A Report on Proactive Analytics in Information System Research Role
VerifiedAdded on 2023/06/04
|8
|2744
|391
Report
AI Summary
This report delves into the significant role of predictive analytics within information systems research, highlighting its use in predicting future events through statistical modeling, data mining, and other tools. It emphasizes how organizations leverage predictive analytics to transform collected data into actionable insights, enabling proactive decision-making across various industries. The report discusses the application of predictive analytics in building and testing theories, improving existing models, and supporting empirical research by analyzing causal models and assessing predictive power. It contrasts predictive analytics with explanatory models, focusing on the importance of data-driven predictions, and underscores its relevance in today's rapidly changing business environment for innovation, resource management, and strategic planning. The report concludes that predictive analytics is essential for organizations to effectively utilize their information systems, anticipate future trends, and make informed decisions.
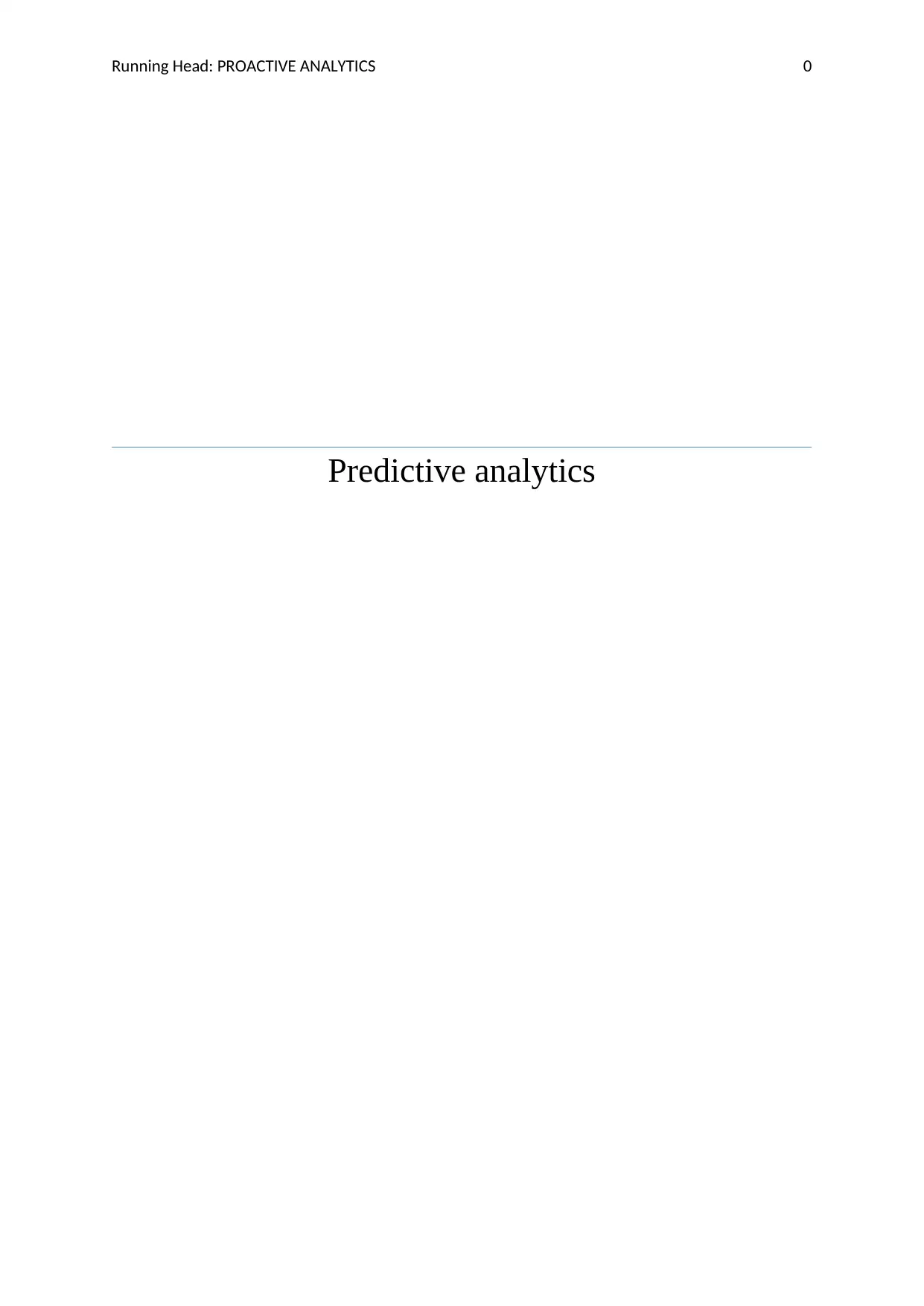
Running Head: PROACTIVE ANALYTICS 0
Predictive analytics
Predictive analytics
Paraphrase This Document
Need a fresh take? Get an instant paraphrase of this document with our AI Paraphraser
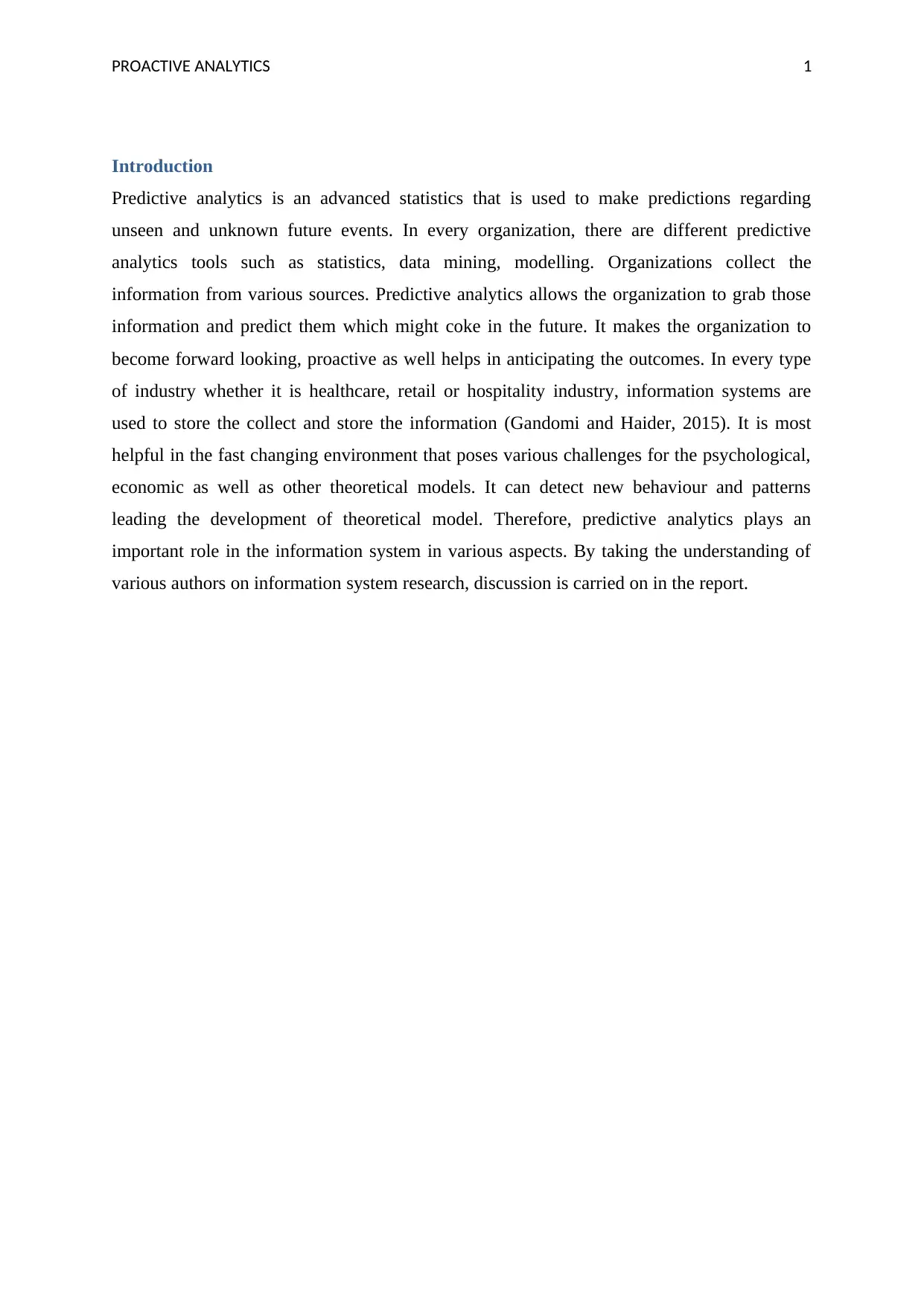
PROACTIVE ANALYTICS 1
Introduction
Predictive analytics is an advanced statistics that is used to make predictions regarding
unseen and unknown future events. In every organization, there are different predictive
analytics tools such as statistics, data mining, modelling. Organizations collect the
information from various sources. Predictive analytics allows the organization to grab those
information and predict them which might coke in the future. It makes the organization to
