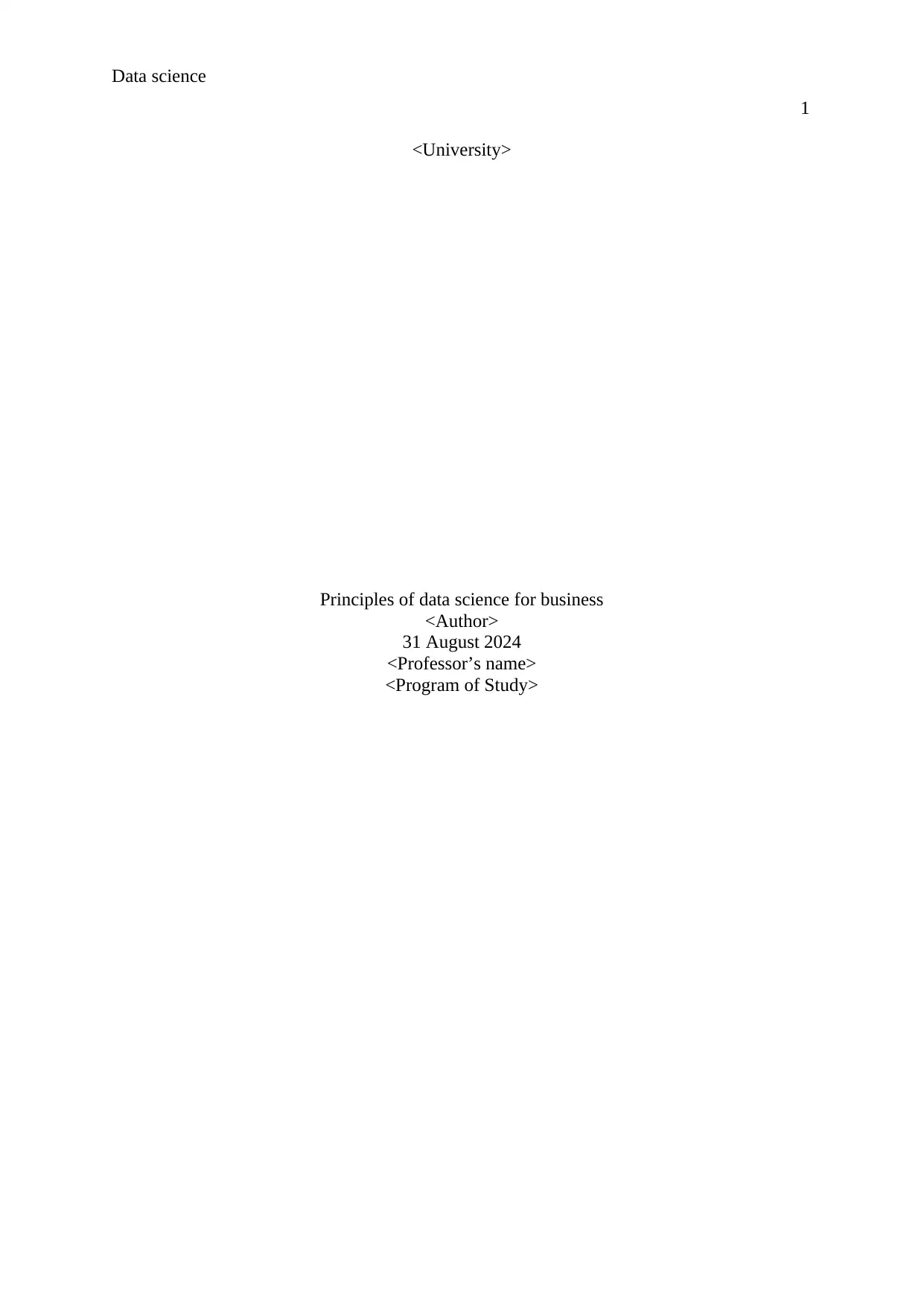
Data science
1
<University>
Principles of data science for business
<Author>
31 August 2024
<Professor’s name>
<Program of Study>
1
<University>
Principles of data science for business
<Author>
31 August 2024
<Professor’s name>
<Program of Study>
Secure Best Marks with AI Grader
Need help grading? Try our AI Grader for instant feedback on your assignments.
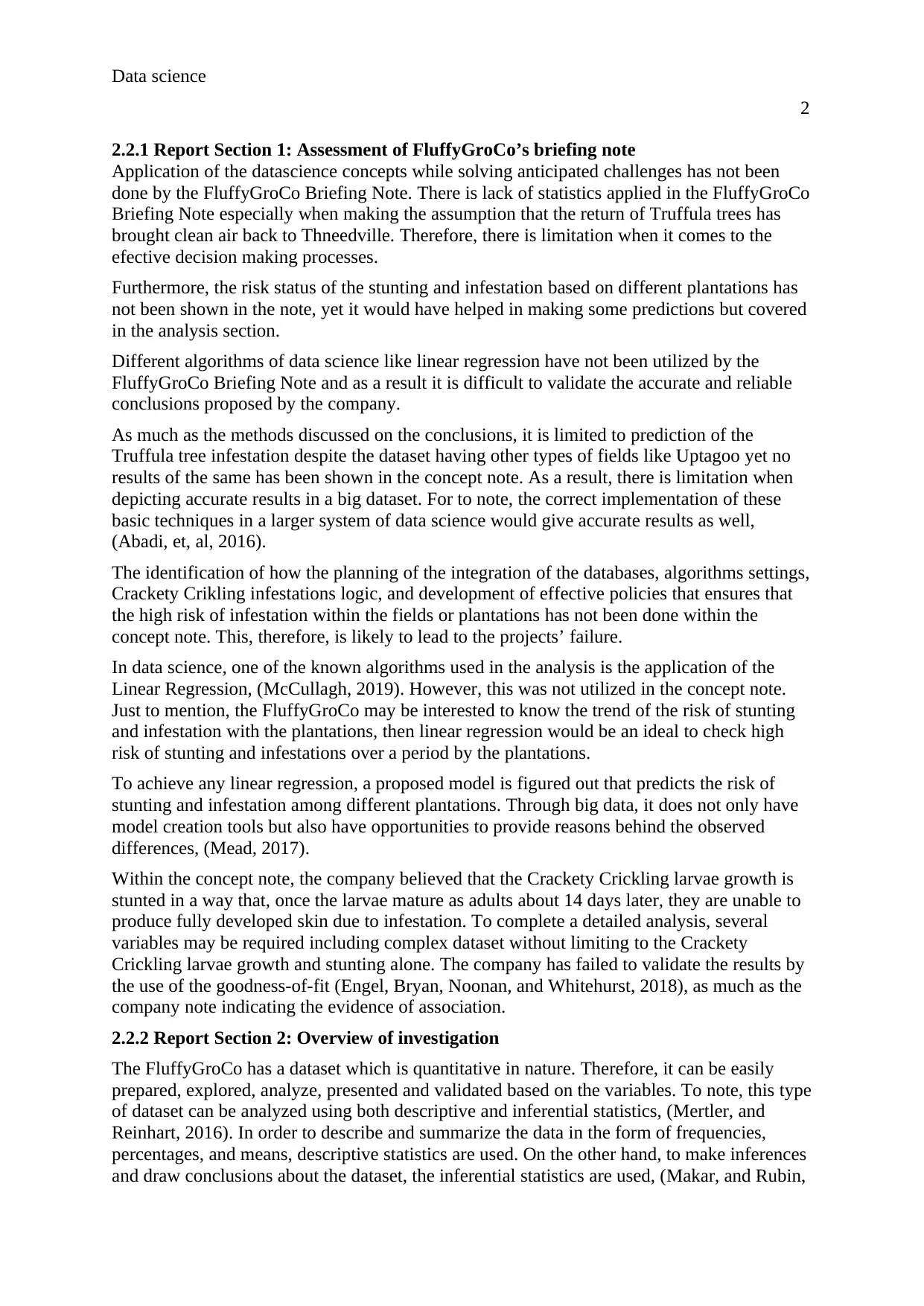
Data science
2
2.2.1 Report Section 1: Assessment of FluffyGroCo’s briefing note
Application of the datascience concepts while solving anticipated challenges has not been
done by the FluffyGroCo Briefing Note. There is lack of statistics applied in the FluffyGroCo
Briefing Note especially when making the assumption that the return of Truffula trees has
brought clean air back to Thneedville. Therefore, there is limitation when it comes to the
efective decision making processes.
Furthermore, the risk status of the stunting and infestation based on different plantations has
not been shown in the note, yet it would have helped in making some predictions but covered
in the analysis section.
Different algorithms of data science like linear regression have not been utilized by the
FluffyGroCo Briefing Note and as a result it is difficult to validate the accurate and reliable
conclusions proposed by the company.
As much as the methods discussed on the conclusions, it is limited to prediction of the
Truffula tree infestation despite the dataset having other types of fields like Uptagoo yet no
results of the same has been shown in the concept note. As a result, there is limitation when
depicting accurate results in a big dataset. For to note, the correct implementation of these
basic techniques in a larger system of data science would give accurate results as well,
(Abadi, et, al, 2016).
The identification of how the planning of the integration of the databases, algorithms settings,
Crackety Crikling infestations logic, and development of effective policies that ensures that
the high risk of infestation within the fields or plantations has not been done within the
concept note. This, therefore, is likely to lead to the projects’ failure.
In data science, one of the known algorithms used in the analysis is the application of the
Linear Regression, (McCullagh, 2019). However, this was not utilized in the concept note.
Just to mention, the FluffyGroCo may be interested to know the trend of the risk of stunting
and infestation with the plantations, then linear regression would be an ideal to check high
risk of stunting and infestations over a period by the plantations.
To achieve any linear regression, a proposed model is figured out that predicts the risk of
stunting and infestation among different plantations. Through big data, it does not only have
model creation tools but also have opportunities to provide reasons behind the observed
differences, (Mead, 2017).
Within the concept note, the company believed that the Crackety Crickling larvae growth is
stunted in a way that, once the larvae mature as adults about 14 days later, they are unable to
produce fully developed skin due to infestation. To complete a detailed analysis, several
variables may be required including complex dataset without limiting to the Crackety
Crickling larvae growth and stunting alone. The company has failed to validate the results by
the use of the goodness-of-fit (Engel, Bryan, Noonan, and Whitehurst, 2018), as much as the
company note indicating the evidence of association.
2.2.2 Report Section 2: Overview of investigation
The FluffyGroCo has a dataset which is quantitative in nature. Therefore, it can be easily
prepared, explored, analyze, presented and validated based on the variables. To note, this type
of dataset can be analyzed using both descriptive and inferential statistics, (Mertler, and
Reinhart, 2016). In order to describe and summarize the data in the form of frequencies,
percentages, and means, descriptive statistics are used. On the other hand, to make inferences
and draw conclusions about the dataset, the inferential statistics are used, (Makar, and Rubin,
2
2.2.1 Report Section 1: Assessment of FluffyGroCo’s briefing note
Application of the datascience concepts while solving anticipated challenges has not been
done by the FluffyGroCo Briefing Note. There is lack of statistics applied in the FluffyGroCo
Briefing Note especially when making the assumption that the return of Truffula trees has
brought clean air back to Thneedville. Therefore, there is limitation when it comes to the
efective decision making processes.
Furthermore, the risk status of the stunting and infestation based on different plantations has
not been shown in the note, yet it would have helped in making some predictions but covered
in the analysis section.
Different algorithms of data science like linear regression have not been utilized by the
FluffyGroCo Briefing Note and as a result it is difficult to validate the accurate and reliable
conclusions proposed by the company.
As much as the methods discussed on the conclusions, it is limited to prediction of the
Truffula tree infestation despite the dataset having other types of fields like Uptagoo yet no
results of the same has been shown in the concept note. As a result, there is limitation when
depicting accurate results in a big dataset. For to note, the correct implementation of these
basic techniques in a larger system of data science would give accurate results as well,
(Abadi, et, al, 2016).
The identification of how the planning of the integration of the databases, algorithms settings,
Crackety Crikling infestations logic, and development of effective policies that ensures that
the high risk of infestation within the fields or plantations has not been done within the
concept note. This, therefore, is likely to lead to the projects’ failure.
In data science, one of the known algorithms used in the analysis is the application of the
Linear Regression, (McCullagh, 2019). However, this was not utilized in the concept note.
