Reviewing Project Cash Flow Models in Australian Construction Industry
VerifiedAdded on 2023/06/03
|10
|2731
|331
Literature Review
AI Summary
This literature review examines various models for project cash flow analysis within the context of Australian construction projects. It highlights the significance of effective cash flow management for construction companies, referencing studies that link poor financial management to company failures. The review covers several models, including the MWM model, Bayesian Belief Networks (BBNs), the logit project cash flow model, Least Squares Support Vector Machine (LS-SVMAT), Cash Flow Forecasting System (CAFFS), and an Algorithm model, discussing their methodologies, advantages, and limitations. The review emphasizes the importance of factors such as time lag, risk management, and accurate cost estimation in cash flow forecasting, concluding with a focus on the Algorithm of Cash Flow Forecasting Model for its consideration of time lag in strategic decision-making during the planning phase.
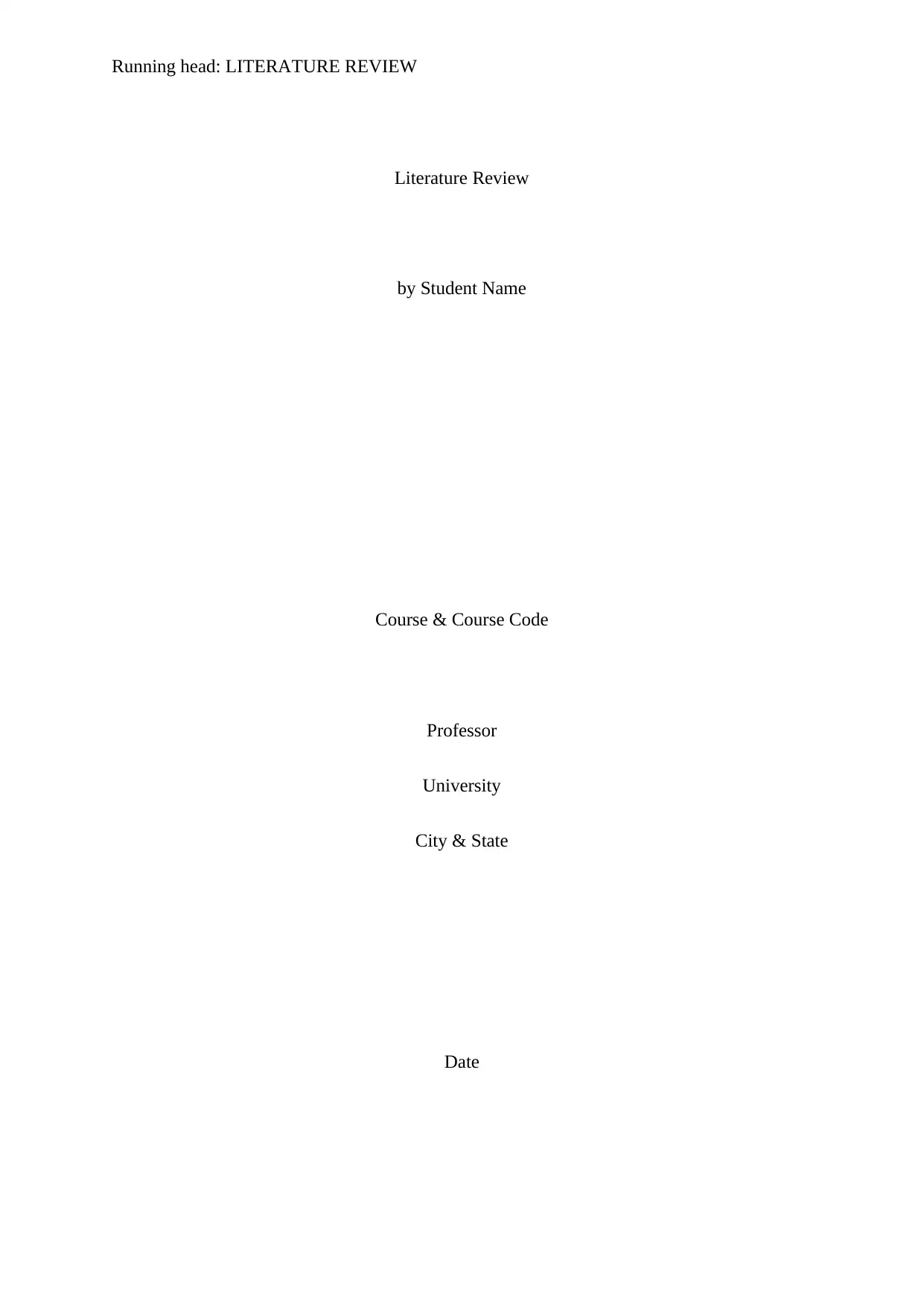
Running head: LITERATURE REVIEW
Literature Review
by Student Name
Course & Course Code
Professor
University
City & State
Date
Literature Review
by Student Name
Course & Course Code
Professor
University
City & State
Date
Paraphrase This Document
Need a fresh take? Get an instant paraphrase of this document with our AI Paraphraser
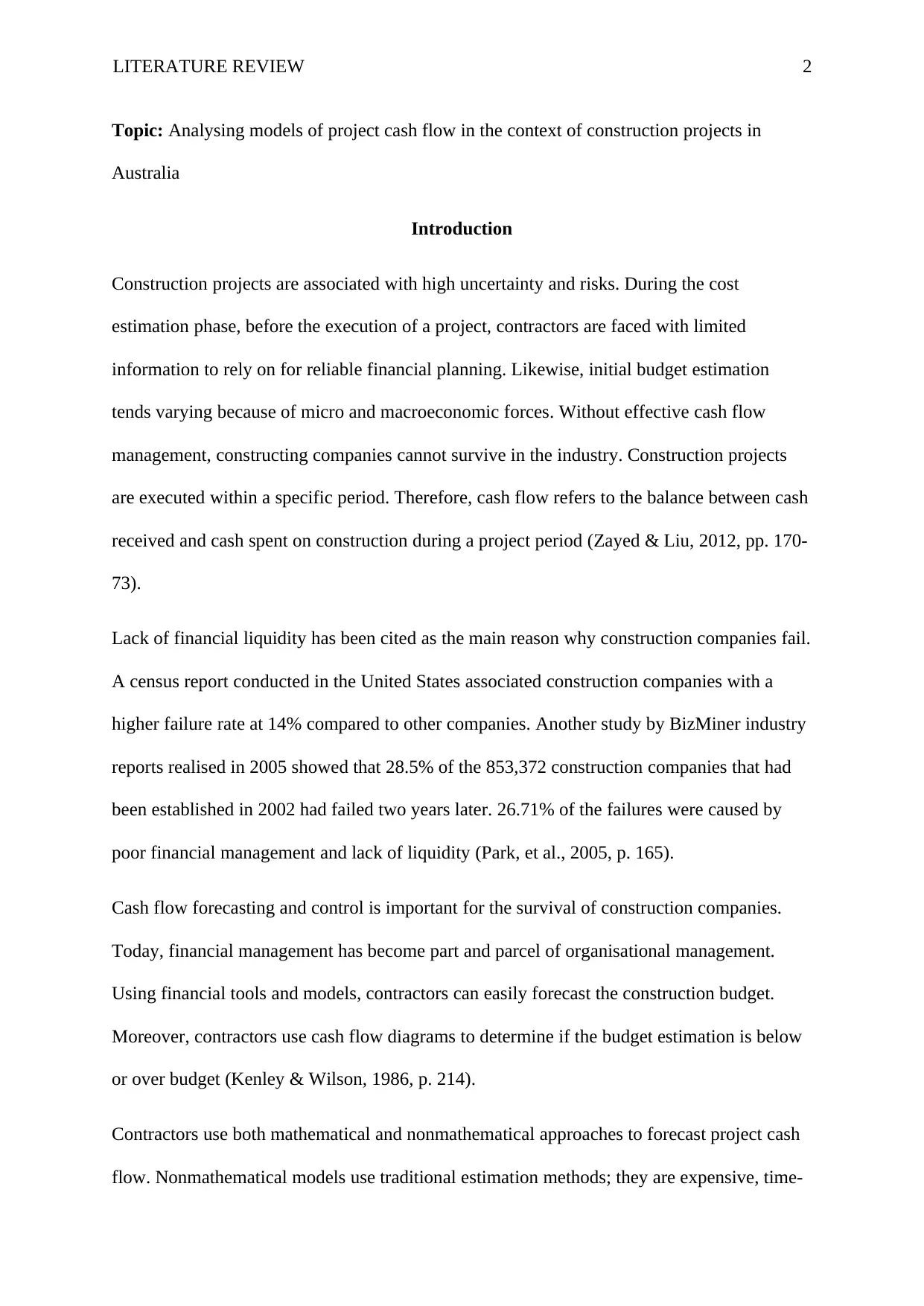
LITERATURE REVIEW 2
Topic: Analysing models of project cash flow in the context of construction projects in
Australia
Introduction
Construction projects are associated with high uncertainty and risks. During the cost
estimation phase, before the execution of a project, contractors are faced with limited
information to rely on for reliable financial planning. Likewise, initial budget estimation
tends varying because of micro and macroeconomic forces. Without effective cash flow
management, constructing companies cannot survive in the industry. Construction projects
are executed within a specific period. Therefore, cash flow refers to the balance between cash
received and cash spent on construction during a project period (Zayed & Liu, 2012, pp. 170-
73).
