Statistical Analysis of Hydrological Data Imputation in Brisbane
VerifiedAdded on 2023/01/11
|8
|1417
|23
Report
AI Summary
This report addresses the challenge of missing data in hydrological databases, which often hinders the construction of accurate runoff prediction models. It explores various statistical methods, with a specific focus on the R language, to fill these data gaps. The study highlights the significance of reliab...
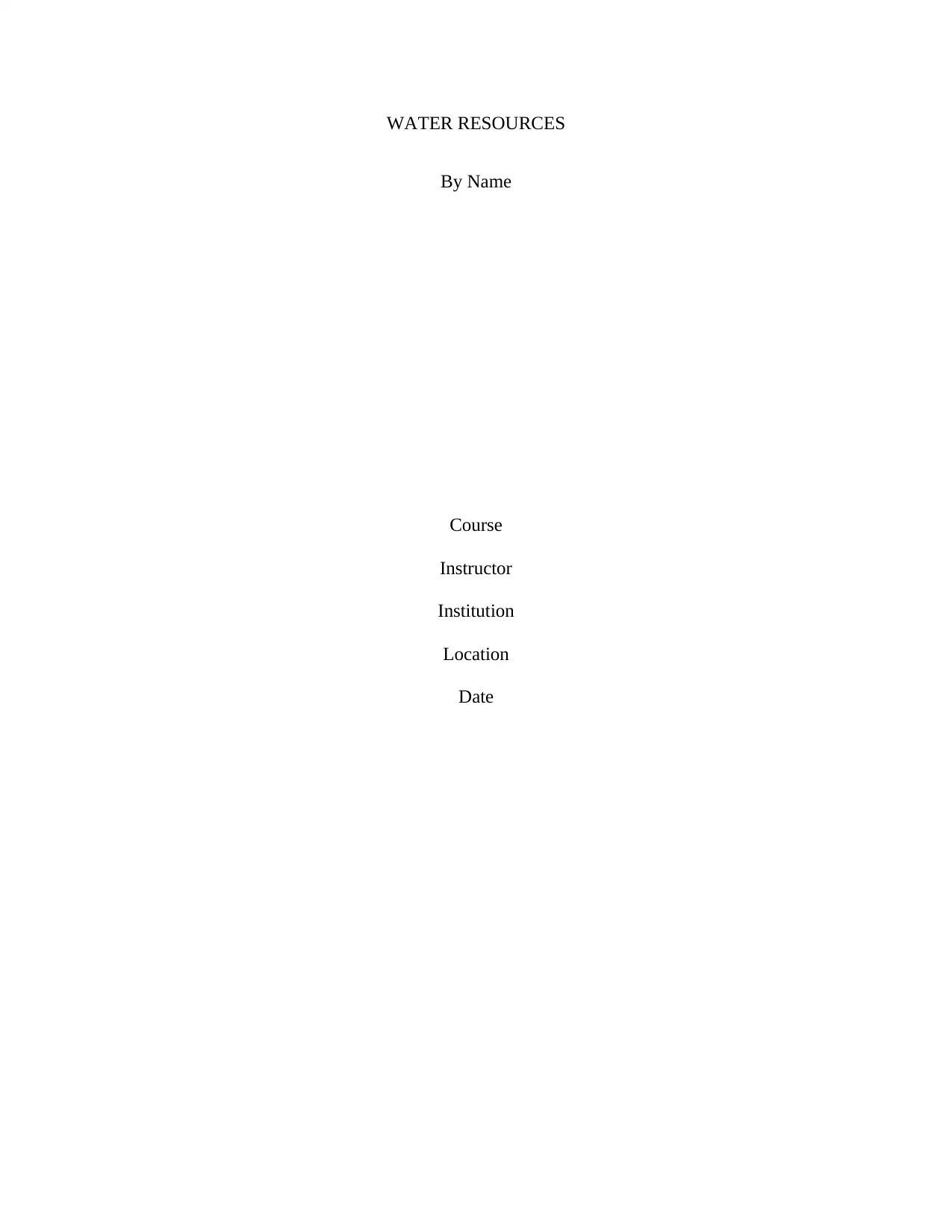
WATER RESOURCES
By Name
Course
Instructor
Institution
Location
Date
By Name
Course
Instructor
Institution
Location
Date
Paraphrase This Document
Need a fresh take? Get an instant paraphrase of this document with our AI Paraphraser
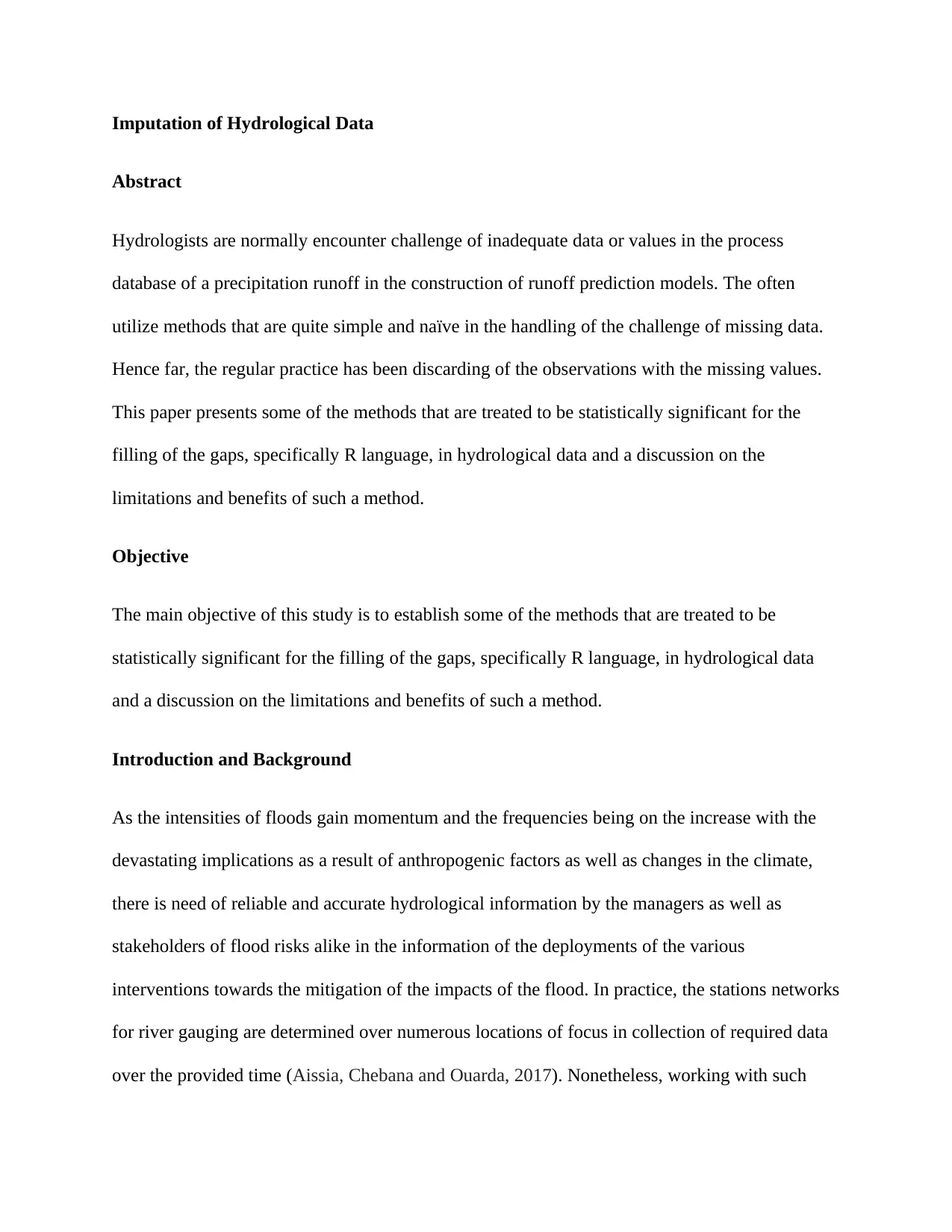
Imputation of Hydrological Data
Abstract
Hydrologists are normally encounter challenge of inadequate data or values in the process
database of a precipitation runoff in the construction of runoff prediction models. The often
utilize methods that are quite simple and naïve in the handling of the challenge of missing data.
Hence far, the regular practice has been discarding of the observations with the missing values.
This paper presents some of the methods that are treated to be statistically significant for the
filling of the gaps, specifically R language, in hydrological data and a discussion on the
limitations and benefits of such a method.
Objective
The main objective of this study is to establish some of the methods that are treated to be
statistically significant for the filling of the gaps, specifically R language, in hydrological data
and a discussion on the limitations and benefits of such a method.
Introduction and Background
As the intensities of floods gain momentum and the frequencies being on the increase with the
devastating implications as a result of anthropogenic factors as well as changes in the climate,
there is need of reliable and accurate hydrological information by the managers as well as
stakeholders of flood risks alike in the information of the deployments of the various
interventions towards the mitigation of the impacts of the flood. In practice, the stations networks
for river gauging are determined over numerous locations of focus in collection of required data
over the provided time (Aissia, Chebana and Ouarda, 2017). Nonetheless, working with such
Abstract
Hydrologists are normally encounter challenge of inadequate data or values in the process
database of a precipitation runoff in the construction of runoff prediction models. The often
utilize methods that are quite simple and naïve in the handling of the challenge of missing data.
Hence far, the regular practice has been discarding of the observations with the missing values.
This paper presents some of the methods that are treated to be statistically significant for the
filling of the gaps, specifically R language, in hydrological data and a discussion on the
limitations and benefits of such a method.
Objective
The main objective of this study is to establish some of the methods that are treated to be
statistically significant for the filling of the gaps, specifically R language, in hydrological data
and a discussion on the limitations and benefits of such a method.