Just to mention, the FluffyGroCo may be interested to know the trend of the risk of stunting
and infestation with the plantations, then linear regression would be an ideal to check high
risk of stunting and infestations over a period by the plantations.
To achieve any linear regression, a proposed model is figured out that predicts the risk of
stunting and infestation among different plantations. Through big data, it does not only have
model creation tools but also have opportunities to provide reasons behind the observed
differences, (Mead, 2017).
Within the concept note, the company believed that the Crackety Crickling larvae growth is
stunted in a way that, once the larvae mature as adults about 14 days later, they are unable to
produce fully developed skin due to infestation. To complete a detailed analysis, several
variables may be required including complex dataset without limiting to the Crackety
Crickling larvae growth and stunting alone. The company has failed to validate the results by
the use of the goodness-of-fit (Engel, Bryan, Noonan, and Whitehurst, 2018), as much as the
company note indicating the evidence of association.
2.2.2 Report Section 2: Overview of investigation
The FluffyGroCo has a dataset which is quantitative in nature. Therefore, it can be easily
prepared, explored, analyze, presented and validated based on the variables. To note, this type
of dataset can be analyzed using both descriptive and inferential statistics, (Mertler, and
Reinhart, 2016). In order to describe and summarize the data in the form of frequencies,
percentages, and means, descriptive statistics are used. On the other hand, to make inferences
and draw conclusions about the dataset, the inferential statistics are used, (Makar, and Rubin,
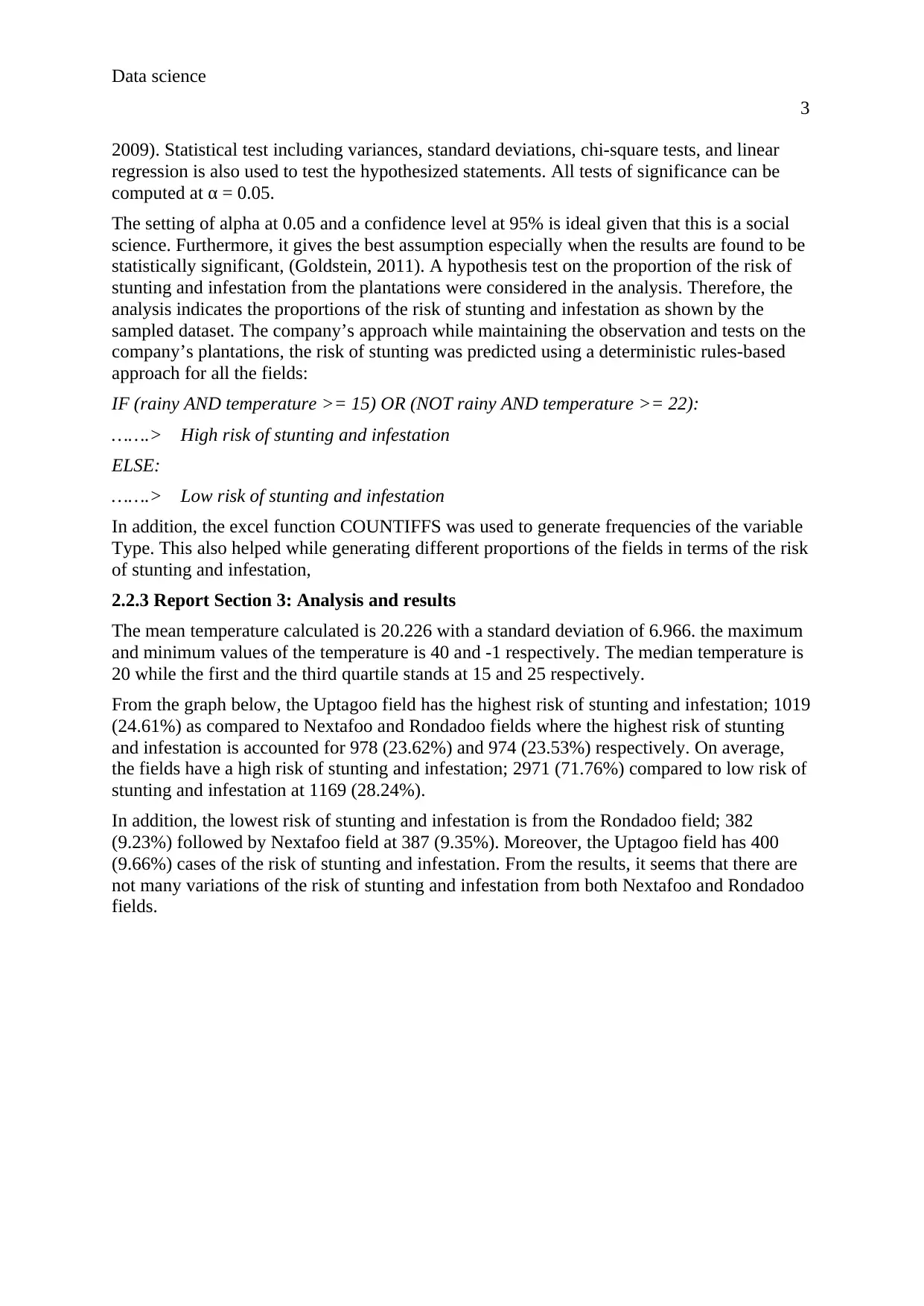
Data science
3
2009). Statistical test including variances, standard deviations, chi-square tests, and linear
regression is also used to test the hypothesized statements. All tests of significance can be
computed at α = 0.05.
The setting of alpha at 0.05 and a confidence level at 95% is ideal given that this is a social
science. Furthermore, it gives the best assumption especially when the results are found to be
statistically significant, (Goldstein, 2011). A hypothesis test on the proportion of the risk of
stunting and infestation from the plantations were considered in the analysis. Therefore, the
analysis indicates the proportions of the risk of stunting and infestation as shown by the
sampled dataset. The company’s approach while maintaining the observation and tests on the
company’s plantations, the risk of stunting was predicted using a deterministic rules-based
approach for all the fields:
IF (rainy AND temperature >= 15) OR (NOT rainy AND temperature >= 22):
…….> High risk of stunting and infestation
ELSE:
…….> Low risk of stunting and infestation
In addition, the excel function COUNTIFFS was used to generate frequencies of the variable
Type. This also helped while generating different proportions of the fields in terms of the risk
of stunting and infestation,
2.2.3 Report Section 3: Analysis and results
The mean temperature calculated is 20.226 with a standard deviation of 6.966. the maximum
and minimum values of the temperature is 40 and -1 respectively. The median temperature is
20 while the first and the third quartile stands at 15 and 25 respectively.
From the graph below, the Uptagoo field has the highest risk of stunting and infestation; 1019
(24.61%) as compared to Nextafoo and Rondadoo fields where the highest risk of stunting
and infestation is accounted for 978 (23.62%) and 974 (23.53%) respectively. On average,
the fields have a high risk of stunting and infestation; 2971 (71.76%) compared to low risk of
stunting and infestation at 1169 (28.24%).
In addition, the lowest risk of stunting and infestation is from the Rondadoo field; 382
(9.23%) followed by Nextafoo field at 387 (9.35%). Moreover, the Uptagoo field has 400
(9.66%) cases of the risk of stunting and infestation. From the results, it seems that there are
not many variations of the risk of stunting and infestation from both Nextafoo and Rondadoo
fields.
3
2009). Statistical test including variances, standard deviations, chi-square tests, and linear
regression is also used to test the hypothesized statements. All tests of significance can be
computed at α = 0.05.
The setting of alpha at 0.05 and a confidence level at 95% is ideal given that this is a social
science. Furthermore, it gives the best assumption especially when the results are found to be
statistically significant, (Goldstein, 2011). A hypothesis test on the proportion of the risk of
stunting and infestation from the plantations were considered in the analysis. Therefore, the
analysis indicates the proportions of the risk of stunting and infestation as shown by the
sampled dataset. The company’s approach while maintaining the observation and tests on the
company’s plantations, the risk of stunting was predicted using a deterministic rules-based
approach for all the fields:
IF (rainy AND temperature >= 15) OR (NOT rainy AND temperature >= 22):
…….> High risk of stunting and infestation
ELSE:
…….> Low risk of stunting and infestation
In addition, the excel function COUNTIFFS was used to generate frequencies of the variable
Type. This also helped while generating different proportions of the fields in terms of the risk
of stunting and infestation,
2.2.3 Report Section 3: Analysis and results
The mean temperature calculated is 20.226 with a standard deviation of 6.966. the maximum
and minimum values of the temperature is 40 and -1 respectively. The median temperature is
20 while the first and the third quartile stands at 15 and 25 respectively.
From the graph below, the Uptagoo field has the highest risk of stunting and infestation; 1019
(24.61%) as compared to Nextafoo and Rondadoo fields where the highest risk of stunting
and infestation is accounted for 978 (23.62%) and 974 (23.53%) respectively. On average,
the fields have a high risk of stunting and infestation; 2971 (71.76%) compared to low risk of
stunting and infestation at 1169 (28.24%).
In addition, the lowest risk of stunting and infestation is from the Rondadoo field; 382
(9.23%) followed by Nextafoo field at 387 (9.35%). Moreover, the Uptagoo field has 400
(9.66%) cases of the risk of stunting and infestation. From the results, it seems that there are
not many variations of the risk of stunting and infestation from both Nextafoo and Rondadoo
fields.
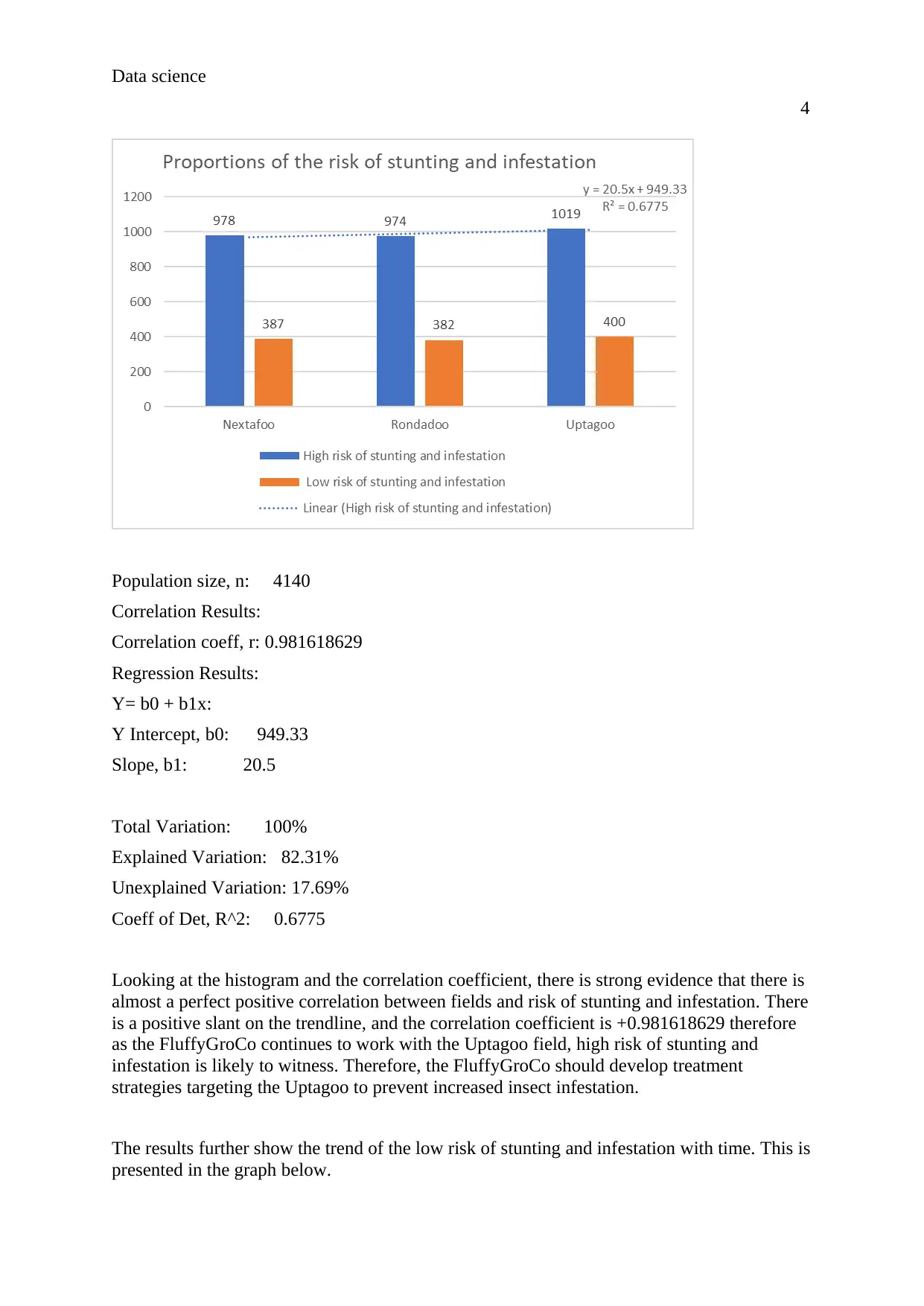
Data science
4
Population size, n: 4140
Correlation Results:
Correlation coeff, r: 0.981618629
Regression Results:
Y= b0 + b1x:
Y Intercept, b0: 949.33
Slope, b1: 20.5
Total Variation: 100%
Explained Variation: 82.31%
Unexplained Variation: 17.69%
Coeff of Det, R^2: 0.6775
Looking at the histogram and the correlation coefficient, there is strong evidence that there is
almost a perfect positive correlation between fields and risk of stunting and infestation. There
is a positive slant on the trendline, and the correlation coefficient is +0.981618629 therefore
as the FluffyGroCo continues to work with the Uptagoo field, high risk of stunting and
infestation is likely to witness. Therefore, the FluffyGroCo should develop treatment
strategies targeting the Uptagoo to prevent increased insect infestation.