Lack of financial liquidity has been cited as the main reason why construction companies fail.
A census report conducted in the United States associated construction companies with a
higher failure rate at 14% compared to other companies. Another study by BizMiner industry
reports realised in 2005 showed that 28.5% of the 853,372 construction companies that had
been established in 2002 had failed two years later. 26.71% of the failures were caused by
poor financial management and lack of liquidity (Park, et al., 2005, p. 165).
Cash flow forecasting and control is important for the survival of construction companies.
Today, financial management has become part and parcel of organisational management.
Using financial tools and models, contractors can easily forecast the construction budget.
Moreover, contractors use cash flow diagrams to determine if the budget estimation is below
or over budget (Kenley & Wilson, 1986, p. 214).
Contractors use both mathematical and nonmathematical approaches to forecast project cash
flow. Nonmathematical models use traditional estimation methods; they are expensive, time-
Topic: Analysing models of project cash flow in the context of construction projects in
Australia
Introduction
Construction projects are associated with high uncertainty and risks. During the cost
estimation phase, before the execution of a project, contractors are faced with limited
information to rely on for reliable financial planning. Likewise, initial budget estimation
tends varying because of micro and macroeconomic forces. Without effective cash flow
management, constructing companies cannot survive in the industry. Construction projects
are executed within a specific period. Therefore, cash flow refers to the balance between cash
received and cash spent on construction during a project period (Zayed & Liu, 2012, pp. 170-
73).
Lack of financial liquidity has been cited as the main reason why construction companies fail.
A census report conducted in the United States associated construction companies with a
higher failure rate at 14% compared to other companies. Another study by BizMiner industry
reports realised in 2005 showed that 28.5% of the 853,372 construction companies that had
been established in 2002 had failed two years later. 26.71% of the failures were caused by
poor financial management and lack of liquidity (Park, et al., 2005, p. 165).
Cash flow forecasting and control is important for the survival of construction companies.
Today, financial management has become part and parcel of organisational management.
Using financial tools and models, contractors can easily forecast the construction budget.
Moreover, contractors use cash flow diagrams to determine if the budget estimation is below
or over budget (Kenley & Wilson, 1986, p. 214).
Contractors use both mathematical and nonmathematical approaches to forecast project cash
flow. Nonmathematical models use traditional estimation methods; they are expensive, time-
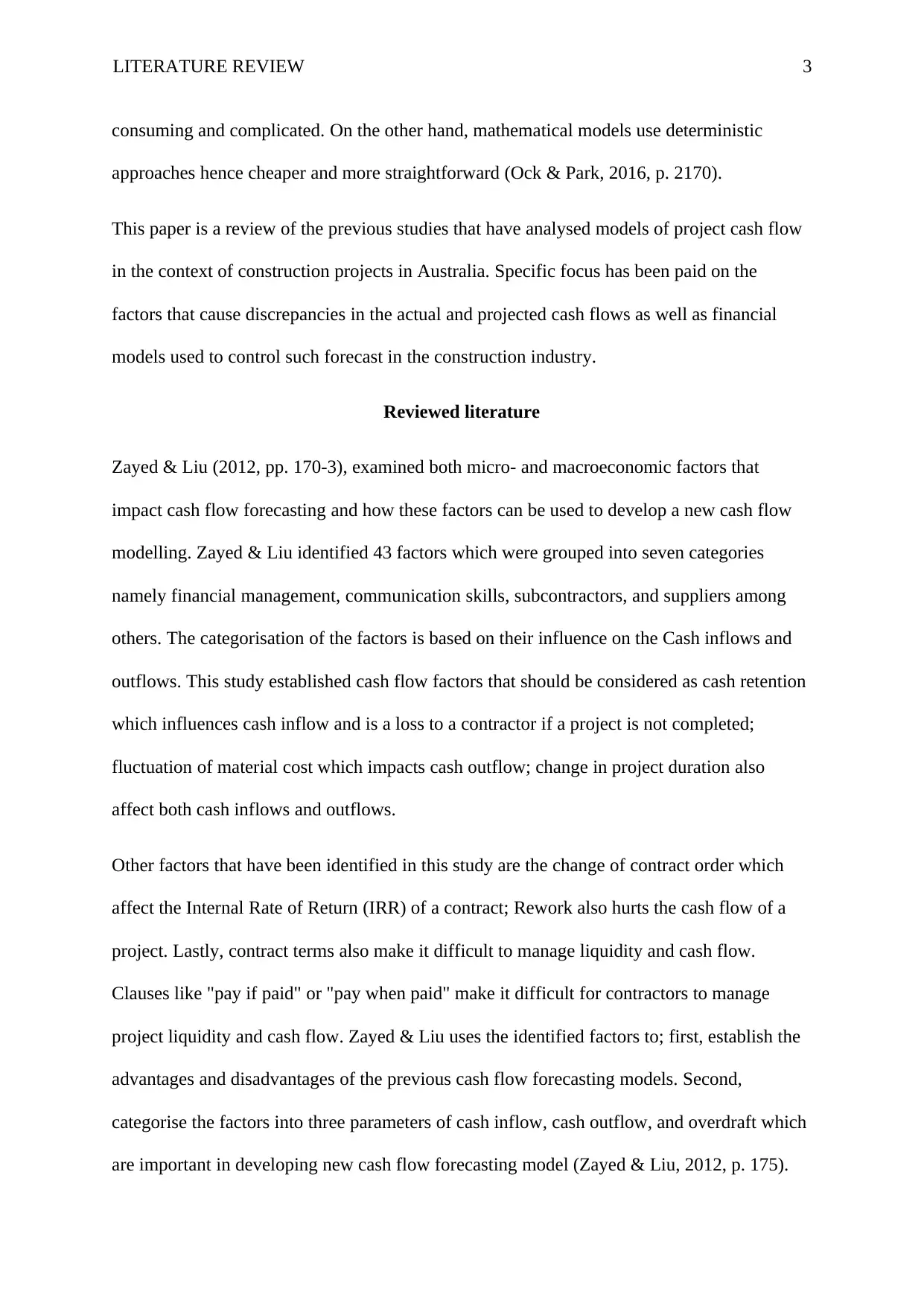
LITERATURE REVIEW 3
consuming and complicated. On the other hand, mathematical models use deterministic
approaches hence cheaper and more straightforward (Ock & Park, 2016, p. 2170).
This paper is a review of the previous studies that have analysed models of project cash flow
in the context of construction projects in Australia. Specific focus has been paid on the
factors that cause discrepancies in the actual and projected cash flows as well as financial
models used to control such forecast in the construction industry.