Introduction and Background
As the intensities of floods gain momentum and the frequencies being on the increase with the
devastating implications as a result of anthropogenic factors as well as changes in the climate,
there is need of reliable and accurate hydrological information by the managers as well as
stakeholders of flood risks alike in the information of the deployments of the various
interventions towards the mitigation of the impacts of the flood. In practice, the stations networks
for river gauging are determined over numerous locations of focus in collection of required data
over the provided time (Aissia, Chebana and Ouarda, 2017). Nonetheless, working with such
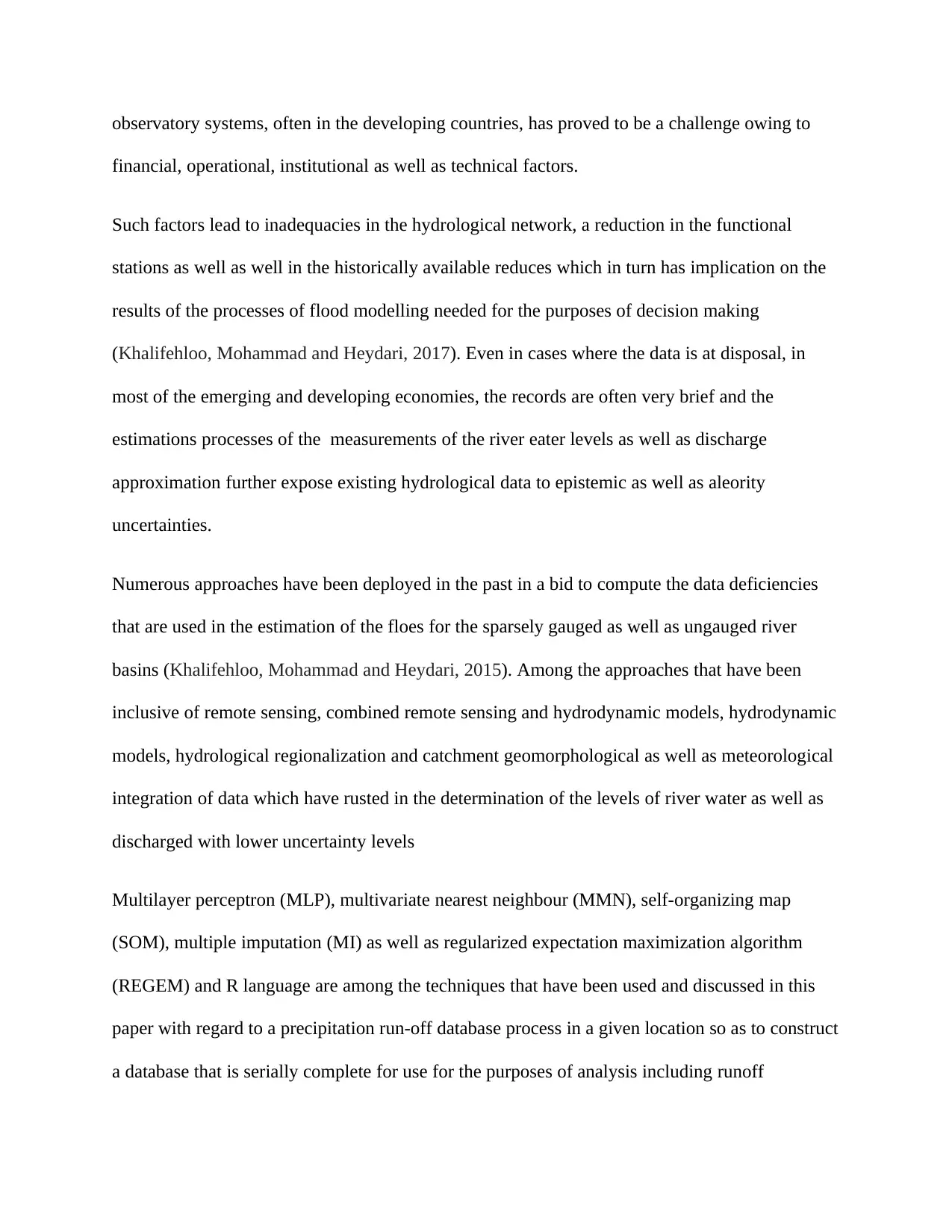
observatory systems, often in the developing countries, has proved to be a challenge owing to
financial, operational, institutional as well as technical factors.
Such factors lead to inadequacies in the hydrological network, a reduction in the functional
stations as well as well in the historically available reduces which in turn has implication on the
results of the processes of flood modelling needed for the purposes of decision making
(Khalifehloo, Mohammad and Heydari, 2017). Even in cases where the data is at disposal, in
most of the emerging and developing economies, the records are often very brief and the
estimations processes of the measurements of the river eater levels as well as discharge
approximation further expose existing hydrological data to epistemic as well as aleority
uncertainties.
Numerous approaches have been deployed in the past in a bid to compute the data deficiencies
that are used in the estimation of the floes for the sparsely gauged as well as ungauged river
basins (Khalifehloo, Mohammad and Heydari, 2015). Among the approaches that have been
inclusive of remote sensing, combined remote sensing and hydrodynamic models, hydrodynamic
models, hydrological regionalization and catchment geomorphological as well as meteorological
integration of data which have rusted in the determination of the levels of river water as well as
discharged with lower uncertainty levels
Multilayer perceptron (MLP), multivariate nearest neighbour (MMN), self-organizing map
(SOM), multiple imputation (MI) as well as regularized expectation maximization algorithm
(REGEM) and R language are among the techniques that have been used and discussed in this
paper with regard to a precipitation run-off database process in a given location so as to construct
a database that is serially complete for use for the purposes of analysis including runoff
financial, operational, institutional as well as technical factors.
Such factors lead to inadequacies in the hydrological network, a reduction in the functional
stations as well as well in the historically available reduces which in turn has implication on the
results of the processes of flood modelling needed for the purposes of decision making
(Khalifehloo, Mohammad and Heydari, 2017). Even in cases where the data is at disposal, in
most of the emerging and developing economies, the records are often very brief and the
estimations processes of the measurements of the river eater levels as well as discharge
approximation further expose existing hydrological data to epistemic as well as aleority
uncertainties.
Numerous approaches have been deployed in the past in a bid to compute the data deficiencies
that are used in the estimation of the floes for the sparsely gauged as well as ungauged river
basins (Khalifehloo, Mohammad and Heydari, 2015). Among the approaches that have been
inclusive of remote sensing, combined remote sensing and hydrodynamic models, hydrodynamic
models, hydrological regionalization and catchment geomorphological as well as meteorological
integration of data which have rusted in the determination of the levels of river water as well as
discharged with lower uncertainty levels
Multilayer perceptron (MLP), multivariate nearest neighbour (MMN), self-organizing map
(SOM), multiple imputation (MI) as well as regularized expectation maximization algorithm
(REGEM) and R language are among the techniques that have been used and discussed in this
paper with regard to a precipitation run-off database process in a given location so as to construct
a database that is serially complete for use for the purposes of analysis including runoff
⊘ This is a preview!⊘
Do you want full access?
