Data Mining Application in Wildfires Forecasting: Project Report
VerifiedAdded on 2023/06/04
|9
|1748
|261
Project
AI Summary
This project investigates the application of data mining techniques to improve wildfire forecasting. It begins with an introduction to the increasing frequency and intensity of wildfires, emphasizing the need for better predictive models. The problem statement highlights the inefficiency of current model...
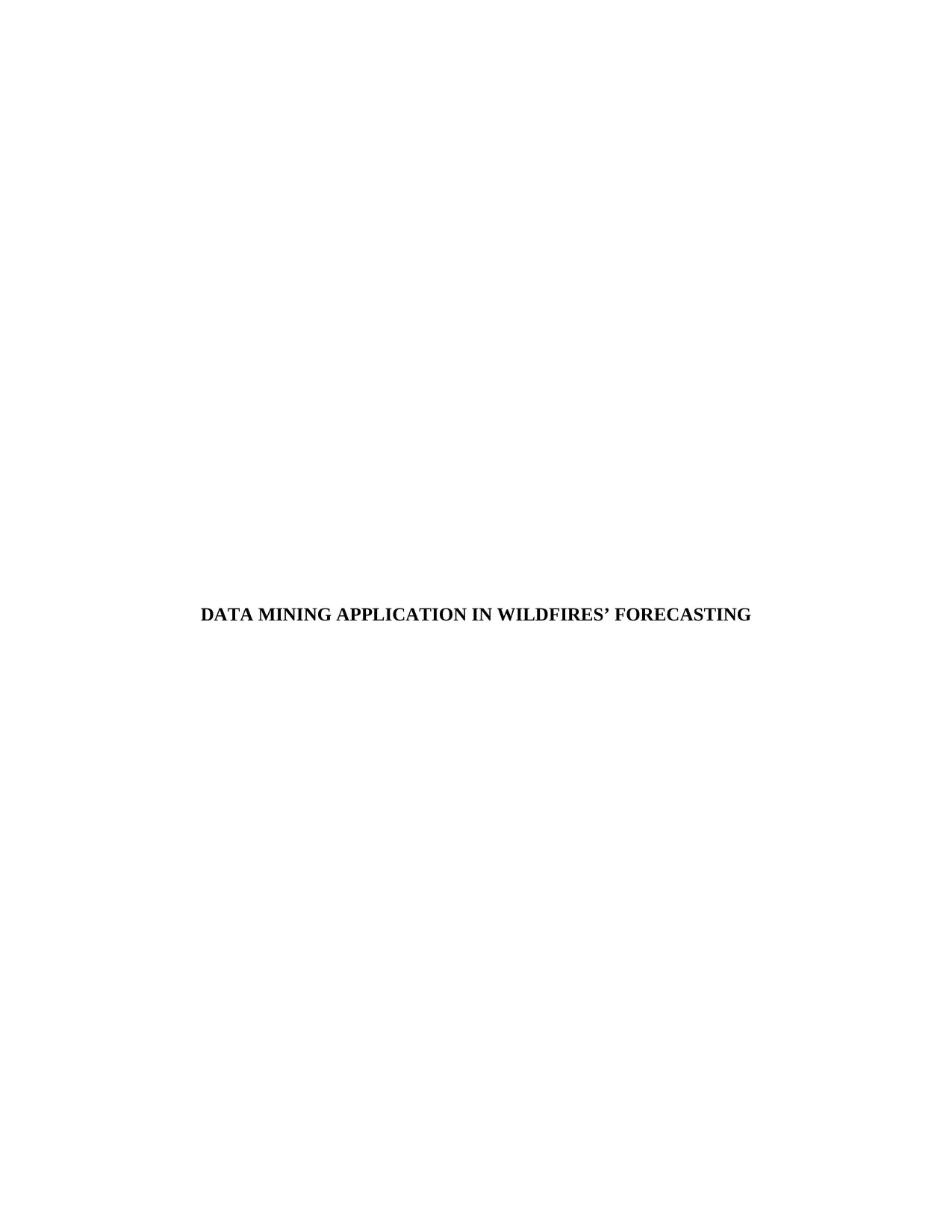
DATA MINING APPLICATION IN WILDFIRES’ FORECASTING
Paraphrase This Document
Need a fresh take? Get an instant paraphrase of this document with our AI Paraphraser

DATA MINING APPLICATION IN WILDFIRES’ FORECASTING
Table of Contents
INTRODUCTION...........................................................................................................................3
PROBLEM STATEMENT..............................................................................................................4
LITERATURE REVIEW................................................................................................................5
DATA COLLECTION AND PREPARATION..............................................................................7
DATA ANALYSIS AND EVALUATION OF RESULTS............................................................8
CONCLUSION................................................................................................................................9
REFERENCES..............................................................................................................................10
2
Table of Contents
INTRODUCTION...........................................................................................................................3
PROBLEM STATEMENT..............................................................................................................4
LITERATURE REVIEW................................................................................................................5
DATA COLLECTION AND PREPARATION..............................................................................7
DATA ANALYSIS AND EVALUATION OF RESULTS............................................................8
CONCLUSION................................................................................................................................9