become forward looking, proactive as well helps in anticipating the outcomes. In every type
of industry whether it is healthcare, retail or hospitality industry, information systems are
used to store the collect and store the information (Gandomi and Haider, 2015). It is most
helpful in the fast changing environment that poses various challenges for the psychological,
economic as well as other theoretical models. It can detect new behaviour and patterns
leading the development of theoretical model. Therefore, predictive analytics plays an
important role in the information system in various aspects. By taking the understanding of
various authors on information system research, discussion is carried on in the report.
Introduction
Predictive analytics is an advanced statistics that is used to make predictions regarding
unseen and unknown future events. In every organization, there are different predictive
analytics tools such as statistics, data mining, modelling. Organizations collect the
information from various sources. Predictive analytics allows the organization to grab those
information and predict them which might coke in the future. It makes the organization to
become forward looking, proactive as well helps in anticipating the outcomes. In every type
of industry whether it is healthcare, retail or hospitality industry, information systems are
used to store the collect and store the information (Gandomi and Haider, 2015). It is most
helpful in the fast changing environment that poses various challenges for the psychological,
economic as well as other theoretical models. It can detect new behaviour and patterns
leading the development of theoretical model. Therefore, predictive analytics plays an
important role in the information system in various aspects. By taking the understanding of
various authors on information system research, discussion is carried on in the report.