Reviewed literature
Zayed & Liu (2012, pp. 170-3), examined both micro- and macroeconomic factors that
impact cash flow forecasting and how these factors can be used to develop a new cash flow
modelling. Zayed & Liu identified 43 factors which were grouped into seven categories
namely financial management, communication skills, subcontractors, and suppliers among
others. The categorisation of the factors is based on their influence on the Cash inflows and
outflows. This study established cash flow factors that should be considered as cash retention
which influences cash inflow and is a loss to a contractor if a project is not completed;
fluctuation of material cost which impacts cash outflow; change in project duration also
affect both cash inflows and outflows.
Other factors that have been identified in this study are the change of contract order which
affect the Internal Rate of Return (IRR) of a contract; Rework also hurts the cash flow of a
project. Lastly, contract terms also make it difficult to manage liquidity and cash flow.
Clauses like "pay if paid" or "pay when paid" make it difficult for contractors to manage
project liquidity and cash flow. Zayed & Liu uses the identified factors to; first, establish the
advantages and disadvantages of the previous cash flow forecasting models. Second,
categorise the factors into three parameters of cash inflow, cash outflow, and overdraft which
are important in developing new cash flow forecasting model (Zayed & Liu, 2012, p. 175).
consuming and complicated. On the other hand, mathematical models use deterministic
approaches hence cheaper and more straightforward (Ock & Park, 2016, p. 2170).
This paper is a review of the previous studies that have analysed models of project cash flow
in the context of construction projects in Australia. Specific focus has been paid on the
factors that cause discrepancies in the actual and projected cash flows as well as financial
models used to control such forecast in the construction industry.
Reviewed literature
Zayed & Liu (2012, pp. 170-3), examined both micro- and macroeconomic factors that
impact cash flow forecasting and how these factors can be used to develop a new cash flow
modelling. Zayed & Liu identified 43 factors which were grouped into seven categories
namely financial management, communication skills, subcontractors, and suppliers among
others. The categorisation of the factors is based on their influence on the Cash inflows and
outflows. This study established cash flow factors that should be considered as cash retention
which influences cash inflow and is a loss to a contractor if a project is not completed;
fluctuation of material cost which impacts cash outflow; change in project duration also
affect both cash inflows and outflows.
Other factors that have been identified in this study are the change of contract order which
affect the Internal Rate of Return (IRR) of a contract; Rework also hurts the cash flow of a
project. Lastly, contract terms also make it difficult to manage liquidity and cash flow.
Clauses like "pay if paid" or "pay when paid" make it difficult for contractors to manage
project liquidity and cash flow. Zayed & Liu uses the identified factors to; first, establish the
advantages and disadvantages of the previous cash flow forecasting models. Second,
categorise the factors into three parameters of cash inflow, cash outflow, and overdraft which
are important in developing new cash flow forecasting model (Zayed & Liu, 2012, p. 175).
You're viewing a preview
Unlock full access by subscribing today!
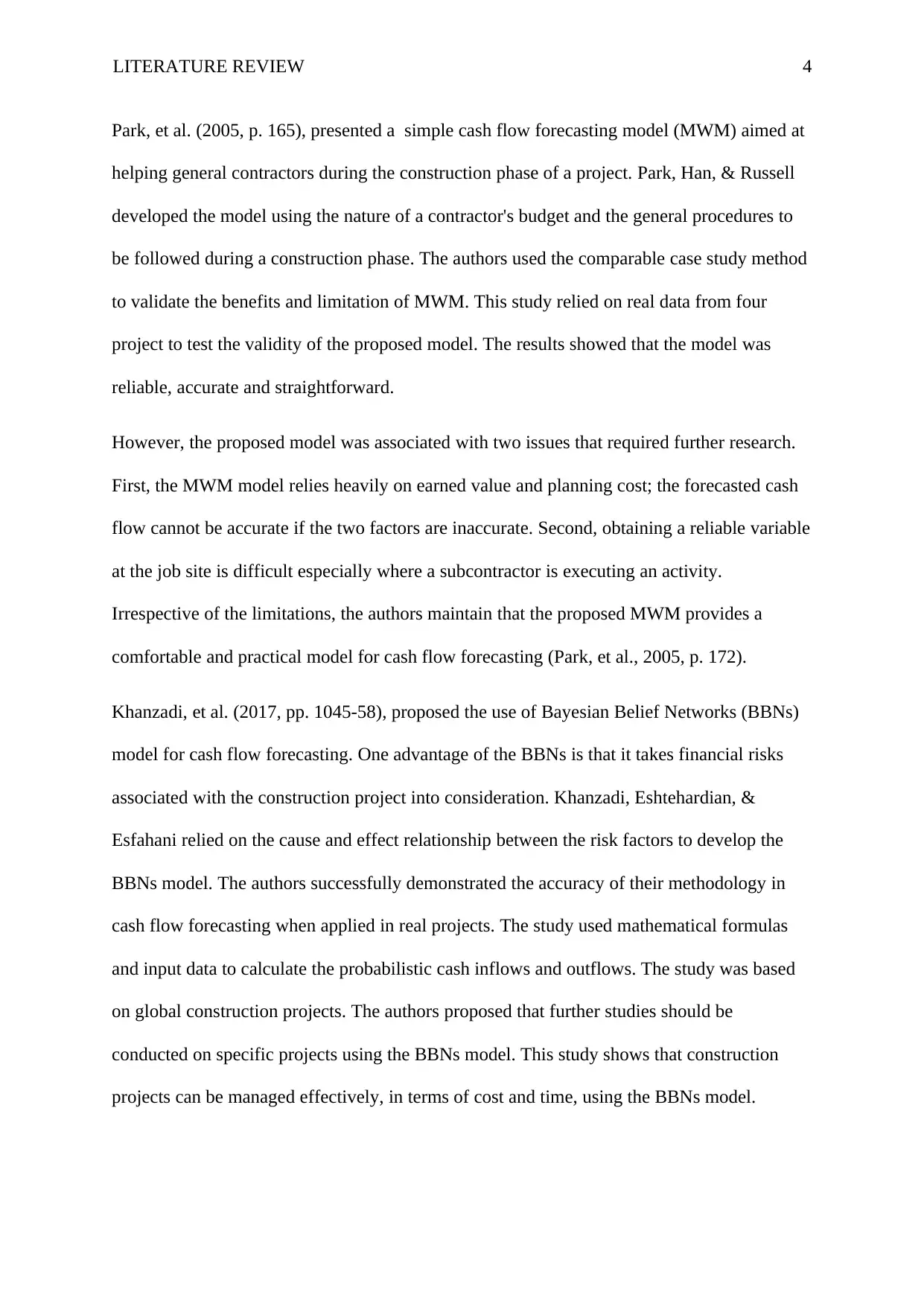
LITERATURE REVIEW 4
Park, et al. (2005, p. 165), presented a simple cash flow forecasting model (MWM) aimed at
helping general contractors during the construction phase of a project. Park, Han, & Russell
developed the model using the nature of a contractor's budget and the general procedures to
be followed during a construction phase. The authors used the comparable case study method
to validate the benefits and limitation of MWM. This study relied on real data from four
project to test the validity of the proposed model. The results showed that the model was
reliable, accurate and straightforward.
However, the proposed model was associated with two issues that required further research.
First, the MWM model relies heavily on earned value and planning cost; the forecasted cash
flow cannot be accurate if the two factors are inaccurate. Second, obtaining a reliable variable
at the job site is difficult especially where a subcontractor is executing an activity.
Irrespective of the limitations, the authors maintain that the proposed MWM provides a
comfortable and practical model for cash flow forecasting (Park, et al., 2005, p. 172).
Khanzadi, et al. (2017, pp. 1045-58), proposed the use of Bayesian Belief Networks (BBNs)
model for cash flow forecasting. One advantage of the BBNs is that it takes financial risks
associated with the construction project into consideration. Khanzadi, Eshtehardian, &
Esfahani relied on the cause and effect relationship between the risk factors to develop the
BBNs model. The authors successfully demonstrated the accuracy of their methodology in
cash flow forecasting when applied in real projects. The study used mathematical formulas
and input data to calculate the probabilistic cash inflows and outflows. The study was based
on global construction projects. The authors proposed that further studies should be
conducted on specific projects using the BBNs model. This study shows that construction
projects can be managed effectively, in terms of cost and time, using the BBNs model.
