MSc Project Proposal: Human Activity Recognition with Wearable Sensors
VerifiedAdded on 2023/01/18
|9
|2082
|53
Project
AI Summary
This document presents a formal proposal for a Master's dissertation at the University of Greenwich, focusing on human activity recognition using data from wearable sensors. The proposal outlines the project's objectives, which include investigating human activity recognition, facilitating the develop...
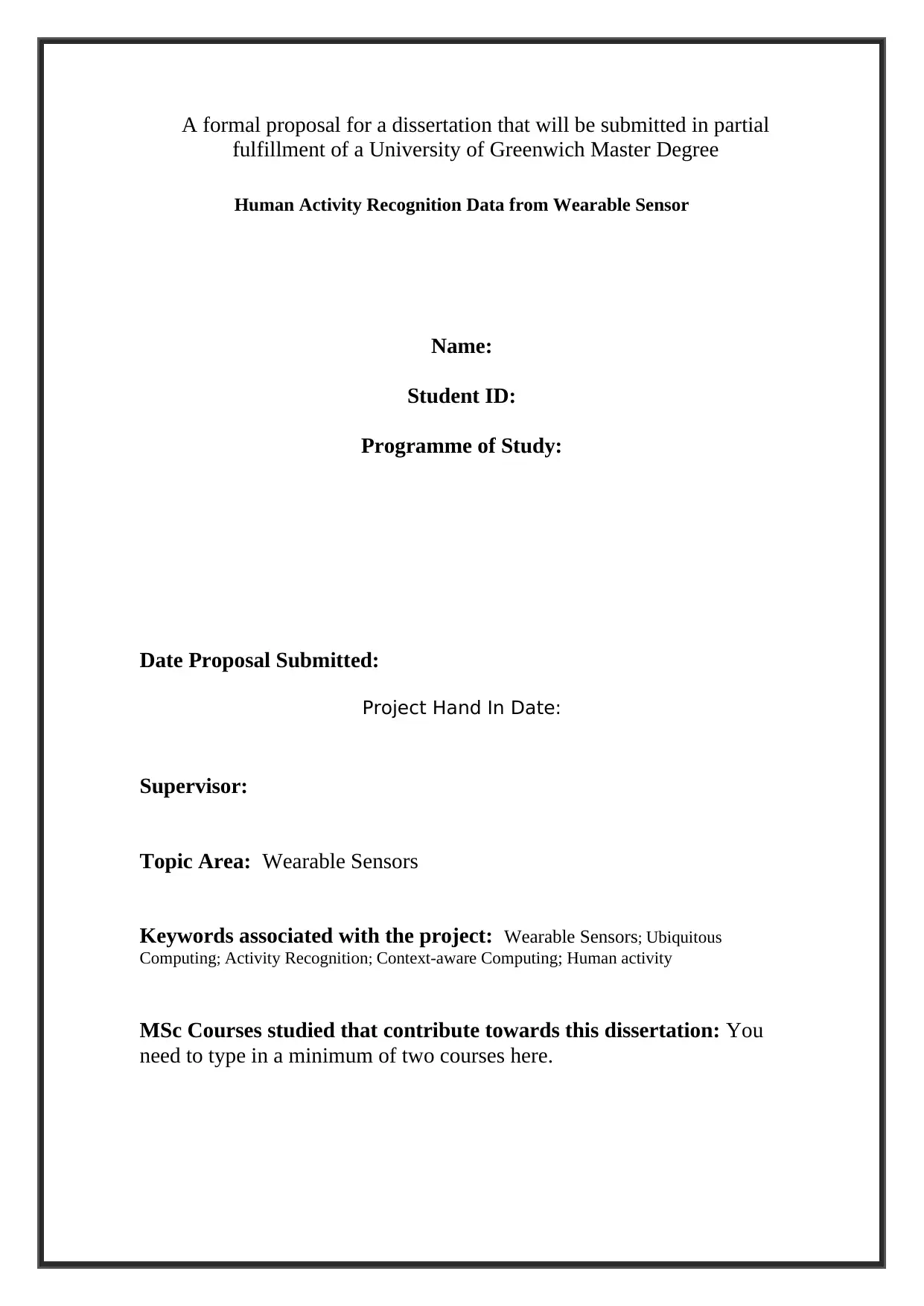
A formal proposal for a dissertation that will be submitted in partial
fulfillment of a University of Greenwich Master Degree
Human Activity Recognition Data from Wearable Sensor
Name:
Student ID:
Programme of Study:
Date Proposal Submitted:
Project Hand In Date:
Supervisor:
Topic Area: Wearable Sensors
Keywords associated with the project: Wearable Sensors; Ubiquitous
Computing; Activity Recognition; Context-aware Computing; Human activity
MSc Courses studied that contribute towards this dissertation: You
need to type in a minimum of two courses here.
fulfillment of a University of Greenwich Master Degree
Human Activity Recognition Data from Wearable Sensor
Name:
Student ID:
Programme of Study:
Date Proposal Submitted:
Project Hand In Date:
Supervisor:
Topic Area: Wearable Sensors
Keywords associated with the project: Wearable Sensors; Ubiquitous
Computing; Activity Recognition; Context-aware Computing; Human activity
MSc Courses studied that contribute towards this dissertation: You
need to type in a minimum of two courses here.