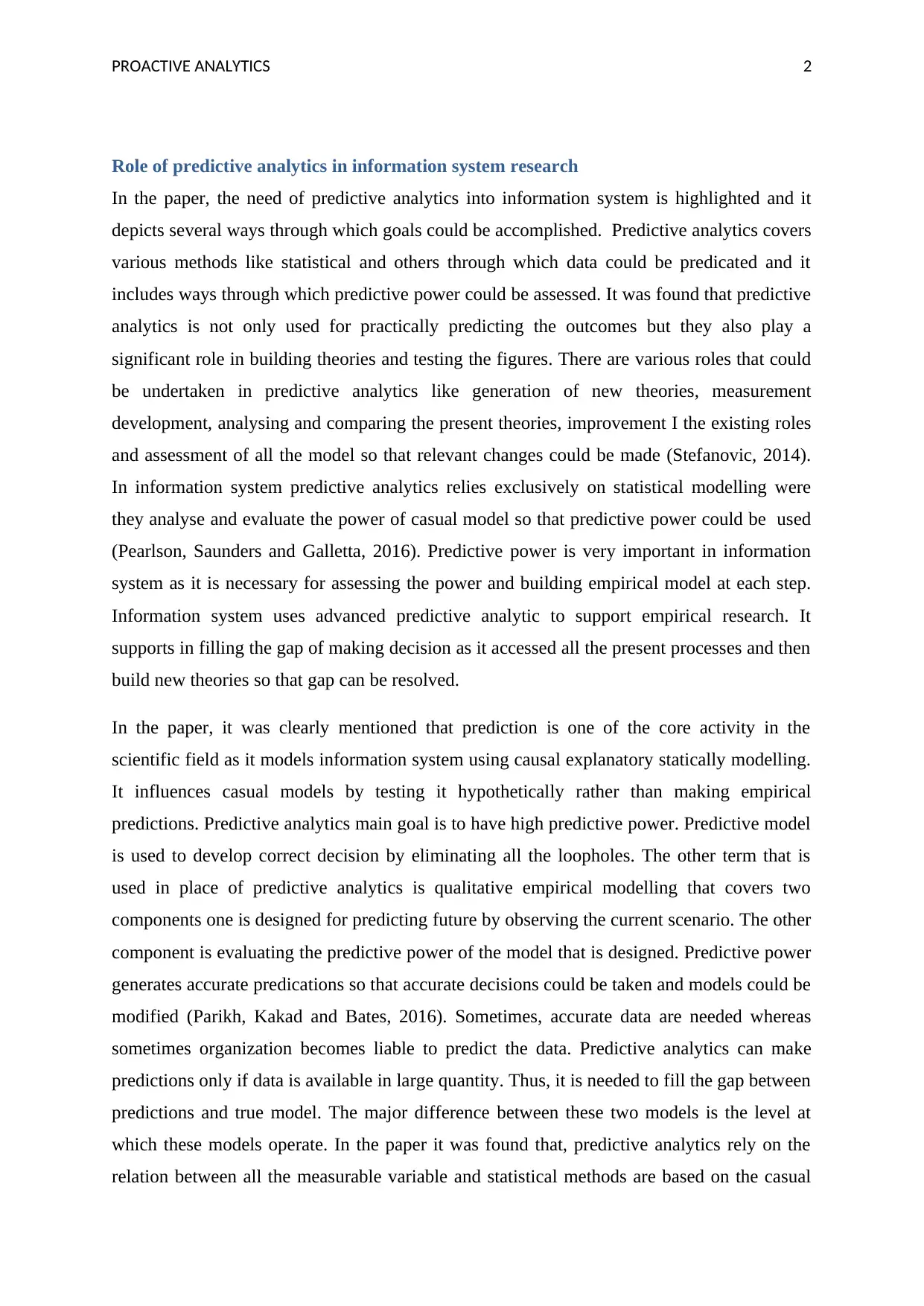
PROACTIVE ANALYTICS 2
Role of predictive analytics in information system research
In the paper, the need of predictive analytics into information system is highlighted and it
depicts several ways through which goals could be accomplished. Predictive analytics covers
various methods like statistical and others through which data could be predicated and it
includes ways through which predictive power could be assessed. It was found that predictive
analytics is not only used for practically predicting the outcomes but they also play a
significant role in building theories and testing the figures. There are various roles that could
be undertaken in predictive analytics like generation of new theories, measurement
development, analysing and comparing the present theories, improvement I the existing roles
and assessment of all the model so that relevant changes could be made (Stefanovic, 2014).
In information system predictive analytics relies exclusively on statistical modelling were
they analyse and evaluate the power of casual model so that predictive power could be used
(Pearlson, Saunders and Galletta, 2016). Predictive power is very important in information
system as it is necessary for assessing the power and building empirical model at each step.
Information system uses advanced predictive analytic to support empirical research. It
supports in filling the gap of making decision as it accessed all the present processes and then
build new theories so that gap can be resolved.
In the paper, it was clearly mentioned that prediction is one of the core activity in the
scientific field as it models information system using causal explanatory statically modelling.