Park, et al. (2005, p. 165), presented a simple cash flow forecasting model (MWM) aimed at
helping general contractors during the construction phase of a project. Park, Han, & Russell
developed the model using the nature of a contractor's budget and the general procedures to
be followed during a construction phase. The authors used the comparable case study method
to validate the benefits and limitation of MWM. This study relied on real data from four
project to test the validity of the proposed model. The results showed that the model was
reliable, accurate and straightforward.
However, the proposed model was associated with two issues that required further research.
First, the MWM model relies heavily on earned value and planning cost; the forecasted cash
flow cannot be accurate if the two factors are inaccurate. Second, obtaining a reliable variable
at the job site is difficult especially where a subcontractor is executing an activity.
Irrespective of the limitations, the authors maintain that the proposed MWM provides a
comfortable and practical model for cash flow forecasting (Park, et al., 2005, p. 172).
Khanzadi, et al. (2017, pp. 1045-58), proposed the use of Bayesian Belief Networks (BBNs)
model for cash flow forecasting. One advantage of the BBNs is that it takes financial risks
associated with the construction project into consideration. Khanzadi, Eshtehardian, &
Esfahani relied on the cause and effect relationship between the risk factors to develop the
BBNs model. The authors successfully demonstrated the accuracy of their methodology in
cash flow forecasting when applied in real projects. The study used mathematical formulas
and input data to calculate the probabilistic cash inflows and outflows. The study was based
on global construction projects. The authors proposed that further studies should be
conducted on specific projects using the BBNs model. This study shows that construction
projects can be managed effectively, in terms of cost and time, using the BBNs model.
Paraphrase This Document
Need a fresh take? Get an instant paraphrase of this document with our AI Paraphraser
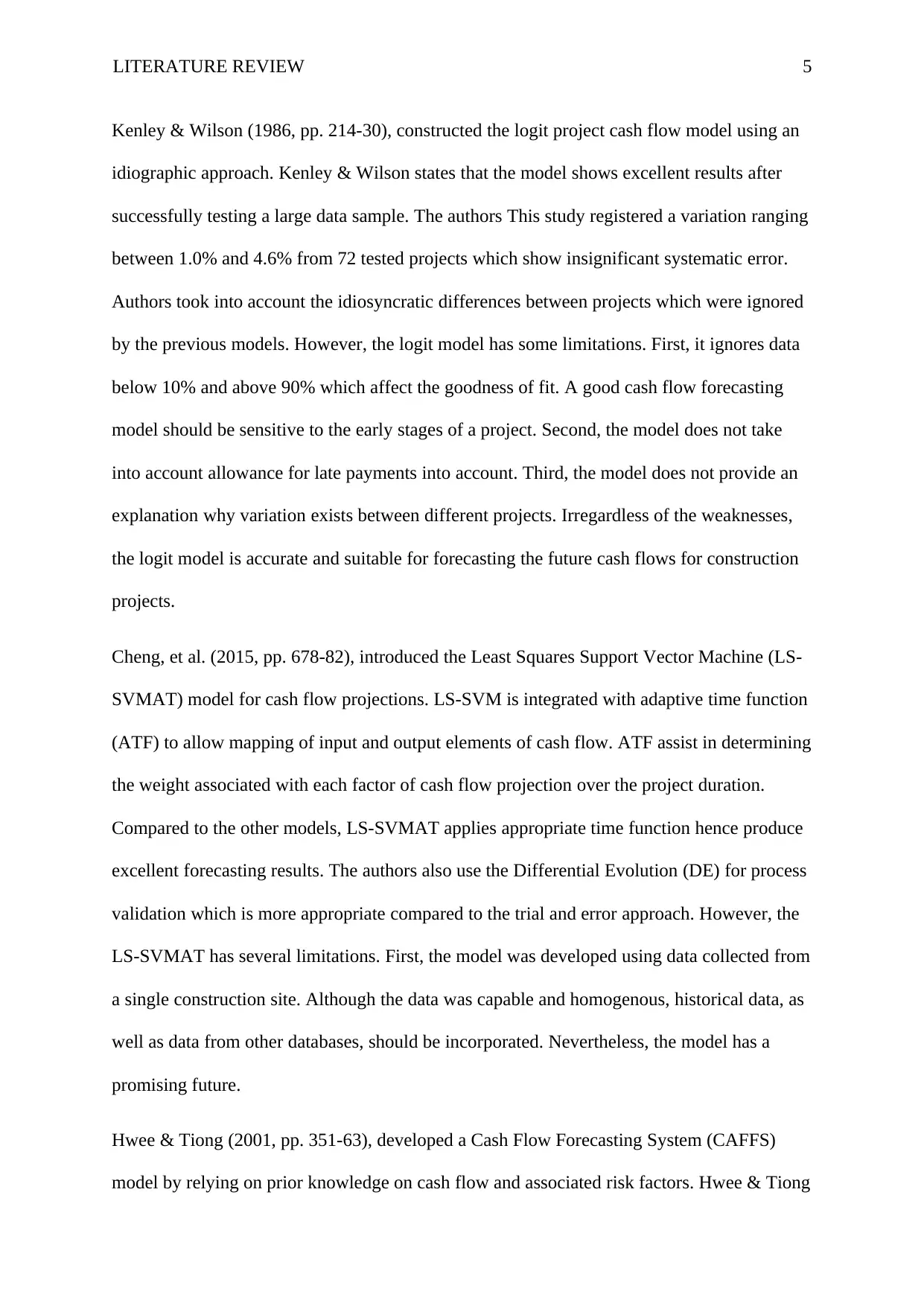
LITERATURE REVIEW 5
Kenley & Wilson (1986, pp. 214-30), constructed the logit project cash flow model using an
idiographic approach. Kenley & Wilson states that the model shows excellent results after
successfully testing a large data sample. The authors This study registered a variation ranging
between 1.0% and 4.6% from 72 tested projects which show insignificant systematic error.
Authors took into account the idiosyncratic differences between projects which were ignored
by the previous models. However, the logit model has some limitations. First, it ignores data
below 10% and above 90% which affect the goodness of fit. A good cash flow forecasting
model should be sensitive to the early stages of a project. Second, the model does not take
into account allowance for late payments into account. Third, the model does not provide an
explanation why variation exists between different projects. Irregardless of the weaknesses,
the logit model is accurate and suitable for forecasting the future cash flows for construction
projects.
Cheng, et al. (2015, pp. 678-82), introduced the Least Squares Support Vector Machine (LS-
SVMAT) model for cash flow projections. LS-SVM is integrated with adaptive time function
(ATF) to allow mapping of input and output elements of cash flow. ATF assist in determining
the weight associated with each factor of cash flow projection over the project duration.
Compared to the other models, LS-SVMAT applies appropriate time function hence produce
excellent forecasting results. The authors also use the Differential Evolution (DE) for process
validation which is more appropriate compared to the trial and error approach. However, the
LS-SVMAT has several limitations. First, the model was developed using data collected from
a single construction site. Although the data was capable and homogenous, historical data, as
well as data from other databases, should be incorporated. Nevertheless, the model has a
promising future.
Hwee & Tiong (2001, pp. 351-63), developed a Cash Flow Forecasting System (CAFFS)
model by relying on prior knowledge on cash flow and associated risk factors. Hwee & Tiong
Kenley & Wilson (1986, pp. 214-30), constructed the logit project cash flow model using an
idiographic approach. Kenley & Wilson states that the model shows excellent results after
successfully testing a large data sample. The authors This study registered a variation ranging
between 1.0% and 4.6% from 72 tested projects which show insignificant systematic error.
Authors took into account the idiosyncratic differences between projects which were ignored
by the previous models. However, the logit model has some limitations. First, it ignores data
below 10% and above 90% which affect the goodness of fit. A good cash flow forecasting
model should be sensitive to the early stages of a project. Second, the model does not take
into account allowance for late payments into account. Third, the model does not provide an
explanation why variation exists between different projects. Irregardless of the weaknesses,
the logit model is accurate and suitable for forecasting the future cash flows for construction
projects.
