Big Data: An Analysis of Advantages, Disadvantages, and Impacts
VerifiedAdded on 2022/08/11
|13
|4009
|16
Essay
AI Summary
This essay delves into the realm of big data, examining its role in modern business practices. It discusses the benefits of utilizing big data, such as improved data management, advanced analytics, and enhanced consumer experiences, highlighting how companies leverage it to analyze trends, patterns, and consumer behavior. The essay also addresses the drawbacks, including data quality issues, security vulnerabilities, and privacy concerns, particularly the risks of cyber-crimes and data breaches. Furthermore, it explores the impact of big data on stakeholders, including investors, and emphasizes the importance of implementing robust security measures and ethical considerations. The conclusion suggests the strategic adoption of big data while advocating for stringent security protocols, employee training, and the use of reliable data sources to mitigate risks and maximize its benefits.
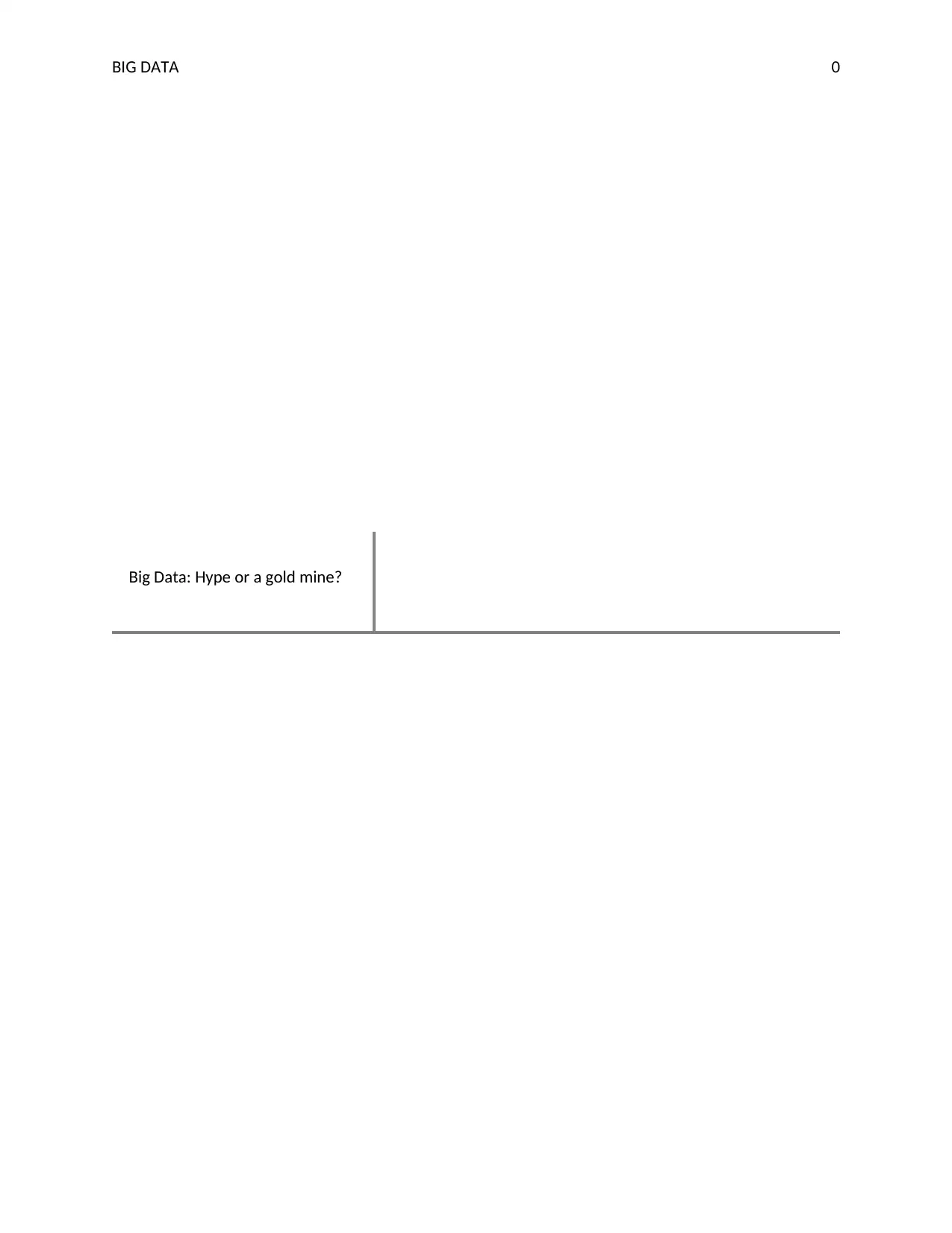
BIG DATA 0
Big Data: Hype or a gold mine?
Big Data: Hype or a gold mine?
Paraphrase This Document
Need a fresh take? Get an instant paraphrase of this document with our AI Paraphraser
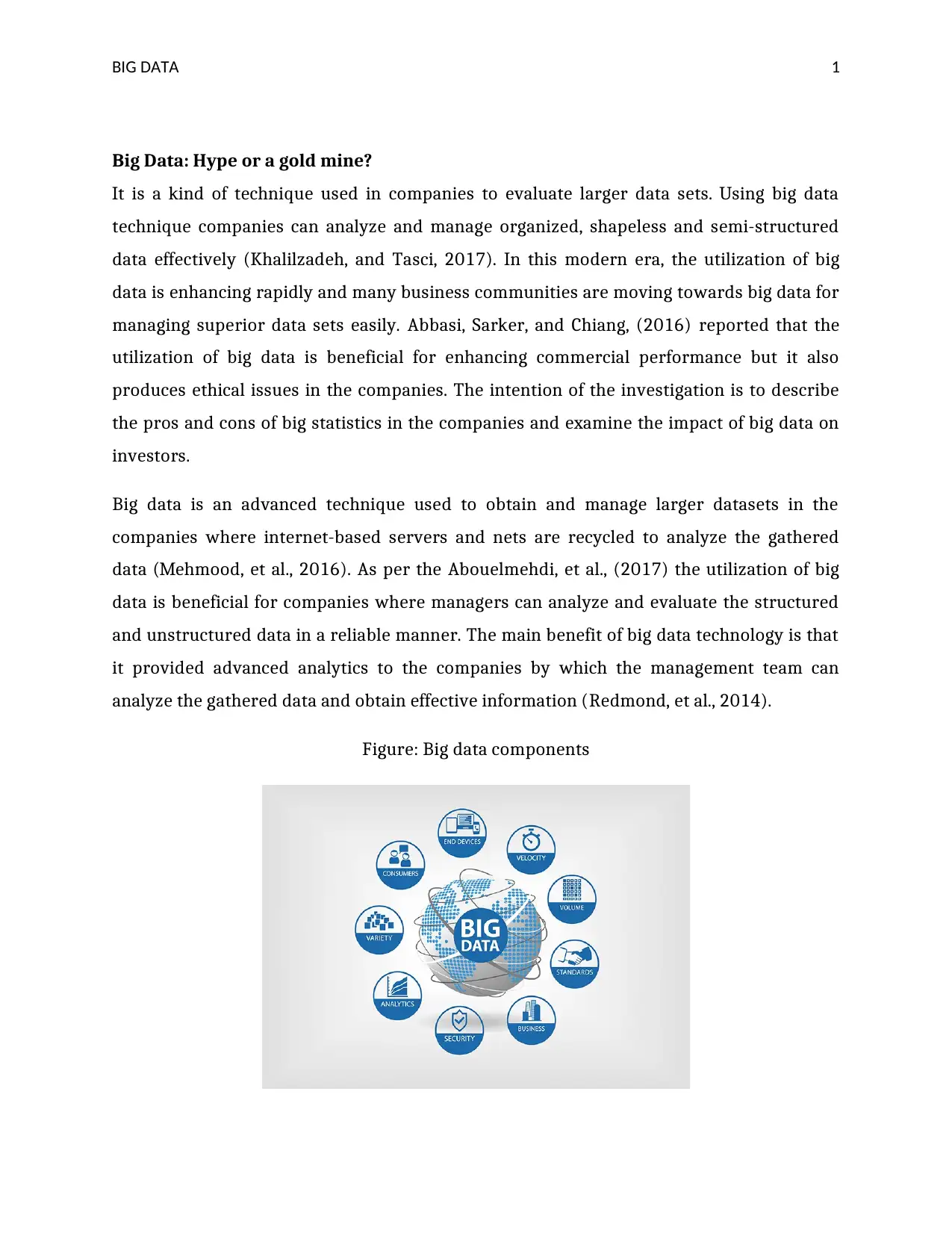
BIG DATA 1
Big Data: Hype or a gold mine?
It is a kind of technique used in companies to evaluate larger data sets. Using big data
technique companies can analyze and manage organized, shapeless and semi-structured
data effectively (Khalilzadeh, and Tasci, 2017). In this modern era, the utilization of big
data is enhancing rapidly and many business communities are moving towards big data for
managing superior data sets easily. Abbasi, Sarker, and Chiang, (2016) reported that the
utilization of big data is beneficial for enhancing commercial performance but it also
produces ethical issues in the companies. The intention of the investigation is to describe
the pros and cons of big statistics in the companies and examine the impact of big data on
investors.
Big data is an advanced technique used to obtain and manage larger datasets in the
companies where internet-based servers and nets are recycled to analyze the gathered
data (Mehmood, et al., 2016). As per the Abouelmehdi, et al., (2017) the utilization of big
data is beneficial for companies where managers can analyze and evaluate the structured
and unstructured data in a reliable manner. The main benefit of big data technology is that
it provided advanced analytics to the companies by which the management team can
analyze the gathered data and obtain effective information (Redmond, et al., 2014).
Figure: Big data components
Big Data: Hype or a gold mine?
It is a kind of technique used in companies to evaluate larger data sets. Using big data
technique companies can analyze and manage organized, shapeless and semi-structured
data effectively (Khalilzadeh, and Tasci, 2017). In this modern era, the utilization of big
data is enhancing rapidly and many business communities are moving towards big data for
managing superior data sets easily. Abbasi, Sarker, and Chiang, (2016) reported that the
utilization of big data is beneficial for enhancing commercial performance but it also
produces ethical issues in the companies. The intention of the investigation is to describe
the pros and cons of big statistics in the companies and examine the impact of big data on
investors.
Big data is an advanced technique used to obtain and manage larger datasets in the
companies where internet-based servers and nets are recycled to analyze the gathered
data (Mehmood, et al., 2016). As per the Abouelmehdi, et al., (2017) the utilization of big
data is beneficial for companies where managers can analyze and evaluate the structured
and unstructured data in a reliable manner. The main benefit of big data technology is that
it provided advanced analytics to the companies by which the management team can
analyze the gathered data and obtain effective information (Redmond, et al., 2014).
