Wireless Sensor Networks in Precision Agriculture for Improved Crop Production
VerifiedAdded on 2023/06/11
|6
|1504
|462
AI Summary
This article discusses the use of Wireless Sensor Networks (WSN) in precision agriculture for improved crop production. It covers the use of Long range radio (LoRa) wireless protocol, channel capacity calculation, noise-level calculations, and maintaining SNR 63 at control centre. It also talks about the application of cloud computing using sensor data in business and agriculture, and the implementation of WSN using IoT.
Contribute Materials
Your contribution can guide someone’s learning journey. Share your
documents today.
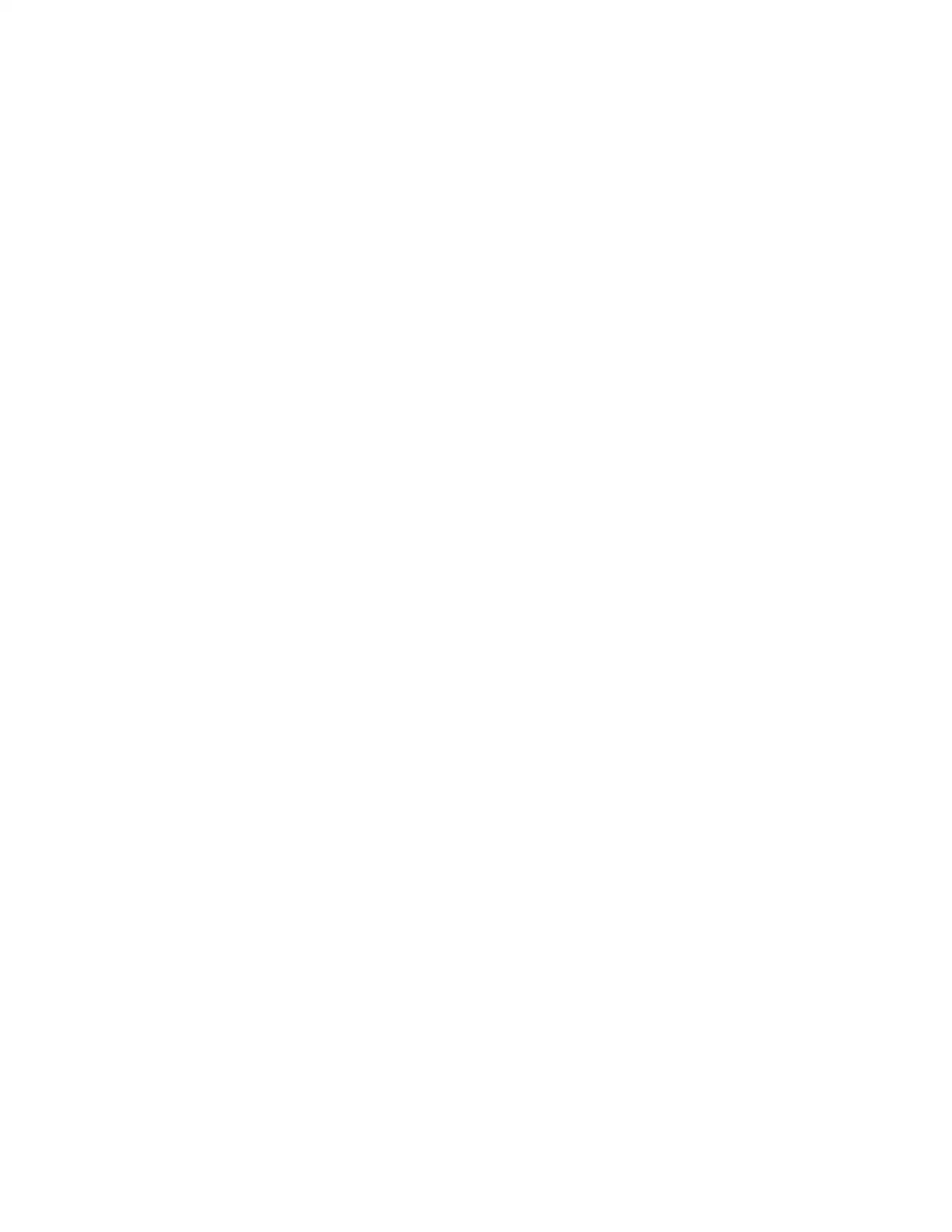
Secure Best Marks with AI Grader
Need help grading? Try our AI Grader for instant feedback on your assignments.