REFERENCES..............................................................................................................................10
2
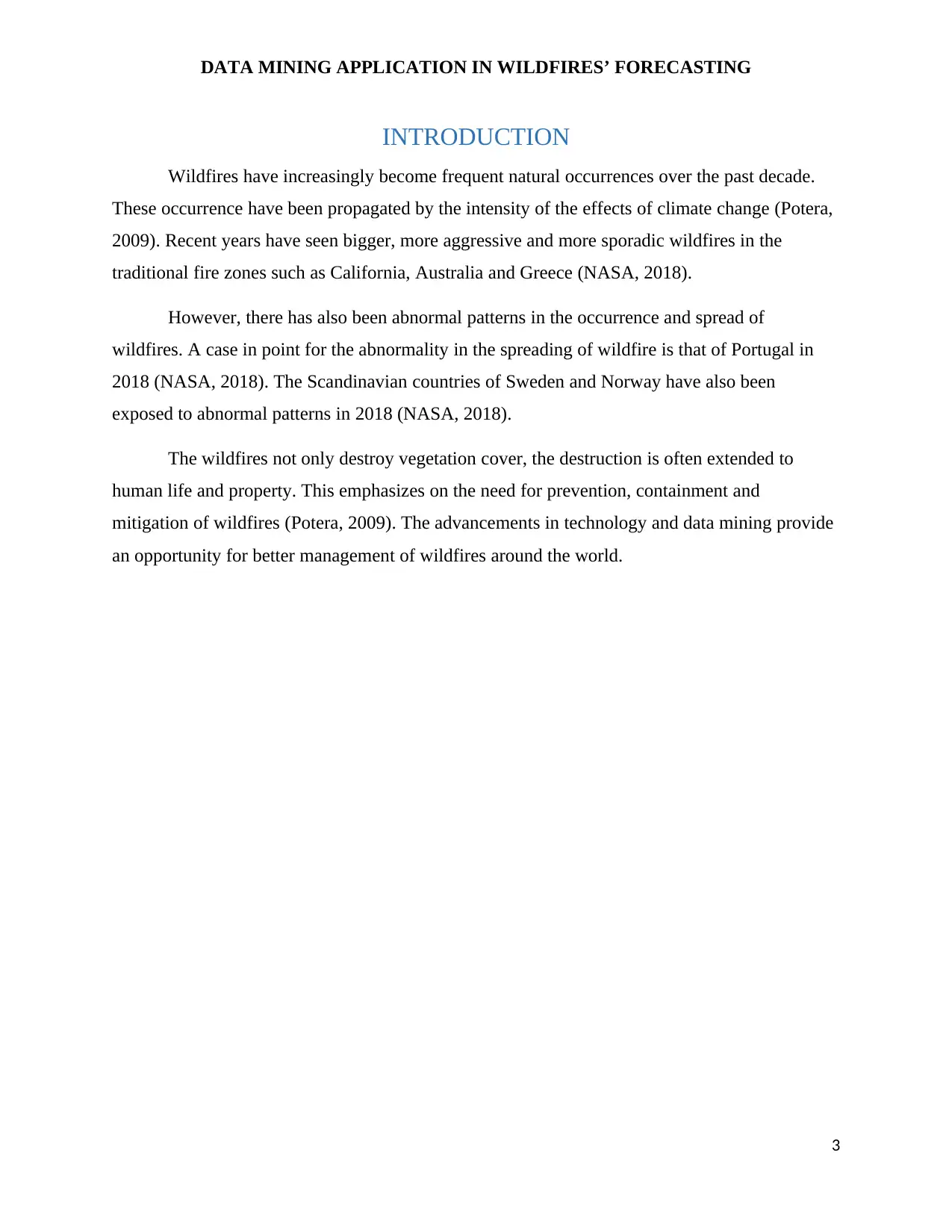
DATA MINING APPLICATION IN WILDFIRES’ FORECASTING
INTRODUCTION
Wildfires have increasingly become frequent natural occurrences over the past decade.
These occurrence have been propagated by the intensity of the effects of climate change (Potera,
2009). Recent years have seen bigger, more aggressive and more sporadic wildfires in the
traditional fire zones such as California, Australia and Greece (NASA, 2018).
However, there has also been abnormal patterns in the occurrence and spread of
wildfires. A case in point for the abnormality in the spreading of wildfire is that of Portugal in
2018 (NASA, 2018). The Scandinavian countries of Sweden and Norway have also been
exposed to abnormal patterns in 2018 (NASA, 2018).
The wildfires not only destroy vegetation cover, the destruction is often extended to
human life and property. This emphasizes on the need for prevention, containment and
mitigation of wildfires (Potera, 2009). The advancements in technology and data mining provide
an opportunity for better management of wildfires around the world.
3
INTRODUCTION
Wildfires have increasingly become frequent natural occurrences over the past decade.
These occurrence have been propagated by the intensity of the effects of climate change (Potera,
2009). Recent years have seen bigger, more aggressive and more sporadic wildfires in the
traditional fire zones such as California, Australia and Greece (NASA, 2018).
However, there has also been abnormal patterns in the occurrence and spread of
wildfires. A case in point for the abnormality in the spreading of wildfire is that of Portugal in
2018 (NASA, 2018). The Scandinavian countries of Sweden and Norway have also been
exposed to abnormal patterns in 2018 (NASA, 2018).
The wildfires not only destroy vegetation cover, the destruction is often extended to
human life and property. This emphasizes on the need for prevention, containment and
mitigation of wildfires (Potera, 2009). The advancements in technology and data mining provide
an opportunity for better management of wildfires around the world.
3
⊘ This is a preview!⊘
Do you want full access?
Subscribe today to unlock all pages.

