Data Analytics Report: Data Significance, Predictions, and Analysis
VerifiedAdded on 2022/11/16
|14
|3775
|281
Report
AI Summary
This report delves into the critical role of data analytics in modern business environments. It emphasizes the significance of data in facilitating informed decision-making, supported by statistical inferences and trends. The report explores how businesses utilize data, including demographic, pricing, and customer feedback, to develop effective strategies. It also highlights the power of predictive analytics in forecasting future events and identifying opportunities, using examples from various industries. The report includes an analysis of unemployment data from California, demonstrating how data can be used to make predictions about economic growth and investment opportunities. The analysis reveals declining unemployment rates, suggesting potential economic expansion. The report concludes with recommendations for maximizing the use of data analytics to enhance business performance.
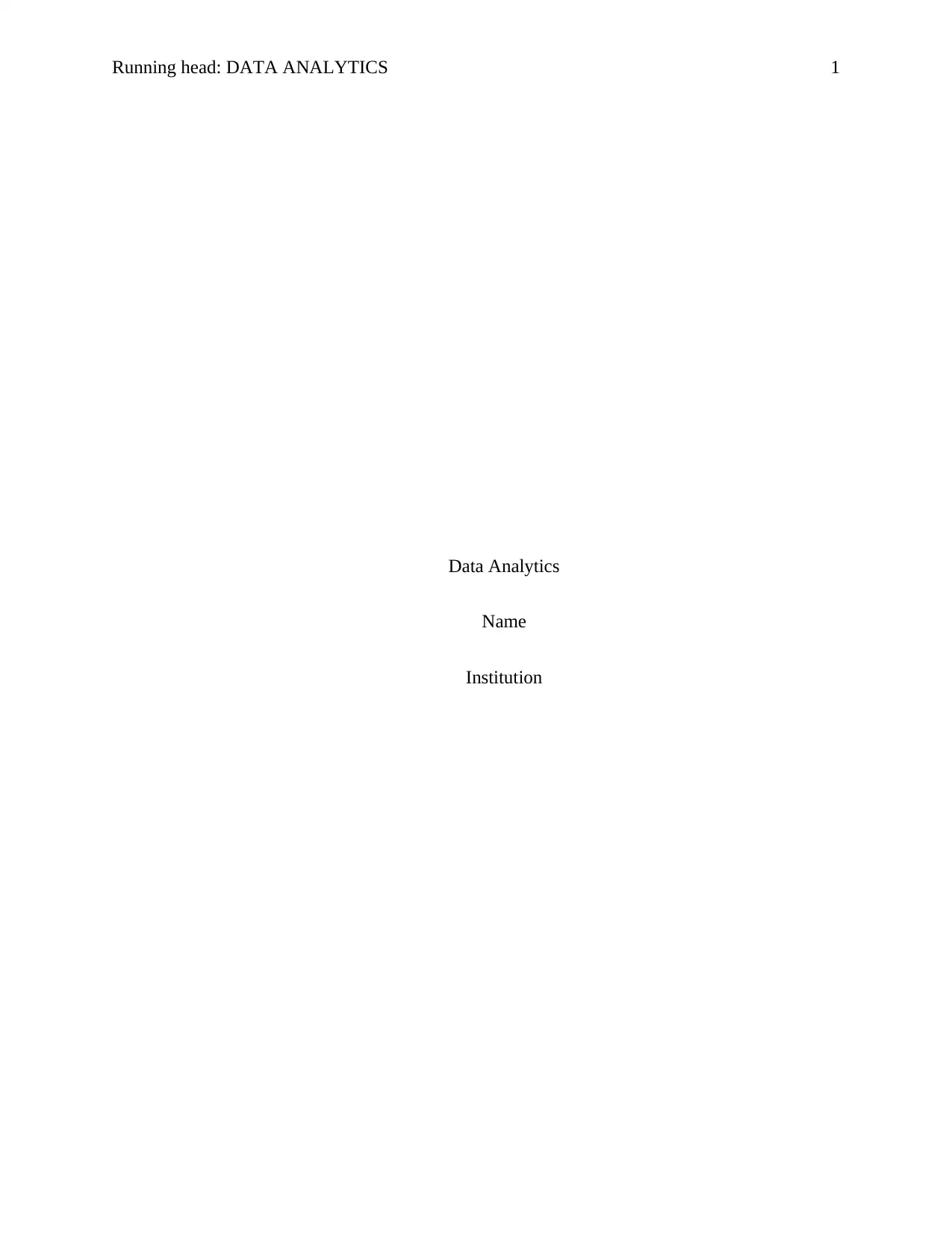
Running head: DATA ANALYTICS 1
Data Analytics
Name
Institution
Data Analytics
Name
Institution
Secure Best Marks with AI Grader
Need help grading? Try our AI Grader for instant feedback on your assignments.
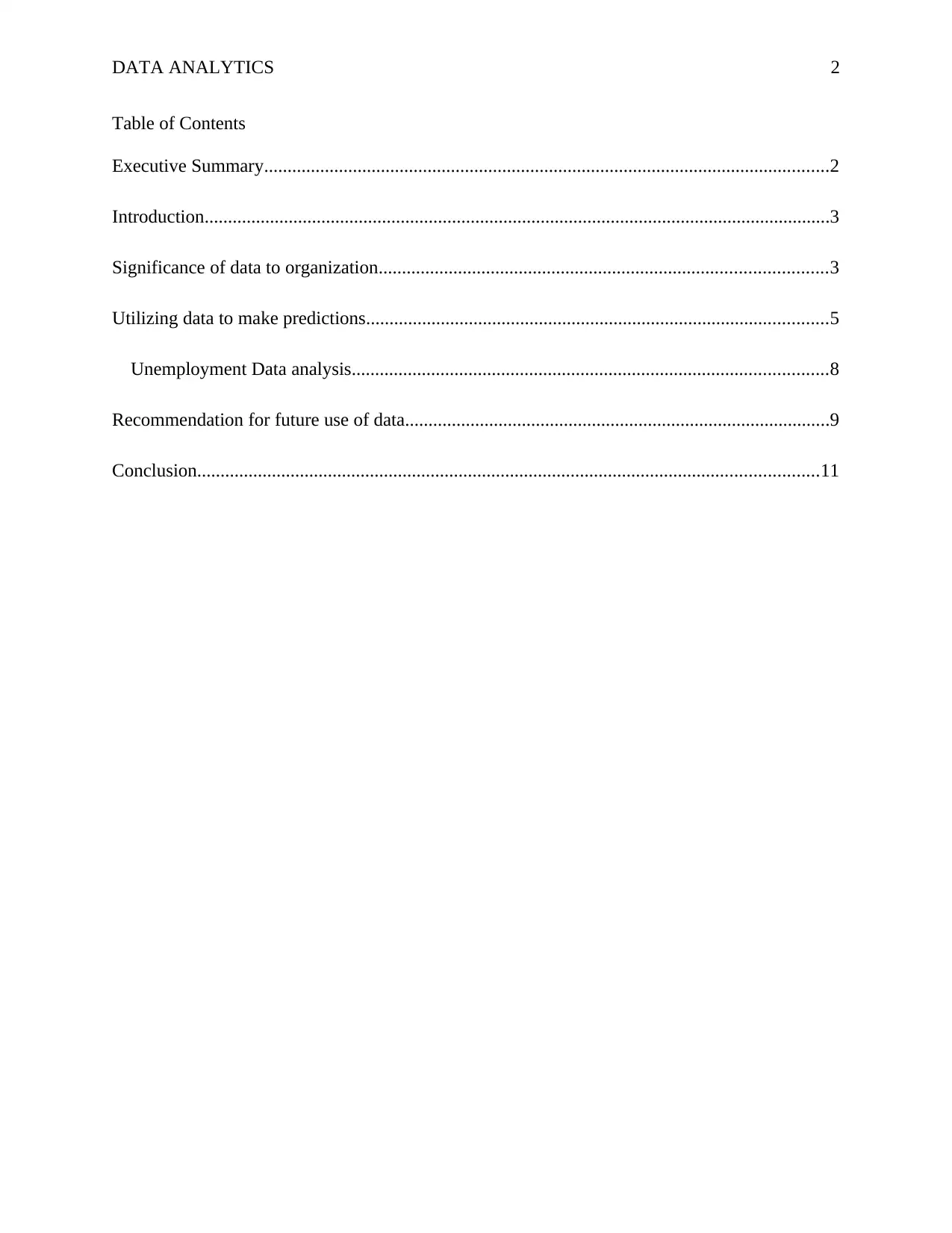
DATA ANALYTICS 2
Table of Contents
Executive Summary.........................................................................................................................2
Introduction......................................................................................................................................3
Significance of data to organization................................................................................................3
Utilizing data to make predictions...................................................................................................5
Unemployment Data analysis......................................................................................................8
Recommendation for future use of data...........................................................................................9
Conclusion.....................................................................................................................................11
Table of Contents
Executive Summary.........................................................................................................................2
Introduction......................................................................................................................................3
Significance of data to organization................................................................................................3
Utilizing data to make predictions...................................................................................................5
Unemployment Data analysis......................................................................................................8
Recommendation for future use of data...........................................................................................9
Conclusion.....................................................................................................................................11
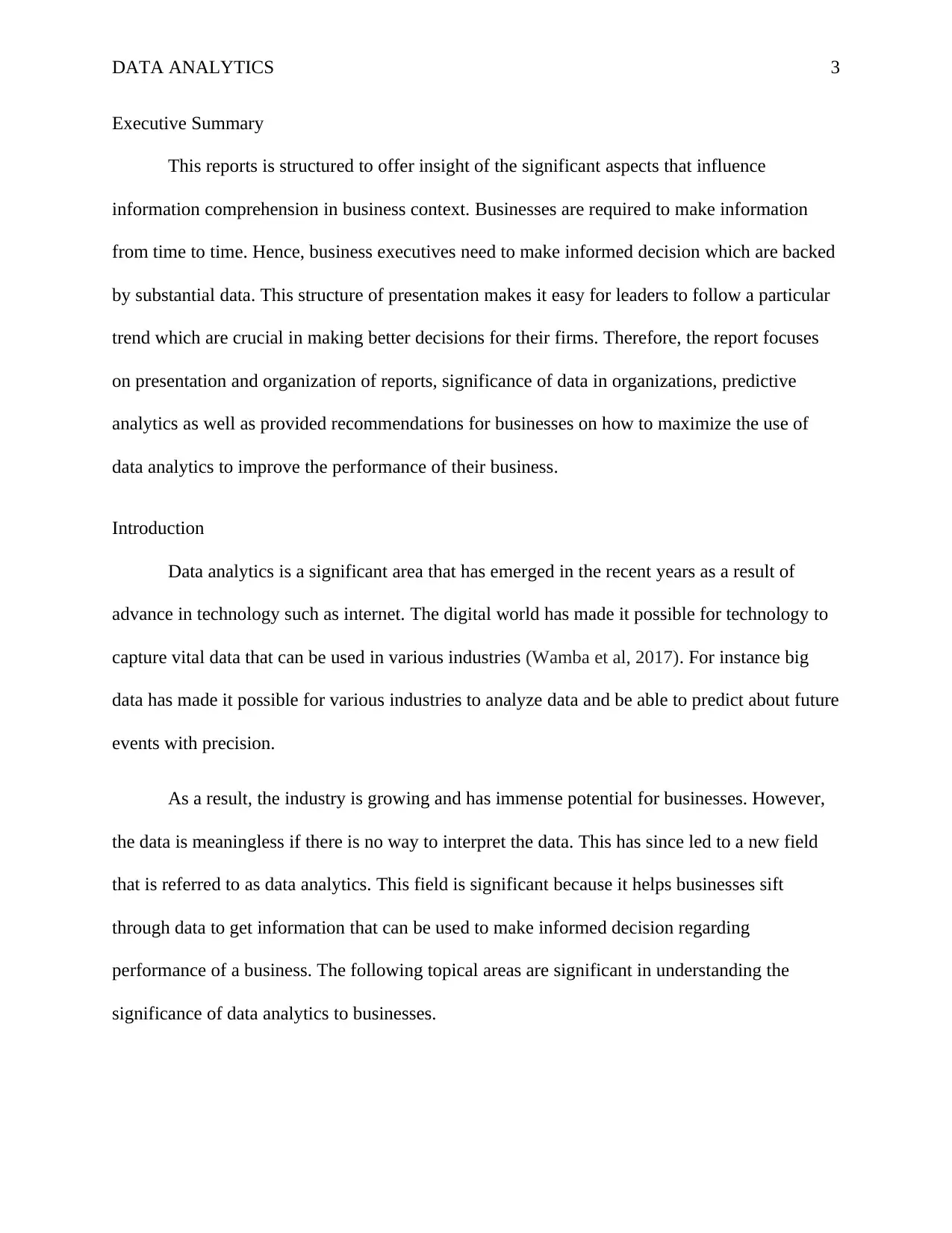
DATA ANALYTICS 3
Executive Summary
This reports is structured to offer insight of the significant aspects that influence
information comprehension in business context. Businesses are required to make information
from time to time. Hence, business executives need to make informed decision which are backed
by substantial data. This structure of presentation makes it easy for leaders to follow a particular
trend which are crucial in making better decisions for their firms. Therefore, the report focuses
on presentation and organization of reports, significance of data in organizations, predictive
analytics as well as provided recommendations for businesses on how to maximize the use of
data analytics to improve the performance of their business.
