Identifying Consumer Preferences: Product Recommendations in Ads
VerifiedAdded on 2022/08/25
|13
|18141
|68
Report
AI Summary
This report, based on research published in the Journal of Retailing and Consumer Services, investigates consumer preferences for personalized product recommendations in advertising across multiple media channels. The study employs a choice-based conjoint experiment to examine the ideal design of personalized product recommendations, focusing on advertising channels, recommendation numbers, and gender differences. Two separate studies were conducted with young male and female consumers in Germany, revealing that the advertising channel is the most critical attribute influencing adoption intentions, followed by the number of recommendations. The research compares the effectiveness of package inserts, email advertising, and banner advertising, highlighting the least preferred channel for both genders and smaller recommendation sets for males. The report also analyzes reasons for advertisement rejection and provides design recommendations for advertisers and retailers to enhance personalized product recommendations. The study extends current research on personalization effects by comparing different advertising channels and considering gender-specific preferences. The findings suggest that advertisers must make informed decisions about allocating their advertising spend across various media channels to maximize effectiveness.
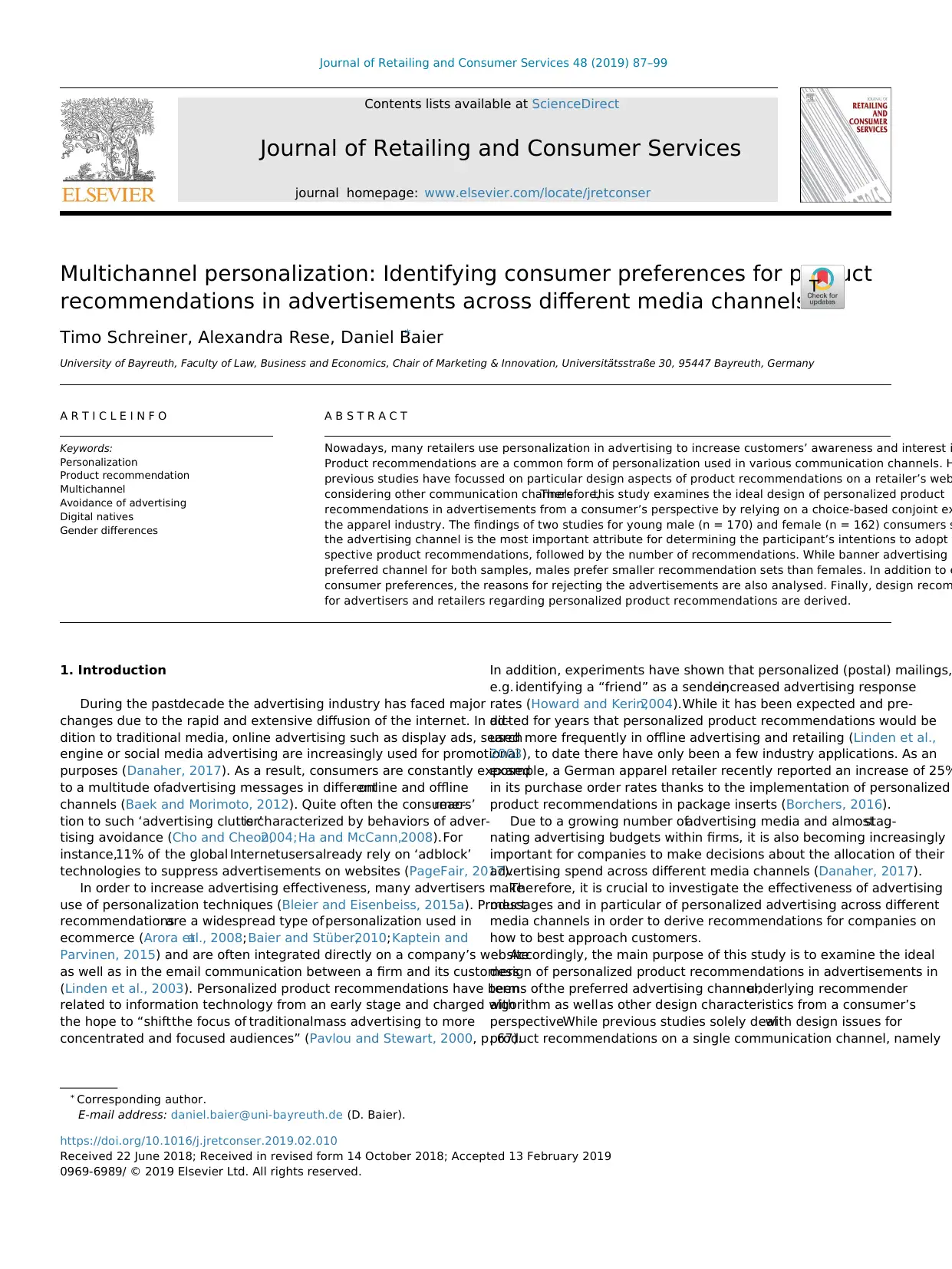
Contents lists available at ScienceDirect
Journal of Retailing and Consumer Services
journal homepage: www.elsevier.com/locate/jretconser
Multichannel personalization: Identifying consumer preferences for product
recommendations in advertisements across different media channels
Timo Schreiner, Alexandra Rese, Daniel Baier
⁎
University of Bayreuth, Faculty of Law, Business and Economics, Chair of Marketing & Innovation, Universitätsstraße 30, 95447 Bayreuth, Germany
A R T I C L E I N F O
Keywords:
Personalization
Product recommendation
Multichannel
Avoidance of advertising
Digital natives
Gender differences
A B S T R A C T
Nowadays, many retailers use personalization in advertising to increase customers’ awareness and interest i
Product recommendations are a common form of personalization used in various communication channels. H
previous studies have focussed on particular design aspects of product recommendations on a retailer’s web
considering other communication channels.Therefore,this study examines the ideal design of personalized product
recommendations in advertisements from a consumer’s perspective by relying on a choice-based conjoint ex
the apparel industry. The findings of two studies for young male (n = 170) and female (n = 162) consumers s
the advertising channel is the most important attribute for determining the participant’s intentions to adopt
spective product recommendations, followed by the number of recommendations. While banner advertising i
preferred channel for both samples, males prefer smaller recommendation sets than females. In addition to e
consumer preferences, the reasons for rejecting the advertisements are also analysed. Finally, design recom
for advertisers and retailers regarding personalized product recommendations are derived.
1. Introduction
During the pastdecade the advertising industry has faced major
changes due to the rapid and extensive diffusion of the internet. In ad-
dition to traditional media, online advertising such as display ads, search
engine or social media advertising are increasingly used for promotional
purposes (Danaher, 2017). As a result, consumers are constantly exposed
to a multitude ofadvertising messages in differentonline and offline
channels (Baek and Morimoto, 2012). Quite often the consumers’reac-
tion to such ‘advertising clutter’is characterized by behaviors of adver-
tising avoidance (Cho and Cheon,2004;Ha and McCann,2008).For
instance,11% of the global Internetusersalready rely on ‘adblock’
technologies to suppress advertisements on websites (PageFair, 2017).
In order to increase advertising effectiveness, many advertisers make
use of personalization techniques (Bleier and Eisenbeiss, 2015a). Product
recommendationsare a widespread type ofpersonalization used in
ecommerce (Arora etal., 2008;Baier and Stüber,2010;Kaptein and
Parvinen, 2015) and are often integrated directly on a company’s website
as well as in the email communication between a firm and its customers
(Linden et al., 2003). Personalized product recommendations have been
related to information technology from an early stage and charged with
the hope to “shiftthe focus of traditionalmass advertising to more
concentrated and focused audiences” (Pavlou and Stewart, 2000, p. 67).
In addition, experiments have shown that personalized (postal) mailings,
e.g. identifying a “friend” as a sender,increased advertising response
rates (Howard and Kerin,2004).While it has been expected and pre-
dicted for years that personalized product recommendations would be
used more frequently in offline advertising and retailing (Linden et al.,
2003), to date there have only been a few industry applications. As an
example, a German apparel retailer recently reported an increase of 25%
in its purchase order rates thanks to the implementation of personalized
product recommendations in package inserts (Borchers, 2016).
Due to a growing number ofadvertising media and almoststag-
nating advertising budgets within firms, it is also becoming increasingly
important for companies to make decisions about the allocation of their
advertising spend across different media channels (Danaher, 2017).
Therefore, it is crucial to investigate the effectiveness of advertising
messages and in particular of personalized advertising across different
media channels in order to derive recommendations for companies on
how to best approach customers.
Accordingly, the main purpose of this study is to examine the ideal
design of personalized product recommendations in advertisements in
terms ofthe preferred advertising channel,underlying recommender
algorithm as wellas other design characteristics from a consumer’s
perspective.While previous studies solely dealwith design issues for
product recommendations on a single communication channel, namely
https://doi.org/10.1016/j.jretconser.2019.02.010
Received 22 June 2018; Received in revised form 14 October 2018; Accepted 13 February 2019
⁎ Corresponding author.
E-mail address: daniel.baier@uni-bayreuth.de (D. Baier).
Journal of Retailing and Consumer Services 48 (2019) 87–99
0969-6989/ © 2019 Elsevier Ltd. All rights reserved.
T
Journal of Retailing and Consumer Services
journal homepage: www.elsevier.com/locate/jretconser
Multichannel personalization: Identifying consumer preferences for product
recommendations in advertisements across different media channels
Timo Schreiner, Alexandra Rese, Daniel Baier
⁎
University of Bayreuth, Faculty of Law, Business and Economics, Chair of Marketing & Innovation, Universitätsstraße 30, 95447 Bayreuth, Germany
A R T I C L E I N F O
Keywords:
Personalization
Product recommendation
Multichannel
Avoidance of advertising
Digital natives
Gender differences
A B S T R A C T
Nowadays, many retailers use personalization in advertising to increase customers’ awareness and interest i
Product recommendations are a common form of personalization used in various communication channels. H
previous studies have focussed on particular design aspects of product recommendations on a retailer’s web
considering other communication channels.Therefore,this study examines the ideal design of personalized product
recommendations in advertisements from a consumer’s perspective by relying on a choice-based conjoint ex
the apparel industry. The findings of two studies for young male (n = 170) and female (n = 162) consumers s
the advertising channel is the most important attribute for determining the participant’s intentions to adopt
spective product recommendations, followed by the number of recommendations. While banner advertising i
preferred channel for both samples, males prefer smaller recommendation sets than females. In addition to e
consumer preferences, the reasons for rejecting the advertisements are also analysed. Finally, design recom
for advertisers and retailers regarding personalized product recommendations are derived.
1. Introduction
During the pastdecade the advertising industry has faced major
changes due to the rapid and extensive diffusion of the internet. In ad-
dition to traditional media, online advertising such as display ads, search
engine or social media advertising are increasingly used for promotional
purposes (Danaher, 2017). As a result, consumers are constantly exposed
to a multitude ofadvertising messages in differentonline and offline
channels (Baek and Morimoto, 2012). Quite often the consumers’reac-
tion to such ‘advertising clutter’is characterized by behaviors of adver-
tising avoidance (Cho and Cheon,2004;Ha and McCann,2008).For
instance,11% of the global Internetusersalready rely on ‘adblock’
technologies to suppress advertisements on websites (PageFair, 2017).
In order to increase advertising effectiveness, many advertisers make
use of personalization techniques (Bleier and Eisenbeiss, 2015a). Product
recommendationsare a widespread type ofpersonalization used in
ecommerce (Arora etal., 2008;Baier and Stüber,2010;Kaptein and
Parvinen, 2015) and are often integrated directly on a company’s website
as well as in the email communication between a firm and its customers
(Linden et al., 2003). Personalized product recommendations have been
related to information technology from an early stage and charged with
the hope to “shiftthe focus of traditionalmass advertising to more
concentrated and focused audiences” (Pavlou and Stewart, 2000, p. 67).
In addition, experiments have shown that personalized (postal) mailings,
e.g. identifying a “friend” as a sender,increased advertising response
rates (Howard and Kerin,2004).While it has been expected and pre-
dicted for years that personalized product recommendations would be
used more frequently in offline advertising and retailing (Linden et al.,
2003), to date there have only been a few industry applications. As an
example, a German apparel retailer recently reported an increase of 25%
in its purchase order rates thanks to the implementation of personalized
product recommendations in package inserts (Borchers, 2016).
Due to a growing number ofadvertising media and almoststag-
nating advertising budgets within firms, it is also becoming increasingly
important for companies to make decisions about the allocation of their
advertising spend across different media channels (Danaher, 2017).
Therefore, it is crucial to investigate the effectiveness of advertising
messages and in particular of personalized advertising across different
media channels in order to derive recommendations for companies on
how to best approach customers.
Accordingly, the main purpose of this study is to examine the ideal
design of personalized product recommendations in advertisements in
terms ofthe preferred advertising channel,underlying recommender
algorithm as wellas other design characteristics from a consumer’s
perspective.While previous studies solely dealwith design issues for
product recommendations on a single communication channel, namely
https://doi.org/10.1016/j.jretconser.2019.02.010
Received 22 June 2018; Received in revised form 14 October 2018; Accepted 13 February 2019
⁎ Corresponding author.
E-mail address: daniel.baier@uni-bayreuth.de (D. Baier).
Journal of Retailing and Consumer Services 48 (2019) 87–99
0969-6989/ © 2019 Elsevier Ltd. All rights reserved.
T
Secure Best Marks with AI Grader
Need help grading? Try our AI Grader for instant feedback on your assignments.
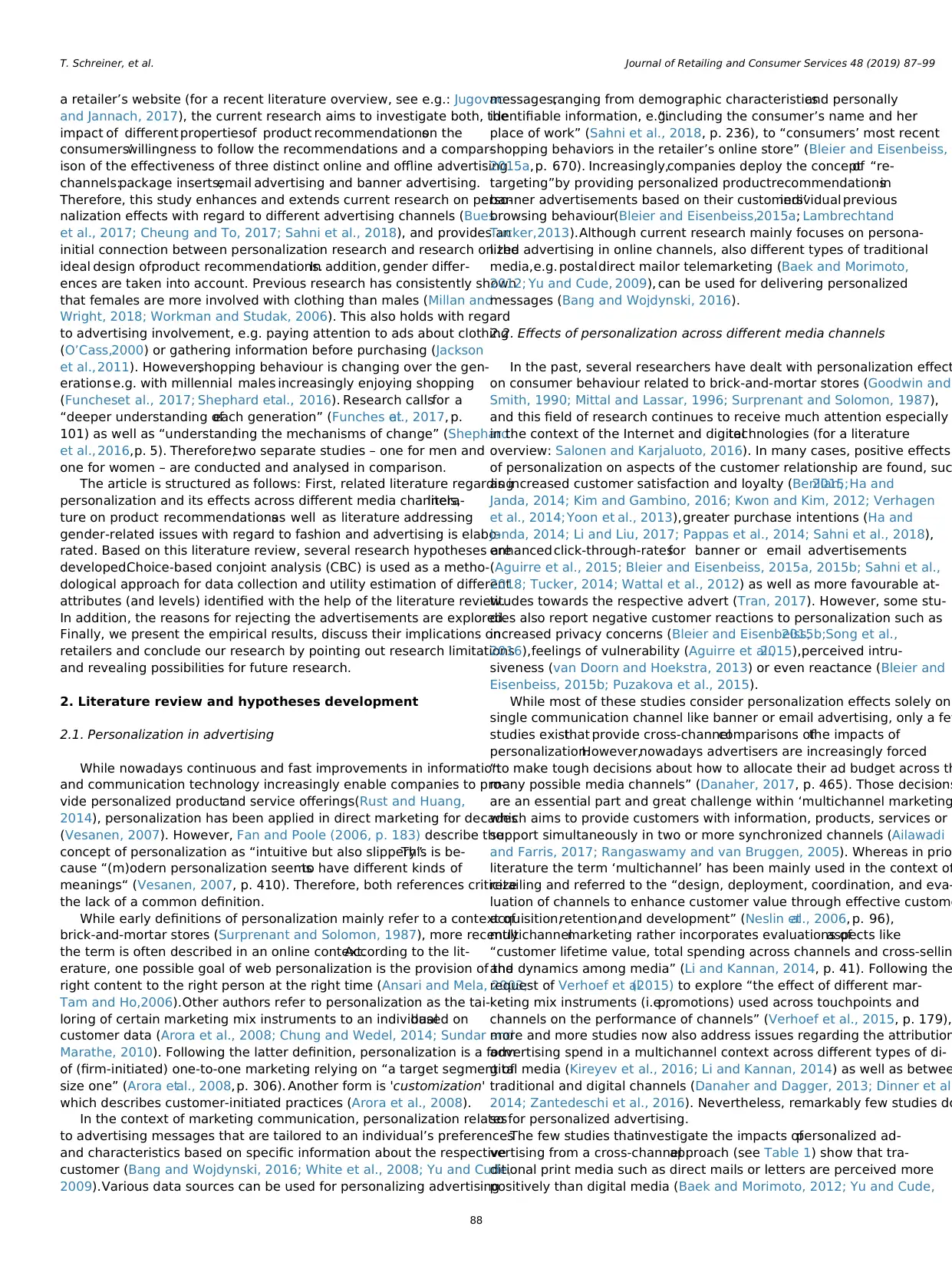
a retailer’s website (for a recent literature overview, see e.g.: Jugovac
and Jannach, 2017), the current research aims to investigate both, the
impact of different propertiesof product recommendationson the
consumers’willingness to follow the recommendations and a compar-
ison of the effectiveness of three distinct online and offline advertising
channels:package inserts,email advertising and banner advertising.
Therefore, this study enhances and extends current research on perso-
nalization effects with regard to different advertising channels (Bues
et al., 2017; Cheung and To, 2017; Sahni et al., 2018), and provides an
initial connection between personalization research and research on the
ideal design ofproduct recommendations.In addition, gender differ-
ences are taken into account. Previous research has consistently shown
that females are more involved with clothing than males (Millan and
Wright, 2018; Workman and Studak, 2006). This also holds with regard
to advertising involvement, e.g. paying attention to ads about clothing
(O’Cass,2000) or gathering information before purchasing (Jackson
et al.,2011). However,shopping behaviour is changing over the gen-
erationse.g. with millennial males increasingly enjoying shopping
(Funcheset al., 2017; Shephard etal., 2016). Research callsfor a
“deeper understanding ofeach generation” (Funches etal., 2017, p.
101) as well as “understanding the mechanisms of change” (Shephard
et al., 2016,p. 5). Therefore,two separate studies – one for men and
one for women – are conducted and analysed in comparison.
The article is structured as follows: First, related literature regarding
personalization and its effects across different media channels,litera-
ture on product recommendationsas well as literature addressing
gender-related issues with regard to fashion and advertising is elabo-
rated. Based on this literature review, several research hypotheses are
developed.Choice-based conjoint analysis (CBC) is used as a metho-
dological approach for data collection and utility estimation of different
attributes (and levels) identified with the help of the literature review.
In addition, the reasons for rejecting the advertisements are explored.
Finally, we present the empirical results, discuss their implications on
retailers and conclude our research by pointing out research limitations
and revealing possibilities for future research.
2. Literature review and hypotheses development
2.1. Personalization in advertising
While nowadays continuous and fast improvements in information
and communication technology increasingly enable companies to pro-
vide personalized productand service offerings(Rust and Huang,
2014), personalization has been applied in direct marketing for decades
(Vesanen, 2007). However, Fan and Poole (2006, p. 183) describe the
concept of personalization as “intuitive but also slippery”.This is be-
cause “(m)odern personalization seemsto have different kinds of
meanings“ (Vesanen, 2007, p. 410). Therefore, both references criticize
the lack of a common definition.
While early definitions of personalization mainly refer to a context of
brick-and-mortar stores (Surprenant and Solomon, 1987), more recently
the term is often described in an online context.According to the lit-
erature, one possible goal of web personalization is the provision of the
right content to the right person at the right time (Ansari and Mela, 2003;
Tam and Ho,2006).Other authors refer to personalization as the tai-
loring of certain marketing mix instruments to an individualbased on
customer data (Arora et al., 2008; Chung and Wedel, 2014; Sundar and
Marathe, 2010). Following the latter definition, personalization is a form
of (firm-initiated) one-to-one marketing relying on “a target segment of
size one” (Arora etal., 2008,p. 306). Another form is 'customization'
which describes customer-initiated practices (Arora et al., 2008).
In the context of marketing communication, personalization relates
to advertising messages that are tailored to an individual’s preferences
and characteristics based on specific information about the respective
customer (Bang and Wojdynski, 2016; White et al., 2008; Yu and Cude,
2009).Various data sources can be used for personalizing advertising
messages,ranging from demographic characteristicsand personally
identifiable information, e.g.“including the consumer’s name and her
place of work” (Sahni et al., 2018, p. 236), to “consumers’ most recent
shopping behaviors in the retailer’s online store” (Bleier and Eisenbeiss,
2015a, p. 670). Increasingly,companies deploy the conceptof “re-
targeting”by providing personalized productrecommendationsin
banner advertisements based on their customers’individual previous
browsing behaviour(Bleier and Eisenbeiss,2015a; Lambrechtand
Tucker,2013).Although current research mainly focuses on persona-
lized advertising in online channels, also different types of traditional
media,e.g. postaldirect mailor telemarketing (Baek and Morimoto,
2012; Yu and Cude, 2009), can be used for delivering personalized
messages (Bang and Wojdynski, 2016).
2.2. Effects of personalization across different media channels
In the past, several researchers have dealt with personalization effect
on consumer behaviour related to brick-and-mortar stores (Goodwin and
Smith, 1990; Mittal and Lassar, 1996; Surprenant and Solomon, 1987),
and this field of research continues to receive much attention especially
in the context of the Internet and digitaltechnologies (for a literature
overview: Salonen and Karjaluoto, 2016). In many cases, positive effects
of personalization on aspects of the customer relationship are found, suc
as increased customer satisfaction and loyalty (Benlian,2015;Ha and
Janda, 2014; Kim and Gambino, 2016; Kwon and Kim, 2012; Verhagen
et al., 2014; Yoon et al., 2013),greater purchase intentions (Ha and
Janda, 2014; Li and Liu, 2017; Pappas et al., 2014; Sahni et al., 2018),
enhanced click-through-ratesfor banner or email advertisements
(Aguirre et al., 2015; Bleier and Eisenbeiss, 2015a, 2015b; Sahni et al.,
2018; Tucker, 2014; Wattal et al., 2012) as well as more favourable at-
titudes towards the respective advert (Tran, 2017). However, some stu-
dies also report negative customer reactions to personalization such as
increased privacy concerns (Bleier and Eisenbeiss,2015b;Song et al.,
2016),feelings of vulnerability (Aguirre et al.,2015),perceived intru-
siveness (van Doorn and Hoekstra, 2013) or even reactance (Bleier and
Eisenbeiss, 2015b; Puzakova et al., 2015).
While most of these studies consider personalization effects solely on
single communication channel like banner or email advertising, only a few
studies existthat provide cross-channelcomparisons ofthe impacts of
personalization.However,nowadays advertisers are increasingly forced
“to make tough decisions about how to allocate their ad budget across th
many possible media channels” (Danaher, 2017, p. 465). Those decisions
are an essential part and great challenge within ‘multichannel marketing
which aims to provide customers with information, products, services or
support simultaneously in two or more synchronized channels (Ailawadi
and Farris, 2017; Rangaswamy and van Bruggen, 2005). Whereas in prio
literature the term ‘multichannel’ has been mainly used in the context of
retailing and referred to the “design, deployment, coordination, and eva-
luation of channels to enhance customer value through effective custome
acquisition,retention,and development” (Neslin etal., 2006, p. 96),
multichannelmarketing rather incorporates evaluations ofaspects like
“customer lifetime value, total spending across channels and cross-sellin
and dynamics among media” (Li and Kannan, 2014, p. 41). Following the
request of Verhoef et al.(2015) to explore “the effect of different mar-
keting mix instruments (i.e.,promotions) used across touchpoints and
channels on the performance of channels” (Verhoef et al., 2015, p. 179),
more and more studies now also address issues regarding the attribution
advertising spend in a multichannel context across different types of di-
gital media (Kireyev et al., 2016; Li and Kannan, 2014) as well as betwee
traditional and digital channels (Danaher and Dagger, 2013; Dinner et al.
2014; Zantedeschi et al., 2016). Nevertheless, remarkably few studies do
so for personalized advertising.
The few studies thatinvestigate the impacts ofpersonalized ad-
vertising from a cross-channelapproach (see Table 1) show that tra-
ditional print media such as direct mails or letters are perceived more
positively than digital media (Baek and Morimoto, 2012; Yu and Cude,
T. Schreiner, et al. Journal of Retailing and Consumer Services 48 (2019) 87–99
88
and Jannach, 2017), the current research aims to investigate both, the
impact of different propertiesof product recommendationson the
consumers’willingness to follow the recommendations and a compar-
ison of the effectiveness of three distinct online and offline advertising
channels:package inserts,email advertising and banner advertising.
Therefore, this study enhances and extends current research on perso-
nalization effects with regard to different advertising channels (Bues
et al., 2017; Cheung and To, 2017; Sahni et al., 2018), and provides an
initial connection between personalization research and research on the
ideal design ofproduct recommendations.In addition, gender differ-
ences are taken into account. Previous research has consistently shown
that females are more involved with clothing than males (Millan and
Wright, 2018; Workman and Studak, 2006). This also holds with regard
to advertising involvement, e.g. paying attention to ads about clothing
(O’Cass,2000) or gathering information before purchasing (Jackson
et al.,2011). However,shopping behaviour is changing over the gen-
erationse.g. with millennial males increasingly enjoying shopping
(Funcheset al., 2017; Shephard etal., 2016). Research callsfor a
“deeper understanding ofeach generation” (Funches etal., 2017, p.
101) as well as “understanding the mechanisms of change” (Shephard
et al., 2016,p. 5). Therefore,two separate studies – one for men and
one for women – are conducted and analysed in comparison.
The article is structured as follows: First, related literature regarding
personalization and its effects across different media channels,litera-
ture on product recommendationsas well as literature addressing
gender-related issues with regard to fashion and advertising is elabo-
rated. Based on this literature review, several research hypotheses are
developed.Choice-based conjoint analysis (CBC) is used as a metho-
dological approach for data collection and utility estimation of different
attributes (and levels) identified with the help of the literature review.
In addition, the reasons for rejecting the advertisements are explored.
Finally, we present the empirical results, discuss their implications on
retailers and conclude our research by pointing out research limitations
and revealing possibilities for future research.
2. Literature review and hypotheses development
2.1. Personalization in advertising
While nowadays continuous and fast improvements in information
and communication technology increasingly enable companies to pro-
vide personalized productand service offerings(Rust and Huang,
2014), personalization has been applied in direct marketing for decades
(Vesanen, 2007). However, Fan and Poole (2006, p. 183) describe the
concept of personalization as “intuitive but also slippery”.This is be-
cause “(m)odern personalization seemsto have different kinds of
meanings“ (Vesanen, 2007, p. 410). Therefore, both references criticize
the lack of a common definition.
While early definitions of personalization mainly refer to a context of
brick-and-mortar stores (Surprenant and Solomon, 1987), more recently
the term is often described in an online context.According to the lit-
erature, one possible goal of web personalization is the provision of the
right content to the right person at the right time (Ansari and Mela, 2003;
Tam and Ho,2006).Other authors refer to personalization as the tai-
loring of certain marketing mix instruments to an individualbased on
customer data (Arora et al., 2008; Chung and Wedel, 2014; Sundar and
Marathe, 2010). Following the latter definition, personalization is a form
of (firm-initiated) one-to-one marketing relying on “a target segment of
size one” (Arora etal., 2008,p. 306). Another form is 'customization'
which describes customer-initiated practices (Arora et al., 2008).
In the context of marketing communication, personalization relates
to advertising messages that are tailored to an individual’s preferences
and characteristics based on specific information about the respective
customer (Bang and Wojdynski, 2016; White et al., 2008; Yu and Cude,
2009).Various data sources can be used for personalizing advertising
messages,ranging from demographic characteristicsand personally
identifiable information, e.g.“including the consumer’s name and her
place of work” (Sahni et al., 2018, p. 236), to “consumers’ most recent
shopping behaviors in the retailer’s online store” (Bleier and Eisenbeiss,
2015a, p. 670). Increasingly,companies deploy the conceptof “re-
targeting”by providing personalized productrecommendationsin
banner advertisements based on their customers’individual previous
browsing behaviour(Bleier and Eisenbeiss,2015a; Lambrechtand
Tucker,2013).Although current research mainly focuses on persona-
lized advertising in online channels, also different types of traditional
media,e.g. postaldirect mailor telemarketing (Baek and Morimoto,
2012; Yu and Cude, 2009), can be used for delivering personalized
messages (Bang and Wojdynski, 2016).