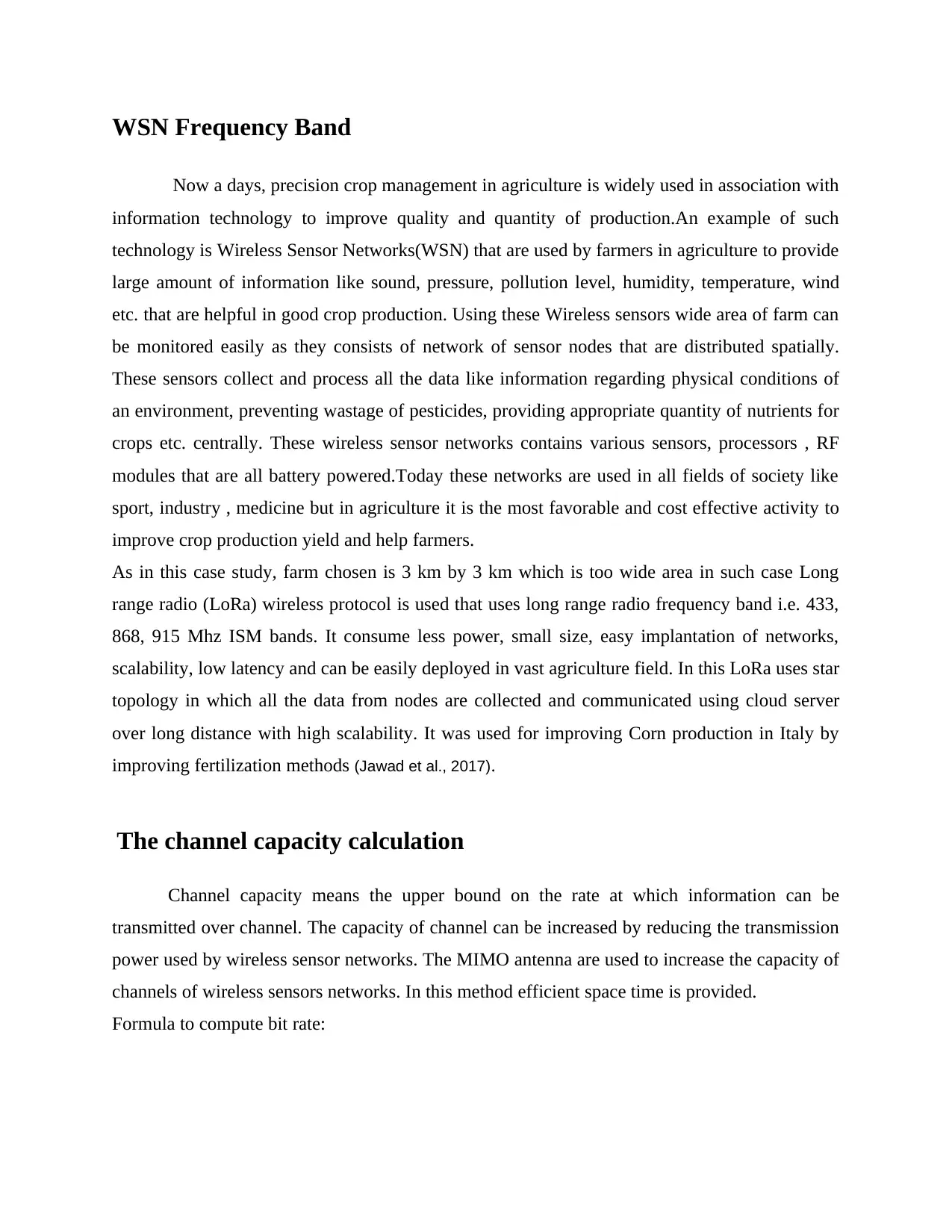
WSN Frequency Band
Now a days, precision crop management in agriculture is widely used in association with
information technology to improve quality and quantity of production.An example of such
technology is Wireless Sensor Networks(WSN) that are used by farmers in agriculture to provide
large amount of information like sound, pressure, pollution level, humidity, temperature, wind
etc. that are helpful in good crop production. Using these Wireless sensors wide area of farm can
be monitored easily as they consists of network of sensor nodes that are distributed spatially.
These sensors collect and process all the data like information regarding physical conditions of
an environment, preventing wastage of pesticides, providing appropriate quantity of nutrients for
crops etc. centrally. These wireless sensor networks contains various sensors, processors , RF
modules that are all battery powered.Today these networks are used in all fields of society like
sport, industry , medicine but in agriculture it is the most favorable and cost effective activity to
improve crop production yield and help farmers.
As in this case study, farm chosen is 3 km by 3 km which is too wide area in such case Long
range radio (LoRa) wireless protocol is used that uses long range radio frequency band i.e. 433,
868, 915 Mhz ISM bands. It consume less power, small size, easy implantation of networks,
scalability, low latency and can be easily deployed in vast agriculture field. In this LoRa uses star
topology in which all the data from nodes are collected and communicated using cloud server
over long distance with high scalability. It was used for improving Corn production in Italy by
improving fertilization methods (Jawad et al., 2017).