Figure: Big data components
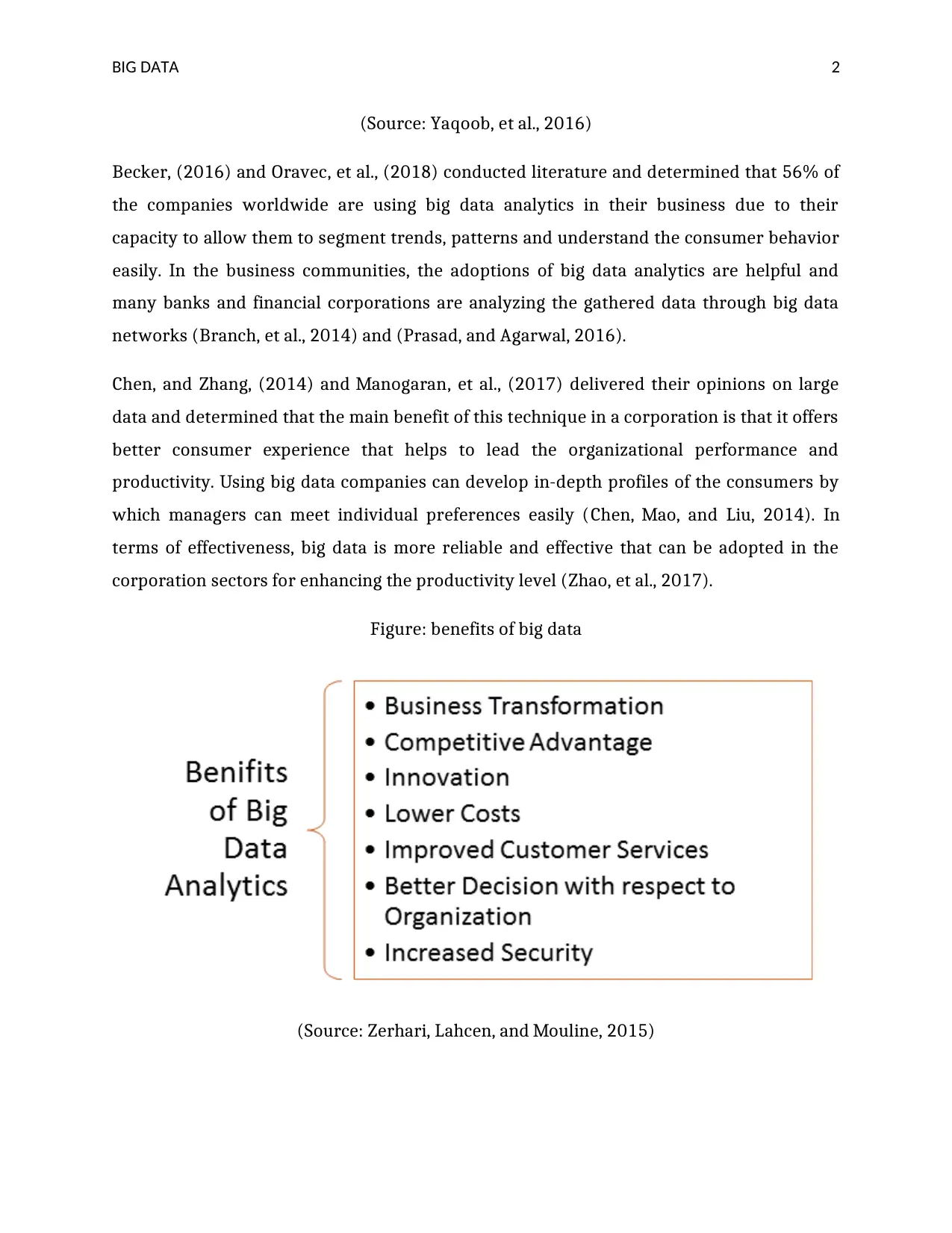
BIG DATA 2
(Source: Yaqoob, et al., 2016)
Becker, (2016) and Oravec, et al., (2018) conducted literature and determined that 56% of
the companies worldwide are using big data analytics in their business due to their
capacity to allow them to segment trends, patterns and understand the consumer behavior
easily. In the business communities, the adoptions of big data analytics are helpful and
many banks and financial corporations are analyzing the gathered data through big data
networks (Branch, et al., 2014) and (Prasad, and Agarwal, 2016).
Chen, and Zhang, (2014) and Manogaran, et al., (2017) delivered their opinions on large
data and determined that the main benefit of this technique in a corporation is that it offers
better consumer experience that helps to lead the organizational performance and
productivity. Using big data companies can develop in-depth profiles of the consumers by
which managers can meet individual preferences easily (Chen, Mao, and Liu, 2014). In
terms of effectiveness, big data is more reliable and effective that can be adopted in the
corporation sectors for enhancing the productivity level (Zhao, et al., 2017).
Figure: benefits of big data
(Source: Zerhari, Lahcen, and Mouline, 2015)
(Source: Yaqoob, et al., 2016)
Becker, (2016) and Oravec, et al., (2018) conducted literature and determined that 56% of
the companies worldwide are using big data analytics in their business due to their
capacity to allow them to segment trends, patterns and understand the consumer behavior
easily. In the business communities, the adoptions of big data analytics are helpful and
many banks and financial corporations are analyzing the gathered data through big data
networks (Branch, et al., 2014) and (Prasad, and Agarwal, 2016).
Chen, and Zhang, (2014) and Manogaran, et al., (2017) delivered their opinions on large
data and determined that the main benefit of this technique in a corporation is that it offers
better consumer experience that helps to lead the organizational performance and
productivity. Using big data companies can develop in-depth profiles of the consumers by
which managers can meet individual preferences easily (Chen, Mao, and Liu, 2014). In
terms of effectiveness, big data is more reliable and effective that can be adopted in the
corporation sectors for enhancing the productivity level (Zhao, et al., 2017).
Figure: benefits of big data
(Source: Zerhari, Lahcen, and Mouline, 2015)
You're viewing a preview
Unlock full access by subscribing today!
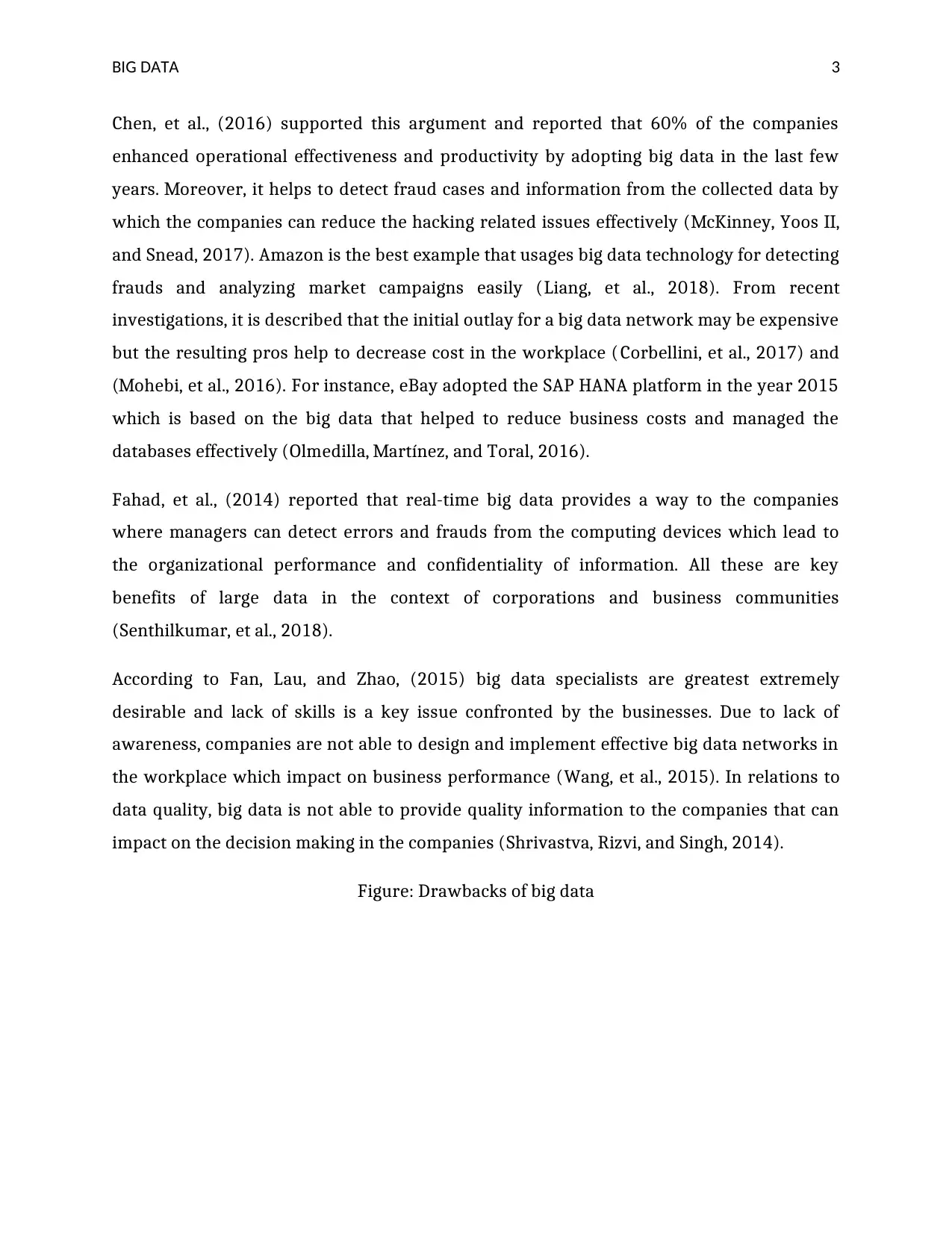
BIG DATA 3
Chen, et al., (2016) supported this argument and reported that 60% of the companies
enhanced operational effectiveness and productivity by adopting big data in the last few
years. Moreover, it helps to detect fraud cases and information from the collected data by
which the companies can reduce the hacking related issues effectively (McKinney, Yoos II,
and Snead, 2017). Amazon is the best example that usages big data technology for detecting
frauds and analyzing market campaigns easily (Liang, et al., 2018). From recent
investigations, it is described that the initial outlay for a big data network may be expensive
but the resulting pros help to decrease cost in the workplace (Corbellini, et al., 2017) and
(Mohebi, et al., 2016). For instance, eBay adopted the SAP HANA platform in the year 2015
which is based on the big data that helped to reduce business costs and managed the
databases effectively (Olmedilla, Martínez, and Toral, 2016).
Fahad, et al., (2014) reported that real-time big data provides a way to the companies
where managers can detect errors and frauds from the computing devices which lead to
the organizational performance and confidentiality of information. All these are key
benefits of large data in the context of corporations and business communities
(Senthilkumar, et al., 2018).
