Business Understanding Report: AIH College Data Mining Project
VerifiedAdded on 2019/10/31
|10
|2653
|283
Report
AI Summary
This report analyzes a data mining project for AIH College, focusing on the "We can pay you to study now" initiative. The report begins with an introduction to data mining and its application in business understanding, emphasizing the CRISP-DM model. It details the business objectives of AIH, including improving student enrollment and providing financial assistance. The assessment of the situation covers resource inventory, data sources, and project constraints, including ethical and legal considerations. The report also outlines the project's schedule, assumptions, and potential risks. The data mining process involves understanding business objectives, assessing the situation, establishing data mining goals, and presenting a project plan to the top management. The report recommends a repetitive data mining process to ensure the initiative's sustainability, providing insights into market demographics and student performance to aid in marketing and financial program development.
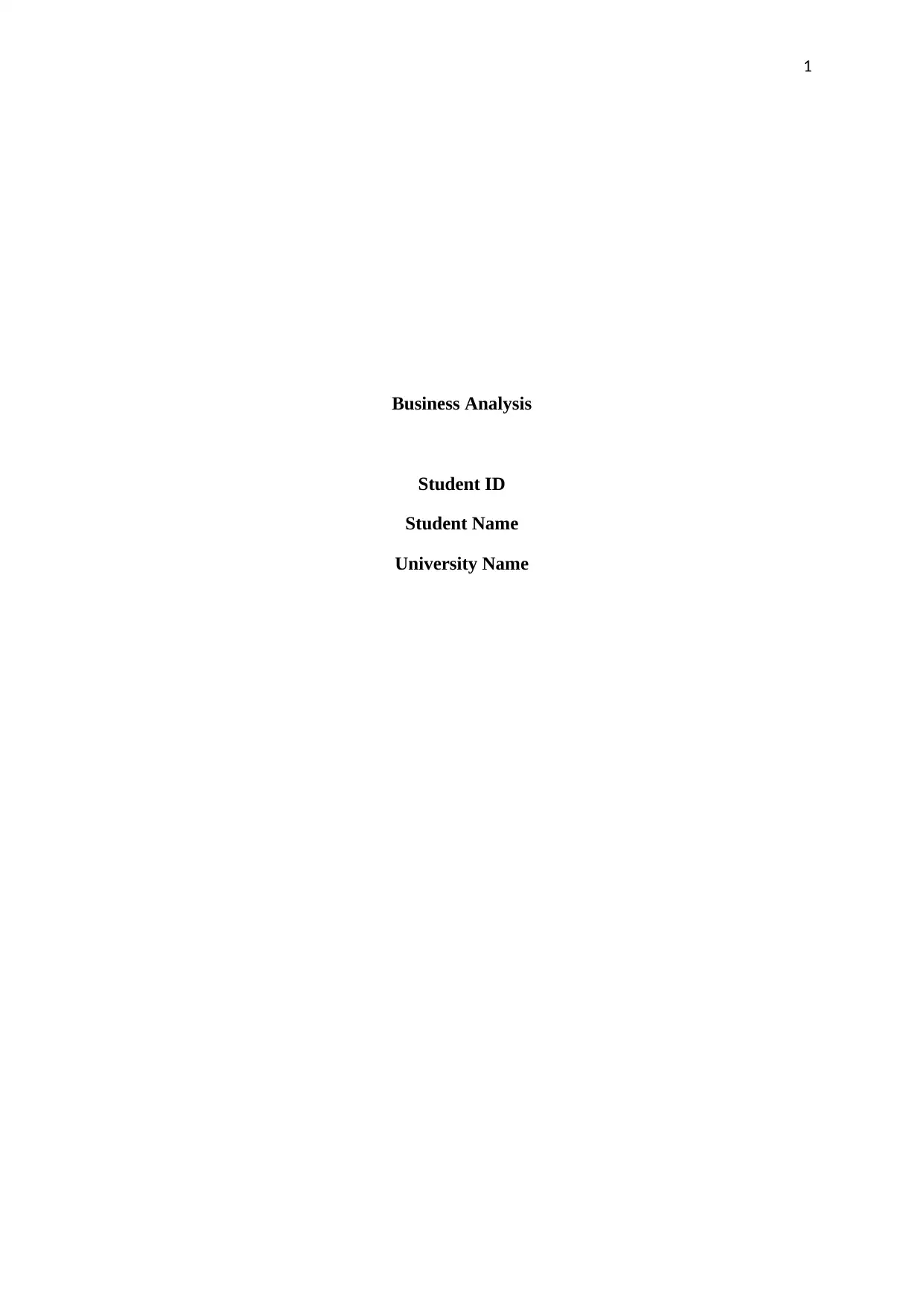
1
Business Analysis
Student ID
Student Name
University Name
Business Analysis
Student ID
Student Name
University Name
Secure Best Marks with AI Grader
Need help grading? Try our AI Grader for instant feedback on your assignments.
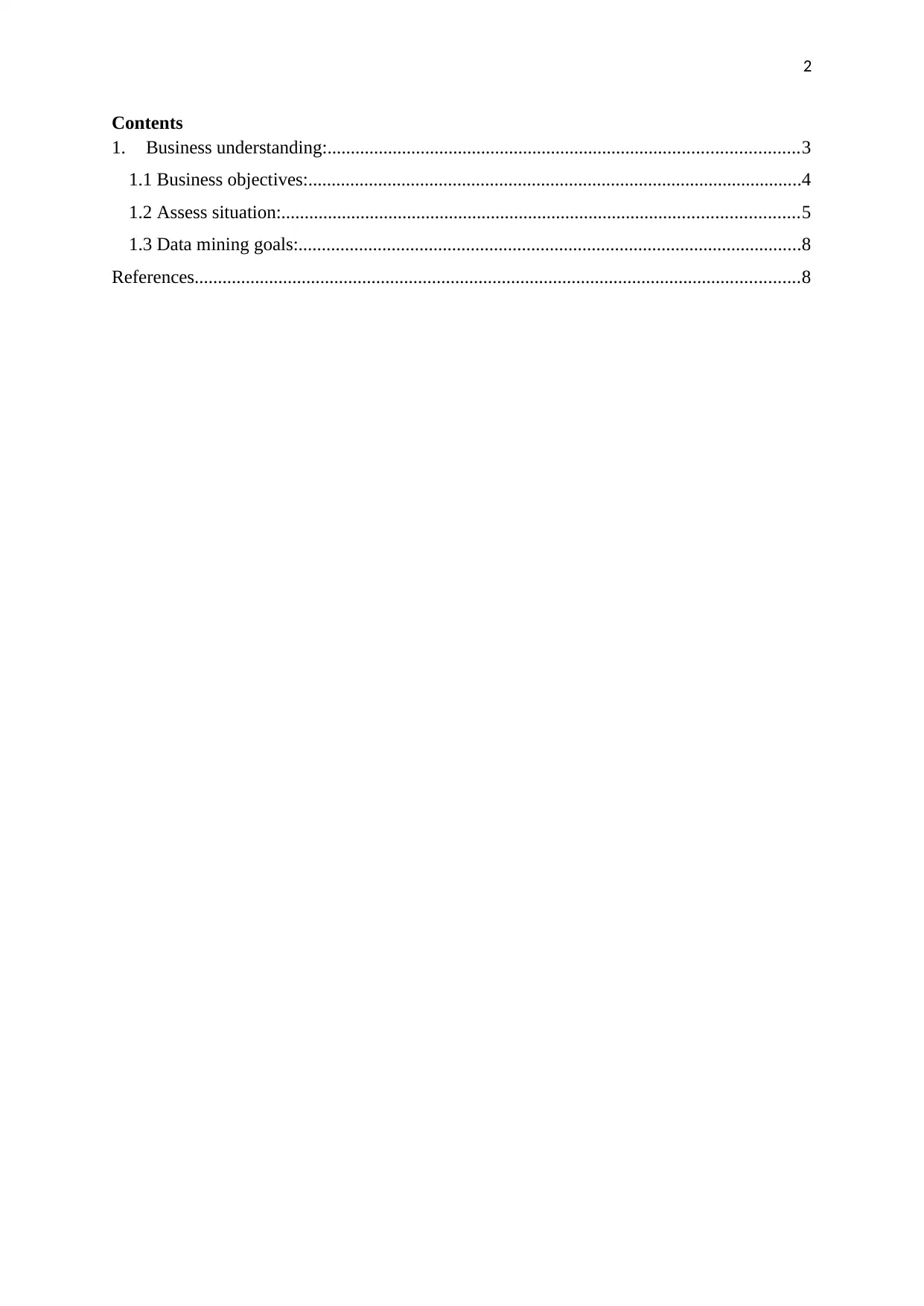
2
Contents
1. Business understanding:.....................................................................................................3
1.1 Business objectives:..........................................................................................................4
1.2 Assess situation:...............................................................................................................5
1.3 Data mining goals:............................................................................................................8
References..................................................................................................................................8
Contents
1. Business understanding:.....................................................................................................3
1.1 Business objectives:..........................................................................................................4
1.2 Assess situation:...............................................................................................................5
1.3 Data mining goals:............................................................................................................8
References..................................................................................................................................8
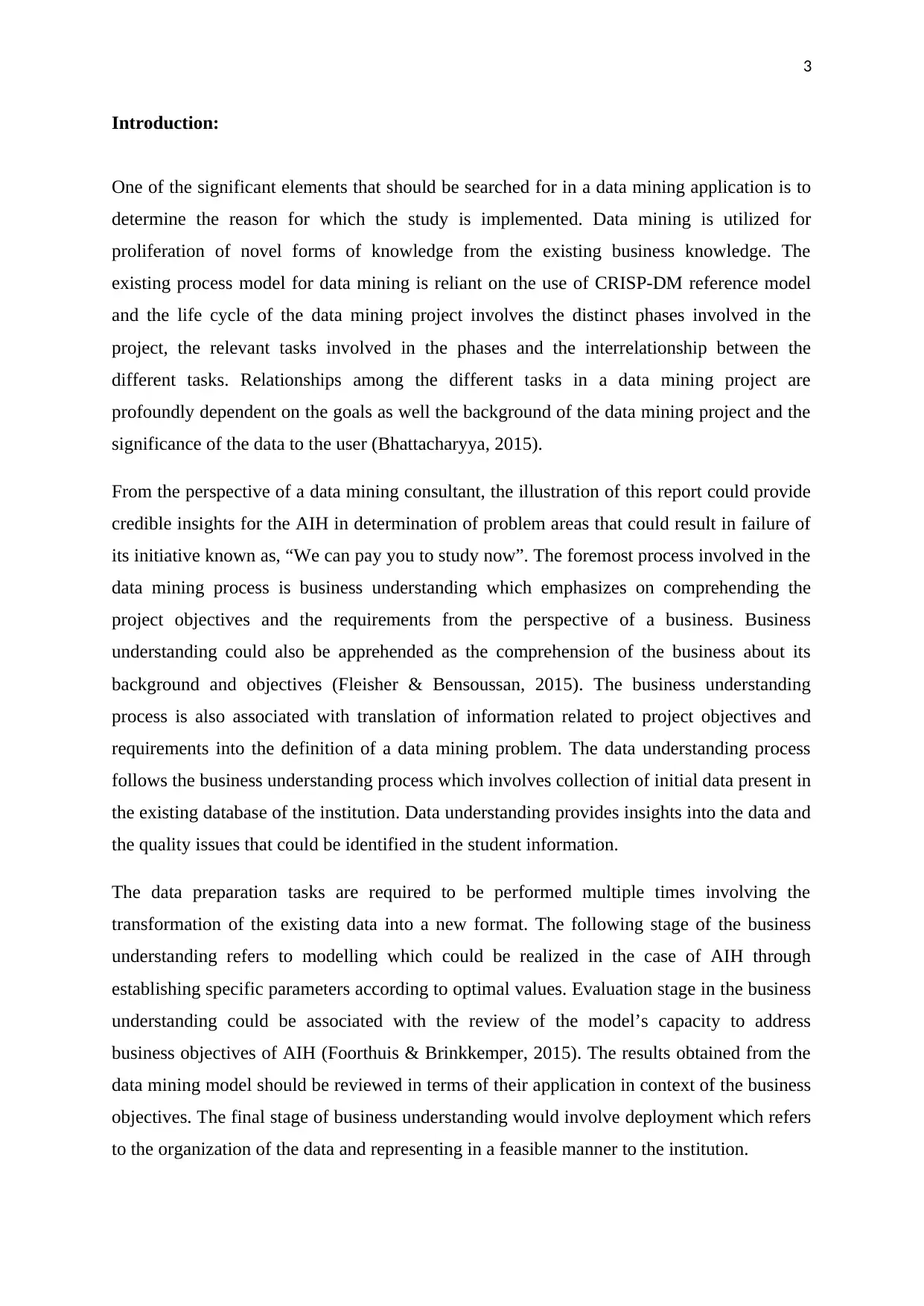
3
Introduction:
One of the significant elements that should be searched for in a data mining application is to
determine the reason for which the study is implemented. Data mining is utilized for
proliferation of novel forms of knowledge from the existing business knowledge. The