The channel capacity calculation
Channel capacity means the upper bound on the rate at which information can be
transmitted over channel. The capacity of channel can be increased by reducing the transmission
power used by wireless sensor networks. The MIMO antenna are used to increase the capacity of
channels of wireless sensors networks. In this method efficient space time is provided.
Formula to compute bit rate:
Now a days, precision crop management in agriculture is widely used in association with
information technology to improve quality and quantity of production.An example of such
technology is Wireless Sensor Networks(WSN) that are used by farmers in agriculture to provide
large amount of information like sound, pressure, pollution level, humidity, temperature, wind
etc. that are helpful in good crop production. Using these Wireless sensors wide area of farm can
be monitored easily as they consists of network of sensor nodes that are distributed spatially.
These sensors collect and process all the data like information regarding physical conditions of
an environment, preventing wastage of pesticides, providing appropriate quantity of nutrients for
crops etc. centrally. These wireless sensor networks contains various sensors, processors , RF
modules that are all battery powered.Today these networks are used in all fields of society like
sport, industry , medicine but in agriculture it is the most favorable and cost effective activity to
improve crop production yield and help farmers.
As in this case study, farm chosen is 3 km by 3 km which is too wide area in such case Long
range radio (LoRa) wireless protocol is used that uses long range radio frequency band i.e. 433,
868, 915 Mhz ISM bands. It consume less power, small size, easy implantation of networks,
scalability, low latency and can be easily deployed in vast agriculture field. In this LoRa uses star
topology in which all the data from nodes are collected and communicated using cloud server
over long distance with high scalability. It was used for improving Corn production in Italy by
improving fertilization methods (Jawad et al., 2017).
The channel capacity calculation
Channel capacity means the upper bound on the rate at which information can be
transmitted over channel. The capacity of channel can be increased by reducing the transmission
power used by wireless sensor networks. The MIMO antenna are used to increase the capacity of
channels of wireless sensors networks. In this method efficient space time is provided.
Formula to compute bit rate:
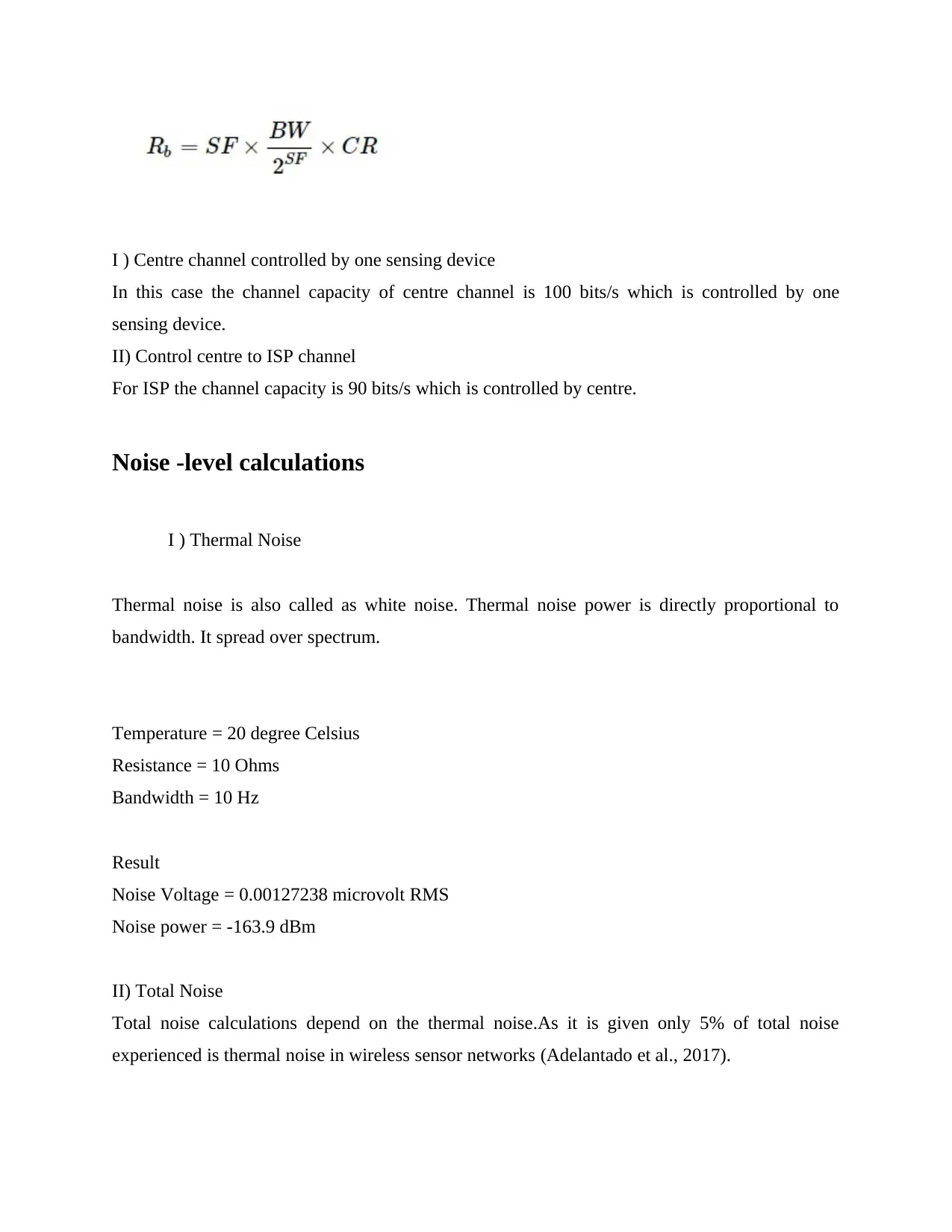
I ) Centre channel controlled by one sensing device
In this case the channel capacity of centre channel is 100 bits/s which is controlled by one
sensing device.
