Airline Financial Performance Analysis
VerifiedAdded on 2020/02/14
|20
|5455
|250
AI Summary
This assignment delves into the financial performance of an airline by analyzing key revenue metrics. Students will calculate and interpret Revenue per Available Seat Mile (RASM), Revenue Passenger Miles (RPM), and Revenue Passenger Kilometers (RPK). The analysis will focus on understanding the relationship between these metrics, their impact on profitability, and factors influencing their fluctuations. The assignment provides a framework for assessing an airline's financial health and identifying areas for improvement.
Contribute Materials
Your contribution can guide someone’s learning journey. Share your
documents today.
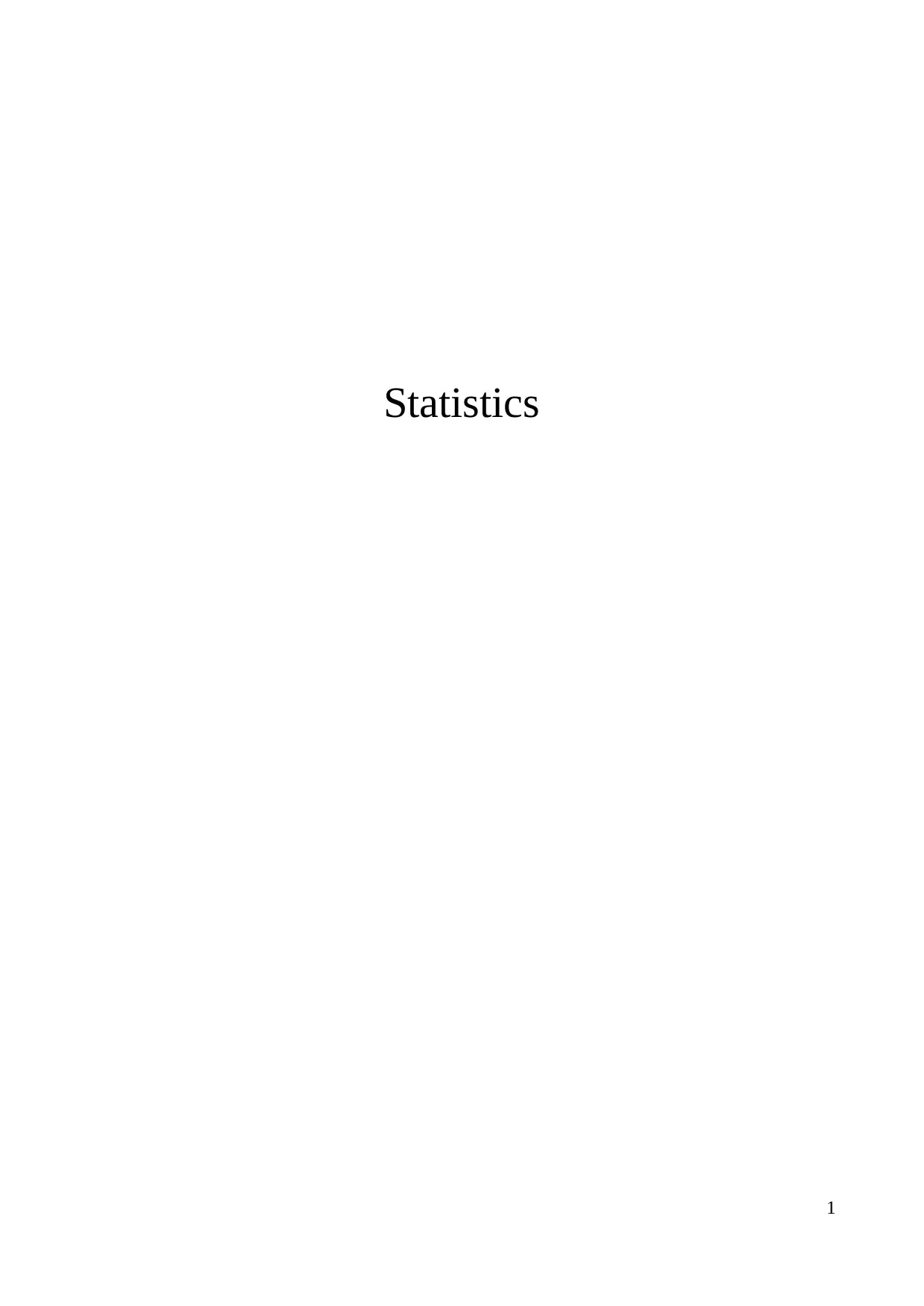
Statistics
1
1
Secure Best Marks with AI Grader
Need help grading? Try our AI Grader for instant feedback on your assignments.
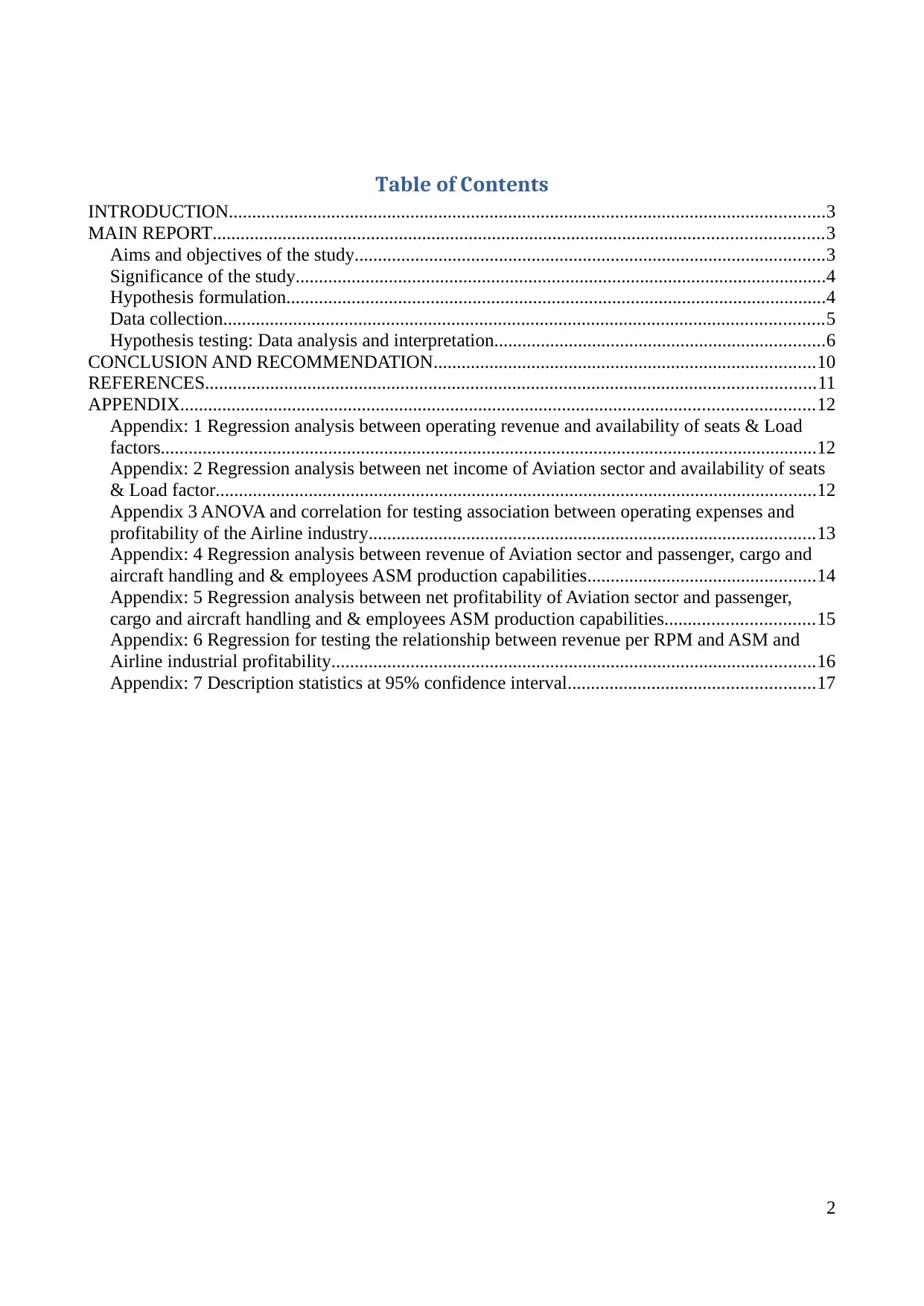
Table of Contents
INTRODUCTION................................................................................................................................3
MAIN REPORT...................................................................................................................................3
Aims and objectives of the study.....................................................................................................3
Significance of the study..................................................................................................................4
Hypothesis formulation....................................................................................................................4
Data collection.................................................................................................................................5
Hypothesis testing: Data analysis and interpretation.......................................................................6
CONCLUSION AND RECOMMENDATION..................................................................................10
REFERENCES...................................................................................................................................11
APPENDIX........................................................................................................................................12
Appendix: 1 Regression analysis between operating revenue and availability of seats & Load
factors.............................................................................................................................................12
Appendix: 2 Regression analysis between net income of Aviation sector and availability of seats
& Load factor.................................................................................................................................12
Appendix 3 ANOVA and correlation for testing association between operating expenses and
profitability of the Airline industry................................................................................................13
Appendix: 4 Regression analysis between revenue of Aviation sector and passenger, cargo and
aircraft handling and & employees ASM production capabilities.................................................14
Appendix: 5 Regression analysis between net profitability of Aviation sector and passenger,
cargo and aircraft handling and & employees ASM production capabilities................................15
Appendix: 6 Regression for testing the relationship between revenue per RPM and ASM and
Airline industrial profitability........................................................................................................16
Appendix: 7 Description statistics at 95% confidence interval.....................................................17
2
INTRODUCTION................................................................................................................................3
MAIN REPORT...................................................................................................................................3
Aims and objectives of the study.....................................................................................................3
Significance of the study..................................................................................................................4
Hypothesis formulation....................................................................................................................4
Data collection.................................................................................................................................5
Hypothesis testing: Data analysis and interpretation.......................................................................6
CONCLUSION AND RECOMMENDATION..................................................................................10
REFERENCES...................................................................................................................................11
APPENDIX........................................................................................................................................12
Appendix: 1 Regression analysis between operating revenue and availability of seats & Load
factors.............................................................................................................................................12
Appendix: 2 Regression analysis between net income of Aviation sector and availability of seats
& Load factor.................................................................................................................................12
Appendix 3 ANOVA and correlation for testing association between operating expenses and
profitability of the Airline industry................................................................................................13
Appendix: 4 Regression analysis between revenue of Aviation sector and passenger, cargo and
aircraft handling and & employees ASM production capabilities.................................................14
Appendix: 5 Regression analysis between net profitability of Aviation sector and passenger,
cargo and aircraft handling and & employees ASM production capabilities................................15
Appendix: 6 Regression for testing the relationship between revenue per RPM and ASM and
Airline industrial profitability........................................................................................................16
Appendix: 7 Description statistics at 95% confidence interval.....................................................17
2
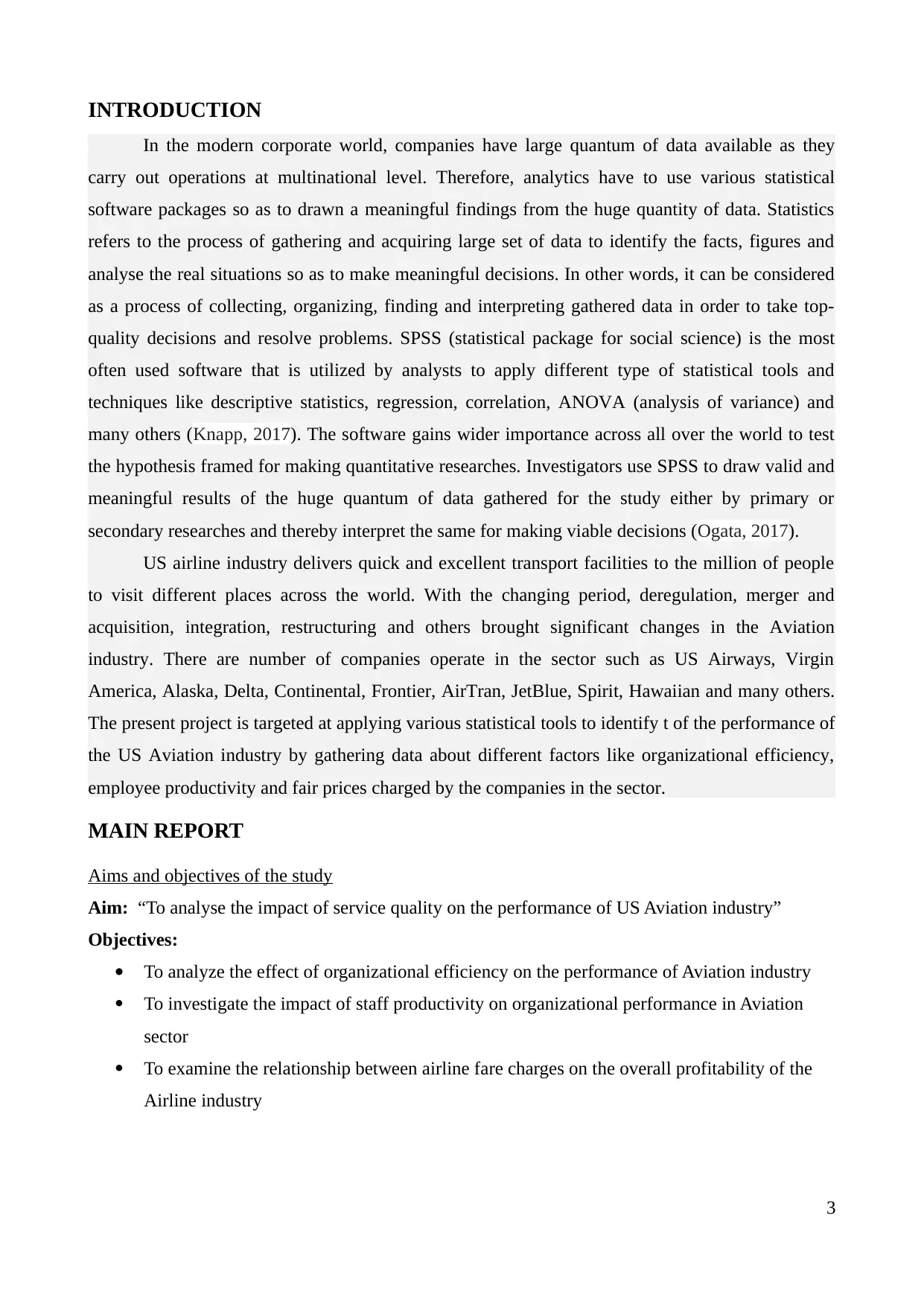
INTRODUCTION
In the modern corporate world, companies have large quantum of data available as they
carry out operations at multinational level. Therefore, analytics have to use various statistical
software packages so as to drawn a meaningful findings from the huge quantity of data. Statistics
refers to the process of gathering and acquiring large set of data to identify the facts, figures and
analyse the real situations so as to make meaningful decisions. In other words, it can be considered
as a process of collecting, organizing, finding and interpreting gathered data in order to take top-
quality decisions and resolve problems. SPSS (statistical package for social science) is the most
often used software that is utilized by analysts to apply different type of statistical tools and
techniques like descriptive statistics, regression, correlation, ANOVA (analysis of variance) and
many others (Knapp, 2017). The software gains wider importance across all over the world to test
the hypothesis framed for making quantitative researches. Investigators use SPSS to draw valid and
meaningful results of the huge quantum of data gathered for the study either by primary or
secondary researches and thereby interpret the same for making viable decisions (Ogata, 2017).
US airline industry delivers quick and excellent transport facilities to the million of people
to visit different places across the world. With the changing period, deregulation, merger and
acquisition, integration, restructuring and others brought significant changes in the Aviation
industry. There are number of companies operate in the sector such as US Airways, Virgin
America, Alaska, Delta, Continental, Frontier, AirTran, JetBlue, Spirit, Hawaiian and many others.
The present project is targeted at applying various statistical tools to identify t of the performance of
the US Aviation industry by gathering data about different factors like organizational efficiency,
employee productivity and fair prices charged by the companies in the sector.
