INF80031 Applied Business Analytics: Textual Data Exploitation
VerifiedAdded on 2022/04/26
|13
|4482
|435
Report
AI Summary
This report examines the application of text analytics within the retail and hospitality industries, focusing on how businesses can better leverage their textual data. It begins with an introduction highlighting the impact of technology and the need for businesses to adapt to evolving customer expectations. The report then provides an overview of the retail and hospitality sectors, identifying key challenges such as understanding customer requirements, addressing issues like human trafficking, improving customer service, and adapting to market trends. It emphasizes the benefits of text analytics, including a better understanding of customer needs and market dynamics. The core of the report explores text analytics strategies like sentiment analysis and cross-channel analytics, along with their associated challenges. Sentiment analysis is discussed as a key strategy for understanding customer opinions, while cross-channel analytics helps in understanding customer purchase processes. The report concludes with recommendations for implementing these strategies to improve business performance and customer satisfaction.
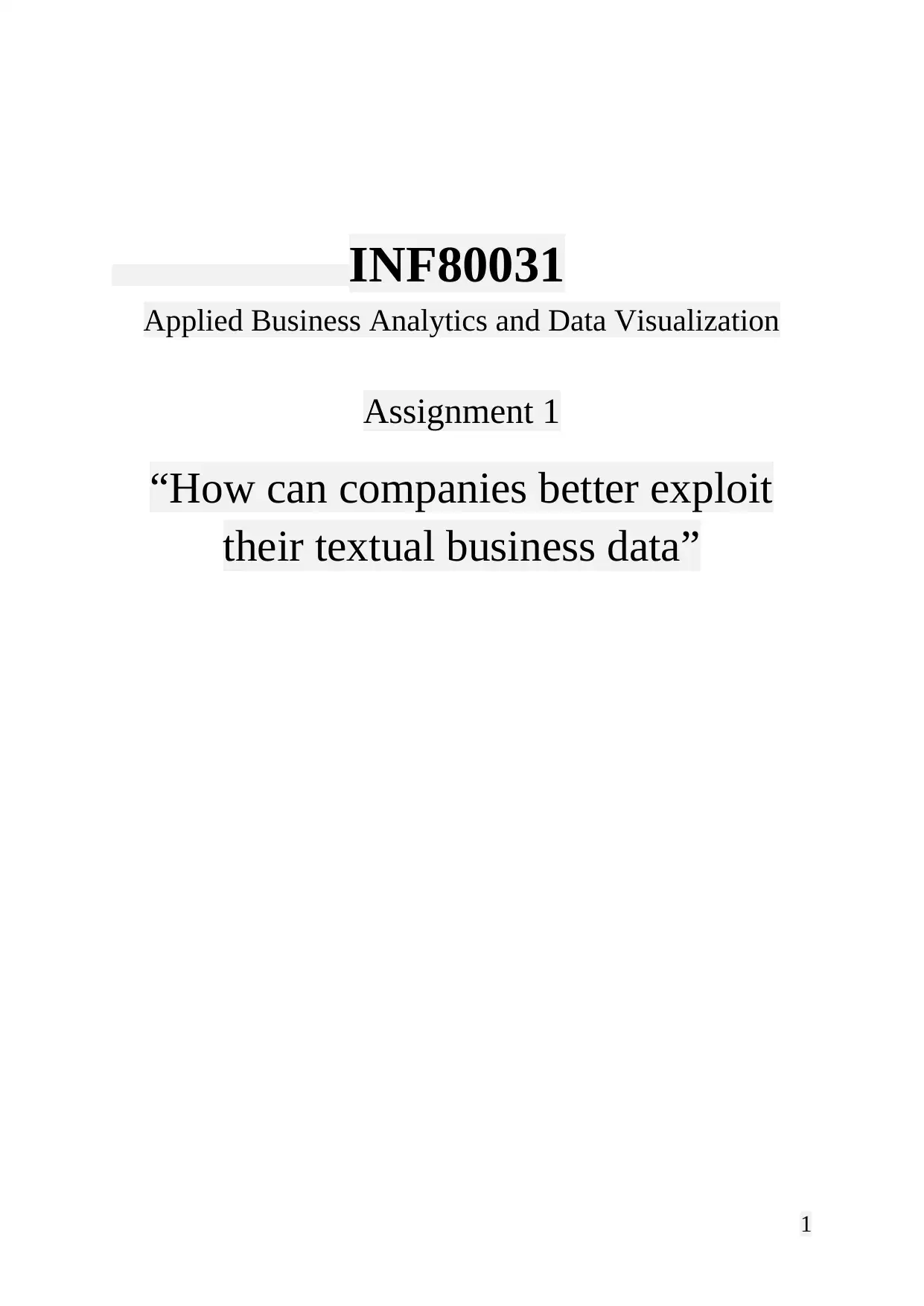
INF80031
Applied Business Analytics and Data Visualization
Assignment 1
“How can companies better exploit
their textual business data”
1
Applied Business Analytics and Data Visualization
Assignment 1
“How can companies better exploit
their textual business data”
1
Paraphrase This Document
Need a fresh take? Get an instant paraphrase of this document with our AI Paraphraser
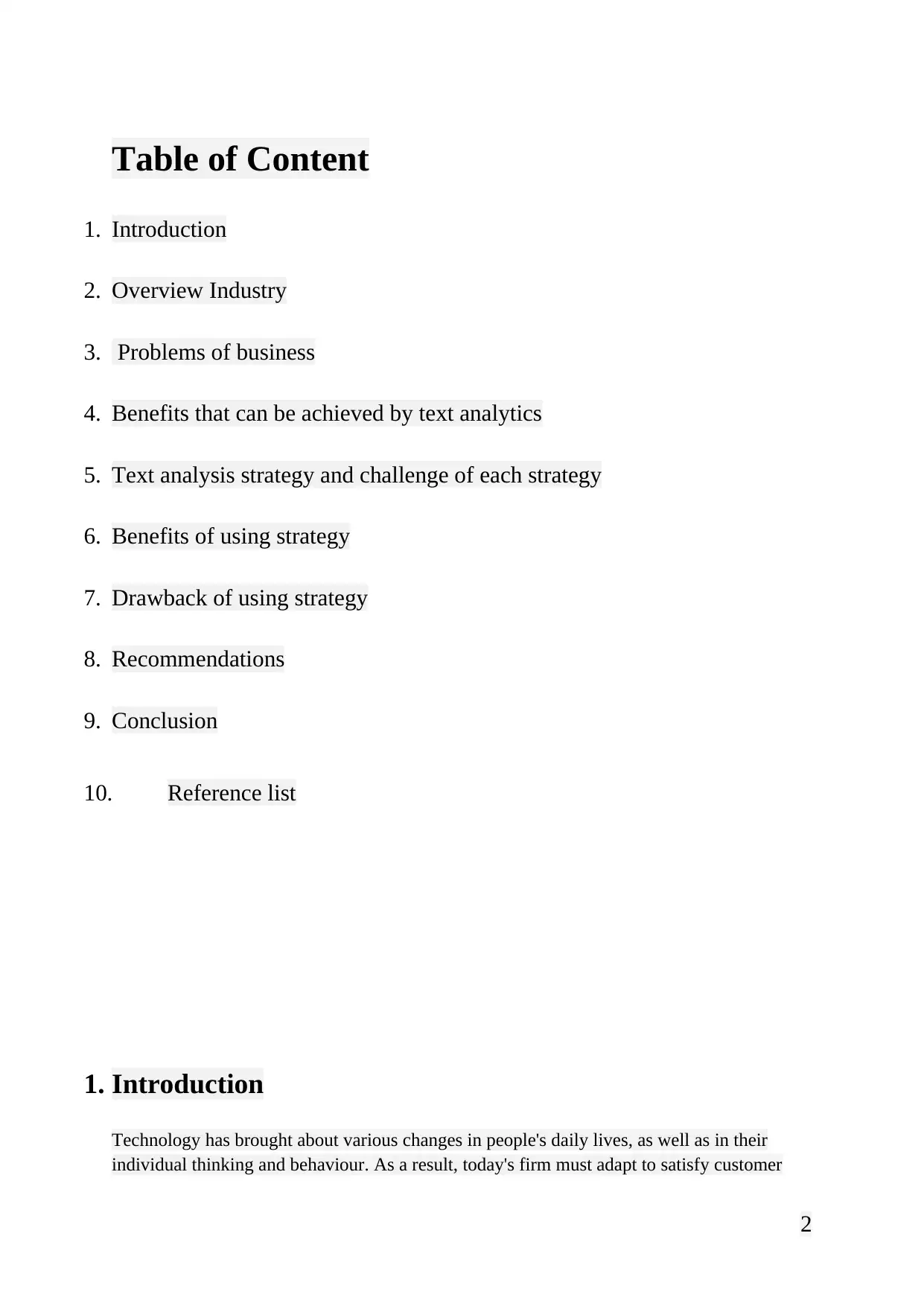
Table of Content
1. Introduction
2. Overview Industry
3. Problems of business
4. Benefits that can be achieved by text analytics
5. Text analysis strategy and challenge of each strategy
6. Benefits of using strategy
7. Drawback of using strategy
8. Recommendations
9. Conclusion
10. Reference list
1. Introduction
Technology has brought about various changes in people's daily lives, as well as in their
individual thinking and behaviour. As a result, today's firm must adapt to satisfy customer
2
1. Introduction
2. Overview Industry
3. Problems of business
4. Benefits that can be achieved by text analytics
5. Text analysis strategy and challenge of each strategy
6. Benefits of using strategy
7. Drawback of using strategy
8. Recommendations
9. Conclusion
10. Reference list
1. Introduction
Technology has brought about various changes in people's daily lives, as well as in their
individual thinking and behaviour. As a result, today's firm must adapt to satisfy customer
2
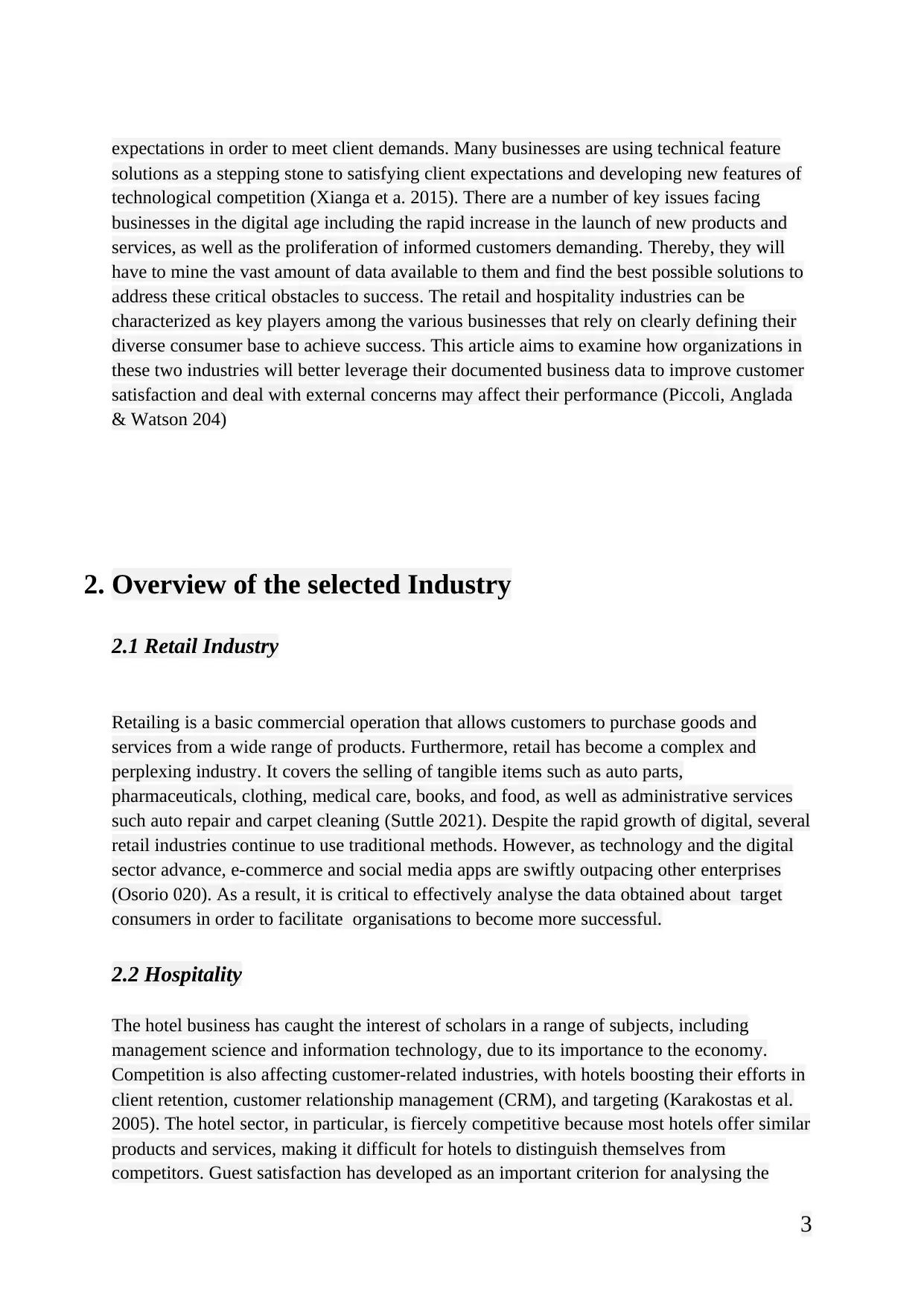
expectations in order to meet client demands. Many businesses are using technical feature
solutions as a stepping stone to satisfying client expectations and developing new features of
technological competition (Xianga et a. 2015). There are a number of key issues facing
businesses in the digital age including the rapid increase in the launch of new products and
services, as well as the proliferation of informed customers demanding. Thereby, they will
have to mine the vast amount of data available to them and find the best possible solutions to
address these critical obstacles to success. The retail and hospitality industries can be
characterized as key players among the various businesses that rely on clearly defining their
diverse consumer base to achieve success. This article aims to examine how organizations in
these two industries will better leverage their documented business data to improve customer
satisfaction and deal with external concerns may affect their performance (Piccoli, Anglada
& Watson 204)
2. Overview of the selected Industry
2.1 Retail Industry
Retailing is a basic commercial operation that allows customers to purchase goods and
services from a wide range of products. Furthermore, retail has become a complex and
perplexing industry. It covers the selling of tangible items such as auto parts,
pharmaceuticals, clothing, medical care, books, and food, as well as administrative services
such auto repair and carpet cleaning (Suttle 2021). Despite the rapid growth of digital, several
retail industries continue to use traditional methods. However, as technology and the digital
sector advance, e-commerce and social media apps are swiftly outpacing other enterprises
(Osorio 020). As a result, it is critical to effectively analyse the data obtained about target
consumers in order to facilitate organisations to become more successful.