Subscribe today to unlock all pages.

Trusted by 1+ million students worldwide
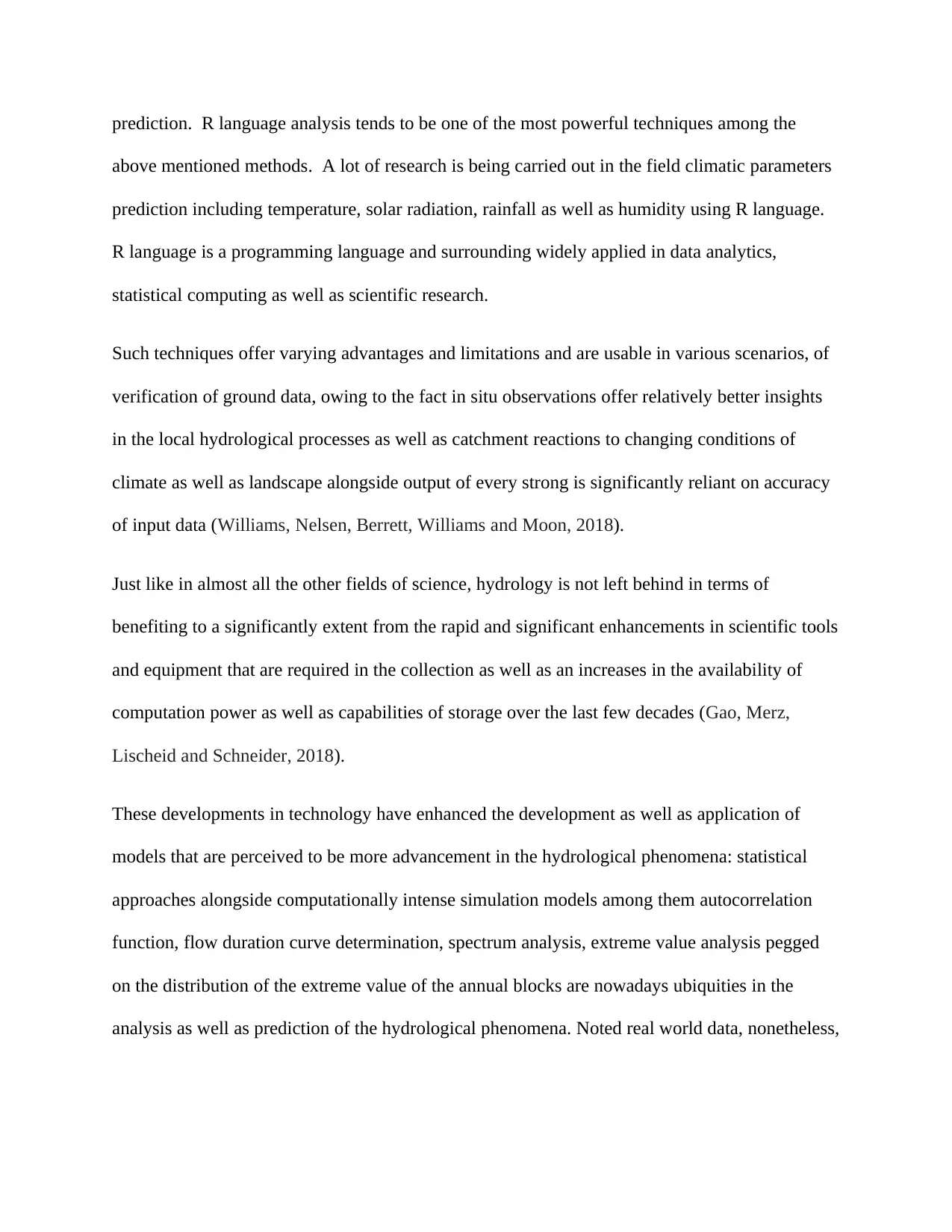
prediction. R language analysis tends to be one of the most powerful techniques among the
above mentioned methods. A lot of research is being carried out in the field climatic parameters
prediction including temperature, solar radiation, rainfall as well as humidity using R language.
R language is a programming language and surrounding widely applied in data analytics,
statistical computing as well as scientific research.
Such techniques offer varying advantages and limitations and are usable in various scenarios, of
verification of ground data, owing to the fact in situ observations offer relatively better insights
in the local hydrological processes as well as catchment reactions to changing conditions of
climate as well as landscape alongside output of every strong is significantly reliant on accuracy
of input data (Williams, Nelsen, Berrett, Williams and Moon, 2018).
Just like in almost all the other fields of science, hydrology is not left behind in terms of
benefiting to a significantly extent from the rapid and significant enhancements in scientific tools
and equipment that are required in the collection as well as an increases in the availability of
computation power as well as capabilities of storage over the last few decades (Gao, Merz,
Lischeid and Schneider, 2018).
These developments in technology have enhanced the development as well as application of
models that are perceived to be more advancement in the hydrological phenomena: statistical
approaches alongside computationally intense simulation models among them autocorrelation
function, flow duration curve determination, spectrum analysis, extreme value analysis pegged
on the distribution of the extreme value of the annual blocks are nowadays ubiquities in the
analysis as well as prediction of the hydrological phenomena. Noted real world data, nonetheless,
above mentioned methods. A lot of research is being carried out in the field climatic parameters
prediction including temperature, solar radiation, rainfall as well as humidity using R language.