Trusted by 1+ million students worldwide
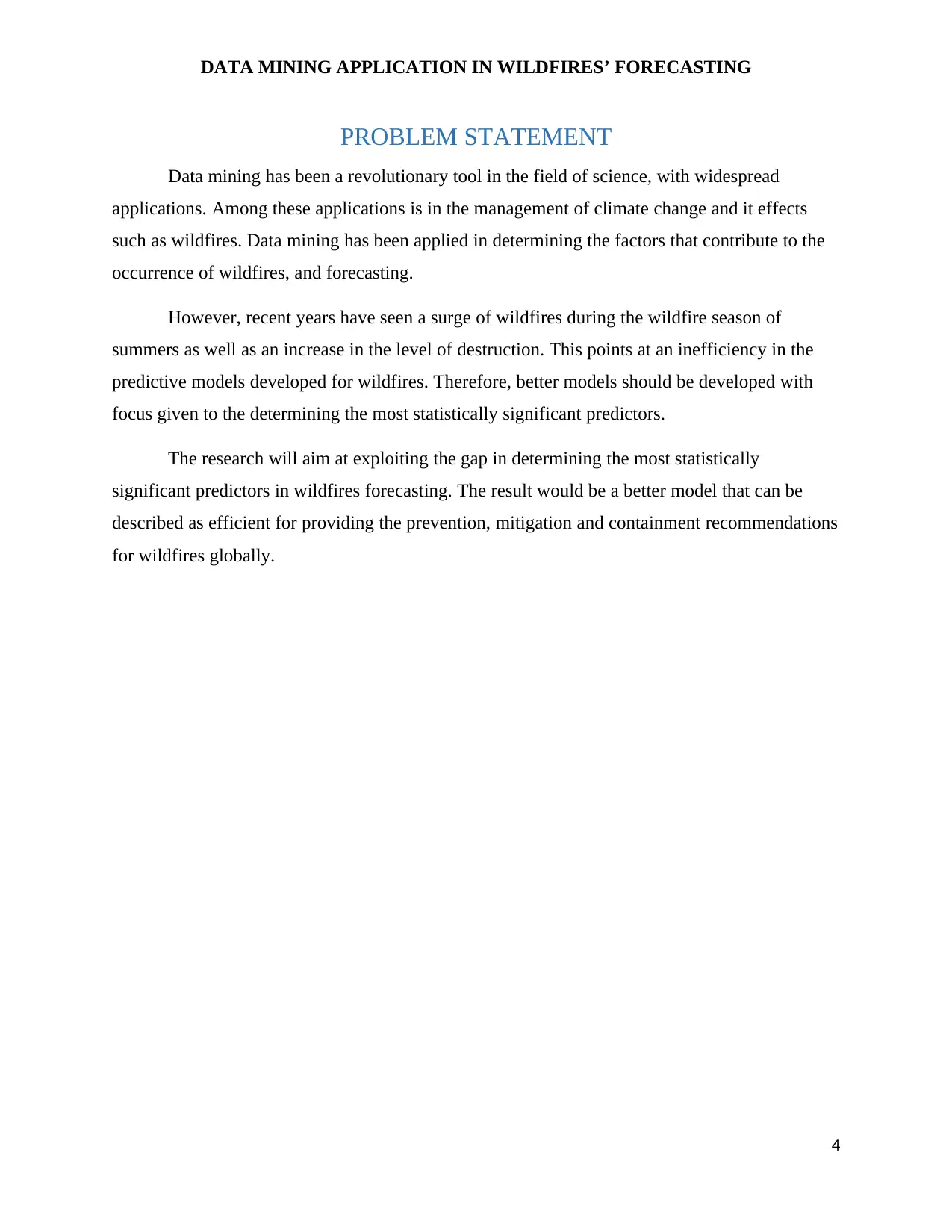
DATA MINING APPLICATION IN WILDFIRES’ FORECASTING
PROBLEM STATEMENT
Data mining has been a revolutionary tool in the field of science, with widespread
applications. Among these applications is in the management of climate change and it effects
such as wildfires. Data mining has been applied in determining the factors that contribute to the
occurrence of wildfires, and forecasting.
However, recent years have seen a surge of wildfires during the wildfire season of
summers as well as an increase in the level of destruction. This points at an inefficiency in the
predictive models developed for wildfires. Therefore, better models should be developed with
focus given to the determining the most statistically significant predictors.
The research will aim at exploiting the gap in determining the most statistically
significant predictors in wildfires forecasting. The result would be a better model that can be
described as efficient for providing the prevention, mitigation and containment recommendations
for wildfires globally.
4
PROBLEM STATEMENT
Data mining has been a revolutionary tool in the field of science, with widespread
applications. Among these applications is in the management of climate change and it effects
such as wildfires. Data mining has been applied in determining the factors that contribute to the
occurrence of wildfires, and forecasting.
However, recent years have seen a surge of wildfires during the wildfire season of
summers as well as an increase in the level of destruction. This points at an inefficiency in the
predictive models developed for wildfires. Therefore, better models should be developed with
focus given to the determining the most statistically significant predictors.
The research will aim at exploiting the gap in determining the most statistically
significant predictors in wildfires forecasting. The result would be a better model that can be
described as efficient for providing the prevention, mitigation and containment recommendations
for wildfires globally.
4
Paraphrase This Document
Need a fresh take? Get an instant paraphrase of this document with our AI Paraphraser
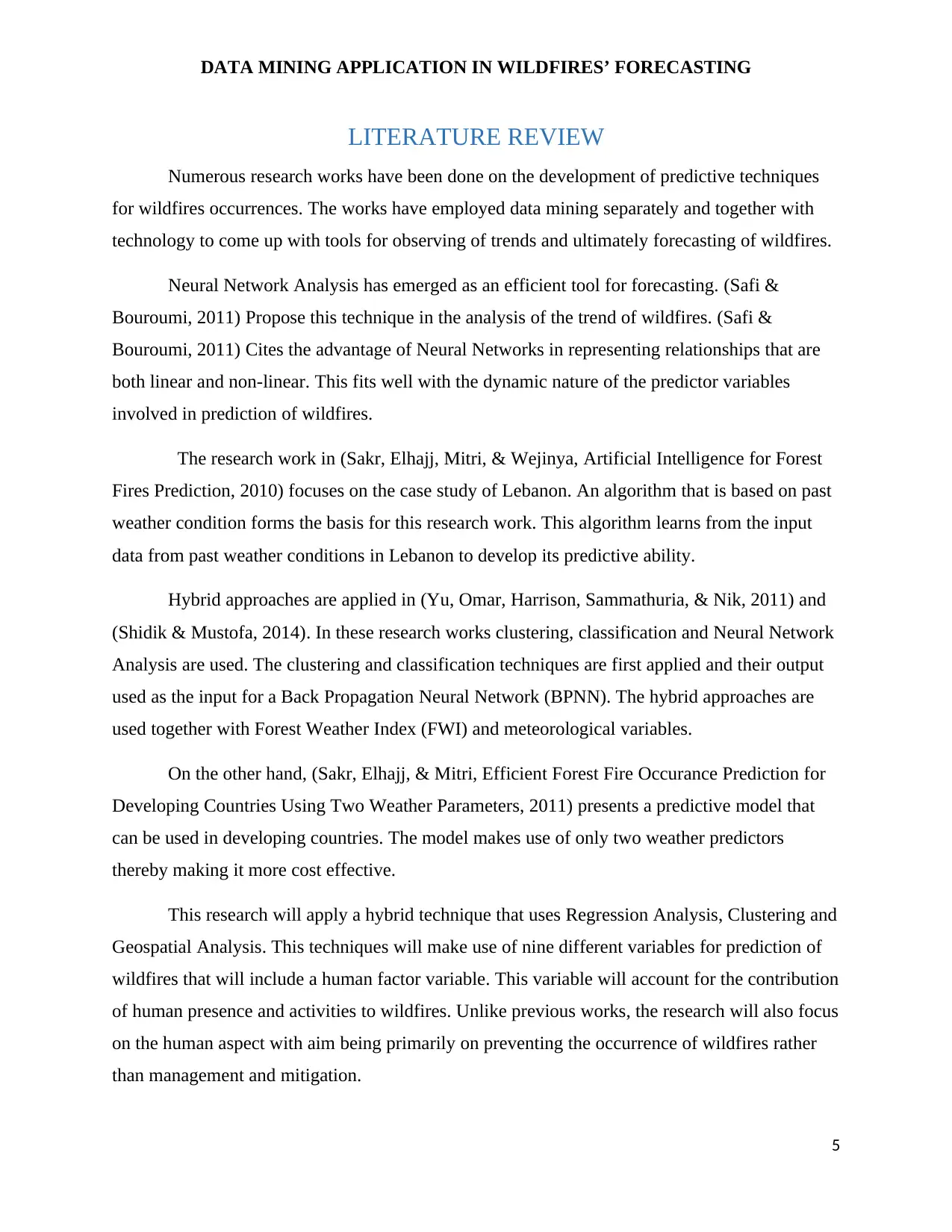
DATA MINING APPLICATION IN WILDFIRES’ FORECASTING
LITERATURE REVIEW
Numerous research works have been done on the development of predictive techniques
for wildfires occurrences. The works have employed data mining separately and together with
technology to come up with tools for observing of trends and ultimately forecasting of wildfires.
Neural Network Analysis has emerged as an efficient tool for forecasting. (Safi &
Bouroumi, 2011) Propose this technique in the analysis of the trend of wildfires. (Safi &
Bouroumi, 2011) Cites the advantage of Neural Networks in representing relationships that are
both linear and non-linear. This fits well with the dynamic nature of the predictor variables
involved in prediction of wildfires.
The research work in (Sakr, Elhajj, Mitri, & Wejinya, Artificial Intelligence for Forest
Fires Prediction, 2010) focuses on the case study of Lebanon. An algorithm that is based on past
weather condition forms the basis for this research work. This algorithm learns from the input
data from past weather conditions in Lebanon to develop its predictive ability.
Hybrid approaches are applied in (Yu, Omar, Harrison, Sammathuria, & Nik, 2011) and