According to Fan, Lau, and Zhao, (2015) big data specialists are greatest extremely
desirable and lack of skills is a key issue confronted by the businesses. Due to lack of
awareness, companies are not able to design and implement effective big data networks in
the workplace which impact on business performance (Wang, et al., 2015). In relations to
data quality, big data is not able to provide quality information to the companies that can
impact on the decision making in the companies (Shrivastva, Rizvi, and Singh, 2014).
Figure: Drawbacks of big data
Chen, et al., (2016) supported this argument and reported that 60% of the companies
enhanced operational effectiveness and productivity by adopting big data in the last few
years. Moreover, it helps to detect fraud cases and information from the collected data by
which the companies can reduce the hacking related issues effectively (McKinney, Yoos II,
and Snead, 2017). Amazon is the best example that usages big data technology for detecting
frauds and analyzing market campaigns easily (Liang, et al., 2018). From recent
investigations, it is described that the initial outlay for a big data network may be expensive
but the resulting pros help to decrease cost in the workplace (Corbellini, et al., 2017) and
(Mohebi, et al., 2016). For instance, eBay adopted the SAP HANA platform in the year 2015
which is based on the big data that helped to reduce business costs and managed the
databases effectively (Olmedilla, Martínez, and Toral, 2016).
Fahad, et al., (2014) reported that real-time big data provides a way to the companies
where managers can detect errors and frauds from the computing devices which lead to
the organizational performance and confidentiality of information. All these are key
benefits of large data in the context of corporations and business communities
(Senthilkumar, et al., 2018).
According to Fan, Lau, and Zhao, (2015) big data specialists are greatest extremely
desirable and lack of skills is a key issue confronted by the businesses. Due to lack of
awareness, companies are not able to design and implement effective big data networks in
the workplace which impact on business performance (Wang, et al., 2015). In relations to
data quality, big data is not able to provide quality information to the companies that can
impact on the decision making in the companies (Shrivastva, Rizvi, and Singh, 2014).
Figure: Drawbacks of big data
Paraphrase This Document
Need a fresh take? Get an instant paraphrase of this document with our AI Paraphraser
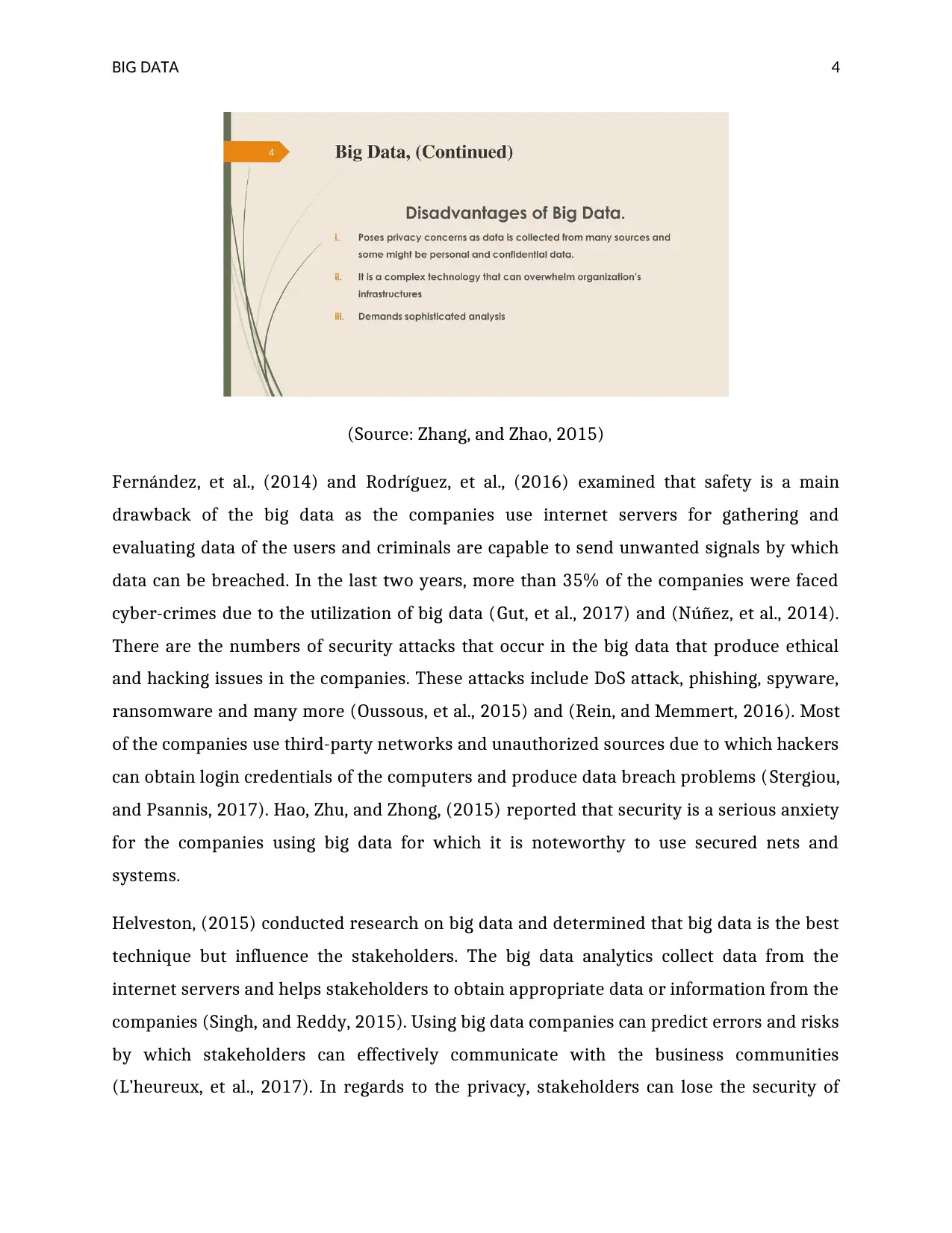
BIG DATA 4
(Source: Zhang, and Zhao, 2015)
Fernández, et al., (2014) and Rodríguez, et al., (2016) examined that safety is a main
drawback of the big data as the companies use internet servers for gathering and
evaluating data of the users and criminals are capable to send unwanted signals by which
data can be breached. In the last two years, more than 35% of the companies were faced
cyber-crimes due to the utilization of big data (Gut, et al., 2017) and (Núñez, et al., 2014).
There are the numbers of security attacks that occur in the big data that produce ethical
and hacking issues in the companies. These attacks include DoS attack, phishing, spyware,
ransomware and many more (Oussous, et al., 2015) and (Rein, and Memmert, 2016). Most
of the companies use third-party networks and unauthorized sources due to which hackers
can obtain login credentials of the computers and produce data breach problems (Stergiou,
and Psannis, 2017). Hao, Zhu, and Zhong, (2015) reported that security is a serious anxiety
for the companies using big data for which it is noteworthy to use secured nets and
systems.
Helveston, (2015) conducted research on big data and determined that big data is the best
technique but influence the stakeholders. The big data analytics collect data from the
internet servers and helps stakeholders to obtain appropriate data or information from the
companies (Singh, and Reddy, 2015). Using big data companies can predict errors and risks
by which stakeholders can effectively communicate with the business communities
(L’heureux, et al., 2017). In regards to the privacy, stakeholders can lose the security of
(Source: Zhang, and Zhao, 2015)
Fernández, et al., (2014) and Rodríguez, et al., (2016) examined that safety is a main
drawback of the big data as the companies use internet servers for gathering and
evaluating data of the users and criminals are capable to send unwanted signals by which
data can be breached. In the last two years, more than 35% of the companies were faced
cyber-crimes due to the utilization of big data (Gut, et al., 2017) and (Núñez, et al., 2014).
There are the numbers of security attacks that occur in the big data that produce ethical
and hacking issues in the companies. These attacks include DoS attack, phishing, spyware,
ransomware and many more (Oussous, et al., 2015) and (Rein, and Memmert, 2016). Most
of the companies use third-party networks and unauthorized sources due to which hackers
can obtain login credentials of the computers and produce data breach problems (Stergiou,
and Psannis, 2017). Hao, Zhu, and Zhong, (2015) reported that security is a serious anxiety
for the companies using big data for which it is noteworthy to use secured nets and
systems.
Helveston, (2015) conducted research on big data and determined that big data is the best
technique but influence the stakeholders. The big data analytics collect data from the
internet servers and helps stakeholders to obtain appropriate data or information from the
companies (Singh, and Reddy, 2015). Using big data companies can predict errors and risks
by which stakeholders can effectively communicate with the business communities
(L’heureux, et al., 2017). In regards to the privacy, stakeholders can lose the security of
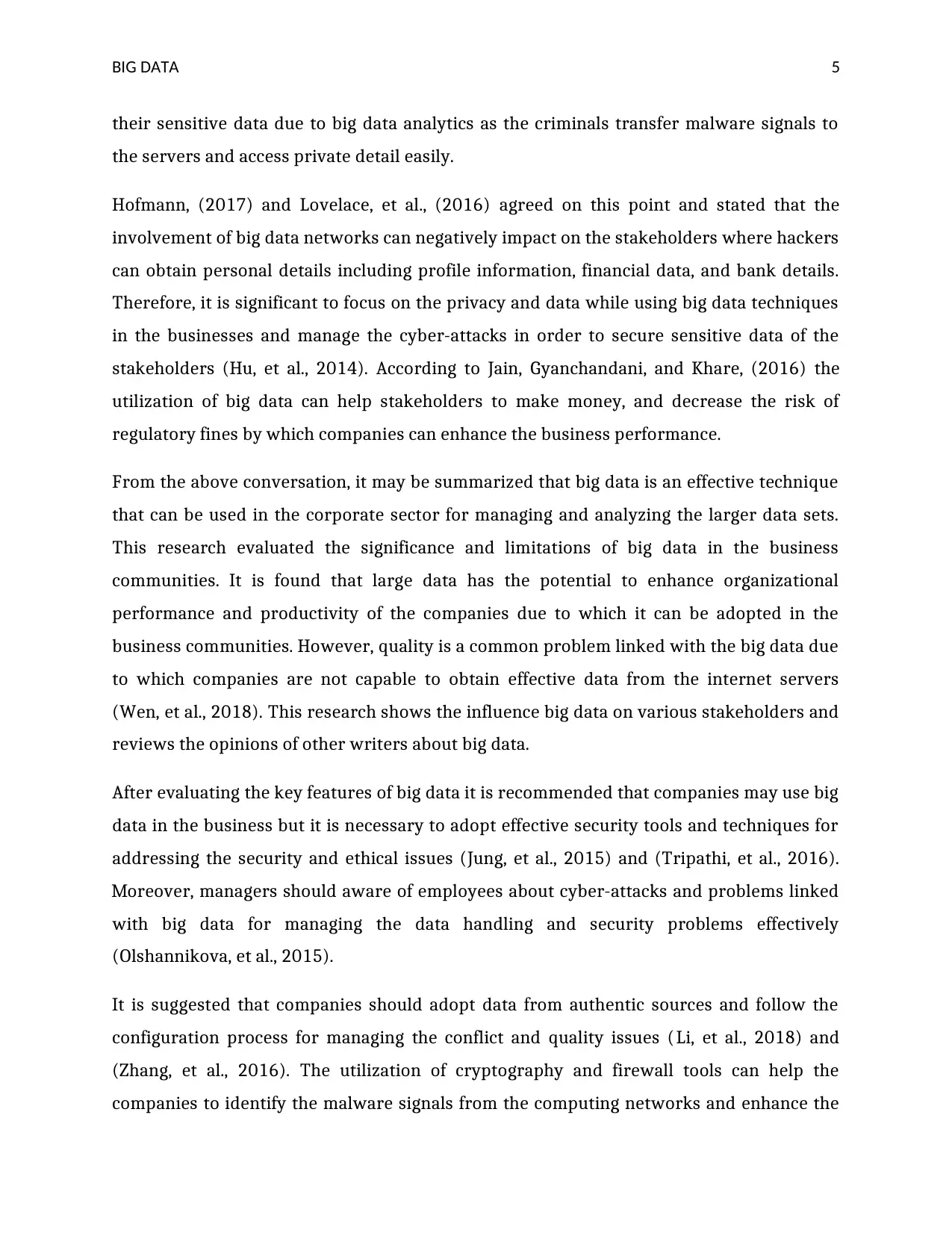
BIG DATA 5
their sensitive data due to big data analytics as the criminals transfer malware signals to
the servers and access private detail easily.