existing process model for data mining is reliant on the use of CRISP-DM reference model
and the life cycle of the data mining project involves the distinct phases involved in the
project, the relevant tasks involved in the phases and the interrelationship between the
different tasks. Relationships among the different tasks in a data mining project are
profoundly dependent on the goals as well the background of the data mining project and the
significance of the data to the user (Bhattacharyya, 2015).
From the perspective of a data mining consultant, the illustration of this report could provide
credible insights for the AIH in determination of problem areas that could result in failure of
its initiative known as, “We can pay you to study now”. The foremost process involved in the
data mining process is business understanding which emphasizes on comprehending the
project objectives and the requirements from the perspective of a business. Business
understanding could also be apprehended as the comprehension of the business about its
background and objectives (Fleisher & Bensoussan, 2015). The business understanding
process is also associated with translation of information related to project objectives and
requirements into the definition of a data mining problem. The data understanding process
follows the business understanding process which involves collection of initial data present in
the existing database of the institution. Data understanding provides insights into the data and
the quality issues that could be identified in the student information.
The data preparation tasks are required to be performed multiple times involving the
transformation of the existing data into a new format. The following stage of the business
understanding refers to modelling which could be realized in the case of AIH through
establishing specific parameters according to optimal values. Evaluation stage in the business
understanding could be associated with the review of the model’s capacity to address
business objectives of AIH (Foorthuis & Brinkkemper, 2015). The results obtained from the
data mining model should be reviewed in terms of their application in context of the business
objectives. The final stage of business understanding would involve deployment which refers
to the organization of the data and representing in a feasible manner to the institution.
Introduction:
One of the significant elements that should be searched for in a data mining application is to
determine the reason for which the study is implemented. Data mining is utilized for
proliferation of novel forms of knowledge from the existing business knowledge. The
existing process model for data mining is reliant on the use of CRISP-DM reference model
and the life cycle of the data mining project involves the distinct phases involved in the
project, the relevant tasks involved in the phases and the interrelationship between the
different tasks. Relationships among the different tasks in a data mining project are
profoundly dependent on the goals as well the background of the data mining project and the
significance of the data to the user (Bhattacharyya, 2015).