R language is a programming language and surrounding widely applied in data analytics,
statistical computing as well as scientific research.
Such techniques offer varying advantages and limitations and are usable in various scenarios, of
verification of ground data, owing to the fact in situ observations offer relatively better insights
in the local hydrological processes as well as catchment reactions to changing conditions of
climate as well as landscape alongside output of every strong is significantly reliant on accuracy
of input data (Williams, Nelsen, Berrett, Williams and Moon, 2018).
Just like in almost all the other fields of science, hydrology is not left behind in terms of
benefiting to a significantly extent from the rapid and significant enhancements in scientific tools
and equipment that are required in the collection as well as an increases in the availability of
computation power as well as capabilities of storage over the last few decades (Gao, Merz,
Lischeid and Schneider, 2018).
These developments in technology have enhanced the development as well as application of
models that are perceived to be more advancement in the hydrological phenomena: statistical
approaches alongside computationally intense simulation models among them autocorrelation
function, flow duration curve determination, spectrum analysis, extreme value analysis pegged
on the distribution of the extreme value of the annual blocks are nowadays ubiquities in the
analysis as well as prediction of the hydrological phenomena. Noted real world data, nonetheless,
Paraphrase This Document
Need a fresh take? Get an instant paraphrase of this document with our AI Paraphraser
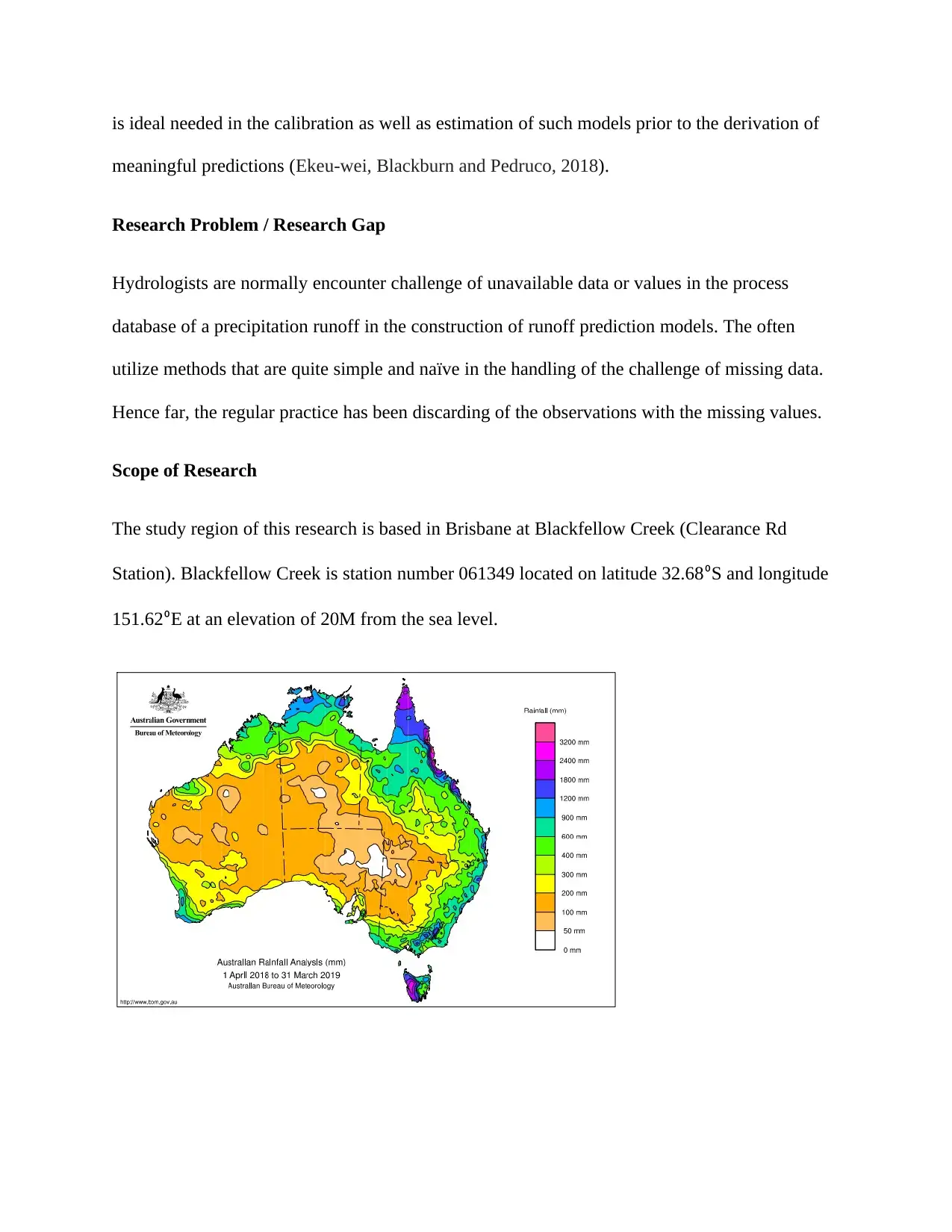
is ideal needed in the calibration as well as estimation of such models prior to the derivation of
meaningful predictions (Ekeu-wei, Blackburn and Pedruco, 2018).