It influences casual models by testing it hypothetically rather than making empirical
predictions. Predictive analytics main goal is to have high predictive power. Predictive model
is used to develop correct decision by eliminating all the loopholes. The other term that is
used in place of predictive analytics is qualitative empirical modelling that covers two
components one is designed for predicting future by observing the current scenario. The other
component is evaluating the predictive power of the model that is designed. Predictive power
generates accurate predications so that accurate decisions could be taken and models could be
modified (Parikh, Kakad and Bates, 2016). Sometimes, accurate data are needed whereas
sometimes organization becomes liable to predict the data. Predictive analytics can make
predictions only if data is available in large quantity. Thus, it is needed to fill the gap between
predictions and true model. The major difference between these two models is the level at
which these models operate. In the paper it was found that, predictive analytics rely on the
relation between all the measurable variable and statistical methods are based on the casual
Role of predictive analytics in information system research
In the paper, the need of predictive analytics into information system is highlighted and it
depicts several ways through which goals could be accomplished. Predictive analytics covers
various methods like statistical and others through which data could be predicated and it
includes ways through which predictive power could be assessed. It was found that predictive
analytics is not only used for practically predicting the outcomes but they also play a
significant role in building theories and testing the figures. There are various roles that could
be undertaken in predictive analytics like generation of new theories, measurement
development, analysing and comparing the present theories, improvement I the existing roles
and assessment of all the model so that relevant changes could be made (Stefanovic, 2014).
In information system predictive analytics relies exclusively on statistical modelling were
they analyse and evaluate the power of casual model so that predictive power could be used
(Pearlson, Saunders and Galletta, 2016). Predictive power is very important in information
system as it is necessary for assessing the power and building empirical model at each step.
Information system uses advanced predictive analytic to support empirical research. It
supports in filling the gap of making decision as it accessed all the present processes and then
build new theories so that gap can be resolved.
In the paper, it was clearly mentioned that prediction is one of the core activity in the
scientific field as it models information system using causal explanatory statically modelling.
It influences casual models by testing it hypothetically rather than making empirical
predictions. Predictive analytics main goal is to have high predictive power. Predictive model
is used to develop correct decision by eliminating all the loopholes. The other term that is
used in place of predictive analytics is qualitative empirical modelling that covers two
components one is designed for predicting future by observing the current scenario. The other
component is evaluating the predictive power of the model that is designed. Predictive power
generates accurate predications so that accurate decisions could be taken and models could be
modified (Parikh, Kakad and Bates, 2016). Sometimes, accurate data are needed whereas
sometimes organization becomes liable to predict the data. Predictive analytics can make
predictions only if data is available in large quantity. Thus, it is needed to fill the gap between
predictions and true model. The major difference between these two models is the level at
which these models operate. In the paper it was found that, predictive analytics rely on the
relation between all the measurable variable and statistical methods are based on the casual
⊘ This is a preview!⊘
Do you want full access?
Subscribe today to unlock all pages.

Trusted by 1+ million students worldwide
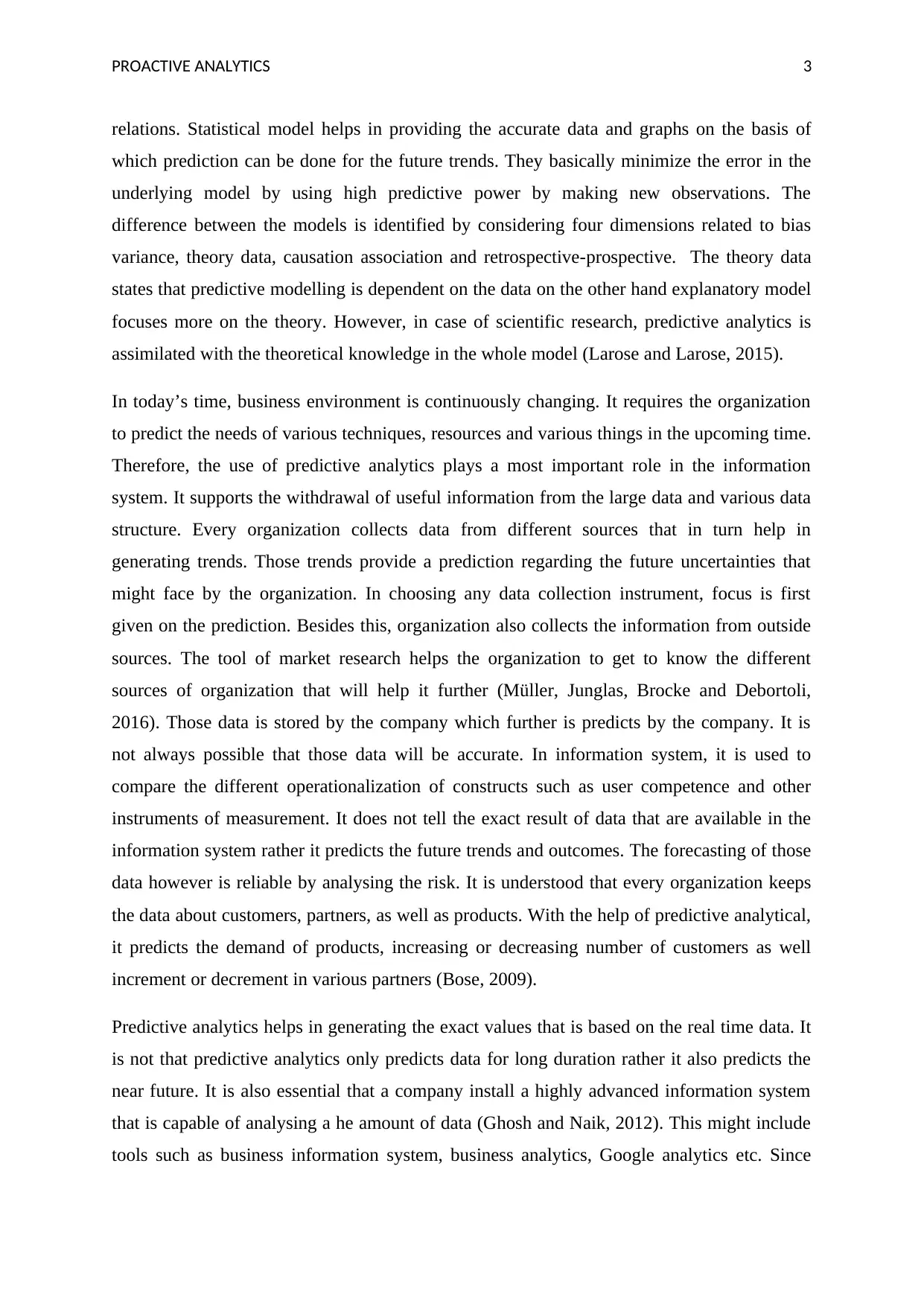
PROACTIVE ANALYTICS 3
relations. Statistical model helps in providing the accurate data and graphs on the basis of
which prediction can be done for the future trends. They basically minimize the error in the
underlying model by using high predictive power by making new observations. The
difference between the models is identified by considering four dimensions related to bias
variance, theory data, causation association and retrospective-prospective. The theory data
states that predictive modelling is dependent on the data on the other hand explanatory model