From the perspective of a data mining consultant, the illustration of this report could provide
credible insights for the AIH in determination of problem areas that could result in failure of
its initiative known as, “We can pay you to study now”. The foremost process involved in the
data mining process is business understanding which emphasizes on comprehending the
project objectives and the requirements from the perspective of a business. Business
understanding could also be apprehended as the comprehension of the business about its
background and objectives (Fleisher & Bensoussan, 2015). The business understanding
process is also associated with translation of information related to project objectives and
requirements into the definition of a data mining problem. The data understanding process
follows the business understanding process which involves collection of initial data present in
the existing database of the institution. Data understanding provides insights into the data and
the quality issues that could be identified in the student information.
The data preparation tasks are required to be performed multiple times involving the
transformation of the existing data into a new format. The following stage of the business
understanding refers to modelling which could be realized in the case of AIH through
establishing specific parameters according to optimal values. Evaluation stage in the business
understanding could be associated with the review of the model’s capacity to address
business objectives of AIH (Foorthuis & Brinkkemper, 2015). The results obtained from the
data mining model should be reviewed in terms of their application in context of the business
objectives. The final stage of business understanding would involve deployment which refers
to the organization of the data and representing in a feasible manner to the institution.
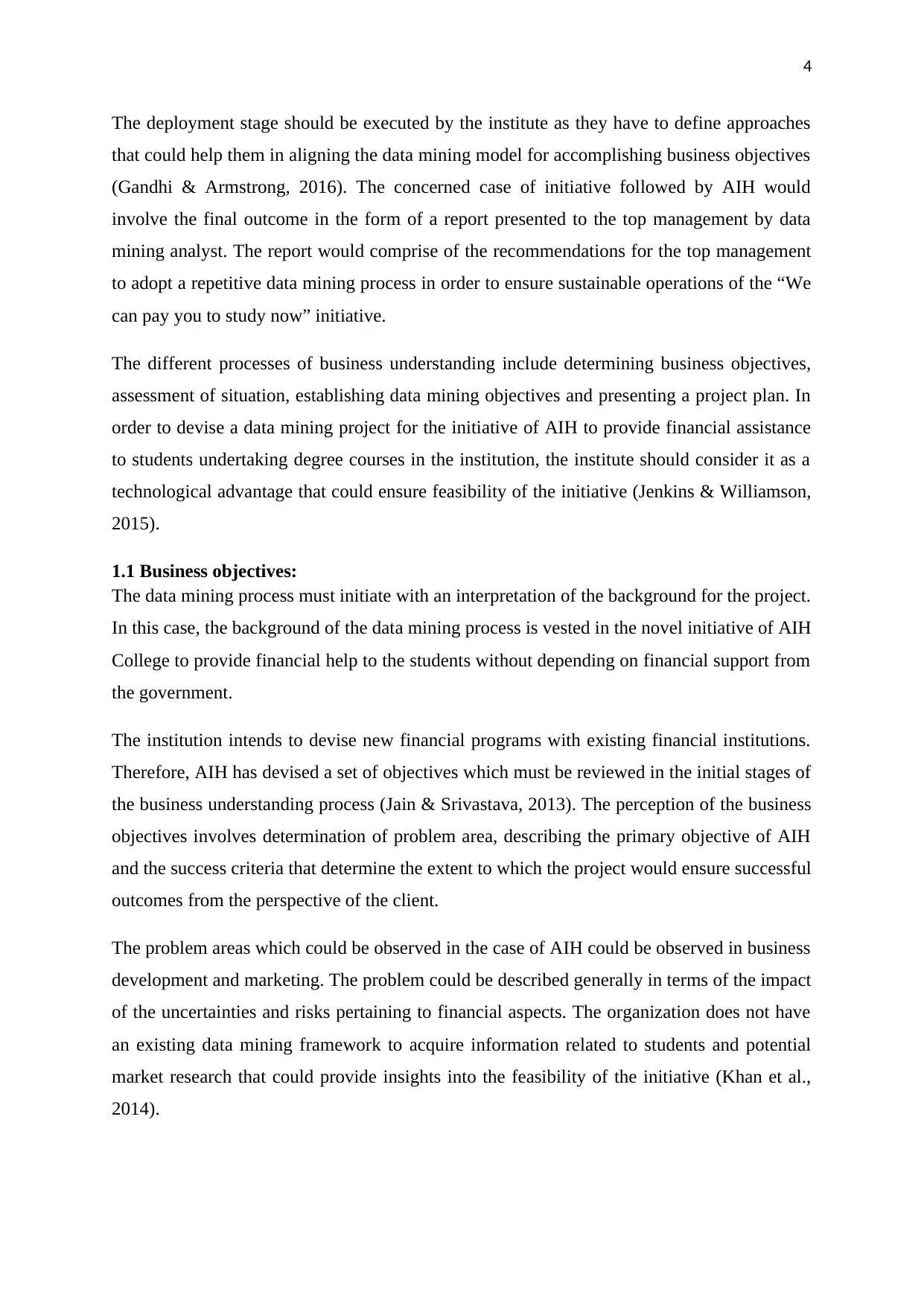
4
The deployment stage should be executed by the institute as they have to define approaches
that could help them in aligning the data mining model for accomplishing business objectives
(Gandhi & Armstrong, 2016). The concerned case of initiative followed by AIH would
involve the final outcome in the form of a report presented to the top management by data
mining analyst. The report would comprise of the recommendations for the top management
to adopt a repetitive data mining process in order to ensure sustainable operations of the “We
can pay you to study now” initiative.
The different processes of business understanding include determining business objectives,
assessment of situation, establishing data mining objectives and presenting a project plan. In
order to devise a data mining project for the initiative of AIH to provide financial assistance
to students undertaking degree courses in the institution, the institute should consider it as a
technological advantage that could ensure feasibility of the initiative (Jenkins & Williamson,
2015).
1.1 Business objectives:
The data mining process must initiate with an interpretation of the background for the project.
In this case, the background of the data mining process is vested in the novel initiative of AIH
College to provide financial help to the students without depending on financial support from
the government.
The institution intends to devise new financial programs with existing financial institutions.
Therefore, AIH has devised a set of objectives which must be reviewed in the initial stages of
the business understanding process (Jain & Srivastava, 2013). The perception of the business
objectives involves determination of problem area, describing the primary objective of AIH
and the success criteria that determine the extent to which the project would ensure successful
outcomes from the perspective of the client.
The problem areas which could be observed in the case of AIH could be observed in business
development and marketing. The problem could be described generally in terms of the impact
of the uncertainties and risks pertaining to financial aspects. The organization does not have
an existing data mining framework to acquire information related to students and potential
market research that could provide insights into the feasibility of the initiative (Khan et al.,
2014).
The deployment stage should be executed by the institute as they have to define approaches
that could help them in aligning the data mining model for accomplishing business objectives
(Gandhi & Armstrong, 2016). The concerned case of initiative followed by AIH would
involve the final outcome in the form of a report presented to the top management by data
mining analyst. The report would comprise of the recommendations for the top management
to adopt a repetitive data mining process in order to ensure sustainable operations of the “We
can pay you to study now” initiative.
The different processes of business understanding include determining business objectives,
assessment of situation, establishing data mining objectives and presenting a project plan. In
order to devise a data mining project for the initiative of AIH to provide financial assistance
to students undertaking degree courses in the institution, the institute should consider it as a
technological advantage that could ensure feasibility of the initiative (Jenkins & Williamson,
2015).
1.1 Business objectives:
The data mining process must initiate with an interpretation of the background for the project.
In this case, the background of the data mining process is vested in the novel initiative of AIH
College to provide financial help to the students without depending on financial support from
the government.
The institution intends to devise new financial programs with existing financial institutions.
Therefore, AIH has devised a set of objectives which must be reviewed in the initial stages of
the business understanding process (Jain & Srivastava, 2013). The perception of the business
objectives involves determination of problem area, describing the primary objective of AIH
and the success criteria that determine the extent to which the project would ensure successful
outcomes from the perspective of the client.