Cheng, et al. (2015, pp. 678-82), introduced the Least Squares Support Vector Machine (LS-
SVMAT) model for cash flow projections. LS-SVM is integrated with adaptive time function
(ATF) to allow mapping of input and output elements of cash flow. ATF assist in determining
the weight associated with each factor of cash flow projection over the project duration.
Compared to the other models, LS-SVMAT applies appropriate time function hence produce
excellent forecasting results. The authors also use the Differential Evolution (DE) for process
validation which is more appropriate compared to the trial and error approach. However, the
LS-SVMAT has several limitations. First, the model was developed using data collected from
a single construction site. Although the data was capable and homogenous, historical data, as
well as data from other databases, should be incorporated. Nevertheless, the model has a
promising future.
Hwee & Tiong (2001, pp. 351-63), developed a Cash Flow Forecasting System (CAFFS)
model by relying on prior knowledge on cash flow and associated risk factors. Hwee & Tiong
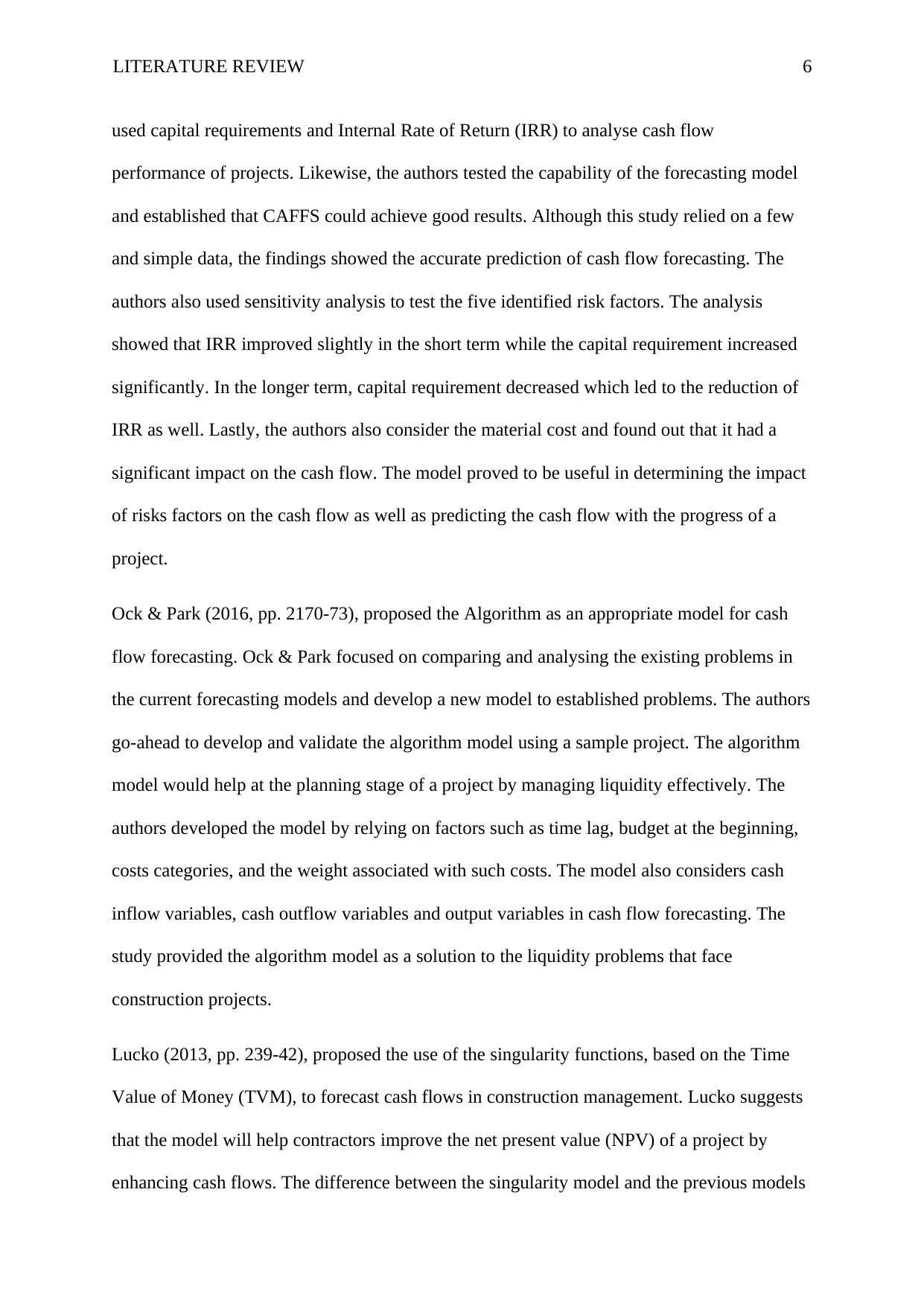
LITERATURE REVIEW 6
used capital requirements and Internal Rate of Return (IRR) to analyse cash flow
performance of projects. Likewise, the authors tested the capability of the forecasting model
and established that CAFFS could achieve good results. Although this study relied on a few
and simple data, the findings showed the accurate prediction of cash flow forecasting. The
authors also used sensitivity analysis to test the five identified risk factors. The analysis
showed that IRR improved slightly in the short term while the capital requirement increased
significantly. In the longer term, capital requirement decreased which led to the reduction of
IRR as well. Lastly, the authors also consider the material cost and found out that it had a
significant impact on the cash flow. The model proved to be useful in determining the impact
of risks factors on the cash flow as well as predicting the cash flow with the progress of a
project.
Ock & Park (2016, pp. 2170-73), proposed the Algorithm as an appropriate model for cash
flow forecasting. Ock & Park focused on comparing and analysing the existing problems in
the current forecasting models and develop a new model to established problems. The authors
go-ahead to develop and validate the algorithm model using a sample project. The algorithm
model would help at the planning stage of a project by managing liquidity effectively. The
authors developed the model by relying on factors such as time lag, budget at the beginning,
costs categories, and the weight associated with such costs. The model also considers cash
inflow variables, cash outflow variables and output variables in cash flow forecasting. The
study provided the algorithm model as a solution to the liquidity problems that face
construction projects.
Lucko (2013, pp. 239-42), proposed the use of the singularity functions, based on the Time
Value of Money (TVM), to forecast cash flows in construction management. Lucko suggests
that the model will help contractors improve the net present value (NPV) of a project by
enhancing cash flows. The difference between the singularity model and the previous models
used capital requirements and Internal Rate of Return (IRR) to analyse cash flow
performance of projects. Likewise, the authors tested the capability of the forecasting model
and established that CAFFS could achieve good results. Although this study relied on a few
and simple data, the findings showed the accurate prediction of cash flow forecasting. The
authors also used sensitivity analysis to test the five identified risk factors. The analysis
showed that IRR improved slightly in the short term while the capital requirement increased
significantly. In the longer term, capital requirement decreased which led to the reduction of
IRR as well. Lastly, the authors also consider the material cost and found out that it had a
significant impact on the cash flow. The model proved to be useful in determining the impact
of risks factors on the cash flow as well as predicting the cash flow with the progress of a
project.
Ock & Park (2016, pp. 2170-73), proposed the Algorithm as an appropriate model for cash
flow forecasting. Ock & Park focused on comparing and analysing the existing problems in
the current forecasting models and develop a new model to established problems. The authors
go-ahead to develop and validate the algorithm model using a sample project. The algorithm
model would help at the planning stage of a project by managing liquidity effectively. The
authors developed the model by relying on factors such as time lag, budget at the beginning,
costs categories, and the weight associated with such costs. The model also considers cash
inflow variables, cash outflow variables and output variables in cash flow forecasting. The
study provided the algorithm model as a solution to the liquidity problems that face
construction projects.
Lucko (2013, pp. 239-42), proposed the use of the singularity functions, based on the Time
Value of Money (TVM), to forecast cash flows in construction management. Lucko suggests
that the model will help contractors improve the net present value (NPV) of a project by
enhancing cash flows. The difference between the singularity model and the previous models
You're viewing a preview
Unlock full access by subscribing today!