Research Problem / Research Gap
Hydrologists are normally encounter challenge of unavailable data or values in the process
database of a precipitation runoff in the construction of runoff prediction models. The often
utilize methods that are quite simple and naïve in the handling of the challenge of missing data.
Hence far, the regular practice has been discarding of the observations with the missing values.
Scope of Research
The study region of this research is based in Brisbane at Blackfellow Creek (Clearance Rd
Station). Blackfellow Creek is station number 061349 located on latitude 32.68⁰S and longitude
151.62⁰E at an elevation of 20M from the sea level.
meaningful predictions (Ekeu-wei, Blackburn and Pedruco, 2018).
Research Problem / Research Gap
Hydrologists are normally encounter challenge of unavailable data or values in the process
database of a precipitation runoff in the construction of runoff prediction models. The often
utilize methods that are quite simple and naïve in the handling of the challenge of missing data.
Hence far, the regular practice has been discarding of the observations with the missing values.
Scope of Research
The study region of this research is based in Brisbane at Blackfellow Creek (Clearance Rd
Station). Blackfellow Creek is station number 061349 located on latitude 32.68⁰S and longitude
151.62⁰E at an elevation of 20M from the sea level.
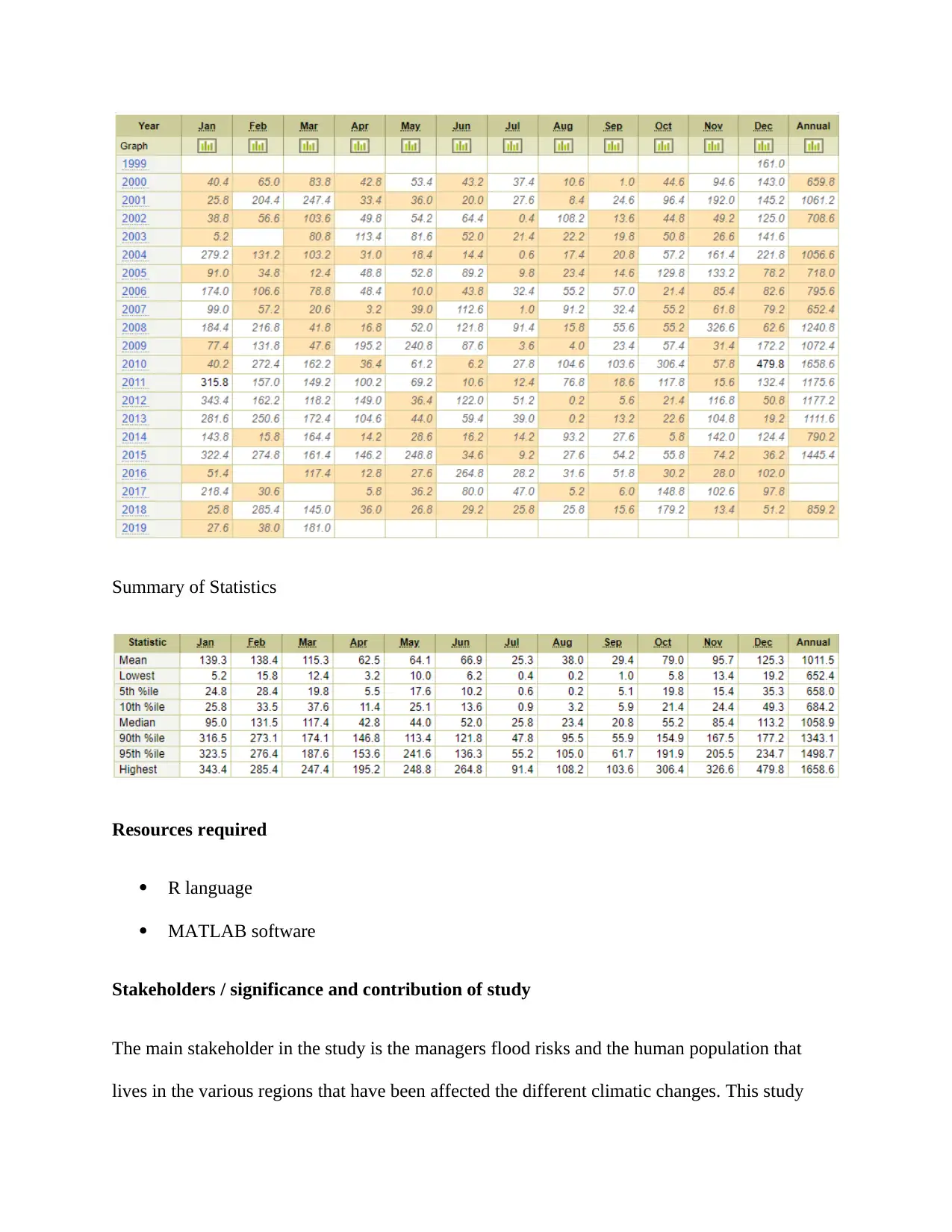
Summary of Statistics
Resources required
R language
MATLAB software
Stakeholders / significance and contribution of study
The main stakeholder in the study is the managers flood risks and the human population that
lives in the various regions that have been affected the different climatic changes. This study
Resources required
R language
MATLAB software
Stakeholders / significance and contribution of study
The main stakeholder in the study is the managers flood risks and the human population that
lives in the various regions that have been affected the different climatic changes. This study
⊘ This is a preview!⊘
Do you want full access?
Subscribe today to unlock all pages.