MAIN REPORT
Aims and objectives of the study
Aim: “To analyse the impact of service quality on the performance of US Aviation industry”
Objectives:
To analyze the effect of organizational efficiency on the performance of Aviation industry
To investigate the impact of staff productivity on organizational performance in Aviation
sector
To examine the relationship between airline fare charges on the overall profitability of the
Airline industry
3
In the modern corporate world, companies have large quantum of data available as they
carry out operations at multinational level. Therefore, analytics have to use various statistical
software packages so as to drawn a meaningful findings from the huge quantity of data. Statistics
refers to the process of gathering and acquiring large set of data to identify the facts, figures and
analyse the real situations so as to make meaningful decisions. In other words, it can be considered
as a process of collecting, organizing, finding and interpreting gathered data in order to take top-
quality decisions and resolve problems. SPSS (statistical package for social science) is the most
often used software that is utilized by analysts to apply different type of statistical tools and
techniques like descriptive statistics, regression, correlation, ANOVA (analysis of variance) and
many others (Knapp, 2017). The software gains wider importance across all over the world to test
the hypothesis framed for making quantitative researches. Investigators use SPSS to draw valid and
meaningful results of the huge quantum of data gathered for the study either by primary or
secondary researches and thereby interpret the same for making viable decisions (Ogata, 2017).
US airline industry delivers quick and excellent transport facilities to the million of people
to visit different places across the world. With the changing period, deregulation, merger and
acquisition, integration, restructuring and others brought significant changes in the Aviation
industry. There are number of companies operate in the sector such as US Airways, Virgin
America, Alaska, Delta, Continental, Frontier, AirTran, JetBlue, Spirit, Hawaiian and many others.
The present project is targeted at applying various statistical tools to identify t of the performance of
the US Aviation industry by gathering data about different factors like organizational efficiency,
employee productivity and fair prices charged by the companies in the sector.
MAIN REPORT
Aims and objectives of the study
Aim: “To analyse the impact of service quality on the performance of US Aviation industry”
Objectives:
To analyze the effect of organizational efficiency on the performance of Aviation industry
To investigate the impact of staff productivity on organizational performance in Aviation
sector
To examine the relationship between airline fare charges on the overall profitability of the
Airline industry
3
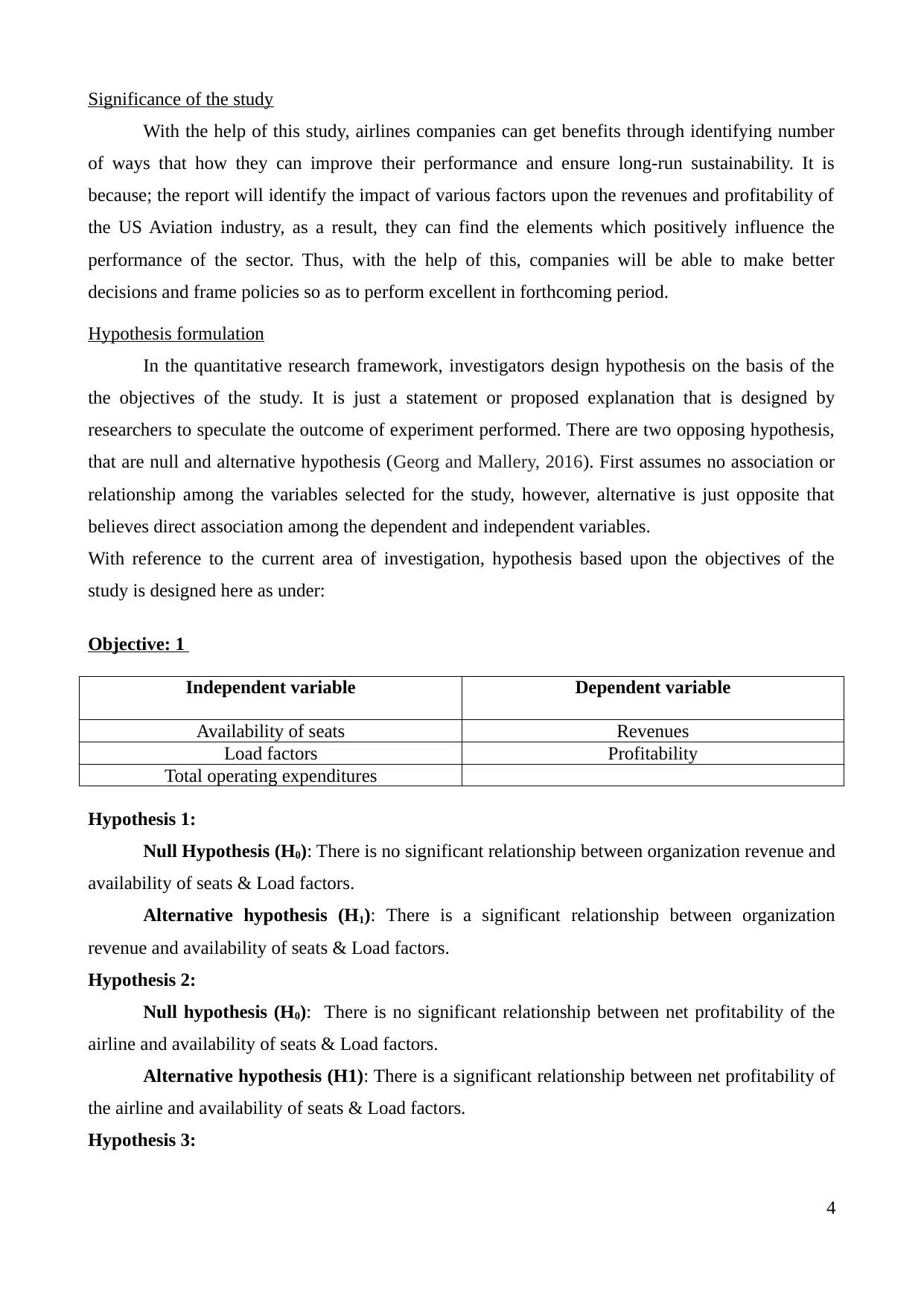
Significance of the study
With the help of this study, airlines companies can get benefits through identifying number
of ways that how they can improve their performance and ensure long-run sustainability. It is
because; the report will identify the impact of various factors upon the revenues and profitability of
the US Aviation industry, as a result, they can find the elements which positively influence the
performance of the sector. Thus, with the help of this, companies will be able to make better
decisions and frame policies so as to perform excellent in forthcoming period.
Hypothesis formulation
In the quantitative research framework, investigators design hypothesis on the basis of the
the objectives of the study. It is just a statement or proposed explanation that is designed by
researchers to speculate the outcome of experiment performed. There are two opposing hypothesis,
that are null and alternative hypothesis (Georg and Mallery, 2016). First assumes no association or
relationship among the variables selected for the study, however, alternative is just opposite that
believes direct association among the dependent and independent variables.
With reference to the current area of investigation, hypothesis based upon the objectives of the
study is designed here as under:
Objective: 1
Independent variable Dependent variable
Availability of seats Revenues
Load factors Profitability
Total operating expenditures
Hypothesis 1:
Null Hypothesis (H0): There is no significant relationship between organization revenue and
availability of seats & Load factors.
Alternative hypothesis (H1): There is a significant relationship between organization
revenue and availability of seats & Load factors.
Hypothesis 2:
Null hypothesis (H0): There is no significant relationship between net profitability of the
airline and availability of seats & Load factors.
Alternative hypothesis (H1): There is a significant relationship between net profitability of
the airline and availability of seats & Load factors.
Hypothesis 3:
4
With the help of this study, airlines companies can get benefits through identifying number
of ways that how they can improve their performance and ensure long-run sustainability. It is
because; the report will identify the impact of various factors upon the revenues and profitability of
the US Aviation industry, as a result, they can find the elements which positively influence the
performance of the sector. Thus, with the help of this, companies will be able to make better
decisions and frame policies so as to perform excellent in forthcoming period.
Hypothesis formulation
In the quantitative research framework, investigators design hypothesis on the basis of the
the objectives of the study. It is just a statement or proposed explanation that is designed by
researchers to speculate the outcome of experiment performed. There are two opposing hypothesis,
that are null and alternative hypothesis (Georg and Mallery, 2016). First assumes no association or
relationship among the variables selected for the study, however, alternative is just opposite that
believes direct association among the dependent and independent variables.
With reference to the current area of investigation, hypothesis based upon the objectives of the
study is designed here as under:
Objective: 1
Independent variable Dependent variable
Availability of seats Revenues
Load factors Profitability
Total operating expenditures
Hypothesis 1:
Null Hypothesis (H0): There is no significant relationship between organization revenue and
availability of seats & Load factors.
Alternative hypothesis (H1): There is a significant relationship between organization
revenue and availability of seats & Load factors.
Hypothesis 2:
Null hypothesis (H0): There is no significant relationship between net profitability of the
airline and availability of seats & Load factors.
Alternative hypothesis (H1): There is a significant relationship between net profitability of
the airline and availability of seats & Load factors.
Hypothesis 3:
4
Secure Best Marks with AI Grader
Need help grading? Try our AI Grader for instant feedback on your assignments.
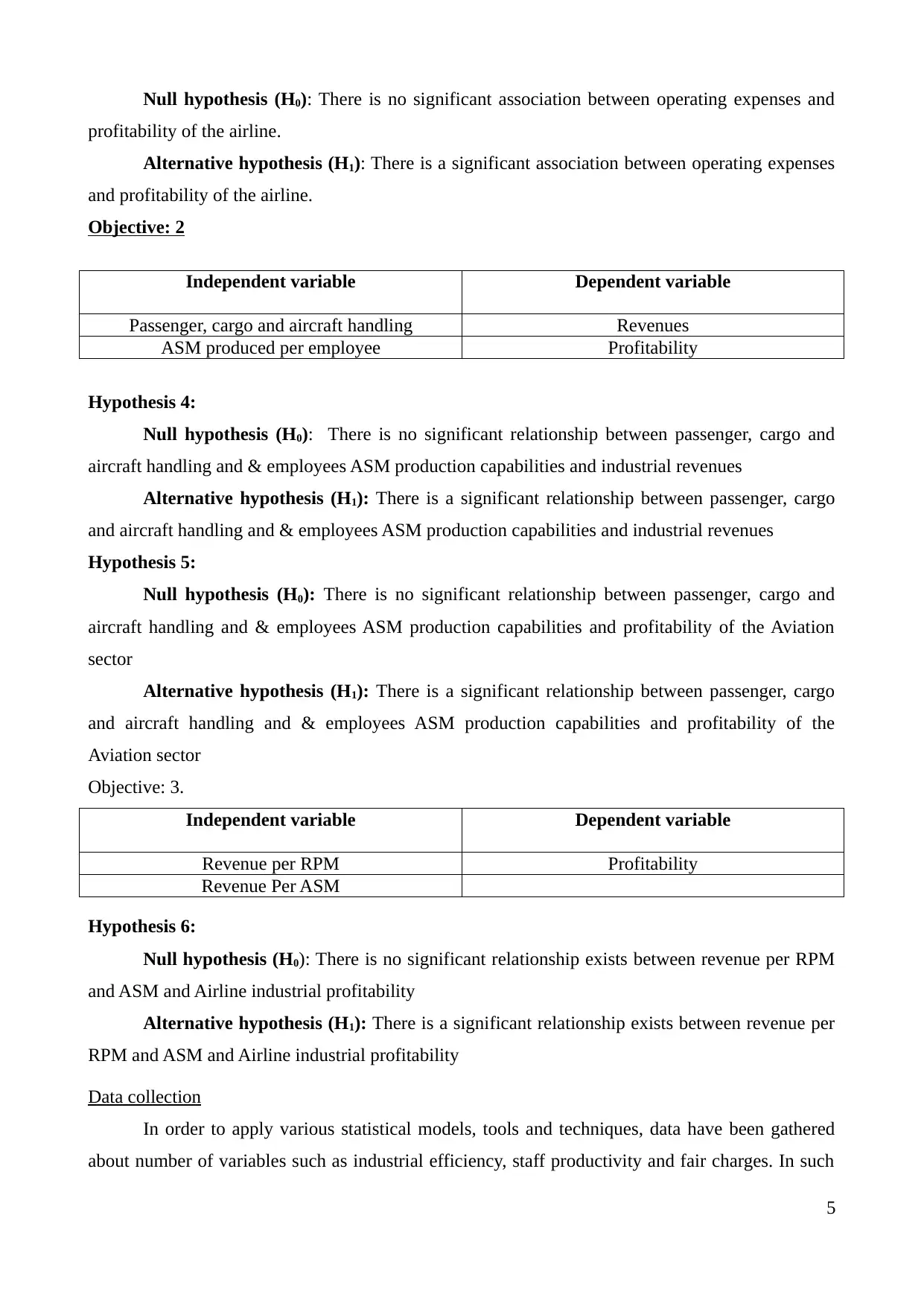
Null hypothesis (H0): There is no significant association between operating expenses and
profitability of the airline.
Alternative hypothesis (H1): There is a significant association between operating expenses
and profitability of the airline.
Objective: 2
Independent variable Dependent variable
Passenger, cargo and aircraft handling Revenues
ASM produced per employee Profitability
Hypothesis 4:
Null hypothesis (H0): There is no significant relationship between passenger, cargo and
aircraft handling and & employees ASM production capabilities and industrial revenues
Alternative hypothesis (H1): There is a significant relationship between passenger, cargo
and aircraft handling and & employees ASM production capabilities and industrial revenues
Hypothesis 5:
Null hypothesis (H0): There is no significant relationship between passenger, cargo and
aircraft handling and & employees ASM production capabilities and profitability of the Aviation
sector
Alternative hypothesis (H1): There is a significant relationship between passenger, cargo
and aircraft handling and & employees ASM production capabilities and profitability of the
Aviation sector
Objective: 3.
Independent variable Dependent variable
Revenue per RPM Profitability
Revenue Per ASM
Hypothesis 6:
Null hypothesis (H0): There is no significant relationship exists between revenue per RPM
and ASM and Airline industrial profitability
Alternative hypothesis (H1): There is a significant relationship exists between revenue per
RPM and ASM and Airline industrial profitability
Data collection
In order to apply various statistical models, tools and techniques, data have been gathered
about number of variables such as industrial efficiency, staff productivity and fair charges. In such
5
profitability of the airline.
Alternative hypothesis (H1): There is a significant association between operating expenses
and profitability of the airline.
Objective: 2
Independent variable Dependent variable
Passenger, cargo and aircraft handling Revenues
ASM produced per employee Profitability
Hypothesis 4:
Null hypothesis (H0): There is no significant relationship between passenger, cargo and
aircraft handling and & employees ASM production capabilities and industrial revenues
Alternative hypothesis (H1): There is a significant relationship between passenger, cargo
and aircraft handling and & employees ASM production capabilities and industrial revenues
Hypothesis 5:
Null hypothesis (H0): There is no significant relationship between passenger, cargo and
aircraft handling and & employees ASM production capabilities and profitability of the Aviation
sector
Alternative hypothesis (H1): There is a significant relationship between passenger, cargo
and aircraft handling and & employees ASM production capabilities and profitability of the
Aviation sector
Objective: 3.