Paraphrase This Document
Need a fresh take? Get an instant paraphrase of this document with our AI Paraphraser
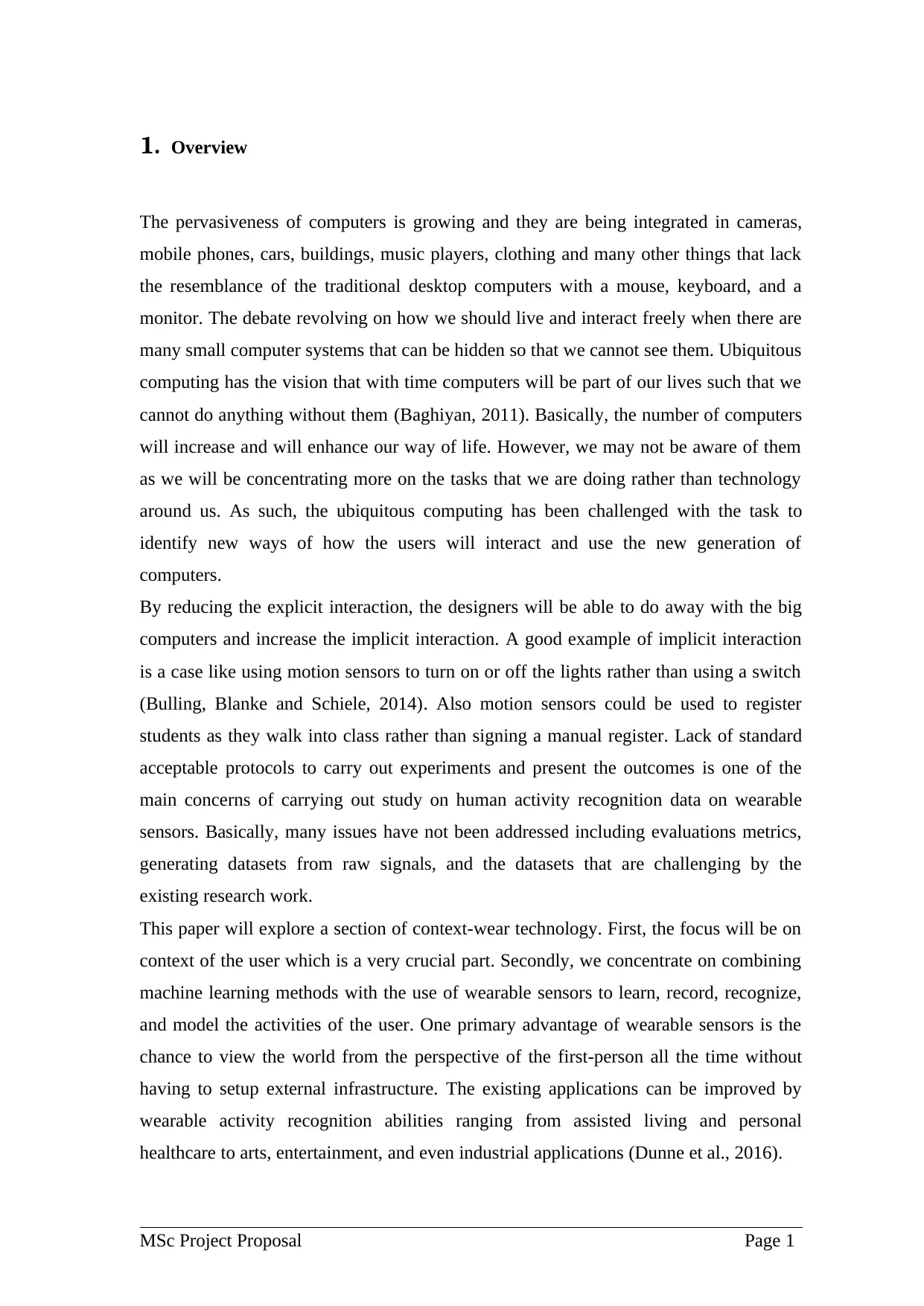
1. Overview
The pervasiveness of computers is growing and they are being integrated in cameras,
mobile phones, cars, buildings, music players, clothing and many other things that lack
the resemblance of the traditional desktop computers with a mouse, keyboard, and a
monitor. The debate revolving on how we should live and interact freely when there are
many small computer systems that can be hidden so that we cannot see them. Ubiquitous
computing has the vision that with time computers will be part of our lives such that we
cannot do anything without them (Baghiyan, 2011). Basically, the number of computers
will increase and will enhance our way of life. However, we may not be aware of them
as we will be concentrating more on the tasks that we are doing rather than technology
around us. As such, the ubiquitous computing has been challenged with the task to
identify new ways of how the users will interact and use the new generation of
computers.
By reducing the explicit interaction, the designers will be able to do away with the big
computers and increase the implicit interaction. A good example of implicit interaction
is a case like using motion sensors to turn on or off the lights rather than using a switch
(Bulling, Blanke and Schiele, 2014). Also motion sensors could be used to register
students as they walk into class rather than signing a manual register. Lack of standard
acceptable protocols to carry out experiments and present the outcomes is one of the
main concerns of carrying out study on human activity recognition data on wearable
sensors. Basically, many issues have not been addressed including evaluations metrics,
generating datasets from raw signals, and the datasets that are challenging by the
existing research work.
This paper will explore a section of context-wear technology. First, the focus will be on
context of the user which is a very crucial part. Secondly, we concentrate on combining
machine learning methods with the use of wearable sensors to learn, record, recognize,
and model the activities of the user. One primary advantage of wearable sensors is the
chance to view the world from the perspective of the first-person all the time without
having to setup external infrastructure. The existing applications can be improved by
wearable activity recognition abilities ranging from assisted living and personal
healthcare to arts, entertainment, and even industrial applications (Dunne et al., 2016).
MSc Project Proposal Page 1
The pervasiveness of computers is growing and they are being integrated in cameras,
mobile phones, cars, buildings, music players, clothing and many other things that lack
the resemblance of the traditional desktop computers with a mouse, keyboard, and a
monitor. The debate revolving on how we should live and interact freely when there are
many small computer systems that can be hidden so that we cannot see them. Ubiquitous
computing has the vision that with time computers will be part of our lives such that we
cannot do anything without them (Baghiyan, 2011). Basically, the number of computers
will increase and will enhance our way of life. However, we may not be aware of them
as we will be concentrating more on the tasks that we are doing rather than technology
around us. As such, the ubiquitous computing has been challenged with the task to
identify new ways of how the users will interact and use the new generation of
computers.
By reducing the explicit interaction, the designers will be able to do away with the big
computers and increase the implicit interaction. A good example of implicit interaction
is a case like using motion sensors to turn on or off the lights rather than using a switch
(Bulling, Blanke and Schiele, 2014). Also motion sensors could be used to register
students as they walk into class rather than signing a manual register. Lack of standard
acceptable protocols to carry out experiments and present the outcomes is one of the
main concerns of carrying out study on human activity recognition data on wearable
sensors. Basically, many issues have not been addressed including evaluations metrics,
generating datasets from raw signals, and the datasets that are challenging by the
existing research work.
This paper will explore a section of context-wear technology. First, the focus will be on
context of the user which is a very crucial part. Secondly, we concentrate on combining
machine learning methods with the use of wearable sensors to learn, record, recognize,
and model the activities of the user. One primary advantage of wearable sensors is the
chance to view the world from the perspective of the first-person all the time without
having to setup external infrastructure. The existing applications can be improved by
wearable activity recognition abilities ranging from assisted living and personal
healthcare to arts, entertainment, and even industrial applications (Dunne et al., 2016).