The problem areas which could be observed in the case of AIH could be observed in business
development and marketing. The problem could be described generally in terms of the impact
of the uncertainties and risks pertaining to financial aspects. The organization does not have
an existing data mining framework to acquire information related to students and potential
market research that could provide insights into the feasibility of the initiative (Khan et al.,
2014).
Secure Best Marks with AI Grader
Need help grading? Try our AI Grader for instant feedback on your assignments.
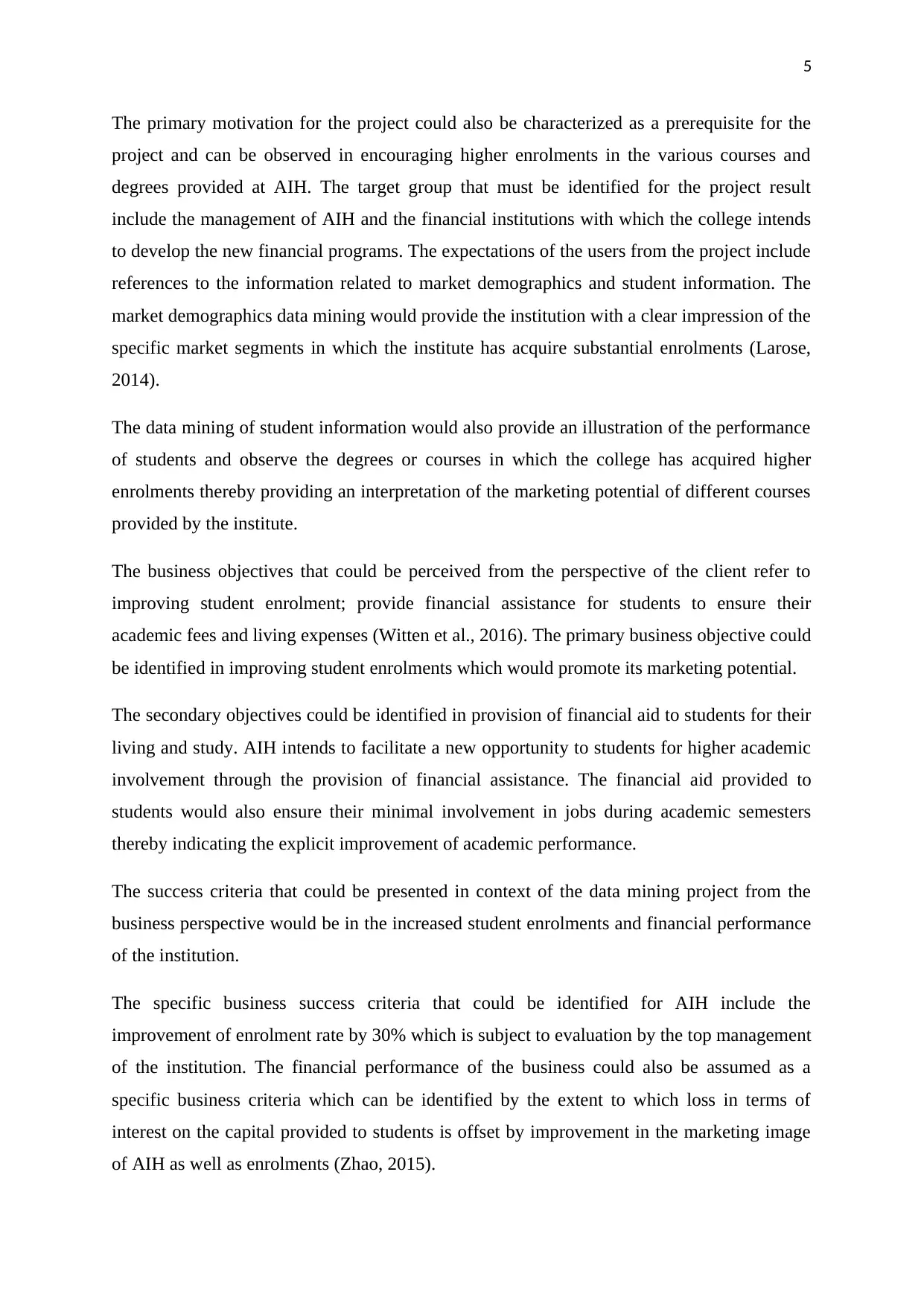
5
The primary motivation for the project could also be characterized as a prerequisite for the
project and can be observed in encouraging higher enrolments in the various courses and
degrees provided at AIH. The target group that must be identified for the project result
include the management of AIH and the financial institutions with which the college intends
to develop the new financial programs. The expectations of the users from the project include
references to the information related to market demographics and student information. The
market demographics data mining would provide the institution with a clear impression of the
specific market segments in which the institute has acquire substantial enrolments (Larose,
2014).
The data mining of student information would also provide an illustration of the performance
of students and observe the degrees or courses in which the college has acquired higher
enrolments thereby providing an interpretation of the marketing potential of different courses
provided by the institute.
The business objectives that could be perceived from the perspective of the client refer to
improving student enrolment; provide financial assistance for students to ensure their
academic fees and living expenses (Witten et al., 2016). The primary business objective could
be identified in improving student enrolments which would promote its marketing potential.
The secondary objectives could be identified in provision of financial aid to students for their
living and study. AIH intends to facilitate a new opportunity to students for higher academic
involvement through the provision of financial assistance. The financial aid provided to
students would also ensure their minimal involvement in jobs during academic semesters
thereby indicating the explicit improvement of academic performance.
The success criteria that could be presented in context of the data mining project from the
business perspective would be in the increased student enrolments and financial performance
of the institution.
The specific business success criteria that could be identified for AIH include the
improvement of enrolment rate by 30% which is subject to evaluation by the top management
of the institution. The financial performance of the business could also be assumed as a
specific business criteria which can be identified by the extent to which loss in terms of
interest on the capital provided to students is offset by improvement in the marketing image
of AIH as well as enrolments (Zhao, 2015).
The primary motivation for the project could also be characterized as a prerequisite for the
project and can be observed in encouraging higher enrolments in the various courses and
degrees provided at AIH. The target group that must be identified for the project result
include the management of AIH and the financial institutions with which the college intends
to develop the new financial programs. The expectations of the users from the project include
references to the information related to market demographics and student information. The
market demographics data mining would provide the institution with a clear impression of the
specific market segments in which the institute has acquire substantial enrolments (Larose,
2014).
The data mining of student information would also provide an illustration of the performance
of students and observe the degrees or courses in which the college has acquired higher
enrolments thereby providing an interpretation of the marketing potential of different courses
provided by the institute.
The business objectives that could be perceived from the perspective of the client refer to
improving student enrolment; provide financial assistance for students to ensure their
academic fees and living expenses (Witten et al., 2016). The primary business objective could
be identified in improving student enrolments which would promote its marketing potential.
The secondary objectives could be identified in provision of financial aid to students for their
living and study. AIH intends to facilitate a new opportunity to students for higher academic
involvement through the provision of financial assistance. The financial aid provided to
students would also ensure their minimal involvement in jobs during academic semesters
thereby indicating the explicit improvement of academic performance.
The success criteria that could be presented in context of the data mining project from the
business perspective would be in the increased student enrolments and financial performance
of the institution.
The specific business success criteria that could be identified for AIH include the
improvement of enrolment rate by 30% which is subject to evaluation by the top management
of the institution. The financial performance of the business could also be assumed as a
specific business criteria which can be identified by the extent to which loss in terms of
interest on the capital provided to students is offset by improvement in the marketing image
of AIH as well as enrolments (Zhao, 2015).