2.2 Hospitality
The hotel business has caught the interest of scholars in a range of subjects, including
management science and information technology, due to its importance to the economy.
Competition is also affecting customer-related industries, with hotels boosting their efforts in
client retention, customer relationship management (CRM), and targeting (Karakostas et al.
2005). The hotel sector, in particular, is fiercely competitive because most hotels offer similar
products and services, making it difficult for hotels to distinguish themselves from
competitors. Guest satisfaction has developed as an important criterion for analysing the
3
solutions as a stepping stone to satisfying client expectations and developing new features of
technological competition (Xianga et a. 2015). There are a number of key issues facing
businesses in the digital age including the rapid increase in the launch of new products and
services, as well as the proliferation of informed customers demanding. Thereby, they will
have to mine the vast amount of data available to them and find the best possible solutions to
address these critical obstacles to success. The retail and hospitality industries can be
characterized as key players among the various businesses that rely on clearly defining their
diverse consumer base to achieve success. This article aims to examine how organizations in
these two industries will better leverage their documented business data to improve customer
satisfaction and deal with external concerns may affect their performance (Piccoli, Anglada
& Watson 204)
2. Overview of the selected Industry
2.1 Retail Industry
Retailing is a basic commercial operation that allows customers to purchase goods and
services from a wide range of products. Furthermore, retail has become a complex and
perplexing industry. It covers the selling of tangible items such as auto parts,
pharmaceuticals, clothing, medical care, books, and food, as well as administrative services
such auto repair and carpet cleaning (Suttle 2021). Despite the rapid growth of digital, several
retail industries continue to use traditional methods. However, as technology and the digital
sector advance, e-commerce and social media apps are swiftly outpacing other enterprises
(Osorio 020). As a result, it is critical to effectively analyse the data obtained about target
consumers in order to facilitate organisations to become more successful.
2.2 Hospitality
The hotel business has caught the interest of scholars in a range of subjects, including
management science and information technology, due to its importance to the economy.
Competition is also affecting customer-related industries, with hotels boosting their efforts in
client retention, customer relationship management (CRM), and targeting (Karakostas et al.
2005). The hotel sector, in particular, is fiercely competitive because most hotels offer similar
products and services, making it difficult for hotels to distinguish themselves from
competitors. Guest satisfaction has developed as an important criterion for analysing the
3
You're viewing a preview
Unlock full access by subscribing today!
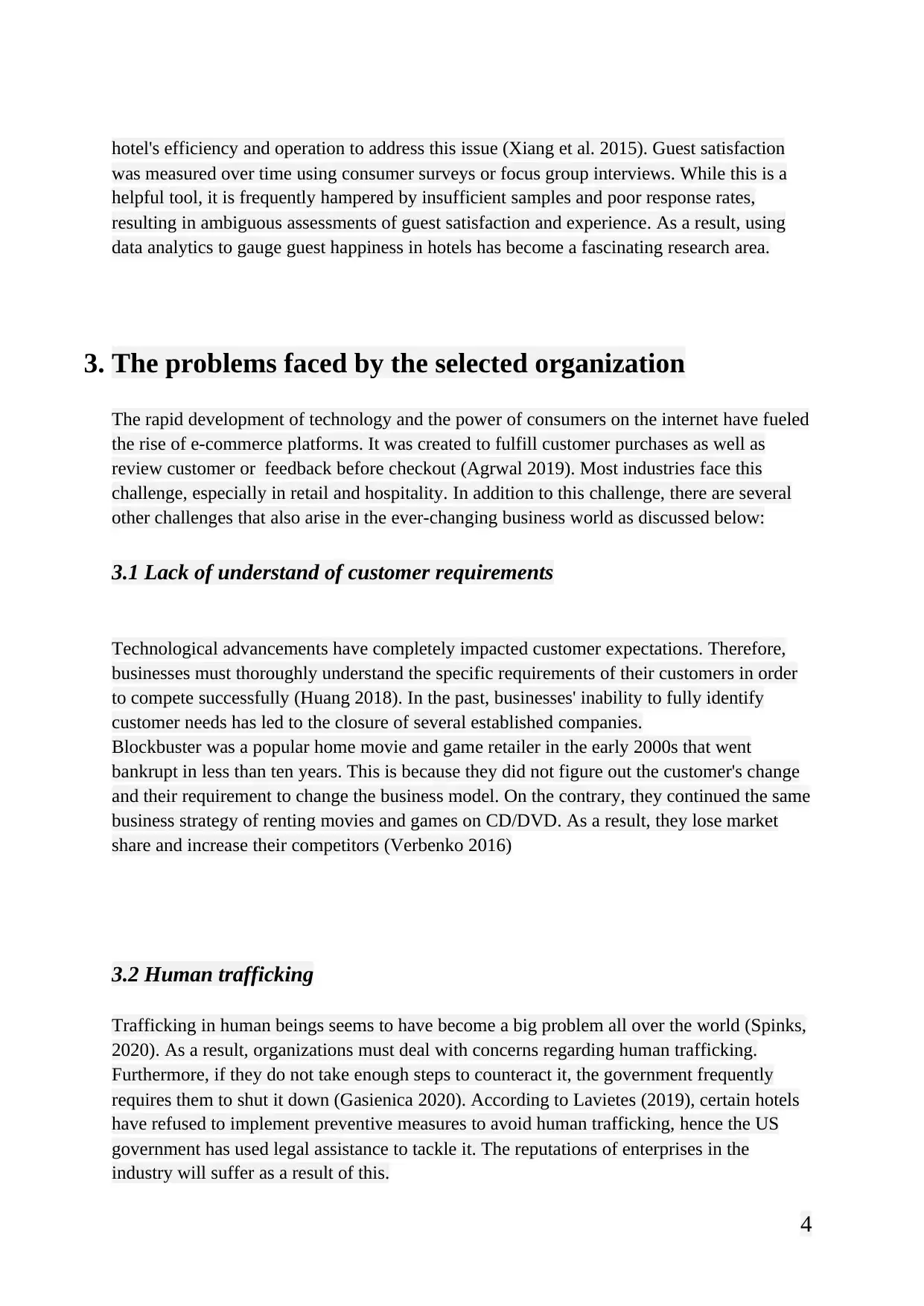
hotel's efficiency and operation to address this issue (Xiang et al. 2015). Guest satisfaction
was measured over time using consumer surveys or focus group interviews. While this is a
helpful tool, it is frequently hampered by insufficient samples and poor response rates,
resulting in ambiguous assessments of guest satisfaction and experience. As a result, using
data analytics to gauge guest happiness in hotels has become a fascinating research area.
3. The problems faced by the selected organization
The rapid development of technology and the power of consumers on the internet have fueled
the rise of e-commerce platforms. It was created to fulfill customer purchases as well as
review customer or feedback before checkout (Agrwal 2019). Most industries face this
challenge, especially in retail and hospitality. In addition to this challenge, there are several
other challenges that also arise in the ever-changing business world as discussed below:
3.1 Lack of understand of customer requirements
Technological advancements have completely impacted customer expectations. Therefore,
businesses must thoroughly understand the specific requirements of their customers in order
to compete successfully (Huang 2018). In the past, businesses' inability to fully identify
customer needs has led to the closure of several established companies.
Blockbuster was a popular home movie and game retailer in the early 2000s that went
bankrupt in less than ten years. This is because they did not figure out the customer's change
and their requirement to change the business model. On the contrary, they continued the same
business strategy of renting movies and games on CD/DVD. As a result, they lose market
share and increase their competitors (Verbenko 2016)
3.2 Human trafficking
Trafficking in human beings seems to have become a big problem all over the world (Spinks,
2020). As a result, organizations must deal with concerns regarding human trafficking.
Furthermore, if they do not take enough steps to counteract it, the government frequently
requires them to shut it down (Gasienica 2020). According to Lavietes (2019), certain hotels
have refused to implement preventive measures to avoid human trafficking, hence the US
government has used legal assistance to tackle it. The reputations of enterprises in the
industry will suffer as a result of this.
4
was measured over time using consumer surveys or focus group interviews. While this is a
helpful tool, it is frequently hampered by insufficient samples and poor response rates,
resulting in ambiguous assessments of guest satisfaction and experience. As a result, using
data analytics to gauge guest happiness in hotels has become a fascinating research area.