II) Control centre to ISP channel
For ISP the channel capacity is 90 bits/s which is controlled by centre.
Noise -level calculations
I ) Thermal Noise
Thermal noise is also called as white noise. Thermal noise power is directly proportional to
bandwidth. It spread over spectrum.
Temperature = 20 degree Celsius
Resistance = 10 Ohms
Bandwidth = 10 Hz
Result
Noise Voltage = 0.00127238 microvolt RMS
Noise power = -163.9 dBm
II) Total Noise
Total noise calculations depend on the thermal noise.As it is given only 5% of total noise
experienced is thermal noise in wireless sensor networks (Adelantado et al., 2017).
In this case the channel capacity of centre channel is 100 bits/s which is controlled by one
sensing device.
II) Control centre to ISP channel
For ISP the channel capacity is 90 bits/s which is controlled by centre.
Noise -level calculations
I ) Thermal Noise
Thermal noise is also called as white noise. Thermal noise power is directly proportional to
bandwidth. It spread over spectrum.
Temperature = 20 degree Celsius
Resistance = 10 Ohms
Bandwidth = 10 Hz
Result
Noise Voltage = 0.00127238 microvolt RMS
Noise power = -163.9 dBm
II) Total Noise
Total noise calculations depend on the thermal noise.As it is given only 5% of total noise
experienced is thermal noise in wireless sensor networks (Adelantado et al., 2017).
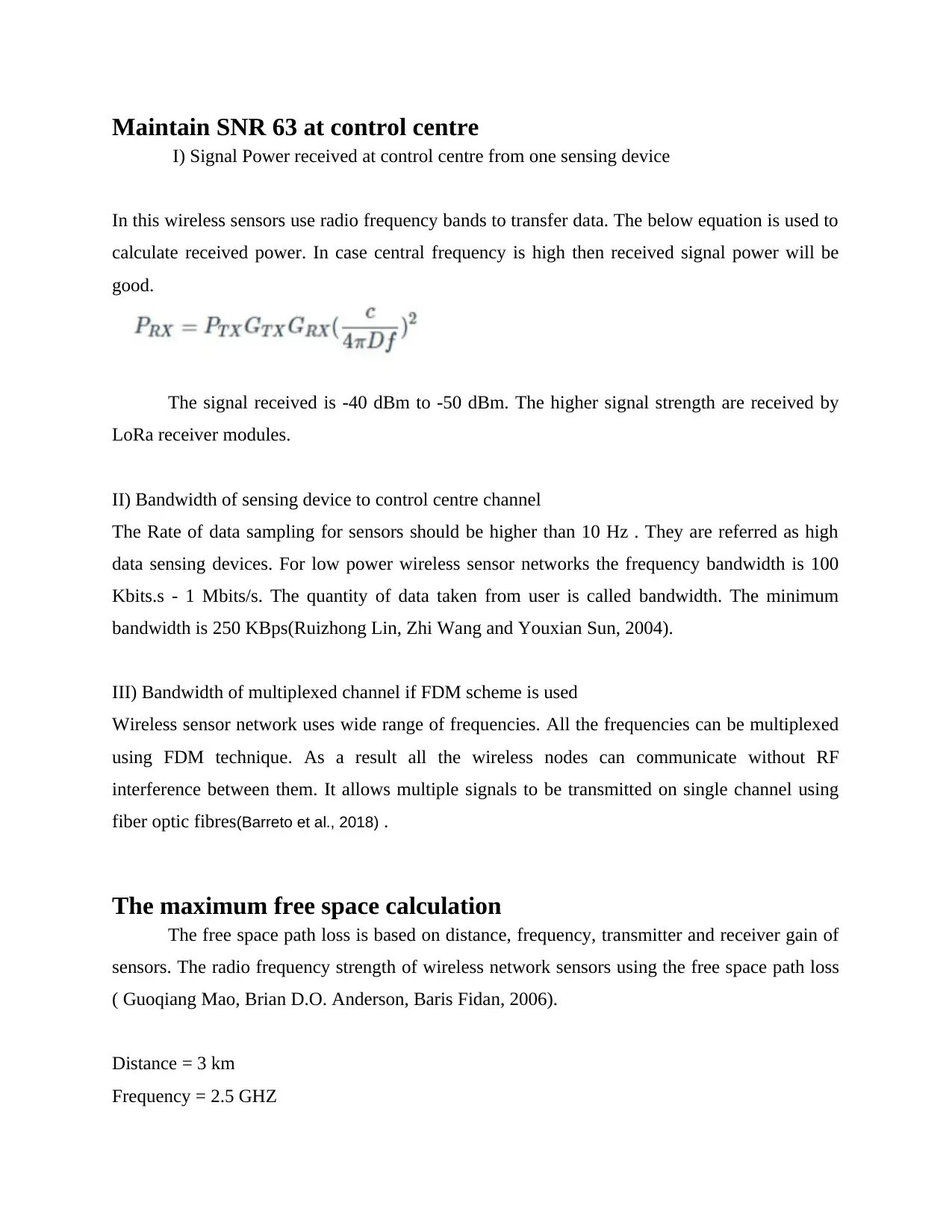
Maintain SNR 63 at control centre
I) Signal Power received at control centre from one sensing device
In this wireless sensors use radio frequency bands to transfer data. The below equation is used to
calculate received power. In case central frequency is high then received signal power will be
good.
The signal received is -40 dBm to -50 dBm. The higher signal strength are received by
LoRa receiver modules.
II) Bandwidth of sensing device to control centre channel
The Rate of data sampling for sensors should be higher than 10 Hz . They are referred as high
data sensing devices. For low power wireless sensor networks the frequency bandwidth is 100
Kbits.s - 1 Mbits/s. The quantity of data taken from user is called bandwidth. The minimum
bandwidth is 250 KBps(Ruizhong Lin, Zhi Wang and Youxian Sun, 2004).
III) Bandwidth of multiplexed channel if FDM scheme is used