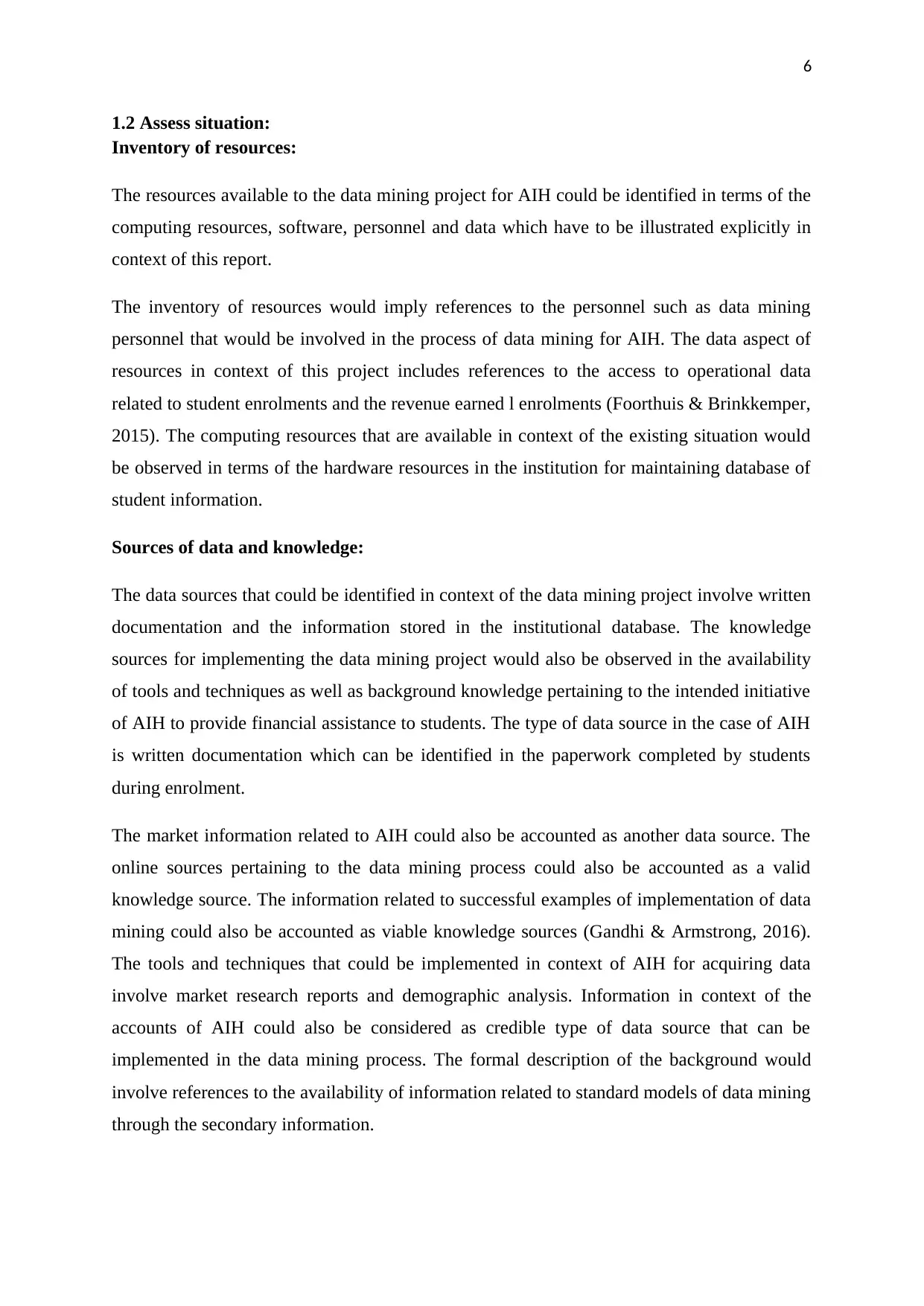
6
1.2 Assess situation:
Inventory of resources:
The resources available to the data mining project for AIH could be identified in terms of the
computing resources, software, personnel and data which have to be illustrated explicitly in
context of this report.
The inventory of resources would imply references to the personnel such as data mining
personnel that would be involved in the process of data mining for AIH. The data aspect of
resources in context of this project includes references to the access to operational data
related to student enrolments and the revenue earned l enrolments (Foorthuis & Brinkkemper,
2015). The computing resources that are available in context of the existing situation would
be observed in terms of the hardware resources in the institution for maintaining database of
student information.
Sources of data and knowledge:
The data sources that could be identified in context of the data mining project involve written
documentation and the information stored in the institutional database. The knowledge
sources for implementing the data mining project would also be observed in the availability
of tools and techniques as well as background knowledge pertaining to the intended initiative
of AIH to provide financial assistance to students. The type of data source in the case of AIH
is written documentation which can be identified in the paperwork completed by students
during enrolment.
The market information related to AIH could also be accounted as another data source. The
online sources pertaining to the data mining process could also be accounted as a valid
knowledge source. The information related to successful examples of implementation of data
mining could also be accounted as viable knowledge sources (Gandhi & Armstrong, 2016).
The tools and techniques that could be implemented in context of AIH for acquiring data
involve market research reports and demographic analysis. Information in context of the
accounts of AIH could also be considered as credible type of data source that can be
implemented in the data mining process. The formal description of the background would
involve references to the availability of information related to standard models of data mining
through the secondary information.
1.2 Assess situation:
Inventory of resources:
The resources available to the data mining project for AIH could be identified in terms of the
computing resources, software, personnel and data which have to be illustrated explicitly in
context of this report.
The inventory of resources would imply references to the personnel such as data mining
personnel that would be involved in the process of data mining for AIH. The data aspect of
resources in context of this project includes references to the access to operational data
related to student enrolments and the revenue earned l enrolments (Foorthuis & Brinkkemper,
2015). The computing resources that are available in context of the existing situation would
be observed in terms of the hardware resources in the institution for maintaining database of
student information.
Sources of data and knowledge:
The data sources that could be identified in context of the data mining project involve written
documentation and the information stored in the institutional database. The knowledge
sources for implementing the data mining project would also be observed in the availability
of tools and techniques as well as background knowledge pertaining to the intended initiative
of AIH to provide financial assistance to students. The type of data source in the case of AIH
is written documentation which can be identified in the paperwork completed by students
during enrolment.
The market information related to AIH could also be accounted as another data source. The
online sources pertaining to the data mining process could also be accounted as a valid
knowledge source. The information related to successful examples of implementation of data
mining could also be accounted as viable knowledge sources (Gandhi & Armstrong, 2016).
The tools and techniques that could be implemented in context of AIH for acquiring data
involve market research reports and demographic analysis. Information in context of the
accounts of AIH could also be considered as credible type of data source that can be
implemented in the data mining process. The formal description of the background would
involve references to the availability of information related to standard models of data mining
through the secondary information.