MSc Project Proposal Page 1
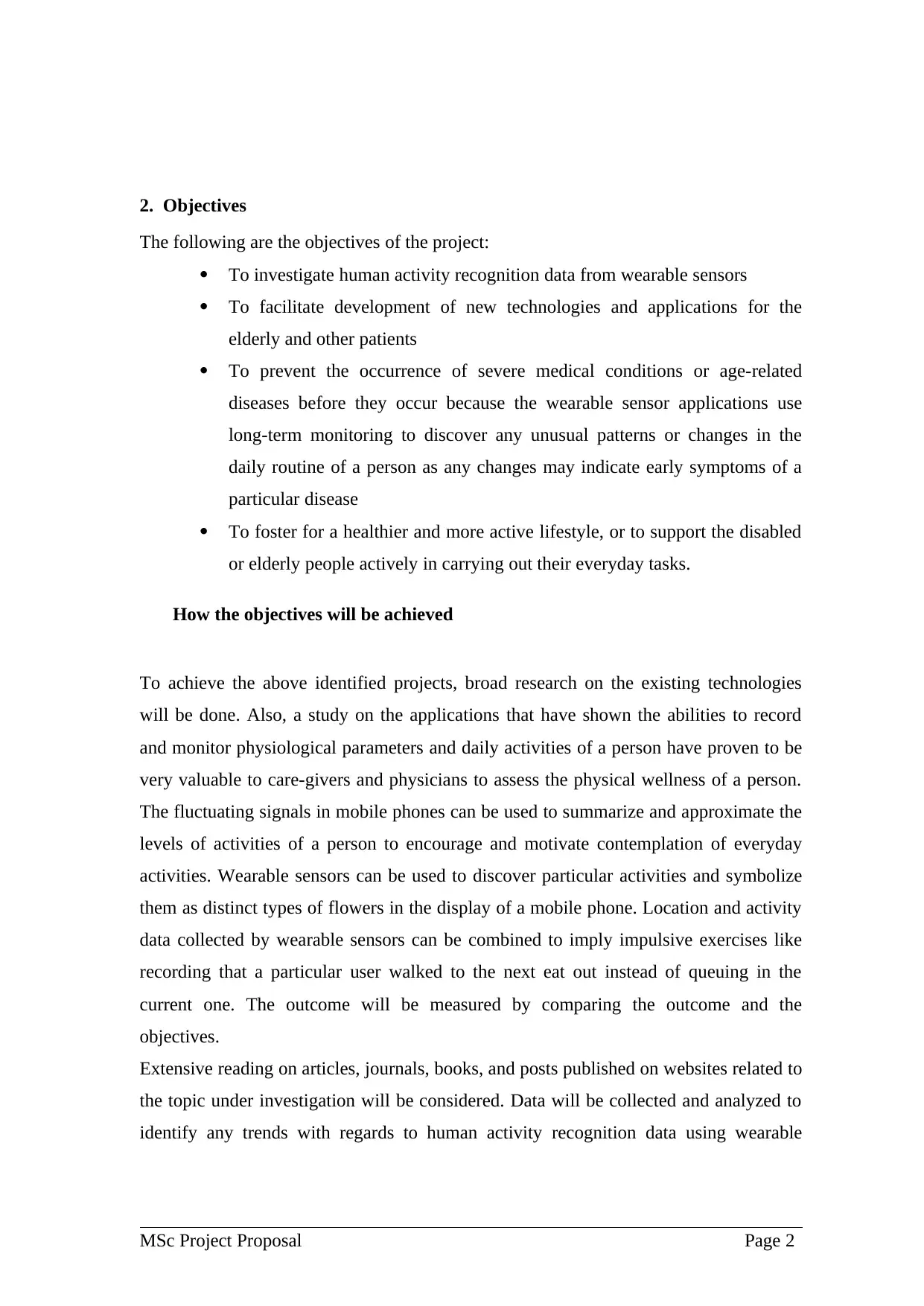
2. Objectives
The following are the objectives of the project:
To investigate human activity recognition data from wearable sensors
To facilitate development of new technologies and applications for the
elderly and other patients
To prevent the occurrence of severe medical conditions or age-related
diseases before they occur because the wearable sensor applications use
long-term monitoring to discover any unusual patterns or changes in the
daily routine of a person as any changes may indicate early symptoms of a
particular disease
To foster for a healthier and more active lifestyle, or to support the disabled
or elderly people actively in carrying out their everyday tasks.
How the objectives will be achieved
To achieve the above identified projects, broad research on the existing technologies
will be done. Also, a study on the applications that have shown the abilities to record
and monitor physiological parameters and daily activities of a person have proven to be
very valuable to care-givers and physicians to assess the physical wellness of a person.
The fluctuating signals in mobile phones can be used to summarize and approximate the
levels of activities of a person to encourage and motivate contemplation of everyday
activities. Wearable sensors can be used to discover particular activities and symbolize
them as distinct types of flowers in the display of a mobile phone. Location and activity
data collected by wearable sensors can be combined to imply impulsive exercises like
recording that a particular user walked to the next eat out instead of queuing in the
current one. The outcome will be measured by comparing the outcome and the
objectives.
Extensive reading on articles, journals, books, and posts published on websites related to
the topic under investigation will be considered. Data will be collected and analyzed to
identify any trends with regards to human activity recognition data using wearable
MSc Project Proposal Page 2
The following are the objectives of the project:
To investigate human activity recognition data from wearable sensors
To facilitate development of new technologies and applications for the
elderly and other patients
To prevent the occurrence of severe medical conditions or age-related
diseases before they occur because the wearable sensor applications use
long-term monitoring to discover any unusual patterns or changes in the
daily routine of a person as any changes may indicate early symptoms of a
particular disease
To foster for a healthier and more active lifestyle, or to support the disabled
or elderly people actively in carrying out their everyday tasks.
How the objectives will be achieved
To achieve the above identified projects, broad research on the existing technologies
will be done. Also, a study on the applications that have shown the abilities to record
and monitor physiological parameters and daily activities of a person have proven to be
very valuable to care-givers and physicians to assess the physical wellness of a person.
The fluctuating signals in mobile phones can be used to summarize and approximate the
levels of activities of a person to encourage and motivate contemplation of everyday
activities. Wearable sensors can be used to discover particular activities and symbolize
them as distinct types of flowers in the display of a mobile phone. Location and activity
data collected by wearable sensors can be combined to imply impulsive exercises like
recording that a particular user walked to the next eat out instead of queuing in the
current one. The outcome will be measured by comparing the outcome and the
objectives.
Extensive reading on articles, journals, books, and posts published on websites related to
the topic under investigation will be considered. Data will be collected and analyzed to
identify any trends with regards to human activity recognition data using wearable
MSc Project Proposal Page 2
⊘ This is a preview!⊘
Do you want full access?
Subscribe today to unlock all pages.