focuses more on the theory. However, in case of scientific research, predictive analytics is
assimilated with the theoretical knowledge in the whole model (Larose and Larose, 2015).
In today’s time, business environment is continuously changing. It requires the organization
to predict the needs of various techniques, resources and various things in the upcoming time.
Therefore, the use of predictive analytics plays a most important role in the information
system. It supports the withdrawal of useful information from the large data and various data
structure. Every organization collects data from different sources that in turn help in
generating trends. Those trends provide a prediction regarding the future uncertainties that
might face by the organization. In choosing any data collection instrument, focus is first
given on the prediction. Besides this, organization also collects the information from outside
sources. The tool of market research helps the organization to get to know the different
sources of organization that will help it further (Müller, Junglas, Brocke and Debortoli,
2016). Those data is stored by the company which further is predicts by the company. It is
not always possible that those data will be accurate. In information system, it is used to
compare the different operationalization of constructs such as user competence and other
instruments of measurement. It does not tell the exact result of data that are available in the
information system rather it predicts the future trends and outcomes. The forecasting of those
data however is reliable by analysing the risk. It is understood that every organization keeps
the data about customers, partners, as well as products. With the help of predictive analytical,
it predicts the demand of products, increasing or decreasing number of customers as well
increment or decrement in various partners (Bose, 2009).
Predictive analytics helps in generating the exact values that is based on the real time data. It
is not that predictive analytics only predicts data for long duration rather it also predicts the
near future. It is also essential that a company install a highly advanced information system
that is capable of analysing a he amount of data (Ghosh and Naik, 2012). This might include
tools such as business information system, business analytics, Google analytics etc. Since
relations. Statistical model helps in providing the accurate data and graphs on the basis of
which prediction can be done for the future trends. They basically minimize the error in the
underlying model by using high predictive power by making new observations. The
difference between the models is identified by considering four dimensions related to bias
variance, theory data, causation association and retrospective-prospective. The theory data
states that predictive modelling is dependent on the data on the other hand explanatory model
focuses more on the theory. However, in case of scientific research, predictive analytics is
assimilated with the theoretical knowledge in the whole model (Larose and Larose, 2015).
In today’s time, business environment is continuously changing. It requires the organization
to predict the needs of various techniques, resources and various things in the upcoming time.
Therefore, the use of predictive analytics plays a most important role in the information
system. It supports the withdrawal of useful information from the large data and various data
structure. Every organization collects data from different sources that in turn help in
generating trends. Those trends provide a prediction regarding the future uncertainties that
might face by the organization. In choosing any data collection instrument, focus is first
given on the prediction. Besides this, organization also collects the information from outside
sources. The tool of market research helps the organization to get to know the different
sources of organization that will help it further (Müller, Junglas, Brocke and Debortoli,
2016). Those data is stored by the company which further is predicts by the company. It is
not always possible that those data will be accurate. In information system, it is used to
compare the different operationalization of constructs such as user competence and other
instruments of measurement. It does not tell the exact result of data that are available in the
information system rather it predicts the future trends and outcomes. The forecasting of those
data however is reliable by analysing the risk. It is understood that every organization keeps
the data about customers, partners, as well as products. With the help of predictive analytical,
it predicts the demand of products, increasing or decreasing number of customers as well
increment or decrement in various partners (Bose, 2009).