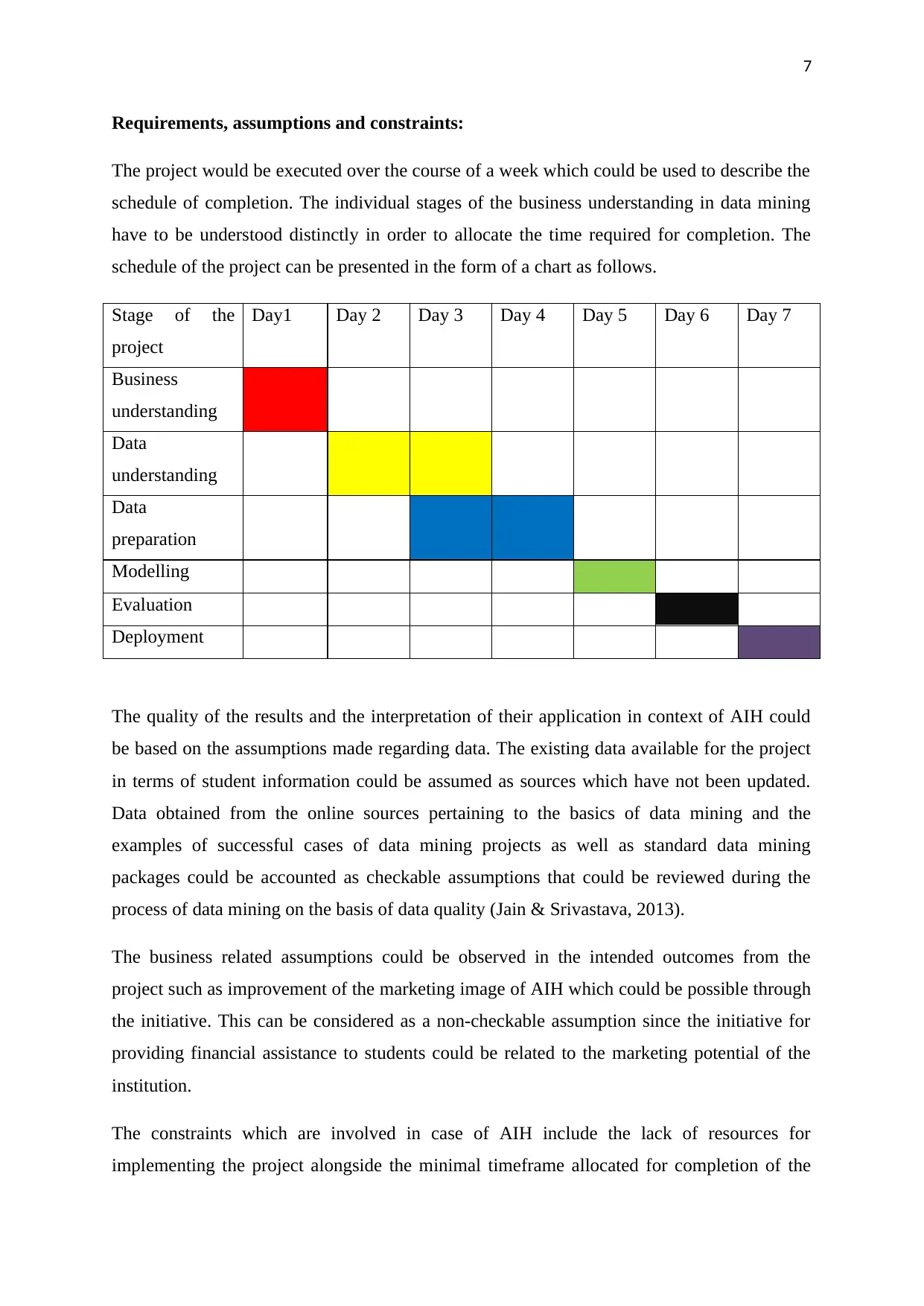
7
Requirements, assumptions and constraints:
The project would be executed over the course of a week which could be used to describe the
schedule of completion. The individual stages of the business understanding in data mining
have to be understood distinctly in order to allocate the time required for completion. The
schedule of the project can be presented in the form of a chart as follows.
Stage of the
project
Day1 Day 2 Day 3 Day 4 Day 5 Day 6 Day 7
Business
understanding
Data
understanding
Data
preparation
Modelling
Evaluation
Deployment
The quality of the results and the interpretation of their application in context of AIH could
be based on the assumptions made regarding data. The existing data available for the project
in terms of student information could be assumed as sources which have not been updated.
Data obtained from the online sources pertaining to the basics of data mining and the
examples of successful cases of data mining projects as well as standard data mining
packages could be accounted as checkable assumptions that could be reviewed during the
process of data mining on the basis of data quality (Jain & Srivastava, 2013).
The business related assumptions could be observed in the intended outcomes from the
project such as improvement of the marketing image of AIH which could be possible through
the initiative. This can be considered as a non-checkable assumption since the initiative for
providing financial assistance to students could be related to the marketing potential of the
institution.
The constraints which are involved in case of AIH include the lack of resources for
implementing the project alongside the minimal timeframe allocated for completion of the
Requirements, assumptions and constraints:
The project would be executed over the course of a week which could be used to describe the
schedule of completion. The individual stages of the business understanding in data mining
have to be understood distinctly in order to allocate the time required for completion. The
schedule of the project can be presented in the form of a chart as follows.
Stage of the
project
Day1 Day 2 Day 3 Day 4 Day 5 Day 6 Day 7
Business
understanding
Data
understanding
Data
preparation
Modelling
Evaluation
Deployment
The quality of the results and the interpretation of their application in context of AIH could
be based on the assumptions made regarding data. The existing data available for the project
in terms of student information could be assumed as sources which have not been updated.
Data obtained from the online sources pertaining to the basics of data mining and the
examples of successful cases of data mining projects as well as standard data mining
packages could be accounted as checkable assumptions that could be reviewed during the
process of data mining on the basis of data quality (Jain & Srivastava, 2013).
The business related assumptions could be observed in the intended outcomes from the
project such as improvement of the marketing image of AIH which could be possible through
the initiative. This can be considered as a non-checkable assumption since the initiative for
providing financial assistance to students could be related to the marketing potential of the
institution.
The constraints which are involved in case of AIH include the lack of resources for
implementing the project alongside the minimal timeframe allocated for completion of the
Paraphrase This Document
Need a fresh take? Get an instant paraphrase of this document with our AI Paraphraser
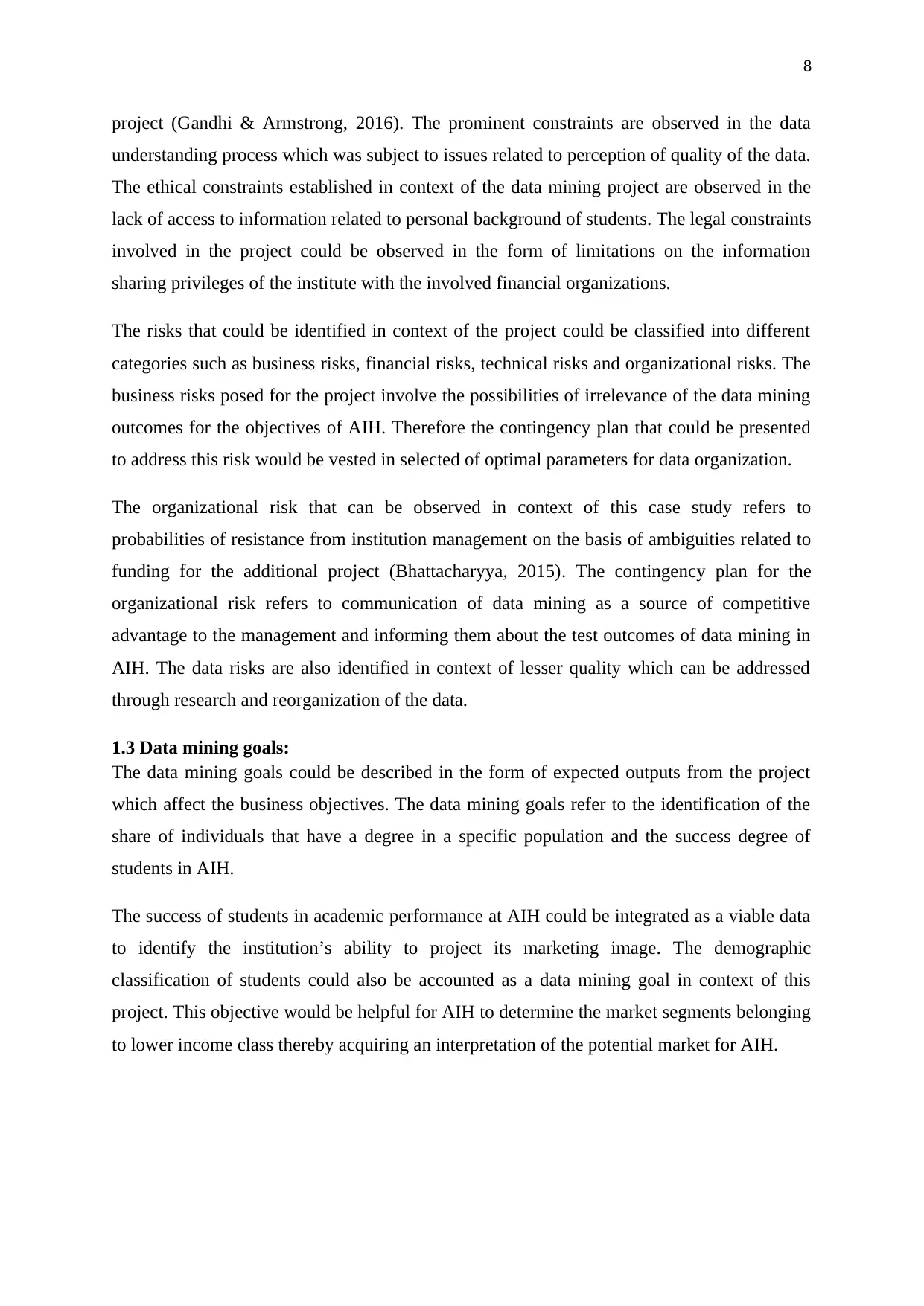
8
project (Gandhi & Armstrong, 2016). The prominent constraints are observed in the data
understanding process which was subject to issues related to perception of quality of the data.