3. The problems faced by the selected organization
The rapid development of technology and the power of consumers on the internet have fueled
the rise of e-commerce platforms. It was created to fulfill customer purchases as well as
review customer or feedback before checkout (Agrwal 2019). Most industries face this
challenge, especially in retail and hospitality. In addition to this challenge, there are several
other challenges that also arise in the ever-changing business world as discussed below:
3.1 Lack of understand of customer requirements
Technological advancements have completely impacted customer expectations. Therefore,
businesses must thoroughly understand the specific requirements of their customers in order
to compete successfully (Huang 2018). In the past, businesses' inability to fully identify
customer needs has led to the closure of several established companies.
Blockbuster was a popular home movie and game retailer in the early 2000s that went
bankrupt in less than ten years. This is because they did not figure out the customer's change
and their requirement to change the business model. On the contrary, they continued the same
business strategy of renting movies and games on CD/DVD. As a result, they lose market
share and increase their competitors (Verbenko 2016)
3.2 Human trafficking
Trafficking in human beings seems to have become a big problem all over the world (Spinks,
2020). As a result, organizations must deal with concerns regarding human trafficking.
Furthermore, if they do not take enough steps to counteract it, the government frequently
requires them to shut it down (Gasienica 2020). According to Lavietes (2019), certain hotels
have refused to implement preventive measures to avoid human trafficking, hence the US
government has used legal assistance to tackle it. The reputations of enterprises in the
industry will suffer as a result of this.
4
Paraphrase This Document
Need a fresh take? Get an instant paraphrase of this document with our AI Paraphraser
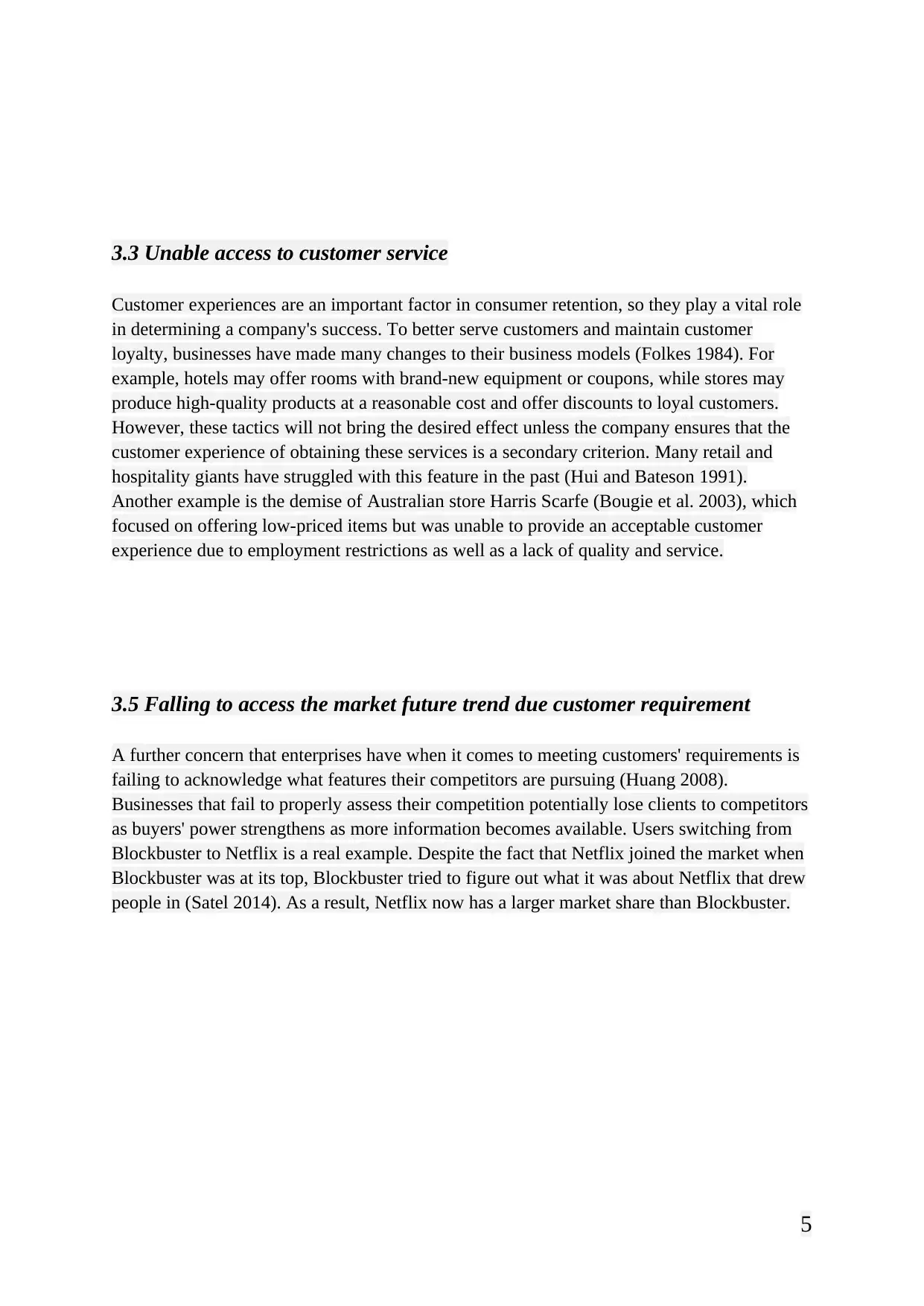
3.3 Unable access to customer service
Customer experiences are an important factor in consumer retention, so they play a vital role
in determining a company's success. To better serve customers and maintain customer
loyalty, businesses have made many changes to their business models (Folkes 1984). For
example, hotels may offer rooms with brand-new equipment or coupons, while stores may
produce high-quality products at a reasonable cost and offer discounts to loyal customers.
However, these tactics will not bring the desired effect unless the company ensures that the
customer experience of obtaining these services is a secondary criterion. Many retail and
hospitality giants have struggled with this feature in the past (Hui and Bateson 1991).
Another example is the demise of Australian store Harris Scarfe (Bougie et al. 2003), which
focused on offering low-priced items but was unable to provide an acceptable customer
experience due to employment restrictions as well as a lack of quality and service.
3.5 Falling to access the market future trend due customer requirement
A further concern that enterprises have when it comes to meeting customers' requirements is
failing to acknowledge what features their competitors are pursuing (Huang 2008).
Businesses that fail to properly assess their competition potentially lose clients to competitors
as buyers' power strengthens as more information becomes available. Users switching from
Blockbuster to Netflix is a real example. Despite the fact that Netflix joined the market when
Blockbuster was at its top, Blockbuster tried to figure out what it was about Netflix that drew
people in (Satel 2014). As a result, Netflix now has a larger market share than Blockbuster.
5
Customer experiences are an important factor in consumer retention, so they play a vital role
in determining a company's success. To better serve customers and maintain customer
loyalty, businesses have made many changes to their business models (Folkes 1984). For
example, hotels may offer rooms with brand-new equipment or coupons, while stores may
produce high-quality products at a reasonable cost and offer discounts to loyal customers.
However, these tactics will not bring the desired effect unless the company ensures that the
customer experience of obtaining these services is a secondary criterion. Many retail and
hospitality giants have struggled with this feature in the past (Hui and Bateson 1991).
Another example is the demise of Australian store Harris Scarfe (Bougie et al. 2003), which
focused on offering low-priced items but was unable to provide an acceptable customer
experience due to employment restrictions as well as a lack of quality and service.
3.5 Falling to access the market future trend due customer requirement
A further concern that enterprises have when it comes to meeting customers' requirements is
failing to acknowledge what features their competitors are pursuing (Huang 2008).
Businesses that fail to properly assess their competition potentially lose clients to competitors
as buyers' power strengthens as more information becomes available. Users switching from
Blockbuster to Netflix is a real example. Despite the fact that Netflix joined the market when
Blockbuster was at its top, Blockbuster tried to figure out what it was about Netflix that drew
people in (Satel 2014). As a result, Netflix now has a larger market share than Blockbuster.
5
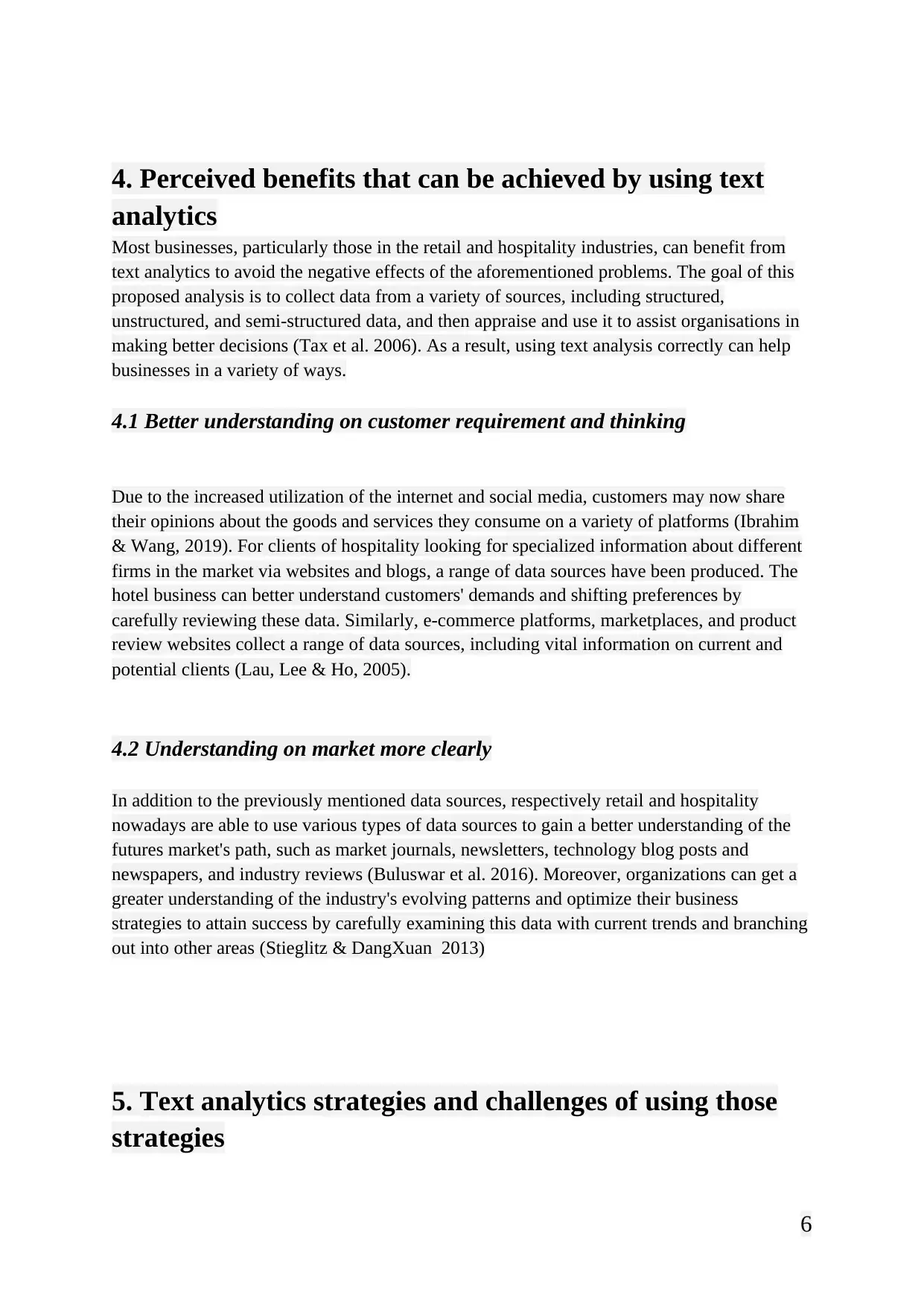
4. Perceived benefits that can be achieved by using text
analytics
Most businesses, particularly those in the retail and hospitality industries, can benefit from
text analytics to avoid the negative effects of the aforementioned problems. The goal of this
proposed analysis is to collect data from a variety of sources, including structured,
unstructured, and semi-structured data, and then appraise and use it to assist organisations in
making better decisions (Tax et al. 2006). As a result, using text analysis correctly can help
businesses in a variety of ways.