Predictive analytics helps in generating the exact values that is based on the real time data. It
is not that predictive analytics only predicts data for long duration rather it also predicts the
near future. It is also essential that a company install a highly advanced information system
that is capable of analysing a he amount of data (Ghosh and Naik, 2012). This might include
tools such as business information system, business analytics, Google analytics etc. Since
Paraphrase This Document
Need a fresh take? Get an instant paraphrase of this document with our AI Paraphraser
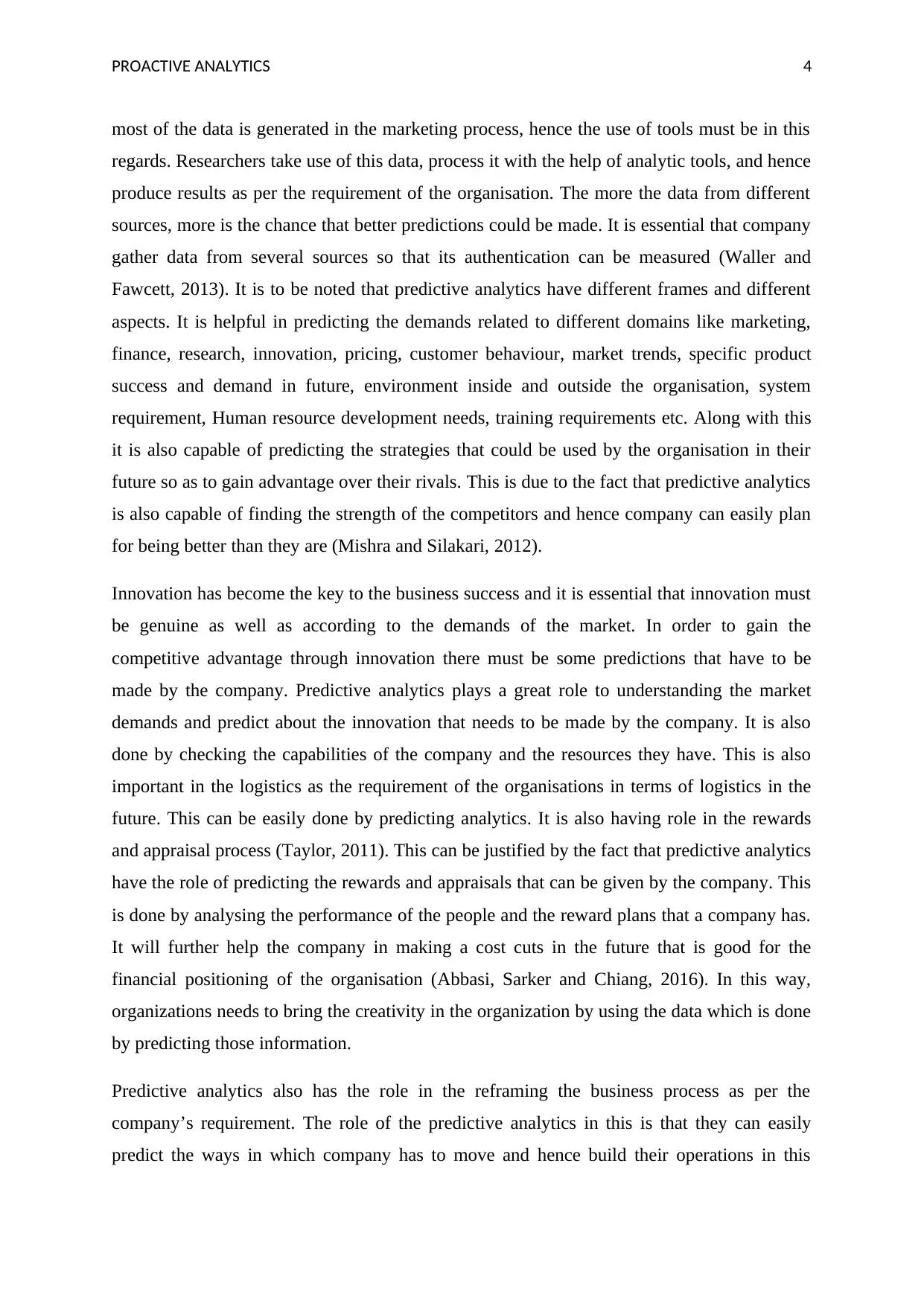
PROACTIVE ANALYTICS 4
most of the data is generated in the marketing process, hence the use of tools must be in this
regards. Researchers take use of this data, process it with the help of analytic tools, and hence
produce results as per the requirement of the organisation. The more the data from different
sources, more is the chance that better predictions could be made. It is essential that company
gather data from several sources so that its authentication can be measured (Waller and
Fawcett, 2013). It is to be noted that predictive analytics have different frames and different
aspects. It is helpful in predicting the demands related to different domains like marketing,
finance, research, innovation, pricing, customer behaviour, market trends, specific product
success and demand in future, environment inside and outside the organisation, system
requirement, Human resource development needs, training requirements etc. Along with this
it is also capable of predicting the strategies that could be used by the organisation in their
future so as to gain advantage over their rivals. This is due to the fact that predictive analytics
is also capable of finding the strength of the competitors and hence company can easily plan
for being better than they are (Mishra and Silakari, 2012).