Hofmann, (2017) and Lovelace, et al., (2016) agreed on this point and stated that the
involvement of big data networks can negatively impact on the stakeholders where hackers
can obtain personal details including profile information, financial data, and bank details.
Therefore, it is significant to focus on the privacy and data while using big data techniques
in the businesses and manage the cyber-attacks in order to secure sensitive data of the
stakeholders (Hu, et al., 2014). According to Jain, Gyanchandani, and Khare, (2016) the
utilization of big data can help stakeholders to make money, and decrease the risk of
regulatory fines by which companies can enhance the business performance.
From the above conversation, it may be summarized that big data is an effective technique
that can be used in the corporate sector for managing and analyzing the larger data sets.
This research evaluated the significance and limitations of big data in the business
communities. It is found that large data has the potential to enhance organizational
performance and productivity of the companies due to which it can be adopted in the
business communities. However, quality is a common problem linked with the big data due
to which companies are not capable to obtain effective data from the internet servers
(Wen, et al., 2018). This research shows the influence big data on various stakeholders and
reviews the opinions of other writers about big data.
After evaluating the key features of big data it is recommended that companies may use big
data in the business but it is necessary to adopt effective security tools and techniques for
addressing the security and ethical issues (Jung, et al., 2015) and (Tripathi, et al., 2016).
Moreover, managers should aware of employees about cyber-attacks and problems linked
with big data for managing the data handling and security problems effectively
(Olshannikova, et al., 2015).
It is suggested that companies should adopt data from authentic sources and follow the
configuration process for managing the conflict and quality issues (Li, et al., 2018) and
(Zhang, et al., 2016). The utilization of cryptography and firewall tools can help the
companies to identify the malware signals from the computing networks and enhance the
their sensitive data due to big data analytics as the criminals transfer malware signals to
the servers and access private detail easily.
Hofmann, (2017) and Lovelace, et al., (2016) agreed on this point and stated that the
involvement of big data networks can negatively impact on the stakeholders where hackers
can obtain personal details including profile information, financial data, and bank details.
Therefore, it is significant to focus on the privacy and data while using big data techniques
in the businesses and manage the cyber-attacks in order to secure sensitive data of the
stakeholders (Hu, et al., 2014). According to Jain, Gyanchandani, and Khare, (2016) the
utilization of big data can help stakeholders to make money, and decrease the risk of
regulatory fines by which companies can enhance the business performance.
From the above conversation, it may be summarized that big data is an effective technique
that can be used in the corporate sector for managing and analyzing the larger data sets.
This research evaluated the significance and limitations of big data in the business
communities. It is found that large data has the potential to enhance organizational
performance and productivity of the companies due to which it can be adopted in the
business communities. However, quality is a common problem linked with the big data due
to which companies are not capable to obtain effective data from the internet servers
(Wen, et al., 2018). This research shows the influence big data on various stakeholders and
reviews the opinions of other writers about big data.
After evaluating the key features of big data it is recommended that companies may use big
data in the business but it is necessary to adopt effective security tools and techniques for
addressing the security and ethical issues (Jung, et al., 2015) and (Tripathi, et al., 2016).
Moreover, managers should aware of employees about cyber-attacks and problems linked
with big data for managing the data handling and security problems effectively
(Olshannikova, et al., 2015).
It is suggested that companies should adopt data from authentic sources and follow the
configuration process for managing the conflict and quality issues (Li, et al., 2018) and
(Zhang, et al., 2016). The utilization of cryptography and firewall tools can help the
companies to identify the malware signals from the computing networks and enhance the
You're viewing a preview
Unlock full access by subscribing today!
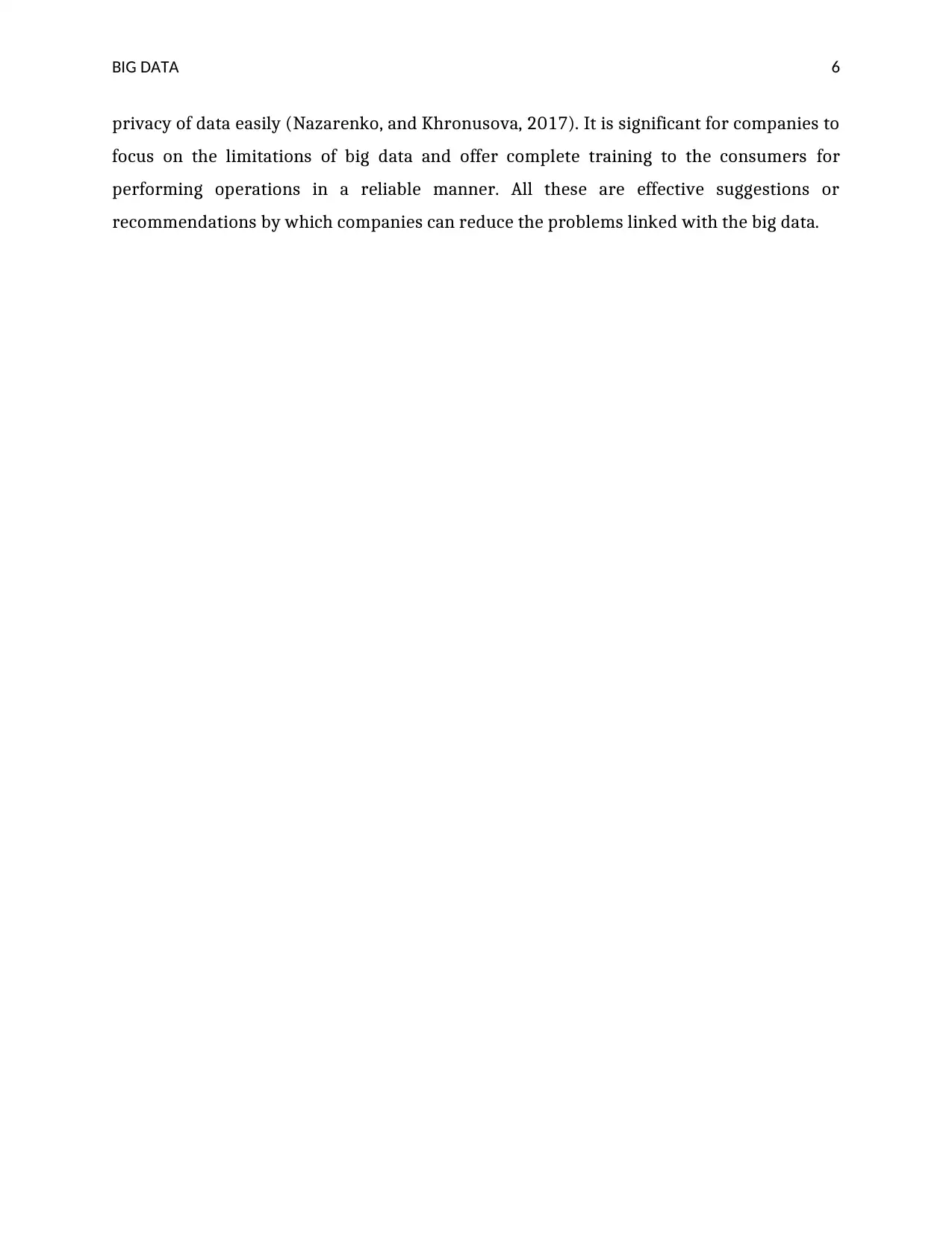
BIG DATA 6
privacy of data easily (Nazarenko, and Khronusova, 2017). It is significant for companies to
focus on the limitations of big data and offer complete training to the consumers for
performing operations in a reliable manner. All these are effective suggestions or
recommendations by which companies can reduce the problems linked with the big data.
privacy of data easily (Nazarenko, and Khronusova, 2017). It is significant for companies to
focus on the limitations of big data and offer complete training to the consumers for
performing operations in a reliable manner. All these are effective suggestions or
recommendations by which companies can reduce the problems linked with the big data.