4.1 Better understanding on customer requirement and thinking
Due to the increased utilization of the internet and social media, customers may now share
their opinions about the goods and services they consume on a variety of platforms (Ibrahim
& Wang, 2019). For clients of hospitality looking for specialized information about different
firms in the market via websites and blogs, a range of data sources have been produced. The
hotel business can better understand customers' demands and shifting preferences by
carefully reviewing these data. Similarly, e-commerce platforms, marketplaces, and product
review websites collect a range of data sources, including vital information on current and
potential clients (Lau, Lee & Ho, 2005).
4.2 Understanding on market more clearly
In addition to the previously mentioned data sources, respectively retail and hospitality
nowadays are able to use various types of data sources to gain a better understanding of the
futures market's path, such as market journals, newsletters, technology blog posts and
newspapers, and industry reviews (Buluswar et al. 2016). Moreover, organizations can get a
greater understanding of the industry's evolving patterns and optimize their business
strategies to attain success by carefully examining this data with current trends and branching
out into other areas (Stieglitz & DangXuan 2013)
5. Text analytics strategies and challenges of using those
strategies
6
analytics
Most businesses, particularly those in the retail and hospitality industries, can benefit from
text analytics to avoid the negative effects of the aforementioned problems. The goal of this
proposed analysis is to collect data from a variety of sources, including structured,
unstructured, and semi-structured data, and then appraise and use it to assist organisations in
making better decisions (Tax et al. 2006). As a result, using text analysis correctly can help
businesses in a variety of ways.
4.1 Better understanding on customer requirement and thinking
Due to the increased utilization of the internet and social media, customers may now share
their opinions about the goods and services they consume on a variety of platforms (Ibrahim
& Wang, 2019). For clients of hospitality looking for specialized information about different
firms in the market via websites and blogs, a range of data sources have been produced. The
hotel business can better understand customers' demands and shifting preferences by
carefully reviewing these data. Similarly, e-commerce platforms, marketplaces, and product
review websites collect a range of data sources, including vital information on current and
potential clients (Lau, Lee & Ho, 2005).
4.2 Understanding on market more clearly
In addition to the previously mentioned data sources, respectively retail and hospitality
nowadays are able to use various types of data sources to gain a better understanding of the
futures market's path, such as market journals, newsletters, technology blog posts and
newspapers, and industry reviews (Buluswar et al. 2016). Moreover, organizations can get a
greater understanding of the industry's evolving patterns and optimize their business
strategies to attain success by carefully examining this data with current trends and branching
out into other areas (Stieglitz & DangXuan 2013)
5. Text analytics strategies and challenges of using those
strategies
6
You're viewing a preview
Unlock full access by subscribing today!
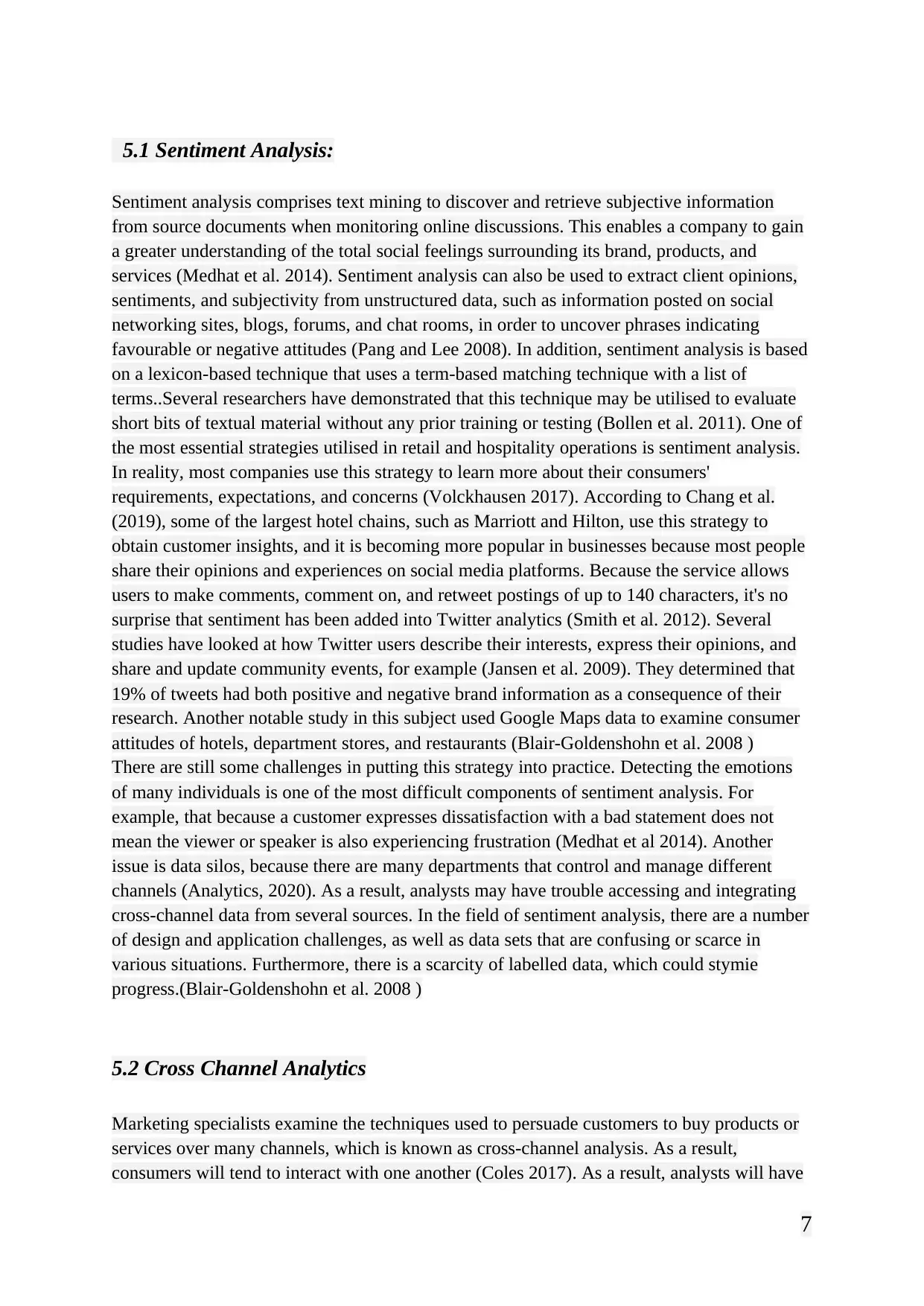
5.1 Sentiment Analysis:
Sentiment analysis comprises text mining to discover and retrieve subjective information
from source documents when monitoring online discussions. This enables a company to gain
a greater understanding of the total social feelings surrounding its brand, products, and
services (Medhat et al. 2014). Sentiment analysis can also be used to extract client opinions,
sentiments, and subjectivity from unstructured data, such as information posted on social
networking sites, blogs, forums, and chat rooms, in order to uncover phrases indicating
favourable or negative attitudes (Pang and Lee 2008). In addition, sentiment analysis is based
on a lexicon-based technique that uses a term-based matching technique with a list of
terms..Several researchers have demonstrated that this technique may be utilised to evaluate
short bits of textual material without any prior training or testing (Bollen et al. 2011). One of
the most essential strategies utilised in retail and hospitality operations is sentiment analysis.
In reality, most companies use this strategy to learn more about their consumers'
requirements, expectations, and concerns (Volckhausen 2017). According to Chang et al.
(2019), some of the largest hotel chains, such as Marriott and Hilton, use this strategy to
obtain customer insights, and it is becoming more popular in businesses because most people
share their opinions and experiences on social media platforms. Because the service allows
users to make comments, comment on, and retweet postings of up to 140 characters, it's no
surprise that sentiment has been added into Twitter analytics (Smith et al. 2012). Several
studies have looked at how Twitter users describe their interests, express their opinions, and
share and update community events, for example (Jansen et al. 2009). They determined that
19% of tweets had both positive and negative brand information as a consequence of their
research. Another notable study in this subject used Google Maps data to examine consumer
attitudes of hotels, department stores, and restaurants (Blair-Goldenshohn et al. 2008 )
There are still some challenges in putting this strategy into practice. Detecting the emotions
of many individuals is one of the most difficult components of sentiment analysis. For
example, that because a customer expresses dissatisfaction with a bad statement does not
mean the viewer or speaker is also experiencing frustration (Medhat et al 2014). Another
issue is data silos, because there are many departments that control and manage different
channels (Analytics, 2020). As a result, analysts may have trouble accessing and integrating
cross-channel data from several sources. In the field of sentiment analysis, there are a number
of design and application challenges, as well as data sets that are confusing or scarce in
various situations. Furthermore, there is a scarcity of labelled data, which could stymie
progress.(Blair-Goldenshohn et al. 2008 )
5.2 Cross Channel Analytics
Marketing specialists examine the techniques used to persuade customers to buy products or
services over many channels, which is known as cross-channel analysis. As a result,
consumers will tend to interact with one another (Coles 2017). As a result, analysts will have
7
Sentiment analysis comprises text mining to discover and retrieve subjective information
from source documents when monitoring online discussions. This enables a company to gain
a greater understanding of the total social feelings surrounding its brand, products, and
services (Medhat et al. 2014). Sentiment analysis can also be used to extract client opinions,
sentiments, and subjectivity from unstructured data, such as information posted on social
networking sites, blogs, forums, and chat rooms, in order to uncover phrases indicating
favourable or negative attitudes (Pang and Lee 2008). In addition, sentiment analysis is based
on a lexicon-based technique that uses a term-based matching technique with a list of
terms..Several researchers have demonstrated that this technique may be utilised to evaluate
short bits of textual material without any prior training or testing (Bollen et al. 2011). One of
the most essential strategies utilised in retail and hospitality operations is sentiment analysis.
In reality, most companies use this strategy to learn more about their consumers'
requirements, expectations, and concerns (Volckhausen 2017). According to Chang et al.