The results further show the trend of the low risk of stunting and infestation with time. This is
presented in the graph below.
4
Population size, n: 4140
Correlation Results:
Correlation coeff, r: 0.981618629
Regression Results:
Y= b0 + b1x:
Y Intercept, b0: 949.33
Slope, b1: 20.5
Total Variation: 100%
Explained Variation: 82.31%
Unexplained Variation: 17.69%
Coeff of Det, R^2: 0.6775
Looking at the histogram and the correlation coefficient, there is strong evidence that there is
almost a perfect positive correlation between fields and risk of stunting and infestation. There
is a positive slant on the trendline, and the correlation coefficient is +0.981618629 therefore
as the FluffyGroCo continues to work with the Uptagoo field, high risk of stunting and
infestation is likely to witness. Therefore, the FluffyGroCo should develop treatment
strategies targeting the Uptagoo to prevent increased insect infestation.
The results further show the trend of the low risk of stunting and infestation with time. This is
presented in the graph below.
Paraphrase This Document
Need a fresh take? Get an instant paraphrase of this document with our AI Paraphraser
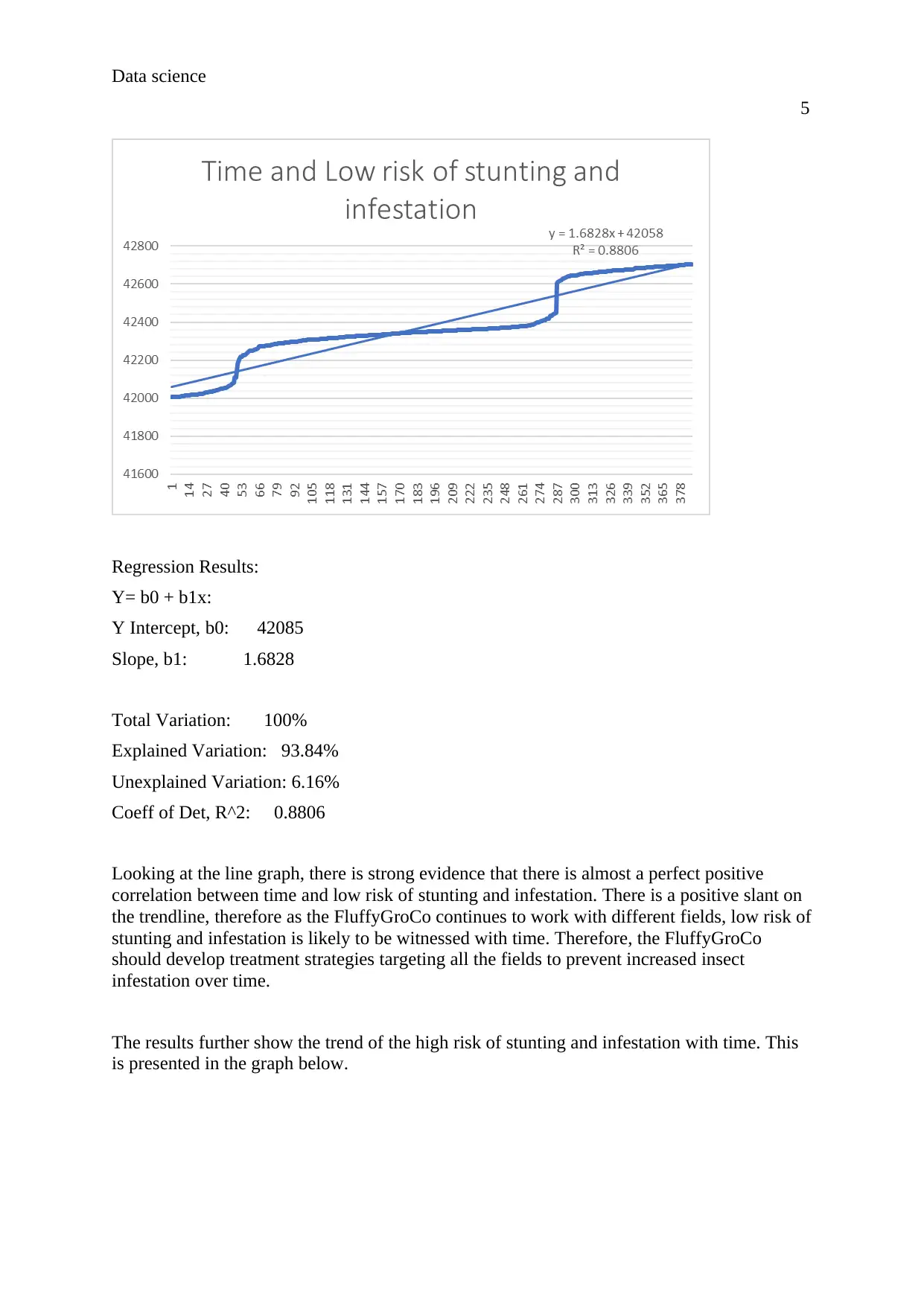
Data science
5
Regression Results:
Y= b0 + b1x:
Y Intercept, b0: 42085
Slope, b1: 1.6828
Total Variation: 100%
Explained Variation: 93.84%
Unexplained Variation: 6.16%
Coeff of Det, R^2: 0.8806
Looking at the line graph, there is strong evidence that there is almost a perfect positive
correlation between time and low risk of stunting and infestation. There is a positive slant on
the trendline, therefore as the FluffyGroCo continues to work with different fields, low risk of
stunting and infestation is likely to be witnessed with time. Therefore, the FluffyGroCo
should develop treatment strategies targeting all the fields to prevent increased insect
infestation over time.
The results further show the trend of the high risk of stunting and infestation with time. This
is presented in the graph below.
5
Regression Results:
Y= b0 + b1x:
Y Intercept, b0: 42085
Slope, b1: 1.6828
Total Variation: 100%
Explained Variation: 93.84%
Unexplained Variation: 6.16%
Coeff of Det, R^2: 0.8806
Looking at the line graph, there is strong evidence that there is almost a perfect positive
correlation between time and low risk of stunting and infestation. There is a positive slant on
the trendline, therefore as the FluffyGroCo continues to work with different fields, low risk of
stunting and infestation is likely to be witnessed with time. Therefore, the FluffyGroCo
should develop treatment strategies targeting all the fields to prevent increased insect
infestation over time.
The results further show the trend of the high risk of stunting and infestation with time. This
is presented in the graph below.
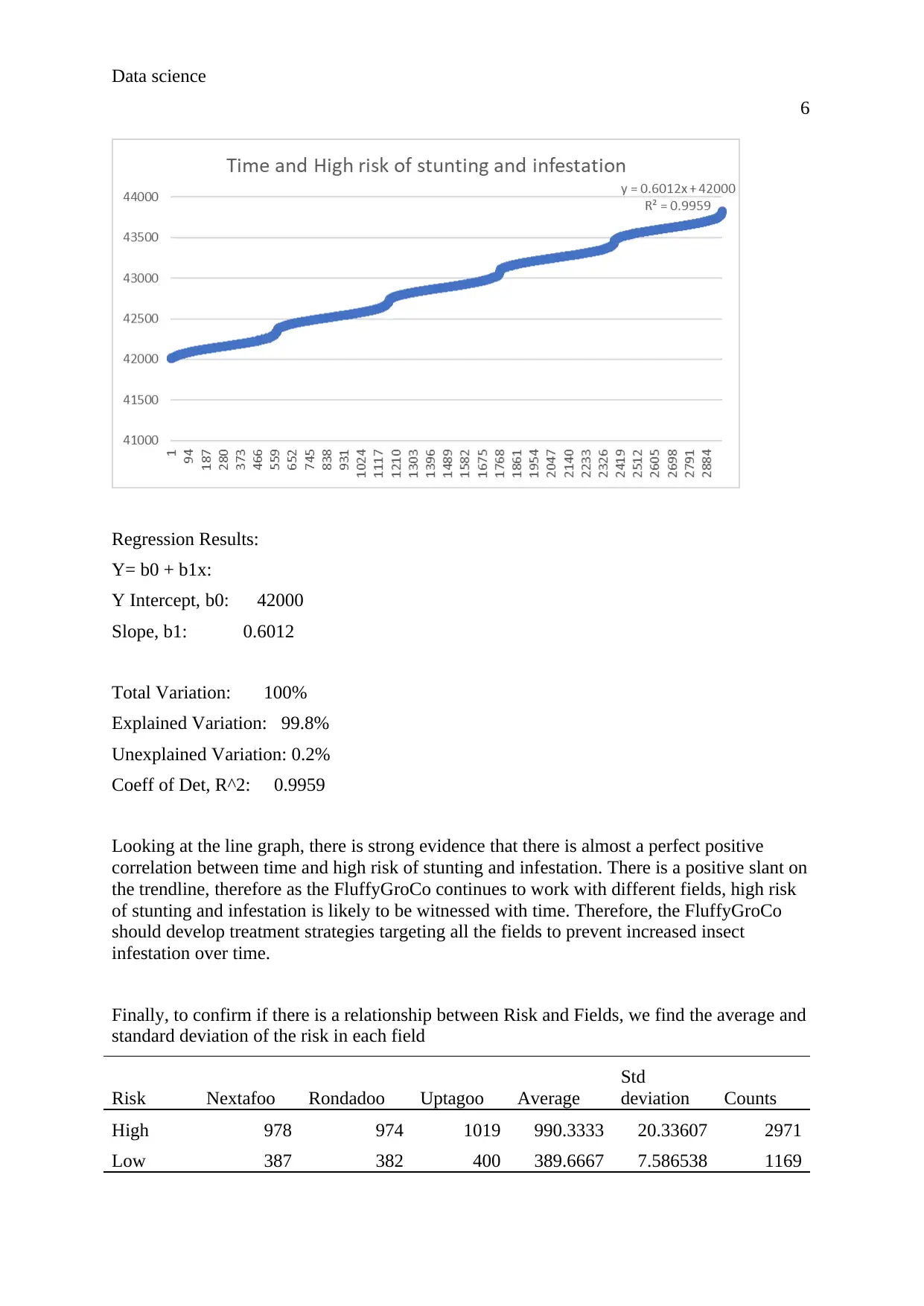
Data science
6
Regression Results:
Y= b0 + b1x:
Y Intercept, b0: 42000
Slope, b1: 0.6012
Total Variation: 100%
Explained Variation: 99.8%
Unexplained Variation: 0.2%
Coeff of Det, R^2: 0.9959
Looking at the line graph, there is strong evidence that there is almost a perfect positive
correlation between time and high risk of stunting and infestation. There is a positive slant on
the trendline, therefore as the FluffyGroCo continues to work with different fields, high risk
of stunting and infestation is likely to be witnessed with time. Therefore, the FluffyGroCo
should develop treatment strategies targeting all the fields to prevent increased insect
infestation over time.