Trusted by 1+ million students worldwide
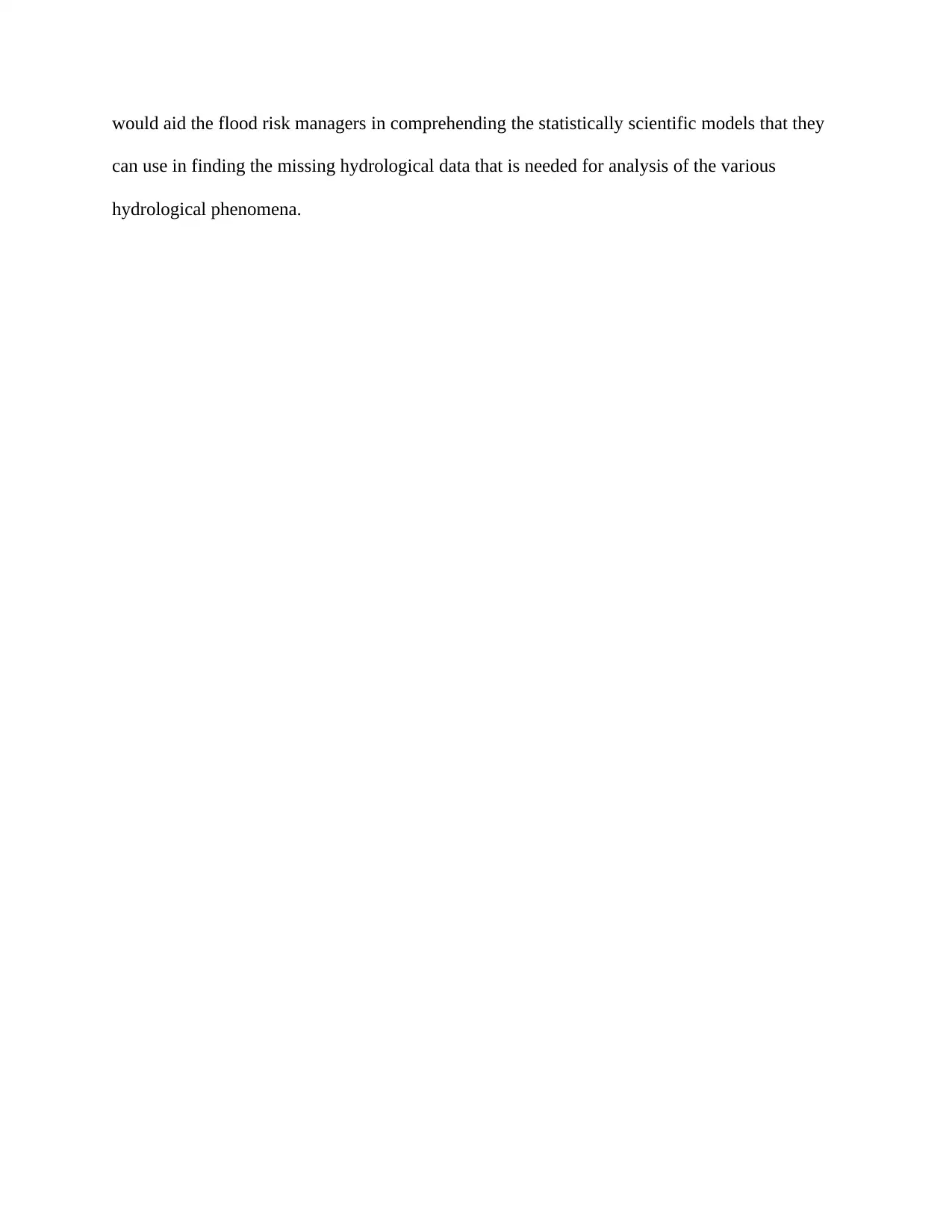
would aid the flood risk managers in comprehending the statistically scientific models that they
can use in finding the missing hydrological data that is needed for analysis of the various
hydrological phenomena.
can use in finding the missing hydrological data that is needed for analysis of the various
hydrological phenomena.
Paraphrase This Document
Need a fresh take? Get an instant paraphrase of this document with our AI Paraphraser
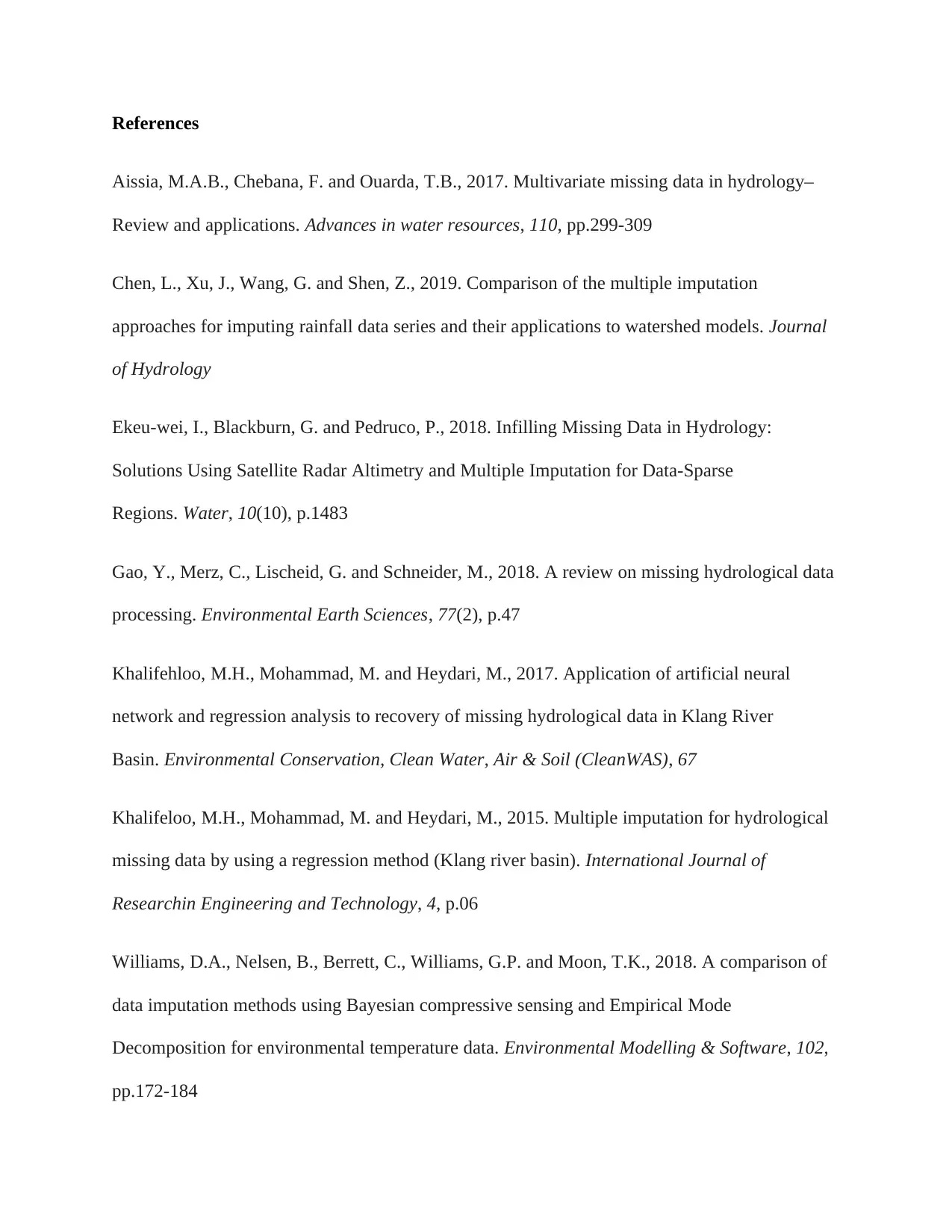
References
Aissia, M.A.B., Chebana, F. and Ouarda, T.B., 2017. Multivariate missing data in hydrology–
Review and applications. Advances in water resources, 110, pp.299-309
Chen, L., Xu, J., Wang, G. and Shen, Z., 2019. Comparison of the multiple imputation
approaches for imputing rainfall data series and their applications to watershed models. Journal
of Hydrology
Ekeu-wei, I., Blackburn, G. and Pedruco, P., 2018. Infilling Missing Data in Hydrology:
Solutions Using Satellite Radar Altimetry and Multiple Imputation for Data-Sparse
Regions. Water, 10(10), p.1483
Gao, Y., Merz, C., Lischeid, G. and Schneider, M., 2018. A review on missing hydrological data
processing. Environmental Earth Sciences, 77(2), p.47
Khalifehloo, M.H., Mohammad, M. and Heydari, M., 2017. Application of artificial neural
network and regression analysis to recovery of missing hydrological data in Klang River
Basin. Environmental Conservation, Clean Water, Air & Soil (CleanWAS), 67
Khalifeloo, M.H., Mohammad, M. and Heydari, M., 2015. Multiple imputation for hydrological
missing data by using a regression method (Klang river basin). International Journal of
Researchin Engineering and Technology, 4, p.06
Williams, D.A., Nelsen, B., Berrett, C., Williams, G.P. and Moon, T.K., 2018. A comparison of