Paraphrase This Document
Need a fresh take? Get an instant paraphrase of this document with our AI Paraphraser
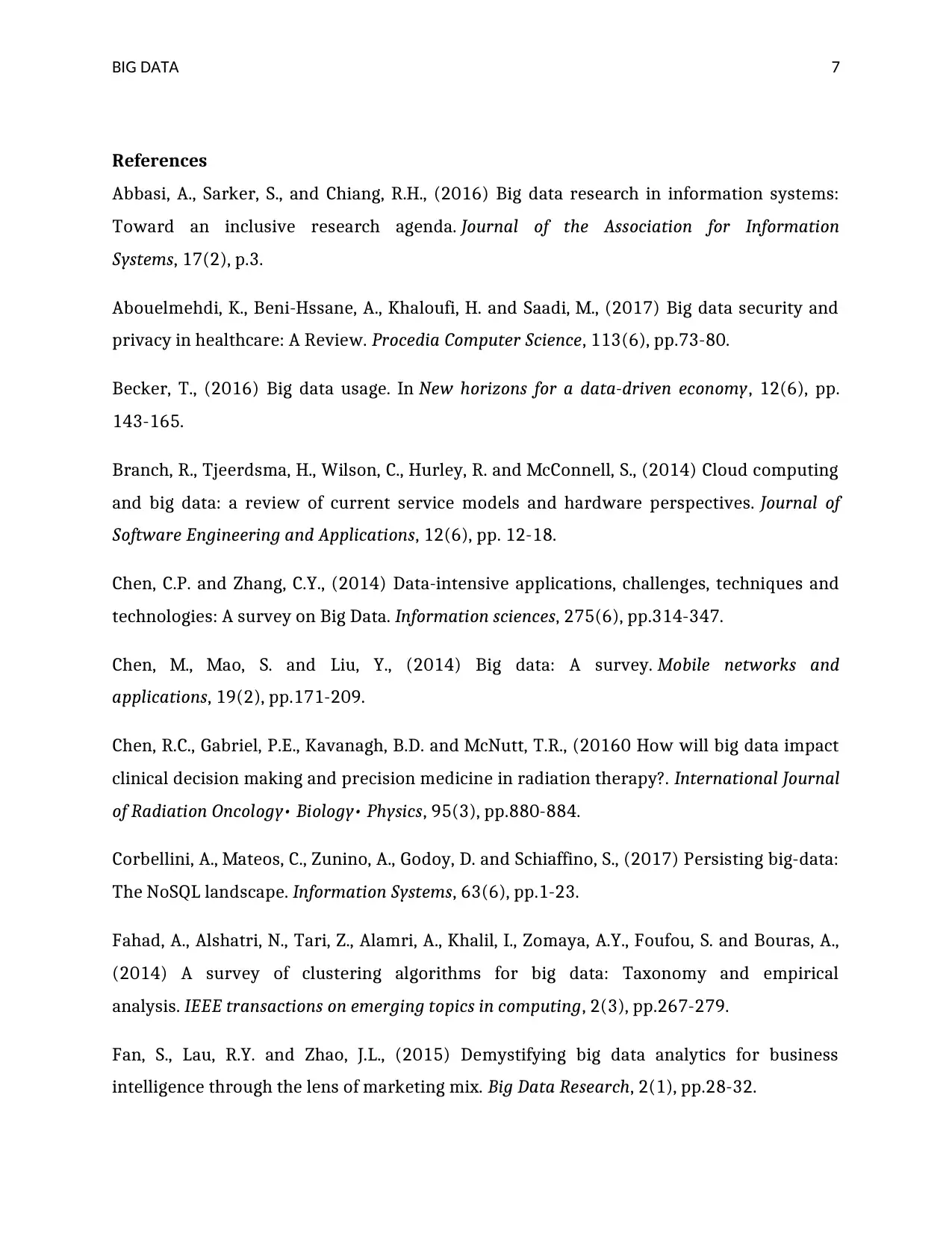
BIG DATA 7
References
Abbasi, A., Sarker, S., and Chiang, R.H., (2016) Big data research in information systems:
Toward an inclusive research agenda. Journal of the Association for Information
Systems, 17(2), p.3.
Abouelmehdi, K., Beni-Hssane, A., Khaloufi, H. and Saadi, M., (2017) Big data security and
privacy in healthcare: A Review. Procedia Computer Science, 113(6), pp.73-80.
Becker, T., (2016) Big data usage. In New horizons for a data-driven economy, 12(6), pp.
143-165.
Branch, R., Tjeerdsma, H., Wilson, C., Hurley, R. and McConnell, S., (2014) Cloud computing
and big data: a review of current service models and hardware perspectives. Journal of
Software Engineering and Applications, 12(6), pp. 12-18.
Chen, C.P. and Zhang, C.Y., (2014) Data-intensive applications, challenges, techniques and
technologies: A survey on Big Data. Information sciences, 275(6), pp.314-347.
Chen, M., Mao, S. and Liu, Y., (2014) Big data: A survey. Mobile networks and
applications, 19(2), pp.171-209.
Chen, R.C., Gabriel, P.E., Kavanagh, B.D. and McNutt, T.R., (20160 How will big data impact
clinical decision making and precision medicine in radiation therapy?. International Journal
of Radiation Oncology• Biology• Physics, 95(3), pp.880-884.
Corbellini, A., Mateos, C., Zunino, A., Godoy, D. and Schiaffino, S., (2017) Persisting big-data:
The NoSQL landscape. Information Systems, 63(6), pp.1-23.
Fahad, A., Alshatri, N., Tari, Z., Alamri, A., Khalil, I., Zomaya, A.Y., Foufou, S. and Bouras, A.,
(2014) A survey of clustering algorithms for big data: Taxonomy and empirical
analysis. IEEE transactions on emerging topics in computing, 2(3), pp.267-279.
Fan, S., Lau, R.Y. and Zhao, J.L., (2015) Demystifying big data analytics for business
intelligence through the lens of marketing mix. Big Data Research, 2(1), pp.28-32.
References
Abbasi, A., Sarker, S., and Chiang, R.H., (2016) Big data research in information systems:
Toward an inclusive research agenda. Journal of the Association for Information
Systems, 17(2), p.3.
Abouelmehdi, K., Beni-Hssane, A., Khaloufi, H. and Saadi, M., (2017) Big data security and
privacy in healthcare: A Review. Procedia Computer Science, 113(6), pp.73-80.
Becker, T., (2016) Big data usage. In New horizons for a data-driven economy, 12(6), pp.
143-165.
Branch, R., Tjeerdsma, H., Wilson, C., Hurley, R. and McConnell, S., (2014) Cloud computing
and big data: a review of current service models and hardware perspectives. Journal of
Software Engineering and Applications, 12(6), pp. 12-18.
Chen, C.P. and Zhang, C.Y., (2014) Data-intensive applications, challenges, techniques and
technologies: A survey on Big Data. Information sciences, 275(6), pp.314-347.
Chen, M., Mao, S. and Liu, Y., (2014) Big data: A survey. Mobile networks and
applications, 19(2), pp.171-209.
Chen, R.C., Gabriel, P.E., Kavanagh, B.D. and McNutt, T.R., (20160 How will big data impact
clinical decision making and precision medicine in radiation therapy?. International Journal
of Radiation Oncology• Biology• Physics, 95(3), pp.880-884.
Corbellini, A., Mateos, C., Zunino, A., Godoy, D. and Schiaffino, S., (2017) Persisting big-data:
The NoSQL landscape. Information Systems, 63(6), pp.1-23.
Fahad, A., Alshatri, N., Tari, Z., Alamri, A., Khalil, I., Zomaya, A.Y., Foufou, S. and Bouras, A.,
(2014) A survey of clustering algorithms for big data: Taxonomy and empirical
analysis. IEEE transactions on emerging topics in computing, 2(3), pp.267-279.
Fan, S., Lau, R.Y. and Zhao, J.L., (2015) Demystifying big data analytics for business
intelligence through the lens of marketing mix. Big Data Research, 2(1), pp.28-32.
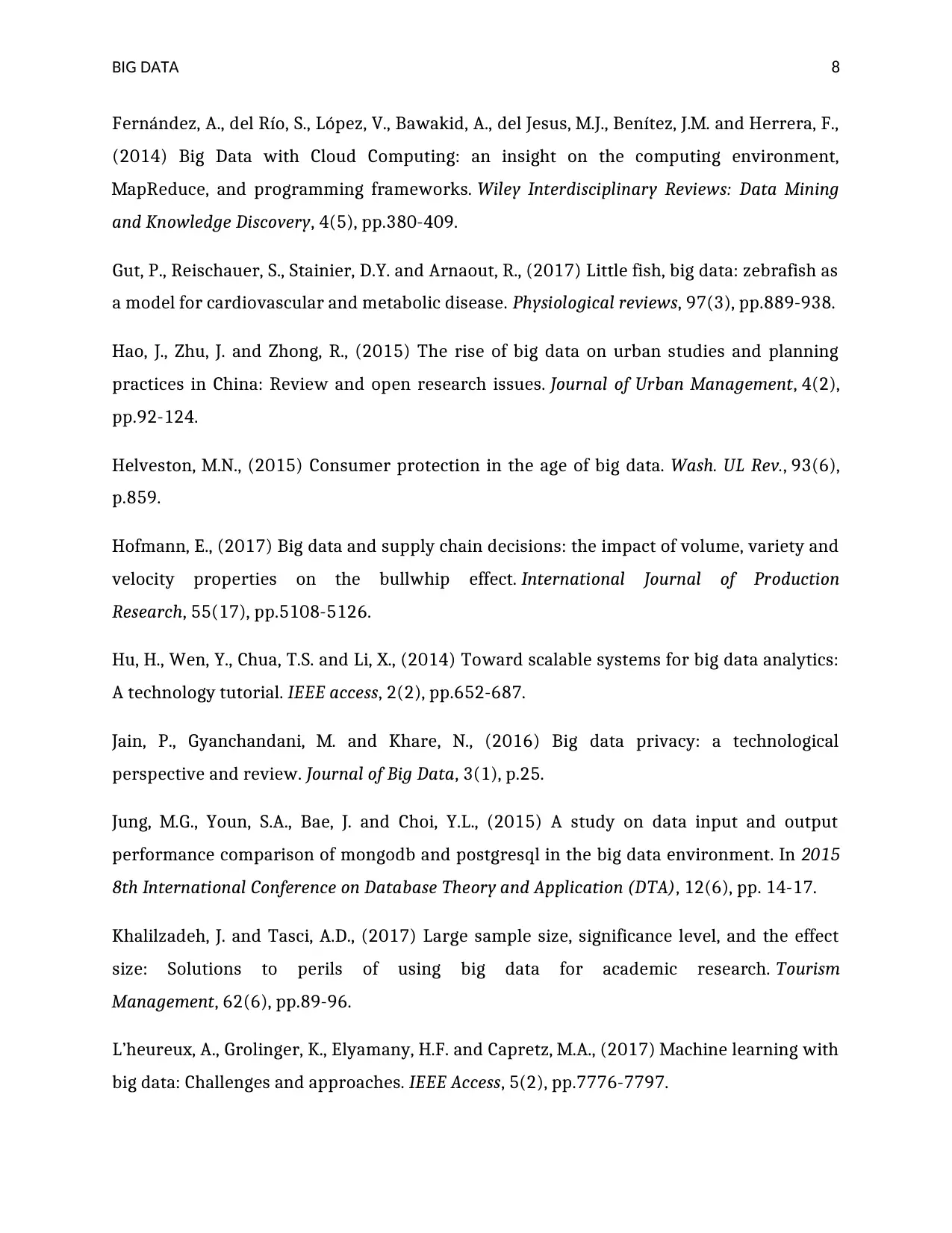
BIG DATA 8
Fernández, A., del Río, S., López, V., Bawakid, A., del Jesus, M.J., Benítez, J.M. and Herrera, F.,
(2014) Big Data with Cloud Computing: an insight on the computing environment,
MapReduce, and programming frameworks. Wiley Interdisciplinary Reviews: Data Mining
and Knowledge Discovery, 4(5), pp.380-409.
Gut, P., Reischauer, S., Stainier, D.Y. and Arnaout, R., (2017) Little fish, big data: zebrafish as
a model for cardiovascular and metabolic disease. Physiological reviews, 97(3), pp.889-938.