The ethical constraints established in context of the data mining project are observed in the
lack of access to information related to personal background of students. The legal constraints
involved in the project could be observed in the form of limitations on the information
sharing privileges of the institute with the involved financial organizations.
The risks that could be identified in context of the project could be classified into different
categories such as business risks, financial risks, technical risks and organizational risks. The
business risks posed for the project involve the possibilities of irrelevance of the data mining
outcomes for the objectives of AIH. Therefore the contingency plan that could be presented
to address this risk would be vested in selected of optimal parameters for data organization.
The organizational risk that can be observed in context of this case study refers to
probabilities of resistance from institution management on the basis of ambiguities related to
funding for the additional project (Bhattacharyya, 2015). The contingency plan for the
organizational risk refers to communication of data mining as a source of competitive
advantage to the management and informing them about the test outcomes of data mining in
AIH. The data risks are also identified in context of lesser quality which can be addressed
through research and reorganization of the data.
1.3 Data mining goals:
The data mining goals could be described in the form of expected outputs from the project
which affect the business objectives. The data mining goals refer to the identification of the
share of individuals that have a degree in a specific population and the success degree of
students in AIH.
The success of students in academic performance at AIH could be integrated as a viable data
to identify the institution’s ability to project its marketing image. The demographic
classification of students could also be accounted as a data mining goal in context of this
project. This objective would be helpful for AIH to determine the market segments belonging
to lower income class thereby acquiring an interpretation of the potential market for AIH.
project (Gandhi & Armstrong, 2016). The prominent constraints are observed in the data
understanding process which was subject to issues related to perception of quality of the data.
The ethical constraints established in context of the data mining project are observed in the
lack of access to information related to personal background of students. The legal constraints
involved in the project could be observed in the form of limitations on the information
sharing privileges of the institute with the involved financial organizations.
The risks that could be identified in context of the project could be classified into different
categories such as business risks, financial risks, technical risks and organizational risks. The
business risks posed for the project involve the possibilities of irrelevance of the data mining
outcomes for the objectives of AIH. Therefore the contingency plan that could be presented
to address this risk would be vested in selected of optimal parameters for data organization.
The organizational risk that can be observed in context of this case study refers to
probabilities of resistance from institution management on the basis of ambiguities related to
funding for the additional project (Bhattacharyya, 2015). The contingency plan for the
organizational risk refers to communication of data mining as a source of competitive
advantage to the management and informing them about the test outcomes of data mining in
AIH. The data risks are also identified in context of lesser quality which can be addressed
through research and reorganization of the data.
1.3 Data mining goals:
The data mining goals could be described in the form of expected outputs from the project
which affect the business objectives. The data mining goals refer to the identification of the
share of individuals that have a degree in a specific population and the success degree of
students in AIH.
The success of students in academic performance at AIH could be integrated as a viable data
to identify the institution’s ability to project its marketing image. The demographic
classification of students could also be accounted as a data mining goal in context of this
project. This objective would be helpful for AIH to determine the market segments belonging
to lower income class thereby acquiring an interpretation of the potential market for AIH.
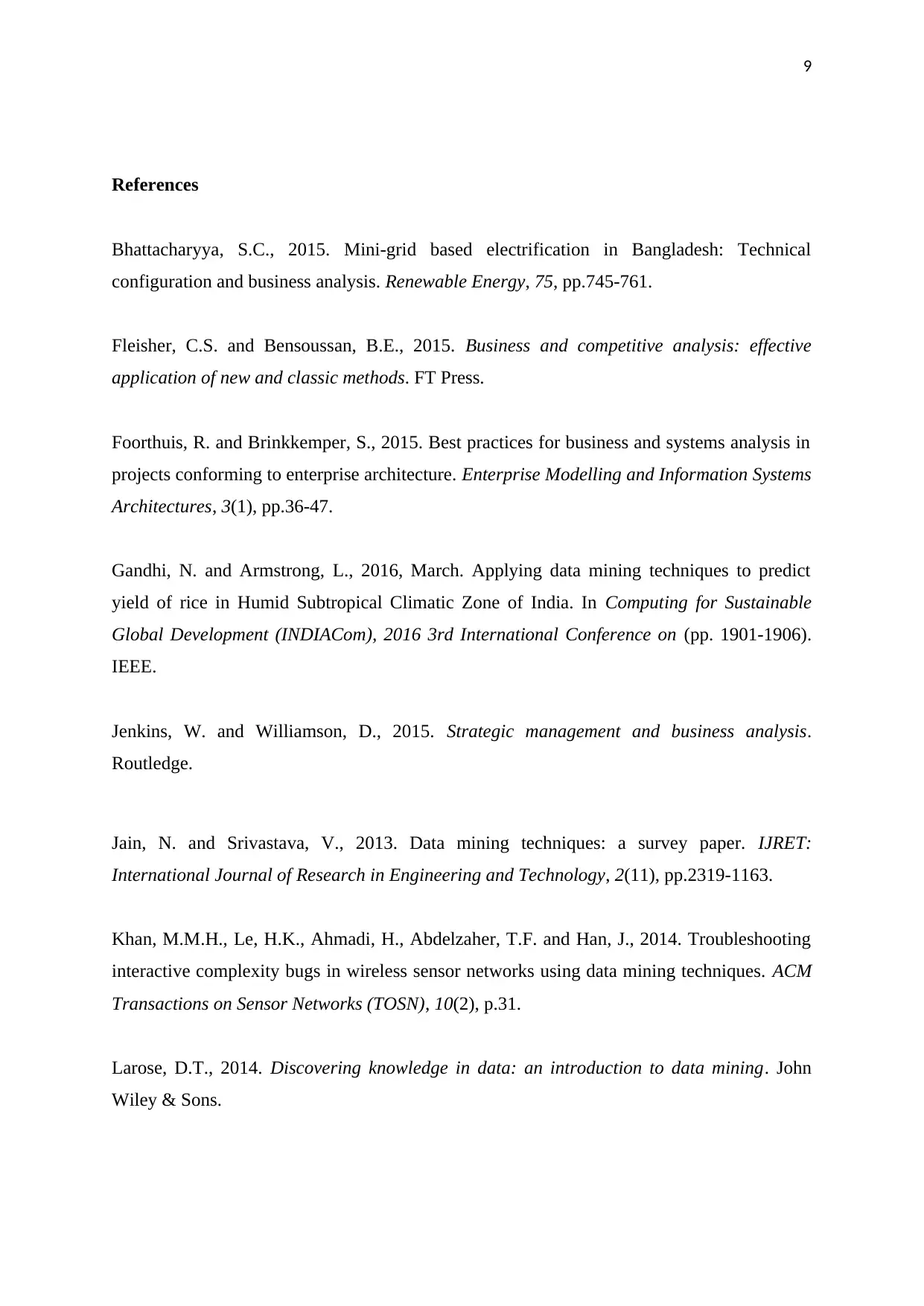
9
References
Bhattacharyya, S.C., 2015. Mini-grid based electrification in Bangladesh: Technical
configuration and business analysis. Renewable Energy, 75, pp.745-761.