Independent variable Dependent variable
Revenue per RPM Profitability
Revenue Per ASM
Hypothesis 6:
Null hypothesis (H0): There is no significant relationship exists between revenue per RPM
and ASM and Airline industrial profitability
Alternative hypothesis (H1): There is a significant relationship exists between revenue per
RPM and ASM and Airline industrial profitability
Data collection
In order to apply various statistical models, tools and techniques, data have been gathered
about number of variables such as industrial efficiency, staff productivity and fair charges. In such
5
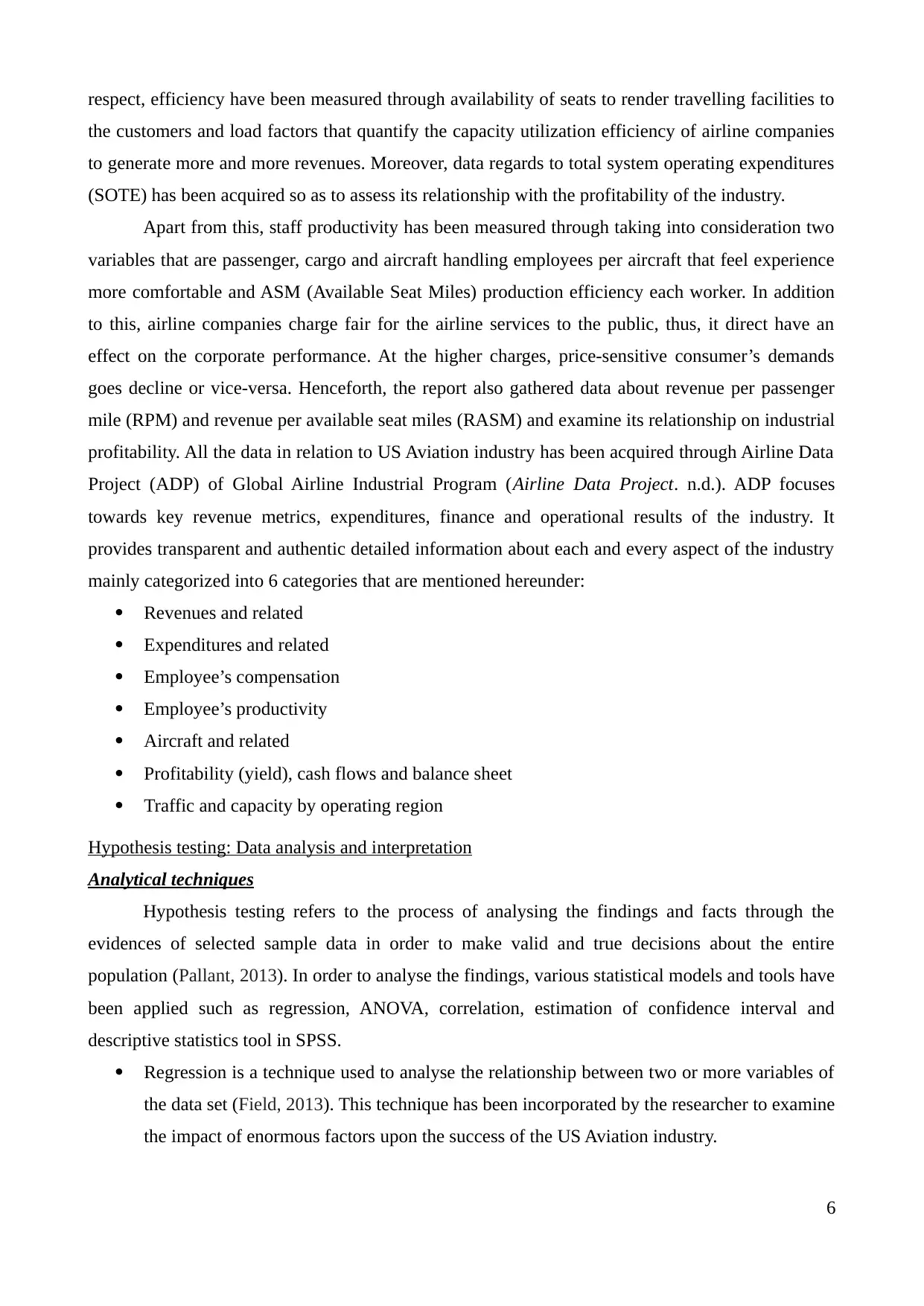
respect, efficiency have been measured through availability of seats to render travelling facilities to
the customers and load factors that quantify the capacity utilization efficiency of airline companies
to generate more and more revenues. Moreover, data regards to total system operating expenditures
(SOTE) has been acquired so as to assess its relationship with the profitability of the industry.
Apart from this, staff productivity has been measured through taking into consideration two
variables that are passenger, cargo and aircraft handling employees per aircraft that feel experience
more comfortable and ASM (Available Seat Miles) production efficiency each worker. In addition
to this, airline companies charge fair for the airline services to the public, thus, it direct have an
effect on the corporate performance. At the higher charges, price-sensitive consumer’s demands
goes decline or vice-versa. Henceforth, the report also gathered data about revenue per passenger
mile (RPM) and revenue per available seat miles (RASM) and examine its relationship on industrial
profitability. All the data in relation to US Aviation industry has been acquired through Airline Data
Project (ADP) of Global Airline Industrial Program (Airline Data Project. n.d.). ADP focuses
towards key revenue metrics, expenditures, finance and operational results of the industry. It
provides transparent and authentic detailed information about each and every aspect of the industry
mainly categorized into 6 categories that are mentioned hereunder:
Revenues and related
Expenditures and related
Employee’s compensation
Employee’s productivity
Aircraft and related
Profitability (yield), cash flows and balance sheet
Traffic and capacity by operating region
Hypothesis testing: Data analysis and interpretation
Analytical techniques
Hypothesis testing refers to the process of analysing the findings and facts through the
evidences of selected sample data in order to make valid and true decisions about the entire
population (Pallant, 2013). In order to analyse the findings, various statistical models and tools have
been applied such as regression, ANOVA, correlation, estimation of confidence interval and
descriptive statistics tool in SPSS.
Regression is a technique used to analyse the relationship between two or more variables of
the data set (Field, 2013). This technique has been incorporated by the researcher to examine
the impact of enormous factors upon the success of the US Aviation industry.
6
the customers and load factors that quantify the capacity utilization efficiency of airline companies
to generate more and more revenues. Moreover, data regards to total system operating expenditures
(SOTE) has been acquired so as to assess its relationship with the profitability of the industry.
Apart from this, staff productivity has been measured through taking into consideration two
variables that are passenger, cargo and aircraft handling employees per aircraft that feel experience
more comfortable and ASM (Available Seat Miles) production efficiency each worker. In addition
to this, airline companies charge fair for the airline services to the public, thus, it direct have an
effect on the corporate performance. At the higher charges, price-sensitive consumer’s demands
goes decline or vice-versa. Henceforth, the report also gathered data about revenue per passenger
mile (RPM) and revenue per available seat miles (RASM) and examine its relationship on industrial
profitability. All the data in relation to US Aviation industry has been acquired through Airline Data
Project (ADP) of Global Airline Industrial Program (Airline Data Project. n.d.). ADP focuses
towards key revenue metrics, expenditures, finance and operational results of the industry. It
provides transparent and authentic detailed information about each and every aspect of the industry
mainly categorized into 6 categories that are mentioned hereunder:
Revenues and related
Expenditures and related
Employee’s compensation
Employee’s productivity
Aircraft and related
Profitability (yield), cash flows and balance sheet
Traffic and capacity by operating region
Hypothesis testing: Data analysis and interpretation
Analytical techniques
Hypothesis testing refers to the process of analysing the findings and facts through the
evidences of selected sample data in order to make valid and true decisions about the entire
population (Pallant, 2013). In order to analyse the findings, various statistical models and tools have
been applied such as regression, ANOVA, correlation, estimation of confidence interval and
descriptive statistics tool in SPSS.
Regression is a technique used to analyse the relationship between two or more variables of
the data set (Field, 2013). This technique has been incorporated by the researcher to examine
the impact of enormous factors upon the success of the US Aviation industry.
6
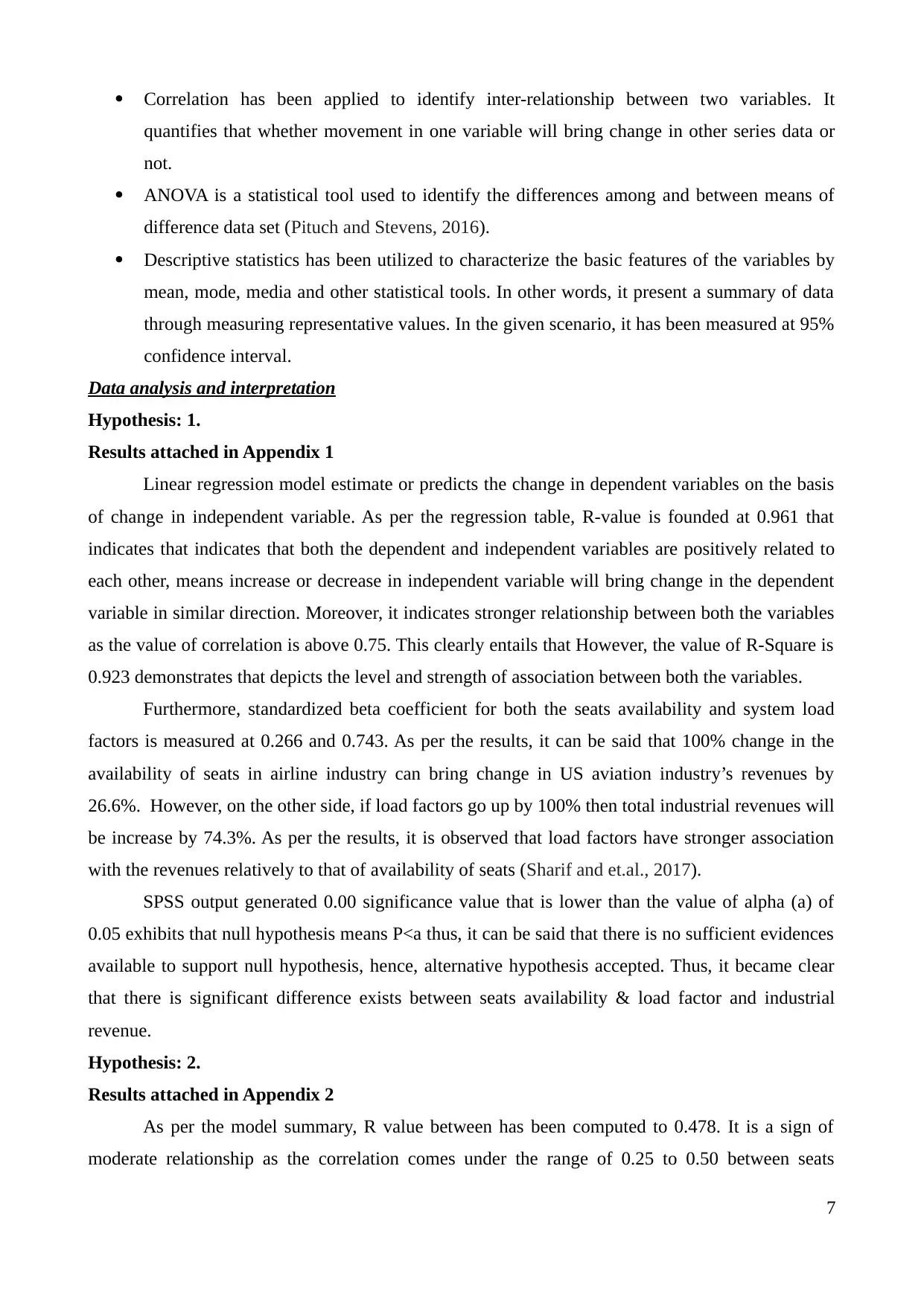
Correlation has been applied to identify inter-relationship between two variables. It
quantifies that whether movement in one variable will bring change in other series data or
not.
ANOVA is a statistical tool used to identify the differences among and between means of
difference data set (Pituch and Stevens, 2016).
Descriptive statistics has been utilized to characterize the basic features of the variables by
mean, mode, media and other statistical tools. In other words, it present a summary of data
through measuring representative values. In the given scenario, it has been measured at 95%
confidence interval.
Data analysis and interpretation
Hypothesis: 1.
Results attached in Appendix 1
Linear regression model estimate or predicts the change in dependent variables on the basis
of change in independent variable. As per the regression table, R-value is founded at 0.961 that
indicates that indicates that both the dependent and independent variables are positively related to
each other, means increase or decrease in independent variable will bring change in the dependent
variable in similar direction. Moreover, it indicates stronger relationship between both the variables
as the value of correlation is above 0.75. This clearly entails that However, the value of R-Square is
0.923 demonstrates that depicts the level and strength of association between both the variables.
Furthermore, standardized beta coefficient for both the seats availability and system load
factors is measured at 0.266 and 0.743. As per the results, it can be said that 100% change in the
availability of seats in airline industry can bring change in US aviation industry’s revenues by
26.6%. However, on the other side, if load factors go up by 100% then total industrial revenues will
be increase by 74.3%. As per the results, it is observed that load factors have stronger association
with the revenues relatively to that of availability of seats (Sharif and et.al., 2017).
SPSS output generated 0.00 significance value that is lower than the value of alpha (a) of
0.05 exhibits that null hypothesis means P<a thus, it can be said that there is no sufficient evidences
available to support null hypothesis, hence, alternative hypothesis accepted. Thus, it became clear
that there is significant difference exists between seats availability & load factor and industrial
revenue.
Hypothesis: 2.
Results attached in Appendix 2
As per the model summary, R value between has been computed to 0.478. It is a sign of
moderate relationship as the correlation comes under the range of 0.25 to 0.50 between seats
7
quantifies that whether movement in one variable will bring change in other series data or
not.
ANOVA is a statistical tool used to identify the differences among and between means of
difference data set (Pituch and Stevens, 2016).
Descriptive statistics has been utilized to characterize the basic features of the variables by
mean, mode, media and other statistical tools. In other words, it present a summary of data
through measuring representative values. In the given scenario, it has been measured at 95%
confidence interval.
Data analysis and interpretation
Hypothesis: 1.
Results attached in Appendix 1
Linear regression model estimate or predicts the change in dependent variables on the basis
of change in independent variable. As per the regression table, R-value is founded at 0.961 that
indicates that indicates that both the dependent and independent variables are positively related to
each other, means increase or decrease in independent variable will bring change in the dependent
variable in similar direction. Moreover, it indicates stronger relationship between both the variables
as the value of correlation is above 0.75. This clearly entails that However, the value of R-Square is
0.923 demonstrates that depicts the level and strength of association between both the variables.
Furthermore, standardized beta coefficient for both the seats availability and system load
factors is measured at 0.266 and 0.743. As per the results, it can be said that 100% change in the
availability of seats in airline industry can bring change in US aviation industry’s revenues by
26.6%. However, on the other side, if load factors go up by 100% then total industrial revenues will
be increase by 74.3%. As per the results, it is observed that load factors have stronger association
with the revenues relatively to that of availability of seats (Sharif and et.al., 2017).
SPSS output generated 0.00 significance value that is lower than the value of alpha (a) of
0.05 exhibits that null hypothesis means P<a thus, it can be said that there is no sufficient evidences
available to support null hypothesis, hence, alternative hypothesis accepted. Thus, it became clear
that there is significant difference exists between seats availability & load factor and industrial
revenue.
Hypothesis: 2.
Results attached in Appendix 2
As per the model summary, R value between has been computed to 0.478. It is a sign of
moderate relationship as the correlation comes under the range of 0.25 to 0.50 between seats
7
Paraphrase This Document
Need a fresh take? Get an instant paraphrase of this document with our AI Paraphraser
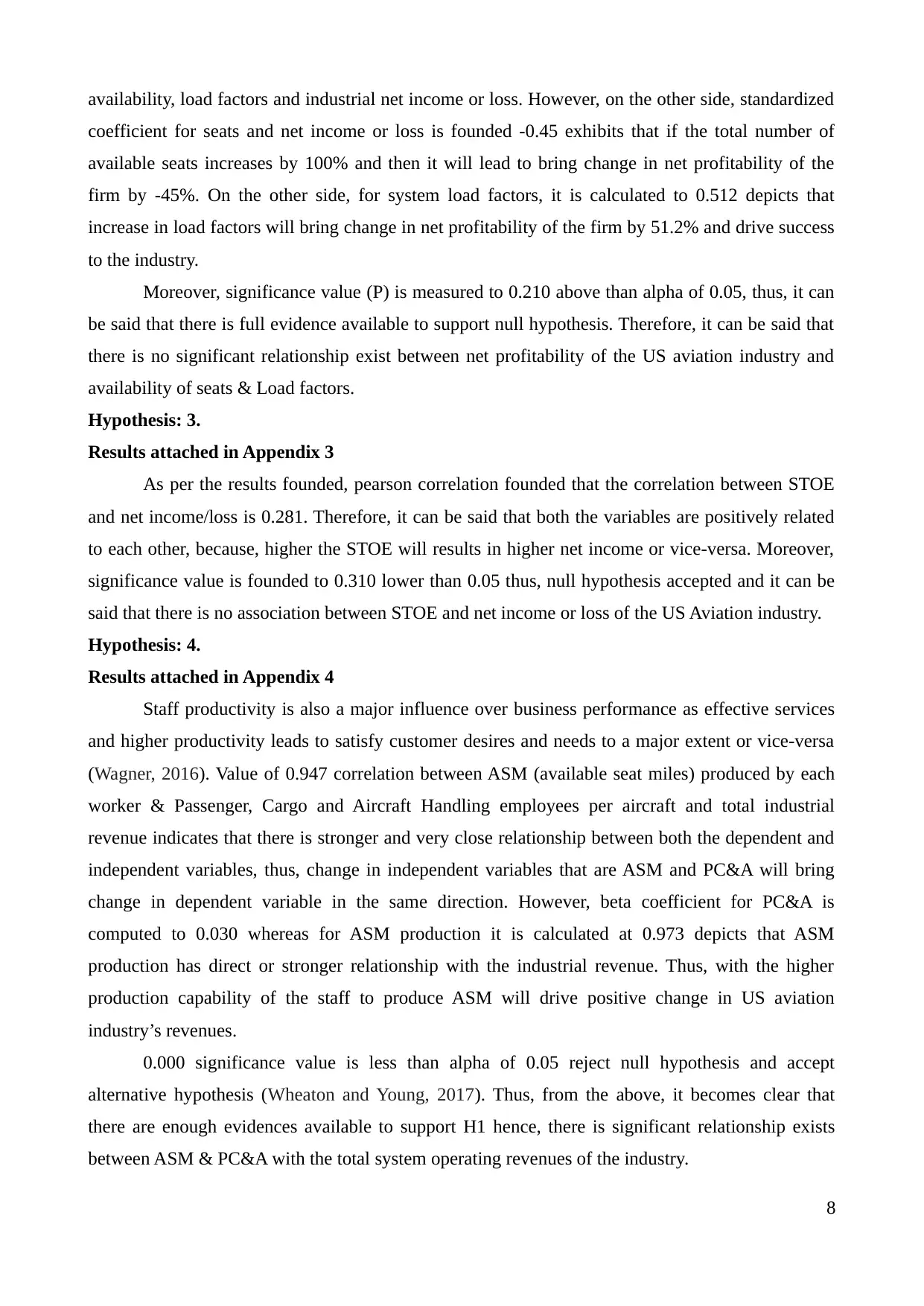
availability, load factors and industrial net income or loss. However, on the other side, standardized
coefficient for seats and net income or loss is founded -0.45 exhibits that if the total number of
available seats increases by 100% and then it will lead to bring change in net profitability of the
firm by -45%. On the other side, for system load factors, it is calculated to 0.512 depicts that
increase in load factors will bring change in net profitability of the firm by 51.2% and drive success
to the industry.