2.2. Effects of personalization across different media channels
In the past, several researchers have dealt with personalization effect
on consumer behaviour related to brick-and-mortar stores (Goodwin and
Smith, 1990; Mittal and Lassar, 1996; Surprenant and Solomon, 1987),
and this field of research continues to receive much attention especially
in the context of the Internet and digitaltechnologies (for a literature
overview: Salonen and Karjaluoto, 2016). In many cases, positive effects
of personalization on aspects of the customer relationship are found, suc
as increased customer satisfaction and loyalty (Benlian,2015;Ha and
Janda, 2014; Kim and Gambino, 2016; Kwon and Kim, 2012; Verhagen
et al., 2014; Yoon et al., 2013),greater purchase intentions (Ha and
Janda, 2014; Li and Liu, 2017; Pappas et al., 2014; Sahni et al., 2018),
enhanced click-through-ratesfor banner or email advertisements
(Aguirre et al., 2015; Bleier and Eisenbeiss, 2015a, 2015b; Sahni et al.,
2018; Tucker, 2014; Wattal et al., 2012) as well as more favourable at-
titudes towards the respective advert (Tran, 2017). However, some stu-
dies also report negative customer reactions to personalization such as
increased privacy concerns (Bleier and Eisenbeiss,2015b;Song et al.,
2016),feelings of vulnerability (Aguirre et al.,2015),perceived intru-
siveness (van Doorn and Hoekstra, 2013) or even reactance (Bleier and
Eisenbeiss, 2015b; Puzakova et al., 2015).
While most of these studies consider personalization effects solely on
single communication channel like banner or email advertising, only a few
studies existthat provide cross-channelcomparisons ofthe impacts of
personalization.However,nowadays advertisers are increasingly forced
“to make tough decisions about how to allocate their ad budget across th
many possible media channels” (Danaher, 2017, p. 465). Those decisions
are an essential part and great challenge within ‘multichannel marketing
which aims to provide customers with information, products, services or
support simultaneously in two or more synchronized channels (Ailawadi
and Farris, 2017; Rangaswamy and van Bruggen, 2005). Whereas in prio
literature the term ‘multichannel’ has been mainly used in the context of
retailing and referred to the “design, deployment, coordination, and eva-
luation of channels to enhance customer value through effective custome
acquisition,retention,and development” (Neslin etal., 2006, p. 96),
multichannelmarketing rather incorporates evaluations ofaspects like
“customer lifetime value, total spending across channels and cross-sellin
and dynamics among media” (Li and Kannan, 2014, p. 41). Following the
request of Verhoef et al.(2015) to explore “the effect of different mar-
keting mix instruments (i.e.,promotions) used across touchpoints and
channels on the performance of channels” (Verhoef et al., 2015, p. 179),
more and more studies now also address issues regarding the attribution
advertising spend in a multichannel context across different types of di-
gital media (Kireyev et al., 2016; Li and Kannan, 2014) as well as betwee
traditional and digital channels (Danaher and Dagger, 2013; Dinner et al.
2014; Zantedeschi et al., 2016). Nevertheless, remarkably few studies do
so for personalized advertising.
The few studies thatinvestigate the impacts ofpersonalized ad-
vertising from a cross-channelapproach (see Table 1) show that tra-
ditional print media such as direct mails or letters are perceived more
positively than digital media (Baek and Morimoto, 2012; Yu and Cude,
T. Schreiner, et al. Journal of Retailing and Consumer Services 48 (2019) 87–99
88
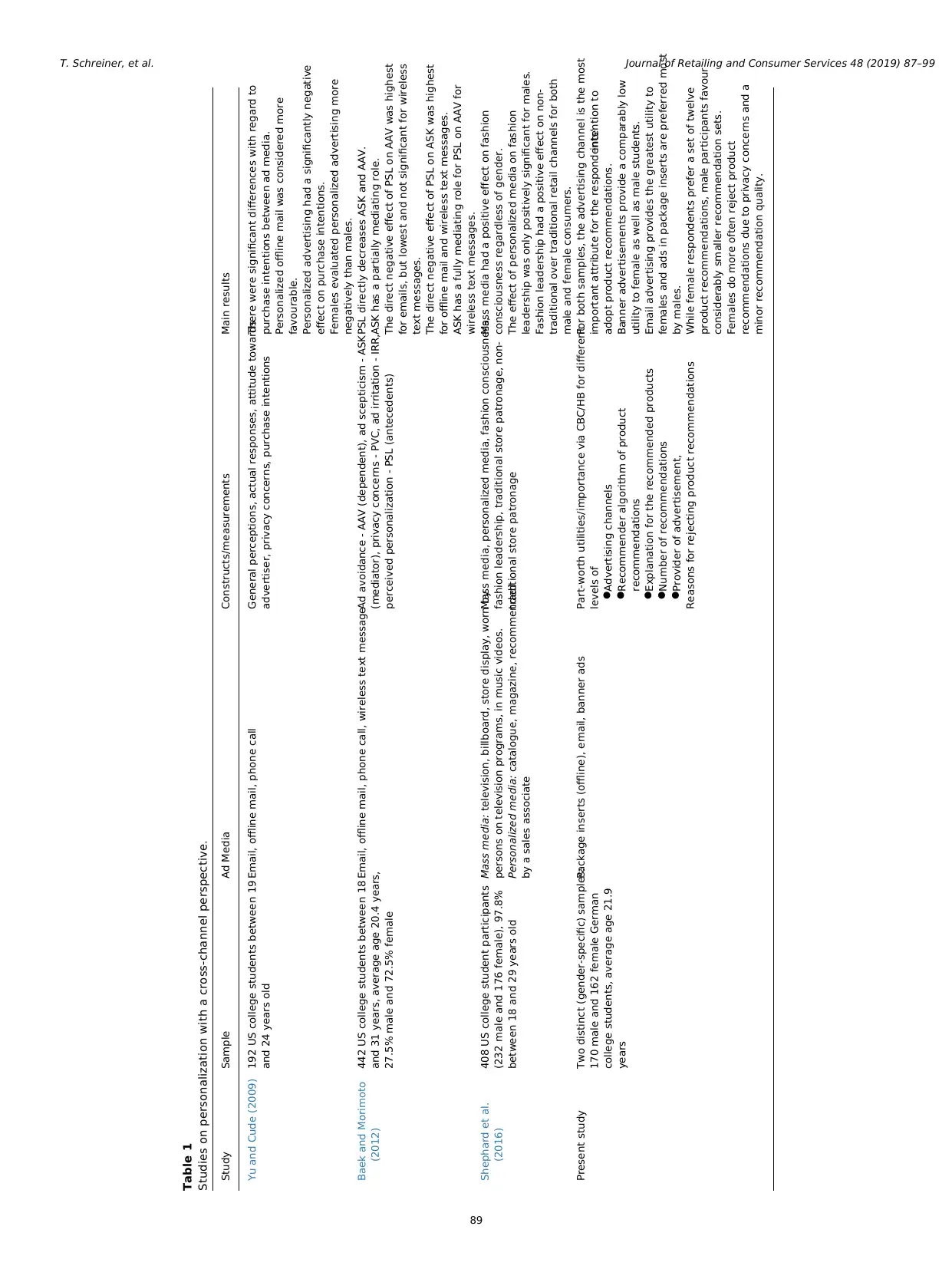
Table 1
Studies on personalization with a cross-channel perspective.
Study Sample Ad Media Constructs/measurements Main results
Yu and Cude (2009) 192 US college students between 19
and 24 years old
Email, offline mail, phone call General perceptions, actual responses, attitude towards
advertiser, privacy concerns, purchase intentions
There were significant differences with regard to
purchase intentions between ad media.
Personalized offline mail was considered more
favourable.
Personalized advertising had a significantly negative
effect on purchase intentions.
Females evaluated personalized advertising more
negatively than males.
Baek and Morimoto
(2012)
442 US college students between 18
and 31 years, average age 20.4 years,
27.5% male and 72.5% female
Email, offline mail, phone call, wireless text messageAd avoidance - AAV (dependent), ad scepticism - ASK
(mediator), privacy concerns - PVC, ad irritation - IRR,
perceived personalization - PSL (antecedents)
PSL directly decreases ASK and AAV.
ASK has a partially mediating role.
The direct negative effect of PSL on AAV was highest
for emails, but lowest and not significant for wireless
text messages.
The direct negative effect of PSL on ASK was highest
for offline mail and wireless text messages.
ASK has a fully mediating role for PSL on AAV for
wireless text messages.
Shephard et al.
(2016)
408 US college student participants
(232 male and 176 female), 97.8%
between 18 and 29 years old
Mass media: television, billboard, store display, worn by
persons on television programs, in music videos.
Personalized media: catalogue, magazine, recommended
by a sales associate
Mass media, personalized media, fashion consciousness,
fashion leadership, traditional store patronage, non-
traditional store patronage
Mass media had a positive effect on fashion
consciousness regardless of gender.
The effect of personalized media on fashion
leadership was only positively significant for males.
Fashion leadership had a positive effect on non-
traditional over traditional retail channels for both
male and female consumers.
Present study Two distinct (gender-specific) samples:
170 male and 162 female German
college students, average age 21.9
years
Package inserts (offline), email, banner ads Part-worth utilities/importance via CBC/HB for different
levels of
For both samples, the advertising channel is the most
important attribute for the respondents’intention to
adopt product recommendations.•Advertising channels
•Recommender algorithm of product
recommendations
•Explanation for the recommended products
•Number of recommendations
•Provider of advertisement,
Banner advertisements provide a comparably low
utility to female as well as male students.
Email advertising provides the greatest utility to
females and ads in package inserts are preferred most
by males.
While female respondents prefer a set of twelve
product recommendations, male participants favour
considerably smaller recommendation sets.
Reasons for rejecting product recommendations
Females do more often reject product
recommendations due to privacy concerns and a
minor recommendation quality.
T. Schreiner, et al. Journal of Retailing and Consumer Services 48 (2019) 87–99
89
Studies on personalization with a cross-channel perspective.
Study Sample Ad Media Constructs/measurements Main results
Yu and Cude (2009) 192 US college students between 19
and 24 years old
Email, offline mail, phone call General perceptions, actual responses, attitude towards
advertiser, privacy concerns, purchase intentions
There were significant differences with regard to
purchase intentions between ad media.
Personalized offline mail was considered more
favourable.
Personalized advertising had a significantly negative
effect on purchase intentions.
Females evaluated personalized advertising more
negatively than males.
Baek and Morimoto
(2012)
442 US college students between 18
and 31 years, average age 20.4 years,
27.5% male and 72.5% female
Email, offline mail, phone call, wireless text messageAd avoidance - AAV (dependent), ad scepticism - ASK
(mediator), privacy concerns - PVC, ad irritation - IRR,
perceived personalization - PSL (antecedents)
PSL directly decreases ASK and AAV.
ASK has a partially mediating role.
The direct negative effect of PSL on AAV was highest
for emails, but lowest and not significant for wireless
text messages.
The direct negative effect of PSL on ASK was highest
for offline mail and wireless text messages.
ASK has a fully mediating role for PSL on AAV for
wireless text messages.
Shephard et al.
(2016)
408 US college student participants
(232 male and 176 female), 97.8%
between 18 and 29 years old
Mass media: television, billboard, store display, worn by
persons on television programs, in music videos.
Personalized media: catalogue, magazine, recommended
by a sales associate
Mass media, personalized media, fashion consciousness,
fashion leadership, traditional store patronage, non-
traditional store patronage
Mass media had a positive effect on fashion
consciousness regardless of gender.
The effect of personalized media on fashion
leadership was only positively significant for males.
Fashion leadership had a positive effect on non-
traditional over traditional retail channels for both
male and female consumers.
Present study Two distinct (gender-specific) samples:
170 male and 162 female German
college students, average age 21.9
years
Package inserts (offline), email, banner ads Part-worth utilities/importance via CBC/HB for different
levels of
For both samples, the advertising channel is the most
important attribute for the respondents’intention to
adopt product recommendations.•Advertising channels
•Recommender algorithm of product
recommendations
•Explanation for the recommended products
•Number of recommendations
•Provider of advertisement,
Banner advertisements provide a comparably low
utility to female as well as male students.
Email advertising provides the greatest utility to
females and ads in package inserts are preferred most
by males.
While female respondents prefer a set of twelve
product recommendations, male participants favour
considerably smaller recommendation sets.
Reasons for rejecting product recommendations
Females do more often reject product
recommendations due to privacy concerns and a
minor recommendation quality.
T. Schreiner, et al. Journal of Retailing and Consumer Services 48 (2019) 87–99
89
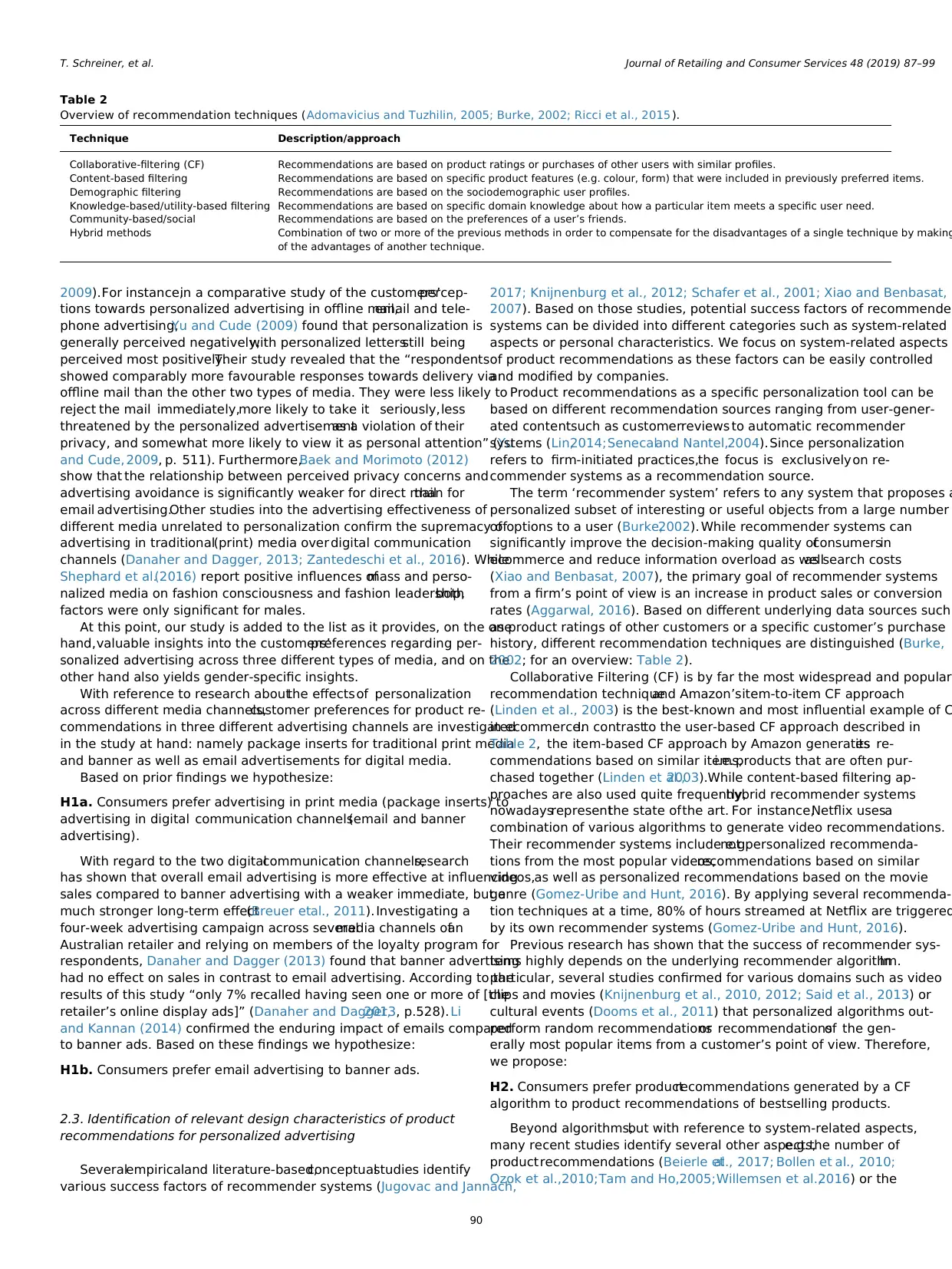
2009).For instance,in a comparative study of the customers’percep-
tions towards personalized advertising in offline mail,email and tele-
phone advertising,Yu and Cude (2009) found that personalization is
generally perceived negatively,with personalized lettersstill being
perceived most positively.Their study revealed that the “respondents
showed comparably more favourable responses towards delivery via
offline mail than the other two types of media. They were less likely to
reject the mail immediately,more likely to take it seriously,less
threatened by the personalized advertisementas a violation of their
privacy, and somewhat more likely to view it as personal attention” (Yu
and Cude, 2009, p. 511). Furthermore,Baek and Morimoto (2012)
show that the relationship between perceived privacy concerns and
advertising avoidance is significantly weaker for direct mailthan for
email advertising.Other studies into the advertising effectiveness of
different media unrelated to personalization confirm the supremacy of
advertising in traditional(print) media over digital communication
channels (Danaher and Dagger, 2013; Zantedeschi et al., 2016). While
Shephard et al.(2016) report positive influences ofmass and perso-
nalized media on fashion consciousness and fashion leadership,both
factors were only significant for males.
At this point, our study is added to the list as it provides, on the one
hand,valuable insights into the customers’preferences regarding per-
sonalized advertising across three different types of media, and on the
other hand also yields gender-specific insights.
With reference to research aboutthe effectsof personalization
across different media channels,customer preferences for product re-
commendations in three different advertising channels are investigated
in the study at hand: namely package inserts for traditional print media
and banner as well as email advertisements for digital media.
Based on prior findings we hypothesize:
H1a. Consumers prefer advertising in print media (package inserts) to
advertising in digital communication channels(email and banner
advertising).
With regard to the two digitalcommunication channels,research
has shown that overall email advertising is more effective at influencing
sales compared to banner advertising with a weaker immediate, but a
much stronger long-term effect(Breuer etal., 2011). Investigating a
four-week advertising campaign across severalmedia channels ofan
Australian retailer and relying on members of the loyalty program for
respondents, Danaher and Dagger (2013) found that banner advertising
had no effect on sales in contrast to email advertising. According to the
results of this study “only 7% recalled having seen one or more of [the
retailer’s online display ads]” (Danaher and Dagger,2013, p.528). Li
and Kannan (2014) confirmed the enduring impact of emails compared
to banner ads. Based on these findings we hypothesize:
H1b. Consumers prefer email advertising to banner ads.
2.3. Identification of relevant design characteristics of product
recommendations for personalized advertising
Severalempiricaland literature-based,conceptualstudies identify
various success factors of recommender systems (Jugovac and Jannach,
2017; Knijnenburg et al., 2012; Schafer et al., 2001; Xiao and Benbasat,
2007). Based on those studies, potential success factors of recommender
systems can be divided into different categories such as system-related
aspects or personal characteristics. We focus on system-related aspects
of product recommendations as these factors can be easily controlled
and modified by companies.
Product recommendations as a specific personalization tool can be
based on different recommendation sources ranging from user-gener-
ated contentsuch as customerreviews to automatic recommender
systems (Lin,2014;Senecaland Nantel,2004).Since personalization
refers to firm-initiated practices,the focus is exclusivelyon re-
commender systems as a recommendation source.
The term ‘recommender system’ refers to any system that proposes a
personalized subset of interesting or useful objects from a large number
of options to a user (Burke,2002). While recommender systems can
significantly improve the decision-making quality ofconsumersin
ecommerce and reduce information overload as wellas search costs
(Xiao and Benbasat, 2007), the primary goal of recommender systems
from a firm’s point of view is an increase in product sales or conversion
rates (Aggarwal, 2016). Based on different underlying data sources such
as product ratings of other customers or a specific customer’s purchase
history, different recommendation techniques are distinguished (Burke,
2002; for an overview: Table 2).
Collaborative Filtering (CF) is by far the most widespread and popular
recommendation techniqueand Amazon’sitem-to-item CF approach
(Linden et al., 2003) is the best-known and most influential example of C
in ecommerce.In contrastto the user-based CF approach described in
Table 2, the item-based CF approach by Amazon generatesits re-
commendations based on similar items,i.e. products that are often pur-
chased together (Linden et al.,2003).While content-based filtering ap-
proaches are also used quite frequently,hybrid recommender systems
nowadaysrepresentthe state ofthe art. For instance,Netflix usesa
combination of various algorithms to generate video recommendations.
Their recommender systems include e.g.not personalized recommenda-
tions from the most popular videos,recommendations based on similar
videos,as well as personalized recommendations based on the movie
genre (Gomez-Uribe and Hunt, 2016). By applying several recommenda-
tion techniques at a time, 80% of hours streamed at Netflix are triggered
by its own recommender systems (Gomez-Uribe and Hunt, 2016).
Previous research has shown that the success of recommender sys-
tems highly depends on the underlying recommender algorithm.In
particular, several studies confirmed for various domains such as video
clips and movies (Knijnenburg et al., 2010, 2012; Said et al., 2013) or
cultural events (Dooms et al., 2011) that personalized algorithms out-
perform random recommendationsor recommendationsof the gen-
erally most popular items from a customer’s point of view. Therefore,
we propose:
H2. Consumers prefer productrecommendations generated by a CF
algorithm to product recommendations of bestselling products.
Beyond algorithms,but with reference to system-related aspects,
many recent studies identify several other aspects,e.g.the number of
productrecommendations (Beierle etal., 2017; Bollen et al., 2010;
Ozok et al.,2010;Tam and Ho,2005;Willemsen et al.,2016) or the
Table 2
Overview of recommendation techniques (Adomavicius and Tuzhilin, 2005; Burke, 2002; Ricci et al., 2015 ).
Technique Description/approach
Collaborative-filtering (CF) Recommendations are based on product ratings or purchases of other users with similar profiles.
Content-based filtering Recommendations are based on specific product features (e.g. colour, form) that were included in previously preferred items.
Demographic filtering Recommendations are based on the sociodemographic user profiles.
Knowledge-based/utility-based filtering Recommendations are based on specific domain knowledge about how a particular item meets a specific user need.
Community-based/social Recommendations are based on the preferences of a user’s friends.
Hybrid methods Combination of two or more of the previous methods in order to compensate for the disadvantages of a single technique by making
of the advantages of another technique.
T. Schreiner, et al. Journal of Retailing and Consumer Services 48 (2019) 87–99
90
tions towards personalized advertising in offline mail,email and tele-
phone advertising,Yu and Cude (2009) found that personalization is
generally perceived negatively,with personalized lettersstill being
perceived most positively.Their study revealed that the “respondents
showed comparably more favourable responses towards delivery via
offline mail than the other two types of media. They were less likely to
reject the mail immediately,more likely to take it seriously,less
threatened by the personalized advertisementas a violation of their
privacy, and somewhat more likely to view it as personal attention” (Yu
and Cude, 2009, p. 511). Furthermore,Baek and Morimoto (2012)
show that the relationship between perceived privacy concerns and
advertising avoidance is significantly weaker for direct mailthan for
email advertising.Other studies into the advertising effectiveness of
different media unrelated to personalization confirm the supremacy of
advertising in traditional(print) media over digital communication
channels (Danaher and Dagger, 2013; Zantedeschi et al., 2016). While
Shephard et al.(2016) report positive influences ofmass and perso-
nalized media on fashion consciousness and fashion leadership,both
factors were only significant for males.
At this point, our study is added to the list as it provides, on the one
hand,valuable insights into the customers’preferences regarding per-
sonalized advertising across three different types of media, and on the
other hand also yields gender-specific insights.
With reference to research aboutthe effectsof personalization
across different media channels,customer preferences for product re-
commendations in three different advertising channels are investigated
in the study at hand: namely package inserts for traditional print media
and banner as well as email advertisements for digital media.
Based on prior findings we hypothesize:
H1a. Consumers prefer advertising in print media (package inserts) to
advertising in digital communication channels(email and banner
advertising).
With regard to the two digitalcommunication channels,research
has shown that overall email advertising is more effective at influencing
sales compared to banner advertising with a weaker immediate, but a
much stronger long-term effect(Breuer etal., 2011). Investigating a
four-week advertising campaign across severalmedia channels ofan
Australian retailer and relying on members of the loyalty program for
respondents, Danaher and Dagger (2013) found that banner advertising
had no effect on sales in contrast to email advertising. According to the
results of this study “only 7% recalled having seen one or more of [the
retailer’s online display ads]” (Danaher and Dagger,2013, p.528). Li
and Kannan (2014) confirmed the enduring impact of emails compared
to banner ads. Based on these findings we hypothesize:
H1b. Consumers prefer email advertising to banner ads.
2.3. Identification of relevant design characteristics of product
recommendations for personalized advertising
Severalempiricaland literature-based,conceptualstudies identify
various success factors of recommender systems (Jugovac and Jannach,
2017; Knijnenburg et al., 2012; Schafer et al., 2001; Xiao and Benbasat,
2007). Based on those studies, potential success factors of recommender
systems can be divided into different categories such as system-related
aspects or personal characteristics. We focus on system-related aspects
of product recommendations as these factors can be easily controlled
and modified by companies.
Product recommendations as a specific personalization tool can be
based on different recommendation sources ranging from user-gener-
ated contentsuch as customerreviews to automatic recommender
systems (Lin,2014;Senecaland Nantel,2004).Since personalization
refers to firm-initiated practices,the focus is exclusivelyon re-
commender systems as a recommendation source.
The term ‘recommender system’ refers to any system that proposes a
personalized subset of interesting or useful objects from a large number
of options to a user (Burke,2002). While recommender systems can
significantly improve the decision-making quality ofconsumersin
ecommerce and reduce information overload as wellas search costs
(Xiao and Benbasat, 2007), the primary goal of recommender systems
from a firm’s point of view is an increase in product sales or conversion
rates (Aggarwal, 2016). Based on different underlying data sources such
as product ratings of other customers or a specific customer’s purchase
history, different recommendation techniques are distinguished (Burke,
2002; for an overview: Table 2).
Collaborative Filtering (CF) is by far the most widespread and popular
recommendation techniqueand Amazon’sitem-to-item CF approach
(Linden et al., 2003) is the best-known and most influential example of C
in ecommerce.In contrastto the user-based CF approach described in
Table 2, the item-based CF approach by Amazon generatesits re-
commendations based on similar items,i.e. products that are often pur-
chased together (Linden et al.,2003).While content-based filtering ap-
proaches are also used quite frequently,hybrid recommender systems
nowadaysrepresentthe state ofthe art. For instance,Netflix usesa
combination of various algorithms to generate video recommendations.
Their recommender systems include e.g.not personalized recommenda-
tions from the most popular videos,recommendations based on similar
videos,as well as personalized recommendations based on the movie
genre (Gomez-Uribe and Hunt, 2016). By applying several recommenda-
tion techniques at a time, 80% of hours streamed at Netflix are triggered
by its own recommender systems (Gomez-Uribe and Hunt, 2016).
Previous research has shown that the success of recommender sys-
tems highly depends on the underlying recommender algorithm.In
particular, several studies confirmed for various domains such as video
clips and movies (Knijnenburg et al., 2010, 2012; Said et al., 2013) or
cultural events (Dooms et al., 2011) that personalized algorithms out-
perform random recommendationsor recommendationsof the gen-
erally most popular items from a customer’s point of view. Therefore,
we propose:
H2. Consumers prefer productrecommendations generated by a CF
algorithm to product recommendations of bestselling products.
Beyond algorithms,but with reference to system-related aspects,
many recent studies identify several other aspects,e.g.the number of
productrecommendations (Beierle etal., 2017; Bollen et al., 2010;
Ozok et al.,2010;Tam and Ho,2005;Willemsen et al.,2016) or the
Table 2
Overview of recommendation techniques (Adomavicius and Tuzhilin, 2005; Burke, 2002; Ricci et al., 2015 ).
Technique Description/approach
Collaborative-filtering (CF) Recommendations are based on product ratings or purchases of other users with similar profiles.
Content-based filtering Recommendations are based on specific product features (e.g. colour, form) that were included in previously preferred items.
Demographic filtering Recommendations are based on the sociodemographic user profiles.
Knowledge-based/utility-based filtering Recommendations are based on specific domain knowledge about how a particular item meets a specific user need.
Community-based/social Recommendations are based on the preferences of a user’s friends.
Hybrid methods Combination of two or more of the previous methods in order to compensate for the disadvantages of a single technique by making
of the advantages of another technique.
T. Schreiner, et al. Journal of Retailing and Consumer Services 48 (2019) 87–99
90
Secure Best Marks with AI Grader
Need help grading? Try our AI Grader for instant feedback on your assignments.