Introduction
Data analytics is a significant area that has emerged in the recent years as a result of
advance in technology such as internet. The digital world has made it possible for technology to
capture vital data that can be used in various industries (Wamba et al, 2017). For instance big
data has made it possible for various industries to analyze data and be able to predict about future
events with precision.
As a result, the industry is growing and has immense potential for businesses. However,
the data is meaningless if there is no way to interpret the data. This has since led to a new field
that is referred to as data analytics. This field is significant because it helps businesses sift
through data to get information that can be used to make informed decision regarding
performance of a business. The following topical areas are significant in understanding the
significance of data analytics to businesses.
Executive Summary
This reports is structured to offer insight of the significant aspects that influence
information comprehension in business context. Businesses are required to make information
from time to time. Hence, business executives need to make informed decision which are backed
by substantial data. This structure of presentation makes it easy for leaders to follow a particular
trend which are crucial in making better decisions for their firms. Therefore, the report focuses
on presentation and organization of reports, significance of data in organizations, predictive
analytics as well as provided recommendations for businesses on how to maximize the use of
data analytics to improve the performance of their business.
Introduction
Data analytics is a significant area that has emerged in the recent years as a result of
advance in technology such as internet. The digital world has made it possible for technology to
capture vital data that can be used in various industries (Wamba et al, 2017). For instance big
data has made it possible for various industries to analyze data and be able to predict about future
events with precision.
As a result, the industry is growing and has immense potential for businesses. However,
the data is meaningless if there is no way to interpret the data. This has since led to a new field
that is referred to as data analytics. This field is significant because it helps businesses sift
through data to get information that can be used to make informed decision regarding
performance of a business. The following topical areas are significant in understanding the
significance of data analytics to businesses.
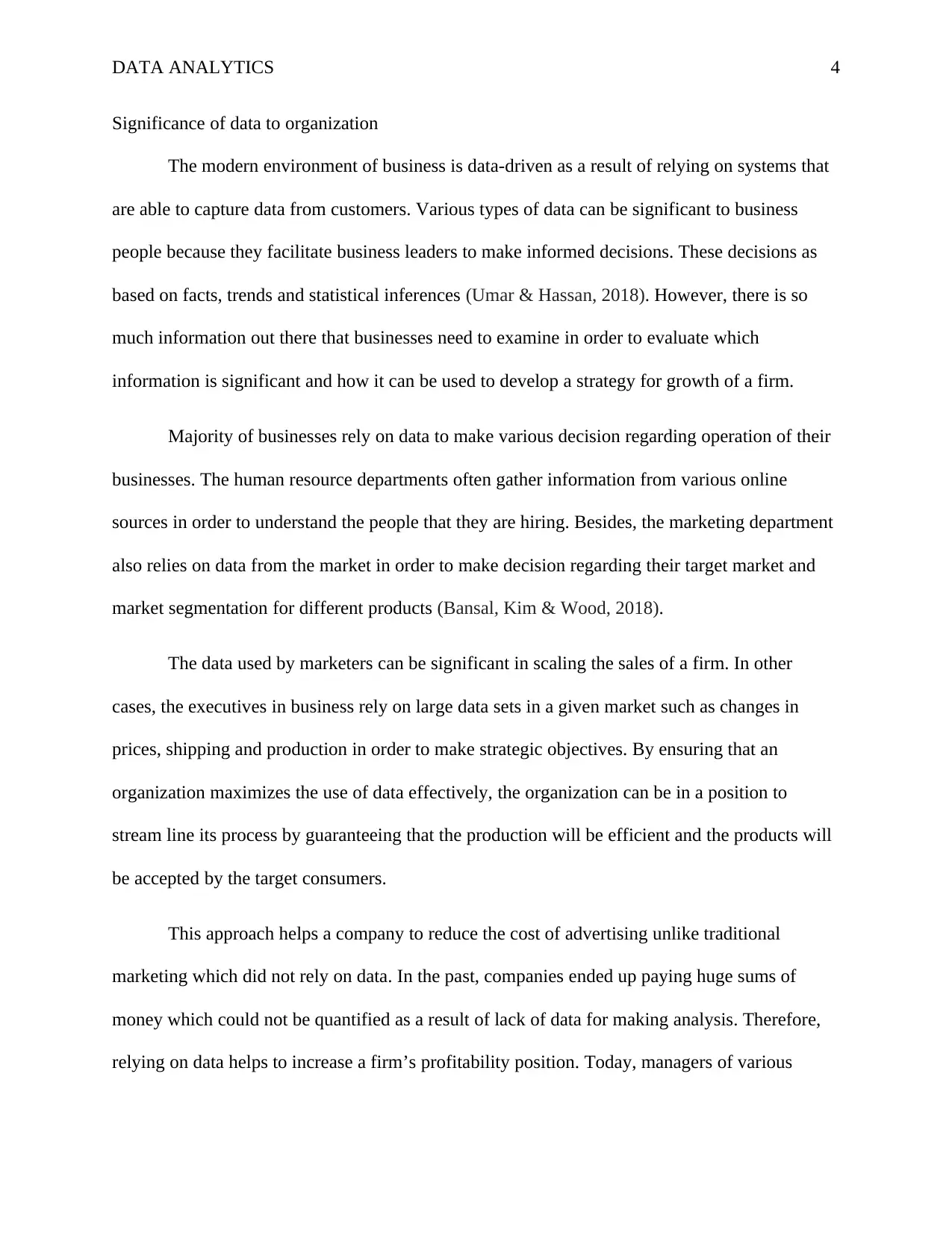
DATA ANALYTICS 4
Significance of data to organization
The modern environment of business is data-driven as a result of relying on systems that
are able to capture data from customers. Various types of data can be significant to business
people because they facilitate business leaders to make informed decisions. These decisions as
based on facts, trends and statistical inferences (Umar & Hassan, 2018). However, there is so
much information out there that businesses need to examine in order to evaluate which
information is significant and how it can be used to develop a strategy for growth of a firm.
Majority of businesses rely on data to make various decision regarding operation of their
businesses. The human resource departments often gather information from various online
sources in order to understand the people that they are hiring. Besides, the marketing department
also relies on data from the market in order to make decision regarding their target market and
market segmentation for different products (Bansal, Kim & Wood, 2018).
The data used by marketers can be significant in scaling the sales of a firm. In other
cases, the executives in business rely on large data sets in a given market such as changes in
prices, shipping and production in order to make strategic objectives. By ensuring that an
organization maximizes the use of data effectively, the organization can be in a position to
stream line its process by guaranteeing that the production will be efficient and the products will
be accepted by the target consumers.
This approach helps a company to reduce the cost of advertising unlike traditional
marketing which did not rely on data. In the past, companies ended up paying huge sums of
money which could not be quantified as a result of lack of data for making analysis. Therefore,
relying on data helps to increase a firm’s profitability position. Today, managers of various
Significance of data to organization
The modern environment of business is data-driven as a result of relying on systems that
are able to capture data from customers. Various types of data can be significant to business
people because they facilitate business leaders to make informed decisions. These decisions as
based on facts, trends and statistical inferences (Umar & Hassan, 2018). However, there is so
much information out there that businesses need to examine in order to evaluate which
information is significant and how it can be used to develop a strategy for growth of a firm.
Majority of businesses rely on data to make various decision regarding operation of their
businesses. The human resource departments often gather information from various online
sources in order to understand the people that they are hiring. Besides, the marketing department
also relies on data from the market in order to make decision regarding their target market and
market segmentation for different products (Bansal, Kim & Wood, 2018).
The data used by marketers can be significant in scaling the sales of a firm. In other
cases, the executives in business rely on large data sets in a given market such as changes in
prices, shipping and production in order to make strategic objectives. By ensuring that an
organization maximizes the use of data effectively, the organization can be in a position to
stream line its process by guaranteeing that the production will be efficient and the products will
be accepted by the target consumers.
This approach helps a company to reduce the cost of advertising unlike traditional
marketing which did not rely on data. In the past, companies ended up paying huge sums of
money which could not be quantified as a result of lack of data for making analysis. Therefore,
relying on data helps to increase a firm’s profitability position. Today, managers of various
Secure Best Marks with AI Grader
Need help grading? Try our AI Grader for instant feedback on your assignments.