(Shidik & Mustofa, 2014). In these research works clustering, classification and Neural Network
Analysis are used. The clustering and classification techniques are first applied and their output
used as the input for a Back Propagation Neural Network (BPNN). The hybrid approaches are
used together with Forest Weather Index (FWI) and meteorological variables.
On the other hand, (Sakr, Elhajj, & Mitri, Efficient Forest Fire Occurance Prediction for
Developing Countries Using Two Weather Parameters, 2011) presents a predictive model that
can be used in developing countries. The model makes use of only two weather predictors
thereby making it more cost effective.
This research will apply a hybrid technique that uses Regression Analysis, Clustering and
Geospatial Analysis. This techniques will make use of nine different variables for prediction of
wildfires that will include a human factor variable. This variable will account for the contribution
of human presence and activities to wildfires. Unlike previous works, the research will also focus
on the human aspect with aim being primarily on preventing the occurrence of wildfires rather
than management and mitigation.
5
LITERATURE REVIEW
Numerous research works have been done on the development of predictive techniques
for wildfires occurrences. The works have employed data mining separately and together with
technology to come up with tools for observing of trends and ultimately forecasting of wildfires.
Neural Network Analysis has emerged as an efficient tool for forecasting. (Safi &
Bouroumi, 2011) Propose this technique in the analysis of the trend of wildfires. (Safi &
Bouroumi, 2011) Cites the advantage of Neural Networks in representing relationships that are
both linear and non-linear. This fits well with the dynamic nature of the predictor variables
involved in prediction of wildfires.
The research work in (Sakr, Elhajj, Mitri, & Wejinya, Artificial Intelligence for Forest
Fires Prediction, 2010) focuses on the case study of Lebanon. An algorithm that is based on past
weather condition forms the basis for this research work. This algorithm learns from the input
data from past weather conditions in Lebanon to develop its predictive ability.
Hybrid approaches are applied in (Yu, Omar, Harrison, Sammathuria, & Nik, 2011) and
(Shidik & Mustofa, 2014). In these research works clustering, classification and Neural Network
Analysis are used. The clustering and classification techniques are first applied and their output
used as the input for a Back Propagation Neural Network (BPNN). The hybrid approaches are
used together with Forest Weather Index (FWI) and meteorological variables.
On the other hand, (Sakr, Elhajj, & Mitri, Efficient Forest Fire Occurance Prediction for
Developing Countries Using Two Weather Parameters, 2011) presents a predictive model that
can be used in developing countries. The model makes use of only two weather predictors
thereby making it more cost effective.
This research will apply a hybrid technique that uses Regression Analysis, Clustering and
Geospatial Analysis. This techniques will make use of nine different variables for prediction of
wildfires that will include a human factor variable. This variable will account for the contribution
of human presence and activities to wildfires. Unlike previous works, the research will also focus
on the human aspect with aim being primarily on preventing the occurrence of wildfires rather
than management and mitigation.