Moreover, significance value (P) is measured to 0.210 above than alpha of 0.05, thus, it can
be said that there is full evidence available to support null hypothesis. Therefore, it can be said that
there is no significant relationship exist between net profitability of the US aviation industry and
availability of seats & Load factors.
Hypothesis: 3.
Results attached in Appendix 3
As per the results founded, pearson correlation founded that the correlation between STOE
and net income/loss is 0.281. Therefore, it can be said that both the variables are positively related
to each other, because, higher the STOE will results in higher net income or vice-versa. Moreover,
significance value is founded to 0.310 lower than 0.05 thus, null hypothesis accepted and it can be
said that there is no association between STOE and net income or loss of the US Aviation industry.
Hypothesis: 4.
Results attached in Appendix 4
Staff productivity is also a major influence over business performance as effective services
and higher productivity leads to satisfy customer desires and needs to a major extent or vice-versa
(Wagner, 2016). Value of 0.947 correlation between ASM (available seat miles) produced by each
worker & Passenger, Cargo and Aircraft Handling employees per aircraft and total industrial
revenue indicates that there is stronger and very close relationship between both the dependent and
independent variables, thus, change in independent variables that are ASM and PC&A will bring
change in dependent variable in the same direction. However, beta coefficient for PC&A is
computed to 0.030 whereas for ASM production it is calculated at 0.973 depicts that ASM
production has direct or stronger relationship with the industrial revenue. Thus, with the higher
production capability of the staff to produce ASM will drive positive change in US aviation
industry’s revenues.
0.000 significance value is less than alpha of 0.05 reject null hypothesis and accept
alternative hypothesis (Wheaton and Young, 2017). Thus, from the above, it becomes clear that
there are enough evidences available to support H1 hence, there is significant relationship exists
between ASM & PC&A with the total system operating revenues of the industry.
8
coefficient for seats and net income or loss is founded -0.45 exhibits that if the total number of
available seats increases by 100% and then it will lead to bring change in net profitability of the
firm by -45%. On the other side, for system load factors, it is calculated to 0.512 depicts that
increase in load factors will bring change in net profitability of the firm by 51.2% and drive success
to the industry.
Moreover, significance value (P) is measured to 0.210 above than alpha of 0.05, thus, it can
be said that there is full evidence available to support null hypothesis. Therefore, it can be said that
there is no significant relationship exist between net profitability of the US aviation industry and
availability of seats & Load factors.
Hypothesis: 3.
Results attached in Appendix 3
As per the results founded, pearson correlation founded that the correlation between STOE
and net income/loss is 0.281. Therefore, it can be said that both the variables are positively related
to each other, because, higher the STOE will results in higher net income or vice-versa. Moreover,
significance value is founded to 0.310 lower than 0.05 thus, null hypothesis accepted and it can be
said that there is no association between STOE and net income or loss of the US Aviation industry.
Hypothesis: 4.
Results attached in Appendix 4
Staff productivity is also a major influence over business performance as effective services
and higher productivity leads to satisfy customer desires and needs to a major extent or vice-versa
(Wagner, 2016). Value of 0.947 correlation between ASM (available seat miles) produced by each
worker & Passenger, Cargo and Aircraft Handling employees per aircraft and total industrial
revenue indicates that there is stronger and very close relationship between both the dependent and
independent variables, thus, change in independent variables that are ASM and PC&A will bring
change in dependent variable in the same direction. However, beta coefficient for PC&A is
computed to 0.030 whereas for ASM production it is calculated at 0.973 depicts that ASM
production has direct or stronger relationship with the industrial revenue. Thus, with the higher
production capability of the staff to produce ASM will drive positive change in US aviation
industry’s revenues.
0.000 significance value is less than alpha of 0.05 reject null hypothesis and accept
alternative hypothesis (Wheaton and Young, 2017). Thus, from the above, it becomes clear that
there are enough evidences available to support H1 hence, there is significant relationship exists
between ASM & PC&A with the total system operating revenues of the industry.
8
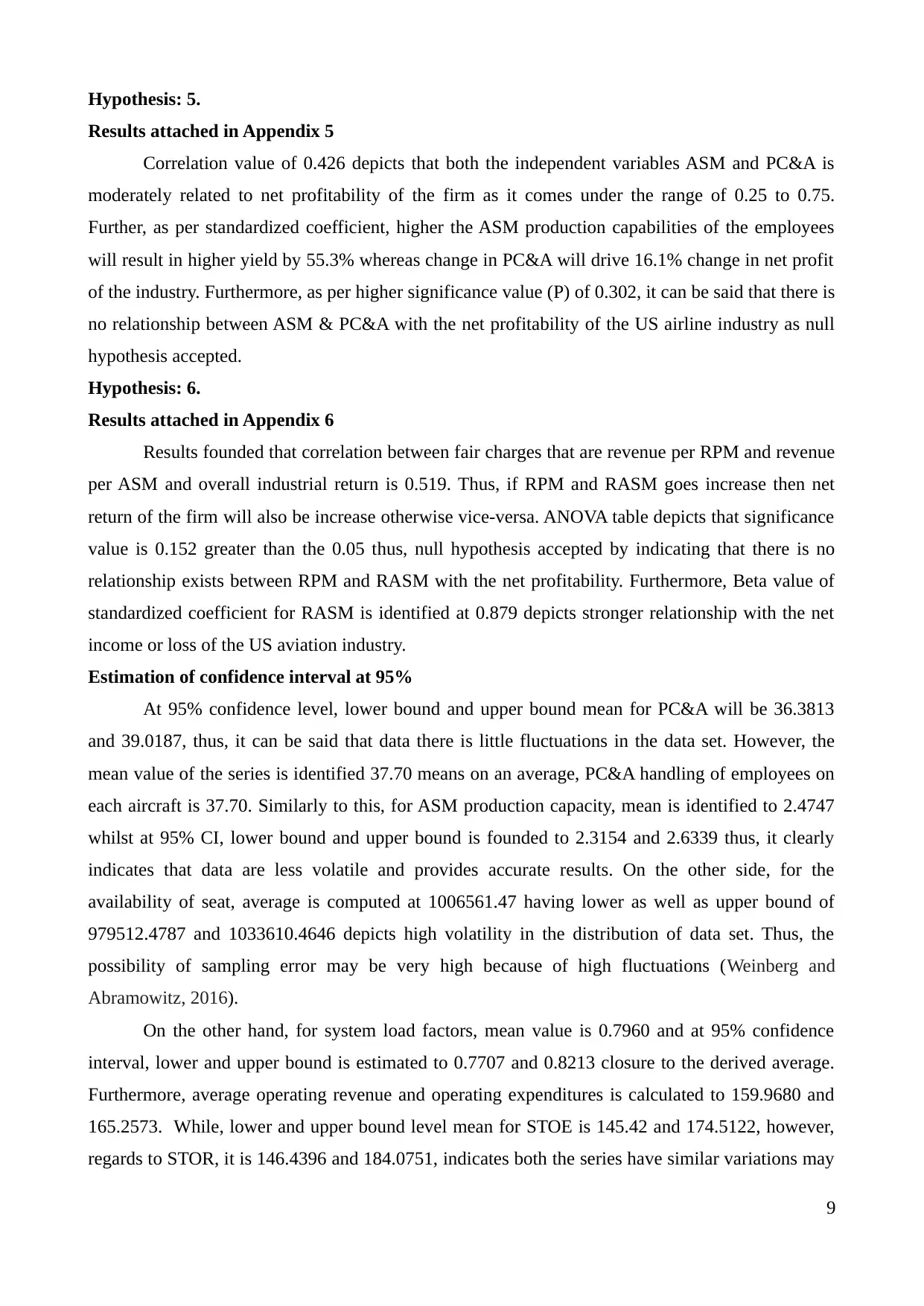
Hypothesis: 5.
Results attached in Appendix 5
Correlation value of 0.426 depicts that both the independent variables ASM and PC&A is
moderately related to net profitability of the firm as it comes under the range of 0.25 to 0.75.
Further, as per standardized coefficient, higher the ASM production capabilities of the employees
will result in higher yield by 55.3% whereas change in PC&A will drive 16.1% change in net profit
of the industry. Furthermore, as per higher significance value (P) of 0.302, it can be said that there is
no relationship between ASM & PC&A with the net profitability of the US airline industry as null
hypothesis accepted.
Hypothesis: 6.
Results attached in Appendix 6
Results founded that correlation between fair charges that are revenue per RPM and revenue
per ASM and overall industrial return is 0.519. Thus, if RPM and RASM goes increase then net
return of the firm will also be increase otherwise vice-versa. ANOVA table depicts that significance
value is 0.152 greater than the 0.05 thus, null hypothesis accepted by indicating that there is no
relationship exists between RPM and RASM with the net profitability. Furthermore, Beta value of
standardized coefficient for RASM is identified at 0.879 depicts stronger relationship with the net
income or loss of the US aviation industry.
Estimation of confidence interval at 95%
At 95% confidence level, lower bound and upper bound mean for PC&A will be 36.3813
and 39.0187, thus, it can be said that data there is little fluctuations in the data set. However, the
mean value of the series is identified 37.70 means on an average, PC&A handling of employees on
each aircraft is 37.70. Similarly to this, for ASM production capacity, mean is identified to 2.4747
whilst at 95% CI, lower bound and upper bound is founded to 2.3154 and 2.6339 thus, it clearly
indicates that data are less volatile and provides accurate results. On the other side, for the
availability of seat, average is computed at 1006561.47 having lower as well as upper bound of
979512.4787 and 1033610.4646 depicts high volatility in the distribution of data set. Thus, the
possibility of sampling error may be very high because of high fluctuations (Weinberg and
Abramowitz, 2016).
On the other hand, for system load factors, mean value is 0.7960 and at 95% confidence
interval, lower and upper bound is estimated to 0.7707 and 0.8213 closure to the derived average.
Furthermore, average operating revenue and operating expenditures is calculated to 159.9680 and
165.2573. While, lower and upper bound level mean for STOE is 145.42 and 174.5122, however,
regards to STOR, it is 146.4396 and 184.0751, indicates both the series have similar variations may
9
Results attached in Appendix 5
Correlation value of 0.426 depicts that both the independent variables ASM and PC&A is
moderately related to net profitability of the firm as it comes under the range of 0.25 to 0.75.
Further, as per standardized coefficient, higher the ASM production capabilities of the employees
will result in higher yield by 55.3% whereas change in PC&A will drive 16.1% change in net profit
of the industry. Furthermore, as per higher significance value (P) of 0.302, it can be said that there is
no relationship between ASM & PC&A with the net profitability of the US airline industry as null
hypothesis accepted.
Hypothesis: 6.
Results attached in Appendix 6
Results founded that correlation between fair charges that are revenue per RPM and revenue
per ASM and overall industrial return is 0.519. Thus, if RPM and RASM goes increase then net
return of the firm will also be increase otherwise vice-versa. ANOVA table depicts that significance
value is 0.152 greater than the 0.05 thus, null hypothesis accepted by indicating that there is no
relationship exists between RPM and RASM with the net profitability. Furthermore, Beta value of
standardized coefficient for RASM is identified at 0.879 depicts stronger relationship with the net
income or loss of the US aviation industry.
Estimation of confidence interval at 95%
At 95% confidence level, lower bound and upper bound mean for PC&A will be 36.3813
and 39.0187, thus, it can be said that data there is little fluctuations in the data set. However, the
mean value of the series is identified 37.70 means on an average, PC&A handling of employees on
each aircraft is 37.70. Similarly to this, for ASM production capacity, mean is identified to 2.4747
whilst at 95% CI, lower bound and upper bound is founded to 2.3154 and 2.6339 thus, it clearly
indicates that data are less volatile and provides accurate results. On the other side, for the
availability of seat, average is computed at 1006561.47 having lower as well as upper bound of
979512.4787 and 1033610.4646 depicts high volatility in the distribution of data set. Thus, the
possibility of sampling error may be very high because of high fluctuations (Weinberg and
Abramowitz, 2016).
On the other hand, for system load factors, mean value is 0.7960 and at 95% confidence
interval, lower and upper bound is estimated to 0.7707 and 0.8213 closure to the derived average.
Furthermore, average operating revenue and operating expenditures is calculated to 159.9680 and
165.2573. While, lower and upper bound level mean for STOE is 145.42 and 174.5122, however,
regards to STOR, it is 146.4396 and 184.0751, indicates both the series have similar variations may
9
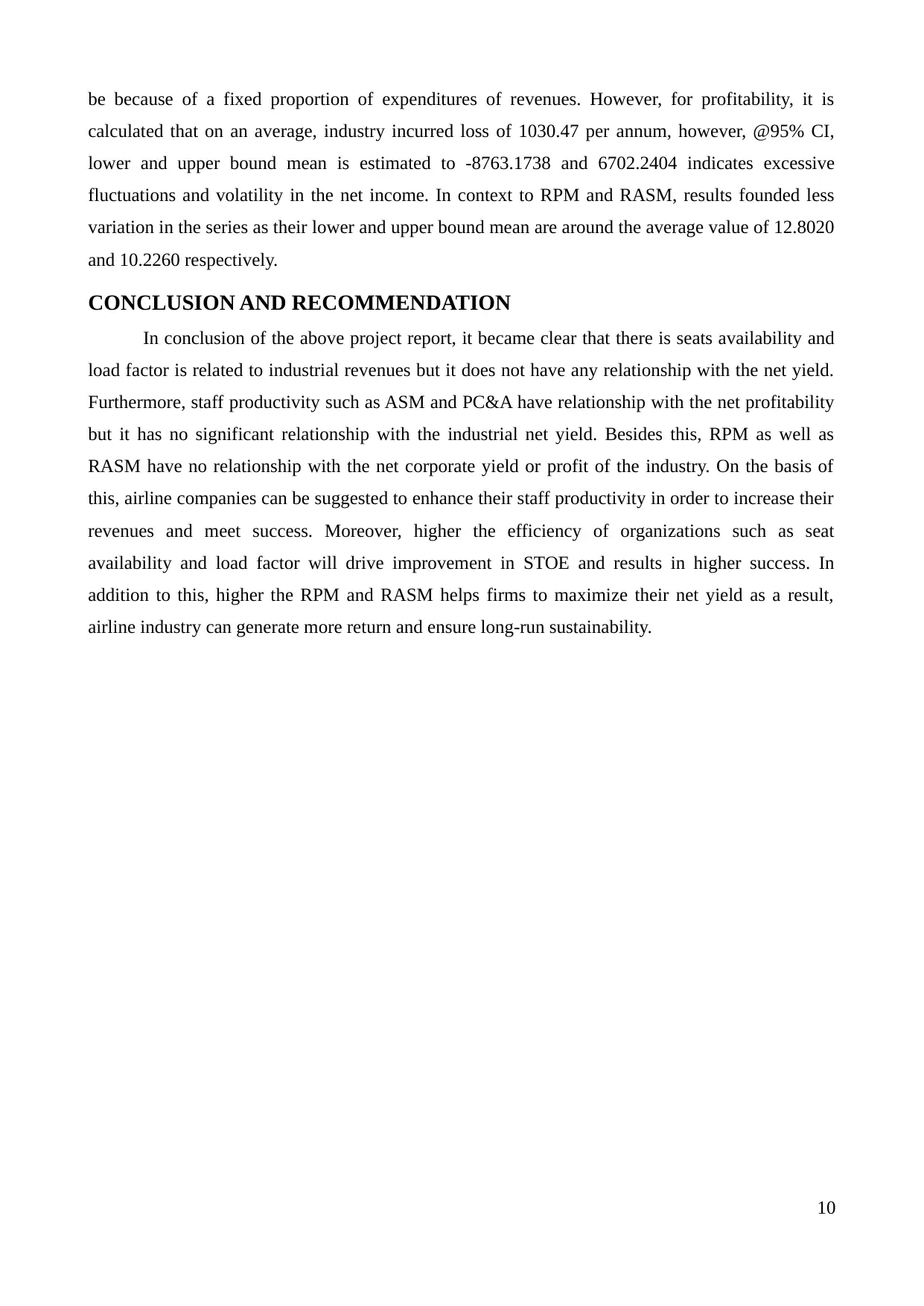
be because of a fixed proportion of expenditures of revenues. However, for profitability, it is
calculated that on an average, industry incurred loss of 1030.47 per annum, however, @95% CI,
lower and upper bound mean is estimated to -8763.1738 and 6702.2404 indicates excessive
fluctuations and volatility in the net income. In context to RPM and RASM, results founded less
variation in the series as their lower and upper bound mean are around the average value of 12.8020
and 10.2260 respectively.