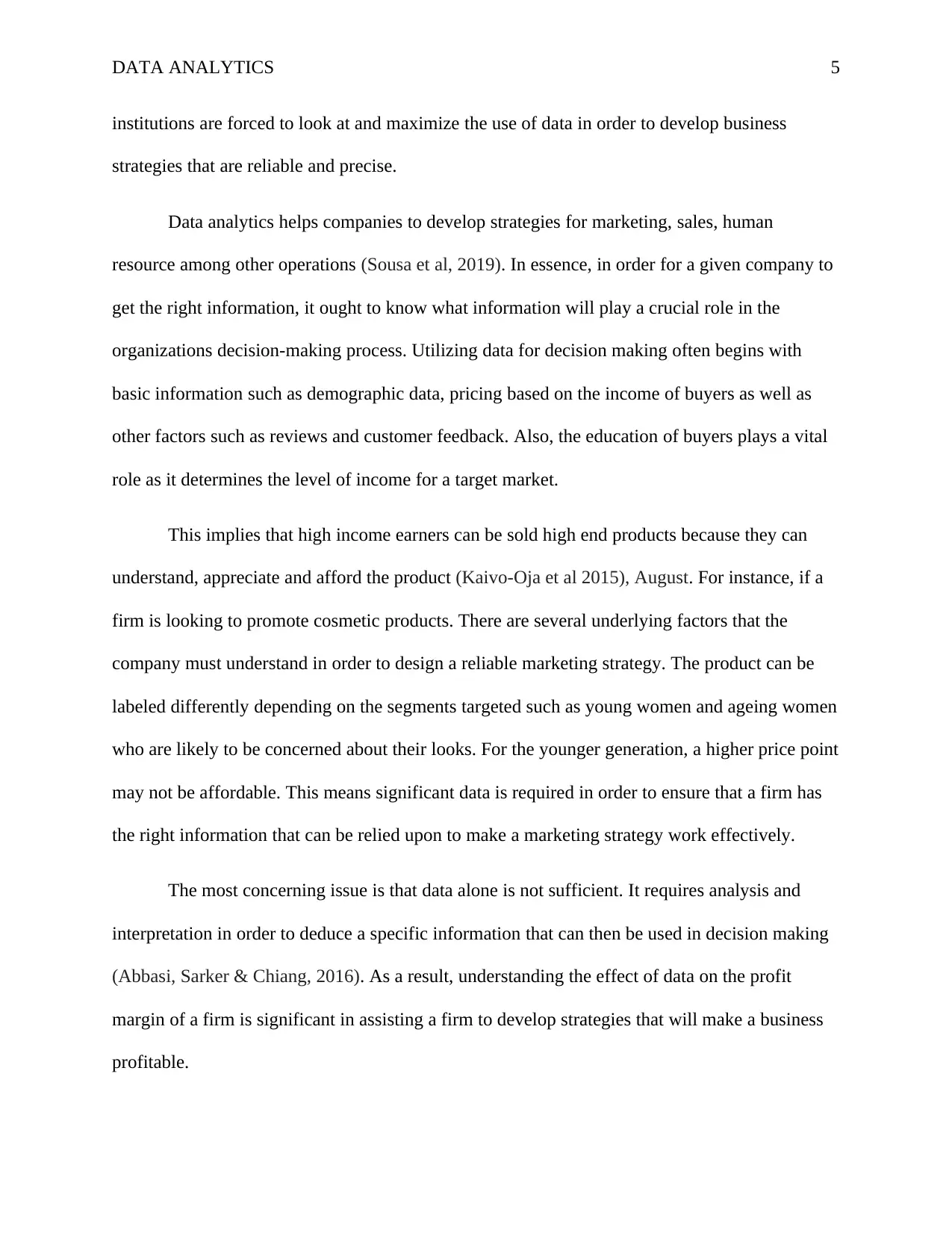
DATA ANALYTICS 5
institutions are forced to look at and maximize the use of data in order to develop business
strategies that are reliable and precise.
Data analytics helps companies to develop strategies for marketing, sales, human
resource among other operations (Sousa et al, 2019). In essence, in order for a given company to
get the right information, it ought to know what information will play a crucial role in the
organizations decision-making process. Utilizing data for decision making often begins with
basic information such as demographic data, pricing based on the income of buyers as well as
other factors such as reviews and customer feedback. Also, the education of buyers plays a vital
role as it determines the level of income for a target market.
This implies that high income earners can be sold high end products because they can
understand, appreciate and afford the product (Kaivo-Oja et al 2015), August. For instance, if a
firm is looking to promote cosmetic products. There are several underlying factors that the
company must understand in order to design a reliable marketing strategy. The product can be
labeled differently depending on the segments targeted such as young women and ageing women
who are likely to be concerned about their looks. For the younger generation, a higher price point
may not be affordable. This means significant data is required in order to ensure that a firm has
the right information that can be relied upon to make a marketing strategy work effectively.
The most concerning issue is that data alone is not sufficient. It requires analysis and
interpretation in order to deduce a specific information that can then be used in decision making
(Abbasi, Sarker & Chiang, 2016). As a result, understanding the effect of data on the profit
margin of a firm is significant in assisting a firm to develop strategies that will make a business
profitable.
institutions are forced to look at and maximize the use of data in order to develop business
strategies that are reliable and precise.
Data analytics helps companies to develop strategies for marketing, sales, human
resource among other operations (Sousa et al, 2019). In essence, in order for a given company to
get the right information, it ought to know what information will play a crucial role in the
organizations decision-making process. Utilizing data for decision making often begins with
basic information such as demographic data, pricing based on the income of buyers as well as
other factors such as reviews and customer feedback. Also, the education of buyers plays a vital
role as it determines the level of income for a target market.
This implies that high income earners can be sold high end products because they can
understand, appreciate and afford the product (Kaivo-Oja et al 2015), August. For instance, if a
firm is looking to promote cosmetic products. There are several underlying factors that the
company must understand in order to design a reliable marketing strategy. The product can be
labeled differently depending on the segments targeted such as young women and ageing women
who are likely to be concerned about their looks. For the younger generation, a higher price point
may not be affordable. This means significant data is required in order to ensure that a firm has
the right information that can be relied upon to make a marketing strategy work effectively.
The most concerning issue is that data alone is not sufficient. It requires analysis and
interpretation in order to deduce a specific information that can then be used in decision making
(Abbasi, Sarker & Chiang, 2016). As a result, understanding the effect of data on the profit
margin of a firm is significant in assisting a firm to develop strategies that will make a business
profitable.
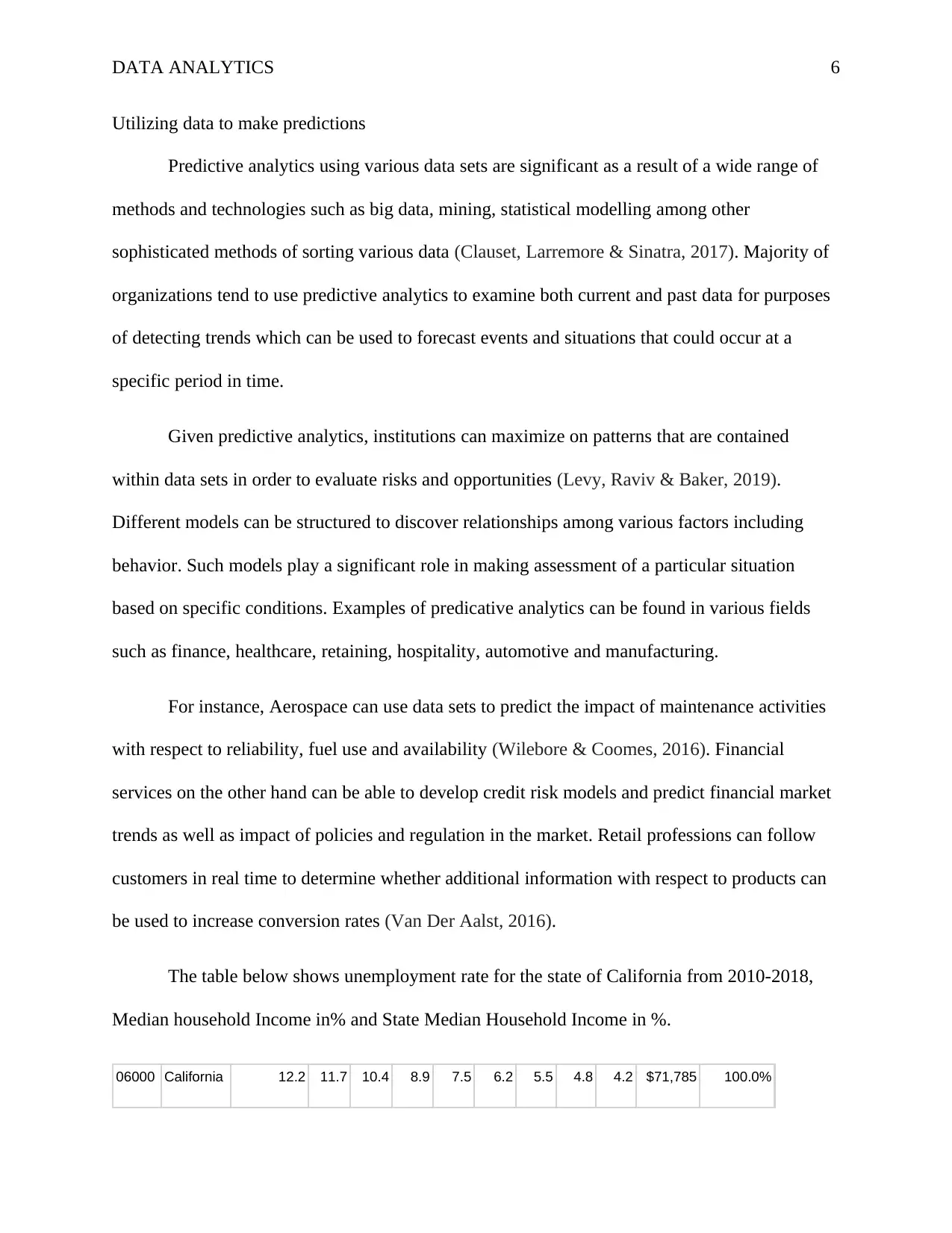
DATA ANALYTICS 6
Utilizing data to make predictions
Predictive analytics using various data sets are significant as a result of a wide range of
methods and technologies such as big data, mining, statistical modelling among other
sophisticated methods of sorting various data (Clauset, Larremore & Sinatra, 2017). Majority of
organizations tend to use predictive analytics to examine both current and past data for purposes
of detecting trends which can be used to forecast events and situations that could occur at a
specific period in time.
Given predictive analytics, institutions can maximize on patterns that are contained
within data sets in order to evaluate risks and opportunities (Levy, Raviv & Baker, 2019).
Different models can be structured to discover relationships among various factors including
behavior. Such models play a significant role in making assessment of a particular situation
based on specific conditions. Examples of predicative analytics can be found in various fields
such as finance, healthcare, retaining, hospitality, automotive and manufacturing.
For instance, Aerospace can use data sets to predict the impact of maintenance activities
with respect to reliability, fuel use and availability (Wilebore & Coomes, 2016). Financial
services on the other hand can be able to develop credit risk models and predict financial market
trends as well as impact of policies and regulation in the market. Retail professions can follow
customers in real time to determine whether additional information with respect to products can
be used to increase conversion rates (Van Der Aalst, 2016).
The table below shows unemployment rate for the state of California from 2010-2018,
Median household Income in% and State Median Household Income in %.
06000 California 12.2 11.7 10.4 8.9 7.5 6.2 5.5 4.8 4.2 $71,785 100.0%
Utilizing data to make predictions
Predictive analytics using various data sets are significant as a result of a wide range of
methods and technologies such as big data, mining, statistical modelling among other
sophisticated methods of sorting various data (Clauset, Larremore & Sinatra, 2017). Majority of
organizations tend to use predictive analytics to examine both current and past data for purposes
of detecting trends which can be used to forecast events and situations that could occur at a
specific period in time.
Given predictive analytics, institutions can maximize on patterns that are contained
within data sets in order to evaluate risks and opportunities (Levy, Raviv & Baker, 2019).
Different models can be structured to discover relationships among various factors including
behavior. Such models play a significant role in making assessment of a particular situation
based on specific conditions. Examples of predicative analytics can be found in various fields
such as finance, healthcare, retaining, hospitality, automotive and manufacturing.