5
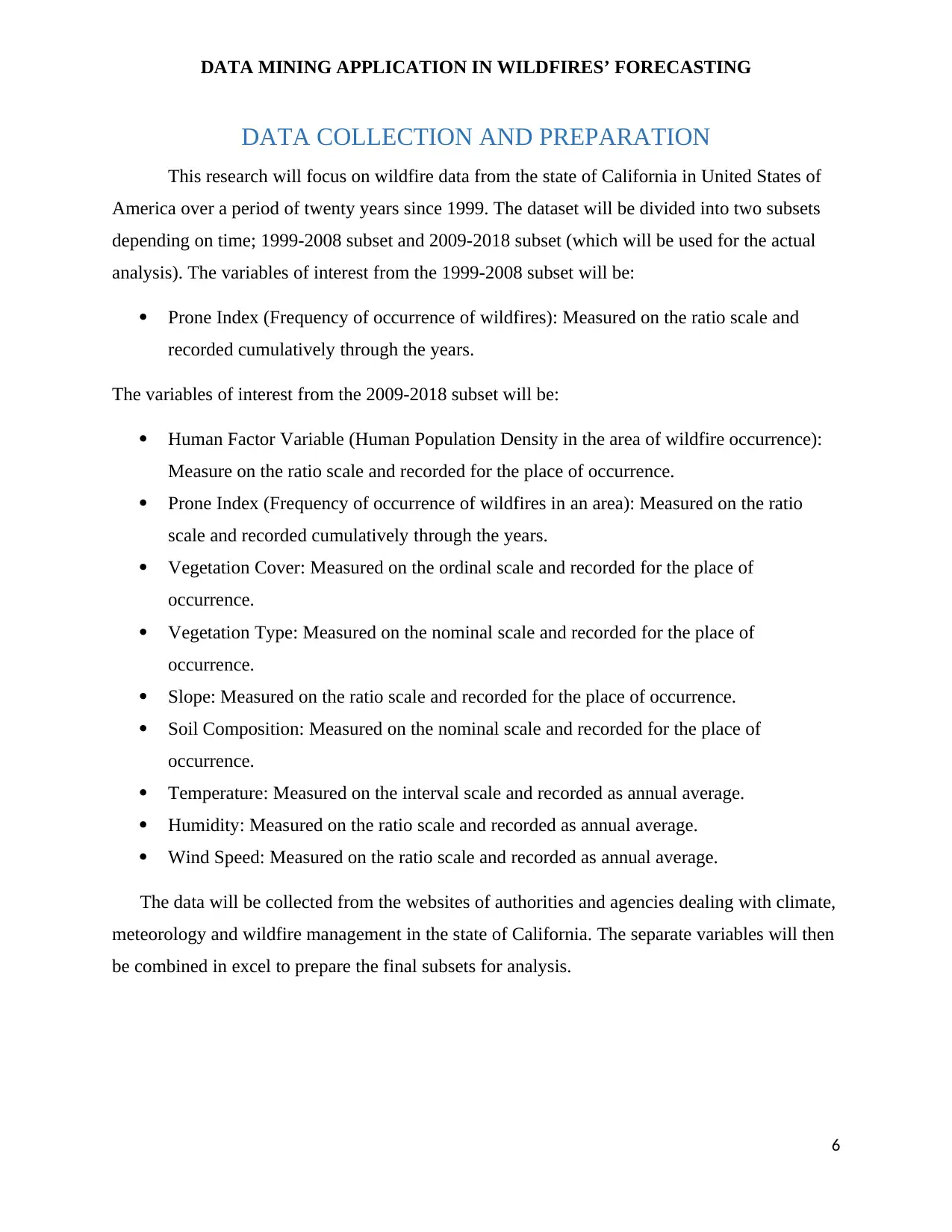
DATA MINING APPLICATION IN WILDFIRES’ FORECASTING
DATA COLLECTION AND PREPARATION
This research will focus on wildfire data from the state of California in United States of
America over a period of twenty years since 1999. The dataset will be divided into two subsets
depending on time; 1999-2008 subset and 2009-2018 subset (which will be used for the actual
analysis). The variables of interest from the 1999-2008 subset will be:
Prone Index (Frequency of occurrence of wildfires): Measured on the ratio scale and
recorded cumulatively through the years.
The variables of interest from the 2009-2018 subset will be:
Human Factor Variable (Human Population Density in the area of wildfire occurrence):
Measure on the ratio scale and recorded for the place of occurrence.
Prone Index (Frequency of occurrence of wildfires in an area): Measured on the ratio
scale and recorded cumulatively through the years.
Vegetation Cover: Measured on the ordinal scale and recorded for the place of
occurrence.
Vegetation Type: Measured on the nominal scale and recorded for the place of
occurrence.
Slope: Measured on the ratio scale and recorded for the place of occurrence.
Soil Composition: Measured on the nominal scale and recorded for the place of
occurrence.
Temperature: Measured on the interval scale and recorded as annual average.
Humidity: Measured on the ratio scale and recorded as annual average.
Wind Speed: Measured on the ratio scale and recorded as annual average.
The data will be collected from the websites of authorities and agencies dealing with climate,
meteorology and wildfire management in the state of California. The separate variables will then
be combined in excel to prepare the final subsets for analysis.
6
DATA COLLECTION AND PREPARATION
This research will focus on wildfire data from the state of California in United States of
America over a period of twenty years since 1999. The dataset will be divided into two subsets
depending on time; 1999-2008 subset and 2009-2018 subset (which will be used for the actual
analysis). The variables of interest from the 1999-2008 subset will be:
Prone Index (Frequency of occurrence of wildfires): Measured on the ratio scale and
recorded cumulatively through the years.
The variables of interest from the 2009-2018 subset will be:
Human Factor Variable (Human Population Density in the area of wildfire occurrence):
Measure on the ratio scale and recorded for the place of occurrence.
Prone Index (Frequency of occurrence of wildfires in an area): Measured on the ratio
scale and recorded cumulatively through the years.
Vegetation Cover: Measured on the ordinal scale and recorded for the place of
occurrence.
Vegetation Type: Measured on the nominal scale and recorded for the place of
occurrence.
Slope: Measured on the ratio scale and recorded for the place of occurrence.
Soil Composition: Measured on the nominal scale and recorded for the place of
occurrence.
Temperature: Measured on the interval scale and recorded as annual average.
Humidity: Measured on the ratio scale and recorded as annual average.
Wind Speed: Measured on the ratio scale and recorded as annual average.
The data will be collected from the websites of authorities and agencies dealing with climate,
meteorology and wildfire management in the state of California. The separate variables will then
be combined in excel to prepare the final subsets for analysis.