Trusted by 1+ million students worldwide
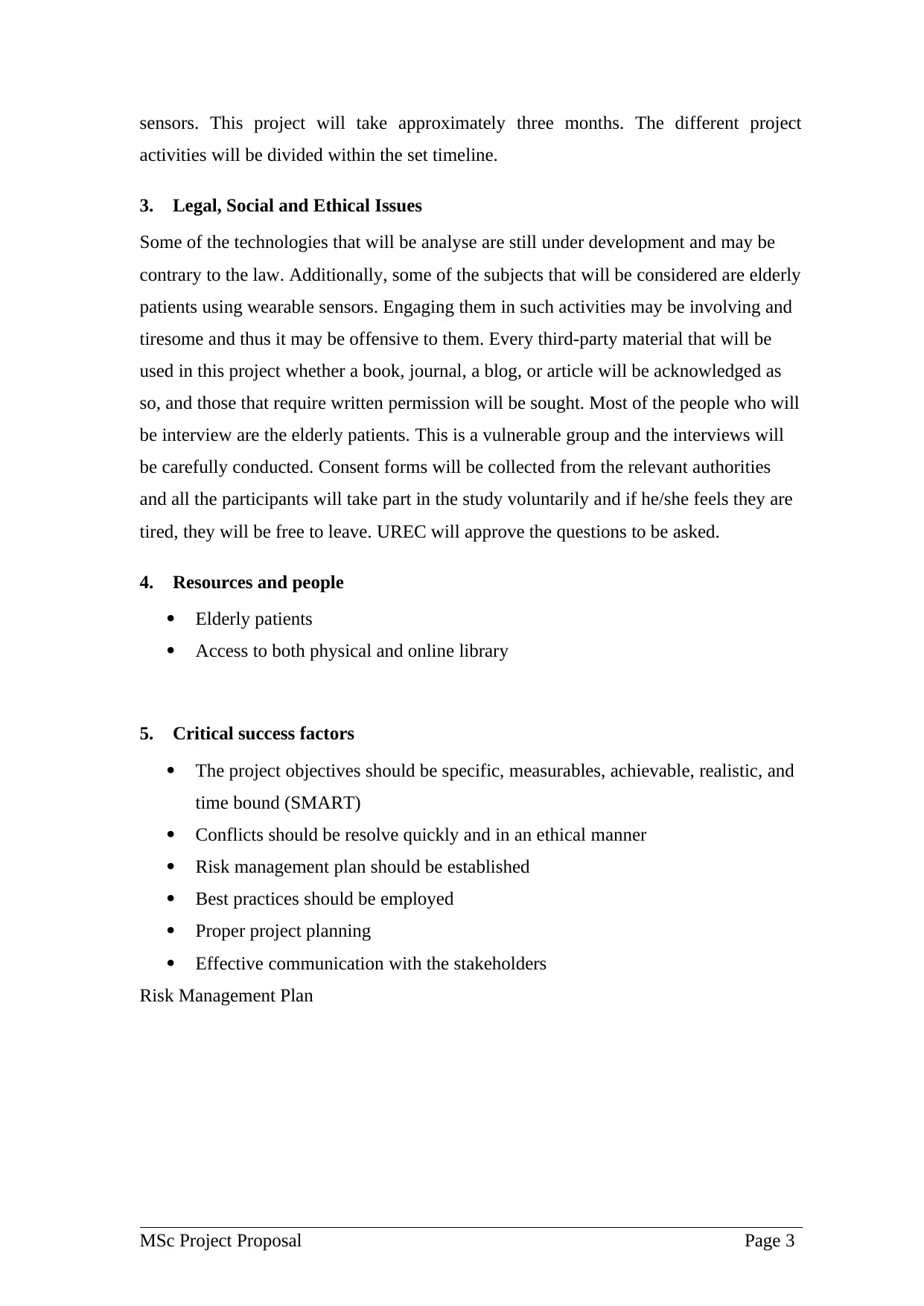
sensors. This project will take approximately three months. The different project
activities will be divided within the set timeline.
3. Legal, Social and Ethical Issues
Some of the technologies that will be analyse are still under development and may be
contrary to the law. Additionally, some of the subjects that will be considered are elderly
patients using wearable sensors. Engaging them in such activities may be involving and
tiresome and thus it may be offensive to them. Every third-party material that will be
used in this project whether a book, journal, a blog, or article will be acknowledged as
so, and those that require written permission will be sought. Most of the people who will
be interview are the elderly patients. This is a vulnerable group and the interviews will
be carefully conducted. Consent forms will be collected from the relevant authorities
and all the participants will take part in the study voluntarily and if he/she feels they are
tired, they will be free to leave. UREC will approve the questions to be asked.
4. Resources and people
Elderly patients
Access to both physical and online library
5. Critical success factors
The project objectives should be specific, measurables, achievable, realistic, and
time bound (SMART)
Conflicts should be resolve quickly and in an ethical manner
Risk management plan should be established
Best practices should be employed
Proper project planning
Effective communication with the stakeholders
Risk Management Plan
MSc Project Proposal Page 3
activities will be divided within the set timeline.
3. Legal, Social and Ethical Issues
Some of the technologies that will be analyse are still under development and may be
contrary to the law. Additionally, some of the subjects that will be considered are elderly
patients using wearable sensors. Engaging them in such activities may be involving and
tiresome and thus it may be offensive to them. Every third-party material that will be