For instance, Aerospace can use data sets to predict the impact of maintenance activities
with respect to reliability, fuel use and availability (Wilebore & Coomes, 2016). Financial
services on the other hand can be able to develop credit risk models and predict financial market
trends as well as impact of policies and regulation in the market. Retail professions can follow
customers in real time to determine whether additional information with respect to products can
be used to increase conversion rates (Van Der Aalst, 2016).
The table below shows unemployment rate for the state of California from 2010-2018,
Median household Income in% and State Median Household Income in %.
06000 California 12.2 11.7 10.4 8.9 7.5 6.2 5.5 4.8 4.2 $71,785 100.0%
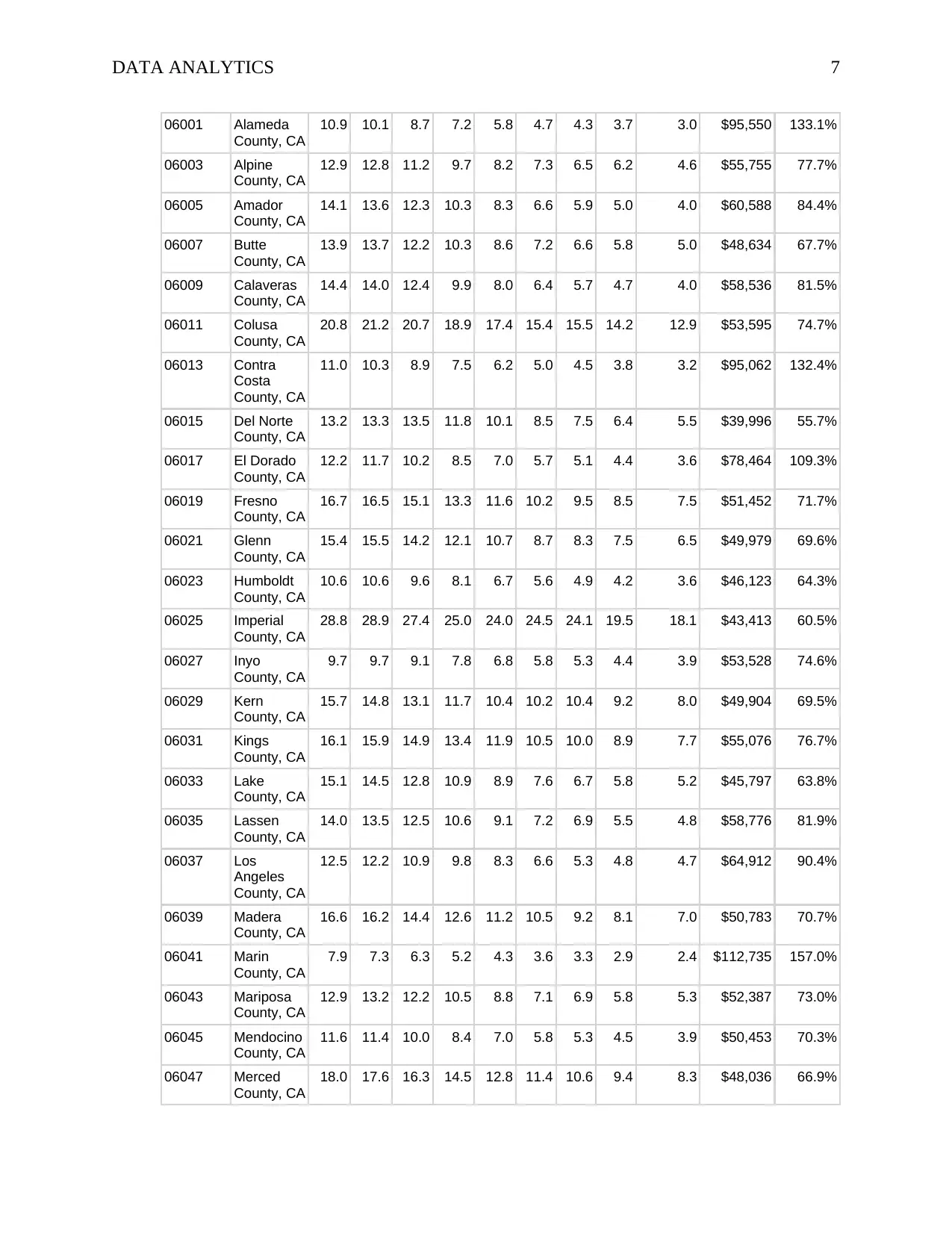
DATA ANALYTICS 7
06001 Alameda
County, CA
10.9 10.1 8.7 7.2 5.8 4.7 4.3 3.7 3.0 $95,550 133.1%
06003 Alpine
County, CA
12.9 12.8 11.2 9.7 8.2 7.3 6.5 6.2 4.6 $55,755 77.7%
06005 Amador
County, CA
14.1 13.6 12.3 10.3 8.3 6.6 5.9 5.0 4.0 $60,588 84.4%
06007 Butte
County, CA
13.9 13.7 12.2 10.3 8.6 7.2 6.6 5.8 5.0 $48,634 67.7%
06009 Calaveras
County, CA
14.4 14.0 12.4 9.9 8.0 6.4 5.7 4.7 4.0 $58,536 81.5%
06011 Colusa
County, CA
20.8 21.2 20.7 18.9 17.4 15.4 15.5 14.2 12.9 $53,595 74.7%
06013 Contra
Costa
County, CA
11.0 10.3 8.9 7.5 6.2 5.0 4.5 3.8 3.2 $95,062 132.4%
06015 Del Norte
County, CA
13.2 13.3 13.5 11.8 10.1 8.5 7.5 6.4 5.5 $39,996 55.7%
06017 El Dorado
County, CA
12.2 11.7 10.2 8.5 7.0 5.7 5.1 4.4 3.6 $78,464 109.3%
06019 Fresno
County, CA
16.7 16.5 15.1 13.3 11.6 10.2 9.5 8.5 7.5 $51,452 71.7%
06021 Glenn
County, CA
15.4 15.5 14.2 12.1 10.7 8.7 8.3 7.5 6.5 $49,979 69.6%
06023 Humboldt
County, CA
10.6 10.6 9.6 8.1 6.7 5.6 4.9 4.2 3.6 $46,123 64.3%
06025 Imperial
County, CA
28.8 28.9 27.4 25.0 24.0 24.5 24.1 19.5 18.1 $43,413 60.5%
06027 Inyo
County, CA
9.7 9.7 9.1 7.8 6.8 5.8 5.3 4.4 3.9 $53,528 74.6%
06029 Kern
County, CA
15.7 14.8 13.1 11.7 10.4 10.2 10.4 9.2 8.0 $49,904 69.5%
06031 Kings
County, CA
16.1 15.9 14.9 13.4 11.9 10.5 10.0 8.9 7.7 $55,076 76.7%
06033 Lake
County, CA
15.1 14.5 12.8 10.9 8.9 7.6 6.7 5.8 5.2 $45,797 63.8%
06035 Lassen
County, CA
14.0 13.5 12.5 10.6 9.1 7.2 6.9 5.5 4.8 $58,776 81.9%
06037 Los
Angeles
County, CA
12.5 12.2 10.9 9.8 8.3 6.6 5.3 4.8 4.7 $64,912 90.4%
06039 Madera
County, CA
16.6 16.2 14.4 12.6 11.2 10.5 9.2 8.1 7.0 $50,783 70.7%
06041 Marin
County, CA
7.9 7.3 6.3 5.2 4.3 3.6 3.3 2.9 2.4 $112,735 157.0%
06043 Mariposa
County, CA
12.9 13.2 12.2 10.5 8.8 7.1 6.9 5.8 5.3 $52,387 73.0%
06045 Mendocino
County, CA
11.6 11.4 10.0 8.4 7.0 5.8 5.3 4.5 3.9 $50,453 70.3%
06047 Merced
County, CA
18.0 17.6 16.3 14.5 12.8 11.4 10.6 9.4 8.3 $48,036 66.9%
06001 Alameda
County, CA
10.9 10.1 8.7 7.2 5.8 4.7 4.3 3.7 3.0 $95,550 133.1%
06003 Alpine
County, CA
12.9 12.8 11.2 9.7 8.2 7.3 6.5 6.2 4.6 $55,755 77.7%
06005 Amador
County, CA
14.1 13.6 12.3 10.3 8.3 6.6 5.9 5.0 4.0 $60,588 84.4%
06007 Butte
County, CA
13.9 13.7 12.2 10.3 8.6 7.2 6.6 5.8 5.0 $48,634 67.7%
06009 Calaveras
County, CA
14.4 14.0 12.4 9.9 8.0 6.4 5.7 4.7 4.0 $58,536 81.5%
06011 Colusa
County, CA
20.8 21.2 20.7 18.9 17.4 15.4 15.5 14.2 12.9 $53,595 74.7%
06013 Contra
Costa
County, CA
11.0 10.3 8.9 7.5 6.2 5.0 4.5 3.8 3.2 $95,062 132.4%
06015 Del Norte
County, CA
13.2 13.3 13.5 11.8 10.1 8.5 7.5 6.4 5.5 $39,996 55.7%
06017 El Dorado
County, CA
12.2 11.7 10.2 8.5 7.0 5.7 5.1 4.4 3.6 $78,464 109.3%
06019 Fresno
County, CA
16.7 16.5 15.1 13.3 11.6 10.2 9.5 8.5 7.5 $51,452 71.7%
06021 Glenn
County, CA
15.4 15.5 14.2 12.1 10.7 8.7 8.3 7.5 6.5 $49,979 69.6%
06023 Humboldt
County, CA
10.6 10.6 9.6 8.1 6.7 5.6 4.9 4.2 3.6 $46,123 64.3%
06025 Imperial
County, CA
28.8 28.9 27.4 25.0 24.0 24.5 24.1 19.5 18.1 $43,413 60.5%
06027 Inyo
County, CA
9.7 9.7 9.1 7.8 6.8 5.8 5.3 4.4 3.9 $53,528 74.6%
06029 Kern
County, CA
15.7 14.8 13.1 11.7 10.4 10.2 10.4 9.2 8.0 $49,904 69.5%
06031 Kings
County, CA
16.1 15.9 14.9 13.4 11.9 10.5 10.0 8.9 7.7 $55,076 76.7%
06033 Lake
County, CA
15.1 14.5 12.8 10.9 8.9 7.6 6.7 5.8 5.2 $45,797 63.8%
06035 Lassen
County, CA
14.0 13.5 12.5 10.6 9.1 7.2 6.9 5.5 4.8 $58,776 81.9%
06037 Los
Angeles
County, CA
12.5 12.2 10.9 9.8 8.3 6.6 5.3 4.8 4.7 $64,912 90.4%
06039 Madera
County, CA
16.6 16.2 14.4 12.6 11.2 10.5 9.2 8.1 7.0 $50,783 70.7%
06041 Marin
County, CA
7.9 7.3 6.3 5.2 4.3 3.6 3.3 2.9 2.4 $112,735 157.0%