Hao, J., Zhu, J. and Zhong, R., (2015) The rise of big data on urban studies and planning
practices in China: Review and open research issues. Journal of Urban Management, 4(2),
pp.92-124.
Helveston, M.N., (2015) Consumer protection in the age of big data. Wash. UL Rev., 93(6),
p.859.
Hofmann, E., (2017) Big data and supply chain decisions: the impact of volume, variety and
velocity properties on the bullwhip effect. International Journal of Production
Research, 55(17), pp.5108-5126.
Hu, H., Wen, Y., Chua, T.S. and Li, X., (2014) Toward scalable systems for big data analytics:
A technology tutorial. IEEE access, 2(2), pp.652-687.
Jain, P., Gyanchandani, M. and Khare, N., (2016) Big data privacy: a technological
perspective and review. Journal of Big Data, 3(1), p.25.
Jung, M.G., Youn, S.A., Bae, J. and Choi, Y.L., (2015) A study on data input and output
performance comparison of mongodb and postgresql in the big data environment. In 2015
8th International Conference on Database Theory and Application (DTA), 12(6), pp. 14-17.
Khalilzadeh, J. and Tasci, A.D., (2017) Large sample size, significance level, and the effect
size: Solutions to perils of using big data for academic research. Tourism
Management, 62(6), pp.89-96.
L’heureux, A., Grolinger, K., Elyamany, H.F. and Capretz, M.A., (2017) Machine learning with
big data: Challenges and approaches. IEEE Access, 5(2), pp.7776-7797.
Fernández, A., del Río, S., López, V., Bawakid, A., del Jesus, M.J., Benítez, J.M. and Herrera, F.,
(2014) Big Data with Cloud Computing: an insight on the computing environment,
MapReduce, and programming frameworks. Wiley Interdisciplinary Reviews: Data Mining
and Knowledge Discovery, 4(5), pp.380-409.
Gut, P., Reischauer, S., Stainier, D.Y. and Arnaout, R., (2017) Little fish, big data: zebrafish as
a model for cardiovascular and metabolic disease. Physiological reviews, 97(3), pp.889-938.
Hao, J., Zhu, J. and Zhong, R., (2015) The rise of big data on urban studies and planning
practices in China: Review and open research issues. Journal of Urban Management, 4(2),
pp.92-124.
Helveston, M.N., (2015) Consumer protection in the age of big data. Wash. UL Rev., 93(6),
p.859.
Hofmann, E., (2017) Big data and supply chain decisions: the impact of volume, variety and
velocity properties on the bullwhip effect. International Journal of Production
Research, 55(17), pp.5108-5126.
Hu, H., Wen, Y., Chua, T.S. and Li, X., (2014) Toward scalable systems for big data analytics:
A technology tutorial. IEEE access, 2(2), pp.652-687.
Jain, P., Gyanchandani, M. and Khare, N., (2016) Big data privacy: a technological
perspective and review. Journal of Big Data, 3(1), p.25.
Jung, M.G., Youn, S.A., Bae, J. and Choi, Y.L., (2015) A study on data input and output
performance comparison of mongodb and postgresql in the big data environment. In 2015
8th International Conference on Database Theory and Application (DTA), 12(6), pp. 14-17.
Khalilzadeh, J. and Tasci, A.D., (2017) Large sample size, significance level, and the effect
size: Solutions to perils of using big data for academic research. Tourism
Management, 62(6), pp.89-96.
L’heureux, A., Grolinger, K., Elyamany, H.F. and Capretz, M.A., (2017) Machine learning with
big data: Challenges and approaches. IEEE Access, 5(2), pp.7776-7797.
You're viewing a preview
Unlock full access by subscribing today!
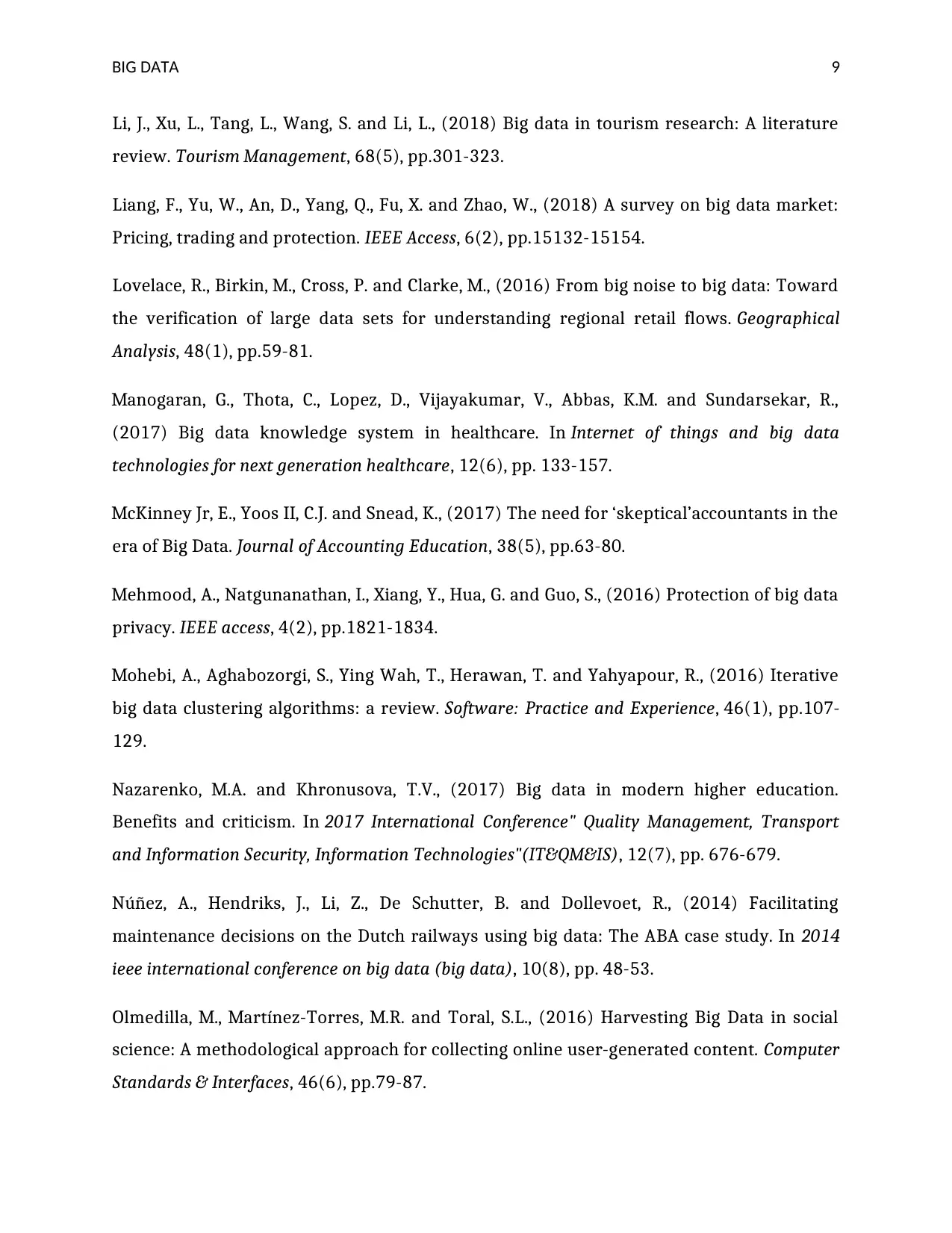
BIG DATA 9
Li, J., Xu, L., Tang, L., Wang, S. and Li, L., (2018) Big data in tourism research: A literature
review. Tourism Management, 68(5), pp.301-323.
Liang, F., Yu, W., An, D., Yang, Q., Fu, X. and Zhao, W., (2018) A survey on big data market:
Pricing, trading and protection. IEEE Access, 6(2), pp.15132-15154.
Lovelace, R., Birkin, M., Cross, P. and Clarke, M., (2016) From big noise to big data: Toward
the verification of large data sets for understanding regional retail flows. Geographical
Analysis, 48(1), pp.59-81.
Manogaran, G., Thota, C., Lopez, D., Vijayakumar, V., Abbas, K.M. and Sundarsekar, R.,
(2017) Big data knowledge system in healthcare. In Internet of things and big data
technologies for next generation healthcare, 12(6), pp. 133-157.
McKinney Jr, E., Yoos II, C.J. and Snead, K., (2017) The need for ‘skeptical’accountants in the
era of Big Data. Journal of Accounting Education, 38(5), pp.63-80.
Mehmood, A., Natgunanathan, I., Xiang, Y., Hua, G. and Guo, S., (2016) Protection of big data
privacy. IEEE access, 4(2), pp.1821-1834.
Mohebi, A., Aghabozorgi, S., Ying Wah, T., Herawan, T. and Yahyapour, R., (2016) Iterative
big data clustering algorithms: a review. Software: Practice and Experience, 46(1), pp.107-
129.
Nazarenko, M.A. and Khronusova, T.V., (2017) Big data in modern higher education.
Benefits and criticism. In 2017 International Conference" Quality Management, Transport
and Information Security, Information Technologies"(IT&QM&IS), 12(7), pp. 676-679.
Núñez, A., Hendriks, J., Li, Z., De Schutter, B. and Dollevoet, R., (2014) Facilitating
maintenance decisions on the Dutch railways using big data: The ABA case study. In 2014
ieee international conference on big data (big data), 10(8), pp. 48-53.
Olmedilla, M., Martínez-Torres, M.R. and Toral, S.L., (2016) Harvesting Big Data in social
science: A methodological approach for collecting online user-generated content. Computer
Standards & Interfaces, 46(6), pp.79-87.
Li, J., Xu, L., Tang, L., Wang, S. and Li, L., (2018) Big data in tourism research: A literature
review. Tourism Management, 68(5), pp.301-323.
Liang, F., Yu, W., An, D., Yang, Q., Fu, X. and Zhao, W., (2018) A survey on big data market:
Pricing, trading and protection. IEEE Access, 6(2), pp.15132-15154.
Lovelace, R., Birkin, M., Cross, P. and Clarke, M., (2016) From big noise to big data: Toward
the verification of large data sets for understanding regional retail flows. Geographical
Analysis, 48(1), pp.59-81.
Manogaran, G., Thota, C., Lopez, D., Vijayakumar, V., Abbas, K.M. and Sundarsekar, R.,
(2017) Big data knowledge system in healthcare. In Internet of things and big data
technologies for next generation healthcare, 12(6), pp. 133-157.
McKinney Jr, E., Yoos II, C.J. and Snead, K., (2017) The need for ‘skeptical’accountants in the
era of Big Data. Journal of Accounting Education, 38(5), pp.63-80.
Mehmood, A., Natgunanathan, I., Xiang, Y., Hua, G. and Guo, S., (2016) Protection of big data
privacy. IEEE access, 4(2), pp.1821-1834.