(2019), some of the largest hotel chains, such as Marriott and Hilton, use this strategy to
obtain customer insights, and it is becoming more popular in businesses because most people
share their opinions and experiences on social media platforms. Because the service allows
users to make comments, comment on, and retweet postings of up to 140 characters, it's no
surprise that sentiment has been added into Twitter analytics (Smith et al. 2012). Several
studies have looked at how Twitter users describe their interests, express their opinions, and
share and update community events, for example (Jansen et al. 2009). They determined that
19% of tweets had both positive and negative brand information as a consequence of their
research. Another notable study in this subject used Google Maps data to examine consumer
attitudes of hotels, department stores, and restaurants (Blair-Goldenshohn et al. 2008 )
There are still some challenges in putting this strategy into practice. Detecting the emotions
of many individuals is one of the most difficult components of sentiment analysis. For
example, that because a customer expresses dissatisfaction with a bad statement does not
mean the viewer or speaker is also experiencing frustration (Medhat et al 2014). Another
issue is data silos, because there are many departments that control and manage different
channels (Analytics, 2020). As a result, analysts may have trouble accessing and integrating
cross-channel data from several sources. In the field of sentiment analysis, there are a number
of design and application challenges, as well as data sets that are confusing or scarce in
various situations. Furthermore, there is a scarcity of labelled data, which could stymie
progress.(Blair-Goldenshohn et al. 2008 )
5.2 Cross Channel Analytics
Marketing specialists examine the techniques used to persuade customers to buy products or
services over many channels, which is known as cross-channel analysis. As a result,
consumers will tend to interact with one another (Coles 2017). As a result, analysts will have
7
Paraphrase This Document
Need a fresh take? Get an instant paraphrase of this document with our AI Paraphraser
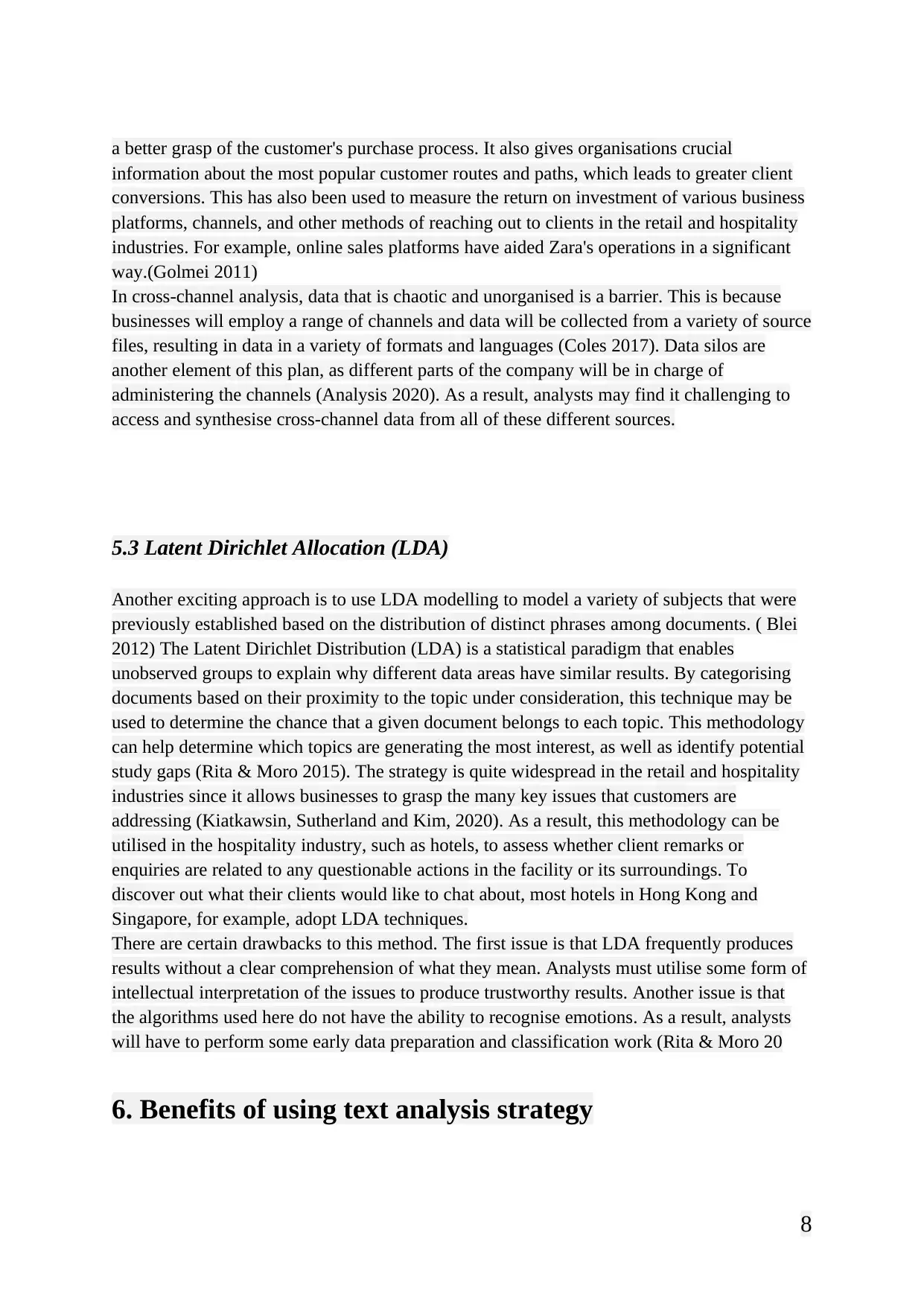
a better grasp of the customer's purchase process. It also gives organisations crucial
information about the most popular customer routes and paths, which leads to greater client
conversions. This has also been used to measure the return on investment of various business
platforms, channels, and other methods of reaching out to clients in the retail and hospitality
industries. For example, online sales platforms have aided Zara's operations in a significant
way.(Golmei 2011)
In cross-channel analysis, data that is chaotic and unorganised is a barrier. This is because
businesses will employ a range of channels and data will be collected from a variety of source
files, resulting in data in a variety of formats and languages (Coles 2017). Data silos are
another element of this plan, as different parts of the company will be in charge of
administering the channels (Analysis 2020). As a result, analysts may find it challenging to
access and synthesise cross-channel data from all of these different sources.
5.3 Latent Dirichlet Allocation (LDA)
Another exciting approach is to use LDA modelling to model a variety of subjects that were
previously established based on the distribution of distinct phrases among documents. ( Blei
2012) The Latent Dirichlet Distribution (LDA) is a statistical paradigm that enables
unobserved groups to explain why different data areas have similar results. By categorising
documents based on their proximity to the topic under consideration, this technique may be
used to determine the chance that a given document belongs to each topic. This methodology
can help determine which topics are generating the most interest, as well as identify potential
study gaps (Rita & Moro 2015). The strategy is quite widespread in the retail and hospitality
industries since it allows businesses to grasp the many key issues that customers are
addressing (Kiatkawsin, Sutherland and Kim, 2020). As a result, this methodology can be
utilised in the hospitality industry, such as hotels, to assess whether client remarks or
enquiries are related to any questionable actions in the facility or its surroundings. To
discover out what their clients would like to chat about, most hotels in Hong Kong and
Singapore, for example, adopt LDA techniques.
There are certain drawbacks to this method. The first issue is that LDA frequently produces
results without a clear comprehension of what they mean. Analysts must utilise some form of
intellectual interpretation of the issues to produce trustworthy results. Another issue is that
the algorithms used here do not have the ability to recognise emotions. As a result, analysts
will have to perform some early data preparation and classification work (Rita & Moro 20
6. Benefits of using text analysis strategy
8
information about the most popular customer routes and paths, which leads to greater client
conversions. This has also been used to measure the return on investment of various business
platforms, channels, and other methods of reaching out to clients in the retail and hospitality
industries. For example, online sales platforms have aided Zara's operations in a significant
way.(Golmei 2011)
In cross-channel analysis, data that is chaotic and unorganised is a barrier. This is because
businesses will employ a range of channels and data will be collected from a variety of source
files, resulting in data in a variety of formats and languages (Coles 2017). Data silos are
another element of this plan, as different parts of the company will be in charge of
administering the channels (Analysis 2020). As a result, analysts may find it challenging to
access and synthesise cross-channel data from all of these different sources.
5.3 Latent Dirichlet Allocation (LDA)
Another exciting approach is to use LDA modelling to model a variety of subjects that were
previously established based on the distribution of distinct phrases among documents. ( Blei
2012) The Latent Dirichlet Distribution (LDA) is a statistical paradigm that enables
unobserved groups to explain why different data areas have similar results. By categorising
documents based on their proximity to the topic under consideration, this technique may be
used to determine the chance that a given document belongs to each topic. This methodology
can help determine which topics are generating the most interest, as well as identify potential
study gaps (Rita & Moro 2015). The strategy is quite widespread in the retail and hospitality
industries since it allows businesses to grasp the many key issues that customers are
addressing (Kiatkawsin, Sutherland and Kim, 2020). As a result, this methodology can be
utilised in the hospitality industry, such as hotels, to assess whether client remarks or
enquiries are related to any questionable actions in the facility or its surroundings. To
discover out what their clients would like to chat about, most hotels in Hong Kong and
Singapore, for example, adopt LDA techniques.
There are certain drawbacks to this method. The first issue is that LDA frequently produces
results without a clear comprehension of what they mean. Analysts must utilise some form of
intellectual interpretation of the issues to produce trustworthy results. Another issue is that
the algorithms used here do not have the ability to recognise emotions. As a result, analysts
will have to perform some early data preparation and classification work (Rita & Moro 20
6. Benefits of using text analysis strategy
8
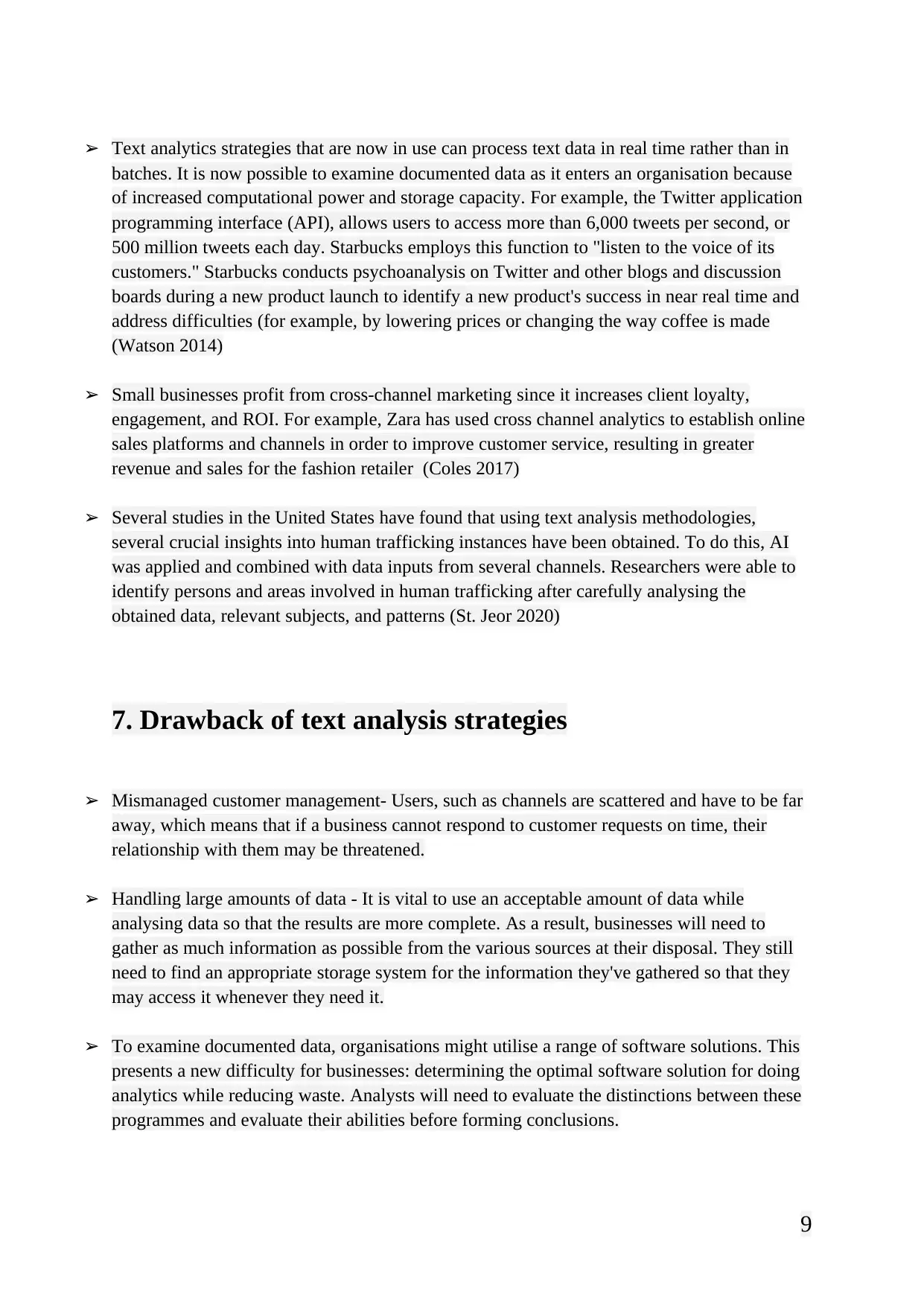
➢ Text analytics strategies that are now in use can process text data in real time rather than in
batches. It is now possible to examine documented data as it enters an organisation because
of increased computational power and storage capacity. For example, the Twitter application
programming interface (API), allows users to access more than 6,000 tweets per second, or
500 million tweets each day. Starbucks employs this function to "listen to the voice of its
customers." Starbucks conducts psychoanalysis on Twitter and other blogs and discussion
boards during a new product launch to identify a new product's success in near real time and
address difficulties (for example, by lowering prices or changing the way coffee is made
(Watson 2014)
➢ Small businesses profit from cross-channel marketing since it increases client loyalty,
engagement, and ROI. For example, Zara has used cross channel analytics to establish online
sales platforms and channels in order to improve customer service, resulting in greater
revenue and sales for the fashion retailer (Coles 2017)
➢ Several studies in the United States have found that using text analysis methodologies,
several crucial insights into human trafficking instances have been obtained. To do this, AI
was applied and combined with data inputs from several channels. Researchers were able to
identify persons and areas involved in human trafficking after carefully analysing the
obtained data, relevant subjects, and patterns (St. Jeor 2020)
7. Drawback of text analysis strategies
➢ Mismanaged customer management- Users, such as channels are scattered and have to be far
away, which means that if a business cannot respond to customer requests on time, their
relationship with them may be threatened.