used in this project whether a book, journal, a blog, or article will be acknowledged as
so, and those that require written permission will be sought. Most of the people who will
be interview are the elderly patients. This is a vulnerable group and the interviews will
be carefully conducted. Consent forms will be collected from the relevant authorities
and all the participants will take part in the study voluntarily and if he/she feels they are
tired, they will be free to leave. UREC will approve the questions to be asked.
4. Resources and people
Elderly patients
Access to both physical and online library
5. Critical success factors
The project objectives should be specific, measurables, achievable, realistic, and
time bound (SMART)
Conflicts should be resolve quickly and in an ethical manner
Risk management plan should be established
Best practices should be employed
Proper project planning
Effective communication with the stakeholders
Risk Management Plan
MSc Project Proposal Page 3
Paraphrase This Document
Need a fresh take? Get an instant paraphrase of this document with our AI Paraphraser
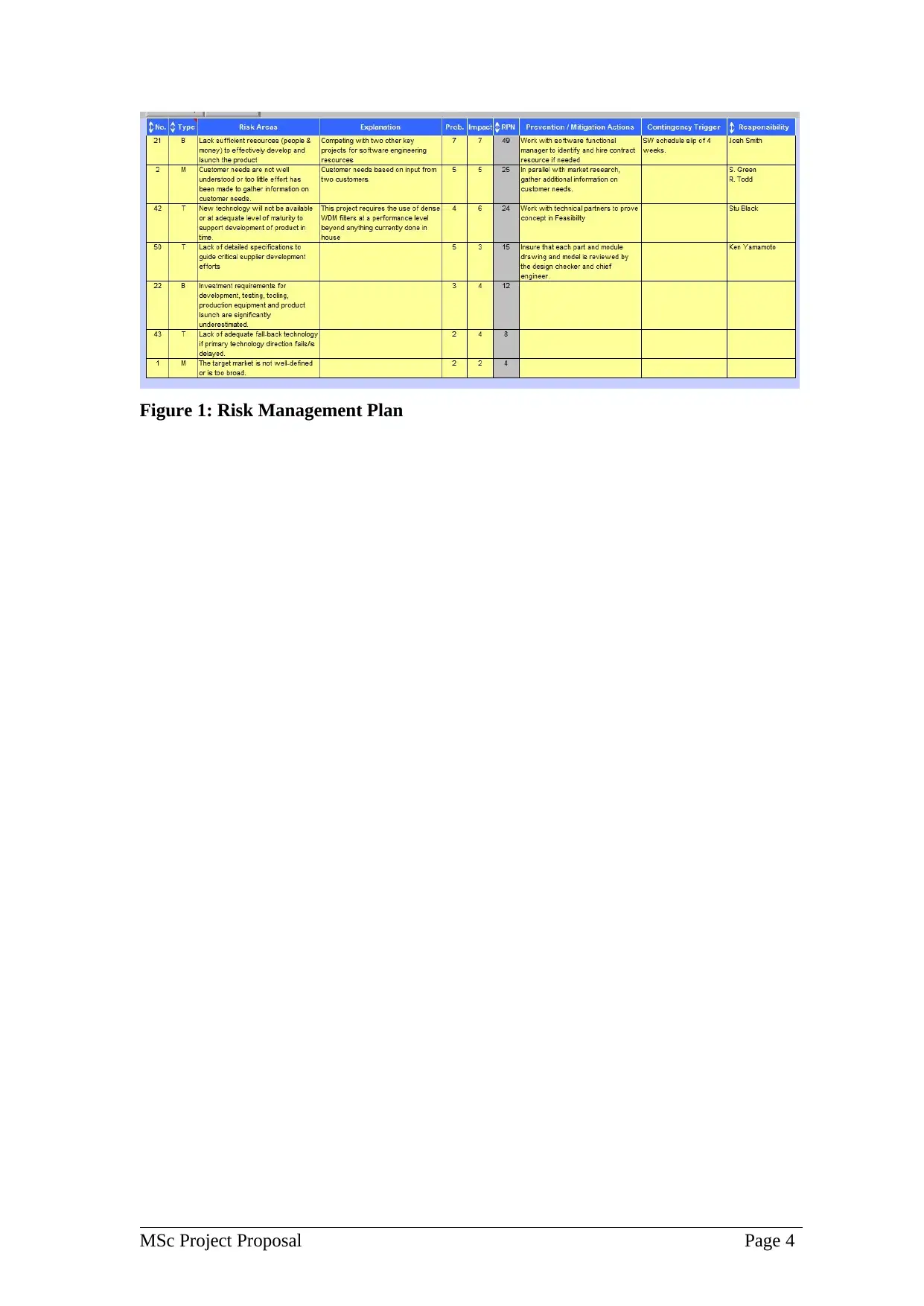
Figure 1: Risk Management Plan
MSc Project Proposal Page 4
MSc Project Proposal Page 4
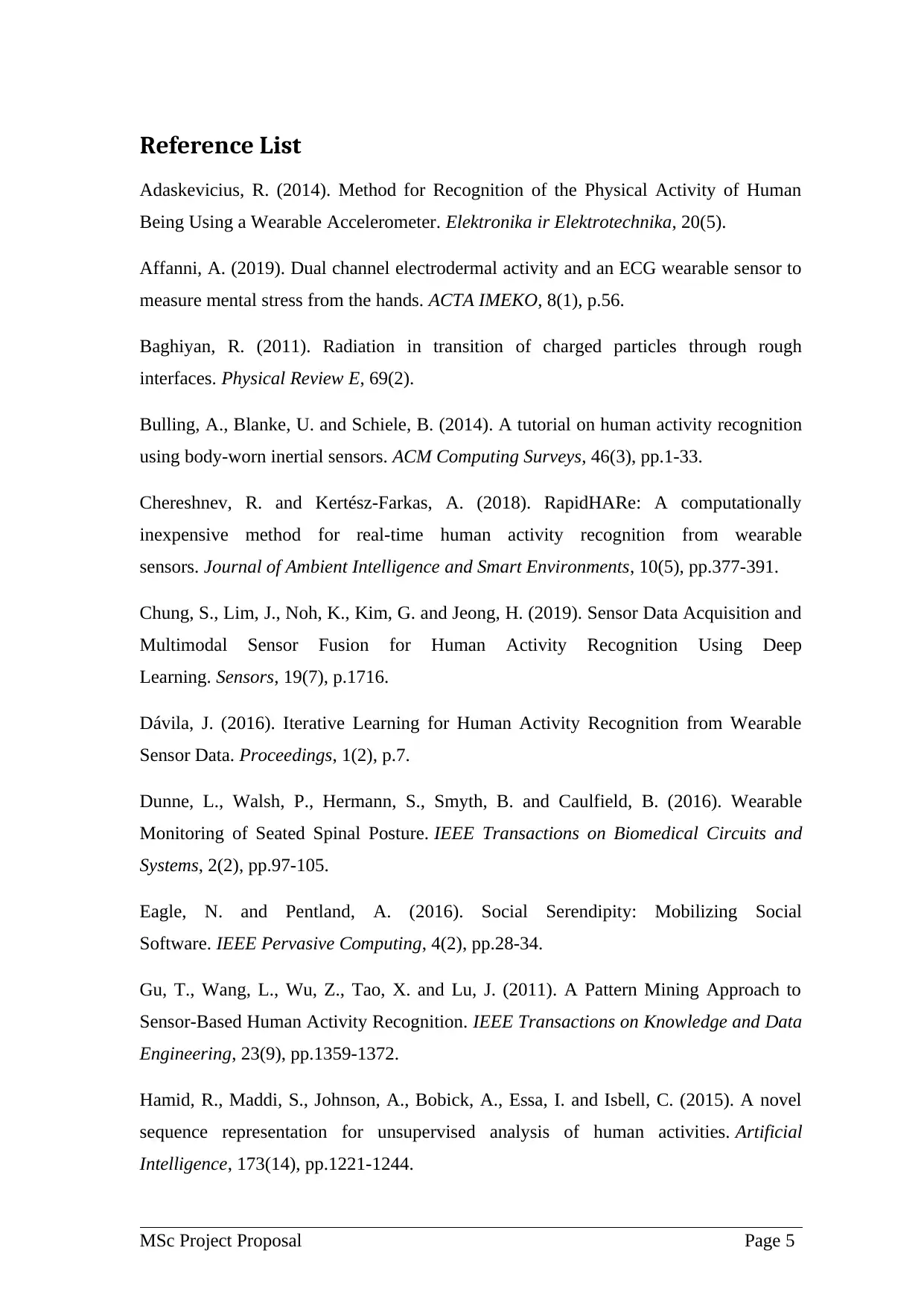
Reference List
Adaskevicius, R. (2014). Method for Recognition of the Physical Activity of Human
Being Using a Wearable Accelerometer. Elektronika ir Elektrotechnika, 20(5).
Affanni, A. (2019). Dual channel electrodermal activity and an ECG wearable sensor to
measure mental stress from the hands. ACTA IMEKO, 8(1), p.56.
Baghiyan, R. (2011). Radiation in transition of charged particles through rough
interfaces. Physical Review E, 69(2).
Bulling, A., Blanke, U. and Schiele, B. (2014). A tutorial on human activity recognition
using body-worn inertial sensors. ACM Computing Surveys, 46(3), pp.1-33.
Chereshnev, R. and Kertész-Farkas, A. (2018). RapidHARe: A computationally
inexpensive method for real-time human activity recognition from wearable
sensors. Journal of Ambient Intelligence and Smart Environments, 10(5), pp.377-391.