Mohebi, A., Aghabozorgi, S., Ying Wah, T., Herawan, T. and Yahyapour, R., (2016) Iterative
big data clustering algorithms: a review. Software: Practice and Experience, 46(1), pp.107-
129.
Nazarenko, M.A. and Khronusova, T.V., (2017) Big data in modern higher education.
Benefits and criticism. In 2017 International Conference" Quality Management, Transport
and Information Security, Information Technologies"(IT&QM&IS), 12(7), pp. 676-679.
Núñez, A., Hendriks, J., Li, Z., De Schutter, B. and Dollevoet, R., (2014) Facilitating
maintenance decisions on the Dutch railways using big data: The ABA case study. In 2014
ieee international conference on big data (big data), 10(8), pp. 48-53.
Olmedilla, M., Martínez-Torres, M.R. and Toral, S.L., (2016) Harvesting Big Data in social
science: A methodological approach for collecting online user-generated content. Computer
Standards & Interfaces, 46(6), pp.79-87.
Paraphrase This Document
Need a fresh take? Get an instant paraphrase of this document with our AI Paraphraser
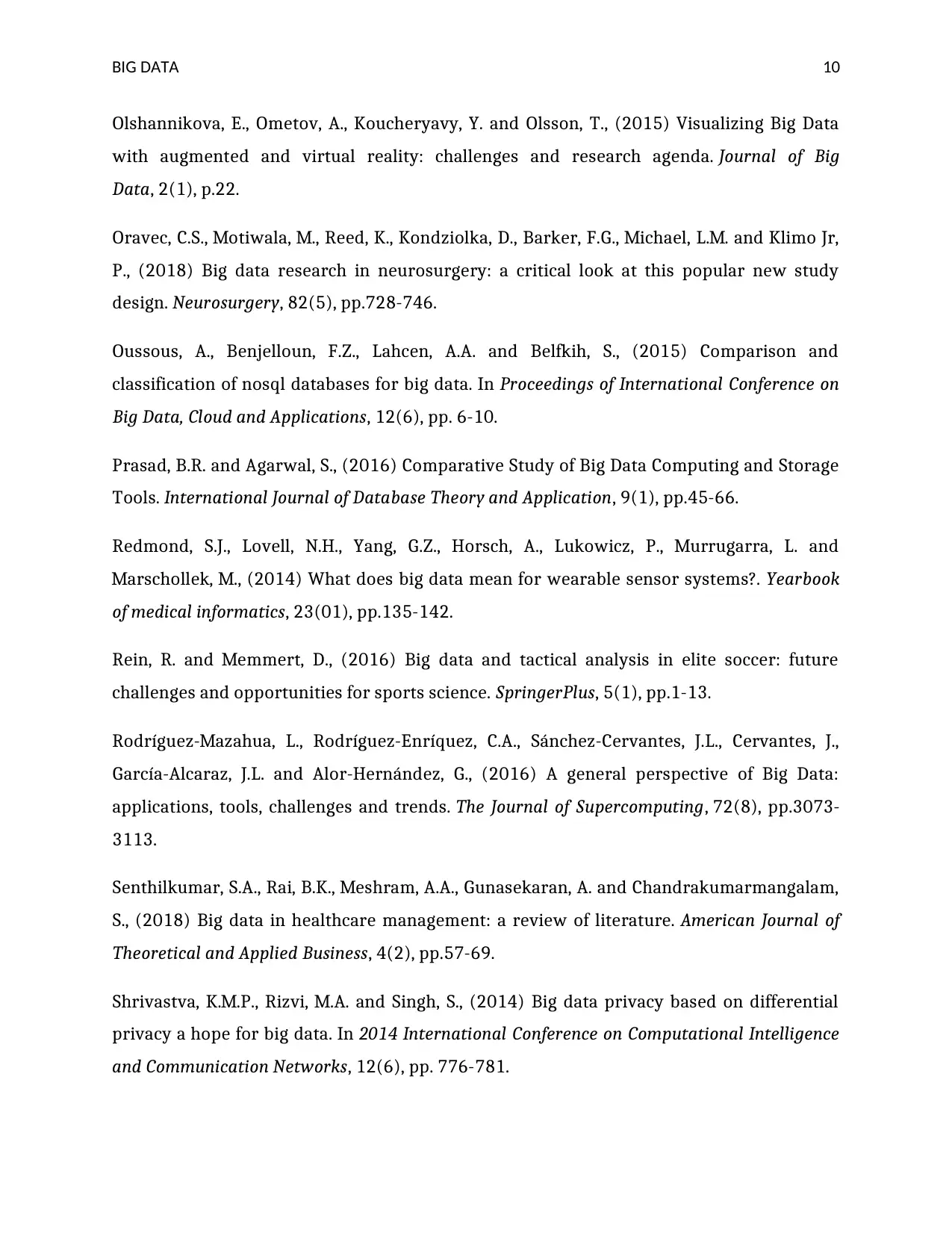
BIG DATA 10
Olshannikova, E., Ometov, A., Koucheryavy, Y. and Olsson, T., (2015) Visualizing Big Data
with augmented and virtual reality: challenges and research agenda. Journal of Big
Data, 2(1), p.22.
Oravec, C.S., Motiwala, M., Reed, K., Kondziolka, D., Barker, F.G., Michael, L.M. and Klimo Jr,
P., (2018) Big data research in neurosurgery: a critical look at this popular new study
design. Neurosurgery, 82(5), pp.728-746.
Oussous, A., Benjelloun, F.Z., Lahcen, A.A. and Belfkih, S., (2015) Comparison and
classification of nosql databases for big data. In Proceedings of International Conference on
Big Data, Cloud and Applications, 12(6), pp. 6-10.
Prasad, B.R. and Agarwal, S., (2016) Comparative Study of Big Data Computing and Storage
Tools. International Journal of Database Theory and Application, 9(1), pp.45-66.
Redmond, S.J., Lovell, N.H., Yang, G.Z., Horsch, A., Lukowicz, P., Murrugarra, L. and
Marschollek, M., (2014) What does big data mean for wearable sensor systems?. Yearbook
of medical informatics, 23(01), pp.135-142.
Rein, R. and Memmert, D., (2016) Big data and tactical analysis in elite soccer: future
challenges and opportunities for sports science. SpringerPlus, 5(1), pp.1-13.
Rodríguez-Mazahua, L., Rodríguez-Enríquez, C.A., Sánchez-Cervantes, J.L., Cervantes, J.,
García-Alcaraz, J.L. and Alor-Hernández, G., (2016) A general perspective of Big Data:
applications, tools, challenges and trends. The Journal of Supercomputing, 72(8), pp.3073-
3113.
Senthilkumar, S.A., Rai, B.K., Meshram, A.A., Gunasekaran, A. and Chandrakumarmangalam,
S., (2018) Big data in healthcare management: a review of literature. American Journal of
Theoretical and Applied Business, 4(2), pp.57-69.
Shrivastva, K.M.P., Rizvi, M.A. and Singh, S., (2014) Big data privacy based on differential
privacy a hope for big data. In 2014 International Conference on Computational Intelligence
and Communication Networks, 12(6), pp. 776-781.
Olshannikova, E., Ometov, A., Koucheryavy, Y. and Olsson, T., (2015) Visualizing Big Data
with augmented and virtual reality: challenges and research agenda. Journal of Big
Data, 2(1), p.22.
Oravec, C.S., Motiwala, M., Reed, K., Kondziolka, D., Barker, F.G., Michael, L.M. and Klimo Jr,
P., (2018) Big data research in neurosurgery: a critical look at this popular new study
design. Neurosurgery, 82(5), pp.728-746.
Oussous, A., Benjelloun, F.Z., Lahcen, A.A. and Belfkih, S., (2015) Comparison and
classification of nosql databases for big data. In Proceedings of International Conference on
Big Data, Cloud and Applications, 12(6), pp. 6-10.
Prasad, B.R. and Agarwal, S., (2016) Comparative Study of Big Data Computing and Storage
Tools. International Journal of Database Theory and Application, 9(1), pp.45-66.
Redmond, S.J., Lovell, N.H., Yang, G.Z., Horsch, A., Lukowicz, P., Murrugarra, L. and
Marschollek, M., (2014) What does big data mean for wearable sensor systems?. Yearbook
of medical informatics, 23(01), pp.135-142.
Rein, R. and Memmert, D., (2016) Big data and tactical analysis in elite soccer: future
challenges and opportunities for sports science. SpringerPlus, 5(1), pp.1-13.
Rodríguez-Mazahua, L., Rodríguez-Enríquez, C.A., Sánchez-Cervantes, J.L., Cervantes, J.,
García-Alcaraz, J.L. and Alor-Hernández, G., (2016) A general perspective of Big Data:
applications, tools, challenges and trends. The Journal of Supercomputing, 72(8), pp.3073-
3113.
Senthilkumar, S.A., Rai, B.K., Meshram, A.A., Gunasekaran, A. and Chandrakumarmangalam,
S., (2018) Big data in healthcare management: a review of literature. American Journal of
Theoretical and Applied Business, 4(2), pp.57-69.
Shrivastva, K.M.P., Rizvi, M.A. and Singh, S., (2014) Big data privacy based on differential
privacy a hope for big data. In 2014 International Conference on Computational Intelligence
and Communication Networks, 12(6), pp. 776-781.