Wireless sensor network uses wide range of frequencies. All the frequencies can be multiplexed
using FDM technique. As a result all the wireless nodes can communicate without RF
interference between them. It allows multiple signals to be transmitted on single channel using
fiber optic fibres(Barreto et al., 2018) .
The maximum free space calculation
The free space path loss is based on distance, frequency, transmitter and receiver gain of
sensors. The radio frequency strength of wireless network sensors using the free space path loss
( Guoqiang Mao, Brian D.O. Anderson, Baris Fidan, 2006).
Distance = 3 km
Frequency = 2.5 GHZ
I) Signal Power received at control centre from one sensing device
In this wireless sensors use radio frequency bands to transfer data. The below equation is used to
calculate received power. In case central frequency is high then received signal power will be
good.
The signal received is -40 dBm to -50 dBm. The higher signal strength are received by
LoRa receiver modules.
II) Bandwidth of sensing device to control centre channel
The Rate of data sampling for sensors should be higher than 10 Hz . They are referred as high
data sensing devices. For low power wireless sensor networks the frequency bandwidth is 100
Kbits.s - 1 Mbits/s. The quantity of data taken from user is called bandwidth. The minimum
bandwidth is 250 KBps(Ruizhong Lin, Zhi Wang and Youxian Sun, 2004).
III) Bandwidth of multiplexed channel if FDM scheme is used
Wireless sensor network uses wide range of frequencies. All the frequencies can be multiplexed
using FDM technique. As a result all the wireless nodes can communicate without RF
interference between them. It allows multiple signals to be transmitted on single channel using
fiber optic fibres(Barreto et al., 2018) .
The maximum free space calculation
The free space path loss is based on distance, frequency, transmitter and receiver gain of
sensors. The radio frequency strength of wireless network sensors using the free space path loss
( Guoqiang Mao, Brian D.O. Anderson, Baris Fidan, 2006).
Distance = 3 km
Frequency = 2.5 GHZ
Secure Best Marks with AI Grader
Need help grading? Try our AI Grader for instant feedback on your assignments.