Chung, S., Lim, J., Noh, K., Kim, G. and Jeong, H. (2019). Sensor Data Acquisition and
Multimodal Sensor Fusion for Human Activity Recognition Using Deep
Learning. Sensors, 19(7), p.1716.
Dávila, J. (2016). Iterative Learning for Human Activity Recognition from Wearable
Sensor Data. Proceedings, 1(2), p.7.
Dunne, L., Walsh, P., Hermann, S., Smyth, B. and Caulfield, B. (2016). Wearable
Monitoring of Seated Spinal Posture. IEEE Transactions on Biomedical Circuits and
Systems, 2(2), pp.97-105.
Eagle, N. and Pentland, A. (2016). Social Serendipity: Mobilizing Social
Software. IEEE Pervasive Computing, 4(2), pp.28-34.
Gu, T., Wang, L., Wu, Z., Tao, X. and Lu, J. (2011). A Pattern Mining Approach to
Sensor-Based Human Activity Recognition. IEEE Transactions on Knowledge and Data
Engineering, 23(9), pp.1359-1372.
Hamid, R., Maddi, S., Johnson, A., Bobick, A., Essa, I. and Isbell, C. (2015). A novel
sequence representation for unsupervised analysis of human activities. Artificial
Intelligence, 173(14), pp.1221-1244.
MSc Project Proposal Page 5
Adaskevicius, R. (2014). Method for Recognition of the Physical Activity of Human
Being Using a Wearable Accelerometer. Elektronika ir Elektrotechnika, 20(5).
Affanni, A. (2019). Dual channel electrodermal activity and an ECG wearable sensor to
measure mental stress from the hands. ACTA IMEKO, 8(1), p.56.
Baghiyan, R. (2011). Radiation in transition of charged particles through rough
interfaces. Physical Review E, 69(2).
Bulling, A., Blanke, U. and Schiele, B. (2014). A tutorial on human activity recognition
using body-worn inertial sensors. ACM Computing Surveys, 46(3), pp.1-33.
Chereshnev, R. and Kertész-Farkas, A. (2018). RapidHARe: A computationally
inexpensive method for real-time human activity recognition from wearable
sensors. Journal of Ambient Intelligence and Smart Environments, 10(5), pp.377-391.
Chung, S., Lim, J., Noh, K., Kim, G. and Jeong, H. (2019). Sensor Data Acquisition and
Multimodal Sensor Fusion for Human Activity Recognition Using Deep
Learning. Sensors, 19(7), p.1716.
Dávila, J. (2016). Iterative Learning for Human Activity Recognition from Wearable
Sensor Data. Proceedings, 1(2), p.7.
Dunne, L., Walsh, P., Hermann, S., Smyth, B. and Caulfield, B. (2016). Wearable
Monitoring of Seated Spinal Posture. IEEE Transactions on Biomedical Circuits and
Systems, 2(2), pp.97-105.
Eagle, N. and Pentland, A. (2016). Social Serendipity: Mobilizing Social
Software. IEEE Pervasive Computing, 4(2), pp.28-34.
Gu, T., Wang, L., Wu, Z., Tao, X. and Lu, J. (2011). A Pattern Mining Approach to
Sensor-Based Human Activity Recognition. IEEE Transactions on Knowledge and Data
Engineering, 23(9), pp.1359-1372.
Hamid, R., Maddi, S., Johnson, A., Bobick, A., Essa, I. and Isbell, C. (2015). A novel
sequence representation for unsupervised analysis of human activities. Artificial
Intelligence, 173(14), pp.1221-1244.
MSc Project Proposal Page 5
⊘ This is a preview!⊘
Do you want full access?
Subscribe today to unlock all pages.

Trusted by 1+ million students worldwide
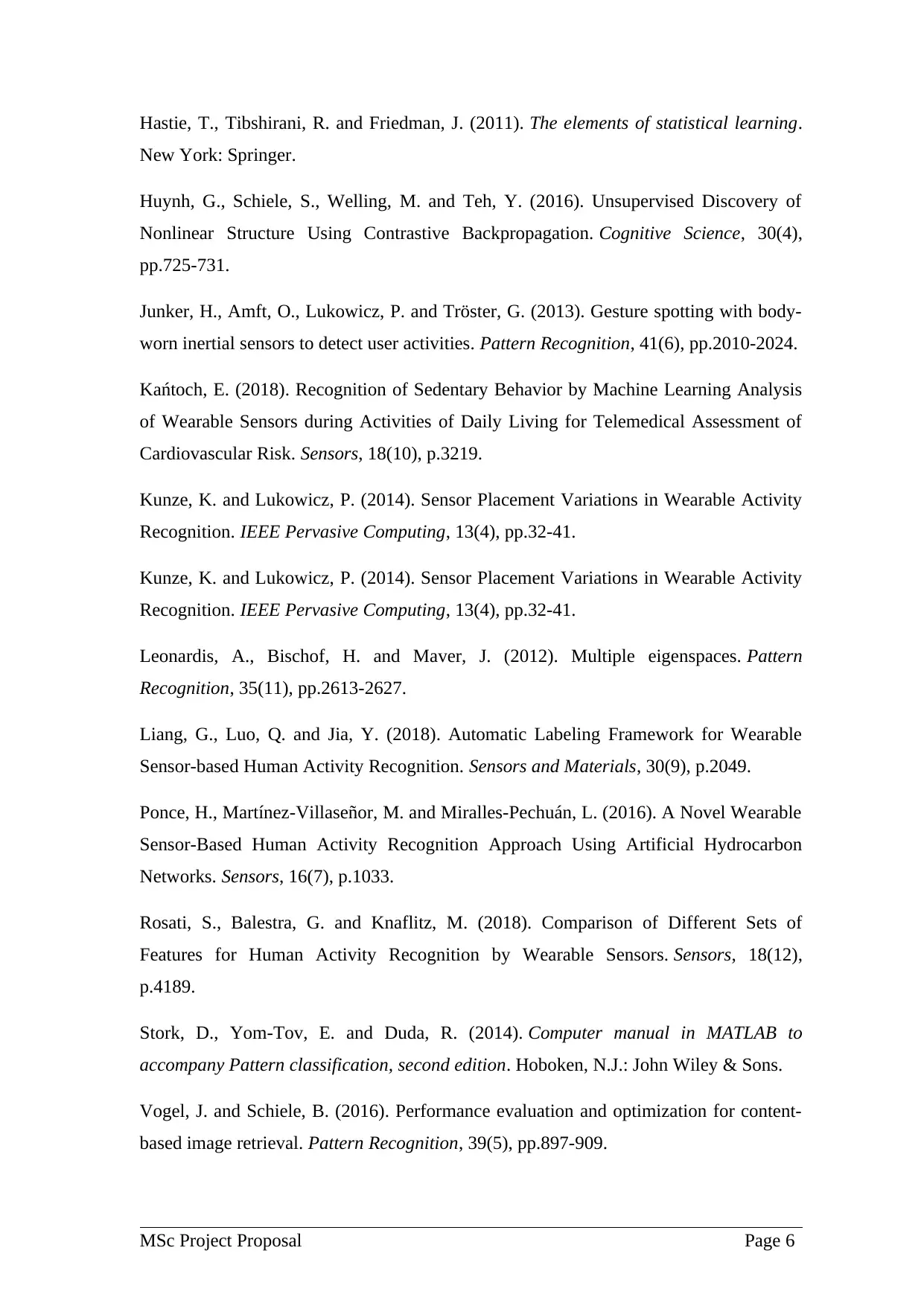
Hastie, T., Tibshirani, R. and Friedman, J. (2011). The elements of statistical learning.