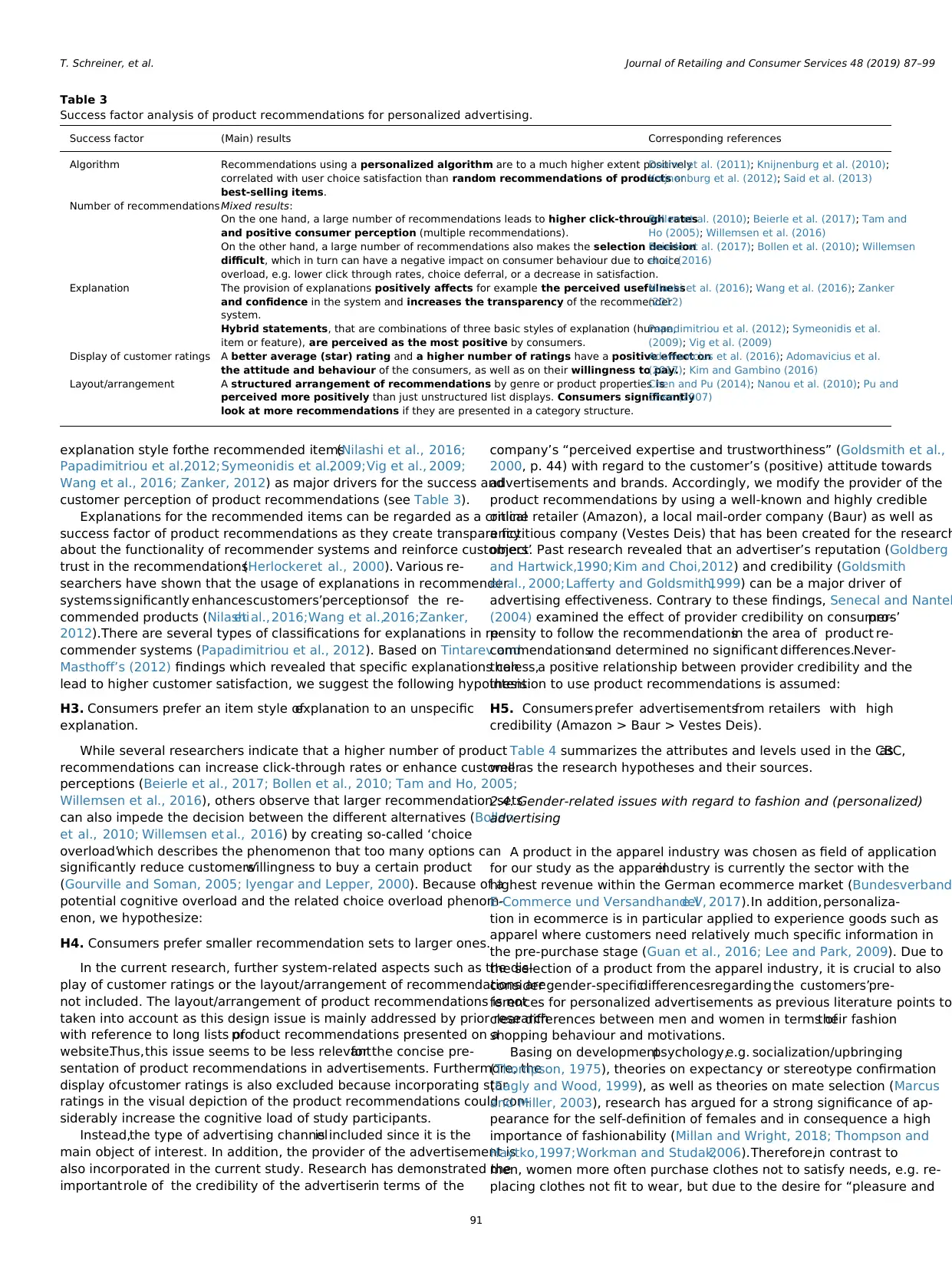
explanation style forthe recommended items(Nilashi et al., 2016;
Papadimitriou et al.,2012;Symeonidis et al.,2009;Vig et al., 2009;
Wang et al., 2016; Zanker, 2012) as major drivers for the success and
customer perception of product recommendations (see Table 3).
Explanations for the recommended items can be regarded as a critical
success factor of product recommendations as they create transparency
about the functionality of recommender systems and reinforce customers’
trust in the recommendations(Herlockeret al., 2000). Various re-
searchers have shown that the usage of explanations in recommender
systemssignificantly enhancescustomers’perceptionsof the re-
commended products (Nilashiet al., 2016;Wang et al.,2016;Zanker,
2012).There are several types of classifications for explanations in re-
commender systems (Papadimitriou et al., 2012). Based on Tintarev and
Masthoff’s (2012) findings which revealed that specific explanations can
lead to higher customer satisfaction, we suggest the following hypothesis:
H3. Consumers prefer an item style ofexplanation to an unspecific
explanation.
While several researchers indicate that a higher number of product
recommendations can increase click-through rates or enhance customer
perceptions (Beierle et al., 2017; Bollen et al., 2010; Tam and Ho, 2005;
Willemsen et al., 2016), others observe that larger recommendation sets
can also impede the decision between the different alternatives (Bollen
et al., 2010; Willemsen et al., 2016) by creating so-called ‘choice
overload’which describes the phenomenon that too many options can
significantly reduce customers’willingness to buy a certain product
(Gourville and Soman, 2005; Iyengar and Lepper, 2000). Because of a
potential cognitive overload and the related choice overload phenom-
enon, we hypothesize:
H4. Consumers prefer smaller recommendation sets to larger ones.
In the current research, further system-related aspects such as the dis-
play of customer ratings or the layout/arrangement of recommendations are
not included. The layout/arrangement of product recommendations is not
taken into account as this design issue is mainly addressed by prior research
with reference to long lists ofproduct recommendations presented on a
website.Thus,this issue seems to be less relevantfor the concise pre-
sentation of product recommendations in advertisements. Furthermore, the
display ofcustomer ratings is also excluded because incorporating star
ratings in the visual depiction of the product recommendations could con-
siderably increase the cognitive load of study participants.
Instead,the type of advertising channelis included since it is the
main object of interest. In addition, the provider of the advertisement is
also incorporated in the current study. Research has demonstrated the
importantrole of the credibility of the advertiserin terms of the
company’s “perceived expertise and trustworthiness” (Goldsmith et al.,
2000, p. 44) with regard to the customer’s (positive) attitude towards
advertisements and brands. Accordingly, we modify the provider of the
product recommendations by using a well-known and highly credible
online retailer (Amazon), a local mail-order company (Baur) as well as
a fictitious company (Vestes Deis) that has been created for the research
object. Past research revealed that an advertiser’s reputation (Goldberg
and Hartwick,1990;Kim and Choi,2012) and credibility (Goldsmith
et al., 2000;Lafferty and Goldsmith,1999) can be a major driver of
advertising effectiveness. Contrary to these findings, Senecal and Nantel
(2004) examined the effect of provider credibility on consumers’pro-
pensity to follow the recommendationsin the area of product re-
commendationsand determined no significant differences.Never-
theless,a positive relationship between provider credibility and the
intention to use product recommendations is assumed:
H5. Consumers prefer advertisementsfrom retailers with high
credibility (Amazon > Baur > Vestes Deis).
Table 4 summarizes the attributes and levels used in the CBC,as
well as the research hypotheses and their sources.
2.4. Gender-related issues with regard to fashion and (personalized)
advertising
A product in the apparel industry was chosen as field of application
for our study as the apparelindustry is currently the sector with the
highest revenue within the German ecommerce market (Bundesverband
E-Commerce und Versandhandele.V, 2017).In addition,personaliza-
tion in ecommerce is in particular applied to experience goods such as
apparel where customers need relatively much specific information in
the pre-purchase stage (Guan et al., 2016; Lee and Park, 2009). Due to
the selection of a product from the apparel industry, it is crucial to also
consider gender-specificdifferencesregarding the customers’pre-
ferences for personalized advertisements as previous literature points to
clear differences between men and women in terms oftheir fashion
shopping behaviour and motivations.
Basing on developmentpsychology,e.g. socialization/upbringing
(Thompson, 1975), theories on expectancy or stereotype confirmation
(Eagly and Wood, 1999), as well as theories on mate selection (Marcus
and Miller, 2003), research has argued for a strong significance of ap-
pearance for the self-definition of females and in consequence a high
importance of fashionability (Millan and Wright, 2018; Thompson and
Haytko,1997;Workman and Studak,2006).Therefore,in contrast to
men, women more often purchase clothes not to satisfy needs, e.g. re-
placing clothes not fit to wear, but due to the desire for “pleasure and
Table 3
Success factor analysis of product recommendations for personalized advertising.
Success factor (Main) results Corresponding references
Algorithm Recommendations using a personalized algorithm are to a much higher extent positively
correlated with user choice satisfaction than random recommendations of products or
best-selling items.
Dooms et al. (2011); Knijnenburg et al. (2010);
Knijnenburg et al. (2012); Said et al. (2013)
Number of recommendations Mixed results:
On the one hand, a large number of recommendations leads to higher click-through rates
and positive consumer perception (multiple recommendations).
Bollen et al. (2010); Beierle et al. (2017); Tam and
Ho (2005); Willemsen et al. (2016)
On the other hand, a large number of recommendations also makes the selection decision
difficult, which in turn can have a negative impact on consumer behaviour due to choice
overload, e.g. lower click through rates, choice deferral, or a decrease in satisfaction.
Beierle et al. (2017); Bollen et al. (2010); Willemsen
et al. (2016)
Explanation The provision of explanations positively affects for example the perceived usefulness
and confidence in the system and increases the transparency of the recommender
system.
Nilashi et al. (2016); Wang et al. (2016); Zanker
(2012)
Hybrid statements, that are combinations of three basic styles of explanation (human,
item or feature), are perceived as the most positive by consumers.
Papadimitriou et al. (2012); Symeonidis et al.
(2009); Vig et al. (2009)
Display of customer ratings A better average (star) rating and a higher number of ratings have a positive effect on
the attitude and behaviour of the consumers, as well as on their willingness to pay.
Adomavicius et al. (2016); Adomavicius et al.
(2017); Kim and Gambino (2016)
Layout/arrangement A structured arrangement of recommendations by genre or product properties is
perceived more positively than just unstructured list displays. Consumers significantly
look at more recommendations if they are presented in a category structure.
Chen and Pu (2014); Nanou et al. (2010); Pu and
Chen (2007)
T. Schreiner, et al. Journal of Retailing and Consumer Services 48 (2019) 87–99
91
Papadimitriou et al.,2012;Symeonidis et al.,2009;Vig et al., 2009;
Wang et al., 2016; Zanker, 2012) as major drivers for the success and
customer perception of product recommendations (see Table 3).
Explanations for the recommended items can be regarded as a critical
success factor of product recommendations as they create transparency
about the functionality of recommender systems and reinforce customers’
trust in the recommendations(Herlockeret al., 2000). Various re-
searchers have shown that the usage of explanations in recommender
systemssignificantly enhancescustomers’perceptionsof the re-
commended products (Nilashiet al., 2016;Wang et al.,2016;Zanker,
2012).There are several types of classifications for explanations in re-
commender systems (Papadimitriou et al., 2012). Based on Tintarev and
Masthoff’s (2012) findings which revealed that specific explanations can
lead to higher customer satisfaction, we suggest the following hypothesis:
H3. Consumers prefer an item style ofexplanation to an unspecific
explanation.
While several researchers indicate that a higher number of product
recommendations can increase click-through rates or enhance customer
perceptions (Beierle et al., 2017; Bollen et al., 2010; Tam and Ho, 2005;
Willemsen et al., 2016), others observe that larger recommendation sets
can also impede the decision between the different alternatives (Bollen
et al., 2010; Willemsen et al., 2016) by creating so-called ‘choice
overload’which describes the phenomenon that too many options can
significantly reduce customers’willingness to buy a certain product
(Gourville and Soman, 2005; Iyengar and Lepper, 2000). Because of a
potential cognitive overload and the related choice overload phenom-
enon, we hypothesize:
H4. Consumers prefer smaller recommendation sets to larger ones.
In the current research, further system-related aspects such as the dis-
play of customer ratings or the layout/arrangement of recommendations are
not included. The layout/arrangement of product recommendations is not
taken into account as this design issue is mainly addressed by prior research
with reference to long lists ofproduct recommendations presented on a
website.Thus,this issue seems to be less relevantfor the concise pre-
sentation of product recommendations in advertisements. Furthermore, the
display ofcustomer ratings is also excluded because incorporating star
ratings in the visual depiction of the product recommendations could con-
siderably increase the cognitive load of study participants.
Instead,the type of advertising channelis included since it is the
main object of interest. In addition, the provider of the advertisement is
also incorporated in the current study. Research has demonstrated the
importantrole of the credibility of the advertiserin terms of the
company’s “perceived expertise and trustworthiness” (Goldsmith et al.,
2000, p. 44) with regard to the customer’s (positive) attitude towards
advertisements and brands. Accordingly, we modify the provider of the
product recommendations by using a well-known and highly credible
online retailer (Amazon), a local mail-order company (Baur) as well as
a fictitious company (Vestes Deis) that has been created for the research
object. Past research revealed that an advertiser’s reputation (Goldberg
and Hartwick,1990;Kim and Choi,2012) and credibility (Goldsmith
et al., 2000;Lafferty and Goldsmith,1999) can be a major driver of
advertising effectiveness. Contrary to these findings, Senecal and Nantel
(2004) examined the effect of provider credibility on consumers’pro-
pensity to follow the recommendationsin the area of product re-
commendationsand determined no significant differences.Never-
theless,a positive relationship between provider credibility and the
intention to use product recommendations is assumed:
H5. Consumers prefer advertisementsfrom retailers with high
credibility (Amazon > Baur > Vestes Deis).
Table 4 summarizes the attributes and levels used in the CBC,as
well as the research hypotheses and their sources.
2.4. Gender-related issues with regard to fashion and (personalized)
advertising
A product in the apparel industry was chosen as field of application
for our study as the apparelindustry is currently the sector with the
highest revenue within the German ecommerce market (Bundesverband
E-Commerce und Versandhandele.V, 2017).In addition,personaliza-
tion in ecommerce is in particular applied to experience goods such as
apparel where customers need relatively much specific information in
the pre-purchase stage (Guan et al., 2016; Lee and Park, 2009). Due to
the selection of a product from the apparel industry, it is crucial to also
consider gender-specificdifferencesregarding the customers’pre-
ferences for personalized advertisements as previous literature points to
clear differences between men and women in terms oftheir fashion
shopping behaviour and motivations.
Basing on developmentpsychology,e.g. socialization/upbringing
(Thompson, 1975), theories on expectancy or stereotype confirmation
(Eagly and Wood, 1999), as well as theories on mate selection (Marcus
and Miller, 2003), research has argued for a strong significance of ap-
pearance for the self-definition of females and in consequence a high
importance of fashionability (Millan and Wright, 2018; Thompson and
Haytko,1997;Workman and Studak,2006).Therefore,in contrast to
men, women more often purchase clothes not to satisfy needs, e.g. re-
placing clothes not fit to wear, but due to the desire for “pleasure and
Table 3
Success factor analysis of product recommendations for personalized advertising.
Success factor (Main) results Corresponding references
Algorithm Recommendations using a personalized algorithm are to a much higher extent positively
correlated with user choice satisfaction than random recommendations of products or
best-selling items.
Dooms et al. (2011); Knijnenburg et al. (2010);
Knijnenburg et al. (2012); Said et al. (2013)
Number of recommendations Mixed results:
On the one hand, a large number of recommendations leads to higher click-through rates
and positive consumer perception (multiple recommendations).
Bollen et al. (2010); Beierle et al. (2017); Tam and
Ho (2005); Willemsen et al. (2016)
On the other hand, a large number of recommendations also makes the selection decision
difficult, which in turn can have a negative impact on consumer behaviour due to choice
overload, e.g. lower click through rates, choice deferral, or a decrease in satisfaction.
Beierle et al. (2017); Bollen et al. (2010); Willemsen
et al. (2016)
Explanation The provision of explanations positively affects for example the perceived usefulness
and confidence in the system and increases the transparency of the recommender
system.
Nilashi et al. (2016); Wang et al. (2016); Zanker
(2012)
Hybrid statements, that are combinations of three basic styles of explanation (human,
item or feature), are perceived as the most positive by consumers.
Papadimitriou et al. (2012); Symeonidis et al.
(2009); Vig et al. (2009)
Display of customer ratings A better average (star) rating and a higher number of ratings have a positive effect on
the attitude and behaviour of the consumers, as well as on their willingness to pay.
Adomavicius et al. (2016); Adomavicius et al.
(2017); Kim and Gambino (2016)
Layout/arrangement A structured arrangement of recommendations by genre or product properties is
perceived more positively than just unstructured list displays. Consumers significantly
look at more recommendations if they are presented in a category structure.
Chen and Pu (2014); Nanou et al. (2010); Pu and
Chen (2007)
T. Schreiner, et al. Journal of Retailing and Consumer Services 48 (2019) 87–99
91
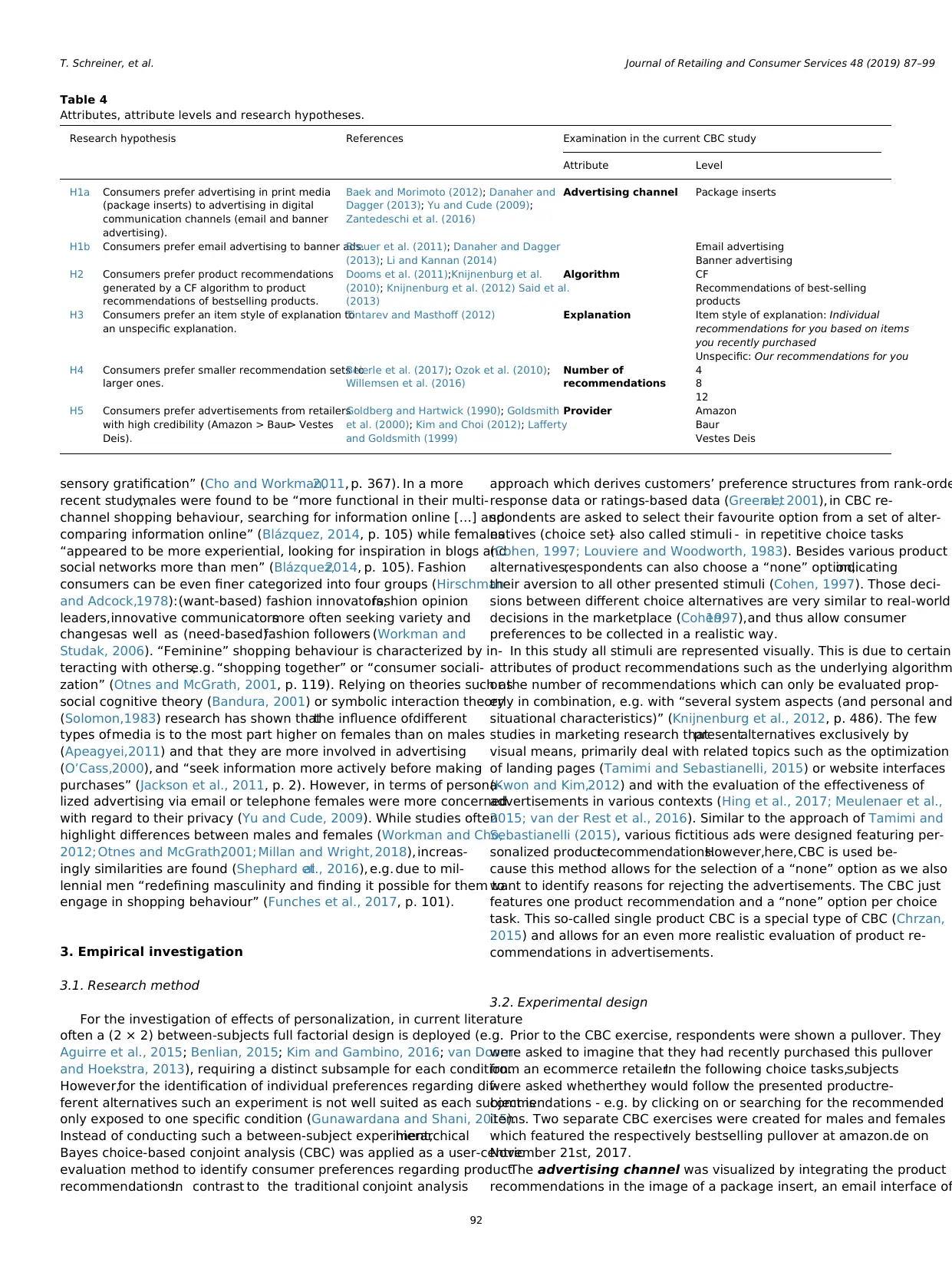
sensory gratification” (Cho and Workman,2011, p. 367). In a more
recent study,males were found to be “more functional in their multi-
channel shopping behaviour, searching for information online […] and
comparing information online” (Blázquez, 2014, p. 105) while females
“appeared to be more experiential, looking for inspiration in blogs and
social networks more than men” (Blázquez,2014, p. 105). Fashion
consumers can be even finer categorized into four groups (Hirschman
and Adcock,1978):(want-based) fashion innovators,fashion opinion
leaders,innovative communicatorsmore often seeking variety and
changesas well as (need-based)fashion followers (Workman and
Studak, 2006). “Feminine” shopping behaviour is characterized by in-
teracting with others,e.g. “shopping together” or “consumer sociali-
zation” (Otnes and McGrath, 2001, p. 119). Relying on theories such as
social cognitive theory (Bandura, 2001) or symbolic interaction theory
(Solomon,1983) research has shown thatthe influence ofdifferent
types ofmedia is to the most part higher on females than on males
(Apeagyei,2011) and that they are more involved in advertising
(O’Cass,2000), and “seek information more actively before making
purchases” (Jackson et al., 2011, p. 2). However, in terms of persona-
lized advertising via email or telephone females were more concerned
with regard to their privacy (Yu and Cude, 2009). While studies often
highlight differences between males and females (Workman and Cho,
2012; Otnes and McGrath,2001; Millan and Wright, 2018), increas-
ingly similarities are found (Shephard etal., 2016), e.g. due to mil-
lennial men “redefining masculinity and finding it possible for them to
engage in shopping behaviour” (Funches et al., 2017, p. 101).
3. Empirical investigation
3.1. Research method
For the investigation of effects of personalization, in current literature
often a (2 × 2) between-subjects full factorial design is deployed (e.g.
Aguirre et al., 2015; Benlian, 2015; Kim and Gambino, 2016; van Doorn
and Hoekstra, 2013), requiring a distinct subsample for each condition.
However,for the identification of individual preferences regarding dif-
ferent alternatives such an experiment is not well suited as each subject is
only exposed to one specific condition (Gunawardana and Shani, 2015).
Instead of conducting such a between-subject experiment,hierarchical
Bayes choice-based conjoint analysis (CBC) was applied as a user-centric
evaluation method to identify consumer preferences regarding product
recommendations.In contrast to the traditional conjoint analysis
approach which derives customers’ preference structures from rank-orde
response data or ratings-based data (Green etal., 2001), in CBC re-
spondents are asked to select their favourite option from a set of alter-
natives (choice set)– also called stimuli - in repetitive choice tasks
(Cohen, 1997; Louviere and Woodworth, 1983). Besides various product
alternatives,respondents can also choose a “none” option,indicating
their aversion to all other presented stimuli (Cohen, 1997). Those deci-
sions between different choice alternatives are very similar to real-world
decisions in the marketplace (Cohen,1997),and thus allow consumer
preferences to be collected in a realistic way.
In this study all stimuli are represented visually. This is due to certain
attributes of product recommendations such as the underlying algorithm
or the number of recommendations which can only be evaluated prop-
erly in combination, e.g. with “several system aspects (and personal and
situational characteristics)” (Knijnenburg et al., 2012, p. 486). The few
studies in marketing research thatpresentalternatives exclusively by
visual means, primarily deal with related topics such as the optimization
of landing pages (Tamimi and Sebastianelli, 2015) or website interfaces
(Kwon and Kim,2012) and with the evaluation of the effectiveness of
advertisements in various contexts (Hing et al., 2017; Meulenaer et al.,
2015; van der Rest et al., 2016). Similar to the approach of Tamimi and
Sebastianelli (2015), various fictitious ads were designed featuring per-
sonalized productrecommendations.However,here,CBC is used be-
cause this method allows for the selection of a “none” option as we also
want to identify reasons for rejecting the advertisements. The CBC just
features one product recommendation and a “none” option per choice
task. This so-called single product CBC is a special type of CBC (Chrzan,
2015) and allows for an even more realistic evaluation of product re-
commendations in advertisements.
3.2. Experimental design
Prior to the CBC exercise, respondents were shown a pullover. They
were asked to imagine that they had recently purchased this pullover
from an ecommerce retailer.In the following choice tasks,subjects
were asked whetherthey would follow the presented productre-
commendations - e.g. by clicking on or searching for the recommended
items. Two separate CBC exercises were created for males and females
which featured the respectively bestselling pullover at amazon.de on
November 21st, 2017.
The advertising channel was visualized by integrating the product
recommendations in the image of a package insert, an email interface of
Table 4
Attributes, attribute levels and research hypotheses.
Research hypothesis References Examination in the current CBC study
Attribute Level
H1a Consumers prefer advertising in print media
(package inserts) to advertising in digital
communication channels (email and banner
advertising).
Baek and Morimoto (2012); Danaher and
Dagger (2013); Yu and Cude (2009);
Zantedeschi et al. (2016)
Advertising channel Package inserts
H1b Consumers prefer email advertising to banner ads.Breuer et al. (2011); Danaher and Dagger
(2013); Li and Kannan (2014)
Email advertising
Banner advertising
H2 Consumers prefer product recommendations
generated by a CF algorithm to product
recommendations of bestselling products.
Dooms et al. (2011);Knijnenburg et al.
(2010); Knijnenburg et al. (2012) Said et al.
(2013)
Algorithm CF
Recommendations of best-selling
products
H3 Consumers prefer an item style of explanation to
an unspecific explanation.
Tintarev and Masthoff (2012) Explanation Item style of explanation: Individual
recommendations for you based on items
you recently purchased
Unspecific: Our recommendations for you
H4 Consumers prefer smaller recommendation sets to
larger ones.
Beierle et al. (2017); Ozok et al. (2010);
Willemsen et al. (2016)
Number of
recommendations
4
8
12
H5 Consumers prefer advertisements from retailers
with high credibility (Amazon > Baur> Vestes
Deis).
Goldberg and Hartwick (1990); Goldsmith
et al. (2000); Kim and Choi (2012); Lafferty
and Goldsmith (1999)
Provider Amazon
Baur
Vestes Deis
T. Schreiner, et al. Journal of Retailing and Consumer Services 48 (2019) 87–99
92
recent study,males were found to be “more functional in their multi-
channel shopping behaviour, searching for information online […] and
comparing information online” (Blázquez, 2014, p. 105) while females
“appeared to be more experiential, looking for inspiration in blogs and
social networks more than men” (Blázquez,2014, p. 105). Fashion
consumers can be even finer categorized into four groups (Hirschman
and Adcock,1978):(want-based) fashion innovators,fashion opinion
leaders,innovative communicatorsmore often seeking variety and
changesas well as (need-based)fashion followers (Workman and
Studak, 2006). “Feminine” shopping behaviour is characterized by in-
teracting with others,e.g. “shopping together” or “consumer sociali-
zation” (Otnes and McGrath, 2001, p. 119). Relying on theories such as
social cognitive theory (Bandura, 2001) or symbolic interaction theory
(Solomon,1983) research has shown thatthe influence ofdifferent
types ofmedia is to the most part higher on females than on males
(Apeagyei,2011) and that they are more involved in advertising
(O’Cass,2000), and “seek information more actively before making
purchases” (Jackson et al., 2011, p. 2). However, in terms of persona-
lized advertising via email or telephone females were more concerned
with regard to their privacy (Yu and Cude, 2009). While studies often
highlight differences between males and females (Workman and Cho,
2012; Otnes and McGrath,2001; Millan and Wright, 2018), increas-
ingly similarities are found (Shephard etal., 2016), e.g. due to mil-
lennial men “redefining masculinity and finding it possible for them to
engage in shopping behaviour” (Funches et al., 2017, p. 101).
3. Empirical investigation
3.1. Research method
For the investigation of effects of personalization, in current literature
often a (2 × 2) between-subjects full factorial design is deployed (e.g.
Aguirre et al., 2015; Benlian, 2015; Kim and Gambino, 2016; van Doorn
and Hoekstra, 2013), requiring a distinct subsample for each condition.
However,for the identification of individual preferences regarding dif-
ferent alternatives such an experiment is not well suited as each subject is
only exposed to one specific condition (Gunawardana and Shani, 2015).
Instead of conducting such a between-subject experiment,hierarchical
Bayes choice-based conjoint analysis (CBC) was applied as a user-centric
evaluation method to identify consumer preferences regarding product
recommendations.In contrast to the traditional conjoint analysis
approach which derives customers’ preference structures from rank-orde
response data or ratings-based data (Green etal., 2001), in CBC re-
spondents are asked to select their favourite option from a set of alter-
natives (choice set)– also called stimuli - in repetitive choice tasks
(Cohen, 1997; Louviere and Woodworth, 1983). Besides various product
alternatives,respondents can also choose a “none” option,indicating
their aversion to all other presented stimuli (Cohen, 1997). Those deci-
sions between different choice alternatives are very similar to real-world
decisions in the marketplace (Cohen,1997),and thus allow consumer
preferences to be collected in a realistic way.