Innovation has become the key to the business success and it is essential that innovation must
be genuine as well as according to the demands of the market. In order to gain the
competitive advantage through innovation there must be some predictions that have to be
made by the company. Predictive analytics plays a great role to understanding the market
demands and predict about the innovation that needs to be made by the company. It is also
done by checking the capabilities of the company and the resources they have. This is also
important in the logistics as the requirement of the organisations in terms of logistics in the
future. This can be easily done by predicting analytics. It is also having role in the rewards
and appraisal process (Taylor, 2011). This can be justified by the fact that predictive analytics
have the role of predicting the rewards and appraisals that can be given by the company. This
is done by analysing the performance of the people and the reward plans that a company has.
It will further help the company in making a cost cuts in the future that is good for the
financial positioning of the organisation (Abbasi, Sarker and Chiang, 2016). In this way,
organizations needs to bring the creativity in the organization by using the data which is done
by predicting those information.
Predictive analytics also has the role in the reframing the business process as per the
company’s requirement. The role of the predictive analytics in this is that they can easily
predict the ways in which company has to move and hence build their operations in this
most of the data is generated in the marketing process, hence the use of tools must be in this
regards. Researchers take use of this data, process it with the help of analytic tools, and hence
produce results as per the requirement of the organisation. The more the data from different
sources, more is the chance that better predictions could be made. It is essential that company
gather data from several sources so that its authentication can be measured (Waller and
Fawcett, 2013). It is to be noted that predictive analytics have different frames and different
aspects. It is helpful in predicting the demands related to different domains like marketing,
finance, research, innovation, pricing, customer behaviour, market trends, specific product
success and demand in future, environment inside and outside the organisation, system
requirement, Human resource development needs, training requirements etc. Along with this
it is also capable of predicting the strategies that could be used by the organisation in their
future so as to gain advantage over their rivals. This is due to the fact that predictive analytics
is also capable of finding the strength of the competitors and hence company can easily plan
for being better than they are (Mishra and Silakari, 2012).
Innovation has become the key to the business success and it is essential that innovation must
be genuine as well as according to the demands of the market. In order to gain the
competitive advantage through innovation there must be some predictions that have to be
made by the company. Predictive analytics plays a great role to understanding the market
demands and predict about the innovation that needs to be made by the company. It is also
done by checking the capabilities of the company and the resources they have. This is also
important in the logistics as the requirement of the organisations in terms of logistics in the
future. This can be easily done by predicting analytics. It is also having role in the rewards
and appraisal process (Taylor, 2011). This can be justified by the fact that predictive analytics
have the role of predicting the rewards and appraisals that can be given by the company. This
is done by analysing the performance of the people and the reward plans that a company has.
It will further help the company in making a cost cuts in the future that is good for the
financial positioning of the organisation (Abbasi, Sarker and Chiang, 2016). In this way,
organizations needs to bring the creativity in the organization by using the data which is done
by predicting those information.
Predictive analytics also has the role in the reframing the business process as per the
company’s requirement. The role of the predictive analytics in this is that they can easily
predict the ways in which company has to move and hence build their operations in this
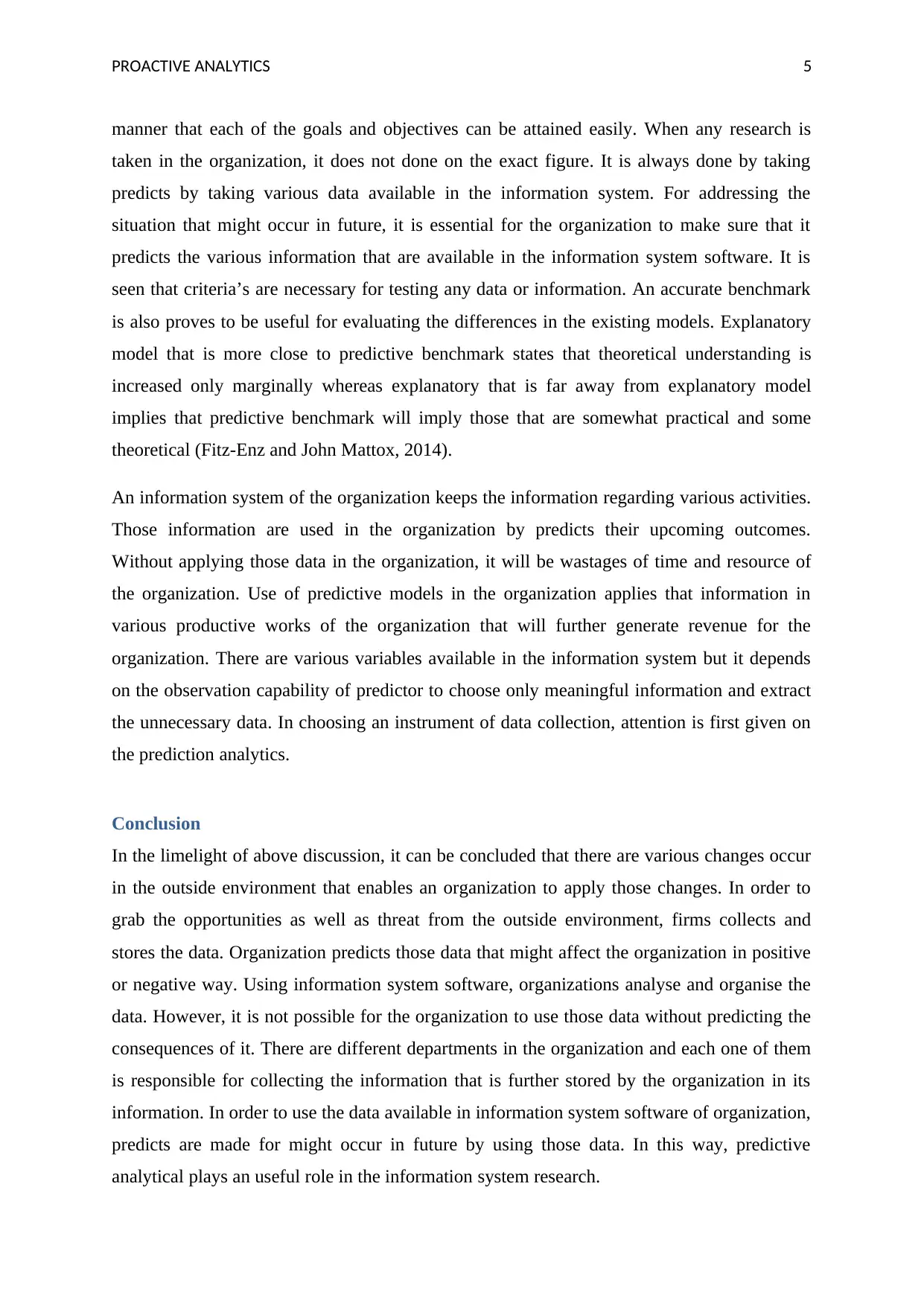
PROACTIVE ANALYTICS 5
manner that each of the goals and objectives can be attained easily. When any research is
taken in the organization, it does not done on the exact figure. It is always done by taking
predicts by taking various data available in the information system. For addressing the
situation that might occur in future, it is essential for the organization to make sure that it
predicts the various information that are available in the information system software. It is
seen that criteria’s are necessary for testing any data or information. An accurate benchmark