CONCLUSION AND RECOMMENDATION
In conclusion of the above project report, it became clear that there is seats availability and
load factor is related to industrial revenues but it does not have any relationship with the net yield.
Furthermore, staff productivity such as ASM and PC&A have relationship with the net profitability
but it has no significant relationship with the industrial net yield. Besides this, RPM as well as
RASM have no relationship with the net corporate yield or profit of the industry. On the basis of
this, airline companies can be suggested to enhance their staff productivity in order to increase their
revenues and meet success. Moreover, higher the efficiency of organizations such as seat
availability and load factor will drive improvement in STOE and results in higher success. In
addition to this, higher the RPM and RASM helps firms to maximize their net yield as a result,
airline industry can generate more return and ensure long-run sustainability.
10
calculated that on an average, industry incurred loss of 1030.47 per annum, however, @95% CI,
lower and upper bound mean is estimated to -8763.1738 and 6702.2404 indicates excessive
fluctuations and volatility in the net income. In context to RPM and RASM, results founded less
variation in the series as their lower and upper bound mean are around the average value of 12.8020
and 10.2260 respectively.
CONCLUSION AND RECOMMENDATION
In conclusion of the above project report, it became clear that there is seats availability and
load factor is related to industrial revenues but it does not have any relationship with the net yield.
Furthermore, staff productivity such as ASM and PC&A have relationship with the net profitability
but it has no significant relationship with the industrial net yield. Besides this, RPM as well as
RASM have no relationship with the net corporate yield or profit of the industry. On the basis of
this, airline companies can be suggested to enhance their staff productivity in order to increase their
revenues and meet success. Moreover, higher the efficiency of organizations such as seat
availability and load factor will drive improvement in STOE and results in higher success. In
addition to this, higher the RPM and RASM helps firms to maximize their net yield as a result,
airline industry can generate more return and ensure long-run sustainability.
10
Secure Best Marks with AI Grader
Need help grading? Try our AI Grader for instant feedback on your assignments.
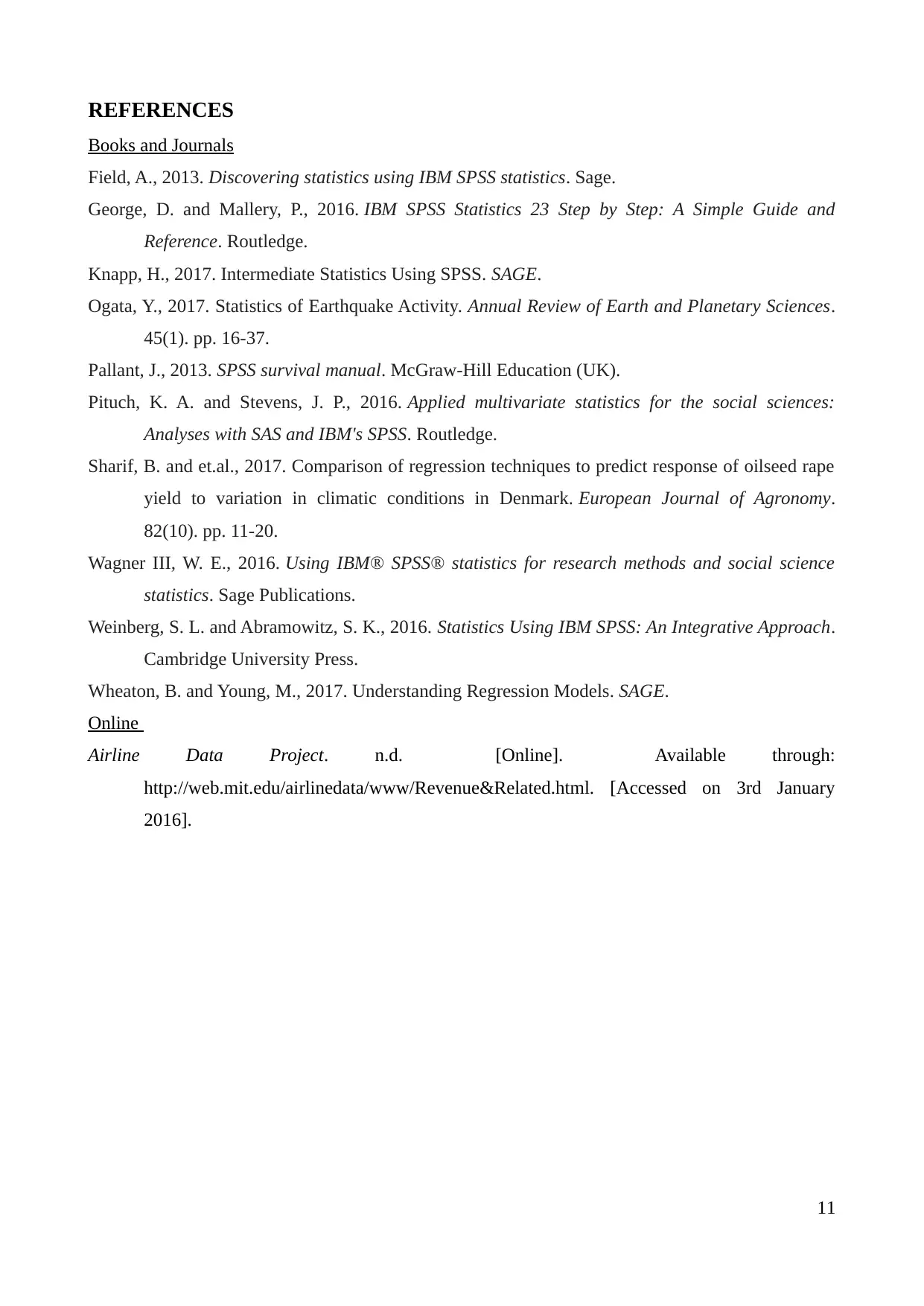
REFERENCES
Books and Journals
Field, A., 2013. Discovering statistics using IBM SPSS statistics. Sage.
George, D. and Mallery, P., 2016. IBM SPSS Statistics 23 Step by Step: A Simple Guide and
Reference. Routledge.
Knapp, H., 2017. Intermediate Statistics Using SPSS. SAGE.
Ogata, Y., 2017. Statistics of Earthquake Activity. Annual Review of Earth and Planetary Sciences.
45(1). pp. 16-37.
Pallant, J., 2013. SPSS survival manual. McGraw-Hill Education (UK).
Pituch, K. A. and Stevens, J. P., 2016. Applied multivariate statistics for the social sciences:
Analyses with SAS and IBM's SPSS. Routledge.
Sharif, B. and et.al., 2017. Comparison of regression techniques to predict response of oilseed rape
yield to variation in climatic conditions in Denmark. European Journal of Agronomy.
82(10). pp. 11-20.
Wagner III, W. E., 2016. Using IBM® SPSS® statistics for research methods and social science
statistics. Sage Publications.
Weinberg, S. L. and Abramowitz, S. K., 2016. Statistics Using IBM SPSS: An Integrative Approach.
Cambridge University Press.
Wheaton, B. and Young, M., 2017. Understanding Regression Models. SAGE.
Online
Airline Data Project. n.d. [Online]. Available through:
http://web.mit.edu/airlinedata/www/Revenue&Related.html. [Accessed on 3rd January
2016].
11
Books and Journals
Field, A., 2013. Discovering statistics using IBM SPSS statistics. Sage.
George, D. and Mallery, P., 2016. IBM SPSS Statistics 23 Step by Step: A Simple Guide and
Reference. Routledge.
Knapp, H., 2017. Intermediate Statistics Using SPSS. SAGE.
Ogata, Y., 2017. Statistics of Earthquake Activity. Annual Review of Earth and Planetary Sciences.
45(1). pp. 16-37.
Pallant, J., 2013. SPSS survival manual. McGraw-Hill Education (UK).
Pituch, K. A. and Stevens, J. P., 2016. Applied multivariate statistics for the social sciences:
Analyses with SAS and IBM's SPSS. Routledge.
Sharif, B. and et.al., 2017. Comparison of regression techniques to predict response of oilseed rape
yield to variation in climatic conditions in Denmark. European Journal of Agronomy.
82(10). pp. 11-20.
Wagner III, W. E., 2016. Using IBM® SPSS® statistics for research methods and social science
statistics. Sage Publications.
Weinberg, S. L. and Abramowitz, S. K., 2016. Statistics Using IBM SPSS: An Integrative Approach.
Cambridge University Press.
Wheaton, B. and Young, M., 2017. Understanding Regression Models. SAGE.
Online
Airline Data Project. n.d. [Online]. Available through:
http://web.mit.edu/airlinedata/www/Revenue&Related.html. [Accessed on 3rd January
2016].
11
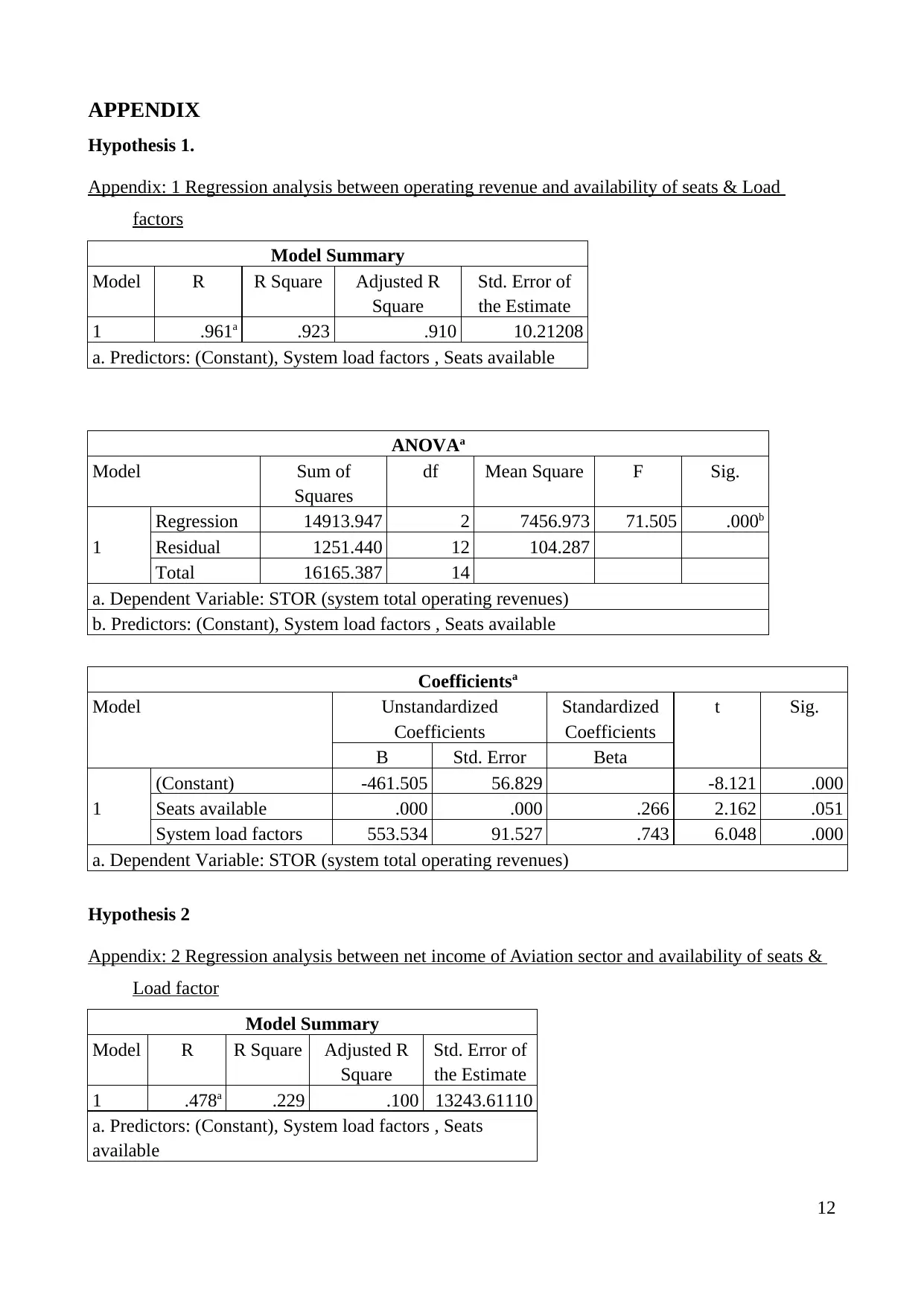
APPENDIX
Hypothesis 1.
Appendix: 1 Regression analysis between operating revenue and availability of seats & Load
factors
Model Summary
Model R R Square Adjusted R
Square
Std. Error of
the Estimate
1 .961a .923 .910 10.21208
a. Predictors: (Constant), System load factors , Seats available
ANOVAa
Model Sum of
Squares
df Mean Square F Sig.
1
Regression 14913.947 2 7456.973 71.505 .000b
Residual 1251.440 12 104.287
Total 16165.387 14
a. Dependent Variable: STOR (system total operating revenues)
b. Predictors: (Constant), System load factors , Seats available
Coefficientsa
Model Unstandardized
Coefficients
Standardized
Coefficients
t Sig.
B Std. Error Beta
1
(Constant) -461.505 56.829 -8.121 .000
Seats available .000 .000 .266 2.162 .051
System load factors 553.534 91.527 .743 6.048 .000
a. Dependent Variable: STOR (system total operating revenues)
Hypothesis 2
Appendix: 2 Regression analysis between net income of Aviation sector and availability of seats &
Load factor
Model Summary
Model R R Square Adjusted R
Square
Std. Error of
the Estimate
1 .478a .229 .100 13243.61110
a. Predictors: (Constant), System load factors , Seats
available
12
Hypothesis 1.
Appendix: 1 Regression analysis between operating revenue and availability of seats & Load
factors
Model Summary
Model R R Square Adjusted R
Square
Std. Error of
the Estimate
1 .961a .923 .910 10.21208
a. Predictors: (Constant), System load factors , Seats available
ANOVAa
Model Sum of
Squares
df Mean Square F Sig.
1
Regression 14913.947 2 7456.973 71.505 .000b
Residual 1251.440 12 104.287
Total 16165.387 14
a. Dependent Variable: STOR (system total operating revenues)
b. Predictors: (Constant), System load factors , Seats available
Coefficientsa
Model Unstandardized
Coefficients
Standardized
Coefficients
t Sig.