In this study all stimuli are represented visually. This is due to certain
attributes of product recommendations such as the underlying algorithm
or the number of recommendations which can only be evaluated prop-
erly in combination, e.g. with “several system aspects (and personal and
situational characteristics)” (Knijnenburg et al., 2012, p. 486). The few
studies in marketing research thatpresentalternatives exclusively by
visual means, primarily deal with related topics such as the optimization
of landing pages (Tamimi and Sebastianelli, 2015) or website interfaces
(Kwon and Kim,2012) and with the evaluation of the effectiveness of
advertisements in various contexts (Hing et al., 2017; Meulenaer et al.,
2015; van der Rest et al., 2016). Similar to the approach of Tamimi and
Sebastianelli (2015), various fictitious ads were designed featuring per-
sonalized productrecommendations.However,here,CBC is used be-
cause this method allows for the selection of a “none” option as we also
want to identify reasons for rejecting the advertisements. The CBC just
features one product recommendation and a “none” option per choice
task. This so-called single product CBC is a special type of CBC (Chrzan,
2015) and allows for an even more realistic evaluation of product re-
commendations in advertisements.
3.2. Experimental design
Prior to the CBC exercise, respondents were shown a pullover. They
were asked to imagine that they had recently purchased this pullover
from an ecommerce retailer.In the following choice tasks,subjects
were asked whetherthey would follow the presented productre-
commendations - e.g. by clicking on or searching for the recommended
items. Two separate CBC exercises were created for males and females
which featured the respectively bestselling pullover at amazon.de on
November 21st, 2017.
The advertising channel was visualized by integrating the product
recommendations in the image of a package insert, an email interface of
Table 4
Attributes, attribute levels and research hypotheses.
Research hypothesis References Examination in the current CBC study
Attribute Level
H1a Consumers prefer advertising in print media
(package inserts) to advertising in digital
communication channels (email and banner
advertising).
Baek and Morimoto (2012); Danaher and
Dagger (2013); Yu and Cude (2009);
Zantedeschi et al. (2016)
Advertising channel Package inserts
H1b Consumers prefer email advertising to banner ads.Breuer et al. (2011); Danaher and Dagger
(2013); Li and Kannan (2014)
Email advertising
Banner advertising
H2 Consumers prefer product recommendations
generated by a CF algorithm to product
recommendations of bestselling products.
Dooms et al. (2011);Knijnenburg et al.
(2010); Knijnenburg et al. (2012) Said et al.
(2013)
Algorithm CF
Recommendations of best-selling
products
H3 Consumers prefer an item style of explanation to
an unspecific explanation.
Tintarev and Masthoff (2012) Explanation Item style of explanation: Individual
recommendations for you based on items
you recently purchased
Unspecific: Our recommendations for you
H4 Consumers prefer smaller recommendation sets to
larger ones.
Beierle et al. (2017); Ozok et al. (2010);
Willemsen et al. (2016)
Number of
recommendations
4
8
12
H5 Consumers prefer advertisements from retailers
with high credibility (Amazon > Baur> Vestes
Deis).
Goldberg and Hartwick (1990); Goldsmith
et al. (2000); Kim and Choi (2012); Lafferty
and Goldsmith (1999)
Provider Amazon
Baur
Vestes Deis
T. Schreiner, et al. Journal of Retailing and Consumer Services 48 (2019) 87–99
92
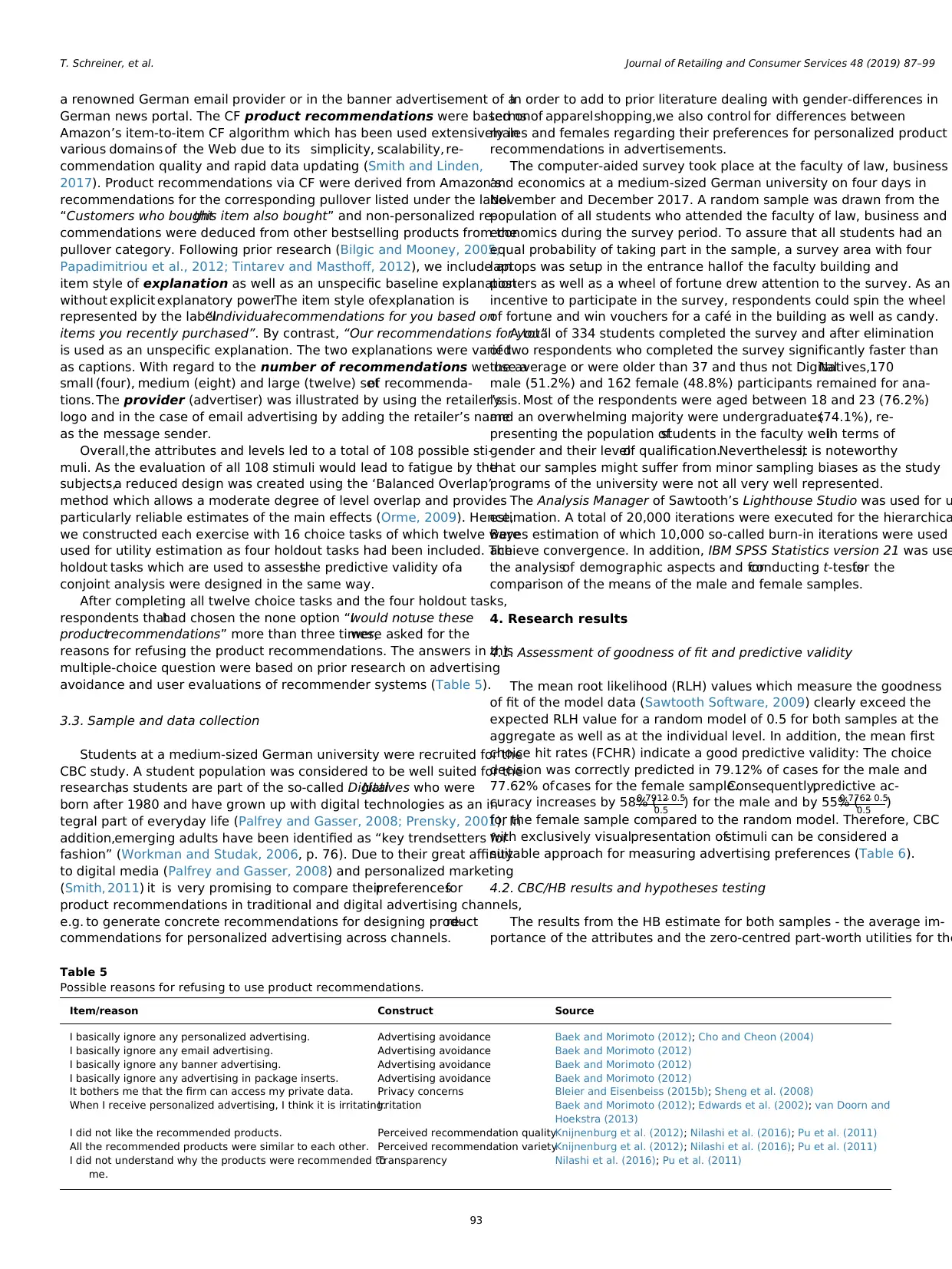
a renowned German email provider or in the banner advertisement of a
German news portal. The CF product recommendations were based on
Amazon’s item-to-item CF algorithm which has been used extensively in
various domains of the Web due to its simplicity, scalability, re-
commendation quality and rapid data updating (Smith and Linden,
2017). Product recommendations via CF were derived from Amazon’s
recommendations for the corresponding pullover listed under the label
“Customers who boughtthis item also bought” and non-personalized re-
commendations were deduced from other bestselling products from the
pullover category. Following prior research (Bilgic and Mooney, 2005;
Papadimitriou et al., 2012; Tintarev and Masthoff, 2012), we include an
item style of explanation as well as an unspecific baseline explanation
without explicit explanatory power.The item style ofexplanation is
represented by the label“Individualrecommendations for you based on
items you recently purchased”. By contrast, “Our recommendations for you”
is used as an unspecific explanation. The two explanations were varied
as captions. With regard to the number of recommendations we use a
small (four), medium (eight) and large (twelve) setof recommenda-
tions. The provider (advertiser) was illustrated by using the retailer’s
logo and in the case of email advertising by adding the retailer’s name
as the message sender.
Overall,the attributes and levels led to a total of 108 possible sti-
muli. As the evaluation of all 108 stimuli would lead to fatigue by the
subjects,a reduced design was created using the ‘Balanced Overlap’
method which allows a moderate degree of level overlap and provides
particularly reliable estimates of the main effects (Orme, 2009). Hence,
we constructed each exercise with 16 choice tasks of which twelve were
used for utility estimation as four holdout tasks had been included. The
holdout tasks which are used to assessthe predictive validity ofa
conjoint analysis were designed in the same way.
After completing all twelve choice tasks and the four holdout tasks,
respondents thathad chosen the none option “Iwould notuse these
productrecommendations” more than three times,were asked for the
reasons for refusing the product recommendations. The answers in this
multiple-choice question were based on prior research on advertising
avoidance and user evaluations of recommender systems (Table 5).
3.3. Sample and data collection
Students at a medium-sized German university were recruited for the
CBC study. A student population was considered to be well suited for the
research,as students are part of the so-called DigitalNatives who were
born after 1980 and have grown up with digital technologies as an in-
tegral part of everyday life (Palfrey and Gasser, 2008; Prensky, 2001). In
addition,emerging adults have been identified as “key trendsetters for
fashion” (Workman and Studak, 2006, p. 76). Due to their great affinity
to digital media (Palfrey and Gasser, 2008) and personalized marketing
(Smith, 2011) it is very promising to compare theirpreferencesfor
product recommendations in traditional and digital advertising channels,
e.g. to generate concrete recommendations for designing productre-
commendations for personalized advertising across channels.
In order to add to prior literature dealing with gender-differences in
terms of apparelshopping,we also control for differences between
males and females regarding their preferences for personalized product
recommendations in advertisements.
The computer-aided survey took place at the faculty of law, business
and economics at a medium-sized German university on four days in
November and December 2017. A random sample was drawn from the
population of all students who attended the faculty of law, business and
economics during the survey period. To assure that all students had an
equal probability of taking part in the sample, a survey area with four
laptops was setup in the entrance hallof the faculty building and
posters as well as a wheel of fortune drew attention to the survey. As an
incentive to participate in the survey, respondents could spin the wheel
of fortune and win vouchers for a café in the building as well as candy.
A total of 334 students completed the survey and after elimination
of two respondents who completed the survey significantly faster than
the average or were older than 37 and thus not DigitalNatives,170
male (51.2%) and 162 female (48.8%) participants remained for ana-
lysis. Most of the respondents were aged between 18 and 23 (76.2%)
and an overwhelming majority were undergraduates(74.1%), re-
presenting the population ofstudents in the faculty wellin terms of
gender and their levelof qualification.Nevertheless,it is noteworthy
that our samples might suffer from minor sampling biases as the study
programs of the university were not all very well represented.
The Analysis Manager of Sawtooth’s Lighthouse Studio was used for u
estimation. A total of 20,000 iterations were executed for the hierarchica
Bayes estimation of which 10,000 so-called burn-in iterations were used
achieve convergence. In addition, IBM SPSS Statistics version 21 was use
the analysisof demographic aspects and forconducting t-testsfor the
comparison of the means of the male and female samples.
4. Research results
4.1. Assessment of goodness of fit and predictive validity
The mean root likelihood (RLH) values which measure the goodness
of fit of the model data (Sawtooth Software, 2009) clearly exceed the
expected RLH value for a random model of 0.5 for both samples at the
aggregate as well as at the individual level. In addition, the mean first
choice hit rates (FCHR) indicate a good predictive validity: The choice
decision was correctly predicted in 79.12% of cases for the male and
77.62% ofcases for the female sample.Consequently,predictive ac-
curacy increases by 58% (0.7912 0.5
0.5 ) for the male and by 55% (0.7762 0.5
0.5 )
for the female sample compared to the random model. Therefore, CBC
with exclusively visualpresentation ofstimuli can be considered a
suitable approach for measuring advertising preferences (Table 6).
4.2. CBC/HB results and hypotheses testing
The results from the HB estimate for both samples - the average im-
portance of the attributes and the zero-centred part-worth utilities for the
Table 5
Possible reasons for refusing to use product recommendations.
Item/reason Construct Source
I basically ignore any personalized advertising. Advertising avoidance Baek and Morimoto (2012); Cho and Cheon (2004)
I basically ignore any email advertising. Advertising avoidance Baek and Morimoto (2012)
I basically ignore any banner advertising. Advertising avoidance Baek and Morimoto (2012)
I basically ignore any advertising in package inserts. Advertising avoidance Baek and Morimoto (2012)
It bothers me that the firm can access my private data. Privacy concerns Bleier and Eisenbeiss (2015b); Sheng et al. (2008)
When I receive personalized advertising, I think it is irritating.Irritation Baek and Morimoto (2012); Edwards et al. (2002); van Doorn and
Hoekstra (2013)
I did not like the recommended products. Perceived recommendation qualityKnijnenburg et al. (2012); Nilashi et al. (2016); Pu et al. (2011)
All the recommended products were similar to each other. Perceived recommendation varietyKnijnenburg et al. (2012); Nilashi et al. (2016); Pu et al. (2011)
I did not understand why the products were recommended to
me.
Transparency Nilashi et al. (2016); Pu et al. (2011)
T. Schreiner, et al. Journal of Retailing and Consumer Services 48 (2019) 87–99
93
German news portal. The CF product recommendations were based on
Amazon’s item-to-item CF algorithm which has been used extensively in
various domains of the Web due to its simplicity, scalability, re-
commendation quality and rapid data updating (Smith and Linden,
2017). Product recommendations via CF were derived from Amazon’s
recommendations for the corresponding pullover listed under the label
“Customers who boughtthis item also bought” and non-personalized re-
commendations were deduced from other bestselling products from the
pullover category. Following prior research (Bilgic and Mooney, 2005;
Papadimitriou et al., 2012; Tintarev and Masthoff, 2012), we include an
item style of explanation as well as an unspecific baseline explanation
without explicit explanatory power.The item style ofexplanation is
represented by the label“Individualrecommendations for you based on
items you recently purchased”. By contrast, “Our recommendations for you”
is used as an unspecific explanation. The two explanations were varied
as captions. With regard to the number of recommendations we use a
small (four), medium (eight) and large (twelve) setof recommenda-
tions. The provider (advertiser) was illustrated by using the retailer’s
logo and in the case of email advertising by adding the retailer’s name
as the message sender.
Overall,the attributes and levels led to a total of 108 possible sti-
muli. As the evaluation of all 108 stimuli would lead to fatigue by the
subjects,a reduced design was created using the ‘Balanced Overlap’
method which allows a moderate degree of level overlap and provides
particularly reliable estimates of the main effects (Orme, 2009). Hence,
we constructed each exercise with 16 choice tasks of which twelve were
used for utility estimation as four holdout tasks had been included. The
holdout tasks which are used to assessthe predictive validity ofa
conjoint analysis were designed in the same way.
After completing all twelve choice tasks and the four holdout tasks,
respondents thathad chosen the none option “Iwould notuse these
productrecommendations” more than three times,were asked for the
reasons for refusing the product recommendations. The answers in this
multiple-choice question were based on prior research on advertising
avoidance and user evaluations of recommender systems (Table 5).
3.3. Sample and data collection
Students at a medium-sized German university were recruited for the
CBC study. A student population was considered to be well suited for the
research,as students are part of the so-called DigitalNatives who were
born after 1980 and have grown up with digital technologies as an in-
tegral part of everyday life (Palfrey and Gasser, 2008; Prensky, 2001). In
addition,emerging adults have been identified as “key trendsetters for
fashion” (Workman and Studak, 2006, p. 76). Due to their great affinity
to digital media (Palfrey and Gasser, 2008) and personalized marketing
(Smith, 2011) it is very promising to compare theirpreferencesfor
product recommendations in traditional and digital advertising channels,
e.g. to generate concrete recommendations for designing productre-
commendations for personalized advertising across channels.
In order to add to prior literature dealing with gender-differences in
terms of apparelshopping,we also control for differences between
males and females regarding their preferences for personalized product
recommendations in advertisements.
The computer-aided survey took place at the faculty of law, business
and economics at a medium-sized German university on four days in
November and December 2017. A random sample was drawn from the
population of all students who attended the faculty of law, business and
economics during the survey period. To assure that all students had an
equal probability of taking part in the sample, a survey area with four
laptops was setup in the entrance hallof the faculty building and
posters as well as a wheel of fortune drew attention to the survey. As an
incentive to participate in the survey, respondents could spin the wheel
of fortune and win vouchers for a café in the building as well as candy.
A total of 334 students completed the survey and after elimination
of two respondents who completed the survey significantly faster than
the average or were older than 37 and thus not DigitalNatives,170
male (51.2%) and 162 female (48.8%) participants remained for ana-
lysis. Most of the respondents were aged between 18 and 23 (76.2%)
and an overwhelming majority were undergraduates(74.1%), re-
presenting the population ofstudents in the faculty wellin terms of
gender and their levelof qualification.Nevertheless,it is noteworthy
that our samples might suffer from minor sampling biases as the study
programs of the university were not all very well represented.
The Analysis Manager of Sawtooth’s Lighthouse Studio was used for u
estimation. A total of 20,000 iterations were executed for the hierarchica
Bayes estimation of which 10,000 so-called burn-in iterations were used
achieve convergence. In addition, IBM SPSS Statistics version 21 was use
the analysisof demographic aspects and forconducting t-testsfor the
comparison of the means of the male and female samples.
4. Research results
4.1. Assessment of goodness of fit and predictive validity
The mean root likelihood (RLH) values which measure the goodness
of fit of the model data (Sawtooth Software, 2009) clearly exceed the
expected RLH value for a random model of 0.5 for both samples at the
aggregate as well as at the individual level. In addition, the mean first
choice hit rates (FCHR) indicate a good predictive validity: The choice
decision was correctly predicted in 79.12% of cases for the male and
77.62% ofcases for the female sample.Consequently,predictive ac-
curacy increases by 58% (0.7912 0.5
0.5 ) for the male and by 55% (0.7762 0.5
0.5 )
for the female sample compared to the random model. Therefore, CBC
with exclusively visualpresentation ofstimuli can be considered a
suitable approach for measuring advertising preferences (Table 6).
4.2. CBC/HB results and hypotheses testing
The results from the HB estimate for both samples - the average im-
portance of the attributes and the zero-centred part-worth utilities for the
Table 5
Possible reasons for refusing to use product recommendations.
Item/reason Construct Source
I basically ignore any personalized advertising. Advertising avoidance Baek and Morimoto (2012); Cho and Cheon (2004)
I basically ignore any email advertising. Advertising avoidance Baek and Morimoto (2012)
I basically ignore any banner advertising. Advertising avoidance Baek and Morimoto (2012)
I basically ignore any advertising in package inserts. Advertising avoidance Baek and Morimoto (2012)
It bothers me that the firm can access my private data. Privacy concerns Bleier and Eisenbeiss (2015b); Sheng et al. (2008)
When I receive personalized advertising, I think it is irritating.Irritation Baek and Morimoto (2012); Edwards et al. (2002); van Doorn and
Hoekstra (2013)
I did not like the recommended products. Perceived recommendation qualityKnijnenburg et al. (2012); Nilashi et al. (2016); Pu et al. (2011)
All the recommended products were similar to each other. Perceived recommendation varietyKnijnenburg et al. (2012); Nilashi et al. (2016); Pu et al. (2011)
I did not understand why the products were recommended to
me.
Transparency Nilashi et al. (2016); Pu et al. (2011)
T. Schreiner, et al. Journal of Retailing and Consumer Services 48 (2019) 87–99
93
Paraphrase This Document
Need a fresh take? Get an instant paraphrase of this document with our AI Paraphraser
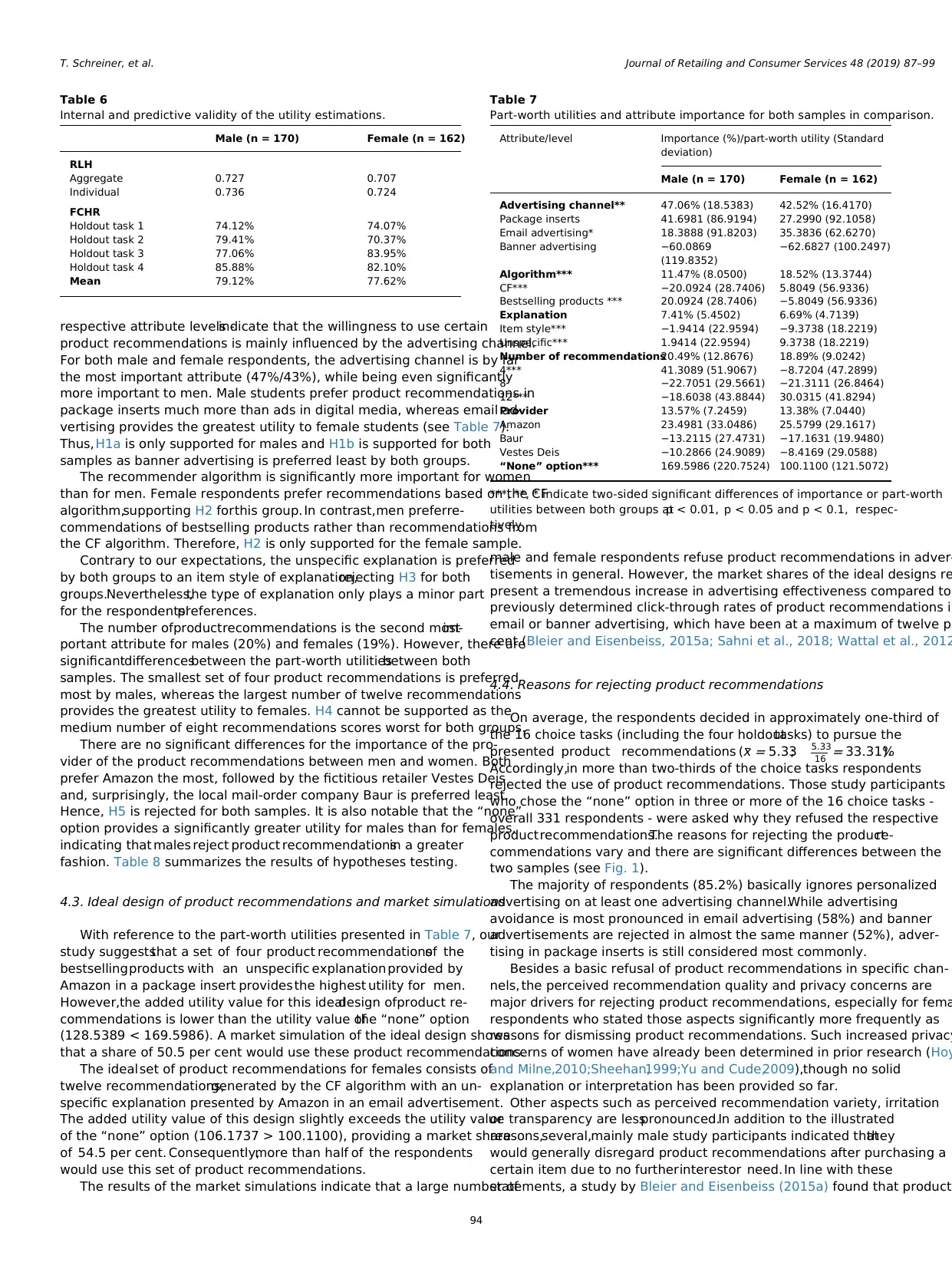
respective attribute levels -indicate that the willingness to use certain
product recommendations is mainly influenced by the advertising channel.
For both male and female respondents, the advertising channel is by far
the most important attribute (47%/43%), while being even significantly
more important to men. Male students prefer product recommendations in
package inserts much more than ads in digital media, whereas email ad-
vertising provides the greatest utility to female students (see Table 7).
Thus,H1a is only supported for males and H1b is supported for both
samples as banner advertising is preferred least by both groups.
The recommender algorithm is significantly more important for women
than for men. Female respondents prefer recommendations based on the CF
algorithm,supporting H2 forthis group. In contrast,men preferre-
commendations of bestselling products rather than recommendations from
the CF algorithm. Therefore, H2 is only supported for the female sample.
Contrary to our expectations, the unspecific explanation is preferred
by both groups to an item style of explanation,rejecting H3 for both
groups.Nevertheless,the type of explanation only plays a minor part
for the respondents’preferences.
The number ofproductrecommendations is the second mostim-
portant attribute for males (20%) and females (19%). However, there are
significantdifferencesbetween the part-worth utilitiesbetween both
samples. The smallest set of four product recommendations is preferred
most by males, whereas the largest number of twelve recommendations
provides the greatest utility to females. H4 cannot be supported as the
medium number of eight recommendations scores worst for both groups.
There are no significant differences for the importance of the pro-
vider of the product recommendations between men and women. Both
prefer Amazon the most, followed by the fictitious retailer Vestes Deis
and, surprisingly, the local mail-order company Baur is preferred least.
Hence, H5 is rejected for both samples. It is also notable that the “none”
option provides a significantly greater utility for males than for females,
indicating that males reject product recommendationsin a greater
fashion. Table 8 summarizes the results of hypotheses testing.
4.3. Ideal design of product recommendations and market simulations
With reference to the part-worth utilities presented in Table 7, our
study suggeststhat a set of four product recommendationsof the
bestsellingproducts with an unspecific explanation provided by
Amazon in a package insert provides the highest utility for men.
However,the added utility value for this idealdesign ofproduct re-
commendations is lower than the utility value ofthe “none” option
(128.5389 < 169.5986). A market simulation of the ideal design shows
that a share of 50.5 per cent would use these product recommendations.
The idealset of product recommendations for females consists of
twelve recommendations,generated by the CF algorithm with an un-
specific explanation presented by Amazon in an email advertisement.
The added utility value of this design slightly exceeds the utility value
of the “none” option (106.1737 > 100.1100), providing a market share
of 54.5 per cent. Consequently,more than half of the respondents
would use this set of product recommendations.
The results of the market simulations indicate that a large number of
male and female respondents refuse product recommendations in adver-
tisements in general. However, the market shares of the ideal designs re
present a tremendous increase in advertising effectiveness compared to
previously determined click-through rates of product recommendations in
email or banner advertising, which have been at a maximum of twelve pe
cent (Bleier and Eisenbeiss, 2015a; Sahni et al., 2018; Wattal et al., 2012
4.4. Reasons for rejecting product recommendations
On average, the respondents decided in approximately one-third of
the 16 choice tasks (including the four holdouttasks) to pursue the
presented product recommendations (
=x 5.33;
= 33.31%
5.33
16 ).
Accordingly,in more than two-thirds of the choice tasks respondents
rejected the use of product recommendations. Those study participants
who chose the “none” option in three or more of the 16 choice tasks -
overall 331 respondents - were asked why they refused the respective
productrecommendations.The reasons for rejecting the productre-
commendations vary and there are significant differences between the
two samples (see Fig. 1).
The majority of respondents (85.2%) basically ignores personalized
advertising on at least one advertising channel.While advertising
avoidance is most pronounced in email advertising (58%) and banner
advertisements are rejected in almost the same manner (52%), adver-
tising in package inserts is still considered most commonly.
Besides a basic refusal of product recommendations in specific chan-
nels, the perceived recommendation quality and privacy concerns are
major drivers for rejecting product recommendations, especially for fema
respondents who stated those aspects significantly more frequently as
reasons for dismissing product recommendations. Such increased privacy
concerns of women have already been determined in prior research (Hoy
and Milne,2010;Sheehan,1999;Yu and Cude,2009),though no solid
explanation or interpretation has been provided so far.
Other aspects such as perceived recommendation variety, irritation
or transparency are lesspronounced.In addition to the illustrated
reasons,several,mainly male study participants indicated thatthey
would generally disregard product recommendations after purchasing a
certain item due to no furtherinterestor need.In line with these
statements, a study by Bleier and Eisenbeiss (2015a) found that product
Table 6
Internal and predictive validity of the utility estimations.
Male (n = 170) Female (n = 162)
RLH
Aggregate 0.727 0.707
Individual 0.736 0.724
FCHR
Holdout task 1 74.12% 74.07%
Holdout task 2 79.41% 70.37%
Holdout task 3 77.06% 83.95%
Holdout task 4 85.88% 82.10%
Mean 79.12% 77.62%
Table 7
Part-worth utilities and attribute importance for both samples in comparison.