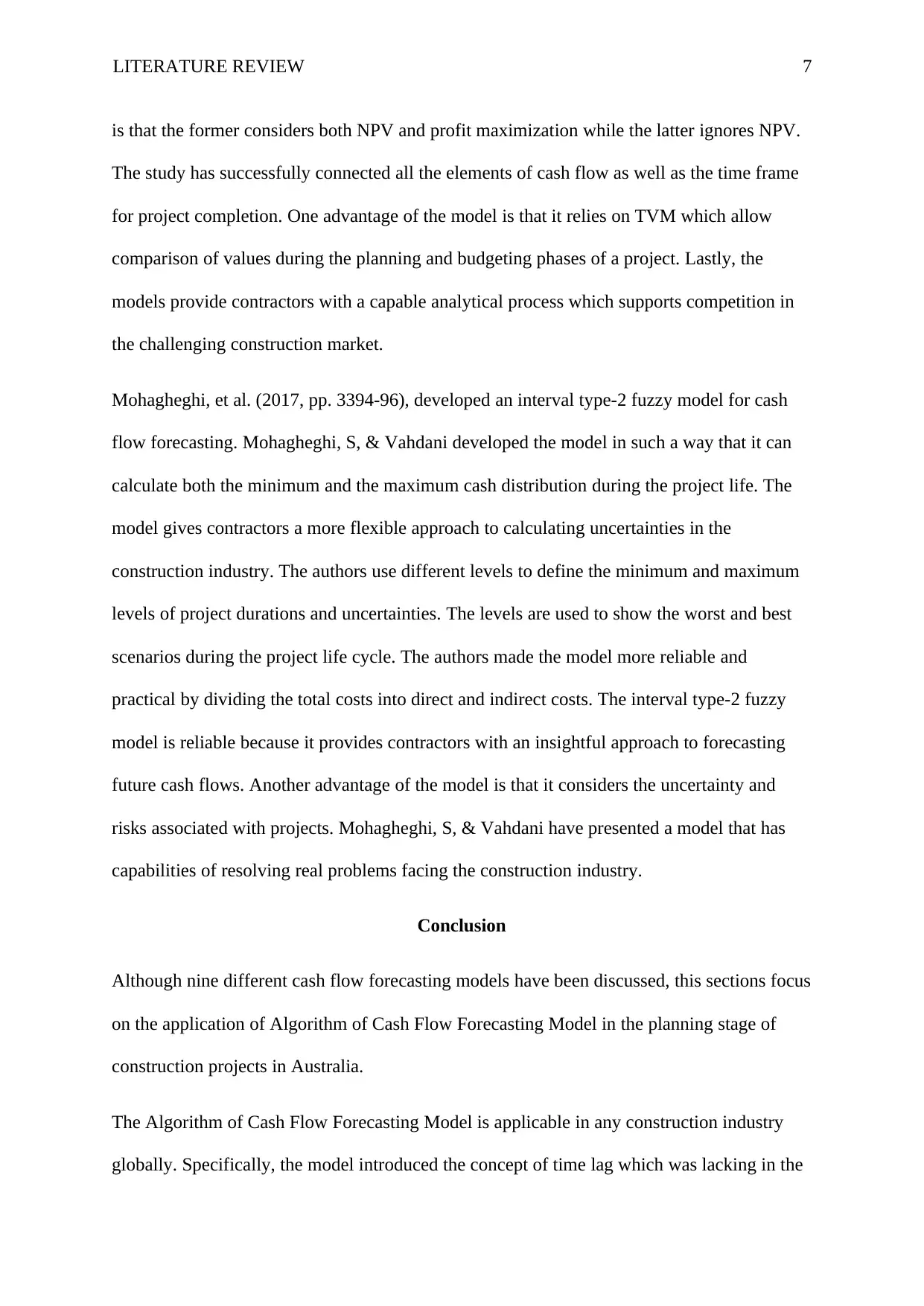
LITERATURE REVIEW 7
is that the former considers both NPV and profit maximization while the latter ignores NPV.
The study has successfully connected all the elements of cash flow as well as the time frame
for project completion. One advantage of the model is that it relies on TVM which allow
comparison of values during the planning and budgeting phases of a project. Lastly, the
models provide contractors with a capable analytical process which supports competition in
the challenging construction market.
Mohagheghi, et al. (2017, pp. 3394-96), developed an interval type-2 fuzzy model for cash
flow forecasting. Mohagheghi, S, & Vahdani developed the model in such a way that it can
calculate both the minimum and the maximum cash distribution during the project life. The
model gives contractors a more flexible approach to calculating uncertainties in the
construction industry. The authors use different levels to define the minimum and maximum
levels of project durations and uncertainties. The levels are used to show the worst and best
scenarios during the project life cycle. The authors made the model more reliable and
practical by dividing the total costs into direct and indirect costs. The interval type-2 fuzzy
model is reliable because it provides contractors with an insightful approach to forecasting
future cash flows. Another advantage of the model is that it considers the uncertainty and
risks associated with projects. Mohagheghi, S, & Vahdani have presented a model that has
capabilities of resolving real problems facing the construction industry.
Conclusion
Although nine different cash flow forecasting models have been discussed, this sections focus
on the application of Algorithm of Cash Flow Forecasting Model in the planning stage of
construction projects in Australia.
The Algorithm of Cash Flow Forecasting Model is applicable in any construction industry
globally. Specifically, the model introduced the concept of time lag which was lacking in the
is that the former considers both NPV and profit maximization while the latter ignores NPV.
The study has successfully connected all the elements of cash flow as well as the time frame
for project completion. One advantage of the model is that it relies on TVM which allow
comparison of values during the planning and budgeting phases of a project. Lastly, the
models provide contractors with a capable analytical process which supports competition in
the challenging construction market.
Mohagheghi, et al. (2017, pp. 3394-96), developed an interval type-2 fuzzy model for cash
flow forecasting. Mohagheghi, S, & Vahdani developed the model in such a way that it can
calculate both the minimum and the maximum cash distribution during the project life. The
model gives contractors a more flexible approach to calculating uncertainties in the
construction industry. The authors use different levels to define the minimum and maximum
levels of project durations and uncertainties. The levels are used to show the worst and best
scenarios during the project life cycle. The authors made the model more reliable and
practical by dividing the total costs into direct and indirect costs. The interval type-2 fuzzy
model is reliable because it provides contractors with an insightful approach to forecasting
future cash flows. Another advantage of the model is that it considers the uncertainty and
risks associated with projects. Mohagheghi, S, & Vahdani have presented a model that has
capabilities of resolving real problems facing the construction industry.
Conclusion
Although nine different cash flow forecasting models have been discussed, this sections focus
on the application of Algorithm of Cash Flow Forecasting Model in the planning stage of
construction projects in Australia.