Fleisher, C.S. and Bensoussan, B.E., 2015. Business and competitive analysis: effective
application of new and classic methods. FT Press.
Foorthuis, R. and Brinkkemper, S., 2015. Best practices for business and systems analysis in
projects conforming to enterprise architecture. Enterprise Modelling and Information Systems
Architectures, 3(1), pp.36-47.
Gandhi, N. and Armstrong, L., 2016, March. Applying data mining techniques to predict
yield of rice in Humid Subtropical Climatic Zone of India. In Computing for Sustainable
Global Development (INDIACom), 2016 3rd International Conference on (pp. 1901-1906).
IEEE.
Jenkins, W. and Williamson, D., 2015. Strategic management and business analysis.
Routledge.
Jain, N. and Srivastava, V., 2013. Data mining techniques: a survey paper. IJRET:
International Journal of Research in Engineering and Technology, 2(11), pp.2319-1163.
Khan, M.M.H., Le, H.K., Ahmadi, H., Abdelzaher, T.F. and Han, J., 2014. Troubleshooting
interactive complexity bugs in wireless sensor networks using data mining techniques. ACM
Transactions on Sensor Networks (TOSN), 10(2), p.31.
Larose, D.T., 2014. Discovering knowledge in data: an introduction to data mining. John
Wiley & Sons.
References
Bhattacharyya, S.C., 2015. Mini-grid based electrification in Bangladesh: Technical
configuration and business analysis. Renewable Energy, 75, pp.745-761.
Fleisher, C.S. and Bensoussan, B.E., 2015. Business and competitive analysis: effective
application of new and classic methods. FT Press.
Foorthuis, R. and Brinkkemper, S., 2015. Best practices for business and systems analysis in
projects conforming to enterprise architecture. Enterprise Modelling and Information Systems
Architectures, 3(1), pp.36-47.
Gandhi, N. and Armstrong, L., 2016, March. Applying data mining techniques to predict
yield of rice in Humid Subtropical Climatic Zone of India. In Computing for Sustainable
Global Development (INDIACom), 2016 3rd International Conference on (pp. 1901-1906).
IEEE.
Jenkins, W. and Williamson, D., 2015. Strategic management and business analysis.
Routledge.
Jain, N. and Srivastava, V., 2013. Data mining techniques: a survey paper. IJRET:
International Journal of Research in Engineering and Technology, 2(11), pp.2319-1163.
Khan, M.M.H., Le, H.K., Ahmadi, H., Abdelzaher, T.F. and Han, J., 2014. Troubleshooting
interactive complexity bugs in wireless sensor networks using data mining techniques. ACM
Transactions on Sensor Networks (TOSN), 10(2), p.31.
Larose, D.T., 2014. Discovering knowledge in data: an introduction to data mining. John
Wiley & Sons.
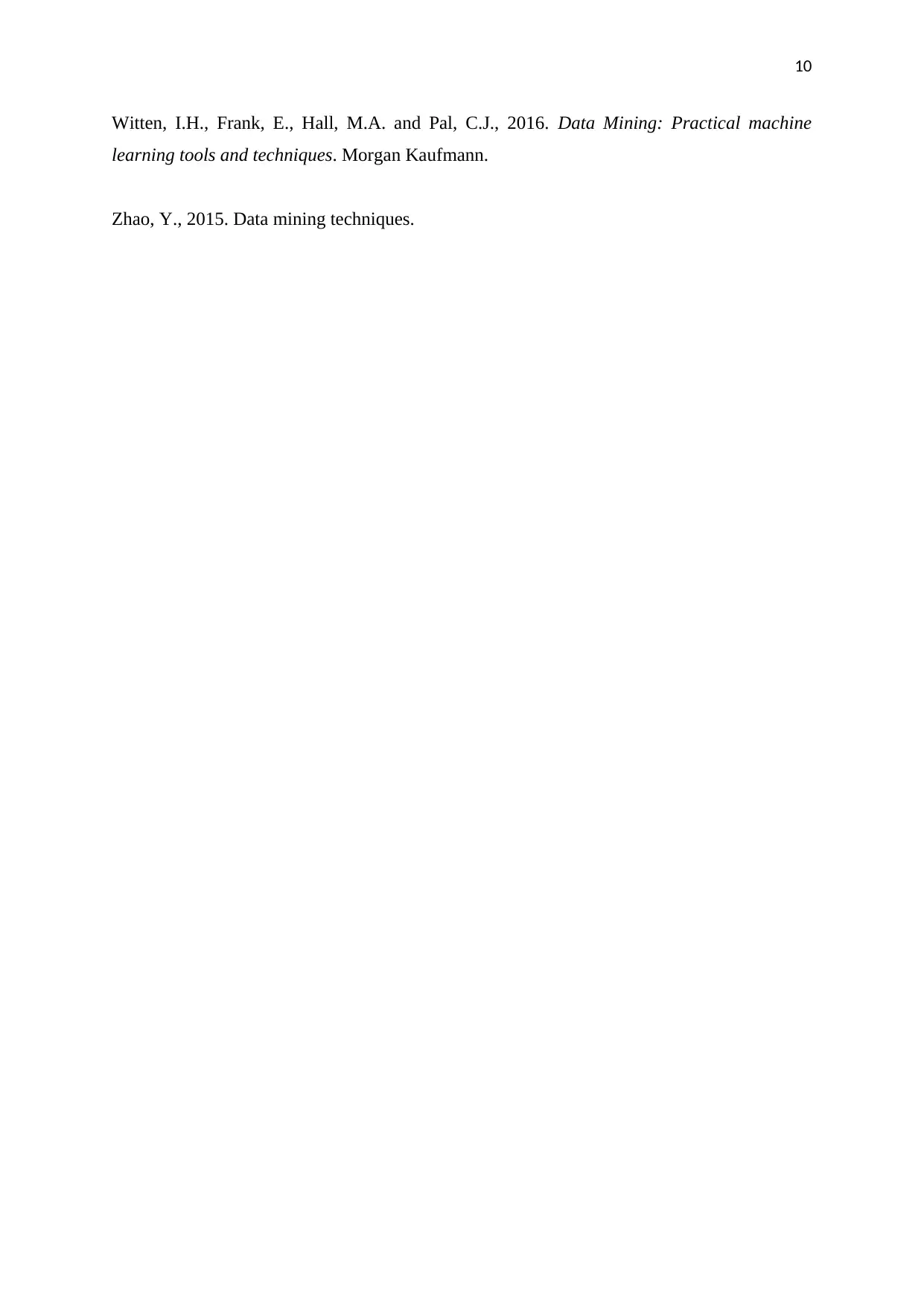
10
Witten, I.H., Frank, E., Hall, M.A. and Pal, C.J., 2016. Data Mining: Practical machine
learning tools and techniques. Morgan Kaufmann.
Zhao, Y., 2015. Data mining techniques.
Witten, I.H., Frank, E., Hall, M.A. and Pal, C.J., 2016. Data Mining: Practical machine
learning tools and techniques. Morgan Kaufmann.
Zhao, Y., 2015. Data mining techniques.
1 out of 10
Related Documents

Your All-in-One AI-Powered Toolkit for Academic Success.
+13062052269
info@desklib.com
Available 24*7 on WhatsApp / Email
Unlock your academic potential
© 2024 | Zucol Services PVT LTD | All rights reserved.