06043 Mariposa
County, CA
12.9 13.2 12.2 10.5 8.8 7.1 6.9 5.8 5.3 $52,387 73.0%
06045 Mendocino
County, CA
11.6 11.4 10.0 8.4 7.0 5.8 5.3 4.5 3.9 $50,453 70.3%
06047 Merced
County, CA
18.0 17.6 16.3 14.5 12.8 11.4 10.6 9.4 8.3 $48,036 66.9%
Paraphrase This Document
Need a fresh take? Get an instant paraphrase of this document with our AI Paraphraser
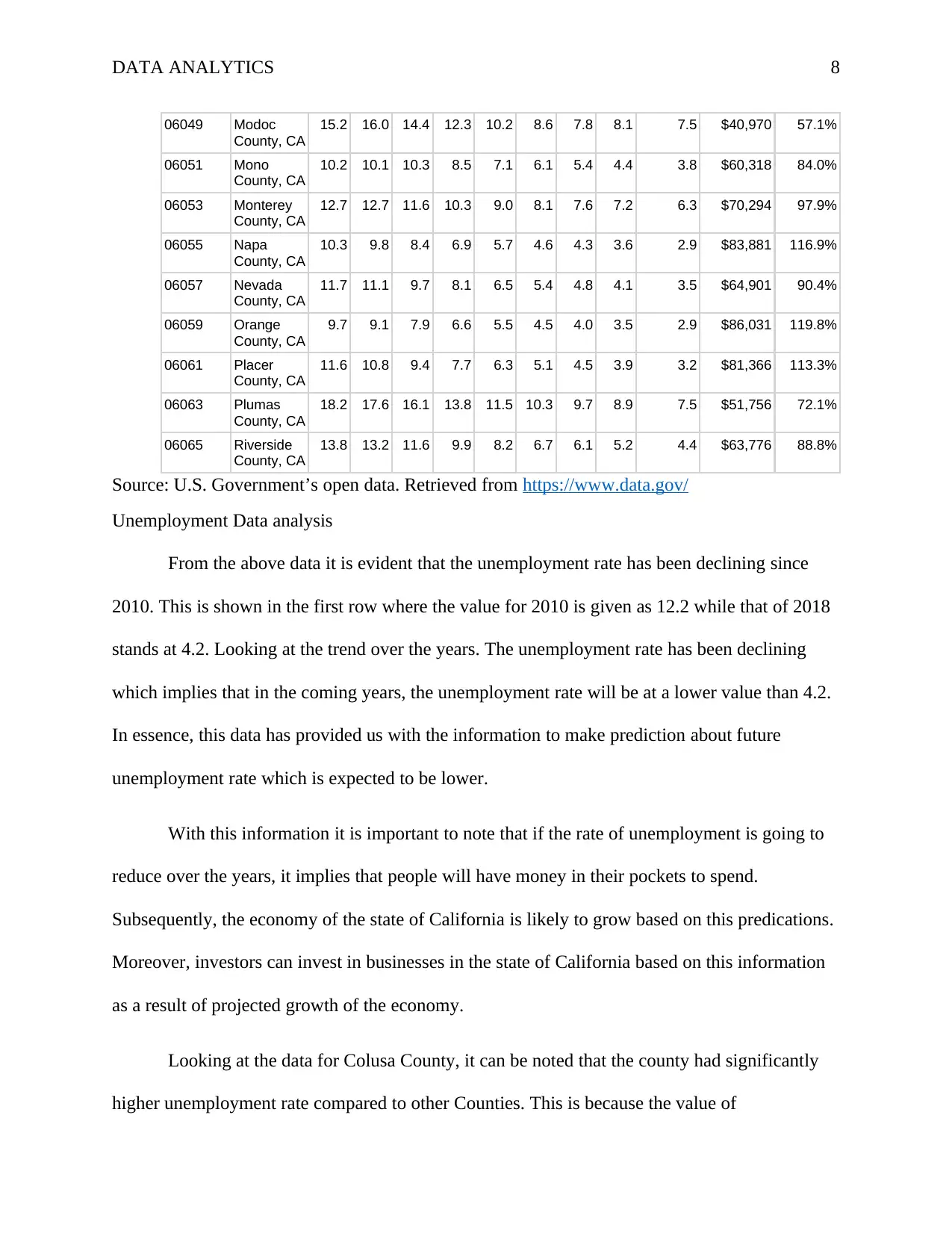
DATA ANALYTICS 8
06049 Modoc
County, CA
15.2 16.0 14.4 12.3 10.2 8.6 7.8 8.1 7.5 $40,970 57.1%
06051 Mono
County, CA
10.2 10.1 10.3 8.5 7.1 6.1 5.4 4.4 3.8 $60,318 84.0%
06053 Monterey
County, CA
12.7 12.7 11.6 10.3 9.0 8.1 7.6 7.2 6.3 $70,294 97.9%
06055 Napa
County, CA
10.3 9.8 8.4 6.9 5.7 4.6 4.3 3.6 2.9 $83,881 116.9%
06057 Nevada
County, CA
11.7 11.1 9.7 8.1 6.5 5.4 4.8 4.1 3.5 $64,901 90.4%
06059 Orange
County, CA
9.7 9.1 7.9 6.6 5.5 4.5 4.0 3.5 2.9 $86,031 119.8%
06061 Placer
County, CA
11.6 10.8 9.4 7.7 6.3 5.1 4.5 3.9 3.2 $81,366 113.3%
06063 Plumas
County, CA
18.2 17.6 16.1 13.8 11.5 10.3 9.7 8.9 7.5 $51,756 72.1%
06065 Riverside
County, CA
13.8 13.2 11.6 9.9 8.2 6.7 6.1 5.2 4.4 $63,776 88.8%
Source: U.S. Government’s open data. Retrieved from https://www.data.gov/
Unemployment Data analysis
From the above data it is evident that the unemployment rate has been declining since
2010. This is shown in the first row where the value for 2010 is given as 12.2 while that of 2018
stands at 4.2. Looking at the trend over the years. The unemployment rate has been declining
which implies that in the coming years, the unemployment rate will be at a lower value than 4.2.
In essence, this data has provided us with the information to make prediction about future
unemployment rate which is expected to be lower.
With this information it is important to note that if the rate of unemployment is going to
reduce over the years, it implies that people will have money in their pockets to spend.
Subsequently, the economy of the state of California is likely to grow based on this predications.
Moreover, investors can invest in businesses in the state of California based on this information
as a result of projected growth of the economy.
Looking at the data for Colusa County, it can be noted that the county had significantly
higher unemployment rate compared to other Counties. This is because the value of
06049 Modoc
County, CA
15.2 16.0 14.4 12.3 10.2 8.6 7.8 8.1 7.5 $40,970 57.1%
06051 Mono
County, CA
10.2 10.1 10.3 8.5 7.1 6.1 5.4 4.4 3.8 $60,318 84.0%
06053 Monterey
County, CA
12.7 12.7 11.6 10.3 9.0 8.1 7.6 7.2 6.3 $70,294 97.9%
06055 Napa
County, CA
10.3 9.8 8.4 6.9 5.7 4.6 4.3 3.6 2.9 $83,881 116.9%
06057 Nevada
County, CA
11.7 11.1 9.7 8.1 6.5 5.4 4.8 4.1 3.5 $64,901 90.4%
06059 Orange
County, CA
9.7 9.1 7.9 6.6 5.5 4.5 4.0 3.5 2.9 $86,031 119.8%
06061 Placer
County, CA
11.6 10.8 9.4 7.7 6.3 5.1 4.5 3.9 3.2 $81,366 113.3%
06063 Plumas
County, CA
18.2 17.6 16.1 13.8 11.5 10.3 9.7 8.9 7.5 $51,756 72.1%
06065 Riverside
County, CA
13.8 13.2 11.6 9.9 8.2 6.7 6.1 5.2 4.4 $63,776 88.8%
Source: U.S. Government’s open data. Retrieved from https://www.data.gov/
Unemployment Data analysis
From the above data it is evident that the unemployment rate has been declining since
2010. This is shown in the first row where the value for 2010 is given as 12.2 while that of 2018
stands at 4.2. Looking at the trend over the years. The unemployment rate has been declining
which implies that in the coming years, the unemployment rate will be at a lower value than 4.2.
In essence, this data has provided us with the information to make prediction about future
unemployment rate which is expected to be lower.
With this information it is important to note that if the rate of unemployment is going to
reduce over the years, it implies that people will have money in their pockets to spend.
Subsequently, the economy of the state of California is likely to grow based on this predications.
Moreover, investors can invest in businesses in the state of California based on this information
as a result of projected growth of the economy.
Looking at the data for Colusa County, it can be noted that the county had significantly
higher unemployment rate compared to other Counties. This is because the value of
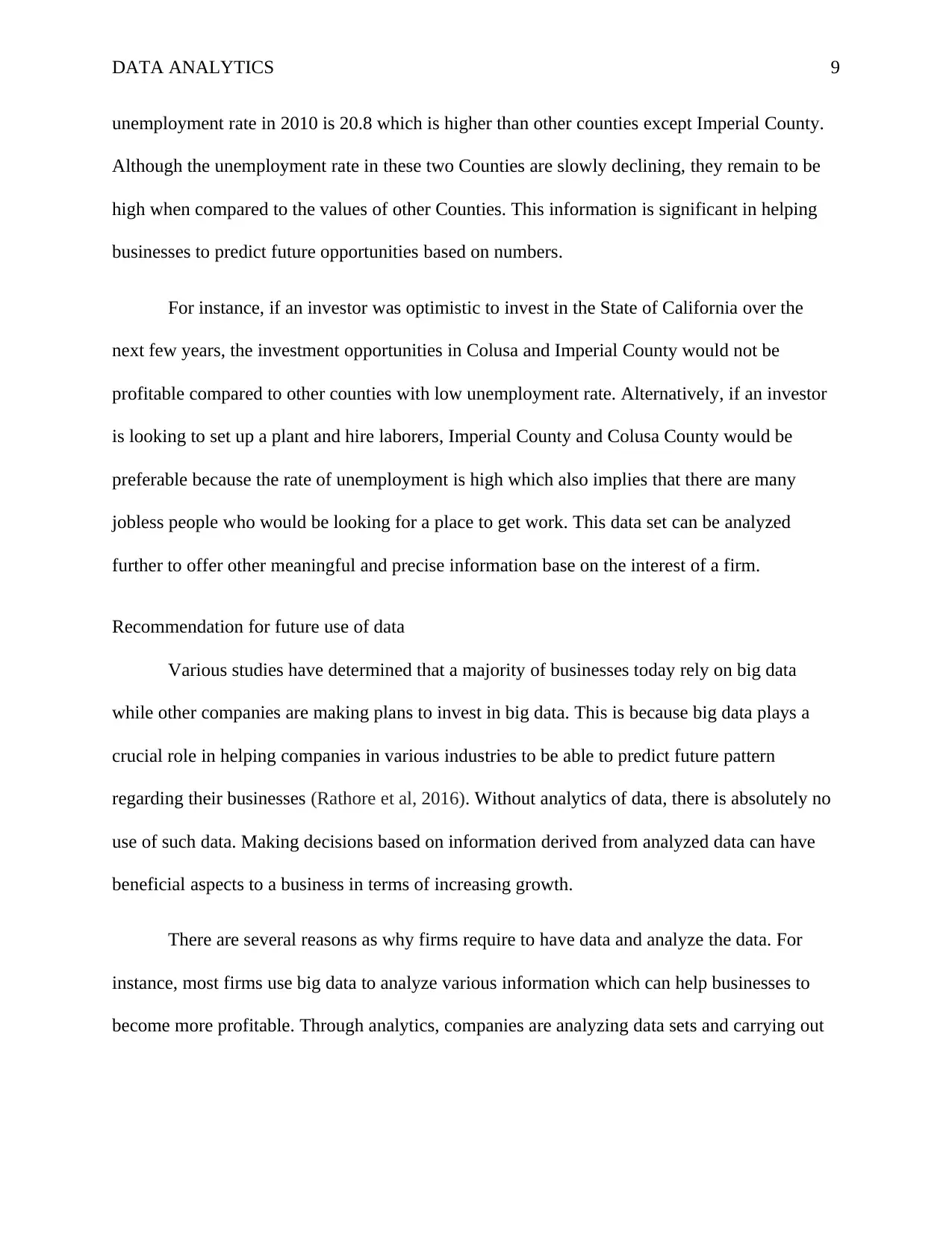
DATA ANALYTICS 9
unemployment rate in 2010 is 20.8 which is higher than other counties except Imperial County.