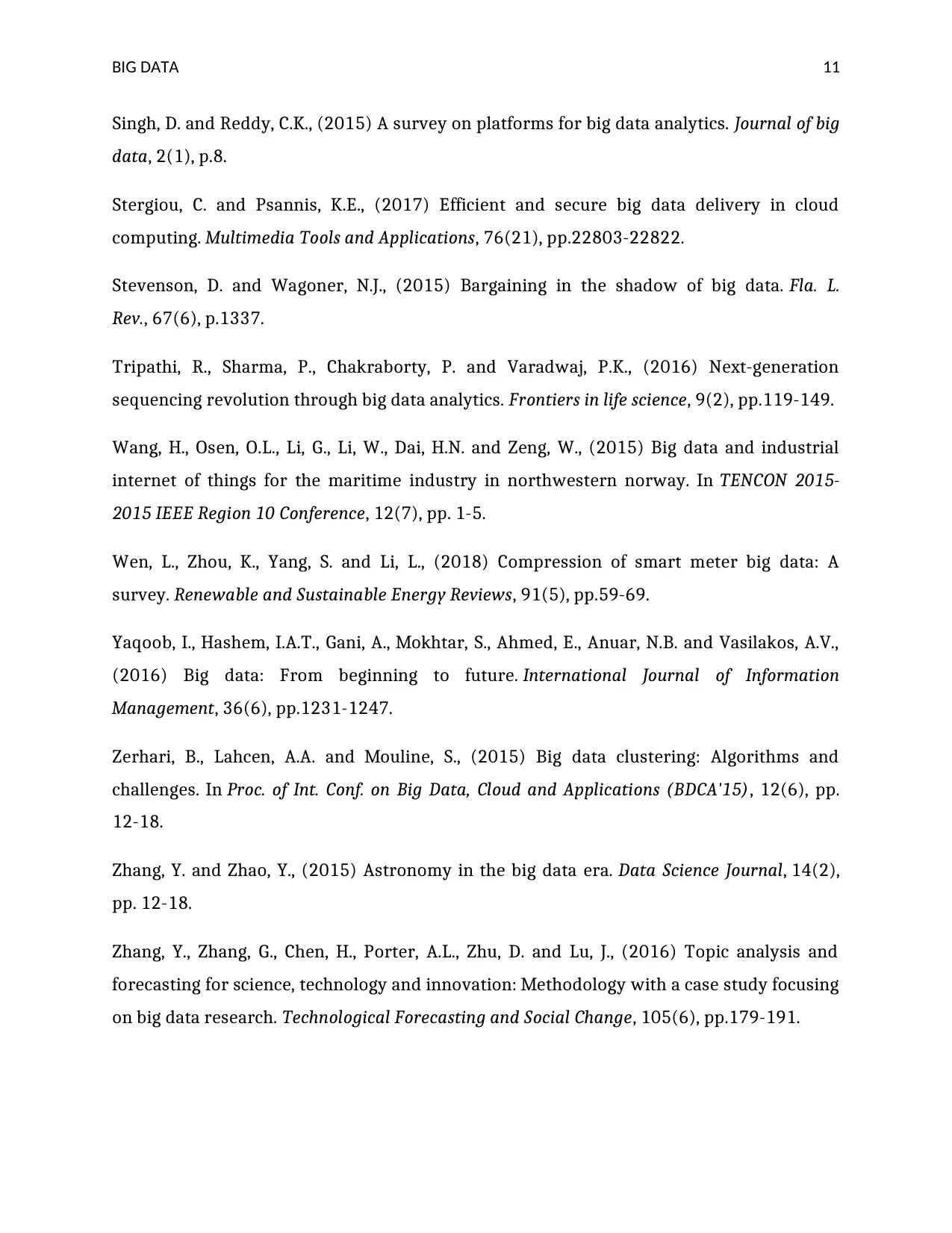
BIG DATA 11
Singh, D. and Reddy, C.K., (2015) A survey on platforms for big data analytics. Journal of big
data, 2(1), p.8.
Stergiou, C. and Psannis, K.E., (2017) Efficient and secure big data delivery in cloud
computing. Multimedia Tools and Applications, 76(21), pp.22803-22822.
Stevenson, D. and Wagoner, N.J., (2015) Bargaining in the shadow of big data. Fla. L.
Rev., 67(6), p.1337.
Tripathi, R., Sharma, P., Chakraborty, P. and Varadwaj, P.K., (2016) Next-generation
sequencing revolution through big data analytics. Frontiers in life science, 9(2), pp.119-149.
Wang, H., Osen, O.L., Li, G., Li, W., Dai, H.N. and Zeng, W., (2015) Big data and industrial
internet of things for the maritime industry in northwestern norway. In TENCON 2015-
2015 IEEE Region 10 Conference, 12(7), pp. 1-5.
Wen, L., Zhou, K., Yang, S. and Li, L., (2018) Compression of smart meter big data: A
survey. Renewable and Sustainable Energy Reviews, 91(5), pp.59-69.
Yaqoob, I., Hashem, I.A.T., Gani, A., Mokhtar, S., Ahmed, E., Anuar, N.B. and Vasilakos, A.V.,
(2016) Big data: From beginning to future. International Journal of Information
Management, 36(6), pp.1231-1247.
Zerhari, B., Lahcen, A.A. and Mouline, S., (2015) Big data clustering: Algorithms and
challenges. In Proc. of Int. Conf. on Big Data, Cloud and Applications (BDCA'15), 12(6), pp.
12-18.
Zhang, Y. and Zhao, Y., (2015) Astronomy in the big data era. Data Science Journal, 14(2),
pp. 12-18.
Zhang, Y., Zhang, G., Chen, H., Porter, A.L., Zhu, D. and Lu, J., (2016) Topic analysis and
forecasting for science, technology and innovation: Methodology with a case study focusing
on big data research. Technological Forecasting and Social Change, 105(6), pp.179-191.
Singh, D. and Reddy, C.K., (2015) A survey on platforms for big data analytics. Journal of big
data, 2(1), p.8.
Stergiou, C. and Psannis, K.E., (2017) Efficient and secure big data delivery in cloud
computing. Multimedia Tools and Applications, 76(21), pp.22803-22822.
Stevenson, D. and Wagoner, N.J., (2015) Bargaining in the shadow of big data. Fla. L.
Rev., 67(6), p.1337.
Tripathi, R., Sharma, P., Chakraborty, P. and Varadwaj, P.K., (2016) Next-generation
sequencing revolution through big data analytics. Frontiers in life science, 9(2), pp.119-149.
Wang, H., Osen, O.L., Li, G., Li, W., Dai, H.N. and Zeng, W., (2015) Big data and industrial
internet of things for the maritime industry in northwestern norway. In TENCON 2015-
2015 IEEE Region 10 Conference, 12(7), pp. 1-5.
Wen, L., Zhou, K., Yang, S. and Li, L., (2018) Compression of smart meter big data: A
survey. Renewable and Sustainable Energy Reviews, 91(5), pp.59-69.
Yaqoob, I., Hashem, I.A.T., Gani, A., Mokhtar, S., Ahmed, E., Anuar, N.B. and Vasilakos, A.V.,
(2016) Big data: From beginning to future. International Journal of Information
Management, 36(6), pp.1231-1247.
Zerhari, B., Lahcen, A.A. and Mouline, S., (2015) Big data clustering: Algorithms and
challenges. In Proc. of Int. Conf. on Big Data, Cloud and Applications (BDCA'15), 12(6), pp.
12-18.
Zhang, Y. and Zhao, Y., (2015) Astronomy in the big data era. Data Science Journal, 14(2),
pp. 12-18.
Zhang, Y., Zhang, G., Chen, H., Porter, A.L., Zhu, D. and Lu, J., (2016) Topic analysis and
forecasting for science, technology and innovation: Methodology with a case study focusing
on big data research. Technological Forecasting and Social Change, 105(6), pp.179-191.
You're viewing a preview
Unlock full access by subscribing today!
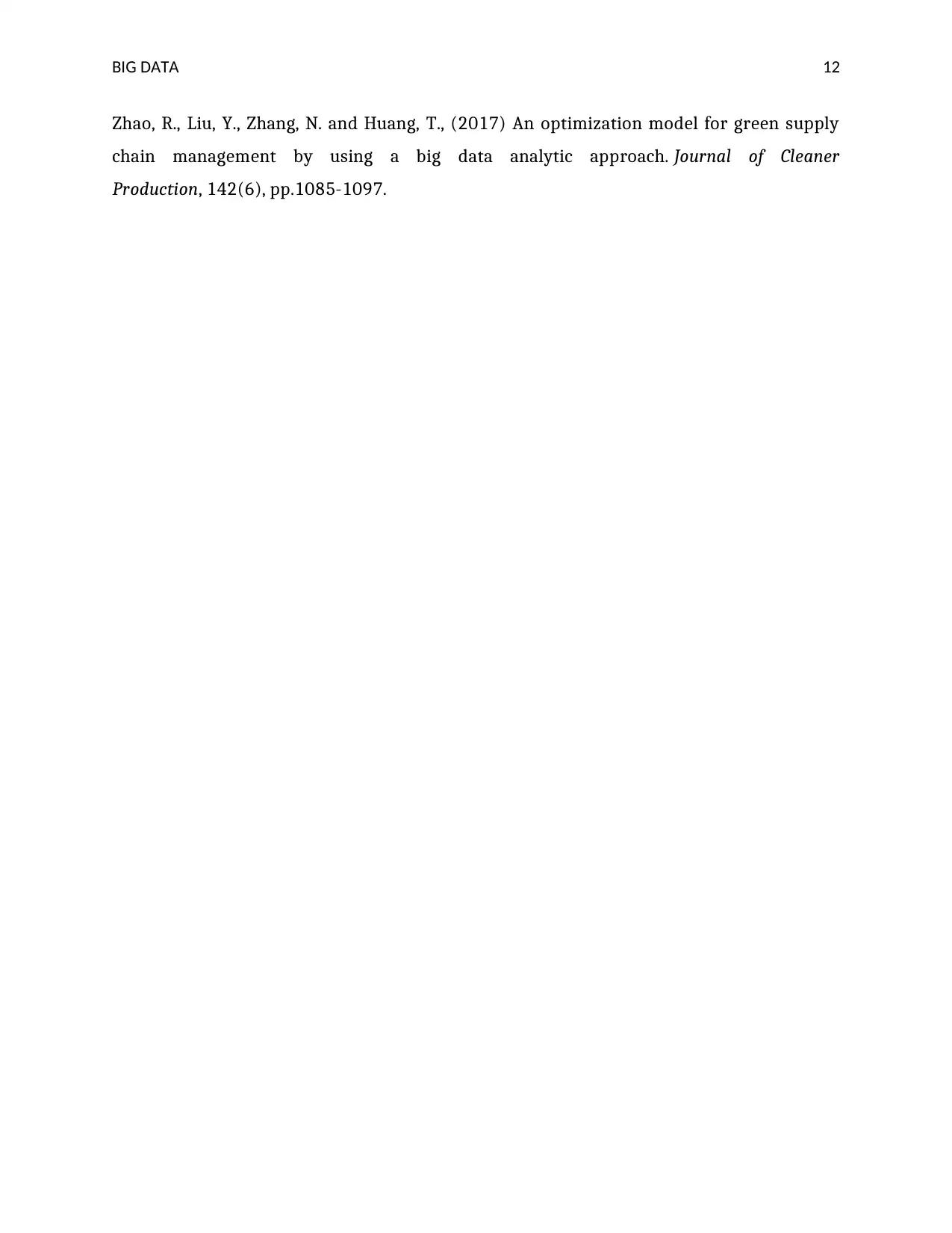
BIG DATA 12
Zhao, R., Liu, Y., Zhang, N. and Huang, T., (2017) An optimization model for green supply
chain management by using a big data analytic approach. Journal of Cleaner
Production, 142(6), pp.1085-1097.
Zhao, R., Liu, Y., Zhang, N. and Huang, T., (2017) An optimization model for green supply
chain management by using a big data analytic approach. Journal of Cleaner
Production, 142(6), pp.1085-1097.
1 out of 13
Related Documents

Your All-in-One AI-Powered Toolkit for Academic Success.
+13062052269
info@desklib.com
Available 24*7 on WhatsApp / Email
Unlock your academic potential
© 2024 | Zucol Services PVT LTD | All rights reserved.