New York: Springer.
Huynh, G., Schiele, S., Welling, M. and Teh, Y. (2016). Unsupervised Discovery of
Nonlinear Structure Using Contrastive Backpropagation. Cognitive Science, 30(4),
pp.725-731.
Junker, H., Amft, O., Lukowicz, P. and Tröster, G. (2013). Gesture spotting with body-
worn inertial sensors to detect user activities. Pattern Recognition, 41(6), pp.2010-2024.
Kańtoch, E. (2018). Recognition of Sedentary Behavior by Machine Learning Analysis
of Wearable Sensors during Activities of Daily Living for Telemedical Assessment of
Cardiovascular Risk. Sensors, 18(10), p.3219.
Kunze, K. and Lukowicz, P. (2014). Sensor Placement Variations in Wearable Activity
Recognition. IEEE Pervasive Computing, 13(4), pp.32-41.
Kunze, K. and Lukowicz, P. (2014). Sensor Placement Variations in Wearable Activity
Recognition. IEEE Pervasive Computing, 13(4), pp.32-41.
Leonardis, A., Bischof, H. and Maver, J. (2012). Multiple eigenspaces. Pattern
Recognition, 35(11), pp.2613-2627.
Liang, G., Luo, Q. and Jia, Y. (2018). Automatic Labeling Framework for Wearable
Sensor-based Human Activity Recognition. Sensors and Materials, 30(9), p.2049.
Ponce, H., Martínez-Villaseñor, M. and Miralles-Pechuán, L. (2016). A Novel Wearable
Sensor-Based Human Activity Recognition Approach Using Artificial Hydrocarbon
Networks. Sensors, 16(7), p.1033.
Rosati, S., Balestra, G. and Knaflitz, M. (2018). Comparison of Different Sets of
Features for Human Activity Recognition by Wearable Sensors. Sensors, 18(12),
p.4189.
Stork, D., Yom-Tov, E. and Duda, R. (2014). Computer manual in MATLAB to
accompany Pattern classification, second edition. Hoboken, N.J.: John Wiley & Sons.
Vogel, J. and Schiele, B. (2016). Performance evaluation and optimization for content-
based image retrieval. Pattern Recognition, 39(5), pp.897-909.
MSc Project Proposal Page 6
New York: Springer.
Huynh, G., Schiele, S., Welling, M. and Teh, Y. (2016). Unsupervised Discovery of
Nonlinear Structure Using Contrastive Backpropagation. Cognitive Science, 30(4),
pp.725-731.
Junker, H., Amft, O., Lukowicz, P. and Tröster, G. (2013). Gesture spotting with body-
worn inertial sensors to detect user activities. Pattern Recognition, 41(6), pp.2010-2024.
Kańtoch, E. (2018). Recognition of Sedentary Behavior by Machine Learning Analysis
of Wearable Sensors during Activities of Daily Living for Telemedical Assessment of
Cardiovascular Risk. Sensors, 18(10), p.3219.
Kunze, K. and Lukowicz, P. (2014). Sensor Placement Variations in Wearable Activity
Recognition. IEEE Pervasive Computing, 13(4), pp.32-41.
Kunze, K. and Lukowicz, P. (2014). Sensor Placement Variations in Wearable Activity
Recognition. IEEE Pervasive Computing, 13(4), pp.32-41.
Leonardis, A., Bischof, H. and Maver, J. (2012). Multiple eigenspaces. Pattern
Recognition, 35(11), pp.2613-2627.
Liang, G., Luo, Q. and Jia, Y. (2018). Automatic Labeling Framework for Wearable
Sensor-based Human Activity Recognition. Sensors and Materials, 30(9), p.2049.
Ponce, H., Martínez-Villaseñor, M. and Miralles-Pechuán, L. (2016). A Novel Wearable
Sensor-Based Human Activity Recognition Approach Using Artificial Hydrocarbon
Networks. Sensors, 16(7), p.1033.
Rosati, S., Balestra, G. and Knaflitz, M. (2018). Comparison of Different Sets of
Features for Human Activity Recognition by Wearable Sensors. Sensors, 18(12),
p.4189.
Stork, D., Yom-Tov, E. and Duda, R. (2014). Computer manual in MATLAB to
accompany Pattern classification, second edition. Hoboken, N.J.: John Wiley & Sons.
Vogel, J. and Schiele, B. (2016). Performance evaluation and optimization for content-
based image retrieval. Pattern Recognition, 39(5), pp.897-909.