Finally, to confirm if there is a relationship between Risk and Fields, we find the average and
standard deviation of the risk in each field
Risk Nextafoo Rondadoo Uptagoo Average
Std
deviation Counts
High 978 974 1019 990.3333 20.33607 2971
Low 387 382 400 389.6667 7.586538 1169
6
Regression Results:
Y= b0 + b1x:
Y Intercept, b0: 42000
Slope, b1: 0.6012
Total Variation: 100%
Explained Variation: 99.8%
Unexplained Variation: 0.2%
Coeff of Det, R^2: 0.9959
Looking at the line graph, there is strong evidence that there is almost a perfect positive
correlation between time and high risk of stunting and infestation. There is a positive slant on
the trendline, therefore as the FluffyGroCo continues to work with different fields, high risk
of stunting and infestation is likely to be witnessed with time. Therefore, the FluffyGroCo
should develop treatment strategies targeting all the fields to prevent increased insect
infestation over time.
Finally, to confirm if there is a relationship between Risk and Fields, we find the average and
standard deviation of the risk in each field
Risk Nextafoo Rondadoo Uptagoo Average
Std
deviation Counts
High 978 974 1019 990.3333 20.33607 2971
Low 387 382 400 389.6667 7.586538 1169
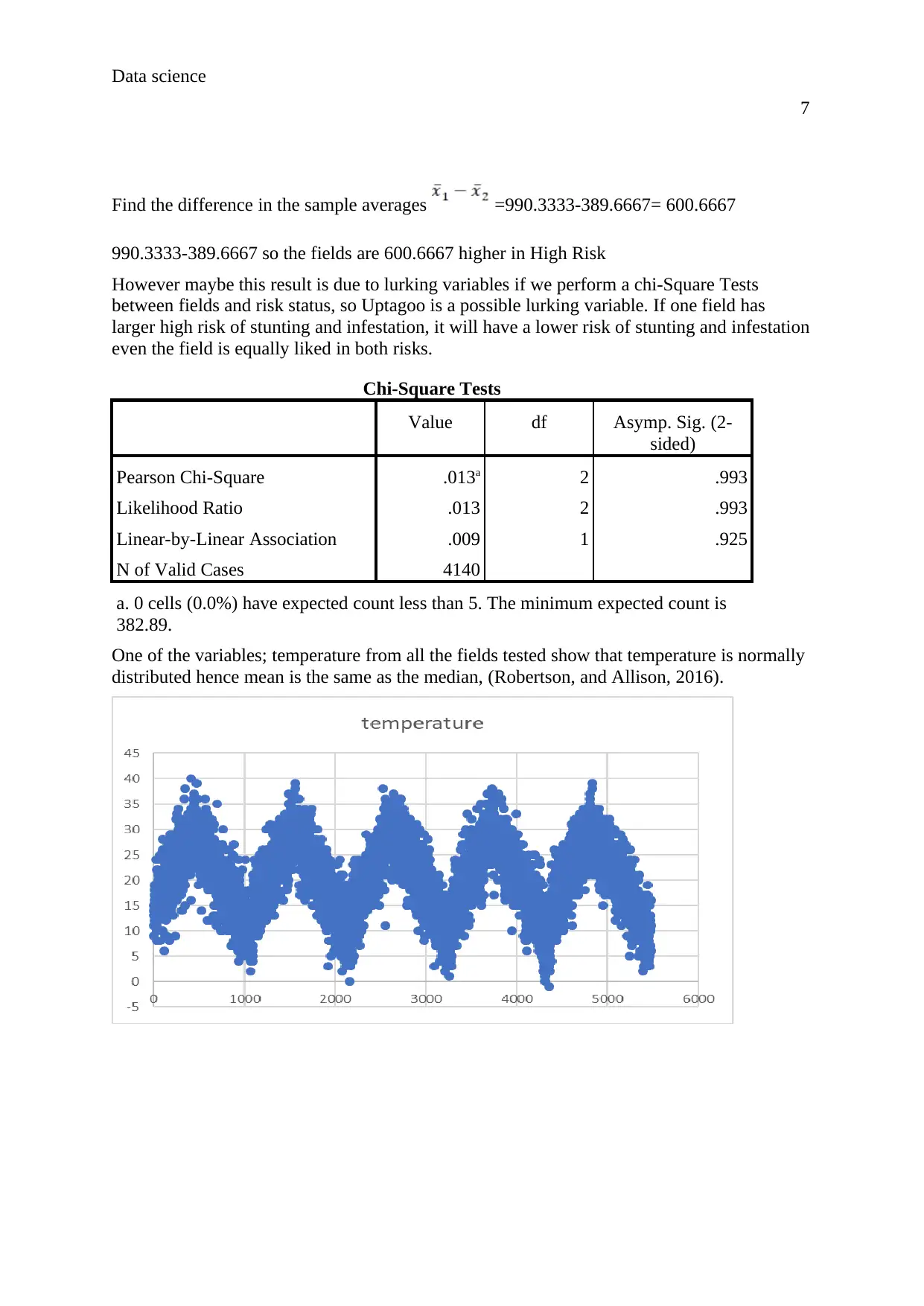
Data science
7
Find the difference in the sample averages =990.3333-389.6667= 600.6667
990.3333-389.6667 so the fields are 600.6667 higher in High Risk
However maybe this result is due to lurking variables if we perform a chi-Square Tests
between fields and risk status, so Uptagoo is a possible lurking variable. If one field has
larger high risk of stunting and infestation, it will have a lower risk of stunting and infestation
even the field is equally liked in both risks.
Chi-Square Tests
Value df Asymp. Sig. (2-
sided)
Pearson Chi-Square .013a 2 .993
Likelihood Ratio .013 2 .993
Linear-by-Linear Association .009 1 .925
N of Valid Cases 4140
a. 0 cells (0.0%) have expected count less than 5. The minimum expected count is
382.89.
One of the variables; temperature from all the fields tested show that temperature is normally
distributed hence mean is the same as the median, (Robertson, and Allison, 2016).
7
Find the difference in the sample averages =990.3333-389.6667= 600.6667
990.3333-389.6667 so the fields are 600.6667 higher in High Risk
However maybe this result is due to lurking variables if we perform a chi-Square Tests
between fields and risk status, so Uptagoo is a possible lurking variable. If one field has
larger high risk of stunting and infestation, it will have a lower risk of stunting and infestation
even the field is equally liked in both risks.
Chi-Square Tests
Value df Asymp. Sig. (2-
sided)
Pearson Chi-Square .013a 2 .993
Likelihood Ratio .013 2 .993
Linear-by-Linear Association .009 1 .925
N of Valid Cases 4140
a. 0 cells (0.0%) have expected count less than 5. The minimum expected count is
382.89.
One of the variables; temperature from all the fields tested show that temperature is normally
distributed hence mean is the same as the median, (Robertson, and Allison, 2016).
Secure Best Marks with AI Grader
Need help grading? Try our AI Grader for instant feedback on your assignments.
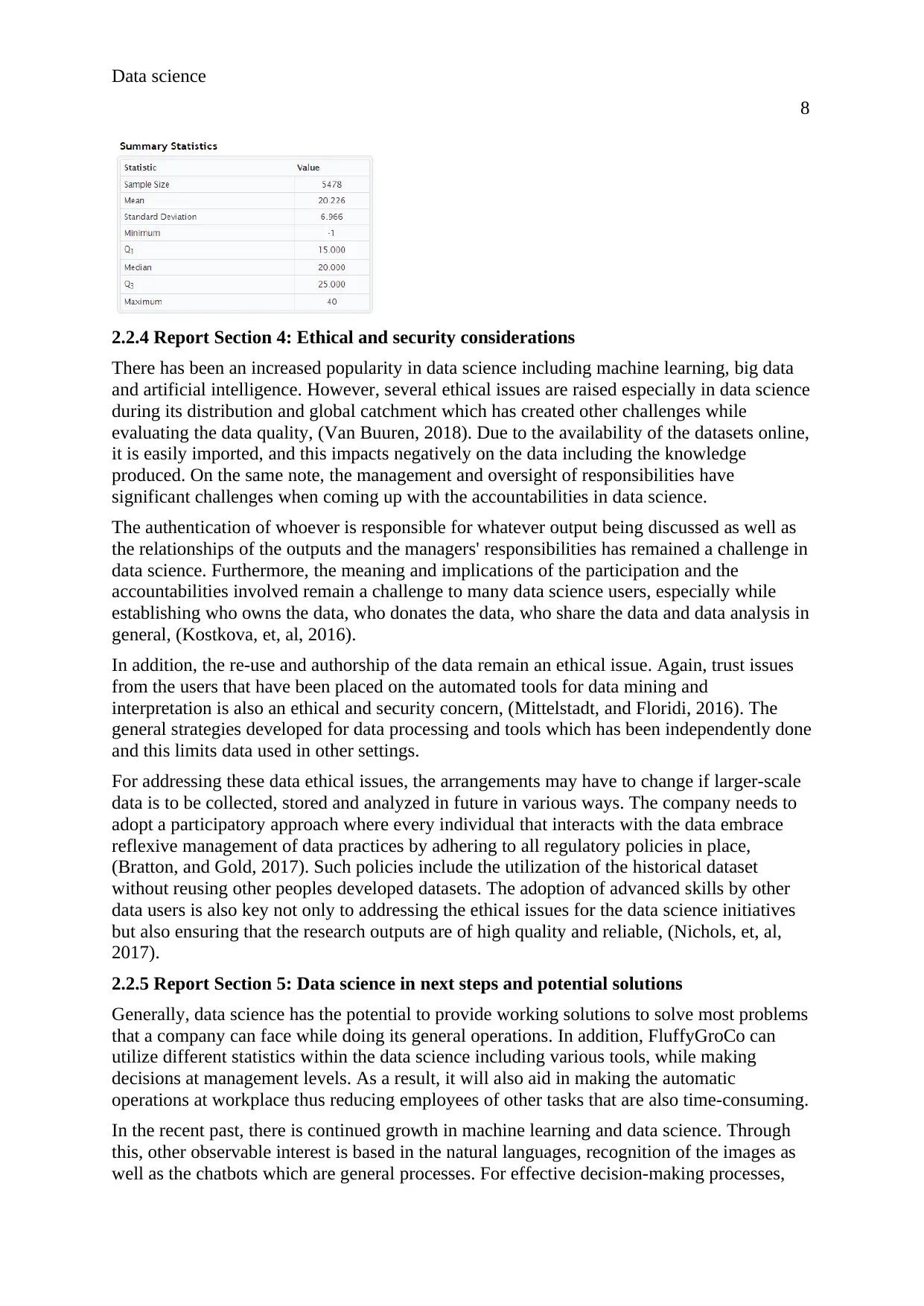
Data science
8
2.2.4 Report Section 4: Ethical and security considerations
There has been an increased popularity in data science including machine learning, big data
and artificial intelligence. However, several ethical issues are raised especially in data science
during its distribution and global catchment which has created other challenges while
evaluating the data quality, (Van Buuren, 2018). Due to the availability of the datasets online,
it is easily imported, and this impacts negatively on the data including the knowledge
produced. On the same note, the management and oversight of responsibilities have
significant challenges when coming up with the accountabilities in data science.
The authentication of whoever is responsible for whatever output being discussed as well as
the relationships of the outputs and the managers' responsibilities has remained a challenge in
data science. Furthermore, the meaning and implications of the participation and the
accountabilities involved remain a challenge to many data science users, especially while
establishing who owns the data, who donates the data, who share the data and data analysis in
general, (Kostkova, et, al, 2016).
In addition, the re-use and authorship of the data remain an ethical issue. Again, trust issues
from the users that have been placed on the automated tools for data mining and
interpretation is also an ethical and security concern, (Mittelstadt, and Floridi, 2016). The
general strategies developed for data processing and tools which has been independently done
and this limits data used in other settings.
For addressing these data ethical issues, the arrangements may have to change if larger-scale
data is to be collected, stored and analyzed in future in various ways. The company needs to
adopt a participatory approach where every individual that interacts with the data embrace
reflexive management of data practices by adhering to all regulatory policies in place,
(Bratton, and Gold, 2017). Such policies include the utilization of the historical dataset
without reusing other peoples developed datasets. The adoption of advanced skills by other
data users is also key not only to addressing the ethical issues for the data science initiatives
but also ensuring that the research outputs are of high quality and reliable, (Nichols, et, al,
2017).
2.2.5 Report Section 5: Data science in next steps and potential solutions
Generally, data science has the potential to provide working solutions to solve most problems
that a company can face while doing its general operations. In addition, FluffyGroCo can
utilize different statistics within the data science including various tools, while making
decisions at management levels. As a result, it will also aid in making the automatic
operations at workplace thus reducing employees of other tasks that are also time-consuming.