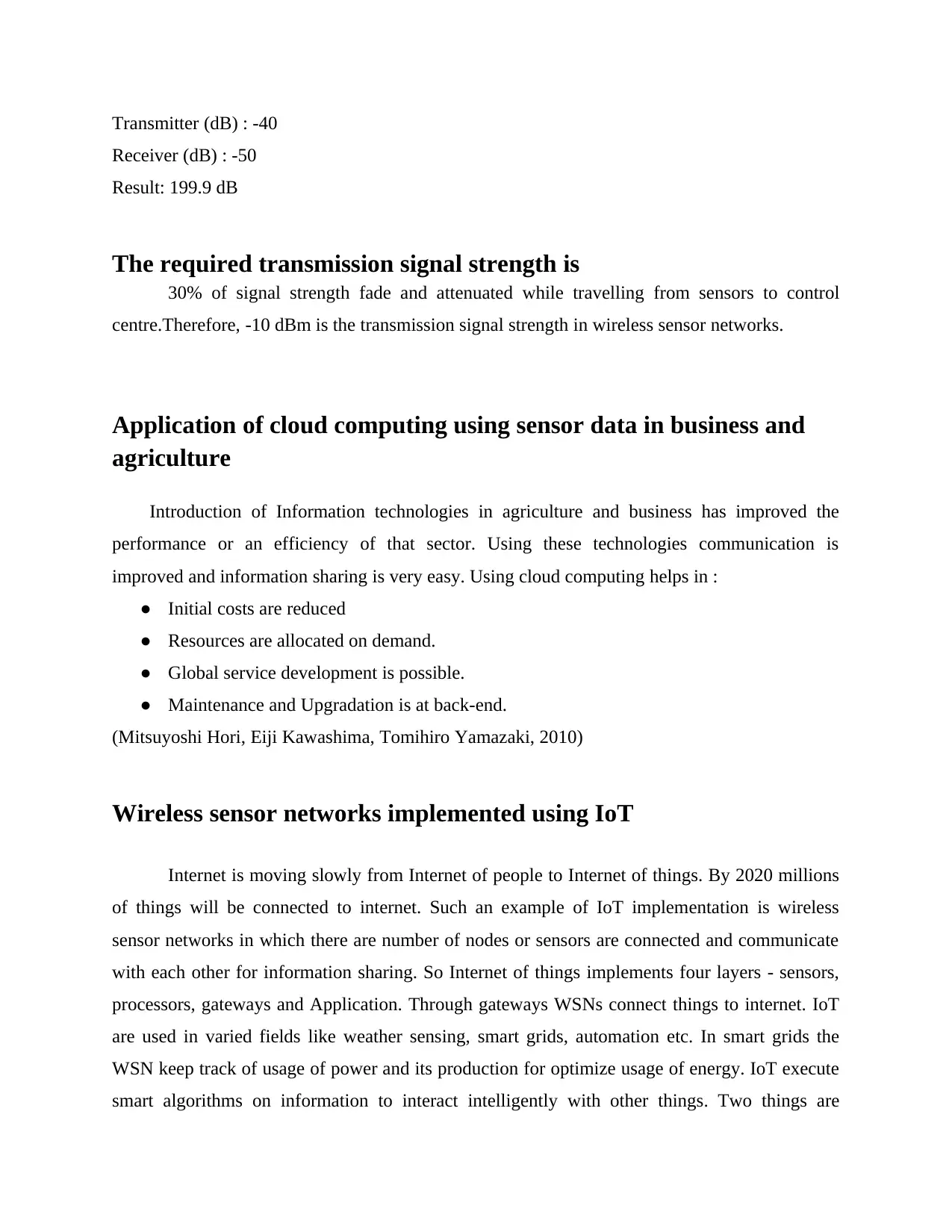
Transmitter (dB) : -40
Receiver (dB) : -50
Result: 199.9 dB
The required transmission signal strength is
30% of signal strength fade and attenuated while travelling from sensors to control
centre.Therefore, -10 dBm is the transmission signal strength in wireless sensor networks.
Application of cloud computing using sensor data in business and
agriculture
Introduction of Information technologies in agriculture and business has improved the
performance or an efficiency of that sector. Using these technologies communication is
improved and information sharing is very easy. Using cloud computing helps in :
● Initial costs are reduced
● Resources are allocated on demand.
● Global service development is possible.
● Maintenance and Upgradation is at back-end.
(Mitsuyoshi Hori, Eiji Kawashima, Tomihiro Yamazaki, 2010)
Wireless sensor networks implemented using IoT
Internet is moving slowly from Internet of people to Internet of things. By 2020 millions
of things will be connected to internet. Such an example of IoT implementation is wireless
sensor networks in which there are number of nodes or sensors are connected and communicate
with each other for information sharing. So Internet of things implements four layers - sensors,
processors, gateways and Application. Through gateways WSNs connect things to internet. IoT
are used in varied fields like weather sensing, smart grids, automation etc. In smart grids the
WSN keep track of usage of power and its production for optimize usage of energy. IoT execute
smart algorithms on information to interact intelligently with other things. Two things are
Receiver (dB) : -50
Result: 199.9 dB
The required transmission signal strength is
30% of signal strength fade and attenuated while travelling from sensors to control
centre.Therefore, -10 dBm is the transmission signal strength in wireless sensor networks.
Application of cloud computing using sensor data in business and
agriculture
Introduction of Information technologies in agriculture and business has improved the
performance or an efficiency of that sector. Using these technologies communication is
improved and information sharing is very easy. Using cloud computing helps in :
● Initial costs are reduced
● Resources are allocated on demand.