The Algorithm of Cash Flow Forecasting Model is applicable in any construction industry
globally. Specifically, the model introduced the concept of time lag which was lacking in the
Paraphrase This Document
Need a fresh take? Get an instant paraphrase of this document with our AI Paraphraser
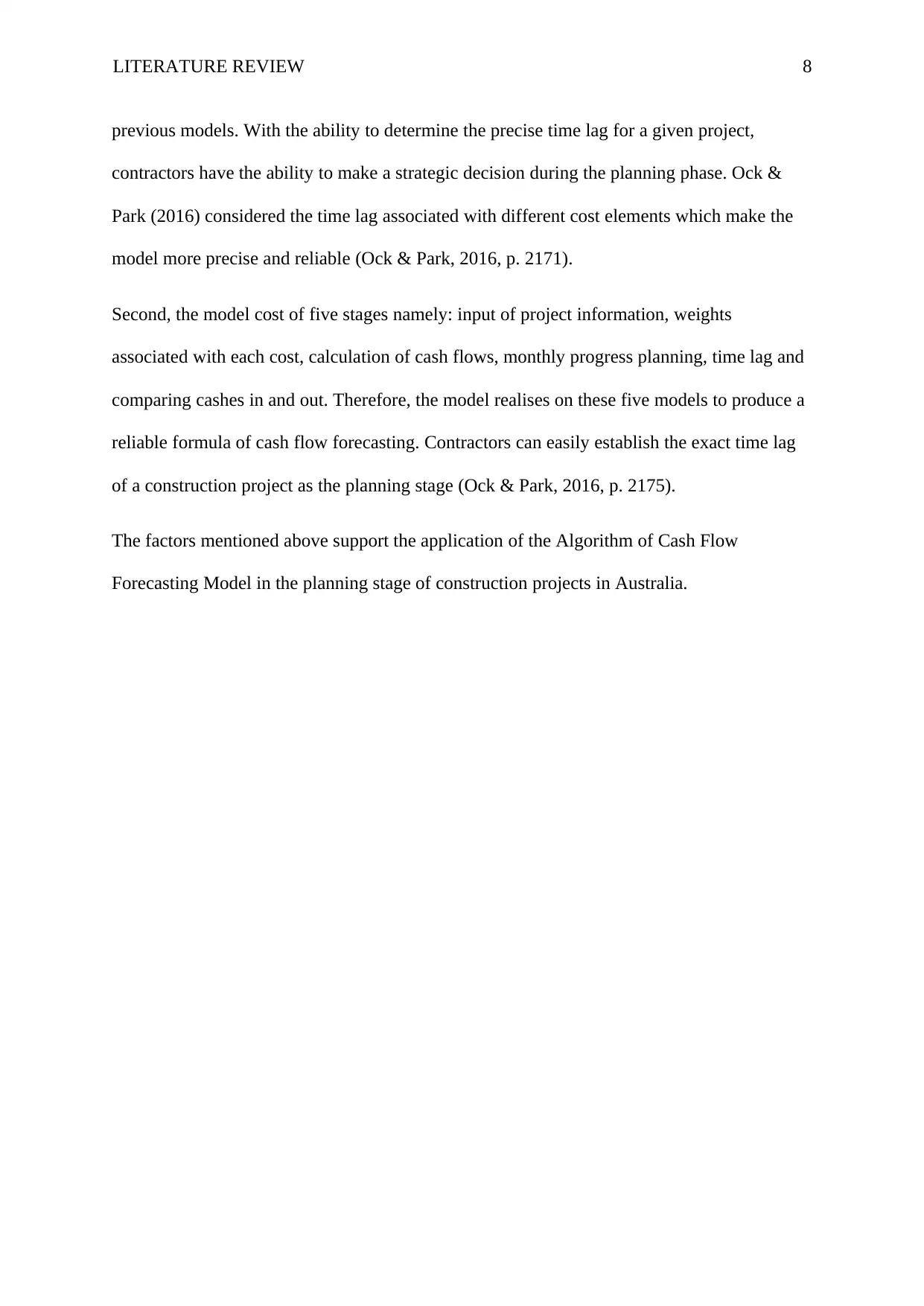
LITERATURE REVIEW 8
previous models. With the ability to determine the precise time lag for a given project,
contractors have the ability to make a strategic decision during the planning phase. Ock &
Park (2016) considered the time lag associated with different cost elements which make the
model more precise and reliable (Ock & Park, 2016, p. 2171).
Second, the model cost of five stages namely: input of project information, weights
associated with each cost, calculation of cash flows, monthly progress planning, time lag and
comparing cashes in and out. Therefore, the model realises on these five models to produce a
reliable formula of cash flow forecasting. Contractors can easily establish the exact time lag
of a construction project as the planning stage (Ock & Park, 2016, p. 2175).
The factors mentioned above support the application of the Algorithm of Cash Flow
Forecasting Model in the planning stage of construction projects in Australia.
previous models. With the ability to determine the precise time lag for a given project,
contractors have the ability to make a strategic decision during the planning phase. Ock &
Park (2016) considered the time lag associated with different cost elements which make the
model more precise and reliable (Ock & Park, 2016, p. 2171).
Second, the model cost of five stages namely: input of project information, weights
associated with each cost, calculation of cash flows, monthly progress planning, time lag and
comparing cashes in and out. Therefore, the model realises on these five models to produce a
reliable formula of cash flow forecasting. Contractors can easily establish the exact time lag
of a construction project as the planning stage (Ock & Park, 2016, p. 2175).
The factors mentioned above support the application of the Algorithm of Cash Flow
Forecasting Model in the planning stage of construction projects in Australia.
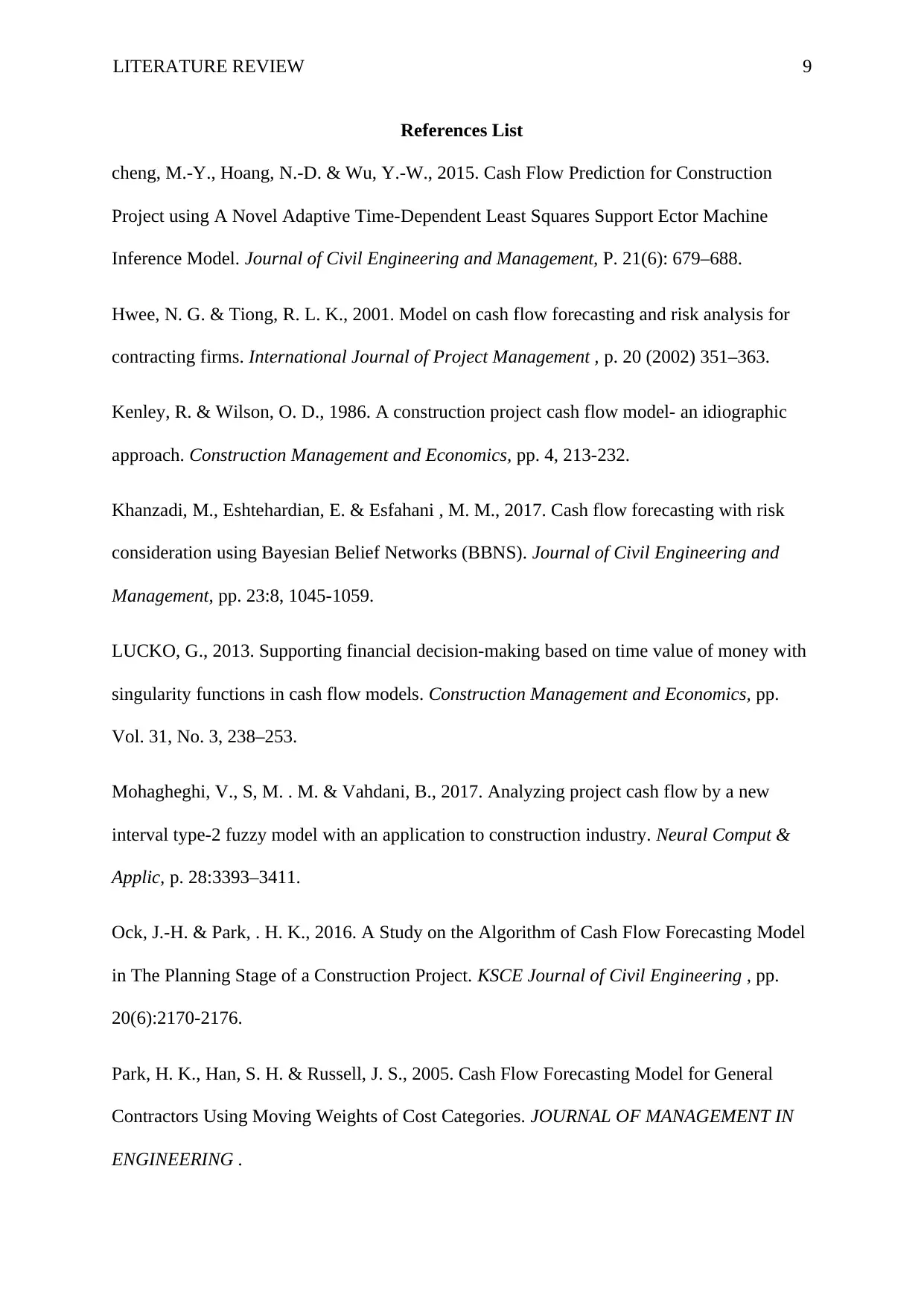
LITERATURE REVIEW 9
References List
cheng, M.-Y., Hoang, N.-D. & Wu, Y.-W., 2015. Cash Flow Prediction for Construction
Project using A Novel Adaptive Time-Dependent Least Squares Support Ector Machine
Inference Model. Journal of Civil Engineering and Management, P. 21(6): 679–688.