In the recent past, there is continued growth in machine learning and data science. Through
this, other observable interest is based in the natural languages, recognition of the images as
well as the chatbots which are general processes. For effective decision-making processes,
8
2.2.4 Report Section 4: Ethical and security considerations
There has been an increased popularity in data science including machine learning, big data
and artificial intelligence. However, several ethical issues are raised especially in data science
during its distribution and global catchment which has created other challenges while
evaluating the data quality, (Van Buuren, 2018). Due to the availability of the datasets online,
it is easily imported, and this impacts negatively on the data including the knowledge
produced. On the same note, the management and oversight of responsibilities have
significant challenges when coming up with the accountabilities in data science.
The authentication of whoever is responsible for whatever output being discussed as well as
the relationships of the outputs and the managers' responsibilities has remained a challenge in
data science. Furthermore, the meaning and implications of the participation and the
accountabilities involved remain a challenge to many data science users, especially while
establishing who owns the data, who donates the data, who share the data and data analysis in
general, (Kostkova, et, al, 2016).
In addition, the re-use and authorship of the data remain an ethical issue. Again, trust issues
from the users that have been placed on the automated tools for data mining and
interpretation is also an ethical and security concern, (Mittelstadt, and Floridi, 2016). The
general strategies developed for data processing and tools which has been independently done
and this limits data used in other settings.
For addressing these data ethical issues, the arrangements may have to change if larger-scale
data is to be collected, stored and analyzed in future in various ways. The company needs to
adopt a participatory approach where every individual that interacts with the data embrace
reflexive management of data practices by adhering to all regulatory policies in place,
(Bratton, and Gold, 2017). Such policies include the utilization of the historical dataset
without reusing other peoples developed datasets. The adoption of advanced skills by other
data users is also key not only to addressing the ethical issues for the data science initiatives
but also ensuring that the research outputs are of high quality and reliable, (Nichols, et, al,
2017).
2.2.5 Report Section 5: Data science in next steps and potential solutions
Generally, data science has the potential to provide working solutions to solve most problems
that a company can face while doing its general operations. In addition, FluffyGroCo can
utilize different statistics within the data science including various tools, while making
decisions at management levels. As a result, it will also aid in making the automatic
operations at workplace thus reducing employees of other tasks that are also time-consuming.
In the recent past, there is continued growth in machine learning and data science. Through
this, other observable interest is based in the natural languages, recognition of the images as
well as the chatbots which are general processes. For effective decision-making processes,
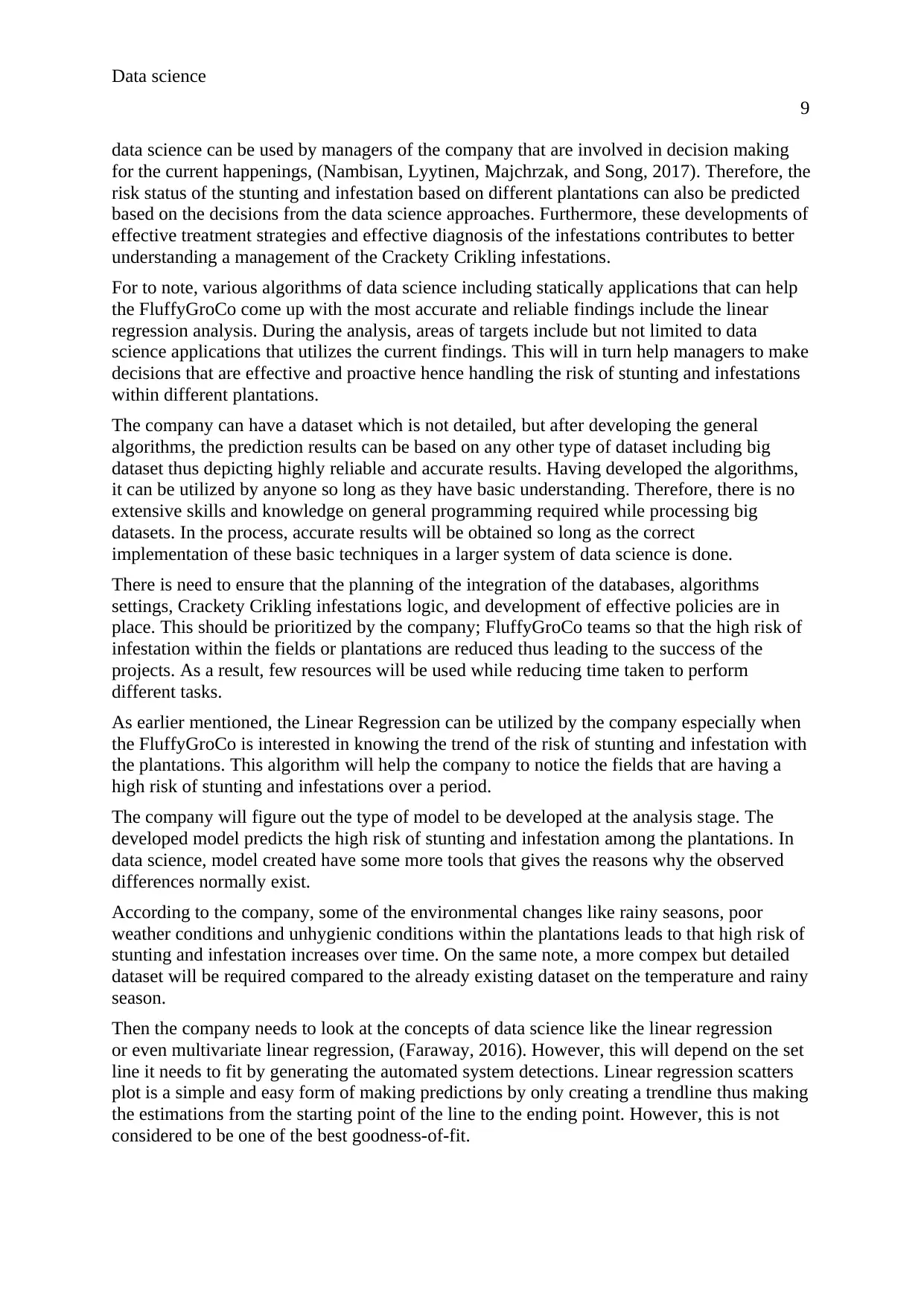
Data science
9
data science can be used by managers of the company that are involved in decision making
for the current happenings, (Nambisan, Lyytinen, Majchrzak, and Song, 2017). Therefore, the
risk status of the stunting and infestation based on different plantations can also be predicted
based on the decisions from the data science approaches. Furthermore, these developments of
effective treatment strategies and effective diagnosis of the infestations contributes to better
understanding a management of the Crackety Crikling infestations.
For to note, various algorithms of data science including statically applications that can help
the FluffyGroCo come up with the most accurate and reliable findings include the linear
regression analysis. During the analysis, areas of targets include but not limited to data
science applications that utilizes the current findings. This will in turn help managers to make
decisions that are effective and proactive hence handling the risk of stunting and infestations
within different plantations.
The company can have a dataset which is not detailed, but after developing the general
algorithms, the prediction results can be based on any other type of dataset including big
dataset thus depicting highly reliable and accurate results. Having developed the algorithms,
it can be utilized by anyone so long as they have basic understanding. Therefore, there is no
extensive skills and knowledge on general programming required while processing big
datasets. In the process, accurate results will be obtained so long as the correct
implementation of these basic techniques in a larger system of data science is done.
There is need to ensure that the planning of the integration of the databases, algorithms
settings, Crackety Crikling infestations logic, and development of effective policies are in
place. This should be prioritized by the company; FluffyGroCo teams so that the high risk of
infestation within the fields or plantations are reduced thus leading to the success of the
projects. As a result, few resources will be used while reducing time taken to perform
different tasks.
As earlier mentioned, the Linear Regression can be utilized by the company especially when
the FluffyGroCo is interested in knowing the trend of the risk of stunting and infestation with
the plantations. This algorithm will help the company to notice the fields that are having a
high risk of stunting and infestations over a period.
The company will figure out the type of model to be developed at the analysis stage. The
developed model predicts the high risk of stunting and infestation among the plantations. In
data science, model created have some more tools that gives the reasons why the observed
differences normally exist.
According to the company, some of the environmental changes like rainy seasons, poor
weather conditions and unhygienic conditions within the plantations leads to that high risk of
stunting and infestation increases over time. On the same note, a more compex but detailed
dataset will be required compared to the already existing dataset on the temperature and rainy
season.
Then the company needs to look at the concepts of data science like the linear regression
or even multivariate linear regression, (Faraway, 2016). However, this will depend on the set
line it needs to fit by generating the automated system detections. Linear regression scatters
plot is a simple and easy form of making predictions by only creating a trendline thus making
the estimations from the starting point of the line to the ending point. However, this is not
considered to be one of the best goodness-of-fit.
9
data science can be used by managers of the company that are involved in decision making
for the current happenings, (Nambisan, Lyytinen, Majchrzak, and Song, 2017). Therefore, the
risk status of the stunting and infestation based on different plantations can also be predicted
based on the decisions from the data science approaches. Furthermore, these developments of
effective treatment strategies and effective diagnosis of the infestations contributes to better
understanding a management of the Crackety Crikling infestations.
For to note, various algorithms of data science including statically applications that can help
the FluffyGroCo come up with the most accurate and reliable findings include the linear
regression analysis. During the analysis, areas of targets include but not limited to data
science applications that utilizes the current findings. This will in turn help managers to make
decisions that are effective and proactive hence handling the risk of stunting and infestations
within different plantations.
The company can have a dataset which is not detailed, but after developing the general
algorithms, the prediction results can be based on any other type of dataset including big
dataset thus depicting highly reliable and accurate results. Having developed the algorithms,
it can be utilized by anyone so long as they have basic understanding. Therefore, there is no
extensive skills and knowledge on general programming required while processing big
datasets. In the process, accurate results will be obtained so long as the correct
implementation of these basic techniques in a larger system of data science is done.
There is need to ensure that the planning of the integration of the databases, algorithms
settings, Crackety Crikling infestations logic, and development of effective policies are in
place. This should be prioritized by the company; FluffyGroCo teams so that the high risk of
infestation within the fields or plantations are reduced thus leading to the success of the
projects. As a result, few resources will be used while reducing time taken to perform
different tasks.
As earlier mentioned, the Linear Regression can be utilized by the company especially when
the FluffyGroCo is interested in knowing the trend of the risk of stunting and infestation with
the plantations. This algorithm will help the company to notice the fields that are having a
high risk of stunting and infestations over a period.
The company will figure out the type of model to be developed at the analysis stage. The
developed model predicts the high risk of stunting and infestation among the plantations. In
data science, model created have some more tools that gives the reasons why the observed
differences normally exist.
According to the company, some of the environmental changes like rainy seasons, poor
weather conditions and unhygienic conditions within the plantations leads to that high risk of
stunting and infestation increases over time. On the same note, a more compex but detailed
dataset will be required compared to the already existing dataset on the temperature and rainy
season.
Then the company needs to look at the concepts of data science like the linear regression
or even multivariate linear regression, (Faraway, 2016). However, this will depend on the set
line it needs to fit by generating the automated system detections. Linear regression scatters
plot is a simple and easy form of making predictions by only creating a trendline thus making
the estimations from the starting point of the line to the ending point. However, this is not
considered to be one of the best goodness-of-fit.