Although the unemployment rate in these two Counties are slowly declining, they remain to be
high when compared to the values of other Counties. This information is significant in helping
businesses to predict future opportunities based on numbers.
For instance, if an investor was optimistic to invest in the State of California over the
next few years, the investment opportunities in Colusa and Imperial County would not be
profitable compared to other counties with low unemployment rate. Alternatively, if an investor
is looking to set up a plant and hire laborers, Imperial County and Colusa County would be
preferable because the rate of unemployment is high which also implies that there are many
jobless people who would be looking for a place to get work. This data set can be analyzed
further to offer other meaningful and precise information base on the interest of a firm.
Recommendation for future use of data
Various studies have determined that a majority of businesses today rely on big data
while other companies are making plans to invest in big data. This is because big data plays a
crucial role in helping companies in various industries to be able to predict future pattern
regarding their businesses (Rathore et al, 2016). Without analytics of data, there is absolutely no
use of such data. Making decisions based on information derived from analyzed data can have
beneficial aspects to a business in terms of increasing growth.
There are several reasons as why firms require to have data and analyze the data. For
instance, most firms use big data to analyze various information which can help businesses to
become more profitable. Through analytics, companies are analyzing data sets and carrying out
unemployment rate in 2010 is 20.8 which is higher than other counties except Imperial County.
Although the unemployment rate in these two Counties are slowly declining, they remain to be
high when compared to the values of other Counties. This information is significant in helping
businesses to predict future opportunities based on numbers.
For instance, if an investor was optimistic to invest in the State of California over the
next few years, the investment opportunities in Colusa and Imperial County would not be
profitable compared to other counties with low unemployment rate. Alternatively, if an investor
is looking to set up a plant and hire laborers, Imperial County and Colusa County would be
preferable because the rate of unemployment is high which also implies that there are many
jobless people who would be looking for a place to get work. This data set can be analyzed
further to offer other meaningful and precise information base on the interest of a firm.
Recommendation for future use of data
Various studies have determined that a majority of businesses today rely on big data
while other companies are making plans to invest in big data. This is because big data plays a
crucial role in helping companies in various industries to be able to predict future pattern
regarding their businesses (Rathore et al, 2016). Without analytics of data, there is absolutely no
use of such data. Making decisions based on information derived from analyzed data can have
beneficial aspects to a business in terms of increasing growth.
There are several reasons as why firms require to have data and analyze the data. For
instance, most firms use big data to analyze various information which can help businesses to
become more profitable. Through analytics, companies are analyzing data sets and carrying out
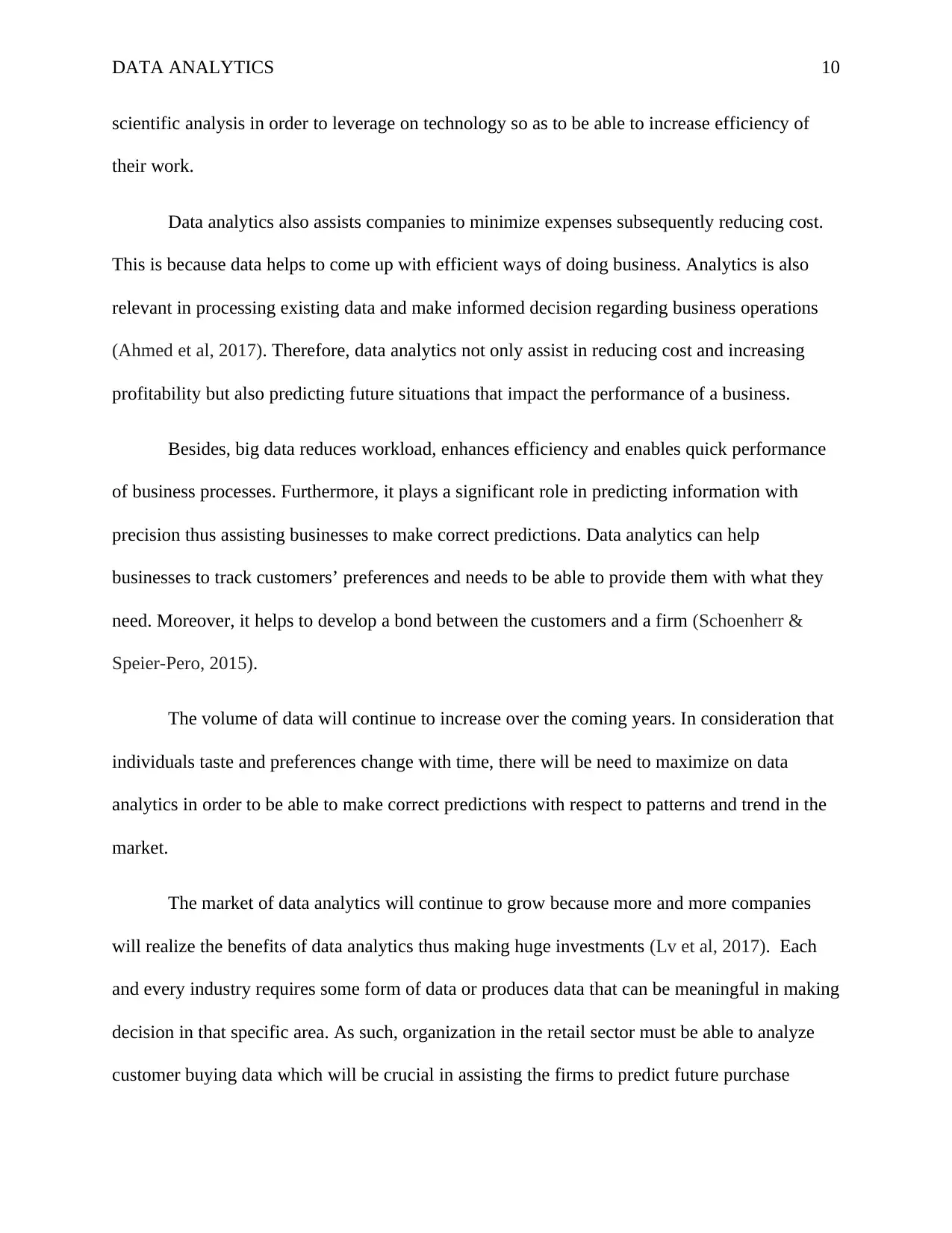
DATA ANALYTICS 10
scientific analysis in order to leverage on technology so as to be able to increase efficiency of
their work.
Data analytics also assists companies to minimize expenses subsequently reducing cost.
This is because data helps to come up with efficient ways of doing business. Analytics is also
relevant in processing existing data and make informed decision regarding business operations
(Ahmed et al, 2017). Therefore, data analytics not only assist in reducing cost and increasing
profitability but also predicting future situations that impact the performance of a business.
Besides, big data reduces workload, enhances efficiency and enables quick performance
of business processes. Furthermore, it plays a significant role in predicting information with
precision thus assisting businesses to make correct predictions. Data analytics can help
businesses to track customers’ preferences and needs to be able to provide them with what they
need. Moreover, it helps to develop a bond between the customers and a firm (Schoenherr &
Speier‐Pero, 2015).
The volume of data will continue to increase over the coming years. In consideration that
individuals taste and preferences change with time, there will be need to maximize on data
analytics in order to be able to make correct predictions with respect to patterns and trend in the
market.
The market of data analytics will continue to grow because more and more companies
will realize the benefits of data analytics thus making huge investments (Lv et al, 2017). Each
and every industry requires some form of data or produces data that can be meaningful in making
decision in that specific area. As such, organization in the retail sector must be able to analyze
customer buying data which will be crucial in assisting the firms to predict future purchase
scientific analysis in order to leverage on technology so as to be able to increase efficiency of
their work.
Data analytics also assists companies to minimize expenses subsequently reducing cost.
This is because data helps to come up with efficient ways of doing business. Analytics is also
relevant in processing existing data and make informed decision regarding business operations
(Ahmed et al, 2017). Therefore, data analytics not only assist in reducing cost and increasing
profitability but also predicting future situations that impact the performance of a business.
Besides, big data reduces workload, enhances efficiency and enables quick performance
of business processes. Furthermore, it plays a significant role in predicting information with
precision thus assisting businesses to make correct predictions. Data analytics can help
businesses to track customers’ preferences and needs to be able to provide them with what they
need. Moreover, it helps to develop a bond between the customers and a firm (Schoenherr &
Speier‐Pero, 2015).
The volume of data will continue to increase over the coming years. In consideration that
individuals taste and preferences change with time, there will be need to maximize on data
analytics in order to be able to make correct predictions with respect to patterns and trend in the
market.
The market of data analytics will continue to grow because more and more companies
will realize the benefits of data analytics thus making huge investments (Lv et al, 2017). Each
and every industry requires some form of data or produces data that can be meaningful in making
decision in that specific area. As such, organization in the retail sector must be able to analyze
customer buying data which will be crucial in assisting the firms to predict future purchase
Secure Best Marks with AI Grader
Need help grading? Try our AI Grader for instant feedback on your assignments.