6
⊘ This is a preview!⊘
Do you want full access?
Subscribe today to unlock all pages.

Trusted by 1+ million students worldwide
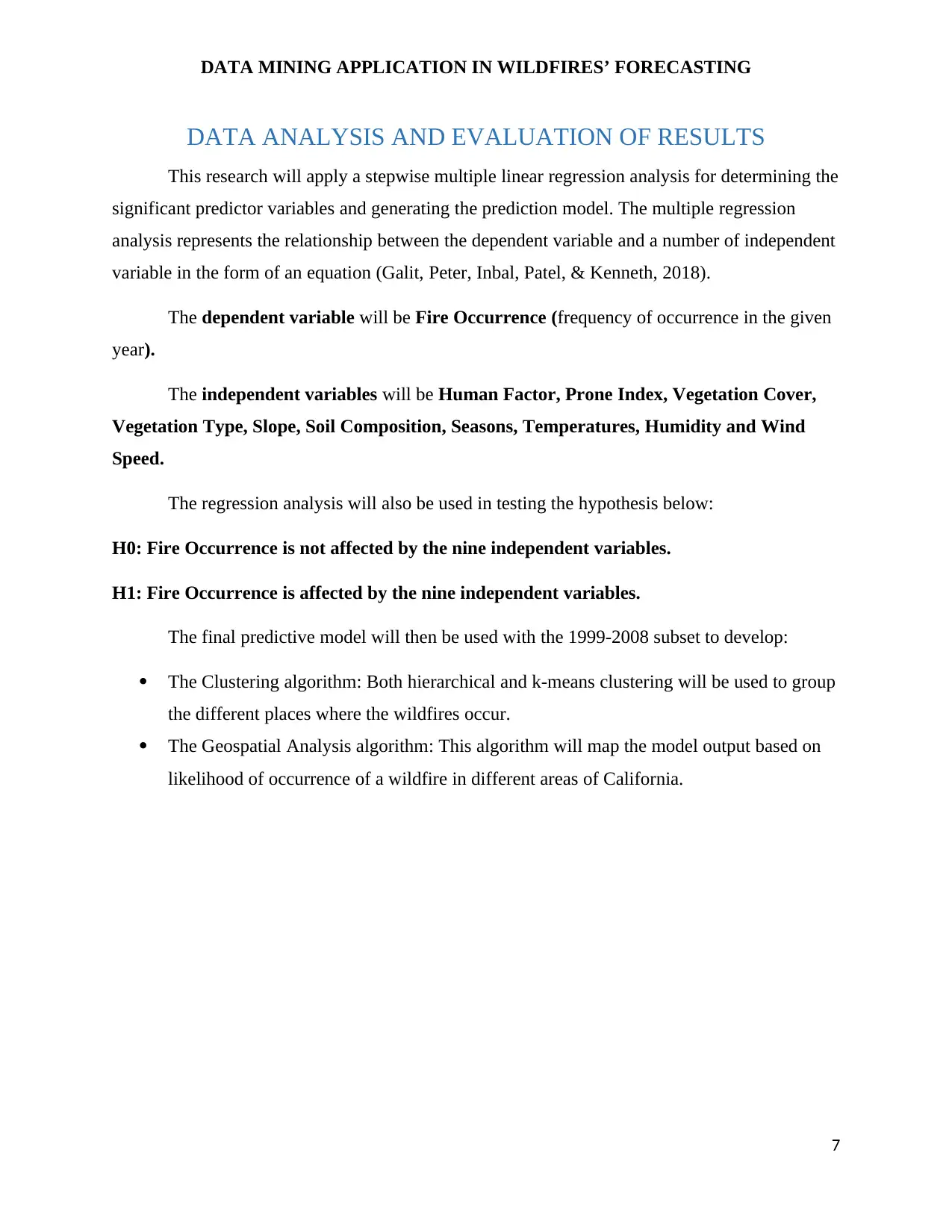
DATA MINING APPLICATION IN WILDFIRES’ FORECASTING
DATA ANALYSIS AND EVALUATION OF RESULTS
This research will apply a stepwise multiple linear regression analysis for determining the
significant predictor variables and generating the prediction model. The multiple regression
analysis represents the relationship between the dependent variable and a number of independent
variable in the form of an equation (Galit, Peter, Inbal, Patel, & Kenneth, 2018).
The dependent variable will be Fire Occurrence (frequency of occurrence in the given
year).
The independent variables will be Human Factor, Prone Index, Vegetation Cover,
Vegetation Type, Slope, Soil Composition, Seasons, Temperatures, Humidity and Wind
Speed.
The regression analysis will also be used in testing the hypothesis below:
H0: Fire Occurrence is not affected by the nine independent variables.
H1: Fire Occurrence is affected by the nine independent variables.
The final predictive model will then be used with the 1999-2008 subset to develop:
The Clustering algorithm: Both hierarchical and k-means clustering will be used to group
the different places where the wildfires occur.
The Geospatial Analysis algorithm: This algorithm will map the model output based on
likelihood of occurrence of a wildfire in different areas of California.
7
DATA ANALYSIS AND EVALUATION OF RESULTS
This research will apply a stepwise multiple linear regression analysis for determining the
significant predictor variables and generating the prediction model. The multiple regression
analysis represents the relationship between the dependent variable and a number of independent
variable in the form of an equation (Galit, Peter, Inbal, Patel, & Kenneth, 2018).
The dependent variable will be Fire Occurrence (frequency of occurrence in the given
year).
The independent variables will be Human Factor, Prone Index, Vegetation Cover,
Vegetation Type, Slope, Soil Composition, Seasons, Temperatures, Humidity and Wind
Speed.
The regression analysis will also be used in testing the hypothesis below:
H0: Fire Occurrence is not affected by the nine independent variables.
H1: Fire Occurrence is affected by the nine independent variables.
The final predictive model will then be used with the 1999-2008 subset to develop:
The Clustering algorithm: Both hierarchical and k-means clustering will be used to group
the different places where the wildfires occur.
The Geospatial Analysis algorithm: This algorithm will map the model output based on
likelihood of occurrence of a wildfire in different areas of California.