B Std. Error Beta
1
(Constant) -461.505 56.829 -8.121 .000
Seats available .000 .000 .266 2.162 .051
System load factors 553.534 91.527 .743 6.048 .000
a. Dependent Variable: STOR (system total operating revenues)
Hypothesis 2
Appendix: 2 Regression analysis between net income of Aviation sector and availability of seats &
Load factor
Model Summary
Model R R Square Adjusted R
Square
Std. Error of
the Estimate
1 .478a .229 .100 13243.61110
a. Predictors: (Constant), System load factors , Seats
available
12
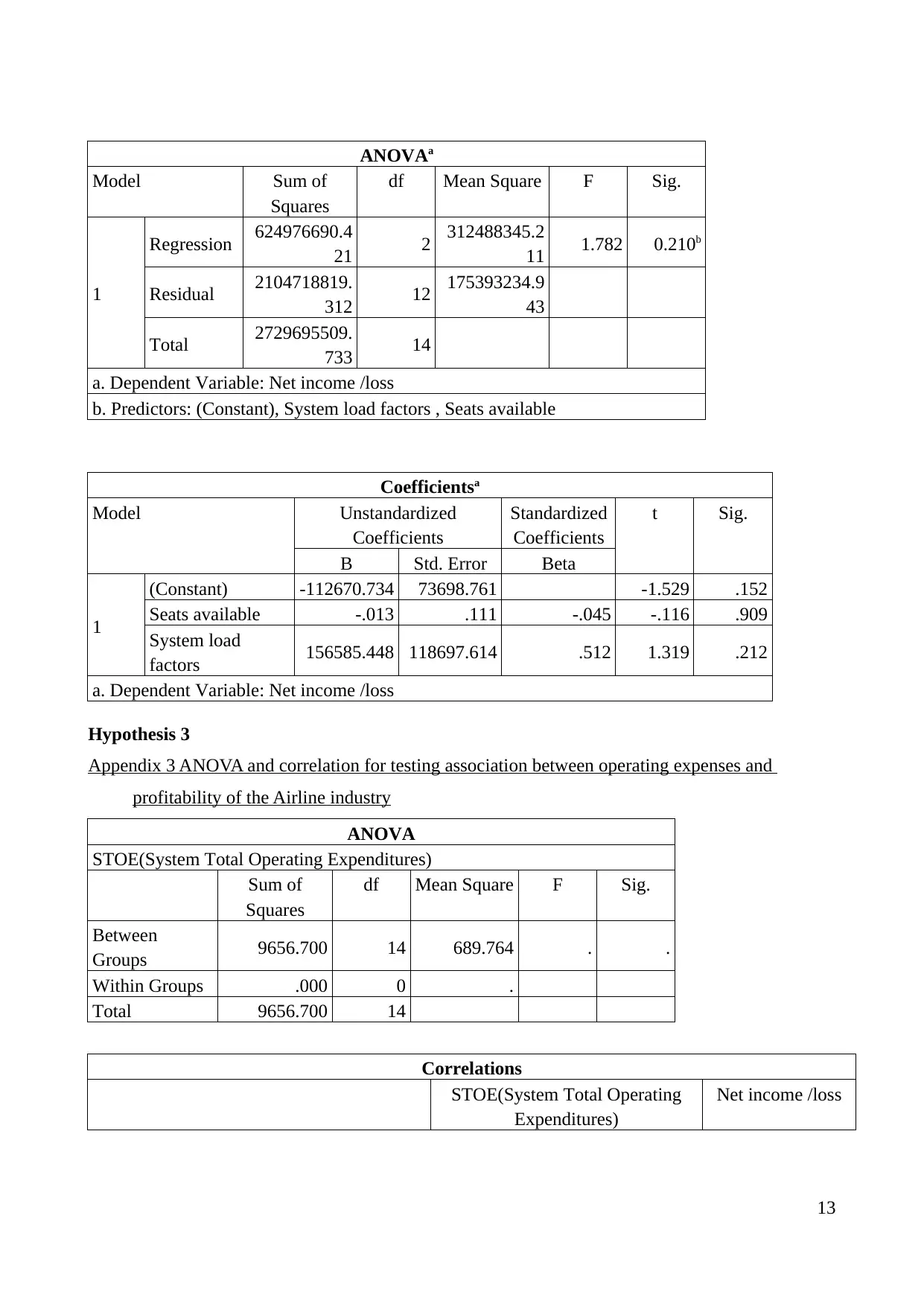
ANOVAa
Model Sum of
Squares
df Mean Square F Sig.
1
Regression 624976690.4
21 2 312488345.2
11 1.782 0.210b
Residual 2104718819.
312 12 175393234.9
43
Total 2729695509.
733 14
a. Dependent Variable: Net income /loss
b. Predictors: (Constant), System load factors , Seats available
Coefficientsa
Model Unstandardized
Coefficients
Standardized
Coefficients
t Sig.
B Std. Error Beta
1
(Constant) -112670.734 73698.761 -1.529 .152
Seats available -.013 .111 -.045 -.116 .909
System load
factors 156585.448 118697.614 .512 1.319 .212
a. Dependent Variable: Net income /loss
Hypothesis 3
Appendix 3 ANOVA and correlation for testing association between operating expenses and
profitability of the Airline industry
ANOVA
STOE(System Total Operating Expenditures)
Sum of
Squares
df Mean Square F Sig.
Between
Groups 9656.700 14 689.764 . .
Within Groups .000 0 .
Total 9656.700 14
Correlations
STOE(System Total Operating
Expenditures)
Net income /loss
13
Model Sum of
Squares
df Mean Square F Sig.
1
Regression 624976690.4
21 2 312488345.2
11 1.782 0.210b
Residual 2104718819.
312 12 175393234.9
43
Total 2729695509.
733 14
a. Dependent Variable: Net income /loss
b. Predictors: (Constant), System load factors , Seats available
Coefficientsa
Model Unstandardized
Coefficients
Standardized
Coefficients
t Sig.
B Std. Error Beta
1
(Constant) -112670.734 73698.761 -1.529 .152
Seats available -.013 .111 -.045 -.116 .909
System load
factors 156585.448 118697.614 .512 1.319 .212
a. Dependent Variable: Net income /loss
Hypothesis 3
Appendix 3 ANOVA and correlation for testing association between operating expenses and
profitability of the Airline industry
ANOVA
STOE(System Total Operating Expenditures)
Sum of
Squares
df Mean Square F Sig.
Between
Groups 9656.700 14 689.764 . .
Within Groups .000 0 .
Total 9656.700 14
Correlations
STOE(System Total Operating
Expenditures)
Net income /loss
13
Paraphrase This Document
Need a fresh take? Get an instant paraphrase of this document with our AI Paraphraser
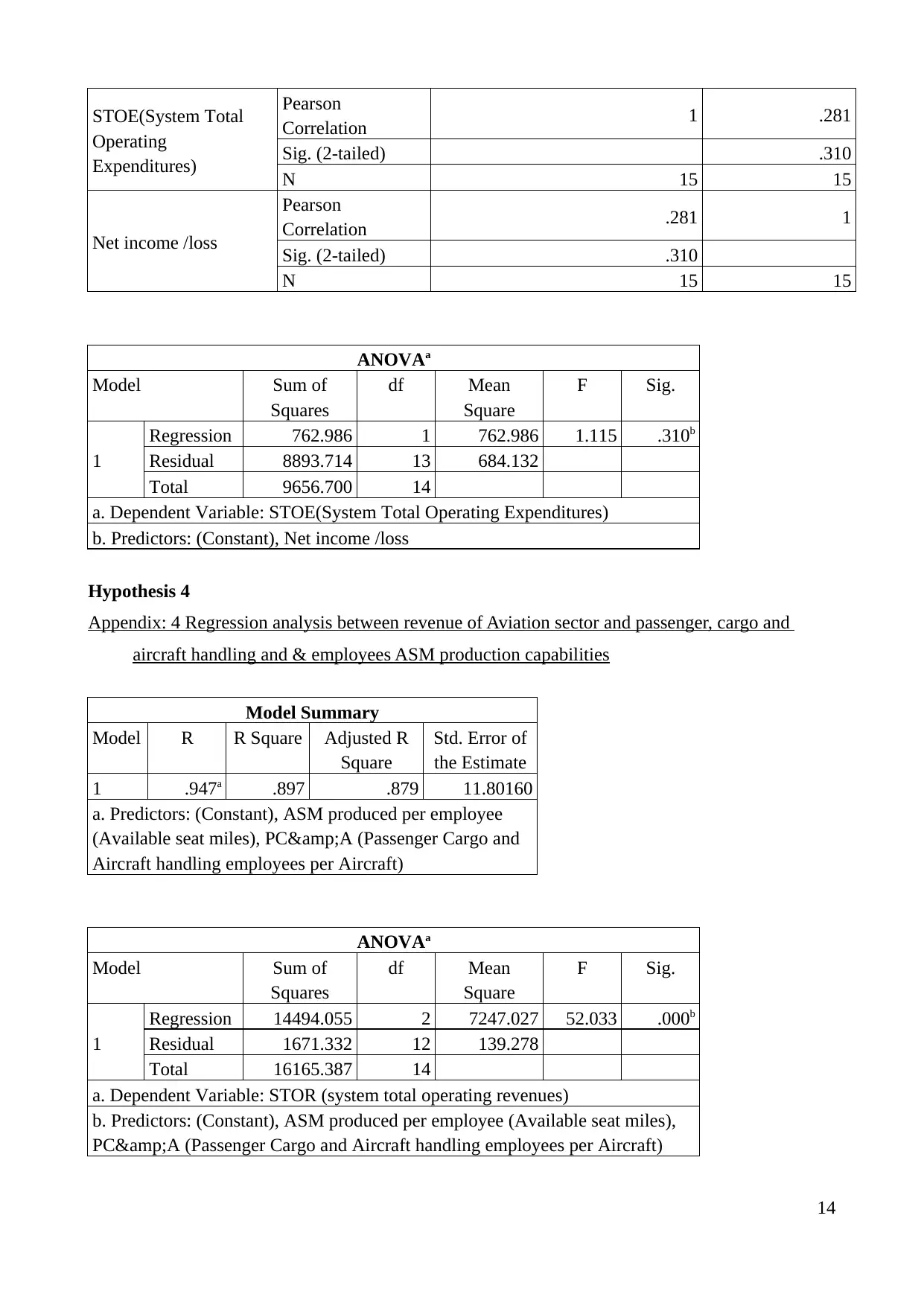
STOE(System Total
Operating
Expenditures)
Pearson
Correlation 1 .281
Sig. (2-tailed) .310
N 15 15
Net income /loss
Pearson
Correlation .281 1
Sig. (2-tailed) .310
N 15 15
ANOVAa
Model Sum of
Squares
df Mean
Square
F Sig.
1
Regression 762.986 1 762.986 1.115 .310b
Residual 8893.714 13 684.132
Total 9656.700 14
a. Dependent Variable: STOE(System Total Operating Expenditures)
b. Predictors: (Constant), Net income /loss
Hypothesis 4
Appendix: 4 Regression analysis between revenue of Aviation sector and passenger, cargo and
aircraft handling and & employees ASM production capabilities
Model Summary
Model R R Square Adjusted R
Square
Std. Error of
the Estimate
1 .947a .897 .879 11.80160
a. Predictors: (Constant), ASM produced per employee
(Available seat miles), PC&A (Passenger Cargo and
Aircraft handling employees per Aircraft)
ANOVAa
Model Sum of
Squares
df Mean
Square
F Sig.
1
Regression 14494.055 2 7247.027 52.033 .000b
Residual 1671.332 12 139.278
Total 16165.387 14
a. Dependent Variable: STOR (system total operating revenues)
b. Predictors: (Constant), ASM produced per employee (Available seat miles),
PC&A (Passenger Cargo and Aircraft handling employees per Aircraft)
14
Operating
Expenditures)
Pearson
Correlation 1 .281
Sig. (2-tailed) .310
N 15 15
Net income /loss
Pearson
Correlation .281 1
Sig. (2-tailed) .310
N 15 15
ANOVAa
Model Sum of
Squares
df Mean
Square
F Sig.
1
Regression 762.986 1 762.986 1.115 .310b
Residual 8893.714 13 684.132
Total 9656.700 14
a. Dependent Variable: STOE(System Total Operating Expenditures)
b. Predictors: (Constant), Net income /loss
Hypothesis 4
Appendix: 4 Regression analysis between revenue of Aviation sector and passenger, cargo and
aircraft handling and & employees ASM production capabilities
Model Summary
Model R R Square Adjusted R
Square
Std. Error of
the Estimate
1 .947a .897 .879 11.80160
a. Predictors: (Constant), ASM produced per employee
(Available seat miles), PC&A (Passenger Cargo and
Aircraft handling employees per Aircraft)
ANOVAa
Model Sum of
Squares
df Mean
Square
F Sig.
1
Regression 14494.055 2 7247.027 52.033 .000b
Residual 1671.332 12 139.278
Total 16165.387 14
a. Dependent Variable: STOR (system total operating revenues)
b. Predictors: (Constant), ASM produced per employee (Available seat miles),
PC&A (Passenger Cargo and Aircraft handling employees per Aircraft)
14
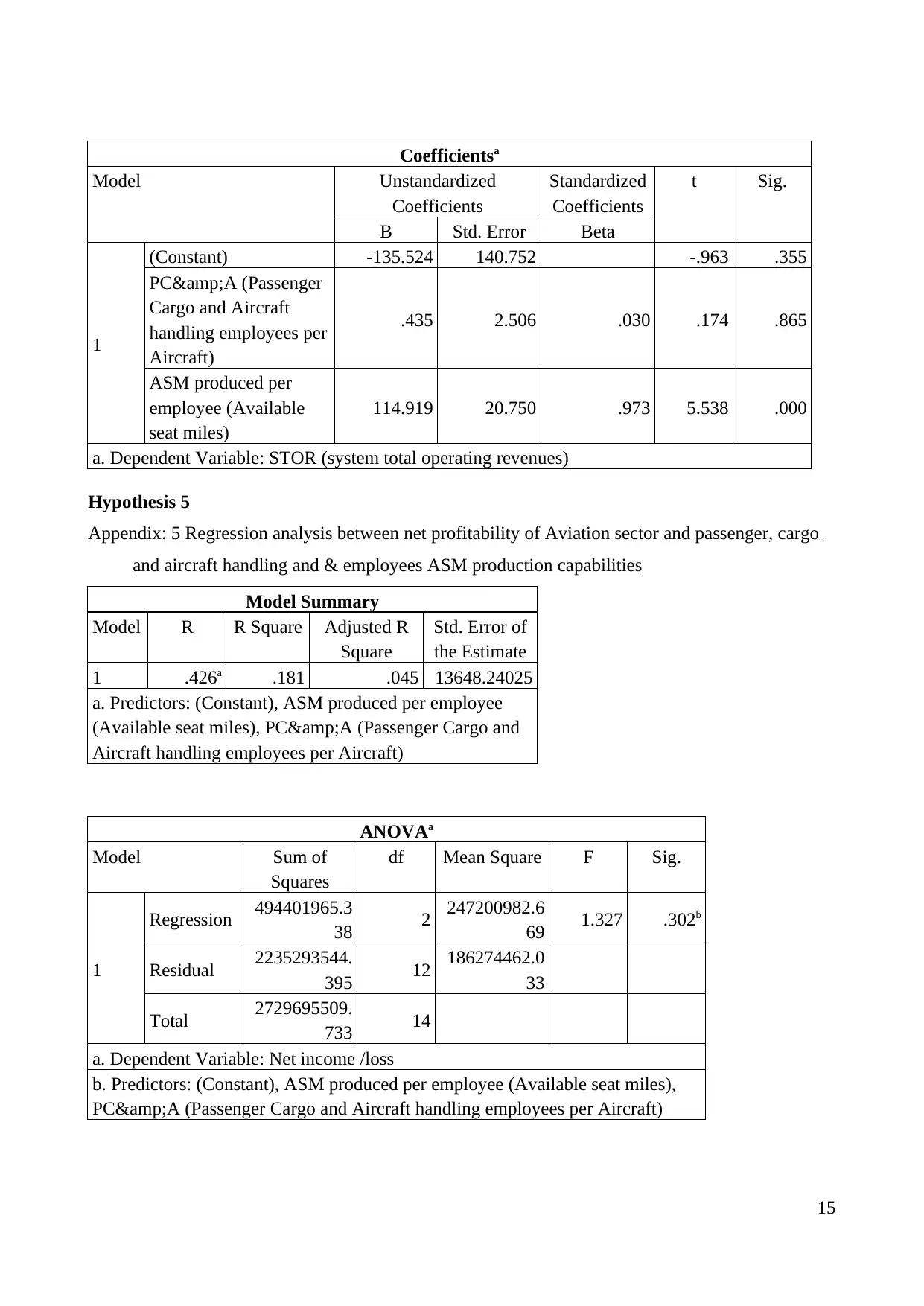
Coefficientsa
Model Unstandardized
Coefficients
Standardized
Coefficients
t Sig.