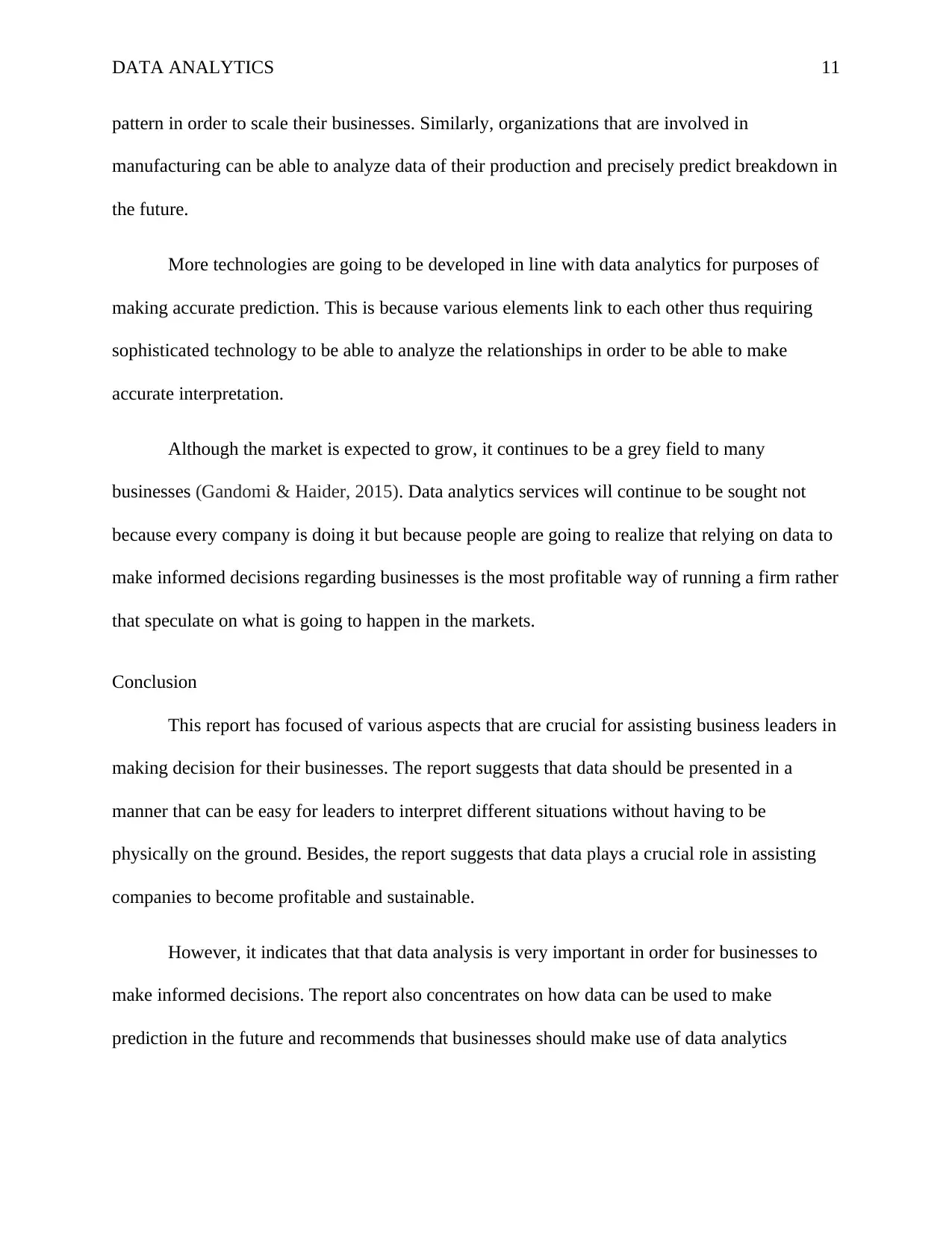
DATA ANALYTICS 11
pattern in order to scale their businesses. Similarly, organizations that are involved in
manufacturing can be able to analyze data of their production and precisely predict breakdown in
the future.
More technologies are going to be developed in line with data analytics for purposes of
making accurate prediction. This is because various elements link to each other thus requiring
sophisticated technology to be able to analyze the relationships in order to be able to make
accurate interpretation.
Although the market is expected to grow, it continues to be a grey field to many
businesses (Gandomi & Haider, 2015). Data analytics services will continue to be sought not
because every company is doing it but because people are going to realize that relying on data to
make informed decisions regarding businesses is the most profitable way of running a firm rather
that speculate on what is going to happen in the markets.
Conclusion
This report has focused of various aspects that are crucial for assisting business leaders in
making decision for their businesses. The report suggests that data should be presented in a
manner that can be easy for leaders to interpret different situations without having to be
physically on the ground. Besides, the report suggests that data plays a crucial role in assisting
companies to become profitable and sustainable.
However, it indicates that that data analysis is very important in order for businesses to
make informed decisions. The report also concentrates on how data can be used to make
prediction in the future and recommends that businesses should make use of data analytics
pattern in order to scale their businesses. Similarly, organizations that are involved in
manufacturing can be able to analyze data of their production and precisely predict breakdown in
the future.
More technologies are going to be developed in line with data analytics for purposes of
making accurate prediction. This is because various elements link to each other thus requiring
sophisticated technology to be able to analyze the relationships in order to be able to make
accurate interpretation.
Although the market is expected to grow, it continues to be a grey field to many
businesses (Gandomi & Haider, 2015). Data analytics services will continue to be sought not
because every company is doing it but because people are going to realize that relying on data to
make informed decisions regarding businesses is the most profitable way of running a firm rather
that speculate on what is going to happen in the markets.
Conclusion
This report has focused of various aspects that are crucial for assisting business leaders in
making decision for their businesses. The report suggests that data should be presented in a
manner that can be easy for leaders to interpret different situations without having to be
physically on the ground. Besides, the report suggests that data plays a crucial role in assisting
companies to become profitable and sustainable.
However, it indicates that that data analysis is very important in order for businesses to
make informed decisions. The report also concentrates on how data can be used to make
prediction in the future and recommends that businesses should make use of data analytics
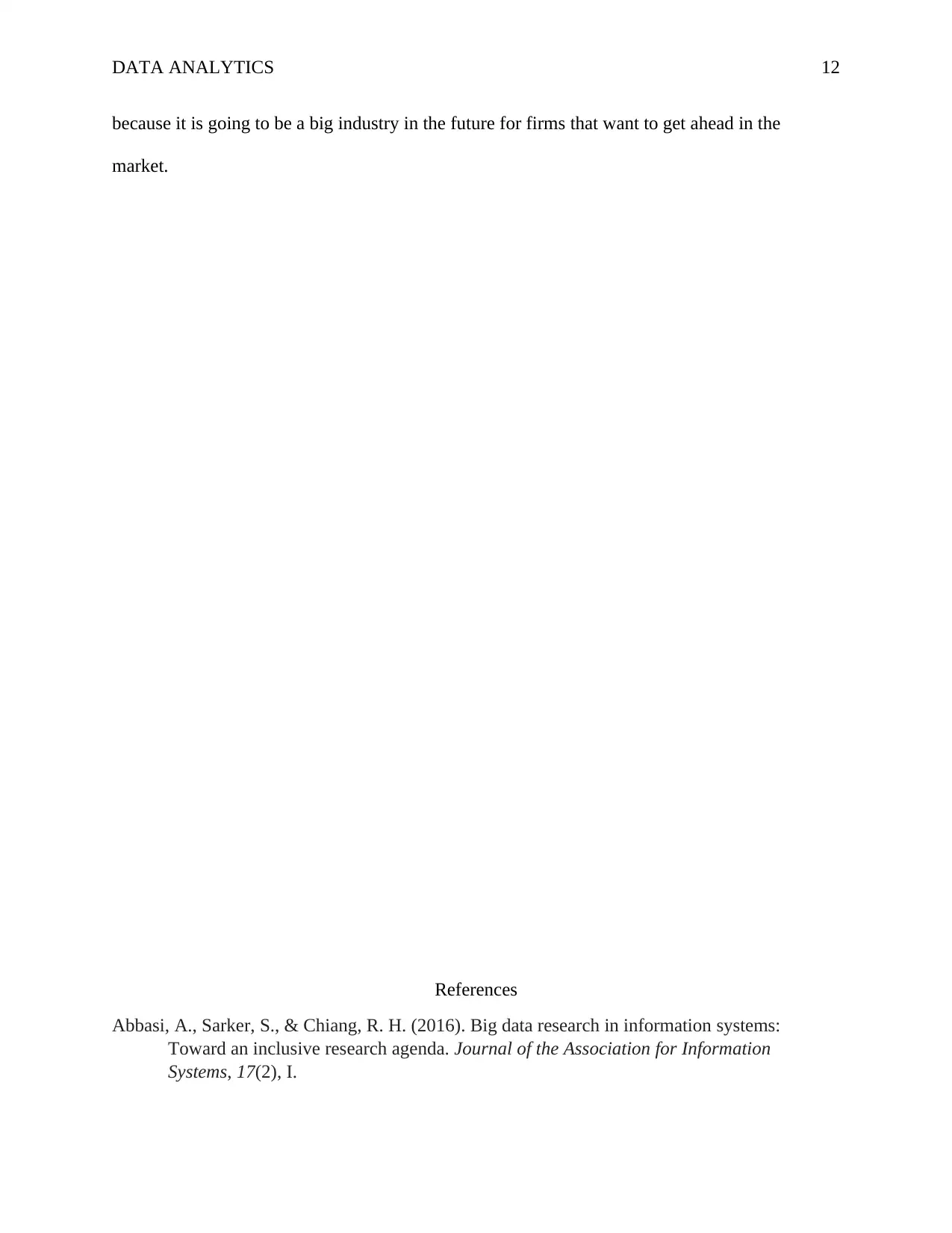
DATA ANALYTICS 12
because it is going to be a big industry in the future for firms that want to get ahead in the
market.
References
Abbasi, A., Sarker, S., & Chiang, R. H. (2016). Big data research in information systems:
Toward an inclusive research agenda. Journal of the Association for Information
Systems, 17(2), I.
because it is going to be a big industry in the future for firms that want to get ahead in the
market.
References
Abbasi, A., Sarker, S., & Chiang, R. H. (2016). Big data research in information systems:
Toward an inclusive research agenda. Journal of the Association for Information
Systems, 17(2), I.
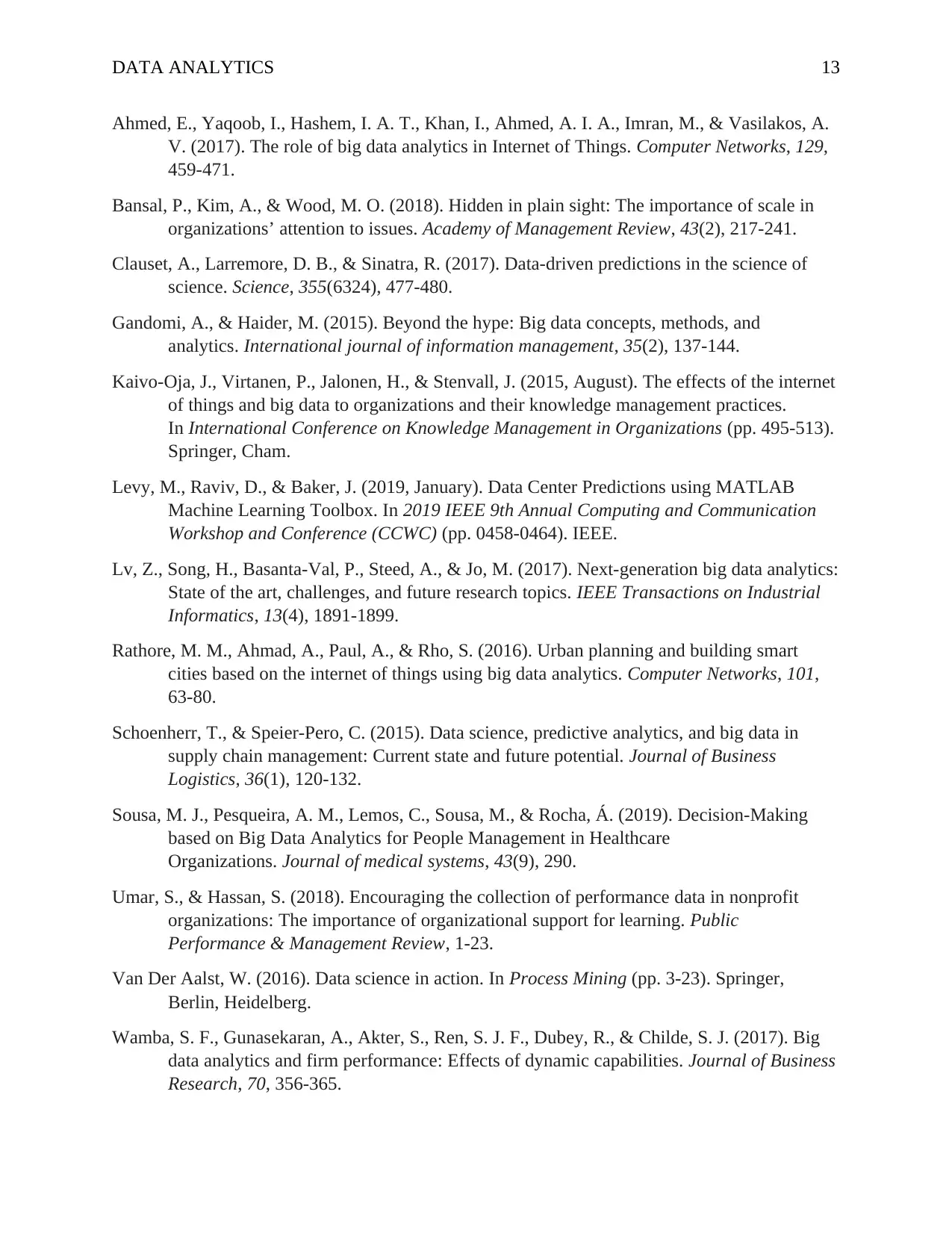
DATA ANALYTICS 13
Ahmed, E., Yaqoob, I., Hashem, I. A. T., Khan, I., Ahmed, A. I. A., Imran, M., & Vasilakos, A.