➢ Handling large amounts of data - It is vital to use an acceptable amount of data while
analysing data so that the results are more complete. As a result, businesses will need to
gather as much information as possible from the various sources at their disposal. They still
need to find an appropriate storage system for the information they've gathered so that they
may access it whenever they need it.
➢ To examine documented data, organisations might utilise a range of software solutions. This
presents a new difficulty for businesses: determining the optimal software solution for doing
analytics while reducing waste. Analysts will need to evaluate the distinctions between these
programmes and evaluate their abilities before forming conclusions.
9
batches. It is now possible to examine documented data as it enters an organisation because
of increased computational power and storage capacity. For example, the Twitter application
programming interface (API), allows users to access more than 6,000 tweets per second, or
500 million tweets each day. Starbucks employs this function to "listen to the voice of its
customers." Starbucks conducts psychoanalysis on Twitter and other blogs and discussion
boards during a new product launch to identify a new product's success in near real time and
address difficulties (for example, by lowering prices or changing the way coffee is made
(Watson 2014)
➢ Small businesses profit from cross-channel marketing since it increases client loyalty,
engagement, and ROI. For example, Zara has used cross channel analytics to establish online
sales platforms and channels in order to improve customer service, resulting in greater
revenue and sales for the fashion retailer (Coles 2017)
➢ Several studies in the United States have found that using text analysis methodologies,
several crucial insights into human trafficking instances have been obtained. To do this, AI
was applied and combined with data inputs from several channels. Researchers were able to
identify persons and areas involved in human trafficking after carefully analysing the
obtained data, relevant subjects, and patterns (St. Jeor 2020)
7. Drawback of text analysis strategies
➢ Mismanaged customer management- Users, such as channels are scattered and have to be far
away, which means that if a business cannot respond to customer requests on time, their
relationship with them may be threatened.
➢ Handling large amounts of data - It is vital to use an acceptable amount of data while
analysing data so that the results are more complete. As a result, businesses will need to
gather as much information as possible from the various sources at their disposal. They still
need to find an appropriate storage system for the information they've gathered so that they
may access it whenever they need it.
➢ To examine documented data, organisations might utilise a range of software solutions. This
presents a new difficulty for businesses: determining the optimal software solution for doing
analytics while reducing waste. Analysts will need to evaluate the distinctions between these
programmes and evaluate their abilities before forming conclusions.
9
You're viewing a preview
Unlock full access by subscribing today!
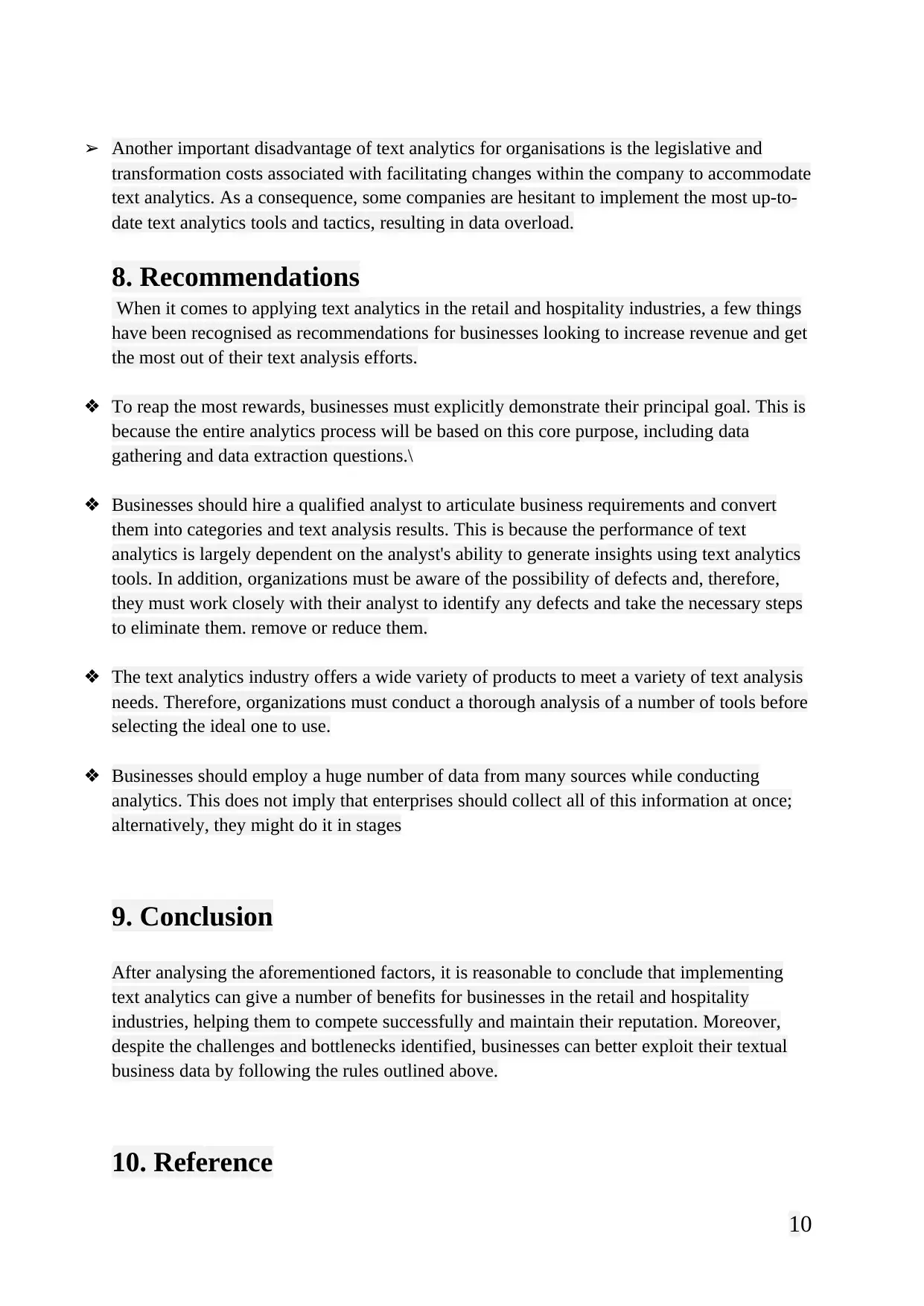
➢ Another important disadvantage of text analytics for organisations is the legislative and
transformation costs associated with facilitating changes within the company to accommodate
text analytics. As a consequence, some companies are hesitant to implement the most up-to-
date text analytics tools and tactics, resulting in data overload.
8. Recommendations
When it comes to applying text analytics in the retail and hospitality industries, a few things
have been recognised as recommendations for businesses looking to increase revenue and get
the most out of their text analysis efforts.
❖ To reap the most rewards, businesses must explicitly demonstrate their principal goal. This is
because the entire analytics process will be based on this core purpose, including data
gathering and data extraction questions.\
❖ Businesses should hire a qualified analyst to articulate business requirements and convert
them into categories and text analysis results. This is because the performance of text
analytics is largely dependent on the analyst's ability to generate insights using text analytics
tools. In addition, organizations must be aware of the possibility of defects and, therefore,
they must work closely with their analyst to identify any defects and take the necessary steps
to eliminate them. remove or reduce them.
❖ The text analytics industry offers a wide variety of products to meet a variety of text analysis
needs. Therefore, organizations must conduct a thorough analysis of a number of tools before
selecting the ideal one to use.
❖ Businesses should employ a huge number of data from many sources while conducting
analytics. This does not imply that enterprises should collect all of this information at once;
alternatively, they might do it in stages
9. Conclusion
After analysing the aforementioned factors, it is reasonable to conclude that implementing
text analytics can give a number of benefits for businesses in the retail and hospitality
industries, helping them to compete successfully and maintain their reputation. Moreover,
despite the challenges and bottlenecks identified, businesses can better exploit their textual
business data by following the rules outlined above.
10. Reference
10
transformation costs associated with facilitating changes within the company to accommodate
text analytics. As a consequence, some companies are hesitant to implement the most up-to-
date text analytics tools and tactics, resulting in data overload.
8. Recommendations
When it comes to applying text analytics in the retail and hospitality industries, a few things
have been recognised as recommendations for businesses looking to increase revenue and get
the most out of their text analysis efforts.
❖ To reap the most rewards, businesses must explicitly demonstrate their principal goal. This is
because the entire analytics process will be based on this core purpose, including data
gathering and data extraction questions.\
❖ Businesses should hire a qualified analyst to articulate business requirements and convert
them into categories and text analysis results. This is because the performance of text
analytics is largely dependent on the analyst's ability to generate insights using text analytics
tools. In addition, organizations must be aware of the possibility of defects and, therefore,
they must work closely with their analyst to identify any defects and take the necessary steps
to eliminate them. remove or reduce them.
❖ The text analytics industry offers a wide variety of products to meet a variety of text analysis
needs. Therefore, organizations must conduct a thorough analysis of a number of tools before
selecting the ideal one to use.
❖ Businesses should employ a huge number of data from many sources while conducting
analytics. This does not imply that enterprises should collect all of this information at once;
alternatively, they might do it in stages
9. Conclusion
After analysing the aforementioned factors, it is reasonable to conclude that implementing
text analytics can give a number of benefits for businesses in the retail and hospitality
industries, helping them to compete successfully and maintain their reputation. Moreover,
despite the challenges and bottlenecks identified, businesses can better exploit their textual
business data by following the rules outlined above.