B Std. Error Beta
1
(Constant) -135.524 140.752 -.963 .355
PC&A (Passenger
Cargo and Aircraft
handling employees per
Aircraft)
.435 2.506 .030 .174 .865
ASM produced per
employee (Available
seat miles)
114.919 20.750 .973 5.538 .000
a. Dependent Variable: STOR (system total operating revenues)
Hypothesis 5
Appendix: 5 Regression analysis between net profitability of Aviation sector and passenger, cargo
and aircraft handling and & employees ASM production capabilities
Model Summary
Model R R Square Adjusted R
Square
Std. Error of
the Estimate
1 .426a .181 .045 13648.24025
a. Predictors: (Constant), ASM produced per employee
(Available seat miles), PC&A (Passenger Cargo and
Aircraft handling employees per Aircraft)
ANOVAa
Model Sum of
Squares
df Mean Square F Sig.
1
Regression 494401965.3
38 2 247200982.6
69 1.327 .302b
Residual 2235293544.
395 12 186274462.0
33
Total 2729695509.
733 14
a. Dependent Variable: Net income /loss
b. Predictors: (Constant), ASM produced per employee (Available seat miles),
PC&A (Passenger Cargo and Aircraft handling employees per Aircraft)
15
Model Unstandardized
Coefficients
Standardized
Coefficients
t Sig.
B Std. Error Beta
1
(Constant) -135.524 140.752 -.963 .355
PC&A (Passenger
Cargo and Aircraft
handling employees per
Aircraft)
.435 2.506 .030 .174 .865
ASM produced per
employee (Available
seat miles)
114.919 20.750 .973 5.538 .000
a. Dependent Variable: STOR (system total operating revenues)
Hypothesis 5
Appendix: 5 Regression analysis between net profitability of Aviation sector and passenger, cargo
and aircraft handling and & employees ASM production capabilities
Model Summary
Model R R Square Adjusted R
Square
Std. Error of
the Estimate
1 .426a .181 .045 13648.24025
a. Predictors: (Constant), ASM produced per employee
(Available seat miles), PC&A (Passenger Cargo and
Aircraft handling employees per Aircraft)
ANOVAa
Model Sum of
Squares
df Mean Square F Sig.
1
Regression 494401965.3
38 2 247200982.6
69 1.327 .302b
Residual 2235293544.
395 12 186274462.0
33
Total 2729695509.
733 14
a. Dependent Variable: Net income /loss
b. Predictors: (Constant), ASM produced per employee (Available seat miles),
PC&A (Passenger Cargo and Aircraft handling employees per Aircraft)
15
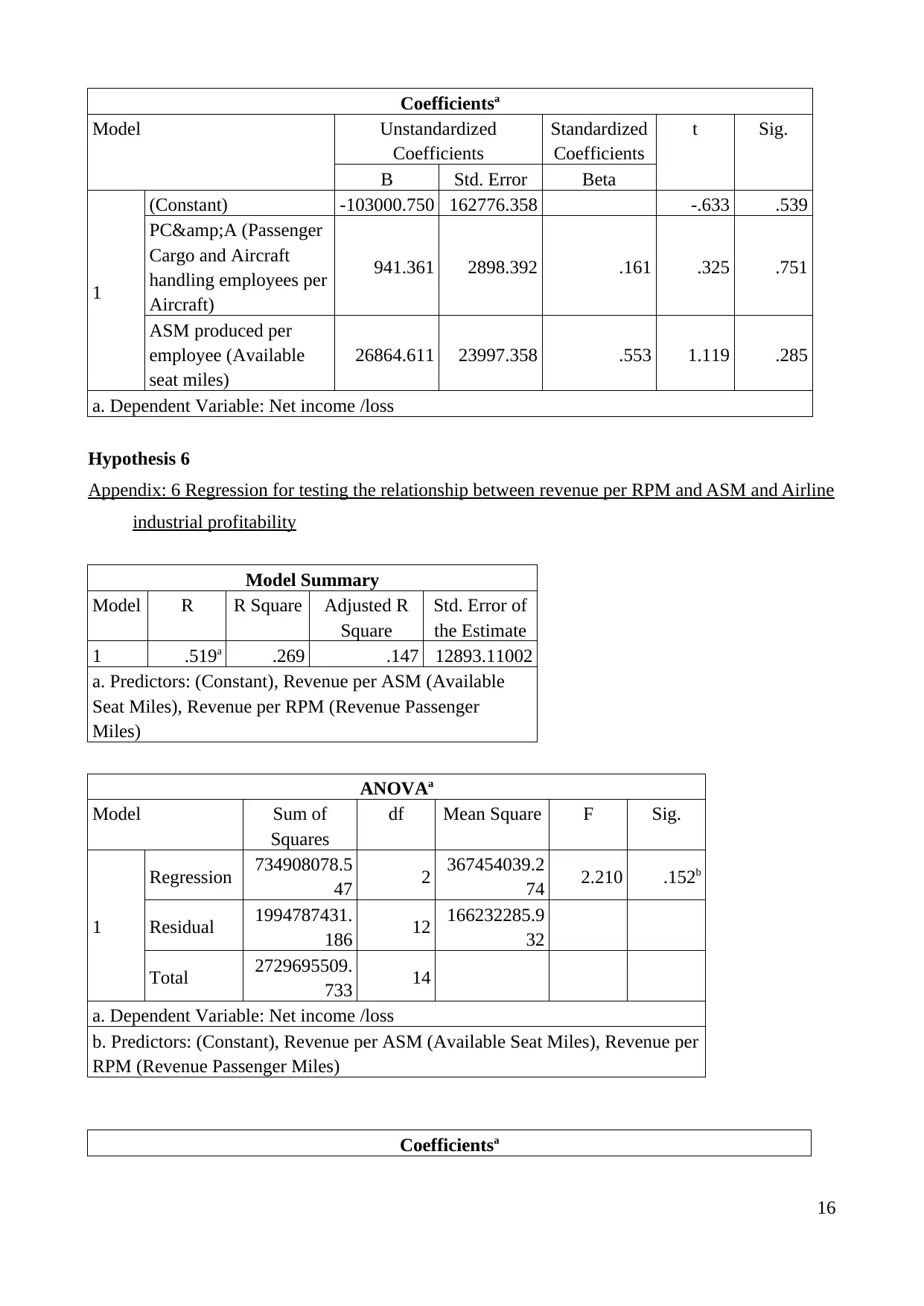
Coefficientsa
Model Unstandardized
Coefficients
Standardized
Coefficients
t Sig.
B Std. Error Beta
1
(Constant) -103000.750 162776.358 -.633 .539
PC&A (Passenger
Cargo and Aircraft
handling employees per
Aircraft)
941.361 2898.392 .161 .325 .751
ASM produced per
employee (Available
seat miles)
26864.611 23997.358 .553 1.119 .285
a. Dependent Variable: Net income /loss
Hypothesis 6
Appendix: 6 Regression for testing the relationship between revenue per RPM and ASM and Airline
industrial profitability
Model Summary
Model R R Square Adjusted R
Square
Std. Error of
the Estimate
1 .519a .269 .147 12893.11002
a. Predictors: (Constant), Revenue per ASM (Available
Seat Miles), Revenue per RPM (Revenue Passenger
Miles)
ANOVAa
Model Sum of
Squares
df Mean Square F Sig.
1
Regression 734908078.5
47 2 367454039.2
74 2.210 .152b
Residual 1994787431.
186 12 166232285.9
32
Total 2729695509.
733 14
a. Dependent Variable: Net income /loss
b. Predictors: (Constant), Revenue per ASM (Available Seat Miles), Revenue per
RPM (Revenue Passenger Miles)
Coefficientsa
16
Model Unstandardized
Coefficients
Standardized
Coefficients
t Sig.
B Std. Error Beta
1
(Constant) -103000.750 162776.358 -.633 .539
PC&A (Passenger
Cargo and Aircraft
handling employees per
Aircraft)
941.361 2898.392 .161 .325 .751
ASM produced per
employee (Available
seat miles)
26864.611 23997.358 .553 1.119 .285
a. Dependent Variable: Net income /loss
Hypothesis 6
Appendix: 6 Regression for testing the relationship between revenue per RPM and ASM and Airline
industrial profitability
Model Summary
Model R R Square Adjusted R
Square
Std. Error of
the Estimate
1 .519a .269 .147 12893.11002
a. Predictors: (Constant), Revenue per ASM (Available
Seat Miles), Revenue per RPM (Revenue Passenger
Miles)
ANOVAa
Model Sum of
Squares
df Mean Square F Sig.
1
Regression 734908078.5
47 2 367454039.2
74 2.210 .152b
Residual 1994787431.
186 12 166232285.9
32
Total 2729695509.
733 14
a. Dependent Variable: Net income /loss
b. Predictors: (Constant), Revenue per ASM (Available Seat Miles), Revenue per
RPM (Revenue Passenger Miles)
Coefficientsa
16
Secure Best Marks with AI Grader
Need help grading? Try our AI Grader for instant feedback on your assignments.
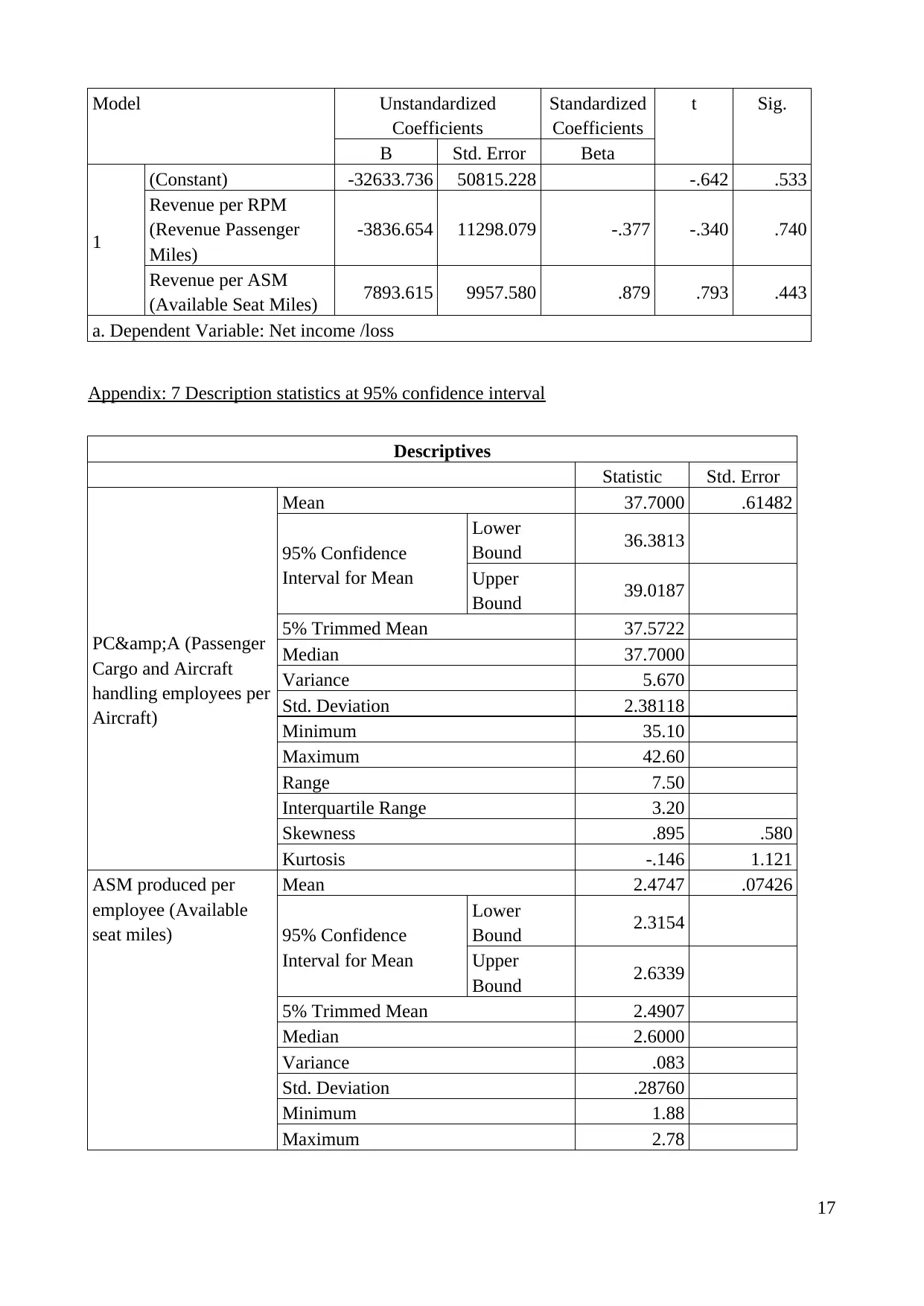
Model Unstandardized
Coefficients
Standardized
Coefficients
t Sig.
B Std. Error Beta
1
(Constant) -32633.736 50815.228 -.642 .533
Revenue per RPM
(Revenue Passenger
Miles)
-3836.654 11298.079 -.377 -.340 .740
Revenue per ASM
(Available Seat Miles) 7893.615 9957.580 .879 .793 .443
a. Dependent Variable: Net income /loss
Appendix: 7 Description statistics at 95% confidence interval
Descriptives
Statistic Std. Error
PC&A (Passenger
Cargo and Aircraft
handling employees per
Aircraft)
Mean 37.7000 .61482
95% Confidence
Interval for Mean
Lower
Bound 36.3813
Upper
Bound 39.0187
5% Trimmed Mean 37.5722
Median 37.7000
Variance 5.670
Std. Deviation 2.38118
Minimum 35.10
Maximum 42.60
Range 7.50
Interquartile Range 3.20
Skewness .895 .580
Kurtosis -.146 1.121
ASM produced per
employee (Available
seat miles)
Mean 2.4747 .07426
95% Confidence
Interval for Mean
Lower
Bound 2.3154
Upper
Bound 2.6339
5% Trimmed Mean 2.4907
Median 2.6000
Variance .083
Std. Deviation .28760
Minimum 1.88
Maximum 2.78
17
Coefficients
Standardized
Coefficients
t Sig.
B Std. Error Beta
1
(Constant) -32633.736 50815.228 -.642 .533
Revenue per RPM
(Revenue Passenger
Miles)
-3836.654 11298.079 -.377 -.340 .740
Revenue per ASM
(Available Seat Miles) 7893.615 9957.580 .879 .793 .443
a. Dependent Variable: Net income /loss
Appendix: 7 Description statistics at 95% confidence interval
Descriptives
Statistic Std. Error
PC&A (Passenger
Cargo and Aircraft
handling employees per
Aircraft)
Mean 37.7000 .61482
95% Confidence
Interval for Mean
Lower
Bound 36.3813
Upper
Bound 39.0187
5% Trimmed Mean 37.5722
Median 37.7000
Variance 5.670
Std. Deviation 2.38118
Minimum 35.10
Maximum 42.60
Range 7.50
Interquartile Range 3.20
Skewness .895 .580
Kurtosis -.146 1.121
ASM produced per
employee (Available
seat miles)
Mean 2.4747 .07426
95% Confidence
Interval for Mean
Lower
Bound 2.3154
Upper
Bound 2.6339
5% Trimmed Mean 2.4907
Median 2.6000
Variance .083
Std. Deviation .28760
Minimum 1.88
Maximum 2.78
17
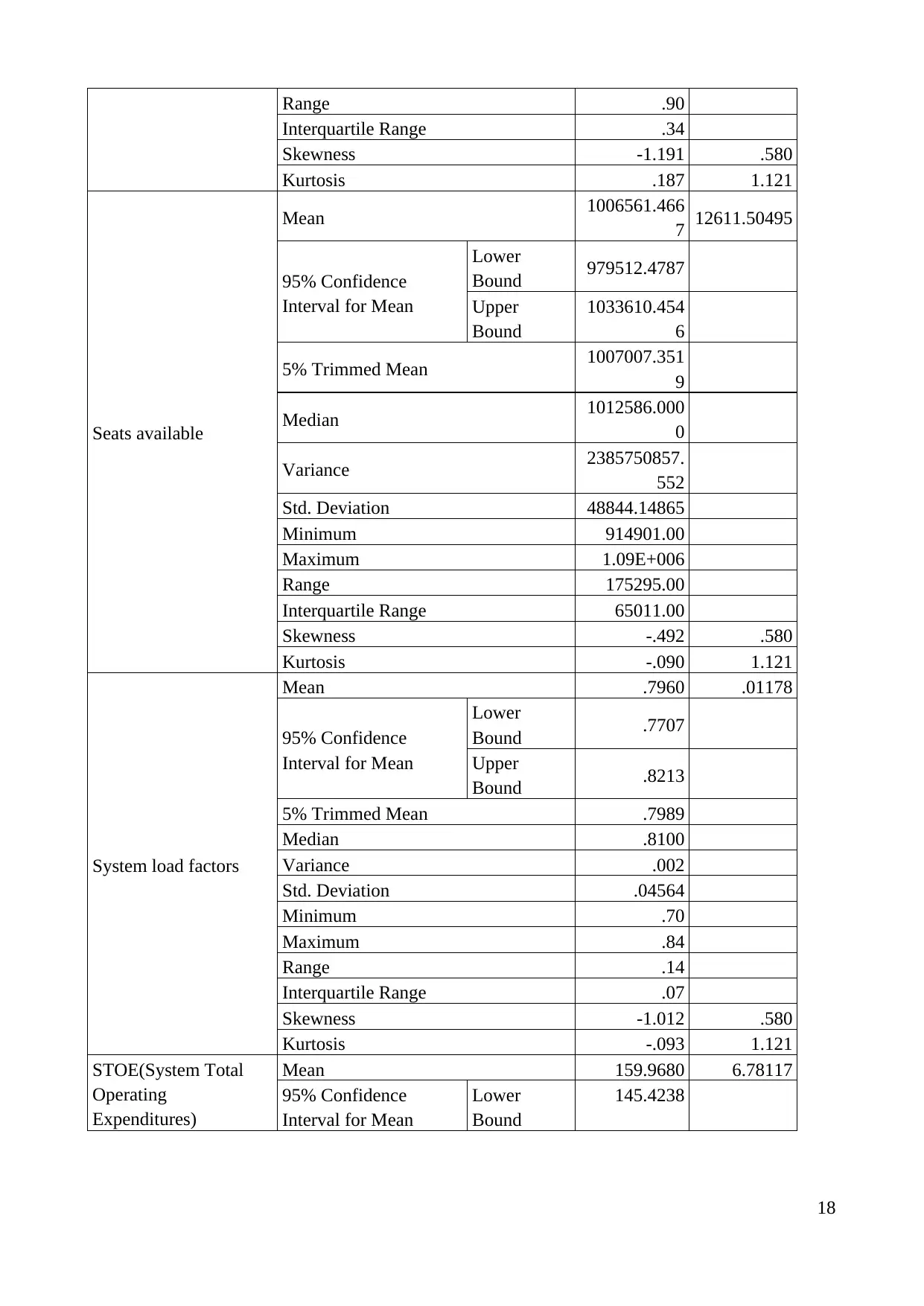
Range .90
Interquartile Range .34
Skewness -1.191 .580
Kurtosis .187 1.121
Seats available
Mean 1006561.466
7 12611.50495
95% Confidence
Interval for Mean
Lower
Bound 979512.4787
Upper
Bound
1033610.454
6
5% Trimmed Mean 1007007.351
9
Median 1012586.000
0
Variance 2385750857.