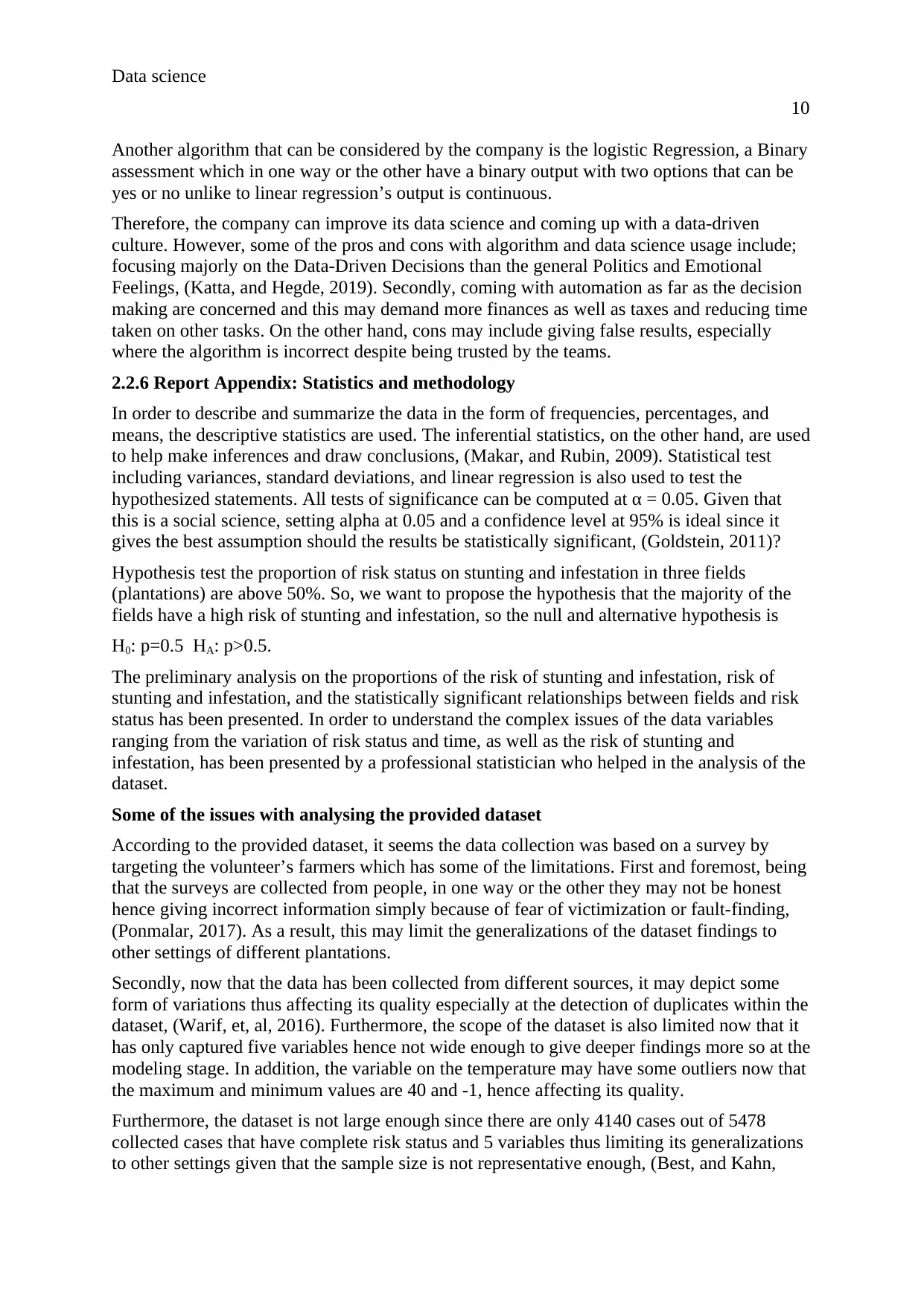
Data science
10
Another algorithm that can be considered by the company is the logistic Regression, a Binary
assessment which in one way or the other have a binary output with two options that can be
yes or no unlike to linear regression’s output is continuous.
Therefore, the company can improve its data science and coming up with a data-driven
culture. However, some of the pros and cons with algorithm and data science usage include;
focusing majorly on the Data-Driven Decisions than the general Politics and Emotional
Feelings, (Katta, and Hegde, 2019). Secondly, coming with automation as far as the decision
making are concerned and this may demand more finances as well as taxes and reducing time
taken on other tasks. On the other hand, cons may include giving false results, especially
where the algorithm is incorrect despite being trusted by the teams.
2.2.6 Report Appendix: Statistics and methodology
In order to describe and summarize the data in the form of frequencies, percentages, and
means, the descriptive statistics are used. The inferential statistics, on the other hand, are used
to help make inferences and draw conclusions, (Makar, and Rubin, 2009). Statistical test
including variances, standard deviations, and linear regression is also used to test the
hypothesized statements. All tests of significance can be computed at α = 0.05. Given that
this is a social science, setting alpha at 0.05 and a confidence level at 95% is ideal since it
gives the best assumption should the results be statistically significant, (Goldstein, 2011)?
Hypothesis test the proportion of risk status on stunting and infestation in three fields
(plantations) are above 50%. So, we want to propose the hypothesis that the majority of the
fields have a high risk of stunting and infestation, so the null and alternative hypothesis is
H0: p=0.5 HA: p>0.5.
The preliminary analysis on the proportions of the risk of stunting and infestation, risk of
stunting and infestation, and the statistically significant relationships between fields and risk
status has been presented. In order to understand the complex issues of the data variables
ranging from the variation of risk status and time, as well as the risk of stunting and
infestation, has been presented by a professional statistician who helped in the analysis of the
dataset.
Some of the issues with analysing the provided dataset
According to the provided dataset, it seems the data collection was based on a survey by
targeting the volunteer’s farmers which has some of the limitations. First and foremost, being
that the surveys are collected from people, in one way or the other they may not be honest
hence giving incorrect information simply because of fear of victimization or fault-finding,
(Ponmalar, 2017). As a result, this may limit the generalizations of the dataset findings to
other settings of different plantations.
Secondly, now that the data has been collected from different sources, it may depict some
form of variations thus affecting its quality especially at the detection of duplicates within the
dataset, (Warif, et, al, 2016). Furthermore, the scope of the dataset is also limited now that it
has only captured five variables hence not wide enough to give deeper findings more so at the
modeling stage. In addition, the variable on the temperature may have some outliers now that
the maximum and minimum values are 40 and -1, hence affecting its quality.
Furthermore, the dataset is not large enough since there are only 4140 cases out of 5478
collected cases that have complete risk status and 5 variables thus limiting its generalizations
to other settings given that the sample size is not representative enough, (Best, and Kahn,
10
Another algorithm that can be considered by the company is the logistic Regression, a Binary
assessment which in one way or the other have a binary output with two options that can be
yes or no unlike to linear regression’s output is continuous.
Therefore, the company can improve its data science and coming up with a data-driven
culture. However, some of the pros and cons with algorithm and data science usage include;
focusing majorly on the Data-Driven Decisions than the general Politics and Emotional
Feelings, (Katta, and Hegde, 2019). Secondly, coming with automation as far as the decision
making are concerned and this may demand more finances as well as taxes and reducing time
taken on other tasks. On the other hand, cons may include giving false results, especially
where the algorithm is incorrect despite being trusted by the teams.
2.2.6 Report Appendix: Statistics and methodology
In order to describe and summarize the data in the form of frequencies, percentages, and
means, the descriptive statistics are used. The inferential statistics, on the other hand, are used
to help make inferences and draw conclusions, (Makar, and Rubin, 2009). Statistical test
including variances, standard deviations, and linear regression is also used to test the
hypothesized statements. All tests of significance can be computed at α = 0.05. Given that
this is a social science, setting alpha at 0.05 and a confidence level at 95% is ideal since it
gives the best assumption should the results be statistically significant, (Goldstein, 2011)?
Hypothesis test the proportion of risk status on stunting and infestation in three fields
(plantations) are above 50%. So, we want to propose the hypothesis that the majority of the
fields have a high risk of stunting and infestation, so the null and alternative hypothesis is
H0: p=0.5 HA: p>0.5.
The preliminary analysis on the proportions of the risk of stunting and infestation, risk of
stunting and infestation, and the statistically significant relationships between fields and risk
status has been presented. In order to understand the complex issues of the data variables
ranging from the variation of risk status and time, as well as the risk of stunting and
infestation, has been presented by a professional statistician who helped in the analysis of the
dataset.
Some of the issues with analysing the provided dataset
According to the provided dataset, it seems the data collection was based on a survey by
targeting the volunteer’s farmers which has some of the limitations. First and foremost, being
that the surveys are collected from people, in one way or the other they may not be honest
hence giving incorrect information simply because of fear of victimization or fault-finding,
(Ponmalar, 2017). As a result, this may limit the generalizations of the dataset findings to
other settings of different plantations.
Secondly, now that the data has been collected from different sources, it may depict some
form of variations thus affecting its quality especially at the detection of duplicates within the
dataset, (Warif, et, al, 2016). Furthermore, the scope of the dataset is also limited now that it
has only captured five variables hence not wide enough to give deeper findings more so at the
modeling stage. In addition, the variable on the temperature may have some outliers now that
the maximum and minimum values are 40 and -1, hence affecting its quality.
Furthermore, the dataset is not large enough since there are only 4140 cases out of 5478
collected cases that have complete risk status and 5 variables thus limiting its generalizations
to other settings given that the sample size is not representative enough, (Best, and Kahn,
Paraphrase This Document
Need a fresh take? Get an instant paraphrase of this document with our AI Paraphraser
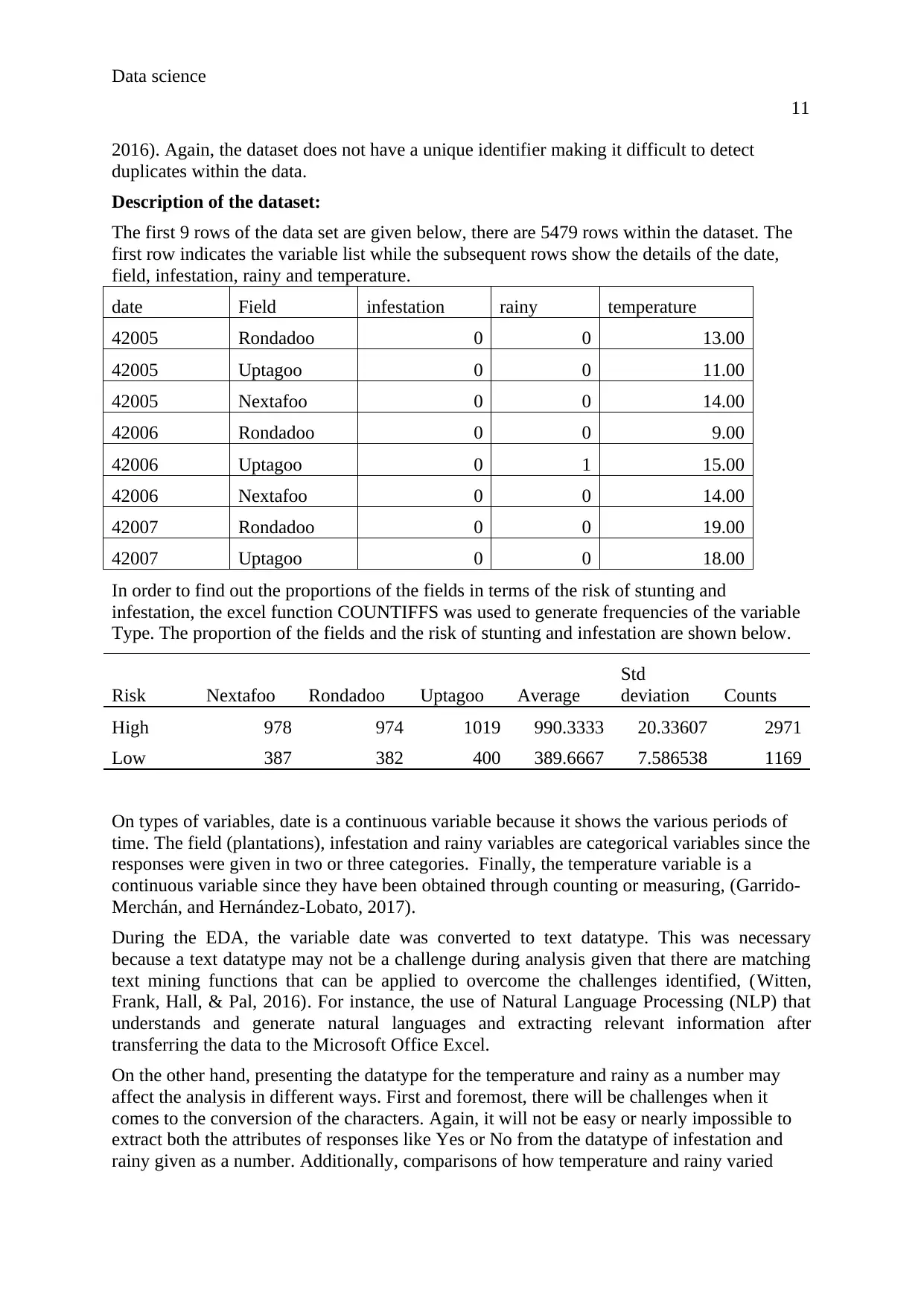
Data science
11
2016). Again, the dataset does not have a unique identifier making it difficult to detect
duplicates within the data.