● Global service development is possible.
● Maintenance and Upgradation is at back-end.
(Mitsuyoshi Hori, Eiji Kawashima, Tomihiro Yamazaki, 2010)
Wireless sensor networks implemented using IoT
Internet is moving slowly from Internet of people to Internet of things. By 2020 millions
of things will be connected to internet. Such an example of IoT implementation is wireless
sensor networks in which there are number of nodes or sensors are connected and communicate
with each other for information sharing. So Internet of things implements four layers - sensors,
processors, gateways and Application. Through gateways WSNs connect things to internet. IoT
are used in varied fields like weather sensing, smart grids, automation etc. In smart grids the
WSN keep track of usage of power and its production for optimize usage of energy. IoT execute
smart algorithms on information to interact intelligently with other things. Two things are
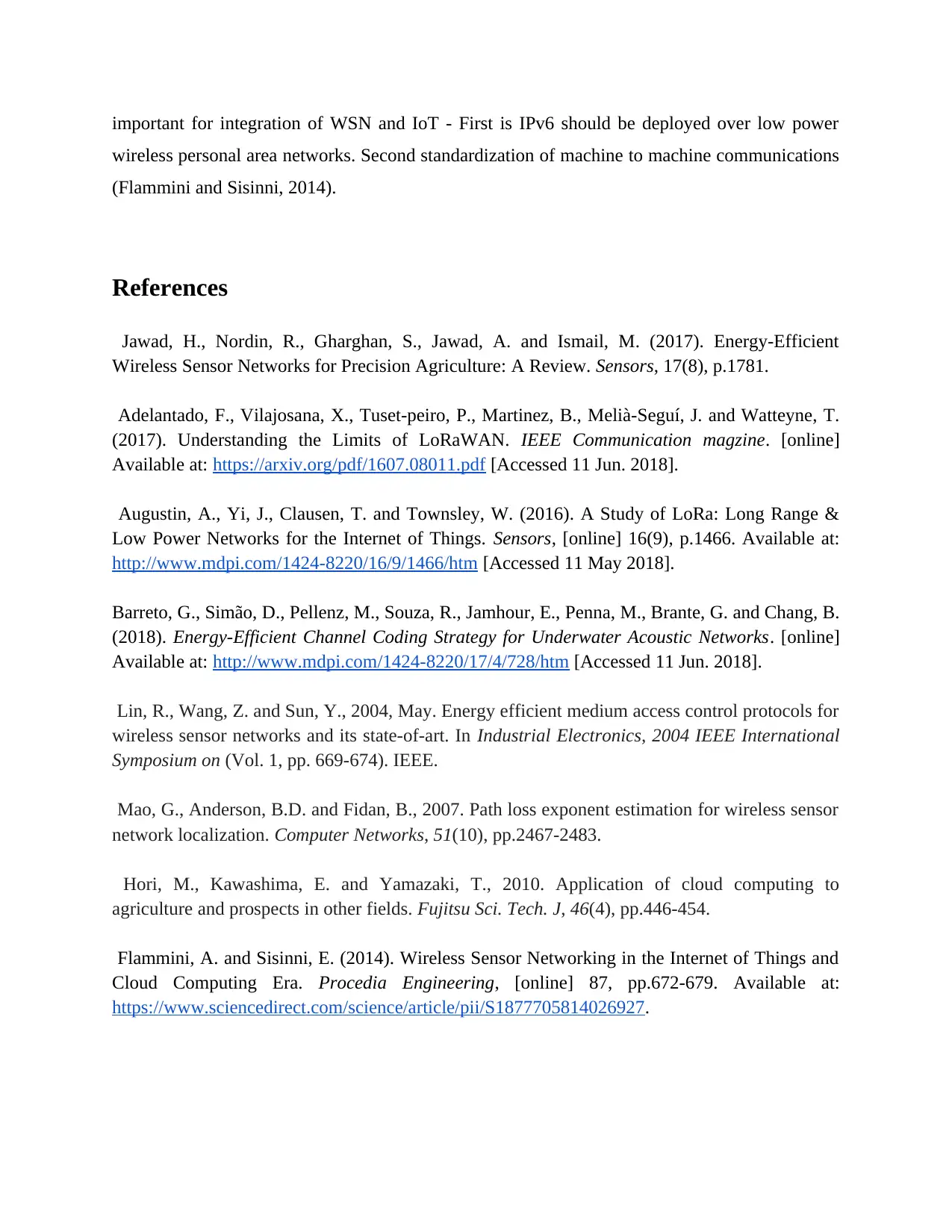
important for integration of WSN and IoT - First is IPv6 should be deployed over low power
wireless personal area networks. Second standardization of machine to machine communications
(Flammini and Sisinni, 2014).