7
Paraphrase This Document
Need a fresh take? Get an instant paraphrase of this document with our AI Paraphraser
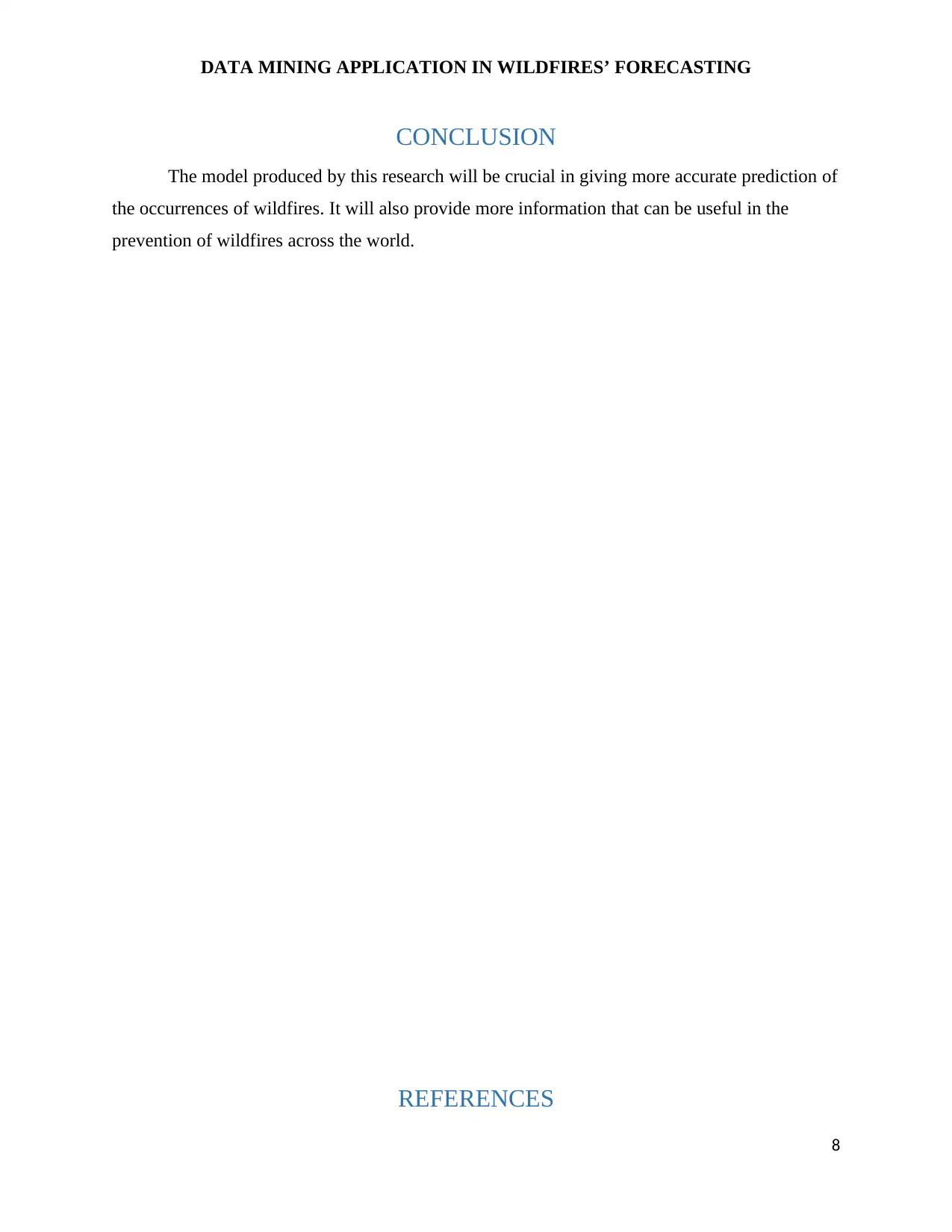
DATA MINING APPLICATION IN WILDFIRES’ FORECASTING
CONCLUSION
The model produced by this research will be crucial in giving more accurate prediction of
the occurrences of wildfires. It will also provide more information that can be useful in the
prevention of wildfires across the world.
REFERENCES
8
CONCLUSION
The model produced by this research will be crucial in giving more accurate prediction of
the occurrences of wildfires. It will also provide more information that can be useful in the
prevention of wildfires across the world.