Description of the dataset:
The first 9 rows of the data set are given below, there are 5479 rows within the dataset. The
first row indicates the variable list while the subsequent rows show the details of the date,
field, infestation, rainy and temperature.
date Field infestation rainy temperature
42005 Rondadoo 0 0 13.00
42005 Uptagoo 0 0 11.00
42005 Nextafoo 0 0 14.00
42006 Rondadoo 0 0 9.00
42006 Uptagoo 0 1 15.00
42006 Nextafoo 0 0 14.00
42007 Rondadoo 0 0 19.00
42007 Uptagoo 0 0 18.00
In order to find out the proportions of the fields in terms of the risk of stunting and
infestation, the excel function COUNTIFFS was used to generate frequencies of the variable
Type. The proportion of the fields and the risk of stunting and infestation are shown below.
Risk Nextafoo Rondadoo Uptagoo Average
Std
deviation Counts
High 978 974 1019 990.3333 20.33607 2971
Low 387 382 400 389.6667 7.586538 1169
On types of variables, date is a continuous variable because it shows the various periods of
time. The field (plantations), infestation and rainy variables are categorical variables since the
responses were given in two or three categories. Finally, the temperature variable is a
continuous variable since they have been obtained through counting or measuring, (Garrido-
Merchán, and Hernández-Lobato, 2017).
During the EDA, the variable date was converted to text datatype. This was necessary
because a text datatype may not be a challenge during analysis given that there are matching
text mining functions that can be applied to overcome the challenges identified, (Witten,
Frank, Hall, & Pal, 2016). For instance, the use of Natural Language Processing (NLP) that
understands and generate natural languages and extracting relevant information after
transferring the data to the Microsoft Office Excel.
On the other hand, presenting the datatype for the temperature and rainy as a number may
affect the analysis in different ways. First and foremost, there will be challenges when it
comes to the conversion of the characters. Again, it will not be easy or nearly impossible to
extract both the attributes of responses like Yes or No from the datatype of infestation and
rainy given as a number. Additionally, comparisons of how temperature and rainy varied
11
2016). Again, the dataset does not have a unique identifier making it difficult to detect
duplicates within the data.
Description of the dataset:
The first 9 rows of the data set are given below, there are 5479 rows within the dataset. The
first row indicates the variable list while the subsequent rows show the details of the date,
field, infestation, rainy and temperature.
date Field infestation rainy temperature
42005 Rondadoo 0 0 13.00
42005 Uptagoo 0 0 11.00
42005 Nextafoo 0 0 14.00
42006 Rondadoo 0 0 9.00
42006 Uptagoo 0 1 15.00
42006 Nextafoo 0 0 14.00
42007 Rondadoo 0 0 19.00
42007 Uptagoo 0 0 18.00
In order to find out the proportions of the fields in terms of the risk of stunting and
infestation, the excel function COUNTIFFS was used to generate frequencies of the variable
Type. The proportion of the fields and the risk of stunting and infestation are shown below.
Risk Nextafoo Rondadoo Uptagoo Average
Std
deviation Counts
High 978 974 1019 990.3333 20.33607 2971
Low 387 382 400 389.6667 7.586538 1169
On types of variables, date is a continuous variable because it shows the various periods of
time. The field (plantations), infestation and rainy variables are categorical variables since the
responses were given in two or three categories. Finally, the temperature variable is a
continuous variable since they have been obtained through counting or measuring, (Garrido-
Merchán, and Hernández-Lobato, 2017).
During the EDA, the variable date was converted to text datatype. This was necessary
because a text datatype may not be a challenge during analysis given that there are matching
text mining functions that can be applied to overcome the challenges identified, (Witten,
Frank, Hall, & Pal, 2016). For instance, the use of Natural Language Processing (NLP) that
understands and generate natural languages and extracting relevant information after
transferring the data to the Microsoft Office Excel.
On the other hand, presenting the datatype for the temperature and rainy as a number may
affect the analysis in different ways. First and foremost, there will be challenges when it
comes to the conversion of the characters. Again, it will not be easy or nearly impossible to
extract both the attributes of responses like Yes or No from the datatype of infestation and
rainy given as a number. Additionally, comparisons of how temperature and rainy varied
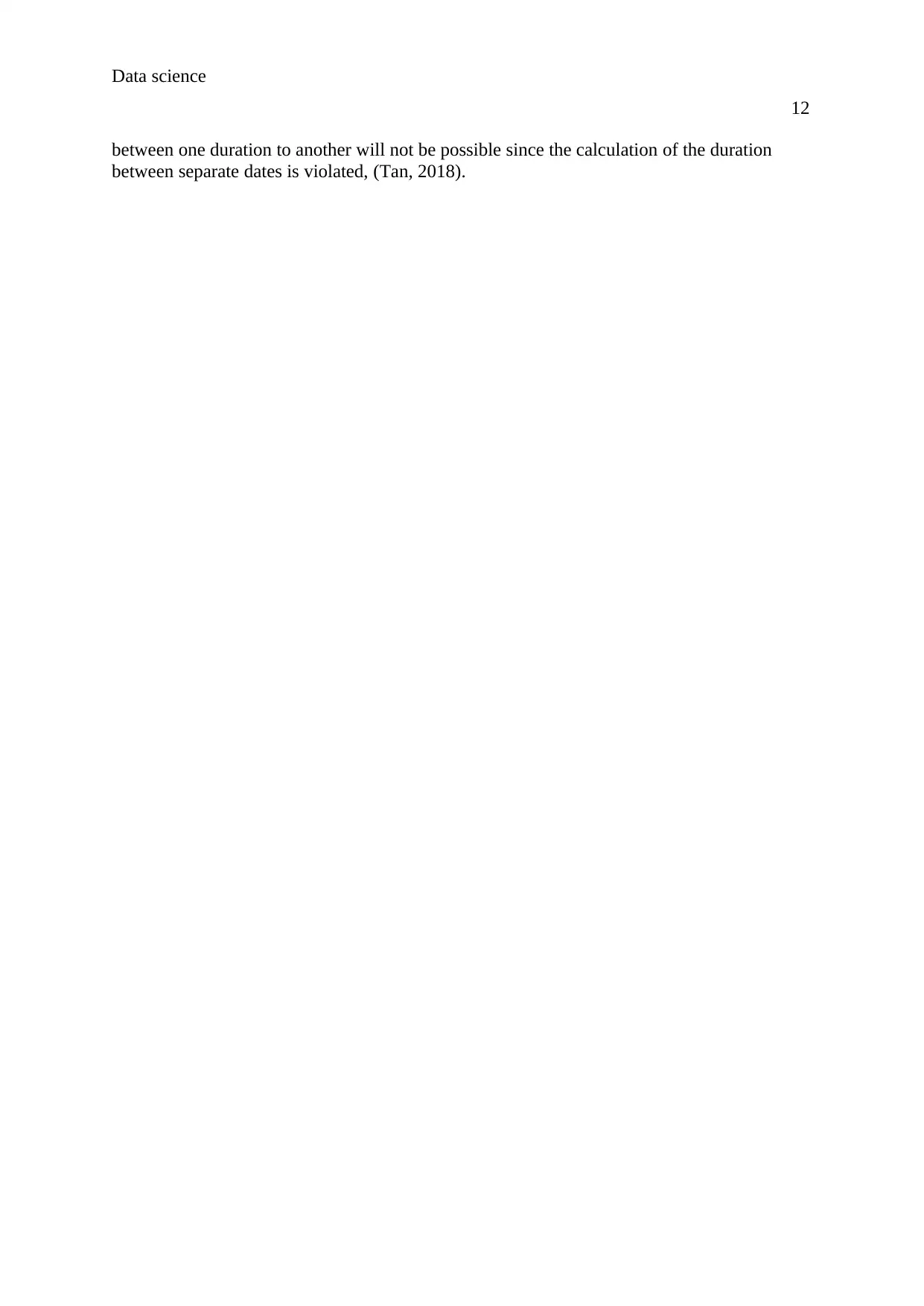
Data science
12
between one duration to another will not be possible since the calculation of the duration
between separate dates is violated, (Tan, 2018).
12
between one duration to another will not be possible since the calculation of the duration
between separate dates is violated, (Tan, 2018).
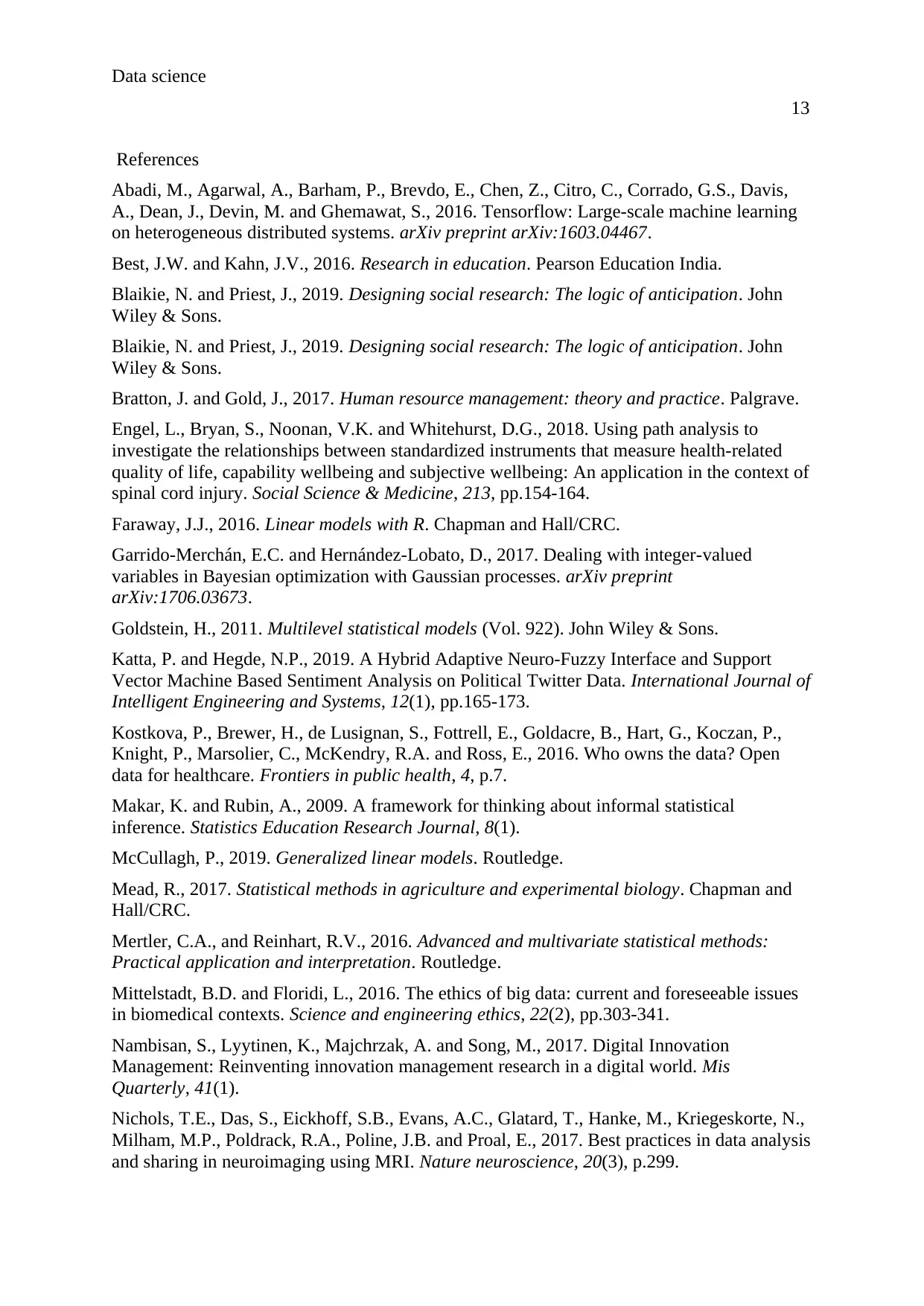
Data science
13
References
Abadi, M., Agarwal, A., Barham, P., Brevdo, E., Chen, Z., Citro, C., Corrado, G.S., Davis,
A., Dean, J., Devin, M. and Ghemawat, S., 2016. Tensorflow: Large-scale machine learning
on heterogeneous distributed systems. arXiv preprint arXiv:1603.04467.