MSc Project Proposal Page 6
Paraphrase This Document
Need a fresh take? Get an instant paraphrase of this document with our AI Paraphraser
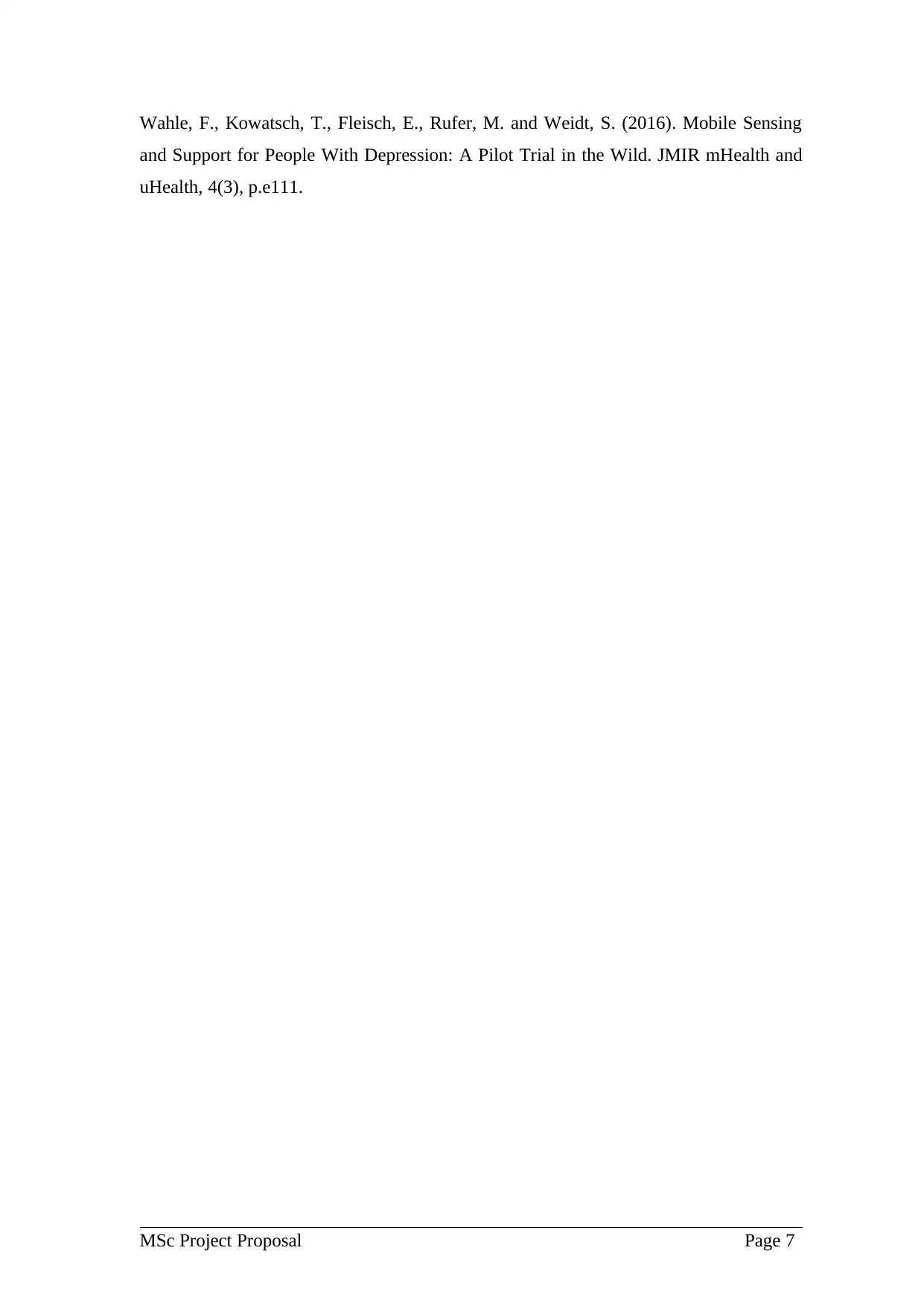
Wahle, F., Kowatsch, T., Fleisch, E., Rufer, M. and Weidt, S. (2016). Mobile Sensing
and Support for People With Depression: A Pilot Trial in the Wild. JMIR mHealth and
uHealth, 4(3), p.e111.
MSc Project Proposal Page 7
and Support for People With Depression: A Pilot Trial in the Wild. JMIR mHealth and
uHealth, 4(3), p.e111.
MSc Project Proposal Page 7
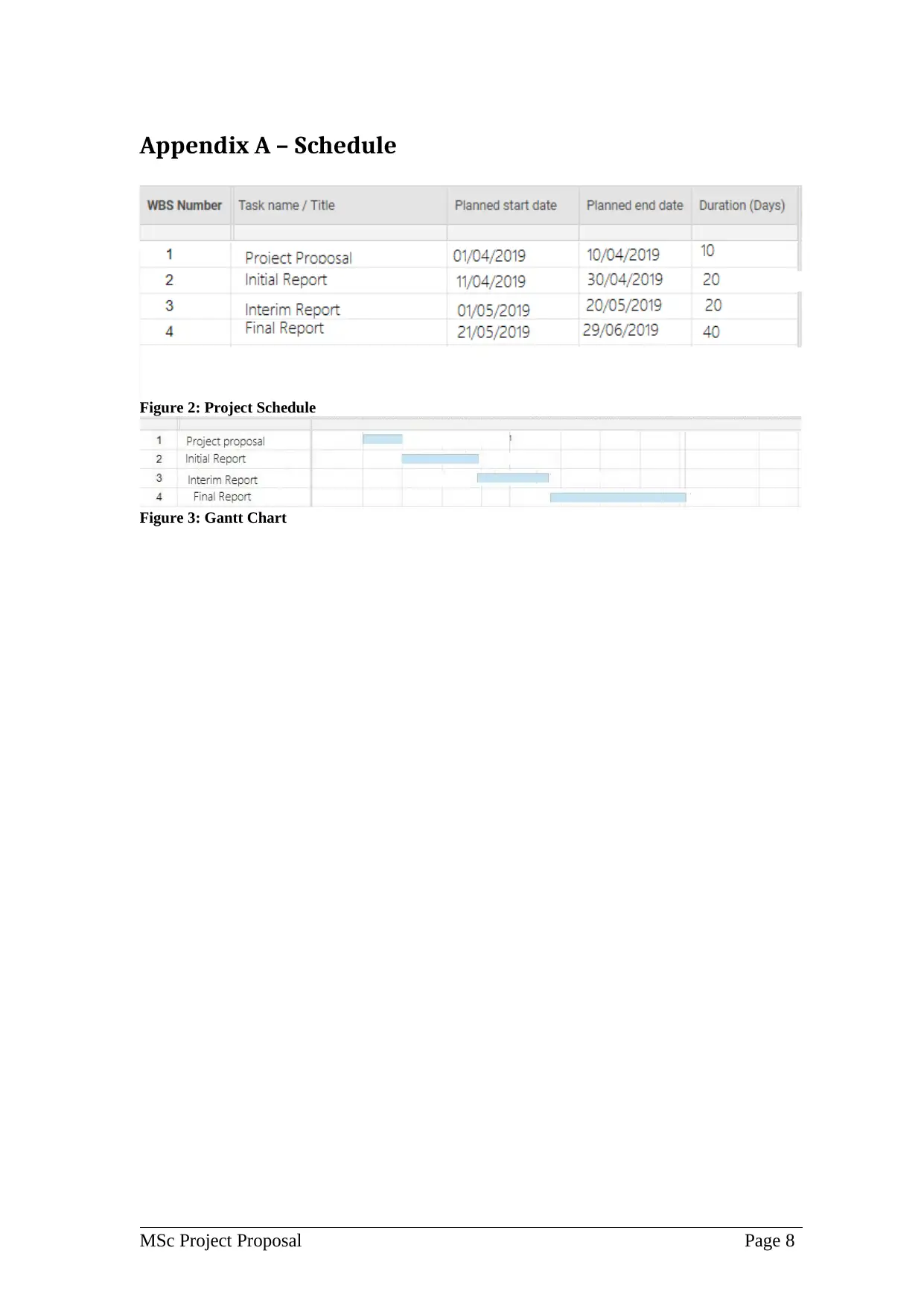
Appendix A – Schedule
Figure 2: Project Schedule
Figure 3: Gantt Chart
MSc Project Proposal Page 8
Figure 2: Project Schedule
Figure 3: Gantt Chart
MSc Project Proposal Page 8
⊘ This is a preview!⊘
Do you want full access?
Subscribe today to unlock all pages.

Trusted by 1+ million students worldwide
1 out of 9
Related Documents

Your All-in-One AI-Powered Toolkit for Academic Success.
+13062052269
info@desklib.com
Available 24*7 on WhatsApp / Email
Unlock your academic potential
© 2024 | Zucol Services PVT LTD | All rights reserved.