References
Jawad, H., Nordin, R., Gharghan, S., Jawad, A. and Ismail, M. (2017). Energy-Efficient
Wireless Sensor Networks for Precision Agriculture: A Review. Sensors, 17(8), p.1781.
Adelantado, F., Vilajosana, X., Tuset-peiro, P., Martinez, B., Melià-Seguí, J. and Watteyne, T.
(2017). Understanding the Limits of LoRaWAN. IEEE Communication magzine. [online]
Available at: https://arxiv.org/pdf/1607.08011.pdf [Accessed 11 Jun. 2018].
Augustin, A., Yi, J., Clausen, T. and Townsley, W. (2016). A Study of LoRa: Long Range &
Low Power Networks for the Internet of Things. Sensors, [online] 16(9), p.1466. Available at:
http://www.mdpi.com/1424-8220/16/9/1466/htm [Accessed 11 May 2018].
Barreto, G., Simão, D., Pellenz, M., Souza, R., Jamhour, E., Penna, M., Brante, G. and Chang, B.
(2018). Energy-Efficient Channel Coding Strategy for Underwater Acoustic Networks. [online]
Available at: http://www.mdpi.com/1424-8220/17/4/728/htm [Accessed 11 Jun. 2018].
Lin, R., Wang, Z. and Sun, Y., 2004, May. Energy efficient medium access control protocols for
wireless sensor networks and its state-of-art. In Industrial Electronics, 2004 IEEE International
Symposium on (Vol. 1, pp. 669-674). IEEE.
Mao, G., Anderson, B.D. and Fidan, B., 2007. Path loss exponent estimation for wireless sensor
network localization. Computer Networks, 51(10), pp.2467-2483.
Hori, M., Kawashima, E. and Yamazaki, T., 2010. Application of cloud computing to
agriculture and prospects in other fields. Fujitsu Sci. Tech. J, 46(4), pp.446-454.
Flammini, A. and Sisinni, E. (2014). Wireless Sensor Networking in the Internet of Things and
Cloud Computing Era. Procedia Engineering, [online] 87, pp.672-679. Available at:
https://www.sciencedirect.com/science/article/pii/S1877705814026927.
wireless personal area networks. Second standardization of machine to machine communications
(Flammini and Sisinni, 2014).
References
Jawad, H., Nordin, R., Gharghan, S., Jawad, A. and Ismail, M. (2017). Energy-Efficient
Wireless Sensor Networks for Precision Agriculture: A Review. Sensors, 17(8), p.1781.
Adelantado, F., Vilajosana, X., Tuset-peiro, P., Martinez, B., Melià-Seguí, J. and Watteyne, T.
(2017). Understanding the Limits of LoRaWAN. IEEE Communication magzine. [online]
Available at: https://arxiv.org/pdf/1607.08011.pdf [Accessed 11 Jun. 2018].
Augustin, A., Yi, J., Clausen, T. and Townsley, W. (2016). A Study of LoRa: Long Range &
Low Power Networks for the Internet of Things. Sensors, [online] 16(9), p.1466. Available at:
http://www.mdpi.com/1424-8220/16/9/1466/htm [Accessed 11 May 2018].
Barreto, G., Simão, D., Pellenz, M., Souza, R., Jamhour, E., Penna, M., Brante, G. and Chang, B.
(2018). Energy-Efficient Channel Coding Strategy for Underwater Acoustic Networks. [online]
Available at: http://www.mdpi.com/1424-8220/17/4/728/htm [Accessed 11 Jun. 2018].
Lin, R., Wang, Z. and Sun, Y., 2004, May. Energy efficient medium access control protocols for
wireless sensor networks and its state-of-art. In Industrial Electronics, 2004 IEEE International
Symposium on (Vol. 1, pp. 669-674). IEEE.
Mao, G., Anderson, B.D. and Fidan, B., 2007. Path loss exponent estimation for wireless sensor
network localization. Computer Networks, 51(10), pp.2467-2483.
Hori, M., Kawashima, E. and Yamazaki, T., 2010. Application of cloud computing to
agriculture and prospects in other fields. Fujitsu Sci. Tech. J, 46(4), pp.446-454.
Flammini, A. and Sisinni, E. (2014). Wireless Sensor Networking in the Internet of Things and
Cloud Computing Era. Procedia Engineering, [online] 87, pp.672-679. Available at:
https://www.sciencedirect.com/science/article/pii/S1877705814026927.
1 out of 6
Related Documents
![[object Object]](/_next/image/?url=%2F_next%2Fstatic%2Fmedia%2Flogo.6d15ce61.png&w=640&q=75)
Your All-in-One AI-Powered Toolkit for Academic Success.
+13062052269
info@desklib.com
Available 24*7 on WhatsApp / Email
Unlock your academic potential
© 2024 | Zucol Services PVT LTD | All rights reserved.