V. (2017). The role of big data analytics in Internet of Things. Computer Networks, 129,
459-471.
Bansal, P., Kim, A., & Wood, M. O. (2018). Hidden in plain sight: The importance of scale in
organizations’ attention to issues. Academy of Management Review, 43(2), 217-241.
Clauset, A., Larremore, D. B., & Sinatra, R. (2017). Data-driven predictions in the science of
science. Science, 355(6324), 477-480.
Gandomi, A., & Haider, M. (2015). Beyond the hype: Big data concepts, methods, and
analytics. International journal of information management, 35(2), 137-144.
Kaivo-Oja, J., Virtanen, P., Jalonen, H., & Stenvall, J. (2015, August). The effects of the internet
of things and big data to organizations and their knowledge management practices.
In International Conference on Knowledge Management in Organizations (pp. 495-513).
Springer, Cham.
Levy, M., Raviv, D., & Baker, J. (2019, January). Data Center Predictions using MATLAB
Machine Learning Toolbox. In 2019 IEEE 9th Annual Computing and Communication
Workshop and Conference (CCWC) (pp. 0458-0464). IEEE.
Lv, Z., Song, H., Basanta-Val, P., Steed, A., & Jo, M. (2017). Next-generation big data analytics:
State of the art, challenges, and future research topics. IEEE Transactions on Industrial
Informatics, 13(4), 1891-1899.
Rathore, M. M., Ahmad, A., Paul, A., & Rho, S. (2016). Urban planning and building smart
cities based on the internet of things using big data analytics. Computer Networks, 101,
63-80.
Schoenherr, T., & Speier‐Pero, C. (2015). Data science, predictive analytics, and big data in
supply chain management: Current state and future potential. Journal of Business
Logistics, 36(1), 120-132.
Sousa, M. J., Pesqueira, A. M., Lemos, C., Sousa, M., & Rocha, Á. (2019). Decision-Making
based on Big Data Analytics for People Management in Healthcare
Organizations. Journal of medical systems, 43(9), 290.
Umar, S., & Hassan, S. (2018). Encouraging the collection of performance data in nonprofit
organizations: The importance of organizational support for learning. Public
Performance & Management Review, 1-23.
Van Der Aalst, W. (2016). Data science in action. In Process Mining (pp. 3-23). Springer,
Berlin, Heidelberg.
Wamba, S. F., Gunasekaran, A., Akter, S., Ren, S. J. F., Dubey, R., & Childe, S. J. (2017). Big
data analytics and firm performance: Effects of dynamic capabilities. Journal of Business
Research, 70, 356-365.
Ahmed, E., Yaqoob, I., Hashem, I. A. T., Khan, I., Ahmed, A. I. A., Imran, M., & Vasilakos, A.
V. (2017). The role of big data analytics in Internet of Things. Computer Networks, 129,
459-471.
Bansal, P., Kim, A., & Wood, M. O. (2018). Hidden in plain sight: The importance of scale in
organizations’ attention to issues. Academy of Management Review, 43(2), 217-241.
Clauset, A., Larremore, D. B., & Sinatra, R. (2017). Data-driven predictions in the science of
science. Science, 355(6324), 477-480.
Gandomi, A., & Haider, M. (2015). Beyond the hype: Big data concepts, methods, and
analytics. International journal of information management, 35(2), 137-144.
Kaivo-Oja, J., Virtanen, P., Jalonen, H., & Stenvall, J. (2015, August). The effects of the internet
of things and big data to organizations and their knowledge management practices.
In International Conference on Knowledge Management in Organizations (pp. 495-513).
Springer, Cham.
Levy, M., Raviv, D., & Baker, J. (2019, January). Data Center Predictions using MATLAB
Machine Learning Toolbox. In 2019 IEEE 9th Annual Computing and Communication
Workshop and Conference (CCWC) (pp. 0458-0464). IEEE.
Lv, Z., Song, H., Basanta-Val, P., Steed, A., & Jo, M. (2017). Next-generation big data analytics:
State of the art, challenges, and future research topics. IEEE Transactions on Industrial
Informatics, 13(4), 1891-1899.
Rathore, M. M., Ahmad, A., Paul, A., & Rho, S. (2016). Urban planning and building smart
cities based on the internet of things using big data analytics. Computer Networks, 101,
63-80.
Schoenherr, T., & Speier‐Pero, C. (2015). Data science, predictive analytics, and big data in
supply chain management: Current state and future potential. Journal of Business
Logistics, 36(1), 120-132.
Sousa, M. J., Pesqueira, A. M., Lemos, C., Sousa, M., & Rocha, Á. (2019). Decision-Making
based on Big Data Analytics for People Management in Healthcare
Organizations. Journal of medical systems, 43(9), 290.
Umar, S., & Hassan, S. (2018). Encouraging the collection of performance data in nonprofit
organizations: The importance of organizational support for learning. Public
Performance & Management Review, 1-23.
Van Der Aalst, W. (2016). Data science in action. In Process Mining (pp. 3-23). Springer,
Berlin, Heidelberg.
Wamba, S. F., Gunasekaran, A., Akter, S., Ren, S. J. F., Dubey, R., & Childe, S. J. (2017). Big
data analytics and firm performance: Effects of dynamic capabilities. Journal of Business
Research, 70, 356-365.
Paraphrase This Document
Need a fresh take? Get an instant paraphrase of this document with our AI Paraphraser
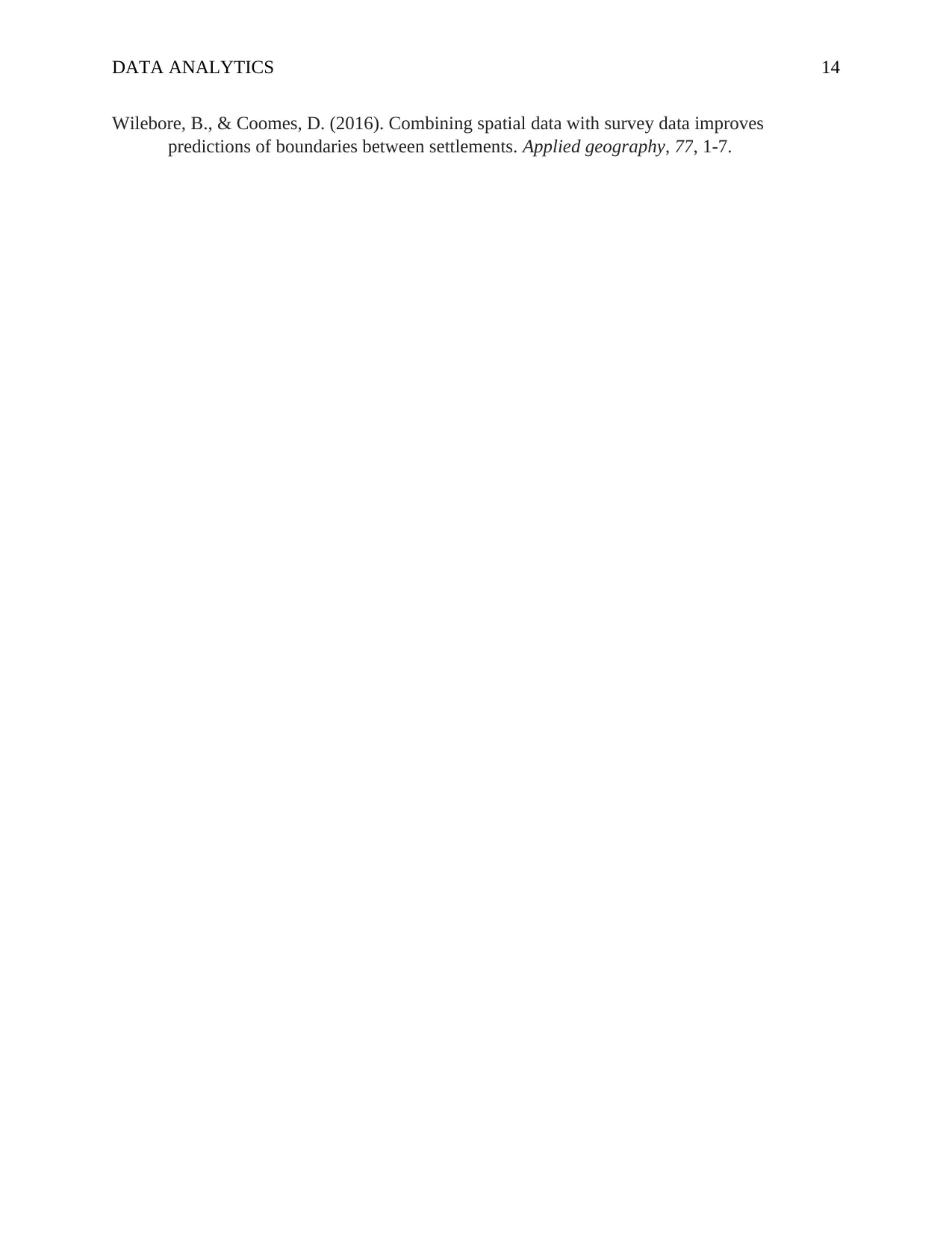
DATA ANALYTICS 14
Wilebore, B., & Coomes, D. (2016). Combining spatial data with survey data improves
predictions of boundaries between settlements. Applied geography, 77, 1-7.
Wilebore, B., & Coomes, D. (2016). Combining spatial data with survey data improves
predictions of boundaries between settlements. Applied geography, 77, 1-7.
1 out of 14
Related Documents

Your All-in-One AI-Powered Toolkit for Academic Success.
+13062052269
info@desklib.com
Available 24*7 on WhatsApp / Email
Unlock your academic potential
© 2024 | Zucol Services PVT LTD | All rights reserved.