10. Reference
10
Paraphrase This Document
Need a fresh take? Get an instant paraphrase of this document with our AI Paraphraser
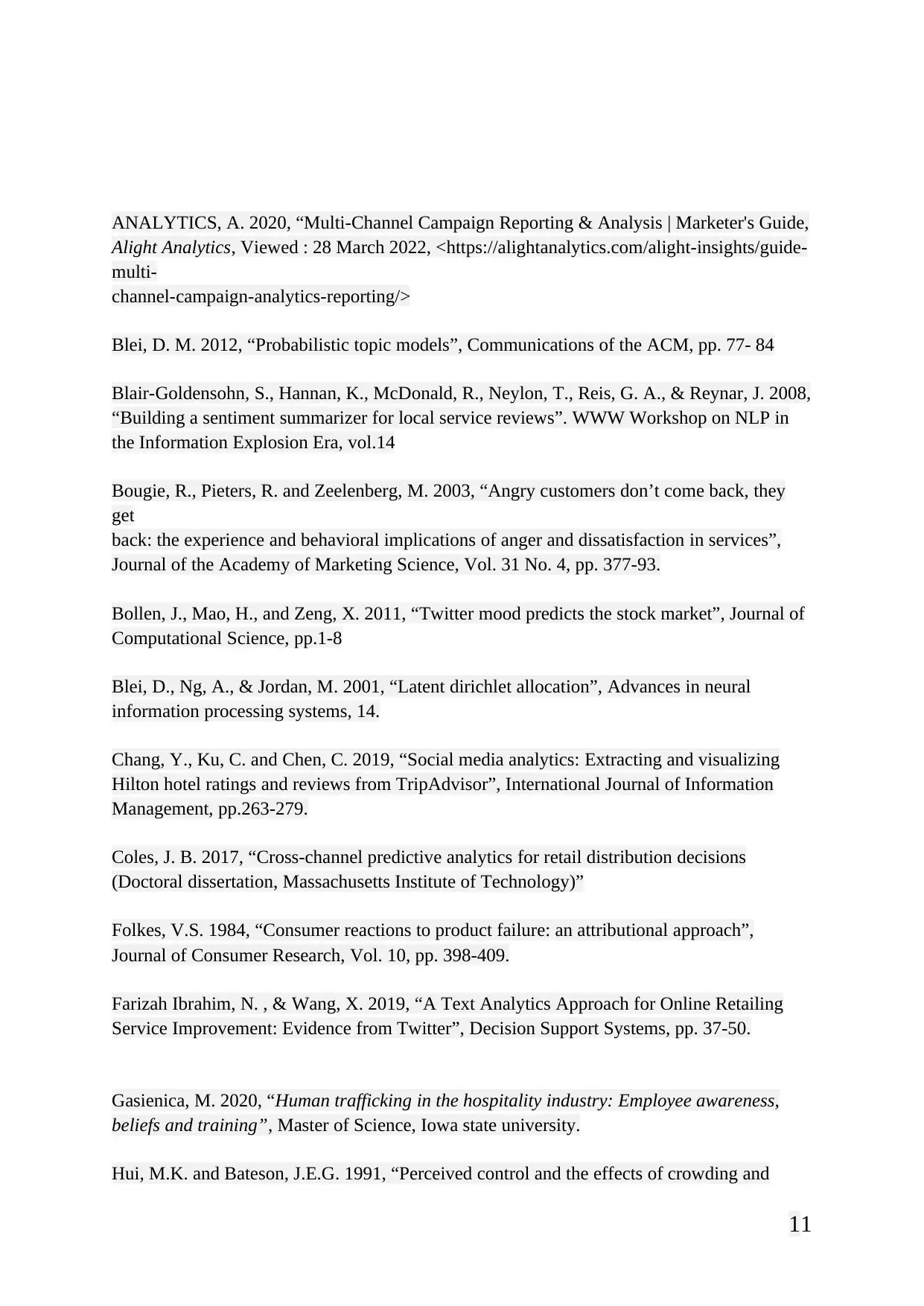
ANALYTICS, A. 2020, “Multi-Channel Campaign Reporting & Analysis | Marketer's Guide,
Alight Analytics, Viewed : 28 March 2022, <https://alightanalytics.com/alight-insights/guide-
multi-
channel-campaign-analytics-reporting/>
Blei, D. M. 2012, “Probabilistic topic models”, Communications of the ACM, pp. 77- 84
Blair-Goldensohn, S., Hannan, K., McDonald, R., Neylon, T., Reis, G. A., & Reynar, J. 2008,
“Building a sentiment summarizer for local service reviews”. WWW Workshop on NLP in
the Information Explosion Era, vol.14
Bougie, R., Pieters, R. and Zeelenberg, M. 2003, “Angry customers don’t come back, they
get
back: the experience and behavioral implications of anger and dissatisfaction in services”,
Journal of the Academy of Marketing Science, Vol. 31 No. 4, pp. 377-93.
Bollen, J., Mao, H., and Zeng, X. 2011, “Twitter mood predicts the stock market”, Journal of
Computational Science, pp.1-8
Blei, D., Ng, A., & Jordan, M. 2001, “Latent dirichlet allocation”, Advances in neural
information processing systems, 14.
Chang, Y., Ku, C. and Chen, C. 2019, “Social media analytics: Extracting and visualizing
Hilton hotel ratings and reviews from TripAdvisor”, International Journal of Information
Management, pp.263-279.
Coles, J. B. 2017, “Cross-channel predictive analytics for retail distribution decisions
(Doctoral dissertation, Massachusetts Institute of Technology)”
Folkes, V.S. 1984, “Consumer reactions to product failure: an attributional approach”,
Journal of Consumer Research, Vol. 10, pp. 398-409.
Farizah Ibrahim, N. , & Wang, X. 2019, “A Text Analytics Approach for Online Retailing
Service Improvement: Evidence from Twitter”, Decision Support Systems, pp. 37-50.
Gasienica, M. 2020, “Human trafficking in the hospitality industry: Employee awareness,
beliefs and training”, Master of Science, Iowa state university.
Hui, M.K. and Bateson, J.E.G. 1991, “Perceived control and the effects of crowding and
11
Alight Analytics, Viewed : 28 March 2022, <https://alightanalytics.com/alight-insights/guide-
multi-
channel-campaign-analytics-reporting/>
Blei, D. M. 2012, “Probabilistic topic models”, Communications of the ACM, pp. 77- 84
Blair-Goldensohn, S., Hannan, K., McDonald, R., Neylon, T., Reis, G. A., & Reynar, J. 2008,
“Building a sentiment summarizer for local service reviews”. WWW Workshop on NLP in
the Information Explosion Era, vol.14
Bougie, R., Pieters, R. and Zeelenberg, M. 2003, “Angry customers don’t come back, they
get
back: the experience and behavioral implications of anger and dissatisfaction in services”,
Journal of the Academy of Marketing Science, Vol. 31 No. 4, pp. 377-93.
Bollen, J., Mao, H., and Zeng, X. 2011, “Twitter mood predicts the stock market”, Journal of
Computational Science, pp.1-8
Blei, D., Ng, A., & Jordan, M. 2001, “Latent dirichlet allocation”, Advances in neural
information processing systems, 14.
Chang, Y., Ku, C. and Chen, C. 2019, “Social media analytics: Extracting and visualizing
Hilton hotel ratings and reviews from TripAdvisor”, International Journal of Information
Management, pp.263-279.
Coles, J. B. 2017, “Cross-channel predictive analytics for retail distribution decisions
(Doctoral dissertation, Massachusetts Institute of Technology)”
Folkes, V.S. 1984, “Consumer reactions to product failure: an attributional approach”,
Journal of Consumer Research, Vol. 10, pp. 398-409.
Farizah Ibrahim, N. , & Wang, X. 2019, “A Text Analytics Approach for Online Retailing
Service Improvement: Evidence from Twitter”, Decision Support Systems, pp. 37-50.
Gasienica, M. 2020, “Human trafficking in the hospitality industry: Employee awareness,
beliefs and training”, Master of Science, Iowa state university.
Hui, M.K. and Bateson, J.E.G. 1991, “Perceived control and the effects of crowding and
11
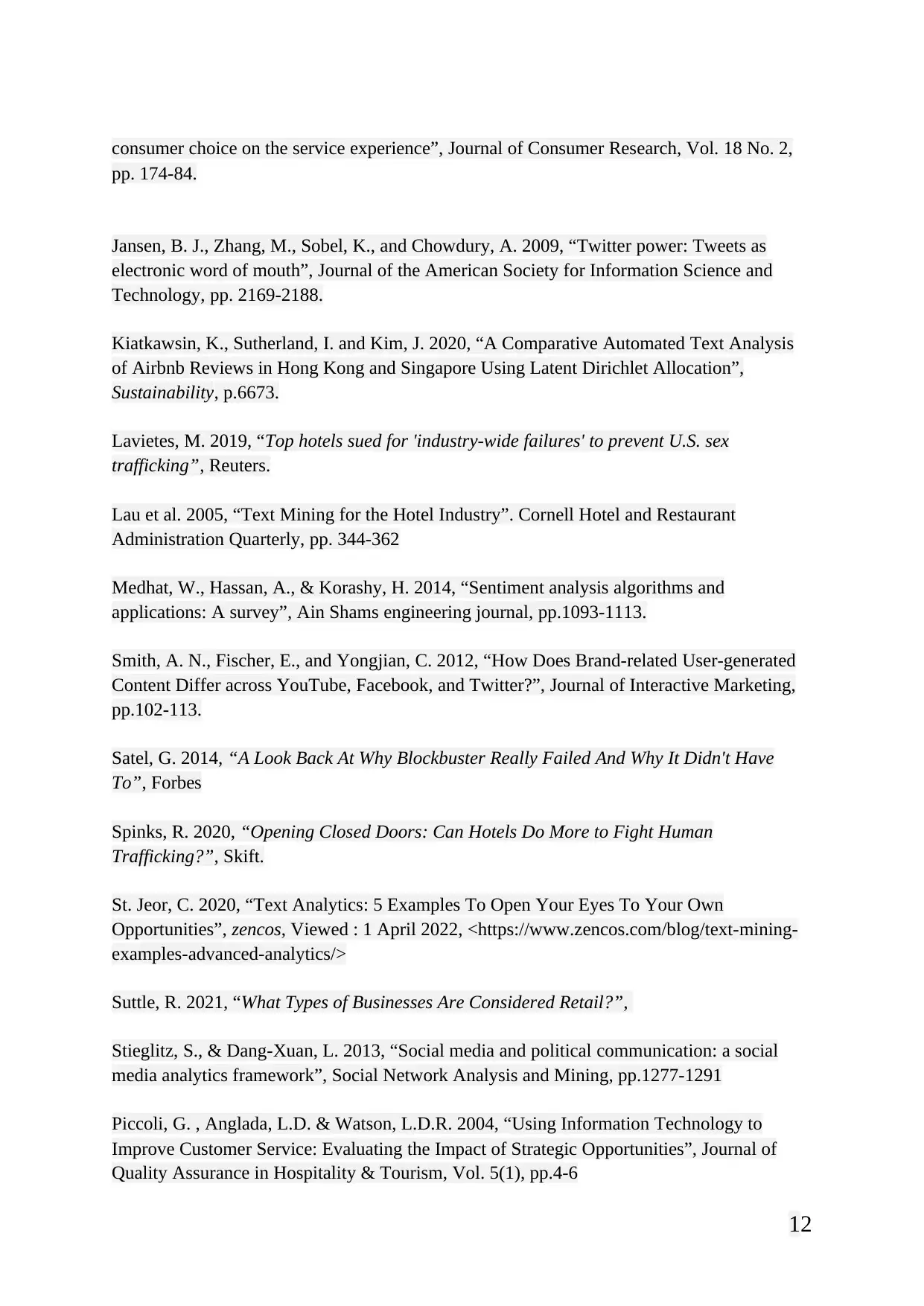
consumer choice on the service experience”, Journal of Consumer Research, Vol. 18 No. 2,
pp. 174-84.