552
Std. Deviation 48844.14865
Minimum 914901.00
Maximum 1.09E+006
Range 175295.00
Interquartile Range 65011.00
Skewness -.492 .580
Kurtosis -.090 1.121
System load factors
Mean .7960 .01178
95% Confidence
Interval for Mean
Lower
Bound .7707
Upper
Bound .8213
5% Trimmed Mean .7989
Median .8100
Variance .002
Std. Deviation .04564
Minimum .70
Maximum .84
Range .14
Interquartile Range .07
Skewness -1.012 .580
Kurtosis -.093 1.121
STOE(System Total
Operating
Expenditures)
Mean 159.9680 6.78117
95% Confidence
Interval for Mean
Lower
Bound
145.4238
18
Interquartile Range .34
Skewness -1.191 .580
Kurtosis .187 1.121
Seats available
Mean 1006561.466
7 12611.50495
95% Confidence
Interval for Mean
Lower
Bound 979512.4787
Upper
Bound
1033610.454
6
5% Trimmed Mean 1007007.351
9
Median 1012586.000
0
Variance 2385750857.
552
Std. Deviation 48844.14865
Minimum 914901.00
Maximum 1.09E+006
Range 175295.00
Interquartile Range 65011.00
Skewness -.492 .580
Kurtosis -.090 1.121
System load factors
Mean .7960 .01178
95% Confidence
Interval for Mean
Lower
Bound .7707
Upper
Bound .8213
5% Trimmed Mean .7989
Median .8100
Variance .002
Std. Deviation .04564
Minimum .70
Maximum .84
Range .14
Interquartile Range .07
Skewness -1.012 .580
Kurtosis -.093 1.121
STOE(System Total
Operating
Expenditures)
Mean 159.9680 6.78117
95% Confidence
Interval for Mean
Lower
Bound
145.4238
18
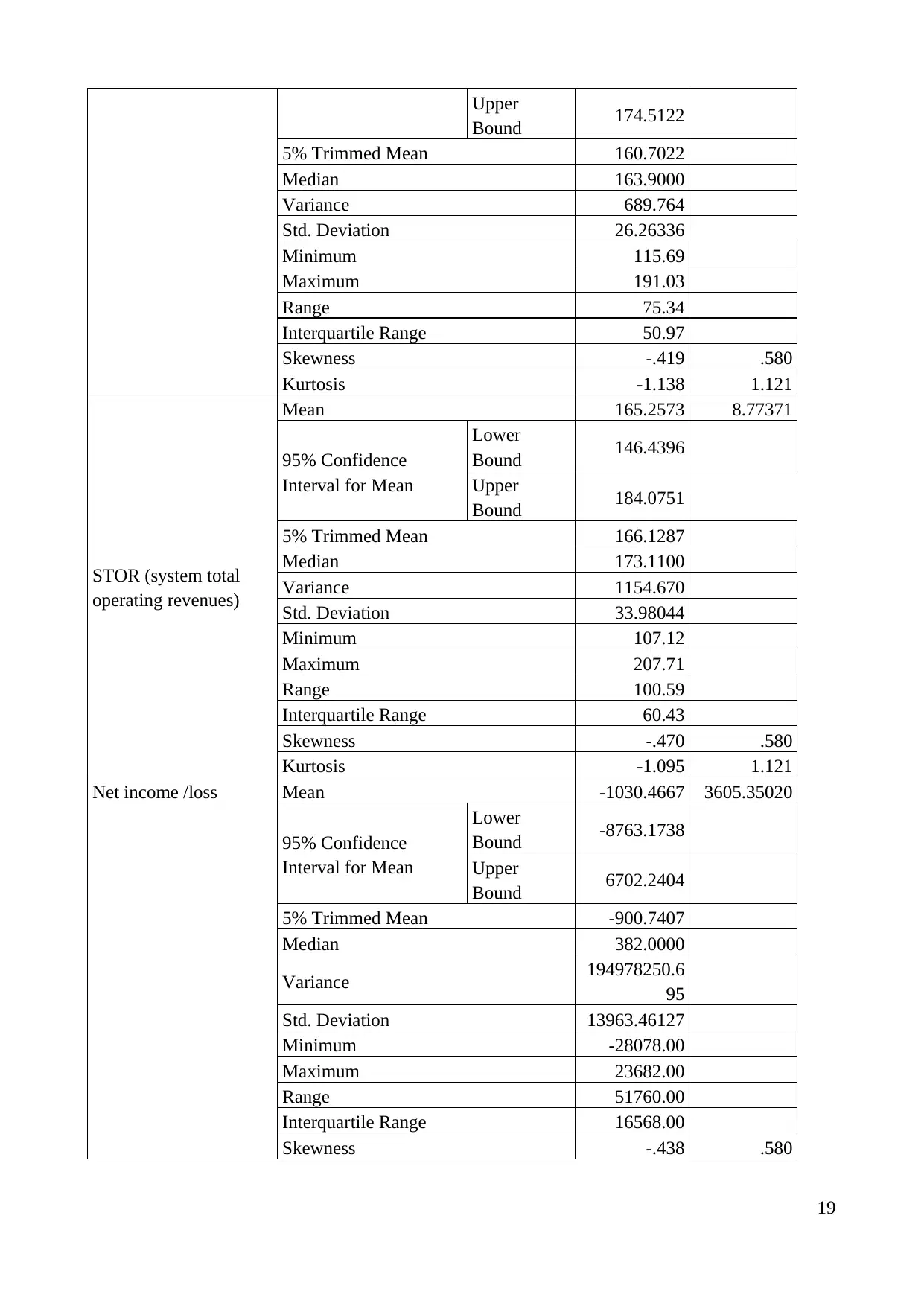
Upper
Bound 174.5122
5% Trimmed Mean 160.7022
Median 163.9000
Variance 689.764
Std. Deviation 26.26336
Minimum 115.69
Maximum 191.03
Range 75.34
Interquartile Range 50.97
Skewness -.419 .580
Kurtosis -1.138 1.121
STOR (system total
operating revenues)
Mean 165.2573 8.77371
95% Confidence
Interval for Mean
Lower
Bound 146.4396
Upper
Bound 184.0751
5% Trimmed Mean 166.1287
Median 173.1100
Variance 1154.670
Std. Deviation 33.98044
Minimum 107.12
Maximum 207.71
Range 100.59
Interquartile Range 60.43
Skewness -.470 .580
Kurtosis -1.095 1.121
Net income /loss Mean -1030.4667 3605.35020
95% Confidence
Interval for Mean
Lower
Bound -8763.1738
Upper
Bound 6702.2404
5% Trimmed Mean -900.7407
Median 382.0000
Variance 194978250.6
95
Std. Deviation 13963.46127
Minimum -28078.00
Maximum 23682.00
Range 51760.00
Interquartile Range 16568.00
Skewness -.438 .580
19
Bound 174.5122
5% Trimmed Mean 160.7022
Median 163.9000
Variance 689.764
Std. Deviation 26.26336
Minimum 115.69
Maximum 191.03
Range 75.34
Interquartile Range 50.97
Skewness -.419 .580
Kurtosis -1.138 1.121
STOR (system total
operating revenues)
Mean 165.2573 8.77371
95% Confidence
Interval for Mean
Lower
Bound 146.4396
Upper
Bound 184.0751
5% Trimmed Mean 166.1287
Median 173.1100
Variance 1154.670
Std. Deviation 33.98044
Minimum 107.12
Maximum 207.71
Range 100.59
Interquartile Range 60.43
Skewness -.470 .580
Kurtosis -1.095 1.121
Net income /loss Mean -1030.4667 3605.35020
95% Confidence
Interval for Mean
Lower
Bound -8763.1738
Upper
Bound 6702.2404
5% Trimmed Mean -900.7407
Median 382.0000
Variance 194978250.6
95
Std. Deviation 13963.46127
Minimum -28078.00
Maximum 23682.00
Range 51760.00
Interquartile Range 16568.00
Skewness -.438 .580
19
Paraphrase This Document
Need a fresh take? Get an instant paraphrase of this document with our AI Paraphraser
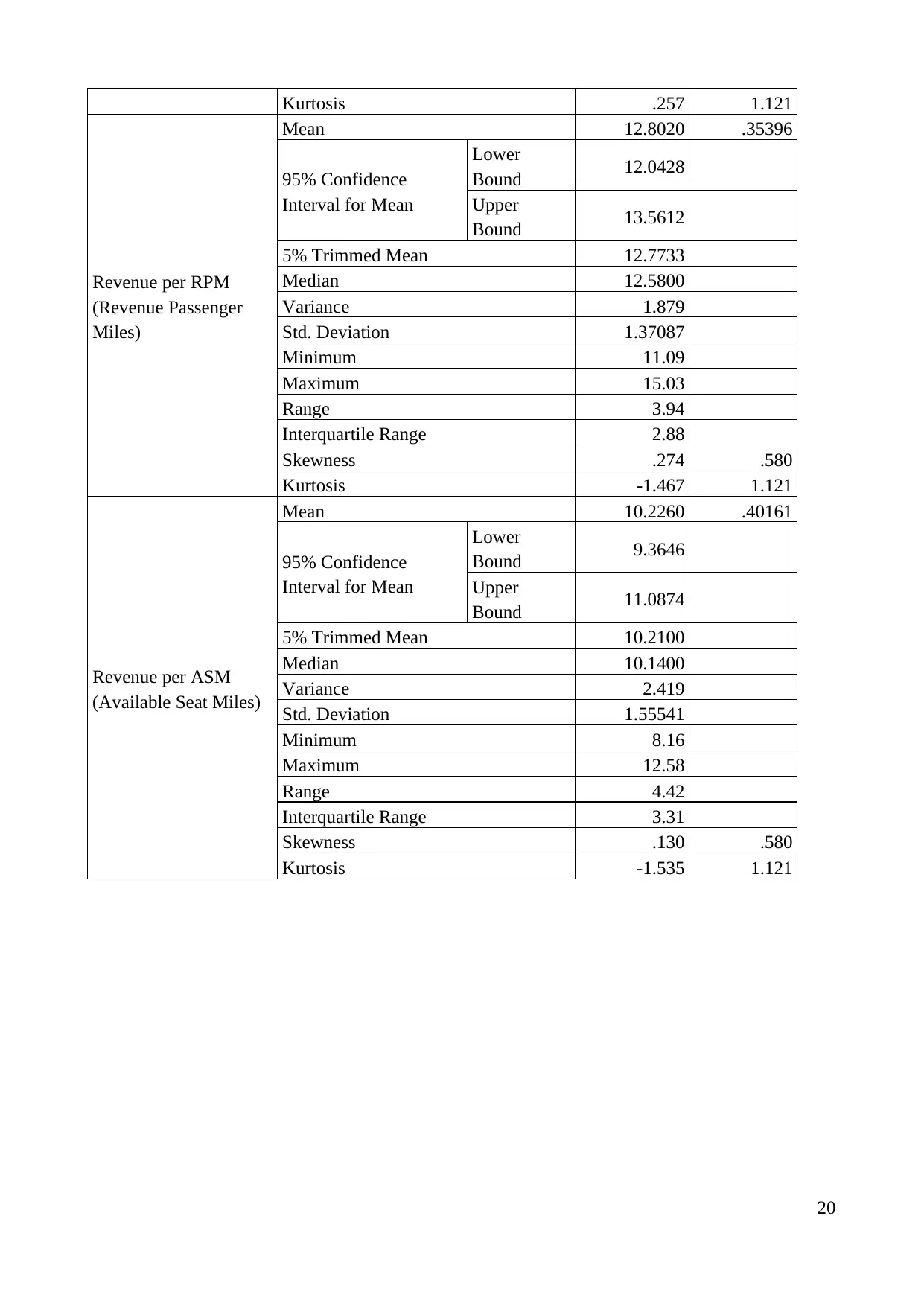
Kurtosis .257 1.121
Revenue per RPM
(Revenue Passenger
Miles)
Mean 12.8020 .35396
95% Confidence
Interval for Mean
Lower
Bound 12.0428
Upper
Bound 13.5612
5% Trimmed Mean 12.7733
Median 12.5800
Variance 1.879
Std. Deviation 1.37087
Minimum 11.09
Maximum 15.03
Range 3.94
Interquartile Range 2.88
Skewness .274 .580
Kurtosis -1.467 1.121
Revenue per ASM
(Available Seat Miles)
Mean 10.2260 .40161
95% Confidence
Interval for Mean
Lower
Bound 9.3646
Upper
Bound 11.0874
5% Trimmed Mean 10.2100
Median 10.1400
Variance 2.419
Std. Deviation 1.55541
Minimum 8.16
Maximum 12.58
Range 4.42
Interquartile Range 3.31
Skewness .130 .580
Kurtosis -1.535 1.121
20
Revenue per RPM
(Revenue Passenger
Miles)
Mean 12.8020 .35396
95% Confidence
Interval for Mean
Lower
Bound 12.0428
Upper
Bound 13.5612
5% Trimmed Mean 12.7733
Median 12.5800
Variance 1.879
Std. Deviation 1.37087
Minimum 11.09
Maximum 15.03
Range 3.94
Interquartile Range 2.88
Skewness .274 .580
Kurtosis -1.467 1.121
Revenue per ASM
(Available Seat Miles)
Mean 10.2260 .40161
95% Confidence
Interval for Mean
Lower
Bound 9.3646
Upper
Bound 11.0874
5% Trimmed Mean 10.2100
Median 10.1400
Variance 2.419
Std. Deviation 1.55541
Minimum 8.16
Maximum 12.58
Range 4.42
Interquartile Range 3.31
Skewness .130 .580
Kurtosis -1.535 1.121
20
1 out of 20
![[object Object]](/_next/image/?url=%2F_next%2Fstatic%2Fmedia%2Flogo.6d15ce61.png&w=640&q=75)
Your All-in-One AI-Powered Toolkit for Academic Success.
+13062052269
info@desklib.com
Available 24*7 on WhatsApp / Email
Unlock your academic potential
© 2024 | Zucol Services PVT LTD | All rights reserved.