data imputation methods using Bayesian compressive sensing and Empirical Mode
Decomposition for environmental temperature data. Environmental Modelling & Software, 102,
pp.172-184
Aissia, M.A.B., Chebana, F. and Ouarda, T.B., 2017. Multivariate missing data in hydrology–
Review and applications. Advances in water resources, 110, pp.299-309
Chen, L., Xu, J., Wang, G. and Shen, Z., 2019. Comparison of the multiple imputation
approaches for imputing rainfall data series and their applications to watershed models. Journal
of Hydrology
Ekeu-wei, I., Blackburn, G. and Pedruco, P., 2018. Infilling Missing Data in Hydrology:
Solutions Using Satellite Radar Altimetry and Multiple Imputation for Data-Sparse
Regions. Water, 10(10), p.1483
Gao, Y., Merz, C., Lischeid, G. and Schneider, M., 2018. A review on missing hydrological data
processing. Environmental Earth Sciences, 77(2), p.47
Khalifehloo, M.H., Mohammad, M. and Heydari, M., 2017. Application of artificial neural
network and regression analysis to recovery of missing hydrological data in Klang River
Basin. Environmental Conservation, Clean Water, Air & Soil (CleanWAS), 67
Khalifeloo, M.H., Mohammad, M. and Heydari, M., 2015. Multiple imputation for hydrological
missing data by using a regression method (Klang river basin). International Journal of
Researchin Engineering and Technology, 4, p.06
Williams, D.A., Nelsen, B., Berrett, C., Williams, G.P. and Moon, T.K., 2018. A comparison of
data imputation methods using Bayesian compressive sensing and Empirical Mode
Decomposition for environmental temperature data. Environmental Modelling & Software, 102,
pp.172-184
1 out of 8

Your All-in-One AI-Powered Toolkit for Academic Success.
+13062052269
info@desklib.com
Available 24*7 on WhatsApp / Email
Unlock your academic potential
© 2024 | Zucol Services PVT LTD | All rights reserved.