Hwee, N. G. & Tiong, R. L. K., 2001. Model on cash flow forecasting and risk analysis for
contracting firms. International Journal of Project Management , p. 20 (2002) 351–363.
Kenley, R. & Wilson, O. D., 1986. A construction project cash flow model- an idiographic
approach. Construction Management and Economics, pp. 4, 213-232.
Khanzadi, M., Eshtehardian, E. & Esfahani , M. M., 2017. Cash flow forecasting with risk
consideration using Bayesian Belief Networks (BBNS). Journal of Civil Engineering and
Management, pp. 23:8, 1045-1059.
LUCKO, G., 2013. Supporting financial decision-making based on time value of money with
singularity functions in cash flow models. Construction Management and Economics, pp.
Vol. 31, No. 3, 238–253.
Mohagheghi, V., S, M. . M. & Vahdani, B., 2017. Analyzing project cash flow by a new
interval type-2 fuzzy model with an application to construction industry. Neural Comput &
Applic, p. 28:3393–3411.
Ock, J.-H. & Park, . H. K., 2016. A Study on the Algorithm of Cash Flow Forecasting Model
in The Planning Stage of a Construction Project. KSCE Journal of Civil Engineering , pp.
20(6):2170-2176.
Park, H. K., Han, S. H. & Russell, J. S., 2005. Cash Flow Forecasting Model for General
Contractors Using Moving Weights of Cost Categories. JOURNAL OF MANAGEMENT IN
ENGINEERING .
References List
cheng, M.-Y., Hoang, N.-D. & Wu, Y.-W., 2015. Cash Flow Prediction for Construction
Project using A Novel Adaptive Time-Dependent Least Squares Support Ector Machine
Inference Model. Journal of Civil Engineering and Management, P. 21(6): 679–688.
Hwee, N. G. & Tiong, R. L. K., 2001. Model on cash flow forecasting and risk analysis for
contracting firms. International Journal of Project Management , p. 20 (2002) 351–363.
Kenley, R. & Wilson, O. D., 1986. A construction project cash flow model- an idiographic
approach. Construction Management and Economics, pp. 4, 213-232.
Khanzadi, M., Eshtehardian, E. & Esfahani , M. M., 2017. Cash flow forecasting with risk
consideration using Bayesian Belief Networks (BBNS). Journal of Civil Engineering and
Management, pp. 23:8, 1045-1059.
LUCKO, G., 2013. Supporting financial decision-making based on time value of money with
singularity functions in cash flow models. Construction Management and Economics, pp.
Vol. 31, No. 3, 238–253.
Mohagheghi, V., S, M. . M. & Vahdani, B., 2017. Analyzing project cash flow by a new
interval type-2 fuzzy model with an application to construction industry. Neural Comput &
Applic, p. 28:3393–3411.
Ock, J.-H. & Park, . H. K., 2016. A Study on the Algorithm of Cash Flow Forecasting Model
in The Planning Stage of a Construction Project. KSCE Journal of Civil Engineering , pp.
20(6):2170-2176.
Park, H. K., Han, S. H. & Russell, J. S., 2005. Cash Flow Forecasting Model for General
Contractors Using Moving Weights of Cost Categories. JOURNAL OF MANAGEMENT IN
ENGINEERING .
You're viewing a preview
Unlock full access by subscribing today!
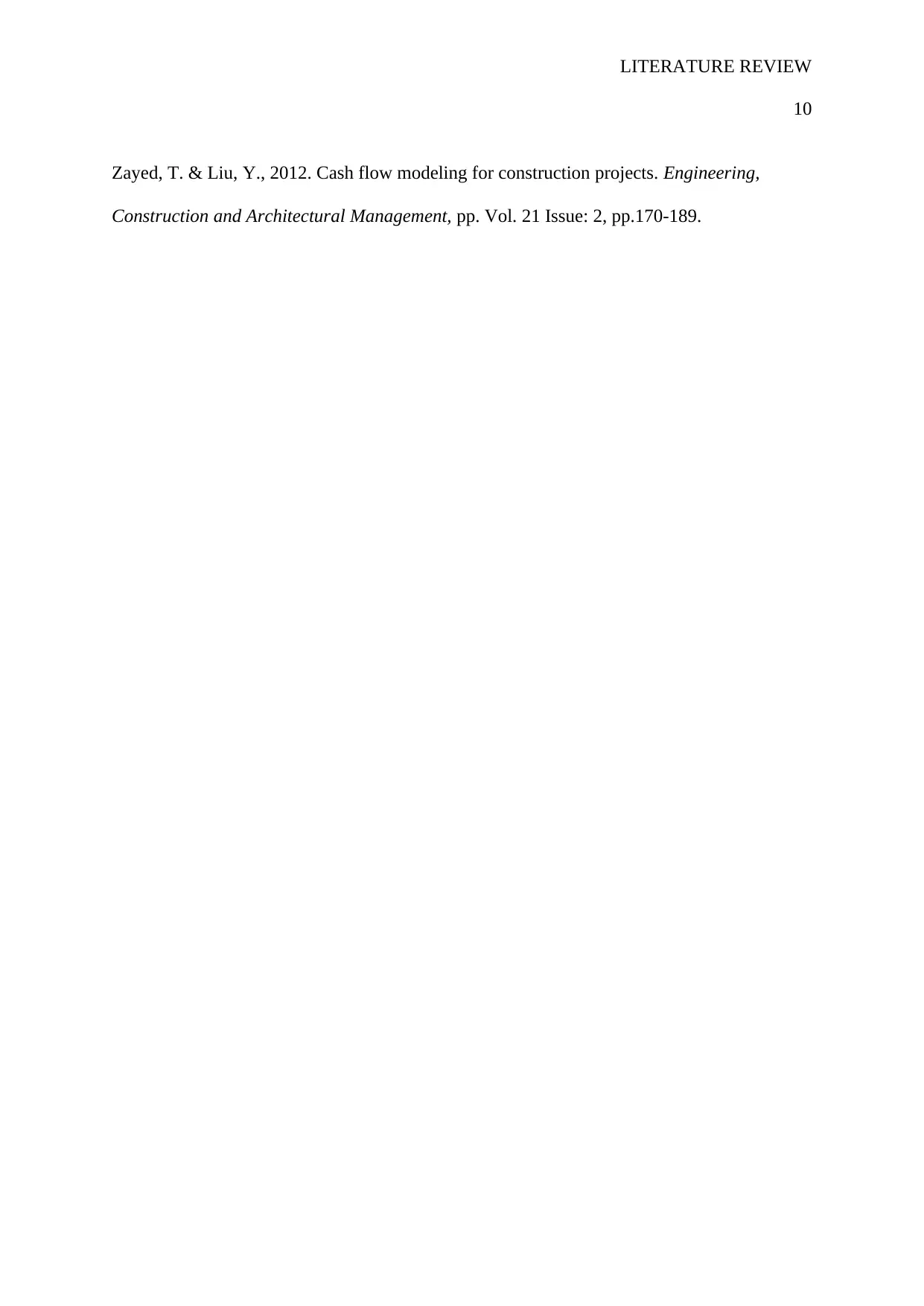
LITERATURE REVIEW
10
Zayed, T. & Liu, Y., 2012. Cash flow modeling for construction projects. Engineering,
Construction and Architectural Management, pp. Vol. 21 Issue: 2, pp.170-189.
10
Zayed, T. & Liu, Y., 2012. Cash flow modeling for construction projects. Engineering,
Construction and Architectural Management, pp. Vol. 21 Issue: 2, pp.170-189.
1 out of 10
Related Documents

Your All-in-One AI-Powered Toolkit for Academic Success.
+13062052269
info@desklib.com
Available 24*7 on WhatsApp / Email
Unlock your academic potential
© 2024 | Zucol Services PVT LTD | All rights reserved.