Attribute/level Importance (%)/part-worth utility (Standard
deviation)
Male (n = 170) Female (n = 162)
Advertising channel** 47.06% (18.5383) 42.52% (16.4170)
Package inserts 41.6981 (86.9194) 27.2990 (92.1058)
Email advertising* 18.3888 (91.8203) 35.3836 (62.6270)
Banner advertising −60.0869
(119.8352)
−62.6827 (100.2497)
Algorithm*** 11.47% (8.0500) 18.52% (13.3744)
CF*** −20.0924 (28.7406) 5.8049 (56.9336)
Bestselling products *** 20.0924 (28.7406) −5.8049 (56.9336)
Explanation 7.41% (5.4502) 6.69% (4.7139)
Item style*** −1.9414 (22.9594) −9.3738 (18.2219)
Unspecific*** 1.9414 (22.9594) 9.3738 (18.2219)
Number of recommendations20.49% (12.8676) 18.89% (9.0242)
4*** 41.3089 (51.9067) −8.7204 (47.2899)
8 −22.7051 (29.5661) −21.3111 (26.8464)
12*** −18.6038 (43.8844) 30.0315 (41.8294)
Provider 13.57% (7.2459) 13.38% (7.0440)
Amazon 23.4981 (33.0486) 25.5799 (29.1617)
Baur −13.2115 (27.4731) −17.1631 (19.9480)
Vestes Deis −10.2866 (24.9089) −8.4169 (29.0588)
“None” option*** 169.5986 (220.7524) 100.1100 (121.5072)
***, **, * indicate two-sided significant differences of importance or part-worth
utilities between both groups atp < 0.01, p < 0.05 and p < 0.1, respec-
tively.
T. Schreiner, et al. Journal of Retailing and Consumer Services 48 (2019) 87–99
94
product recommendations is mainly influenced by the advertising channel.
For both male and female respondents, the advertising channel is by far
the most important attribute (47%/43%), while being even significantly
more important to men. Male students prefer product recommendations in
package inserts much more than ads in digital media, whereas email ad-
vertising provides the greatest utility to female students (see Table 7).
Thus,H1a is only supported for males and H1b is supported for both
samples as banner advertising is preferred least by both groups.
The recommender algorithm is significantly more important for women
than for men. Female respondents prefer recommendations based on the CF
algorithm,supporting H2 forthis group. In contrast,men preferre-
commendations of bestselling products rather than recommendations from
the CF algorithm. Therefore, H2 is only supported for the female sample.
Contrary to our expectations, the unspecific explanation is preferred
by both groups to an item style of explanation,rejecting H3 for both
groups.Nevertheless,the type of explanation only plays a minor part
for the respondents’preferences.
The number ofproductrecommendations is the second mostim-
portant attribute for males (20%) and females (19%). However, there are
significantdifferencesbetween the part-worth utilitiesbetween both
samples. The smallest set of four product recommendations is preferred
most by males, whereas the largest number of twelve recommendations
provides the greatest utility to females. H4 cannot be supported as the
medium number of eight recommendations scores worst for both groups.
There are no significant differences for the importance of the pro-
vider of the product recommendations between men and women. Both
prefer Amazon the most, followed by the fictitious retailer Vestes Deis
and, surprisingly, the local mail-order company Baur is preferred least.
Hence, H5 is rejected for both samples. It is also notable that the “none”
option provides a significantly greater utility for males than for females,
indicating that males reject product recommendationsin a greater
fashion. Table 8 summarizes the results of hypotheses testing.
4.3. Ideal design of product recommendations and market simulations
With reference to the part-worth utilities presented in Table 7, our
study suggeststhat a set of four product recommendationsof the
bestsellingproducts with an unspecific explanation provided by
Amazon in a package insert provides the highest utility for men.
However,the added utility value for this idealdesign ofproduct re-
commendations is lower than the utility value ofthe “none” option
(128.5389 < 169.5986). A market simulation of the ideal design shows
that a share of 50.5 per cent would use these product recommendations.
The idealset of product recommendations for females consists of
twelve recommendations,generated by the CF algorithm with an un-
specific explanation presented by Amazon in an email advertisement.
The added utility value of this design slightly exceeds the utility value
of the “none” option (106.1737 > 100.1100), providing a market share
of 54.5 per cent. Consequently,more than half of the respondents
would use this set of product recommendations.
The results of the market simulations indicate that a large number of
male and female respondents refuse product recommendations in adver-
tisements in general. However, the market shares of the ideal designs re
present a tremendous increase in advertising effectiveness compared to
previously determined click-through rates of product recommendations in
email or banner advertising, which have been at a maximum of twelve pe
cent (Bleier and Eisenbeiss, 2015a; Sahni et al., 2018; Wattal et al., 2012
4.4. Reasons for rejecting product recommendations
On average, the respondents decided in approximately one-third of
the 16 choice tasks (including the four holdouttasks) to pursue the
presented product recommendations (
=x 5.33;
= 33.31%
5.33
16 ).
Accordingly,in more than two-thirds of the choice tasks respondents
rejected the use of product recommendations. Those study participants
who chose the “none” option in three or more of the 16 choice tasks -
overall 331 respondents - were asked why they refused the respective
productrecommendations.The reasons for rejecting the productre-
commendations vary and there are significant differences between the
two samples (see Fig. 1).
The majority of respondents (85.2%) basically ignores personalized
advertising on at least one advertising channel.While advertising
avoidance is most pronounced in email advertising (58%) and banner
advertisements are rejected in almost the same manner (52%), adver-
tising in package inserts is still considered most commonly.
Besides a basic refusal of product recommendations in specific chan-
nels, the perceived recommendation quality and privacy concerns are
major drivers for rejecting product recommendations, especially for fema
respondents who stated those aspects significantly more frequently as
reasons for dismissing product recommendations. Such increased privacy
concerns of women have already been determined in prior research (Hoy
and Milne,2010;Sheehan,1999;Yu and Cude,2009),though no solid
explanation or interpretation has been provided so far.
Other aspects such as perceived recommendation variety, irritation
or transparency are lesspronounced.In addition to the illustrated
reasons,several,mainly male study participants indicated thatthey
would generally disregard product recommendations after purchasing a
certain item due to no furtherinterestor need.In line with these
statements, a study by Bleier and Eisenbeiss (2015a) found that product
Table 6
Internal and predictive validity of the utility estimations.
Male (n = 170) Female (n = 162)
RLH
Aggregate 0.727 0.707
Individual 0.736 0.724
FCHR
Holdout task 1 74.12% 74.07%
Holdout task 2 79.41% 70.37%
Holdout task 3 77.06% 83.95%
Holdout task 4 85.88% 82.10%
Mean 79.12% 77.62%
Table 7
Part-worth utilities and attribute importance for both samples in comparison.
Attribute/level Importance (%)/part-worth utility (Standard
deviation)
Male (n = 170) Female (n = 162)
Advertising channel** 47.06% (18.5383) 42.52% (16.4170)
Package inserts 41.6981 (86.9194) 27.2990 (92.1058)
Email advertising* 18.3888 (91.8203) 35.3836 (62.6270)
Banner advertising −60.0869
(119.8352)
−62.6827 (100.2497)
Algorithm*** 11.47% (8.0500) 18.52% (13.3744)
CF*** −20.0924 (28.7406) 5.8049 (56.9336)
Bestselling products *** 20.0924 (28.7406) −5.8049 (56.9336)
Explanation 7.41% (5.4502) 6.69% (4.7139)
Item style*** −1.9414 (22.9594) −9.3738 (18.2219)
Unspecific*** 1.9414 (22.9594) 9.3738 (18.2219)
Number of recommendations20.49% (12.8676) 18.89% (9.0242)
4*** 41.3089 (51.9067) −8.7204 (47.2899)
8 −22.7051 (29.5661) −21.3111 (26.8464)
12*** −18.6038 (43.8844) 30.0315 (41.8294)
Provider 13.57% (7.2459) 13.38% (7.0440)
Amazon 23.4981 (33.0486) 25.5799 (29.1617)
Baur −13.2115 (27.4731) −17.1631 (19.9480)
Vestes Deis −10.2866 (24.9089) −8.4169 (29.0588)
“None” option*** 169.5986 (220.7524) 100.1100 (121.5072)
***, **, * indicate two-sided significant differences of importance or part-worth
utilities between both groups atp < 0.01, p < 0.05 and p < 0.1, respec-
tively.
T. Schreiner, et al. Journal of Retailing and Consumer Services 48 (2019) 87–99
94
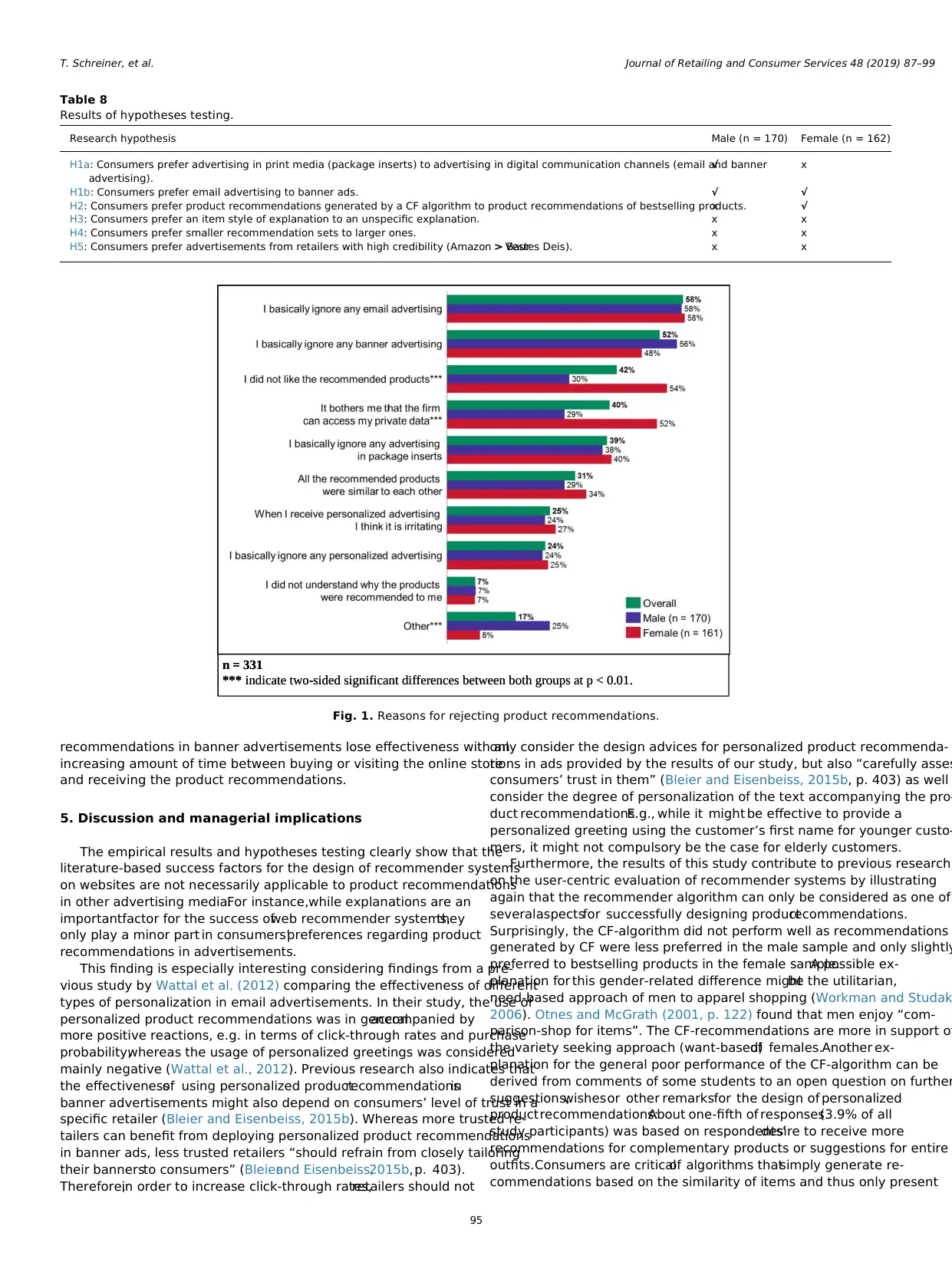
recommendations in banner advertisements lose effectiveness with an
increasing amount of time between buying or visiting the online store
and receiving the product recommendations.
5. Discussion and managerial implications
The empirical results and hypotheses testing clearly show that the
literature-based success factors for the design of recommender systems
on websites are not necessarily applicable to product recommendations
in other advertising media.For instance,while explanations are an
importantfactor for the success ofweb recommender systems,they
only play a minor part in consumers’preferences regarding product
recommendations in advertisements.
This finding is especially interesting considering findings from a pre-
vious study by Wattal et al. (2012) comparing the effectiveness of different
types of personalization in email advertisements. In their study, the use of
personalized product recommendations was in generalaccompanied by
more positive reactions, e.g. in terms of click-through rates and purchase
probability,whereas the usage of personalized greetings was considered
mainly negative (Wattal et al., 2012). Previous research also indicates that
the effectivenessof using personalized productrecommendationsin
banner advertisements might also depend on consumers’ level of trust in a
specific retailer (Bleier and Eisenbeiss, 2015b). Whereas more trusted re-
tailers can benefit from deploying personalized product recommendations
in banner ads, less trusted retailers “should refrain from closely tailoring
their bannersto consumers” (Bleierand Eisenbeiss,2015b,p. 403).
Therefore,in order to increase click-through rates,retailers should not
only consider the design advices for personalized product recommenda-
tions in ads provided by the results of our study, but also “carefully asses
consumers’ trust in them” (Bleier and Eisenbeiss, 2015b, p. 403) as well
consider the degree of personalization of the text accompanying the pro-
duct recommendations.E.g., while it might be effective to provide a
personalized greeting using the customer’s first name for younger custo-
mers, it might not compulsory be the case for elderly customers.
Furthermore, the results of this study contribute to previous research
on the user-centric evaluation of recommender systems by illustrating
again that the recommender algorithm can only be considered as one of
severalaspectsfor successfully designing productrecommendations.
Surprisingly, the CF-algorithm did not perform well as recommendations
generated by CF were less preferred in the male sample and only slightly
preferred to bestselling products in the female sample.A possible ex-
planation for this gender-related difference mightbe the utilitarian,
need-based approach of men to apparel shopping (Workman and Studak
2006). Otnes and McGrath (2001, p. 122) found that men enjoy “com-
parison-shop for items”. The CF-recommendations are more in support of
the variety seeking approach (want-based)of females.Another ex-
planation for the general poor performance of the CF-algorithm can be
derived from comments of some students to an open question on further
suggestions,wishesor other remarksfor the design of personalized
productrecommendations:About one-fifth of responses(3.9% of all
study participants) was based on respondents'desire to receive more
recommendations for complementary products or suggestions for entire
outfits.Consumers are criticalof algorithms thatsimply generate re-
commendations based on the similarity of items and thus only present
Table 8
Results of hypotheses testing.
Research hypothesis Male (n = 170) Female (n = 162)
H1a: Consumers prefer advertising in print media (package inserts) to advertising in digital communication channels (email and banner
advertising).
√ x
H1b: Consumers prefer email advertising to banner ads. √ √
H2: Consumers prefer product recommendations generated by a CF algorithm to product recommendations of bestselling products.x √
H3: Consumers prefer an item style of explanation to an unspecific explanation. x x
H4: Consumers prefer smaller recommendation sets to larger ones. x x
H5: Consumers prefer advertisements from retailers with high credibility (Amazon > Baur> Vestes Deis). x x
n = 331
*** indicate two-sided significant differences between both groups at p < 0.01.
n = 331
*** indicate two-sided significant differences between both groups at p < 0.01.
Fig. 1. Reasons for rejecting product recommendations.
T. Schreiner, et al. Journal of Retailing and Consumer Services 48 (2019) 87–99
95
increasing amount of time between buying or visiting the online store
and receiving the product recommendations.
5. Discussion and managerial implications
The empirical results and hypotheses testing clearly show that the
literature-based success factors for the design of recommender systems
on websites are not necessarily applicable to product recommendations
in other advertising media.For instance,while explanations are an
importantfactor for the success ofweb recommender systems,they
only play a minor part in consumers’preferences regarding product
recommendations in advertisements.
This finding is especially interesting considering findings from a pre-
vious study by Wattal et al. (2012) comparing the effectiveness of different
types of personalization in email advertisements. In their study, the use of
personalized product recommendations was in generalaccompanied by
more positive reactions, e.g. in terms of click-through rates and purchase
probability,whereas the usage of personalized greetings was considered
mainly negative (Wattal et al., 2012). Previous research also indicates that
the effectivenessof using personalized productrecommendationsin
banner advertisements might also depend on consumers’ level of trust in a
specific retailer (Bleier and Eisenbeiss, 2015b). Whereas more trusted re-
tailers can benefit from deploying personalized product recommendations
in banner ads, less trusted retailers “should refrain from closely tailoring
their bannersto consumers” (Bleierand Eisenbeiss,2015b,p. 403).
Therefore,in order to increase click-through rates,retailers should not
only consider the design advices for personalized product recommenda-
tions in ads provided by the results of our study, but also “carefully asses
consumers’ trust in them” (Bleier and Eisenbeiss, 2015b, p. 403) as well
consider the degree of personalization of the text accompanying the pro-
duct recommendations.E.g., while it might be effective to provide a
personalized greeting using the customer’s first name for younger custo-
mers, it might not compulsory be the case for elderly customers.
Furthermore, the results of this study contribute to previous research
on the user-centric evaluation of recommender systems by illustrating
again that the recommender algorithm can only be considered as one of
severalaspectsfor successfully designing productrecommendations.
Surprisingly, the CF-algorithm did not perform well as recommendations
generated by CF were less preferred in the male sample and only slightly
preferred to bestselling products in the female sample.A possible ex-
planation for this gender-related difference mightbe the utilitarian,
need-based approach of men to apparel shopping (Workman and Studak
2006). Otnes and McGrath (2001, p. 122) found that men enjoy “com-
parison-shop for items”. The CF-recommendations are more in support of
the variety seeking approach (want-based)of females.Another ex-
planation for the general poor performance of the CF-algorithm can be
derived from comments of some students to an open question on further
suggestions,wishesor other remarksfor the design of personalized
productrecommendations:About one-fifth of responses(3.9% of all
study participants) was based on respondents'desire to receive more
recommendations for complementary products or suggestions for entire
outfits.Consumers are criticalof algorithms thatsimply generate re-
commendations based on the similarity of items and thus only present
Table 8
Results of hypotheses testing.
Research hypothesis Male (n = 170) Female (n = 162)
H1a: Consumers prefer advertising in print media (package inserts) to advertising in digital communication channels (email and banner
advertising).
√ x
H1b: Consumers prefer email advertising to banner ads. √ √
H2: Consumers prefer product recommendations generated by a CF algorithm to product recommendations of bestselling products.x √
H3: Consumers prefer an item style of explanation to an unspecific explanation. x x
H4: Consumers prefer smaller recommendation sets to larger ones. x x
H5: Consumers prefer advertisements from retailers with high credibility (Amazon > Baur> Vestes Deis). x x
n = 331
*** indicate two-sided significant differences between both groups at p < 0.01.
n = 331
*** indicate two-sided significant differences between both groups at p < 0.01.
Fig. 1. Reasons for rejecting product recommendations.
T. Schreiner, et al. Journal of Retailing and Consumer Services 48 (2019) 87–99
95
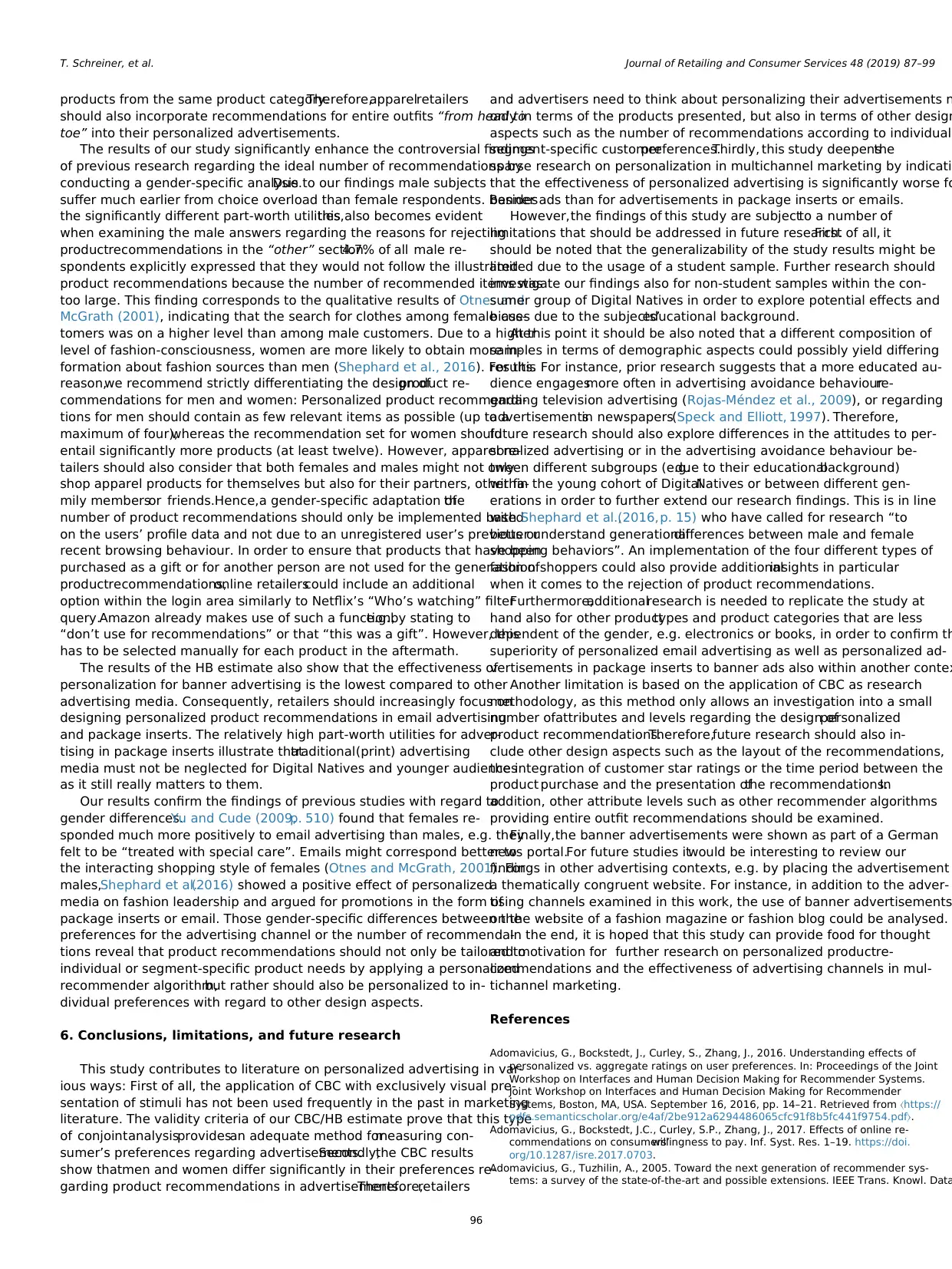
products from the same product category.Therefore,apparelretailers
should also incorporate recommendations for entire outfits “from head to
toe” into their personalized advertisements.
The results of our study significantly enhance the controversial findings
of previous research regarding the ideal number of recommendations by
conducting a gender-specific analysis.Due to our findings male subjects
suffer much earlier from choice overload than female respondents. Besides
the significantly different part-worth utilities,this also becomes evident
when examining the male answers regarding the reasons for rejecting
productrecommendations in the “other” section.4.7% of all male re-
spondents explicitly expressed that they would not follow the illustrated
product recommendations because the number of recommended items was
too large. This finding corresponds to the qualitative results of Otnes and
McGrath (2001), indicating that the search for clothes among female cus-
tomers was on a higher level than among male customers. Due to a higher
level of fashion-consciousness, women are more likely to obtain more in-
formation about fashion sources than men (Shephard et al., 2016). For this
reason,we recommend strictly differentiating the design ofproduct re-
commendations for men and women: Personalized product recommenda-
tions for men should contain as few relevant items as possible (up to a
maximum of four),whereas the recommendation set for women should
entail significantly more products (at least twelve). However, apparel re-
tailers should also consider that both females and males might not only
shop apparel products for themselves but also for their partners, other fa-
mily membersor friends.Hence,a gender-specific adaptation ofthe
number of product recommendations should only be implemented based
on the users’ profile data and not due to an unregistered user’s previous or
recent browsing behaviour. In order to ensure that products that have been
purchased as a gift or for another person are not used for the generation of
productrecommendations,online retailerscould include an additional
option within the login area similarly to Netflix’s “Who’s watching” filter
query.Amazon already makes use of such a function,e.g.by stating to
“don’t use for recommendations” or that “this was a gift”. However, this
has to be selected manually for each product in the aftermath.
The results of the HB estimate also show that the effectiveness of
personalization for banner advertising is the lowest compared to other
advertising media. Consequently, retailers should increasingly focus on
designing personalized product recommendations in email advertising
and package inserts. The relatively high part-worth utilities for adver-
tising in package inserts illustrate thattraditional(print) advertising
media must not be neglected for Digital Natives and younger audiences
as it still really matters to them.
Our results confirm the findings of previous studies with regard to
gender differences.Yu and Cude (2009,p. 510) found that females re-
sponded much more positively to email advertising than males, e.g. they
felt to be “treated with special care”. Emails might correspond better to
the interacting shopping style of females (Otnes and McGrath, 2001). For
males,Shephard et al.(2016) showed a positive effect of personalized
media on fashion leadership and argued for promotions in the form of
package inserts or email. Those gender-specific differences between the
preferences for the advertising channel or the number of recommenda-
tions reveal that product recommendations should not only be tailored to
individual or segment-specific product needs by applying a personalized
recommender algorithm,but rather should also be personalized to in-
dividual preferences with regard to other design aspects.
6. Conclusions, limitations, and future research
This study contributes to literature on personalized advertising in var-
ious ways: First of all, the application of CBC with exclusively visual pre-
sentation of stimuli has not been used frequently in the past in marketing
literature. The validity criteria of our CBC/HB estimate prove that this type
of conjointanalysisprovidesan adequate method formeasuring con-
sumer’s preferences regarding advertisements.Secondly,the CBC results
show thatmen and women differ significantly in their preferences re-
garding product recommendations in advertisements.Therefore,retailers
and advertisers need to think about personalizing their advertisements n
only in terms of the products presented, but also in terms of other design
aspects such as the number of recommendations according to individual
segment-specific customerpreferences.Thirdly, this study deepensthe
sparse research on personalization in multichannel marketing by indicati
that the effectiveness of personalized advertising is significantly worse fo
banner ads than for advertisements in package inserts or emails.
However,the findings of this study are subjectto a number of
limitations that should be addressed in future research.First of all, it
should be noted that the generalizability of the study results might be
limited due to the usage of a student sample. Further research should
investigate our findings also for non-student samples within the con-
sumer group of Digital Natives in order to explore potential effects and
biases due to the subjects’educational background.
At this point it should be also noted that a different composition of
samples in terms of demographic aspects could possibly yield differing
results. For instance, prior research suggests that a more educated au-
dience engagesmore often in advertising avoidance behaviourre-
garding television advertising (Rojas-Méndez et al., 2009), or regarding
advertisementsin newspapers(Speck and Elliott, 1997). Therefore,
future research should also explore differences in the attitudes to per-
sonalized advertising or in the advertising avoidance behaviour be-
tween different subgroups (e.g.due to their educationalbackground)
within the young cohort of DigitalNatives or between different gen-
erations in order to further extend our research findings. This is in line
with Shephard et al.,(2016,p. 15) who have called for research “to
better understand generationaldifferences between male and female
shopping behaviors”. An implementation of the four different types of
fashion shoppers could also provide additionalinsights in particular
when it comes to the rejection of product recommendations.
Furthermore,additionalresearch is needed to replicate the study at
hand also for other producttypes and product categories that are less
dependent of the gender, e.g. electronics or books, in order to confirm th
superiority of personalized email advertising as well as personalized ad-
vertisements in package inserts to banner ads also within another contex
Another limitation is based on the application of CBC as research
methodology, as this method only allows an investigation into a small
number ofattributes and levels regarding the design ofpersonalized
product recommendations.Therefore,future research should also in-
clude other design aspects such as the layout of the recommendations,
the integration of customer star ratings or the time period between the
product purchase and the presentation ofthe recommendations.In
addition, other attribute levels such as other recommender algorithms
providing entire outfit recommendations should be examined.
Finally,the banner advertisements were shown as part of a German
news portal.For future studies itwould be interesting to review our
findings in other advertising contexts, e.g. by placing the advertisement
a thematically congruent website. For instance, in addition to the adver-
tising channels examined in this work, the use of banner advertisements
on the website of a fashion magazine or fashion blog could be analysed.
In the end, it is hoped that this study can provide food for thought
and motivation for further research on personalized productre-
commendations and the effectiveness of advertising channels in mul-
tichannel marketing.
References
Adomavicius, G., Bockstedt, J., Curley, S., Zhang, J., 2016. Understanding effects of
personalized vs. aggregate ratings on user preferences. In: Proceedings of the Joint
Workshop on Interfaces and Human Decision Making for Recommender Systems.
Joint Workshop on Interfaces and Human Decision Making for Recommender
Systems, Boston, MA, USA. September 16, 2016, pp. 14–21. Retrieved from https://〈
pdfs.semanticscholar.org/e4af/2be912a6294486065cfc91f8b5fc441f9754.pdf〉.
Adomavicius, G., Bockstedt, J.C., Curley, S.P., Zhang, J., 2017. Effects of online re-
commendations on consumers’willingness to pay. Inf. Syst. Res. 1–19. https://doi.
org/10.1287/isre.2017.0703.