Jansen, B. J., Zhang, M., Sobel, K., and Chowdury, A. 2009, “Twitter power: Tweets as
electronic word of mouth”, Journal of the American Society for Information Science and
Technology, pp. 2169-2188.
Kiatkawsin, K., Sutherland, I. and Kim, J. 2020, “A Comparative Automated Text Analysis
of Airbnb Reviews in Hong Kong and Singapore Using Latent Dirichlet Allocation”,
Sustainability, p.6673.
Lavietes, M. 2019, “Top hotels sued for 'industry-wide failures' to prevent U.S. sex
trafficking”, Reuters.
Lau et al. 2005, “Text Mining for the Hotel Industry”. Cornell Hotel and Restaurant
Administration Quarterly, pp. 344-362
Medhat, W., Hassan, A., & Korashy, H. 2014, “Sentiment analysis algorithms and
applications: A survey”, Ain Shams engineering journal, pp.1093-1113.
Smith, A. N., Fischer, E., and Yongjian, C. 2012, “How Does Brand-related User-generated
Content Differ across YouTube, Facebook, and Twitter?”, Journal of Interactive Marketing,
pp.102-113.
Satel, G. 2014, “A Look Back At Why Blockbuster Really Failed And Why It Didn't Have
To”, Forbes
Spinks, R. 2020, “Opening Closed Doors: Can Hotels Do More to Fight Human
Trafficking?”, Skift.
St. Jeor, C. 2020, “Text Analytics: 5 Examples To Open Your Eyes To Your Own
Opportunities”, zencos, Viewed : 1 April 2022, <https://www.zencos.com/blog/text-mining-
examples-advanced-analytics/>
Suttle, R. 2021, “What Types of Businesses Are Considered Retail?”,
Stieglitz, S., & Dang-Xuan, L. 2013, “Social media and political communication: a social
media analytics framework”, Social Network Analysis and Mining, pp.1277-1291
Piccoli, G. , Anglada, L.D. & Watson, L.D.R. 2004, “Using Information Technology to
Improve Customer Service: Evaluating the Impact of Strategic Opportunities”, Journal of
Quality Assurance in Hospitality & Tourism, Vol. 5(1), pp.4-6
12
pp. 174-84.
Jansen, B. J., Zhang, M., Sobel, K., and Chowdury, A. 2009, “Twitter power: Tweets as
electronic word of mouth”, Journal of the American Society for Information Science and
Technology, pp. 2169-2188.
Kiatkawsin, K., Sutherland, I. and Kim, J. 2020, “A Comparative Automated Text Analysis
of Airbnb Reviews in Hong Kong and Singapore Using Latent Dirichlet Allocation”,
Sustainability, p.6673.
Lavietes, M. 2019, “Top hotels sued for 'industry-wide failures' to prevent U.S. sex
trafficking”, Reuters.
Lau et al. 2005, “Text Mining for the Hotel Industry”. Cornell Hotel and Restaurant
Administration Quarterly, pp. 344-362
Medhat, W., Hassan, A., & Korashy, H. 2014, “Sentiment analysis algorithms and
applications: A survey”, Ain Shams engineering journal, pp.1093-1113.
Smith, A. N., Fischer, E., and Yongjian, C. 2012, “How Does Brand-related User-generated
Content Differ across YouTube, Facebook, and Twitter?”, Journal of Interactive Marketing,
pp.102-113.
Satel, G. 2014, “A Look Back At Why Blockbuster Really Failed And Why It Didn't Have
To”, Forbes
Spinks, R. 2020, “Opening Closed Doors: Can Hotels Do More to Fight Human
Trafficking?”, Skift.
St. Jeor, C. 2020, “Text Analytics: 5 Examples To Open Your Eyes To Your Own
Opportunities”, zencos, Viewed : 1 April 2022, <https://www.zencos.com/blog/text-mining-
examples-advanced-analytics/>
Suttle, R. 2021, “What Types of Businesses Are Considered Retail?”,
Stieglitz, S., & Dang-Xuan, L. 2013, “Social media and political communication: a social
media analytics framework”, Social Network Analysis and Mining, pp.1277-1291
Piccoli, G. , Anglada, L.D. & Watson, L.D.R. 2004, “Using Information Technology to
Improve Customer Service: Evaluating the Impact of Strategic Opportunities”, Journal of
Quality Assurance in Hospitality & Tourism, Vol. 5(1), pp.4-6
12
You're viewing a preview
Unlock full access by subscribing today!
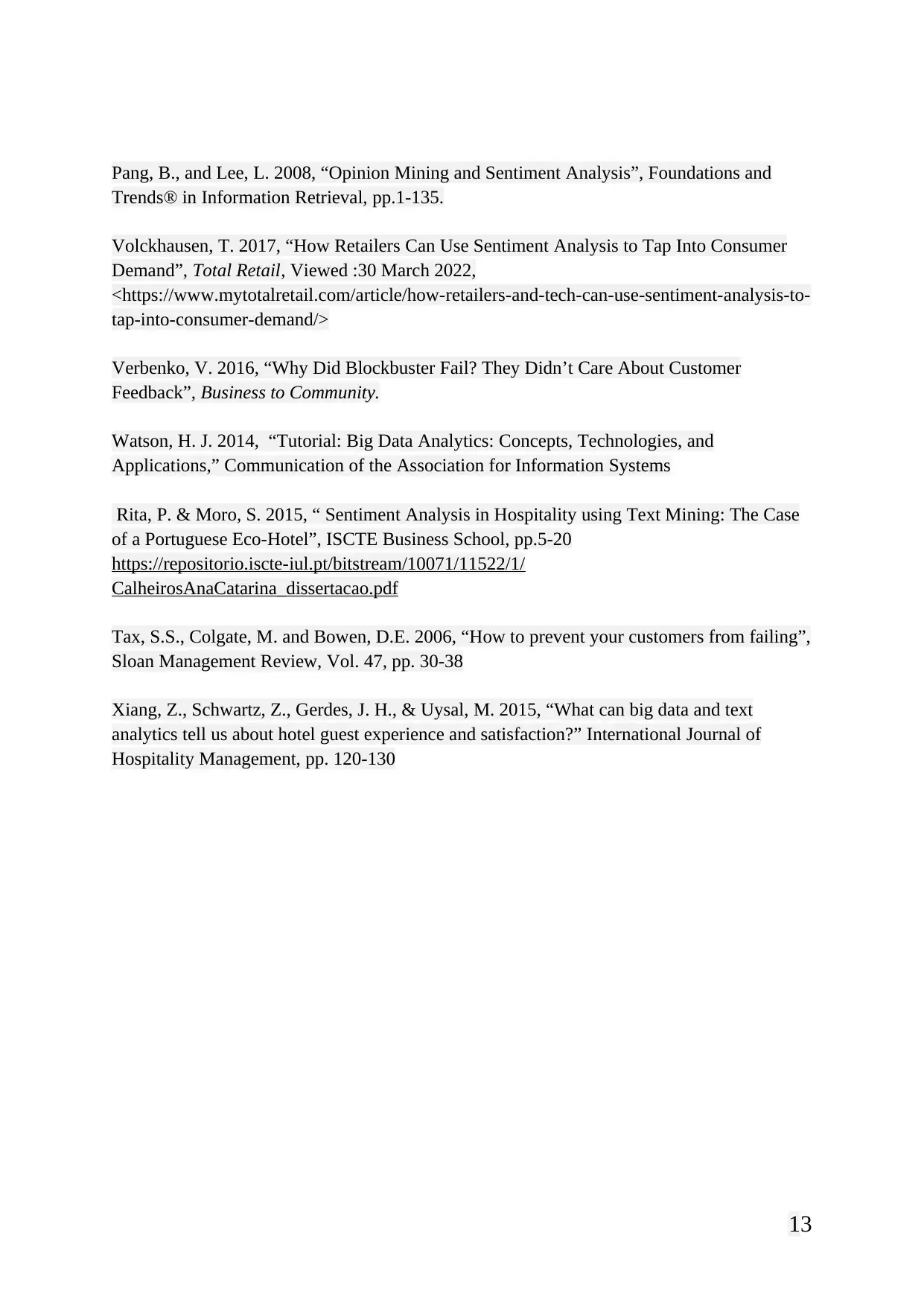
Pang, B., and Lee, L. 2008, “Opinion Mining and Sentiment Analysis”, Foundations and
Trends® in Information Retrieval, pp.1-135.
Volckhausen, T. 2017, “How Retailers Can Use Sentiment Analysis to Tap Into Consumer
Demand”, Total Retail, Viewed :30 March 2022,
<https://www.mytotalretail.com/article/how-retailers-and-tech-can-use-sentiment-analysis-to-
tap-into-consumer-demand/>
Verbenko, V. 2016, “Why Did Blockbuster Fail? They Didn’t Care About Customer
Feedback”, Business to Community.
Watson, H. J. 2014, “Tutorial: Big Data Analytics: Concepts, Technologies, and
Applications,” Communication of the Association for Information Systems
Rita, P. & Moro, S. 2015, “ Sentiment Analysis in Hospitality using Text Mining: The Case
of a Portuguese Eco-Hotel”, ISCTE Business School, pp.5-20
https://repositorio.iscte-iul.pt/bitstream/10071/11522/1/
CalheirosAnaCatarina_dissertacao.pdf
Tax, S.S., Colgate, M. and Bowen, D.E. 2006, “How to prevent your customers from failing”,
Sloan Management Review, Vol. 47, pp. 30-38
Xiang, Z., Schwartz, Z., Gerdes, J. H., & Uysal, M. 2015, “What can big data and text
analytics tell us about hotel guest experience and satisfaction?” International Journal of
Hospitality Management, pp. 120-130
13
Trends® in Information Retrieval, pp.1-135.
Volckhausen, T. 2017, “How Retailers Can Use Sentiment Analysis to Tap Into Consumer
Demand”, Total Retail, Viewed :30 March 2022,
<https://www.mytotalretail.com/article/how-retailers-and-tech-can-use-sentiment-analysis-to-
tap-into-consumer-demand/>
Verbenko, V. 2016, “Why Did Blockbuster Fail? They Didn’t Care About Customer
Feedback”, Business to Community.
Watson, H. J. 2014, “Tutorial: Big Data Analytics: Concepts, Technologies, and
Applications,” Communication of the Association for Information Systems
Rita, P. & Moro, S. 2015, “ Sentiment Analysis in Hospitality using Text Mining: The Case
of a Portuguese Eco-Hotel”, ISCTE Business School, pp.5-20
https://repositorio.iscte-iul.pt/bitstream/10071/11522/1/
CalheirosAnaCatarina_dissertacao.pdf
Tax, S.S., Colgate, M. and Bowen, D.E. 2006, “How to prevent your customers from failing”,
Sloan Management Review, Vol. 47, pp. 30-38
Xiang, Z., Schwartz, Z., Gerdes, J. H., & Uysal, M. 2015, “What can big data and text
analytics tell us about hotel guest experience and satisfaction?” International Journal of
Hospitality Management, pp. 120-130
13
1 out of 13
Related Documents

Your All-in-One AI-Powered Toolkit for Academic Success.
+13062052269
info@desklib.com
Available 24*7 on WhatsApp / Email
Unlock your academic potential
© 2024 | Zucol Services PVT LTD | All rights reserved.