REFERENCES
8
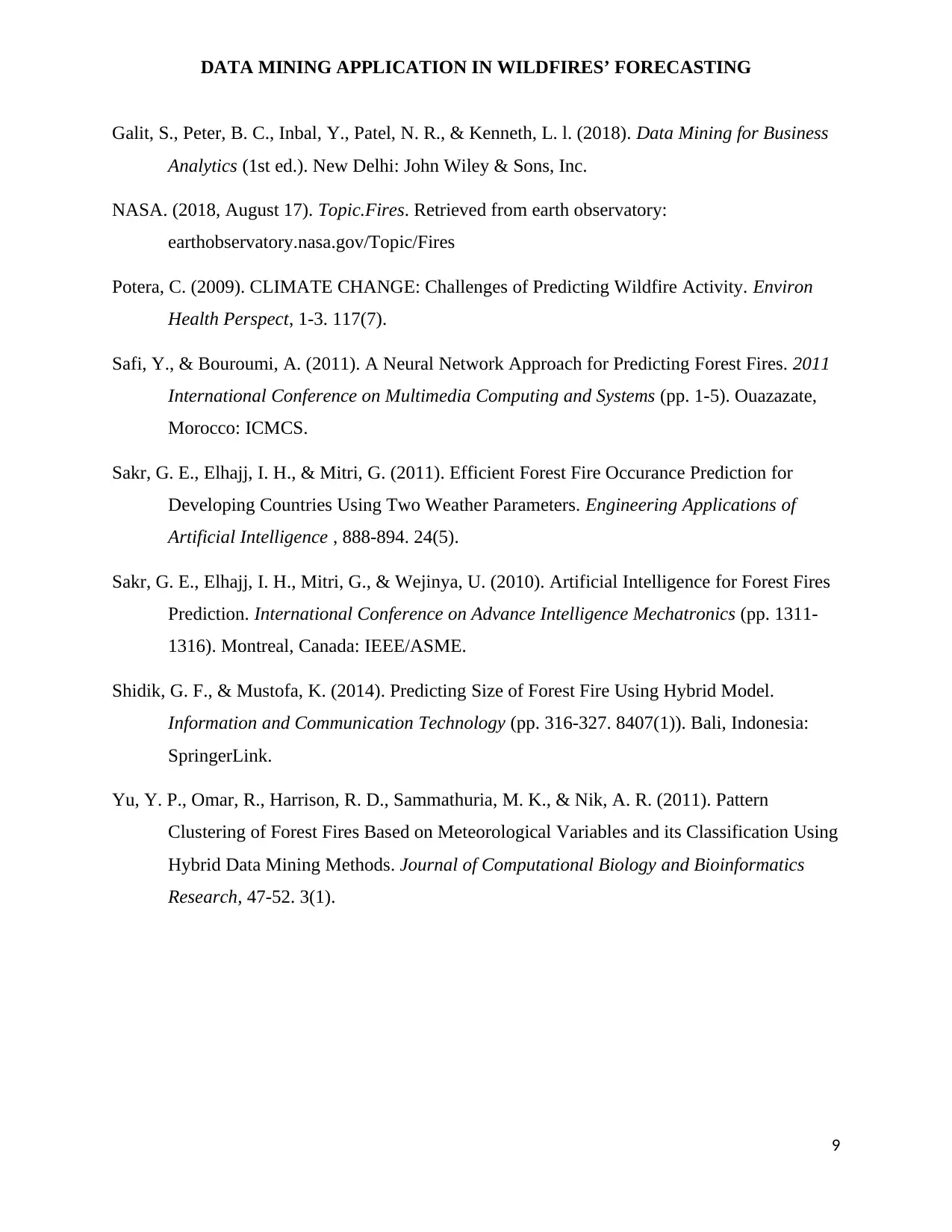
DATA MINING APPLICATION IN WILDFIRES’ FORECASTING
Galit, S., Peter, B. C., Inbal, Y., Patel, N. R., & Kenneth, L. l. (2018). Data Mining for Business
Analytics (1st ed.). New Delhi: John Wiley & Sons, Inc.
NASA. (2018, August 17). Topic.Fires. Retrieved from earth observatory:
earthobservatory.nasa.gov/Topic/Fires
Potera, C. (2009). CLIMATE CHANGE: Challenges of Predicting Wildfire Activity. Environ
Health Perspect, 1-3. 117(7).
Safi, Y., & Bouroumi, A. (2011). A Neural Network Approach for Predicting Forest Fires. 2011
International Conference on Multimedia Computing and Systems (pp. 1-5). Ouazazate,
Morocco: ICMCS.
Sakr, G. E., Elhajj, I. H., & Mitri, G. (2011). Efficient Forest Fire Occurance Prediction for
Developing Countries Using Two Weather Parameters. Engineering Applications of
Artificial Intelligence , 888-894. 24(5).
Sakr, G. E., Elhajj, I. H., Mitri, G., & Wejinya, U. (2010). Artificial Intelligence for Forest Fires
Prediction. International Conference on Advance Intelligence Mechatronics (pp. 1311-
1316). Montreal, Canada: IEEE/ASME.
Shidik, G. F., & Mustofa, K. (2014). Predicting Size of Forest Fire Using Hybrid Model.
Information and Communication Technology (pp. 316-327. 8407(1)). Bali, Indonesia:
SpringerLink.
Yu, Y. P., Omar, R., Harrison, R. D., Sammathuria, M. K., & Nik, A. R. (2011). Pattern
Clustering of Forest Fires Based on Meteorological Variables and its Classification Using
Hybrid Data Mining Methods. Journal of Computational Biology and Bioinformatics
Research, 47-52. 3(1).
9
Galit, S., Peter, B. C., Inbal, Y., Patel, N. R., & Kenneth, L. l. (2018). Data Mining for Business
Analytics (1st ed.). New Delhi: John Wiley & Sons, Inc.
NASA. (2018, August 17). Topic.Fires. Retrieved from earth observatory:
earthobservatory.nasa.gov/Topic/Fires
Potera, C. (2009). CLIMATE CHANGE: Challenges of Predicting Wildfire Activity. Environ
Health Perspect, 1-3. 117(7).
Safi, Y., & Bouroumi, A. (2011). A Neural Network Approach for Predicting Forest Fires. 2011
International Conference on Multimedia Computing and Systems (pp. 1-5). Ouazazate,
Morocco: ICMCS.
Sakr, G. E., Elhajj, I. H., & Mitri, G. (2011). Efficient Forest Fire Occurance Prediction for
Developing Countries Using Two Weather Parameters. Engineering Applications of
Artificial Intelligence , 888-894. 24(5).
Sakr, G. E., Elhajj, I. H., Mitri, G., & Wejinya, U. (2010). Artificial Intelligence for Forest Fires
Prediction. International Conference on Advance Intelligence Mechatronics (pp. 1311-
1316). Montreal, Canada: IEEE/ASME.
Shidik, G. F., & Mustofa, K. (2014). Predicting Size of Forest Fire Using Hybrid Model.
Information and Communication Technology (pp. 316-327. 8407(1)). Bali, Indonesia:
SpringerLink.
Yu, Y. P., Omar, R., Harrison, R. D., Sammathuria, M. K., & Nik, A. R. (2011). Pattern
Clustering of Forest Fires Based on Meteorological Variables and its Classification Using
Hybrid Data Mining Methods. Journal of Computational Biology and Bioinformatics
Research, 47-52. 3(1).
9
⊘ This is a preview!⊘
Do you want full access?
Subscribe today to unlock all pages.

Trusted by 1+ million students worldwide
1 out of 9

Your All-in-One AI-Powered Toolkit for Academic Success.
+13062052269
info@desklib.com
Available 24*7 on WhatsApp / Email
Unlock your academic potential
© 2024 | Zucol Services PVT LTD | All rights reserved.