Adomavicius, G., Tuzhilin, A., 2005. Toward the next generation of recommender sys-
tems: a survey of the state-of-the-art and possible extensions. IEEE Trans. Knowl. Data
T. Schreiner, et al. Journal of Retailing and Consumer Services 48 (2019) 87–99
96
should also incorporate recommendations for entire outfits “from head to
toe” into their personalized advertisements.
The results of our study significantly enhance the controversial findings
of previous research regarding the ideal number of recommendations by
conducting a gender-specific analysis.Due to our findings male subjects
suffer much earlier from choice overload than female respondents. Besides
the significantly different part-worth utilities,this also becomes evident
when examining the male answers regarding the reasons for rejecting
productrecommendations in the “other” section.4.7% of all male re-
spondents explicitly expressed that they would not follow the illustrated
product recommendations because the number of recommended items was
too large. This finding corresponds to the qualitative results of Otnes and
McGrath (2001), indicating that the search for clothes among female cus-
tomers was on a higher level than among male customers. Due to a higher
level of fashion-consciousness, women are more likely to obtain more in-
formation about fashion sources than men (Shephard et al., 2016). For this
reason,we recommend strictly differentiating the design ofproduct re-
commendations for men and women: Personalized product recommenda-
tions for men should contain as few relevant items as possible (up to a
maximum of four),whereas the recommendation set for women should
entail significantly more products (at least twelve). However, apparel re-
tailers should also consider that both females and males might not only
shop apparel products for themselves but also for their partners, other fa-
mily membersor friends.Hence,a gender-specific adaptation ofthe
number of product recommendations should only be implemented based
on the users’ profile data and not due to an unregistered user’s previous or
recent browsing behaviour. In order to ensure that products that have been
purchased as a gift or for another person are not used for the generation of
productrecommendations,online retailerscould include an additional
option within the login area similarly to Netflix’s “Who’s watching” filter
query.Amazon already makes use of such a function,e.g.by stating to
“don’t use for recommendations” or that “this was a gift”. However, this
has to be selected manually for each product in the aftermath.
The results of the HB estimate also show that the effectiveness of
personalization for banner advertising is the lowest compared to other
advertising media. Consequently, retailers should increasingly focus on
designing personalized product recommendations in email advertising
and package inserts. The relatively high part-worth utilities for adver-
tising in package inserts illustrate thattraditional(print) advertising
media must not be neglected for Digital Natives and younger audiences
as it still really matters to them.
Our results confirm the findings of previous studies with regard to
gender differences.Yu and Cude (2009,p. 510) found that females re-
sponded much more positively to email advertising than males, e.g. they
felt to be “treated with special care”. Emails might correspond better to
the interacting shopping style of females (Otnes and McGrath, 2001). For
males,Shephard et al.(2016) showed a positive effect of personalized
media on fashion leadership and argued for promotions in the form of
package inserts or email. Those gender-specific differences between the
preferences for the advertising channel or the number of recommenda-
tions reveal that product recommendations should not only be tailored to
individual or segment-specific product needs by applying a personalized
recommender algorithm,but rather should also be personalized to in-
dividual preferences with regard to other design aspects.
6. Conclusions, limitations, and future research
This study contributes to literature on personalized advertising in var-
ious ways: First of all, the application of CBC with exclusively visual pre-
sentation of stimuli has not been used frequently in the past in marketing
literature. The validity criteria of our CBC/HB estimate prove that this type
of conjointanalysisprovidesan adequate method formeasuring con-
sumer’s preferences regarding advertisements.Secondly,the CBC results
show thatmen and women differ significantly in their preferences re-
garding product recommendations in advertisements.Therefore,retailers
and advertisers need to think about personalizing their advertisements n
only in terms of the products presented, but also in terms of other design
aspects such as the number of recommendations according to individual
segment-specific customerpreferences.Thirdly, this study deepensthe
sparse research on personalization in multichannel marketing by indicati
that the effectiveness of personalized advertising is significantly worse fo
banner ads than for advertisements in package inserts or emails.
However,the findings of this study are subjectto a number of
limitations that should be addressed in future research.First of all, it
should be noted that the generalizability of the study results might be
limited due to the usage of a student sample. Further research should
investigate our findings also for non-student samples within the con-
sumer group of Digital Natives in order to explore potential effects and
biases due to the subjects’educational background.
At this point it should be also noted that a different composition of
samples in terms of demographic aspects could possibly yield differing
results. For instance, prior research suggests that a more educated au-
dience engagesmore often in advertising avoidance behaviourre-
garding television advertising (Rojas-Méndez et al., 2009), or regarding
advertisementsin newspapers(Speck and Elliott, 1997). Therefore,
future research should also explore differences in the attitudes to per-
sonalized advertising or in the advertising avoidance behaviour be-
tween different subgroups (e.g.due to their educationalbackground)
within the young cohort of DigitalNatives or between different gen-
erations in order to further extend our research findings. This is in line
with Shephard et al.,(2016,p. 15) who have called for research “to
better understand generationaldifferences between male and female
shopping behaviors”. An implementation of the four different types of
fashion shoppers could also provide additionalinsights in particular
when it comes to the rejection of product recommendations.
Furthermore,additionalresearch is needed to replicate the study at
hand also for other producttypes and product categories that are less
dependent of the gender, e.g. electronics or books, in order to confirm th
superiority of personalized email advertising as well as personalized ad-
vertisements in package inserts to banner ads also within another contex
Another limitation is based on the application of CBC as research
methodology, as this method only allows an investigation into a small
number ofattributes and levels regarding the design ofpersonalized
product recommendations.Therefore,future research should also in-
clude other design aspects such as the layout of the recommendations,
the integration of customer star ratings or the time period between the
product purchase and the presentation ofthe recommendations.In
addition, other attribute levels such as other recommender algorithms
providing entire outfit recommendations should be examined.
Finally,the banner advertisements were shown as part of a German
news portal.For future studies itwould be interesting to review our
findings in other advertising contexts, e.g. by placing the advertisement
a thematically congruent website. For instance, in addition to the adver-
tising channels examined in this work, the use of banner advertisements
on the website of a fashion magazine or fashion blog could be analysed.
In the end, it is hoped that this study can provide food for thought
and motivation for further research on personalized productre-
commendations and the effectiveness of advertising channels in mul-
tichannel marketing.
References
Adomavicius, G., Bockstedt, J., Curley, S., Zhang, J., 2016. Understanding effects of
personalized vs. aggregate ratings on user preferences. In: Proceedings of the Joint
Workshop on Interfaces and Human Decision Making for Recommender Systems.
Joint Workshop on Interfaces and Human Decision Making for Recommender
Systems, Boston, MA, USA. September 16, 2016, pp. 14–21. Retrieved from https://〈
pdfs.semanticscholar.org/e4af/2be912a6294486065cfc91f8b5fc441f9754.pdf〉.
Adomavicius, G., Bockstedt, J.C., Curley, S.P., Zhang, J., 2017. Effects of online re-
commendations on consumers’willingness to pay. Inf. Syst. Res. 1–19. https://doi.
org/10.1287/isre.2017.0703.
Adomavicius, G., Tuzhilin, A., 2005. Toward the next generation of recommender sys-
tems: a survey of the state-of-the-art and possible extensions. IEEE Trans. Knowl. Data
T. Schreiner, et al. Journal of Retailing and Consumer Services 48 (2019) 87–99
96
Secure Best Marks with AI Grader
Need help grading? Try our AI Grader for instant feedback on your assignments.
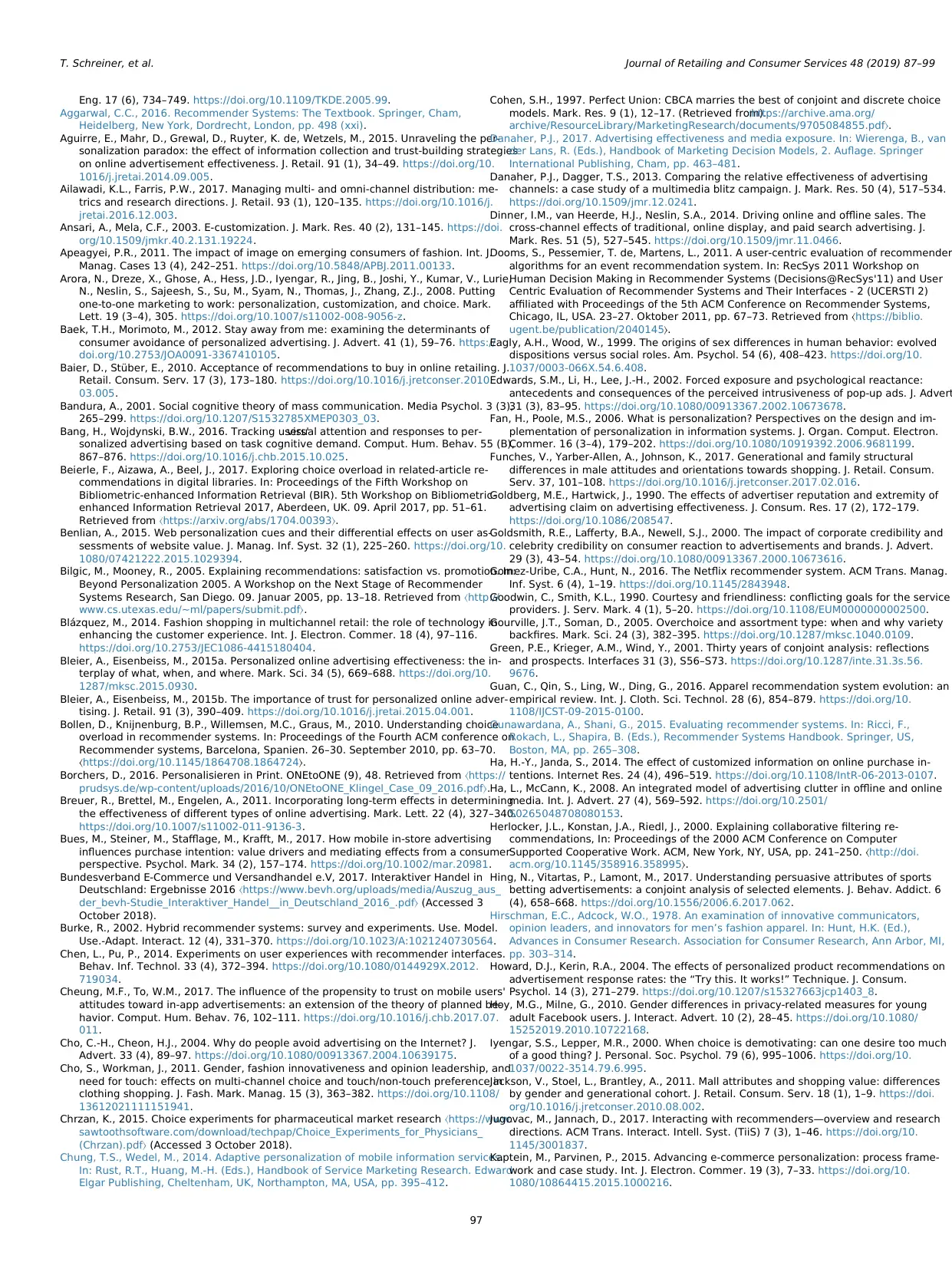
Eng. 17 (6), 734–749. https://doi.org/10.1109/TKDE.2005.99.
Aggarwal, C.C., 2016. Recommender Systems: The Textbook. Springer, Cham,
Heidelberg, New York, Dordrecht, London, pp. 498 (xxi).
Aguirre, E., Mahr, D., Grewal, D., Ruyter, K. de, Wetzels, M., 2015. Unraveling the per-
sonalization paradox: the effect of information collection and trust-building strategies
on online advertisement effectiveness. J. Retail. 91 (1), 34–49. https://doi.org/10.
1016/j.jretai.2014.09.005.
Ailawadi, K.L., Farris, P.W., 2017. Managing multi- and omni-channel distribution: me-
trics and research directions. J. Retail. 93 (1), 120–135. https://doi.org/10.1016/j.
jretai.2016.12.003.
Ansari, A., Mela, C.F., 2003. E-customization. J. Mark. Res. 40 (2), 131–145. https://doi.
org/10.1509/jmkr.40.2.131.19224.
Apeagyei, P.R., 2011. The impact of image on emerging consumers of fashion. Int. J.
Manag. Cases 13 (4), 242–251. https://doi.org/10.5848/APBJ.2011.00133.
Arora, N., Dreze, X., Ghose, A., Hess, J.D., Iyengar, R., Jing, B., Joshi, Y., Kumar, V., Lurie,
N., Neslin, S., Sajeesh, S., Su, M., Syam, N., Thomas, J., Zhang, Z.J., 2008. Putting
one-to-one marketing to work: personalization, customization, and choice. Mark.
Lett. 19 (3–4), 305. https://doi.org/10.1007/s11002-008-9056-z.
Baek, T.H., Morimoto, M., 2012. Stay away from me: examining the determinants of
consumer avoidance of personalized advertising. J. Advert. 41 (1), 59–76. https://
doi.org/10.2753/JOA0091-3367410105.
Baier, D., Stüber, E., 2010. Acceptance of recommendations to buy in online retailing. J.
Retail. Consum. Serv. 17 (3), 173–180. https://doi.org/10.1016/j.jretconser.2010.
03.005.
Bandura, A., 2001. Social cognitive theory of mass communication. Media Psychol. 3 (3),
265–299. https://doi.org/10.1207/S1532785XMEP0303_03.
Bang, H., Wojdynski, B.W., 2016. Tracking users'visual attention and responses to per-
sonalized advertising based on task cognitive demand. Comput. Hum. Behav. 55 (B),
867–876. https://doi.org/10.1016/j.chb.2015.10.025.
Beierle, F., Aizawa, A., Beel, J., 2017. Exploring choice overload in related-article re-
commendations in digital libraries. In: Proceedings of the Fifth Workshop on
Bibliometric-enhanced Information Retrieval (BIR). 5th Workshop on Bibliometric-
enhanced Information Retrieval 2017, Aberdeen, UK. 09. April 2017, pp. 51–61.
Retrieved from https://arxiv.org/abs/1704.00393〈 〉.
Benlian, A., 2015. Web personalization cues and their differential effects on user as-
sessments of website value. J. Manag. Inf. Syst. 32 (1), 225–260. https://doi.org/10.
1080/07421222.2015.1029394.
Bilgic, M., Mooney, R., 2005. Explaining recommendations: satisfaction vs. promotion. In:
Beyond Personalization 2005. A Workshop on the Next Stage of Recommender
Systems Research, San Diego. 09. Januar 2005, pp. 13–18. Retrieved from http://〈
www.cs.utexas.edu/~ml/papers/submit.pdf〉.
Blázquez, M., 2014. Fashion shopping in multichannel retail: the role of technology in
enhancing the customer experience. Int. J. Electron. Commer. 18 (4), 97–116.
https://doi.org/10.2753/JEC1086-4415180404.
Bleier, A., Eisenbeiss, M., 2015a. Personalized online advertising effectiveness: the in-
terplay of what, when, and where. Mark. Sci. 34 (5), 669–688. https://doi.org/10.
1287/mksc.2015.0930.
Bleier, A., Eisenbeiss, M., 2015b. The importance of trust for personalized online adver-
tising. J. Retail. 91 (3), 390–409. https://doi.org/10.1016/j.jretai.2015.04.001.
Bollen, D., Knijnenburg, B.P., Willemsen, M.C., Graus, M., 2010. Understanding choice
overload in recommender systems. In: Proceedings of the Fourth ACM conference on
Recommender systems, Barcelona, Spanien. 26–30. September 2010, pp. 63–70.
〈https://doi.org/10.1145/1864708.1864724 .〉
Borchers, D., 2016. Personalisieren in Print. ONEtoONE (9), 48. Retrieved from https://〈
prudsys.de/wp-content/uploads/2016/10/ONEtoONE_Klingel_Case_09_2016.pdf〉.
Breuer, R., Brettel, M., Engelen, A., 2011. Incorporating long-term effects in determining
the effectiveness of different types of online advertising. Mark. Lett. 22 (4), 327–340.
https://doi.org/10.1007/s11002-011-9136-3.
Bues, M., Steiner, M., Stafflage, M., Krafft, M., 2017. How mobile in‐store advertising
influences purchase intention: value drivers and mediating effects from a consumer
perspective. Psychol. Mark. 34 (2), 157–174. https://doi.org/10.1002/mar.20981.
Bundesverband E-Commerce und Versandhandel e.V, 2017. Interaktiver Handel in
Deutschland: Ergebnisse 2016 https://www.bevh.org/uploads/media/Auszug_aus_〈
der_bevh-Studie_Interaktiver_Handel__in_Deutschland_2016_.pdf〉 (Accessed 3
October 2018).
Burke, R., 2002. Hybrid recommender systems: survey and experiments. Use. Model.
Use.-Adapt. Interact. 12 (4), 331–370. https://doi.org/10.1023/A:1021240730564.
Chen, L., Pu, P., 2014. Experiments on user experiences with recommender interfaces.
Behav. Inf. Technol. 33 (4), 372–394. https://doi.org/10.1080/0144929X.2012.
719034.
Cheung, M.F., To, W.M., 2017. The influence of the propensity to trust on mobile users'
attitudes toward in-app advertisements: an extension of the theory of planned be-
havior. Comput. Hum. Behav. 76, 102–111. https://doi.org/10.1016/j.chb.2017.07.
011.
Cho, C.-H., Cheon, H.J., 2004. Why do people avoid advertising on the Internet? J.
Advert. 33 (4), 89–97. https://doi.org/10.1080/00913367.2004.10639175.
Cho, S., Workman, J., 2011. Gender, fashion innovativeness and opinion leadership, and
need for touch: effects on multi-channel choice and touch/non-touch preference in
clothing shopping. J. Fash. Mark. Manag. 15 (3), 363–382. https://doi.org/10.1108/
13612021111151941.
Chrzan, K., 2015. Choice experiments for pharmaceutical market research https://www.〈
sawtoothsoftware.com/download/techpap/Choice_Experiments_for_Physicians_
(Chrzan).pdf〉 (Accessed 3 October 2018).
Chung, T.S., Wedel, M., 2014. Adaptive personalization of mobile information services.
In: Rust, R.T., Huang, M.-H. (Eds.), Handbook of Service Marketing Research. Edward
Elgar Publishing, Cheltenham, UK, Northampton, MA, USA, pp. 395–412.
Cohen, S.H., 1997. Perfect Union: CBCA marries the best of conjoint and discrete choice
models. Mark. Res. 9 (1), 12–17. (Retrieved from).〈https://archive.ama.org/
archive/ResourceLibrary/MarketingResearch/documents/9705084855.pdf〉.
Danaher, P.J., 2017. Advertising effectiveness and media exposure. In: Wierenga, B., van
der Lans, R. (Eds.), Handbook of Marketing Decision Models, 2. Auflage. Springer
International Publishing, Cham, pp. 463–481.
Danaher, P.J., Dagger, T.S., 2013. Comparing the relative effectiveness of advertising
channels: a case study of a multimedia blitz campaign. J. Mark. Res. 50 (4), 517–534.
https://doi.org/10.1509/jmr.12.0241.
Dinner, I.M., van Heerde, H.J., Neslin, S.A., 2014. Driving online and offline sales. The
cross-channel effects of traditional, online display, and paid search advertising. J.
Mark. Res. 51 (5), 527–545. https://doi.org/10.1509/jmr.11.0466.
Dooms, S., Pessemier, T. de, Martens, L., 2011. A user-centric evaluation of recommender
algorithms for an event recommendation system. In: RecSys 2011 Workshop on
Human Decision Making in Recommender Systems (Decisions@RecSys'11) and User
Centric Evaluation of Recommender Systems and Their Interfaces - 2 (UCERSTI 2)
affiliated with Proceedings of the 5th ACM Conference on Recommender Systems,
Chicago, IL, USA. 23–27. Oktober 2011, pp. 67–73. Retrieved from 〈https://biblio.
ugent.be/publication/2040145 .〉
Eagly, A.H., Wood, W., 1999. The origins of sex differences in human behavior: evolved
dispositions versus social roles. Am. Psychol. 54 (6), 408–423. https://doi.org/10.
1037/0003-066X.54.6.408.
Edwards, S.M., Li, H., Lee, J.-H., 2002. Forced exposure and psychological reactance:
antecedents and consequences of the perceived intrusiveness of pop-up ads. J. Advert
31 (3), 83–95. https://doi.org/10.1080/00913367.2002.10673678.
Fan, H., Poole, M.S., 2006. What is personalization? Perspectives on the design and im-
plementation of personalization in information systems. J. Organ. Comput. Electron.
Commer. 16 (3–4), 179–202. https://doi.org/10.1080/10919392.2006.9681199.
Funches, V., Yarber-Allen, A., Johnson, K., 2017. Generational and family structural
differences in male attitudes and orientations towards shopping. J. Retail. Consum.
Serv. 37, 101–108. https://doi.org/10.1016/j.jretconser.2017.02.016.
Goldberg, M.E., Hartwick, J., 1990. The effects of advertiser reputation and extremity of
advertising claim on advertising effectiveness. J. Consum. Res. 17 (2), 172–179.
https://doi.org/10.1086/208547.
Goldsmith, R.E., Lafferty, B.A., Newell, S.J., 2000. The impact of corporate credibility and
celebrity credibility on consumer reaction to advertisements and brands. J. Advert.
29 (3), 43–54. https://doi.org/10.1080/00913367.2000.10673616.
Gomez-Uribe, C.A., Hunt, N., 2016. The Netflix recommender system. ACM Trans. Manag.
Inf. Syst. 6 (4), 1–19. https://doi.org/10.1145/2843948.
Goodwin, C., Smith, K.L., 1990. Courtesy and friendliness: conflicting goals for the service
providers. J. Serv. Mark. 4 (1), 5–20. https://doi.org/10.1108/EUM0000000002500.
Gourville, J.T., Soman, D., 2005. Overchoice and assortment type: when and why variety
backfires. Mark. Sci. 24 (3), 382–395. https://doi.org/10.1287/mksc.1040.0109.
Green, P.E., Krieger, A.M., Wind, Y., 2001. Thirty years of conjoint analysis: reflections
and prospects. Interfaces 31 (3), S56–S73. https://doi.org/10.1287/inte.31.3s.56.
9676.
Guan, C., Qin, S., Ling, W., Ding, G., 2016. Apparel recommendation system evolution: an
empirical review. Int. J. Cloth. Sci. Technol. 28 (6), 854–879. https://doi.org/10.
1108/IJCST-09-2015-0100.
Gunawardana, A., Shani, G., 2015. Evaluating recommender systems. In: Ricci, F.,
Rokach, L., Shapira, B. (Eds.), Recommender Systems Handbook. Springer, US,
Boston, MA, pp. 265–308.
Ha, H.-Y., Janda, S., 2014. The effect of customized information on online purchase in-
tentions. Internet Res. 24 (4), 496–519. https://doi.org/10.1108/IntR-06-2013-0107.
Ha, L., McCann, K., 2008. An integrated model of advertising clutter in offline and online
media. Int. J. Advert. 27 (4), 569–592. https://doi.org/10.2501/
S0265048708080153.
Herlocker, J.L., Konstan, J.A., Riedl, J., 2000. Explaining collaborative filtering re-
commendations, In: Proceedings of the 2000 ACM Conference on Computer
Supported Cooperative Work. ACM, New York, NY, USA, pp. 241–250. 〈http://doi.
acm.org/10.1145/358916.358995 .〉
Hing, N., Vitartas, P., Lamont, M., 2017. Understanding persuasive attributes of sports
betting advertisements: a conjoint analysis of selected elements. J. Behav. Addict. 6
(4), 658–668. https://doi.org/10.1556/2006.6.2017.062.
Hirschman, E.C., Adcock, W.O., 1978. An examination of innovative communicators,
opinion leaders, and innovators for men’s fashion apparel. In: Hunt, H.K. (Ed.),
Advances in Consumer Research. Association for Consumer Research, Ann Arbor, MI,
pp. 303–314.
Howard, D.J., Kerin, R.A., 2004. The effects of personalized product recommendations on
advertisement response rates: the “Try this. It works!” Technique. J. Consum.
Psychol. 14 (3), 271–279. https://doi.org/10.1207/s15327663jcp1403_8.
Hoy, M.G., Milne, G., 2010. Gender differences in privacy-related measures for young
adult Facebook users. J. Interact. Advert. 10 (2), 28–45. https://doi.org/10.1080/
15252019.2010.10722168.
Iyengar, S.S., Lepper, M.R., 2000. When choice is demotivating: can one desire too much
of a good thing? J. Personal. Soc. Psychol. 79 (6), 995–1006. https://doi.org/10.
1037/0022-3514.79.6.995.
Jackson, V., Stoel, L., Brantley, A., 2011. Mall attributes and shopping value: differences
by gender and generational cohort. J. Retail. Consum. Serv. 18 (1), 1–9. https://doi.
org/10.1016/j.jretconser.2010.08.002.
Jugovac, M., Jannach, D., 2017. Interacting with recommenders—overview and research
directions. ACM Trans. Interact. Intell. Syst. (TiiS) 7 (3), 1–46. https://doi.org/10.
1145/3001837.
Kaptein, M., Parvinen, P., 2015. Advancing e-commerce personalization: process frame-
work and case study. Int. J. Electron. Commer. 19 (3), 7–33. https://doi.org/10.
1080/10864415.2015.1000216.
T. Schreiner, et al. Journal of Retailing and Consumer Services 48 (2019) 87–99
97
Aggarwal, C.C., 2016. Recommender Systems: The Textbook. Springer, Cham,
Heidelberg, New York, Dordrecht, London, pp. 498 (xxi).
Aguirre, E., Mahr, D., Grewal, D., Ruyter, K. de, Wetzels, M., 2015. Unraveling the per-
sonalization paradox: the effect of information collection and trust-building strategies
on online advertisement effectiveness. J. Retail. 91 (1), 34–49. https://doi.org/10.
1016/j.jretai.2014.09.005.
Ailawadi, K.L., Farris, P.W., 2017. Managing multi- and omni-channel distribution: me-
trics and research directions. J. Retail. 93 (1), 120–135. https://doi.org/10.1016/j.
jretai.2016.12.003.
Ansari, A., Mela, C.F., 2003. E-customization. J. Mark. Res. 40 (2), 131–145. https://doi.
org/10.1509/jmkr.40.2.131.19224.
Apeagyei, P.R., 2011. The impact of image on emerging consumers of fashion. Int. J.
Manag. Cases 13 (4), 242–251. https://doi.org/10.5848/APBJ.2011.00133.
Arora, N., Dreze, X., Ghose, A., Hess, J.D., Iyengar, R., Jing, B., Joshi, Y., Kumar, V., Lurie,
N., Neslin, S., Sajeesh, S., Su, M., Syam, N., Thomas, J., Zhang, Z.J., 2008. Putting
one-to-one marketing to work: personalization, customization, and choice. Mark.
Lett. 19 (3–4), 305. https://doi.org/10.1007/s11002-008-9056-z.
Baek, T.H., Morimoto, M., 2012. Stay away from me: examining the determinants of
consumer avoidance of personalized advertising. J. Advert. 41 (1), 59–76. https://
doi.org/10.2753/JOA0091-3367410105.
Baier, D., Stüber, E., 2010. Acceptance of recommendations to buy in online retailing. J.
Retail. Consum. Serv. 17 (3), 173–180. https://doi.org/10.1016/j.jretconser.2010.
03.005.
Bandura, A., 2001. Social cognitive theory of mass communication. Media Psychol. 3 (3),
265–299. https://doi.org/10.1207/S1532785XMEP0303_03.
Bang, H., Wojdynski, B.W., 2016. Tracking users'visual attention and responses to per-
sonalized advertising based on task cognitive demand. Comput. Hum. Behav. 55 (B),
867–876. https://doi.org/10.1016/j.chb.2015.10.025.
Beierle, F., Aizawa, A., Beel, J., 2017. Exploring choice overload in related-article re-
commendations in digital libraries. In: Proceedings of the Fifth Workshop on
Bibliometric-enhanced Information Retrieval (BIR). 5th Workshop on Bibliometric-
enhanced Information Retrieval 2017, Aberdeen, UK. 09. April 2017, pp. 51–61.
Retrieved from https://arxiv.org/abs/1704.00393〈 〉.
Benlian, A., 2015. Web personalization cues and their differential effects on user as-
sessments of website value. J. Manag. Inf. Syst. 32 (1), 225–260. https://doi.org/10.
1080/07421222.2015.1029394.
Bilgic, M., Mooney, R., 2005. Explaining recommendations: satisfaction vs. promotion. In:
Beyond Personalization 2005. A Workshop on the Next Stage of Recommender
Systems Research, San Diego. 09. Januar 2005, pp. 13–18. Retrieved from http://〈
www.cs.utexas.edu/~ml/papers/submit.pdf〉.
Blázquez, M., 2014. Fashion shopping in multichannel retail: the role of technology in
enhancing the customer experience. Int. J. Electron. Commer. 18 (4), 97–116.
https://doi.org/10.2753/JEC1086-4415180404.