Best, J.W. and Kahn, J.V., 2016. Research in education. Pearson Education India.
Blaikie, N. and Priest, J., 2019. Designing social research: The logic of anticipation. John
Wiley & Sons.
Blaikie, N. and Priest, J., 2019. Designing social research: The logic of anticipation. John
Wiley & Sons.
Bratton, J. and Gold, J., 2017. Human resource management: theory and practice. Palgrave.
Engel, L., Bryan, S., Noonan, V.K. and Whitehurst, D.G., 2018. Using path analysis to
investigate the relationships between standardized instruments that measure health-related
quality of life, capability wellbeing and subjective wellbeing: An application in the context of
spinal cord injury. Social Science & Medicine, 213, pp.154-164.
Faraway, J.J., 2016. Linear models with R. Chapman and Hall/CRC.
Garrido-Merchán, E.C. and Hernández-Lobato, D., 2017. Dealing with integer-valued
variables in Bayesian optimization with Gaussian processes. arXiv preprint
arXiv:1706.03673.
Goldstein, H., 2011. Multilevel statistical models (Vol. 922). John Wiley & Sons.
Katta, P. and Hegde, N.P., 2019. A Hybrid Adaptive Neuro-Fuzzy Interface and Support
Vector Machine Based Sentiment Analysis on Political Twitter Data. International Journal of
Intelligent Engineering and Systems, 12(1), pp.165-173.
Kostkova, P., Brewer, H., de Lusignan, S., Fottrell, E., Goldacre, B., Hart, G., Koczan, P.,
Knight, P., Marsolier, C., McKendry, R.A. and Ross, E., 2016. Who owns the data? Open
data for healthcare. Frontiers in public health, 4, p.7.
Makar, K. and Rubin, A., 2009. A framework for thinking about informal statistical
inference. Statistics Education Research Journal, 8(1).
McCullagh, P., 2019. Generalized linear models. Routledge.
Mead, R., 2017. Statistical methods in agriculture and experimental biology. Chapman and
Hall/CRC.
Mertler, C.A., and Reinhart, R.V., 2016. Advanced and multivariate statistical methods:
Practical application and interpretation. Routledge.
Mittelstadt, B.D. and Floridi, L., 2016. The ethics of big data: current and foreseeable issues
in biomedical contexts. Science and engineering ethics, 22(2), pp.303-341.
Nambisan, S., Lyytinen, K., Majchrzak, A. and Song, M., 2017. Digital Innovation
Management: Reinventing innovation management research in a digital world. Mis
Quarterly, 41(1).
Nichols, T.E., Das, S., Eickhoff, S.B., Evans, A.C., Glatard, T., Hanke, M., Kriegeskorte, N.,
Milham, M.P., Poldrack, R.A., Poline, J.B. and Proal, E., 2017. Best practices in data analysis
and sharing in neuroimaging using MRI. Nature neuroscience, 20(3), p.299.
13
References
Abadi, M., Agarwal, A., Barham, P., Brevdo, E., Chen, Z., Citro, C., Corrado, G.S., Davis,
A., Dean, J., Devin, M. and Ghemawat, S., 2016. Tensorflow: Large-scale machine learning
on heterogeneous distributed systems. arXiv preprint arXiv:1603.04467.
Best, J.W. and Kahn, J.V., 2016. Research in education. Pearson Education India.
Blaikie, N. and Priest, J., 2019. Designing social research: The logic of anticipation. John
Wiley & Sons.
Blaikie, N. and Priest, J., 2019. Designing social research: The logic of anticipation. John
Wiley & Sons.
Bratton, J. and Gold, J., 2017. Human resource management: theory and practice. Palgrave.
Engel, L., Bryan, S., Noonan, V.K. and Whitehurst, D.G., 2018. Using path analysis to
investigate the relationships between standardized instruments that measure health-related
quality of life, capability wellbeing and subjective wellbeing: An application in the context of
spinal cord injury. Social Science & Medicine, 213, pp.154-164.
Faraway, J.J., 2016. Linear models with R. Chapman and Hall/CRC.
Garrido-Merchán, E.C. and Hernández-Lobato, D., 2017. Dealing with integer-valued
variables in Bayesian optimization with Gaussian processes. arXiv preprint
arXiv:1706.03673.
Goldstein, H., 2011. Multilevel statistical models (Vol. 922). John Wiley & Sons.
Katta, P. and Hegde, N.P., 2019. A Hybrid Adaptive Neuro-Fuzzy Interface and Support
Vector Machine Based Sentiment Analysis on Political Twitter Data. International Journal of
Intelligent Engineering and Systems, 12(1), pp.165-173.
Kostkova, P., Brewer, H., de Lusignan, S., Fottrell, E., Goldacre, B., Hart, G., Koczan, P.,
Knight, P., Marsolier, C., McKendry, R.A. and Ross, E., 2016. Who owns the data? Open
data for healthcare. Frontiers in public health, 4, p.7.
Makar, K. and Rubin, A., 2009. A framework for thinking about informal statistical
inference. Statistics Education Research Journal, 8(1).
McCullagh, P., 2019. Generalized linear models. Routledge.
Mead, R., 2017. Statistical methods in agriculture and experimental biology. Chapman and
Hall/CRC.
Mertler, C.A., and Reinhart, R.V., 2016. Advanced and multivariate statistical methods:
Practical application and interpretation. Routledge.
Mittelstadt, B.D. and Floridi, L., 2016. The ethics of big data: current and foreseeable issues
in biomedical contexts. Science and engineering ethics, 22(2), pp.303-341.
Nambisan, S., Lyytinen, K., Majchrzak, A. and Song, M., 2017. Digital Innovation
Management: Reinventing innovation management research in a digital world. Mis
Quarterly, 41(1).
Nichols, T.E., Das, S., Eickhoff, S.B., Evans, A.C., Glatard, T., Hanke, M., Kriegeskorte, N.,
Milham, M.P., Poldrack, R.A., Poline, J.B. and Proal, E., 2017. Best practices in data analysis
and sharing in neuroimaging using MRI. Nature neuroscience, 20(3), p.299.
Secure Best Marks with AI Grader
Need help grading? Try our AI Grader for instant feedback on your assignments.
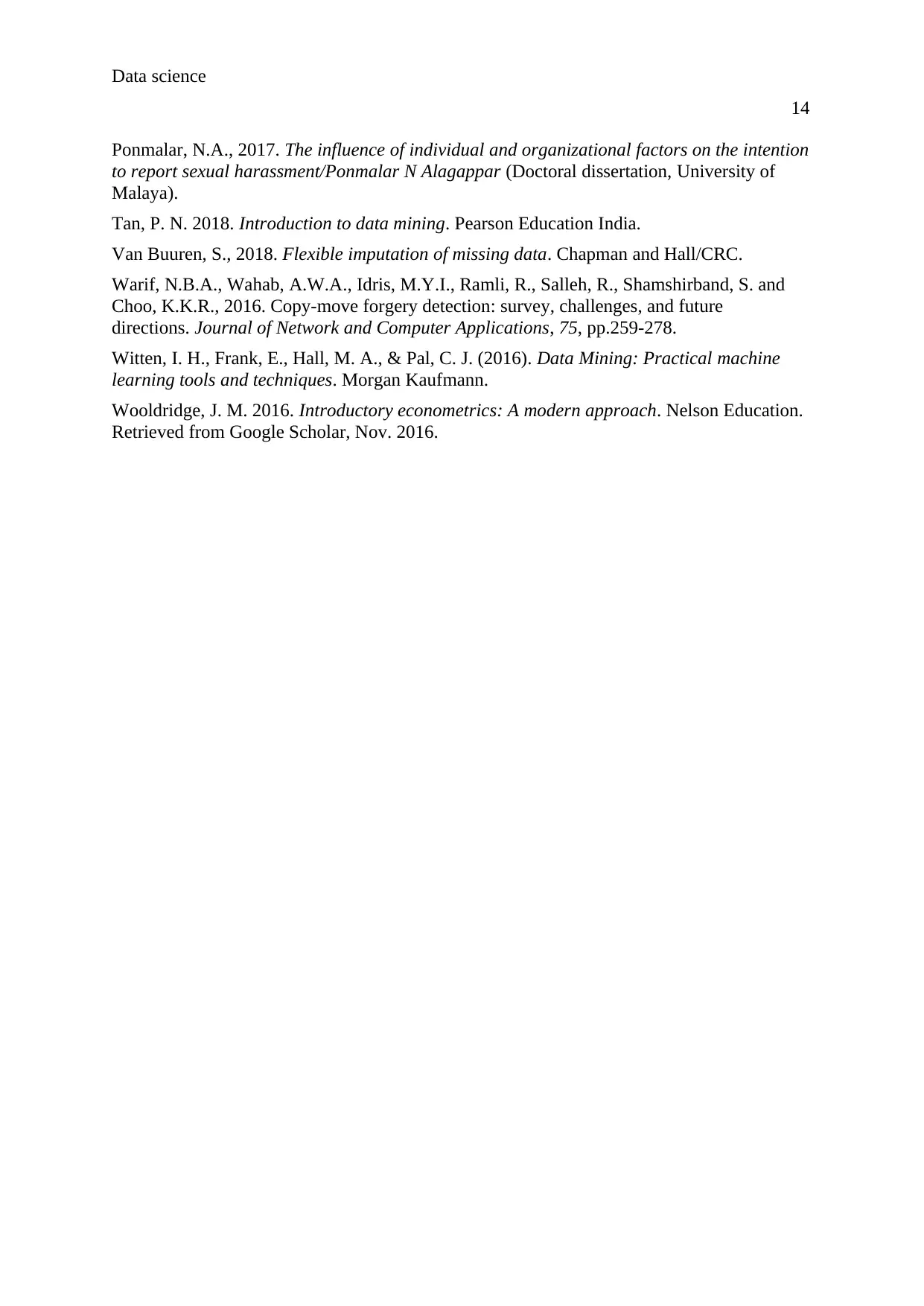
Data science
14
Ponmalar, N.A., 2017. The influence of individual and organizational factors on the intention
to report sexual harassment/Ponmalar N Alagappar (Doctoral dissertation, University of
Malaya).
Tan, P. N. 2018. Introduction to data mining. Pearson Education India.
Van Buuren, S., 2018. Flexible imputation of missing data. Chapman and Hall/CRC.
Warif, N.B.A., Wahab, A.W.A., Idris, M.Y.I., Ramli, R., Salleh, R., Shamshirband, S. and
Choo, K.K.R., 2016. Copy-move forgery detection: survey, challenges, and future
directions. Journal of Network and Computer Applications, 75, pp.259-278.
Witten, I. H., Frank, E., Hall, M. A., & Pal, C. J. (2016). Data Mining: Practical machine
learning tools and techniques. Morgan Kaufmann.
Wooldridge, J. M. 2016. Introductory econometrics: A modern approach. Nelson Education.
Retrieved from Google Scholar, Nov. 2016.
14
Ponmalar, N.A., 2017. The influence of individual and organizational factors on the intention
to report sexual harassment/Ponmalar N Alagappar (Doctoral dissertation, University of
Malaya).
Tan, P. N. 2018. Introduction to data mining. Pearson Education India.
Van Buuren, S., 2018. Flexible imputation of missing data. Chapman and Hall/CRC.
Warif, N.B.A., Wahab, A.W.A., Idris, M.Y.I., Ramli, R., Salleh, R., Shamshirband, S. and
Choo, K.K.R., 2016. Copy-move forgery detection: survey, challenges, and future
directions. Journal of Network and Computer Applications, 75, pp.259-278.
Witten, I. H., Frank, E., Hall, M. A., & Pal, C. J. (2016). Data Mining: Practical machine
learning tools and techniques. Morgan Kaufmann.
Wooldridge, J. M. 2016. Introductory econometrics: A modern approach. Nelson Education.
Retrieved from Google Scholar, Nov. 2016.
1 out of 14
Related Documents
![[object Object]](/_next/image/?url=%2F_next%2Fstatic%2Fmedia%2Flogo.6d15ce61.png&w=640&q=75)
Your All-in-One AI-Powered Toolkit for Academic Success.
+13062052269
info@desklib.com
Available 24*7 on WhatsApp / Email
Unlock your academic potential
© 2024 | Zucol Services PVT LTD | All rights reserved.