Bleier, A., Eisenbeiss, M., 2015a. Personalized online advertising effectiveness: the in-
terplay of what, when, and where. Mark. Sci. 34 (5), 669–688. https://doi.org/10.
1287/mksc.2015.0930.
Bleier, A., Eisenbeiss, M., 2015b. The importance of trust for personalized online adver-
tising. J. Retail. 91 (3), 390–409. https://doi.org/10.1016/j.jretai.2015.04.001.
Bollen, D., Knijnenburg, B.P., Willemsen, M.C., Graus, M., 2010. Understanding choice
overload in recommender systems. In: Proceedings of the Fourth ACM conference on
Recommender systems, Barcelona, Spanien. 26–30. September 2010, pp. 63–70.
〈https://doi.org/10.1145/1864708.1864724 .〉
Borchers, D., 2016. Personalisieren in Print. ONEtoONE (9), 48. Retrieved from https://〈
prudsys.de/wp-content/uploads/2016/10/ONEtoONE_Klingel_Case_09_2016.pdf〉.
Breuer, R., Brettel, M., Engelen, A., 2011. Incorporating long-term effects in determining
the effectiveness of different types of online advertising. Mark. Lett. 22 (4), 327–340.
https://doi.org/10.1007/s11002-011-9136-3.
Bues, M., Steiner, M., Stafflage, M., Krafft, M., 2017. How mobile in‐store advertising
influences purchase intention: value drivers and mediating effects from a consumer
perspective. Psychol. Mark. 34 (2), 157–174. https://doi.org/10.1002/mar.20981.
Bundesverband E-Commerce und Versandhandel e.V, 2017. Interaktiver Handel in
Deutschland: Ergebnisse 2016 https://www.bevh.org/uploads/media/Auszug_aus_〈
der_bevh-Studie_Interaktiver_Handel__in_Deutschland_2016_.pdf〉 (Accessed 3
October 2018).
Burke, R., 2002. Hybrid recommender systems: survey and experiments. Use. Model.
Use.-Adapt. Interact. 12 (4), 331–370. https://doi.org/10.1023/A:1021240730564.
Chen, L., Pu, P., 2014. Experiments on user experiences with recommender interfaces.
Behav. Inf. Technol. 33 (4), 372–394. https://doi.org/10.1080/0144929X.2012.
719034.
Cheung, M.F., To, W.M., 2017. The influence of the propensity to trust on mobile users'
attitudes toward in-app advertisements: an extension of the theory of planned be-
havior. Comput. Hum. Behav. 76, 102–111. https://doi.org/10.1016/j.chb.2017.07.
011.
Cho, C.-H., Cheon, H.J., 2004. Why do people avoid advertising on the Internet? J.
Advert. 33 (4), 89–97. https://doi.org/10.1080/00913367.2004.10639175.
Cho, S., Workman, J., 2011. Gender, fashion innovativeness and opinion leadership, and
need for touch: effects on multi-channel choice and touch/non-touch preference in
clothing shopping. J. Fash. Mark. Manag. 15 (3), 363–382. https://doi.org/10.1108/
13612021111151941.
Chrzan, K., 2015. Choice experiments for pharmaceutical market research https://www.〈
sawtoothsoftware.com/download/techpap/Choice_Experiments_for_Physicians_
(Chrzan).pdf〉 (Accessed 3 October 2018).
Chung, T.S., Wedel, M., 2014. Adaptive personalization of mobile information services.
In: Rust, R.T., Huang, M.-H. (Eds.), Handbook of Service Marketing Research. Edward
Elgar Publishing, Cheltenham, UK, Northampton, MA, USA, pp. 395–412.
Cohen, S.H., 1997. Perfect Union: CBCA marries the best of conjoint and discrete choice
models. Mark. Res. 9 (1), 12–17. (Retrieved from).〈https://archive.ama.org/
archive/ResourceLibrary/MarketingResearch/documents/9705084855.pdf〉.
Danaher, P.J., 2017. Advertising effectiveness and media exposure. In: Wierenga, B., van
der Lans, R. (Eds.), Handbook of Marketing Decision Models, 2. Auflage. Springer
International Publishing, Cham, pp. 463–481.
Danaher, P.J., Dagger, T.S., 2013. Comparing the relative effectiveness of advertising
channels: a case study of a multimedia blitz campaign. J. Mark. Res. 50 (4), 517–534.
https://doi.org/10.1509/jmr.12.0241.
Dinner, I.M., van Heerde, H.J., Neslin, S.A., 2014. Driving online and offline sales. The
cross-channel effects of traditional, online display, and paid search advertising. J.
Mark. Res. 51 (5), 527–545. https://doi.org/10.1509/jmr.11.0466.
Dooms, S., Pessemier, T. de, Martens, L., 2011. A user-centric evaluation of recommender
algorithms for an event recommendation system. In: RecSys 2011 Workshop on
Human Decision Making in Recommender Systems (Decisions@RecSys'11) and User
Centric Evaluation of Recommender Systems and Their Interfaces - 2 (UCERSTI 2)
affiliated with Proceedings of the 5th ACM Conference on Recommender Systems,
Chicago, IL, USA. 23–27. Oktober 2011, pp. 67–73. Retrieved from 〈https://biblio.
ugent.be/publication/2040145 .〉
Eagly, A.H., Wood, W., 1999. The origins of sex differences in human behavior: evolved
dispositions versus social roles. Am. Psychol. 54 (6), 408–423. https://doi.org/10.
1037/0003-066X.54.6.408.
Edwards, S.M., Li, H., Lee, J.-H., 2002. Forced exposure and psychological reactance:
antecedents and consequences of the perceived intrusiveness of pop-up ads. J. Advert
31 (3), 83–95. https://doi.org/10.1080/00913367.2002.10673678.
Fan, H., Poole, M.S., 2006. What is personalization? Perspectives on the design and im-
plementation of personalization in information systems. J. Organ. Comput. Electron.
Commer. 16 (3–4), 179–202. https://doi.org/10.1080/10919392.2006.9681199.
Funches, V., Yarber-Allen, A., Johnson, K., 2017. Generational and family structural
differences in male attitudes and orientations towards shopping. J. Retail. Consum.
Serv. 37, 101–108. https://doi.org/10.1016/j.jretconser.2017.02.016.
Goldberg, M.E., Hartwick, J., 1990. The effects of advertiser reputation and extremity of
advertising claim on advertising effectiveness. J. Consum. Res. 17 (2), 172–179.
https://doi.org/10.1086/208547.
Goldsmith, R.E., Lafferty, B.A., Newell, S.J., 2000. The impact of corporate credibility and
celebrity credibility on consumer reaction to advertisements and brands. J. Advert.
29 (3), 43–54. https://doi.org/10.1080/00913367.2000.10673616.
Gomez-Uribe, C.A., Hunt, N., 2016. The Netflix recommender system. ACM Trans. Manag.
Inf. Syst. 6 (4), 1–19. https://doi.org/10.1145/2843948.
Goodwin, C., Smith, K.L., 1990. Courtesy and friendliness: conflicting goals for the service
providers. J. Serv. Mark. 4 (1), 5–20. https://doi.org/10.1108/EUM0000000002500.
Gourville, J.T., Soman, D., 2005. Overchoice and assortment type: when and why variety
backfires. Mark. Sci. 24 (3), 382–395. https://doi.org/10.1287/mksc.1040.0109.
Green, P.E., Krieger, A.M., Wind, Y., 2001. Thirty years of conjoint analysis: reflections
and prospects. Interfaces 31 (3), S56–S73. https://doi.org/10.1287/inte.31.3s.56.
9676.
Guan, C., Qin, S., Ling, W., Ding, G., 2016. Apparel recommendation system evolution: an
empirical review. Int. J. Cloth. Sci. Technol. 28 (6), 854–879. https://doi.org/10.
1108/IJCST-09-2015-0100.
Gunawardana, A., Shani, G., 2015. Evaluating recommender systems. In: Ricci, F.,
Rokach, L., Shapira, B. (Eds.), Recommender Systems Handbook. Springer, US,
Boston, MA, pp. 265–308.
Ha, H.-Y., Janda, S., 2014. The effect of customized information on online purchase in-
tentions. Internet Res. 24 (4), 496–519. https://doi.org/10.1108/IntR-06-2013-0107.
Ha, L., McCann, K., 2008. An integrated model of advertising clutter in offline and online
media. Int. J. Advert. 27 (4), 569–592. https://doi.org/10.2501/
S0265048708080153.
Herlocker, J.L., Konstan, J.A., Riedl, J., 2000. Explaining collaborative filtering re-
commendations, In: Proceedings of the 2000 ACM Conference on Computer
Supported Cooperative Work. ACM, New York, NY, USA, pp. 241–250. 〈http://doi.
acm.org/10.1145/358916.358995 .〉
Hing, N., Vitartas, P., Lamont, M., 2017. Understanding persuasive attributes of sports
betting advertisements: a conjoint analysis of selected elements. J. Behav. Addict. 6
(4), 658–668. https://doi.org/10.1556/2006.6.2017.062.
Hirschman, E.C., Adcock, W.O., 1978. An examination of innovative communicators,
opinion leaders, and innovators for men’s fashion apparel. In: Hunt, H.K. (Ed.),
Advances in Consumer Research. Association for Consumer Research, Ann Arbor, MI,
pp. 303–314.
Howard, D.J., Kerin, R.A., 2004. The effects of personalized product recommendations on
advertisement response rates: the “Try this. It works!” Technique. J. Consum.
Psychol. 14 (3), 271–279. https://doi.org/10.1207/s15327663jcp1403_8.
Hoy, M.G., Milne, G., 2010. Gender differences in privacy-related measures for young
adult Facebook users. J. Interact. Advert. 10 (2), 28–45. https://doi.org/10.1080/
15252019.2010.10722168.
Iyengar, S.S., Lepper, M.R., 2000. When choice is demotivating: can one desire too much
of a good thing? J. Personal. Soc. Psychol. 79 (6), 995–1006. https://doi.org/10.
1037/0022-3514.79.6.995.
Jackson, V., Stoel, L., Brantley, A., 2011. Mall attributes and shopping value: differences
by gender and generational cohort. J. Retail. Consum. Serv. 18 (1), 1–9. https://doi.
org/10.1016/j.jretconser.2010.08.002.
Jugovac, M., Jannach, D., 2017. Interacting with recommenders—overview and research
directions. ACM Trans. Interact. Intell. Syst. (TiiS) 7 (3), 1–46. https://doi.org/10.
1145/3001837.
Kaptein, M., Parvinen, P., 2015. Advancing e-commerce personalization: process frame-
work and case study. Int. J. Electron. Commer. 19 (3), 7–33. https://doi.org/10.
1080/10864415.2015.1000216.
T. Schreiner, et al. Journal of Retailing and Consumer Services 48 (2019) 87–99
97
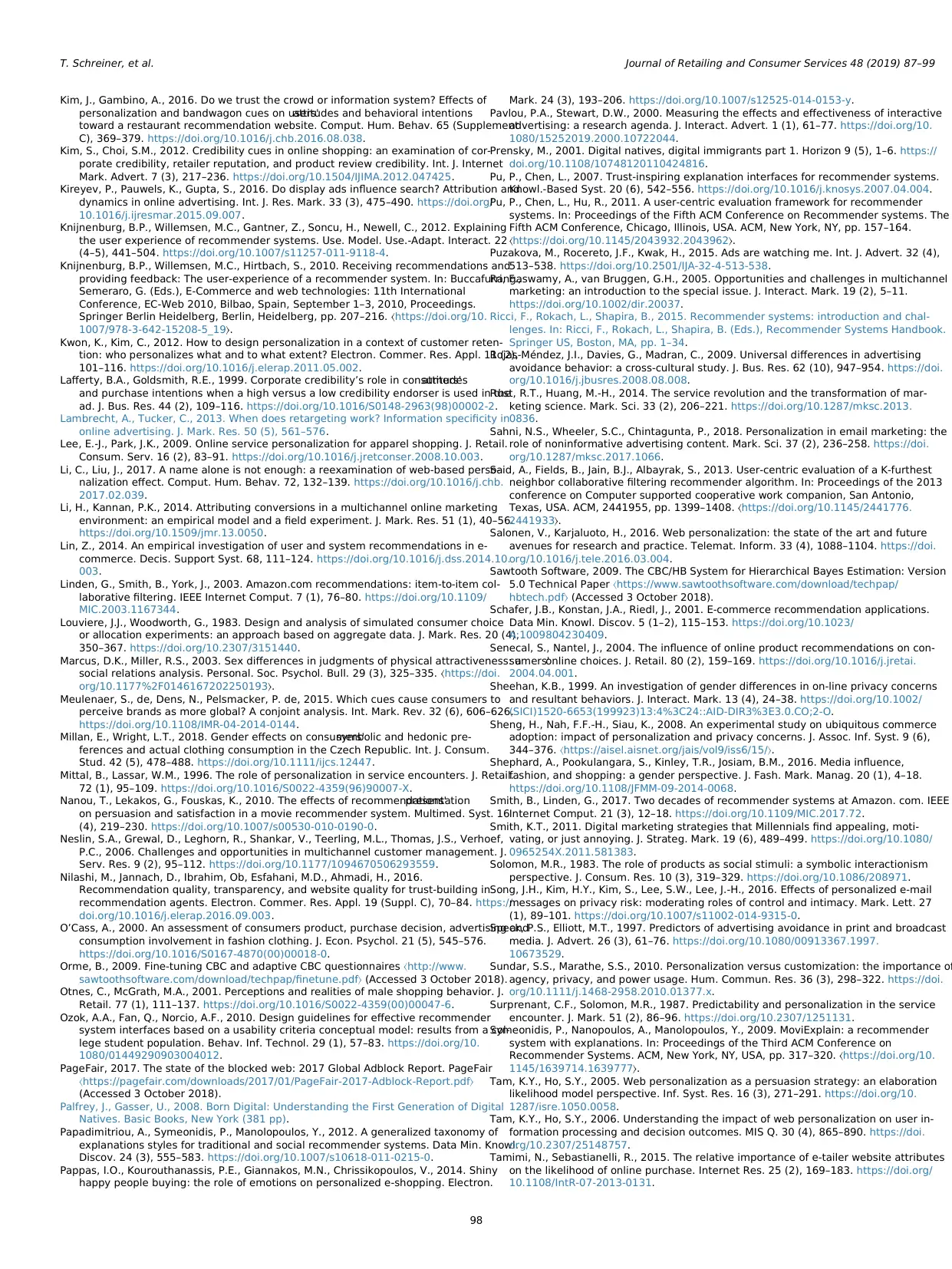
Kim, J., Gambino, A., 2016. Do we trust the crowd or information system? Effects of
personalization and bandwagon cues on users'attitudes and behavioral intentions
toward a restaurant recommendation website. Comput. Hum. Behav. 65 (Supplement
C), 369–379. https://doi.org/10.1016/j.chb.2016.08.038.
Kim, S., Choi, S.M., 2012. Credibility cues in online shopping: an examination of cor-
porate credibility, retailer reputation, and product review credibility. Int. J. Internet
Mark. Advert. 7 (3), 217–236. https://doi.org/10.1504/IJIMA.2012.047425.
Kireyev, P., Pauwels, K., Gupta, S., 2016. Do display ads influence search? Attribution and
dynamics in online advertising. Int. J. Res. Mark. 33 (3), 475–490. https://doi.org/
10.1016/j.ijresmar.2015.09.007.
Knijnenburg, B.P., Willemsen, M.C., Gantner, Z., Soncu, H., Newell, C., 2012. Explaining
the user experience of recommender systems. Use. Model. Use.-Adapt. Interact. 22
(4–5), 441–504. https://doi.org/10.1007/s11257-011-9118-4.
Knijnenburg, B.P., Willemsen, M.C., Hirtbach, S., 2010. Receiving recommendations and
providing feedback: The user-experience of a recommender system. In: Buccafurri, F.,
Semeraro, G. (Eds.), E-Commerce and web technologies: 11th International
Conference, EC-Web 2010, Bilbao, Spain, September 1–3, 2010, Proceedings.
Springer Berlin Heidelberg, Berlin, Heidelberg, pp. 207–216. 〈https://doi.org/10.
1007/978-3-642-15208-5_19 .〉
Kwon, K., Kim, C., 2012. How to design personalization in a context of customer reten-
tion: who personalizes what and to what extent? Electron. Commer. Res. Appl. 11 (2),
101–116. https://doi.org/10.1016/j.elerap.2011.05.002.
Lafferty, B.A., Goldsmith, R.E., 1999. Corporate credibility’s role in consumers'attitudes
and purchase intentions when a high versus a low credibility endorser is used in the
ad. J. Bus. Res. 44 (2), 109–116. https://doi.org/10.1016/S0148-2963(98)00002-2.
Lambrecht, A., Tucker, C., 2013. When does retargeting work? Information specificity in
online advertising. J. Mark. Res. 50 (5), 561–576.
Lee, E.-J., Park, J.K., 2009. Online service personalization for apparel shopping. J. Retail.
Consum. Serv. 16 (2), 83–91. https://doi.org/10.1016/j.jretconser.2008.10.003.
Li, C., Liu, J., 2017. A name alone is not enough: a reexamination of web-based perso-
nalization effect. Comput. Hum. Behav. 72, 132–139. https://doi.org/10.1016/j.chb.
2017.02.039.
Li, H., Kannan, P.K., 2014. Attributing conversions in a multichannel online marketing
environment: an empirical model and a field experiment. J. Mark. Res. 51 (1), 40–56.
https://doi.org/10.1509/jmr.13.0050.
Lin, Z., 2014. An empirical investigation of user and system recommendations in e-
commerce. Decis. Support Syst. 68, 111–124. https://doi.org/10.1016/j.dss.2014.10.
003.
Linden, G., Smith, B., York, J., 2003. Amazon.com recommendations: item-to-item col-
laborative filtering. IEEE Internet Comput. 7 (1), 76–80. https://doi.org/10.1109/
MIC.2003.1167344.
Louviere, J.J., Woodworth, G., 1983. Design and analysis of simulated consumer choice
or allocation experiments: an approach based on aggregate data. J. Mark. Res. 20 (4),
350–367. https://doi.org/10.2307/3151440.
Marcus, D.K., Miller, R.S., 2003. Sex differences in judgments of physical attractiveness: a
social relations analysis. Personal. Soc. Psychol. Bull. 29 (3), 325–335. 〈https://doi.
org/10.1177%2F0146167202250193 .〉
Meulenaer, S., de, Dens, N., Pelsmacker, P. de, 2015. Which cues cause consumers to
perceive brands as more global? A conjoint analysis. Int. Mark. Rev. 32 (6), 606–626.
https://doi.org/10.1108/IMR-04-2014-0144.
Millan, E., Wright, L.T., 2018. Gender effects on consumers'symbolic and hedonic pre-
ferences and actual clothing consumption in the Czech Republic. Int. J. Consum.
Stud. 42 (5), 478–488. https://doi.org/10.1111/ijcs.12447.
Mittal, B., Lassar, W.M., 1996. The role of personalization in service encounters. J. Retail.
72 (1), 95–109. https://doi.org/10.1016/S0022-4359(96)90007-X.
Nanou, T., Lekakos, G., Fouskas, K., 2010. The effects of recommendations'presentation
on persuasion and satisfaction in a movie recommender system. Multimed. Syst. 16
(4), 219–230. https://doi.org/10.1007/s00530-010-0190-0.
Neslin, S.A., Grewal, D., Leghorn, R., Shankar, V., Teerling, M.L., Thomas, J.S., Verhoef,
P.C., 2006. Challenges and opportunities in multichannel customer management. J.
Serv. Res. 9 (2), 95–112. https://doi.org/10.1177/1094670506293559.
Nilashi, M., Jannach, D., Ibrahim, Ob, Esfahani, M.D., Ahmadi, H., 2016.
Recommendation quality, transparency, and website quality for trust-building in
recommendation agents. Electron. Commer. Res. Appl. 19 (Suppl. C), 70–84. https://
doi.org/10.1016/j.elerap.2016.09.003.
O’Cass, A., 2000. An assessment of consumers product, purchase decision, advertising and
consumption involvement in fashion clothing. J. Econ. Psychol. 21 (5), 545–576.
https://doi.org/10.1016/S0167-4870(00)00018-0.
Orme, B., 2009. Fine-tuning CBC and adaptive CBC questionnaires http://www.〈
sawtoothsoftware.com/download/techpap/finetune.pdf〉 (Accessed 3 October 2018).
Otnes, C., McGrath, M.A., 2001. Perceptions and realities of male shopping behavior. J.
Retail. 77 (1), 111–137. https://doi.org/10.1016/S0022-4359(00)00047-6.
Ozok, A.A., Fan, Q., Norcio, A.F., 2010. Design guidelines for effective recommender
system interfaces based on a usability criteria conceptual model: results from a col-
lege student population. Behav. Inf. Technol. 29 (1), 57–83. https://doi.org/10.
1080/01449290903004012.
PageFair, 2017. The state of the blocked web: 2017 Global Adblock Report. PageFair
〈https://pagefair.com/downloads/2017/01/PageFair-2017-Adblock-Report.pdf〉
(Accessed 3 October 2018).
Palfrey, J., Gasser, U., 2008. Born Digital: Understanding the First Generation of Digital
Natives. Basic Books, New York (381 pp).
Papadimitriou, A., Symeonidis, P., Manolopoulos, Y., 2012. A generalized taxonomy of
explanations styles for traditional and social recommender systems. Data Min. Knowl.
Discov. 24 (3), 555–583. https://doi.org/10.1007/s10618-011-0215-0.
Pappas, I.O., Kourouthanassis, P.E., Giannakos, M.N., Chrissikopoulos, V., 2014. Shiny
happy people buying: the role of emotions on personalized e-shopping. Electron.
Mark. 24 (3), 193–206. https://doi.org/10.1007/s12525-014-0153-y.
Pavlou, P.A., Stewart, D.W., 2000. Measuring the effects and effectiveness of interactive
advertising: a research agenda. J. Interact. Advert. 1 (1), 61–77. https://doi.org/10.
1080/15252019.2000.10722044.
Prensky, M., 2001. Digital natives, digital immigrants part 1. Horizon 9 (5), 1–6. https://
doi.org/10.1108/10748120110424816.
Pu, P., Chen, L., 2007. Trust-inspiring explanation interfaces for recommender systems.
Knowl.-Based Syst. 20 (6), 542–556. https://doi.org/10.1016/j.knosys.2007.04.004.
Pu, P., Chen, L., Hu, R., 2011. A user-centric evaluation framework for recommender
systems. In: Proceedings of the Fifth ACM Conference on Recommender systems. The
Fifth ACM Conference, Chicago, Illinois, USA. ACM, New York, NY, pp. 157–164.
〈https://doi.org/10.1145/2043932.2043962 .〉
Puzakova, M., Rocereto, J.F., Kwak, H., 2015. Ads are watching me. Int. J. Advert. 32 (4),
513–538. https://doi.org/10.2501/IJA-32-4-513-538.
Rangaswamy, A., van Bruggen, G.H., 2005. Opportunities and challenges in multichannel
marketing: an introduction to the special issue. J. Interact. Mark. 19 (2), 5–11.
https://doi.org/10.1002/dir.20037.
Ricci, F., Rokach, L., Shapira, B., 2015. Recommender systems: introduction and chal-
lenges. In: Ricci, F., Rokach, L., Shapira, B. (Eds.), Recommender Systems Handbook.
Springer US, Boston, MA, pp. 1–34.
Rojas-Méndez, J.I., Davies, G., Madran, C., 2009. Universal differences in advertising
avoidance behavior: a cross-cultural study. J. Bus. Res. 62 (10), 947–954. https://doi.
org/10.1016/j.jbusres.2008.08.008.
Rust, R.T., Huang, M.-H., 2014. The service revolution and the transformation of mar-
keting science. Mark. Sci. 33 (2), 206–221. https://doi.org/10.1287/mksc.2013.
0836.
Sahni, N.S., Wheeler, S.C., Chintagunta, P., 2018. Personalization in email marketing: the
role of noninformative advertising content. Mark. Sci. 37 (2), 236–258. https://doi.
org/10.1287/mksc.2017.1066.
Said, A., Fields, B., Jain, B.J., Albayrak, S., 2013. User-centric evaluation of a K-furthest
neighbor collaborative filtering recommender algorithm. In: Proceedings of the 2013
conference on Computer supported cooperative work companion, San Antonio,
Texas, USA. ACM, 2441955, pp. 1399–1408. 〈https://doi.org/10.1145/2441776.
2441933 .〉
Salonen, V., Karjaluoto, H., 2016. Web personalization: the state of the art and future
avenues for research and practice. Telemat. Inform. 33 (4), 1088–1104. https://doi.
org/10.1016/j.tele.2016.03.004.
Sawtooth Software, 2009. The CBC/HB System for Hierarchical Bayes Estimation: Version
5.0 Technical Paper https://www.sawtoothsoftware.com/download/techpap/〈
hbtech.pdf〉 (Accessed 3 October 2018).
Schafer, J.B., Konstan, J.A., Riedl, J., 2001. E-commerce recommendation applications.
Data Min. Knowl. Discov. 5 (1–2), 115–153. https://doi.org/10.1023/
A:1009804230409.
Senecal, S., Nantel, J., 2004. The influence of online product recommendations on con-
sumers'online choices. J. Retail. 80 (2), 159–169. https://doi.org/10.1016/j.jretai.
2004.04.001.
Sheehan, K.B., 1999. An investigation of gender differences in on-line privacy concerns
and resultant behaviors. J. Interact. Mark. 13 (4), 24–38. https://doi.org/10.1002/
(SICI)1520-6653(199923)13:4%3C24::AID-DIR3%3E3.0.CO;2-O.
Sheng, H., Nah, F.F.-H., Siau, K., 2008. An experimental study on ubiquitous commerce
adoption: impact of personalization and privacy concerns. J. Assoc. Inf. Syst. 9 (6),
344–376. https://aisel.aisnet.org/jais/vol9/iss6/15/〈 〉.
Shephard, A., Pookulangara, S., Kinley, T.R., Josiam, B.M., 2016. Media influence,
fashion, and shopping: a gender perspective. J. Fash. Mark. Manag. 20 (1), 4–18.
https://doi.org/10.1108/JFMM-09-2014-0068.
Smith, B., Linden, G., 2017. Two decades of recommender systems at Amazon. com. IEEE
Internet Comput. 21 (3), 12–18. https://doi.org/10.1109/MIC.2017.72.
Smith, K.T., 2011. Digital marketing strategies that Millennials find appealing, moti-
vating, or just annoying. J. Strateg. Mark. 19 (6), 489–499. https://doi.org/10.1080/
0965254X.2011.581383.
Solomon, M.R., 1983. The role of products as social stimuli: a symbolic interactionism
perspective. J. Consum. Res. 10 (3), 319–329. https://doi.org/10.1086/208971.
Song, J.H., Kim, H.Y., Kim, S., Lee, S.W., Lee, J.-H., 2016. Effects of personalized e-mail
messages on privacy risk: moderating roles of control and intimacy. Mark. Lett. 27
(1), 89–101. https://doi.org/10.1007/s11002-014-9315-0.
Speck, P.S., Elliott, M.T., 1997. Predictors of advertising avoidance in print and broadcast
media. J. Advert. 26 (3), 61–76. https://doi.org/10.1080/00913367.1997.
10673529.
Sundar, S.S., Marathe, S.S., 2010. Personalization versus customization: the importance of
agency, privacy, and power usage. Hum. Commun. Res. 36 (3), 298–322. https://doi.
org/10.1111/j.1468-2958.2010.01377.x.
Surprenant, C.F., Solomon, M.R., 1987. Predictability and personalization in the service
encounter. J. Mark. 51 (2), 86–96. https://doi.org/10.2307/1251131.
Symeonidis, P., Nanopoulos, A., Manolopoulos, Y., 2009. MoviExplain: a recommender
system with explanations. In: Proceedings of the Third ACM Conference on
Recommender Systems. ACM, New York, NY, USA, pp. 317–320. 〈https://doi.org/10.
1145/1639714.1639777 .〉
Tam, K.Y., Ho, S.Y., 2005. Web personalization as a persuasion strategy: an elaboration
likelihood model perspective. Inf. Syst. Res. 16 (3), 271–291. https://doi.org/10.
1287/isre.1050.0058.
Tam, K.Y., Ho, S.Y., 2006. Understanding the impact of web personalization on user in-
formation processing and decision outcomes. MIS Q. 30 (4), 865–890. https://doi.
org/10.2307/25148757.
Tamimi, N., Sebastianelli, R., 2015. The relative importance of e-tailer website attributes
on the likelihood of online purchase. Internet Res. 25 (2), 169–183. https://doi.org/
10.1108/IntR-07-2013-0131.
T. Schreiner, et al. Journal of Retailing and Consumer Services 48 (2019) 87–99
98
personalization and bandwagon cues on users'attitudes and behavioral intentions
toward a restaurant recommendation website. Comput. Hum. Behav. 65 (Supplement
C), 369–379. https://doi.org/10.1016/j.chb.2016.08.038.
Kim, S., Choi, S.M., 2012. Credibility cues in online shopping: an examination of cor-
porate credibility, retailer reputation, and product review credibility. Int. J. Internet
Mark. Advert. 7 (3), 217–236. https://doi.org/10.1504/IJIMA.2012.047425.
Kireyev, P., Pauwels, K., Gupta, S., 2016. Do display ads influence search? Attribution and
dynamics in online advertising. Int. J. Res. Mark. 33 (3), 475–490. https://doi.org/
10.1016/j.ijresmar.2015.09.007.
Knijnenburg, B.P., Willemsen, M.C., Gantner, Z., Soncu, H., Newell, C., 2012. Explaining
the user experience of recommender systems. Use. Model. Use.-Adapt. Interact. 22
(4–5), 441–504. https://doi.org/10.1007/s11257-011-9118-4.
Knijnenburg, B.P., Willemsen, M.C., Hirtbach, S., 2010. Receiving recommendations and
providing feedback: The user-experience of a recommender system. In: Buccafurri, F.,
Semeraro, G. (Eds.), E-Commerce and web technologies: 11th International
Conference, EC-Web 2010, Bilbao, Spain, September 1–3, 2010, Proceedings.
Springer Berlin Heidelberg, Berlin, Heidelberg, pp. 207–216. 〈https://doi.org/10.
1007/978-3-642-15208-5_19 .〉
Kwon, K., Kim, C., 2012. How to design personalization in a context of customer reten-
tion: who personalizes what and to what extent? Electron. Commer. Res. Appl. 11 (2),
101–116. https://doi.org/10.1016/j.elerap.2011.05.002.
Lafferty, B.A., Goldsmith, R.E., 1999. Corporate credibility’s role in consumers'attitudes
and purchase intentions when a high versus a low credibility endorser is used in the
ad. J. Bus. Res. 44 (2), 109–116. https://doi.org/10.1016/S0148-2963(98)00002-2.
Lambrecht, A., Tucker, C., 2013. When does retargeting work? Information specificity in
online advertising. J. Mark. Res. 50 (5), 561–576.
Lee, E.-J., Park, J.K., 2009. Online service personalization for apparel shopping. J. Retail.
Consum. Serv. 16 (2), 83–91. https://doi.org/10.1016/j.jretconser.2008.10.003.
Li, C., Liu, J., 2017. A name alone is not enough: a reexamination of web-based perso-
nalization effect. Comput. Hum. Behav. 72, 132–139. https://doi.org/10.1016/j.chb.
2017.02.039.
Li, H., Kannan, P.K., 2014. Attributing conversions in a multichannel online marketing
environment: an empirical model and a field experiment. J. Mark. Res. 51 (1), 40–56.
https://doi.org/10.1509/jmr.13.0050.
Lin, Z., 2014. An empirical investigation of user and system recommendations in e-
commerce. Decis. Support Syst. 68, 111–124. https://doi.org/10.1016/j.dss.2014.10.
003.
Linden, G., Smith, B., York, J., 2003. Amazon.com recommendations: item-to-item col-
laborative filtering. IEEE Internet Comput. 7 (1), 76–80. https://doi.org/10.1109/
MIC.2003.1167344.
Louviere, J.J., Woodworth, G., 1983. Design and analysis of simulated consumer choice
or allocation experiments: an approach based on aggregate data. J. Mark. Res. 20 (4),
350–367. https://doi.org/10.2307/3151440.
Marcus, D.K., Miller, R.S., 2003. Sex differences in judgments of physical attractiveness: a
social relations analysis. Personal. Soc. Psychol. Bull. 29 (3), 325–335. 〈https://doi.
org/10.1177%2F0146167202250193 .〉
Meulenaer, S., de, Dens, N., Pelsmacker, P. de, 2015. Which cues cause consumers to
perceive brands as more global? A conjoint analysis. Int. Mark. Rev. 32 (6), 606–626.
https://doi.org/10.1108/IMR-04-2014-0144.
Millan, E., Wright, L.T., 2018. Gender effects on consumers'symbolic and hedonic pre-
ferences and actual clothing consumption in the Czech Republic. Int. J. Consum.
Stud. 42 (5), 478–488. https://doi.org/10.1111/ijcs.12447.
Mittal, B., Lassar, W.M., 1996. The role of personalization in service encounters. J. Retail.
72 (1), 95–109. https://doi.org/10.1016/S0022-4359(96)90007-X.
Nanou, T., Lekakos, G., Fouskas, K., 2010. The effects of recommendations'presentation
on persuasion and satisfaction in a movie recommender system. Multimed. Syst. 16
(4), 219–230. https://doi.org/10.1007/s00530-010-0190-0.
Neslin, S.A., Grewal, D., Leghorn, R., Shankar, V., Teerling, M.L., Thomas, J.S., Verhoef,
P.C., 2006. Challenges and opportunities in multichannel customer management. J.
Serv. Res. 9 (2), 95–112. https://doi.org/10.1177/1094670506293559.
Nilashi, M., Jannach, D., Ibrahim, Ob, Esfahani, M.D., Ahmadi, H., 2016.
Recommendation quality, transparency, and website quality for trust-building in
recommendation agents. Electron. Commer. Res. Appl. 19 (Suppl. C), 70–84. https://
doi.org/10.1016/j.elerap.2016.09.003.
O’Cass, A., 2000. An assessment of consumers product, purchase decision, advertising and
consumption involvement in fashion clothing. J. Econ. Psychol. 21 (5), 545–576.
https://doi.org/10.1016/S0167-4870(00)00018-0.
Orme, B., 2009. Fine-tuning CBC and adaptive CBC questionnaires http://www.〈
sawtoothsoftware.com/download/techpap/finetune.pdf〉 (Accessed 3 October 2018).
Otnes, C., McGrath, M.A., 2001. Perceptions and realities of male shopping behavior. J.
Retail. 77 (1), 111–137. https://doi.org/10.1016/S0022-4359(00)00047-6.
Ozok, A.A., Fan, Q., Norcio, A.F., 2010. Design guidelines for effective recommender
system interfaces based on a usability criteria conceptual model: results from a col-
lege student population. Behav. Inf. Technol. 29 (1), 57–83. https://doi.org/10.
1080/01449290903004012.
PageFair, 2017. The state of the blocked web: 2017 Global Adblock Report. PageFair
〈https://pagefair.com/downloads/2017/01/PageFair-2017-Adblock-Report.pdf〉
(Accessed 3 October 2018).
Palfrey, J., Gasser, U., 2008. Born Digital: Understanding the First Generation of Digital
Natives. Basic Books, New York (381 pp).
Papadimitriou, A., Symeonidis, P., Manolopoulos, Y., 2012. A generalized taxonomy of
explanations styles for traditional and social recommender systems. Data Min. Knowl.
Discov. 24 (3), 555–583. https://doi.org/10.1007/s10618-011-0215-0.
Pappas, I.O., Kourouthanassis, P.E., Giannakos, M.N., Chrissikopoulos, V., 2014. Shiny
happy people buying: the role of emotions on personalized e-shopping. Electron.
Mark. 24 (3), 193–206. https://doi.org/10.1007/s12525-014-0153-y.
Pavlou, P.A., Stewart, D.W., 2000. Measuring the effects and effectiveness of interactive
advertising: a research agenda. J. Interact. Advert. 1 (1), 61–77. https://doi.org/10.
1080/15252019.2000.10722044.
Prensky, M., 2001. Digital natives, digital immigrants part 1. Horizon 9 (5), 1–6. https://
doi.org/10.1108/10748120110424816.
Pu, P., Chen, L., 2007. Trust-inspiring explanation interfaces for recommender systems.
Knowl.-Based Syst. 20 (6), 542–556. https://doi.org/10.1016/j.knosys.2007.04.004.
Pu, P., Chen, L., Hu, R., 2011. A user-centric evaluation framework for recommender
systems. In: Proceedings of the Fifth ACM Conference on Recommender systems. The
Fifth ACM Conference, Chicago, Illinois, USA. ACM, New York, NY, pp. 157–164.
〈https://doi.org/10.1145/2043932.2043962 .〉
Puzakova, M., Rocereto, J.F., Kwak, H., 2015. Ads are watching me. Int. J. Advert. 32 (4),
513–538. https://doi.org/10.2501/IJA-32-4-513-538.
Rangaswamy, A., van Bruggen, G.H., 2005. Opportunities and challenges in multichannel
marketing: an introduction to the special issue. J. Interact. Mark. 19 (2), 5–11.
https://doi.org/10.1002/dir.20037.
Ricci, F., Rokach, L., Shapira, B., 2015. Recommender systems: introduction and chal-
lenges. In: Ricci, F., Rokach, L., Shapira, B. (Eds.), Recommender Systems Handbook.
Springer US, Boston, MA, pp. 1–34.
Rojas-Méndez, J.I., Davies, G., Madran, C., 2009. Universal differences in advertising
avoidance behavior: a cross-cultural study. J. Bus. Res. 62 (10), 947–954. https://doi.
org/10.1016/j.jbusres.2008.08.008.
Rust, R.T., Huang, M.-H., 2014. The service revolution and the transformation of mar-
keting science. Mark. Sci. 33 (2), 206–221. https://doi.org/10.1287/mksc.2013.
0836.
Sahni, N.S., Wheeler, S.C., Chintagunta, P., 2018. Personalization in email marketing: the
role of noninformative advertising content. Mark. Sci. 37 (2), 236–258. https://doi.
org/10.1287/mksc.2017.1066.
Said, A., Fields, B., Jain, B.J., Albayrak, S., 2013. User-centric evaluation of a K-furthest
neighbor collaborative filtering recommender algorithm. In: Proceedings of the 2013
conference on Computer supported cooperative work companion, San Antonio,
Texas, USA. ACM, 2441955, pp. 1399–1408. 〈https://doi.org/10.1145/2441776.
2441933 .〉
Salonen, V., Karjaluoto, H., 2016. Web personalization: the state of the art and future
avenues for research and practice. Telemat. Inform. 33 (4), 1088–1104. https://doi.
org/10.1016/j.tele.2016.03.004.
Sawtooth Software, 2009. The CBC/HB System for Hierarchical Bayes Estimation: Version
5.0 Technical Paper https://www.sawtoothsoftware.com/download/techpap/〈
hbtech.pdf〉 (Accessed 3 October 2018).
Schafer, J.B., Konstan, J.A., Riedl, J., 2001. E-commerce recommendation applications.
Data Min. Knowl. Discov. 5 (1–2), 115–153. https://doi.org/10.1023/
A:1009804230409.
Senecal, S., Nantel, J., 2004. The influence of online product recommendations on con-
sumers'online choices. J. Retail. 80 (2), 159–169. https://doi.org/10.1016/j.jretai.
2004.04.001.
Sheehan, K.B., 1999. An investigation of gender differences in on-line privacy concerns
and resultant behaviors. J. Interact. Mark. 13 (4), 24–38. https://doi.org/10.1002/
(SICI)1520-6653(199923)13:4%3C24::AID-DIR3%3E3.0.CO;2-O.
Sheng, H., Nah, F.F.-H., Siau, K., 2008. An experimental study on ubiquitous commerce
adoption: impact of personalization and privacy concerns. J. Assoc. Inf. Syst. 9 (6),
344–376. https://aisel.aisnet.org/jais/vol9/iss6/15/〈 〉.
Shephard, A., Pookulangara, S., Kinley, T.R., Josiam, B.M., 2016. Media influence,
fashion, and shopping: a gender perspective. J. Fash. Mark. Manag. 20 (1), 4–18.
https://doi.org/10.1108/JFMM-09-2014-0068.
Smith, B., Linden, G., 2017. Two decades of recommender systems at Amazon. com. IEEE
Internet Comput. 21 (3), 12–18. https://doi.org/10.1109/MIC.2017.72.
Smith, K.T., 2011. Digital marketing strategies that Millennials find appealing, moti-
vating, or just annoying. J. Strateg. Mark. 19 (6), 489–499. https://doi.org/10.1080/
0965254X.2011.581383.
Solomon, M.R., 1983. The role of products as social stimuli: a symbolic interactionism
perspective. J. Consum. Res. 10 (3), 319–329. https://doi.org/10.1086/208971.
Song, J.H., Kim, H.Y., Kim, S., Lee, S.W., Lee, J.-H., 2016. Effects of personalized e-mail
messages on privacy risk: moderating roles of control and intimacy. Mark. Lett. 27
(1), 89–101. https://doi.org/10.1007/s11002-014-9315-0.
Speck, P.S., Elliott, M.T., 1997. Predictors of advertising avoidance in print and broadcast
media. J. Advert. 26 (3), 61–76. https://doi.org/10.1080/00913367.1997.
10673529.
Sundar, S.S., Marathe, S.S., 2010. Personalization versus customization: the importance of
agency, privacy, and power usage. Hum. Commun. Res. 36 (3), 298–322. https://doi.
org/10.1111/j.1468-2958.2010.01377.x.
Surprenant, C.F., Solomon, M.R., 1987. Predictability and personalization in the service
encounter. J. Mark. 51 (2), 86–96. https://doi.org/10.2307/1251131.
Symeonidis, P., Nanopoulos, A., Manolopoulos, Y., 2009. MoviExplain: a recommender
system with explanations. In: Proceedings of the Third ACM Conference on
Recommender Systems. ACM, New York, NY, USA, pp. 317–320. 〈https://doi.org/10.
1145/1639714.1639777 .〉
Tam, K.Y., Ho, S.Y., 2005. Web personalization as a persuasion strategy: an elaboration
likelihood model perspective. Inf. Syst. Res. 16 (3), 271–291. https://doi.org/10.
1287/isre.1050.0058.
Tam, K.Y., Ho, S.Y., 2006. Understanding the impact of web personalization on user in-
formation processing and decision outcomes. MIS Q. 30 (4), 865–890. https://doi.
org/10.2307/25148757.
Tamimi, N., Sebastianelli, R., 2015. The relative importance of e-tailer website attributes
on the likelihood of online purchase. Internet Res. 25 (2), 169–183. https://doi.org/
10.1108/IntR-07-2013-0131.
T. Schreiner, et al. Journal of Retailing and Consumer Services 48 (2019) 87–99
98
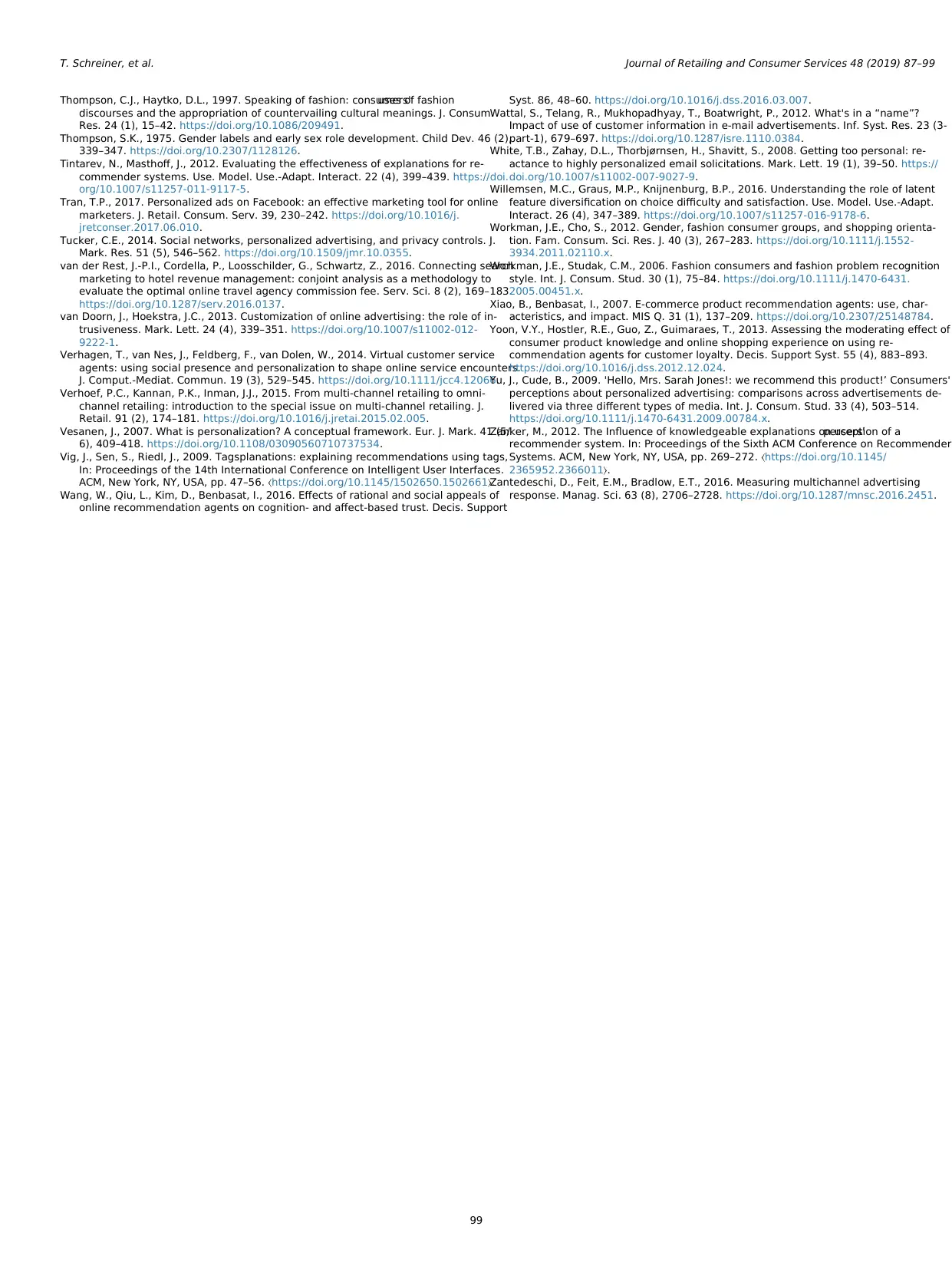
Thompson, C.J., Haytko, D.L., 1997. Speaking of fashion: consumers'uses of fashion
discourses and the appropriation of countervailing cultural meanings. J. Consum.
Res. 24 (1), 15–42. https://doi.org/10.1086/209491.
Thompson, S.K., 1975. Gender labels and early sex role development. Child Dev. 46 (2),
339–347. https://doi.org/10.2307/1128126.
Tintarev, N., Masthoff, J., 2012. Evaluating the effectiveness of explanations for re-
commender systems. Use. Model. Use.-Adapt. Interact. 22 (4), 399–439. https://doi.
org/10.1007/s11257-011-9117-5.
Tran, T.P., 2017. Personalized ads on Facebook: an effective marketing tool for online
marketers. J. Retail. Consum. Serv. 39, 230–242. https://doi.org/10.1016/j.
jretconser.2017.06.010.
Tucker, C.E., 2014. Social networks, personalized advertising, and privacy controls. J.
Mark. Res. 51 (5), 546–562. https://doi.org/10.1509/jmr.10.0355.
van der Rest, J.-P.I., Cordella, P., Loosschilder, G., Schwartz, Z., 2016. Connecting search
marketing to hotel revenue management: conjoint analysis as a methodology to
evaluate the optimal online travel agency commission fee. Serv. Sci. 8 (2), 169–183.
https://doi.org/10.1287/serv.2016.0137.
van Doorn, J., Hoekstra, J.C., 2013. Customization of online advertising: the role of in-
trusiveness. Mark. Lett. 24 (4), 339–351. https://doi.org/10.1007/s11002-012-
9222-1.
Verhagen, T., van Nes, J., Feldberg, F., van Dolen, W., 2014. Virtual customer service
agents: using social presence and personalization to shape online service encounters.
J. Comput.-Mediat. Commun. 19 (3), 529–545. https://doi.org/10.1111/jcc4.12066.
Verhoef, P.C., Kannan, P.K., Inman, J.J., 2015. From multi-channel retailing to omni-
channel retailing: introduction to the special issue on multi-channel retailing. J.
Retail. 91 (2), 174–181. https://doi.org/10.1016/j.jretai.2015.02.005.
Vesanen, J., 2007. What is personalization? A conceptual framework. Eur. J. Mark. 41 (5/
6), 409–418. https://doi.org/10.1108/03090560710737534.
Vig, J., Sen, S., Riedl, J., 2009. Tagsplanations: explaining recommendations using tags,
In: Proceedings of the 14th International Conference on Intelligent User Interfaces.
ACM, New York, NY, USA, pp. 47–56. 〈https://doi.org/10.1145/1502650.1502661 .〉
Wang, W., Qiu, L., Kim, D., Benbasat, I., 2016. Effects of rational and social appeals of
online recommendation agents on cognition- and affect-based trust. Decis. Support
Syst. 86, 48–60. https://doi.org/10.1016/j.dss.2016.03.007.
Wattal, S., Telang, R., Mukhopadhyay, T., Boatwright, P., 2012. What's in a “name”?
Impact of use of customer information in e-mail advertisements. Inf. Syst. Res. 23 (3-
part-1), 679–697. https://doi.org/10.1287/isre.1110.0384.
White, T.B., Zahay, D.L., Thorbjørnsen, H., Shavitt, S., 2008. Getting too personal: re-
actance to highly personalized email solicitations. Mark. Lett. 19 (1), 39–50. https://
doi.org/10.1007/s11002-007-9027-9.
Willemsen, M.C., Graus, M.P., Knijnenburg, B.P., 2016. Understanding the role of latent
feature diversification on choice difficulty and satisfaction. Use. Model. Use.-Adapt.
Interact. 26 (4), 347–389. https://doi.org/10.1007/s11257-016-9178-6.
Workman, J.E., Cho, S., 2012. Gender, fashion consumer groups, and shopping orienta-
tion. Fam. Consum. Sci. Res. J. 40 (3), 267–283. https://doi.org/10.1111/j.1552-
3934.2011.02110.x.
Workman, J.E., Studak, C.M., 2006. Fashion consumers and fashion problem recognition
style. Int. J. Consum. Stud. 30 (1), 75–84. https://doi.org/10.1111/j.1470-6431.
2005.00451.x.
Xiao, B., Benbasat, I., 2007. E-commerce product recommendation agents: use, char-
acteristics, and impact. MIS Q. 31 (1), 137–209. https://doi.org/10.2307/25148784.
Yoon, V.Y., Hostler, R.E., Guo, Z., Guimaraes, T., 2013. Assessing the moderating effect of
consumer product knowledge and online shopping experience on using re-
commendation agents for customer loyalty. Decis. Support Syst. 55 (4), 883–893.
https://doi.org/10.1016/j.dss.2012.12.024.
Yu, J., Cude, B., 2009. 'Hello, Mrs. Sarah Jones!: we recommend this product!’ Consumers'
perceptions about personalized advertising: comparisons across advertisements de-
livered via three different types of media. Int. J. Consum. Stud. 33 (4), 503–514.
https://doi.org/10.1111/j.1470-6431.2009.00784.x.
Zanker, M., 2012. The Influence of knowledgeable explanations on users'perception of a
recommender system. In: Proceedings of the Sixth ACM Conference on Recommender
Systems. ACM, New York, NY, USA, pp. 269–272. 〈https://doi.org/10.1145/
2365952.2366011 .〉
Zantedeschi, D., Feit, E.M., Bradlow, E.T., 2016. Measuring multichannel advertising
response. Manag. Sci. 63 (8), 2706–2728. https://doi.org/10.1287/mnsc.2016.2451.
T. Schreiner, et al. Journal of Retailing and Consumer Services 48 (2019) 87–99
99
discourses and the appropriation of countervailing cultural meanings. J. Consum.
Res. 24 (1), 15–42. https://doi.org/10.1086/209491.
Thompson, S.K., 1975. Gender labels and early sex role development. Child Dev. 46 (2),
339–347. https://doi.org/10.2307/1128126.
Tintarev, N., Masthoff, J., 2012. Evaluating the effectiveness of explanations for re-
commender systems. Use. Model. Use.-Adapt. Interact. 22 (4), 399–439. https://doi.
org/10.1007/s11257-011-9117-5.
Tran, T.P., 2017. Personalized ads on Facebook: an effective marketing tool for online
marketers. J. Retail. Consum. Serv. 39, 230–242. https://doi.org/10.1016/j.
jretconser.2017.06.010.
Tucker, C.E., 2014. Social networks, personalized advertising, and privacy controls. J.
Mark. Res. 51 (5), 546–562. https://doi.org/10.1509/jmr.10.0355.
van der Rest, J.-P.I., Cordella, P., Loosschilder, G., Schwartz, Z., 2016. Connecting search
marketing to hotel revenue management: conjoint analysis as a methodology to
evaluate the optimal online travel agency commission fee. Serv. Sci. 8 (2), 169–183.
https://doi.org/10.1287/serv.2016.0137.
van Doorn, J., Hoekstra, J.C., 2013. Customization of online advertising: the role of in-
trusiveness. Mark. Lett. 24 (4), 339–351. https://doi.org/10.1007/s11002-012-
9222-1.
Verhagen, T., van Nes, J., Feldberg, F., van Dolen, W., 2014. Virtual customer service
agents: using social presence and personalization to shape online service encounters.
J. Comput.-Mediat. Commun. 19 (3), 529–545. https://doi.org/10.1111/jcc4.12066.
Verhoef, P.C., Kannan, P.K., Inman, J.J., 2015. From multi-channel retailing to omni-
channel retailing: introduction to the special issue on multi-channel retailing. J.
Retail. 91 (2), 174–181. https://doi.org/10.1016/j.jretai.2015.02.005.
Vesanen, J., 2007. What is personalization? A conceptual framework. Eur. J. Mark. 41 (5/
6), 409–418. https://doi.org/10.1108/03090560710737534.
Vig, J., Sen, S., Riedl, J., 2009. Tagsplanations: explaining recommendations using tags,
In: Proceedings of the 14th International Conference on Intelligent User Interfaces.
ACM, New York, NY, USA, pp. 47–56. 〈https://doi.org/10.1145/1502650.1502661 .〉
Wang, W., Qiu, L., Kim, D., Benbasat, I., 2016. Effects of rational and social appeals of
online recommendation agents on cognition- and affect-based trust. Decis. Support
Syst. 86, 48–60. https://doi.org/10.1016/j.dss.2016.03.007.
Wattal, S., Telang, R., Mukhopadhyay, T., Boatwright, P., 2012. What's in a “name”?
Impact of use of customer information in e-mail advertisements. Inf. Syst. Res. 23 (3-
part-1), 679–697. https://doi.org/10.1287/isre.1110.0384.
White, T.B., Zahay, D.L., Thorbjørnsen, H., Shavitt, S., 2008. Getting too personal: re-
actance to highly personalized email solicitations. Mark. Lett. 19 (1), 39–50. https://
doi.org/10.1007/s11002-007-9027-9.
Willemsen, M.C., Graus, M.P., Knijnenburg, B.P., 2016. Understanding the role of latent
feature diversification on choice difficulty and satisfaction. Use. Model. Use.-Adapt.
Interact. 26 (4), 347–389. https://doi.org/10.1007/s11257-016-9178-6.
Workman, J.E., Cho, S., 2012. Gender, fashion consumer groups, and shopping orienta-
tion. Fam. Consum. Sci. Res. J. 40 (3), 267–283. https://doi.org/10.1111/j.1552-
3934.2011.02110.x.
Workman, J.E., Studak, C.M., 2006. Fashion consumers and fashion problem recognition
style. Int. J. Consum. Stud. 30 (1), 75–84. https://doi.org/10.1111/j.1470-6431.
2005.00451.x.
Xiao, B., Benbasat, I., 2007. E-commerce product recommendation agents: use, char-
acteristics, and impact. MIS Q. 31 (1), 137–209. https://doi.org/10.2307/25148784.
Yoon, V.Y., Hostler, R.E., Guo, Z., Guimaraes, T., 2013. Assessing the moderating effect of
consumer product knowledge and online shopping experience on using re-
commendation agents for customer loyalty. Decis. Support Syst. 55 (4), 883–893.
https://doi.org/10.1016/j.dss.2012.12.024.
Yu, J., Cude, B., 2009. 'Hello, Mrs. Sarah Jones!: we recommend this product!’ Consumers'
perceptions about personalized advertising: comparisons across advertisements de-
livered via three different types of media. Int. J. Consum. Stud. 33 (4), 503–514.
https://doi.org/10.1111/j.1470-6431.2009.00784.x.
Zanker, M., 2012. The Influence of knowledgeable explanations on users'perception of a
recommender system. In: Proceedings of the Sixth ACM Conference on Recommender
Systems. ACM, New York, NY, USA, pp. 269–272. 〈https://doi.org/10.1145/
2365952.2366011 .〉
Zantedeschi, D., Feit, E.M., Bradlow, E.T., 2016. Measuring multichannel advertising
response. Manag. Sci. 63 (8), 2706–2728. https://doi.org/10.1287/mnsc.2016.2451.
T. Schreiner, et al. Journal of Retailing and Consumer Services 48 (2019) 87–99
99
1 out of 13
Related Documents

Your All-in-One AI-Powered Toolkit for Academic Success.
+13062052269
info@desklib.com
Available 24*7 on WhatsApp / Email
Unlock your academic potential
© 2024 | Zucol Services PVT LTD | All rights reserved.