is also proves to be useful for evaluating the differences in the existing models. Explanatory
model that is more close to predictive benchmark states that theoretical understanding is
increased only marginally whereas explanatory that is far away from explanatory model
implies that predictive benchmark will imply those that are somewhat practical and some
theoretical (Fitz-Enz and John Mattox, 2014).
An information system of the organization keeps the information regarding various activities.
Those information are used in the organization by predicts their upcoming outcomes.
Without applying those data in the organization, it will be wastages of time and resource of
the organization. Use of predictive models in the organization applies that information in
various productive works of the organization that will further generate revenue for the
organization. There are various variables available in the information system but it depends
on the observation capability of predictor to choose only meaningful information and extract
the unnecessary data. In choosing an instrument of data collection, attention is first given on
the prediction analytics.
Conclusion
In the limelight of above discussion, it can be concluded that there are various changes occur
in the outside environment that enables an organization to apply those changes. In order to
grab the opportunities as well as threat from the outside environment, firms collects and
stores the data. Organization predicts those data that might affect the organization in positive
or negative way. Using information system software, organizations analyse and organise the
data. However, it is not possible for the organization to use those data without predicting the
consequences of it. There are different departments in the organization and each one of them
is responsible for collecting the information that is further stored by the organization in its
information. In order to use the data available in information system software of organization,
predicts are made for might occur in future by using those data. In this way, predictive
analytical plays an useful role in the information system research.
manner that each of the goals and objectives can be attained easily. When any research is
taken in the organization, it does not done on the exact figure. It is always done by taking
predicts by taking various data available in the information system. For addressing the
situation that might occur in future, it is essential for the organization to make sure that it
predicts the various information that are available in the information system software. It is
seen that criteria’s are necessary for testing any data or information. An accurate benchmark
is also proves to be useful for evaluating the differences in the existing models. Explanatory
model that is more close to predictive benchmark states that theoretical understanding is
increased only marginally whereas explanatory that is far away from explanatory model
implies that predictive benchmark will imply those that are somewhat practical and some
theoretical (Fitz-Enz and John Mattox, 2014).
An information system of the organization keeps the information regarding various activities.
Those information are used in the organization by predicts their upcoming outcomes.
Without applying those data in the organization, it will be wastages of time and resource of
the organization. Use of predictive models in the organization applies that information in
various productive works of the organization that will further generate revenue for the
organization. There are various variables available in the information system but it depends
on the observation capability of predictor to choose only meaningful information and extract
the unnecessary data. In choosing an instrument of data collection, attention is first given on
the prediction analytics.
Conclusion
In the limelight of above discussion, it can be concluded that there are various changes occur
in the outside environment that enables an organization to apply those changes. In order to
grab the opportunities as well as threat from the outside environment, firms collects and
stores the data. Organization predicts those data that might affect the organization in positive
or negative way. Using information system software, organizations analyse and organise the
data. However, it is not possible for the organization to use those data without predicting the
consequences of it. There are different departments in the organization and each one of them
is responsible for collecting the information that is further stored by the organization in its
information. In order to use the data available in information system software of organization,
predicts are made for might occur in future by using those data. In this way, predictive
analytical plays an useful role in the information system research.
⊘ This is a preview!⊘
Do you want full access?
Subscribe today to unlock all pages.

Trusted by 1+ million students worldwide
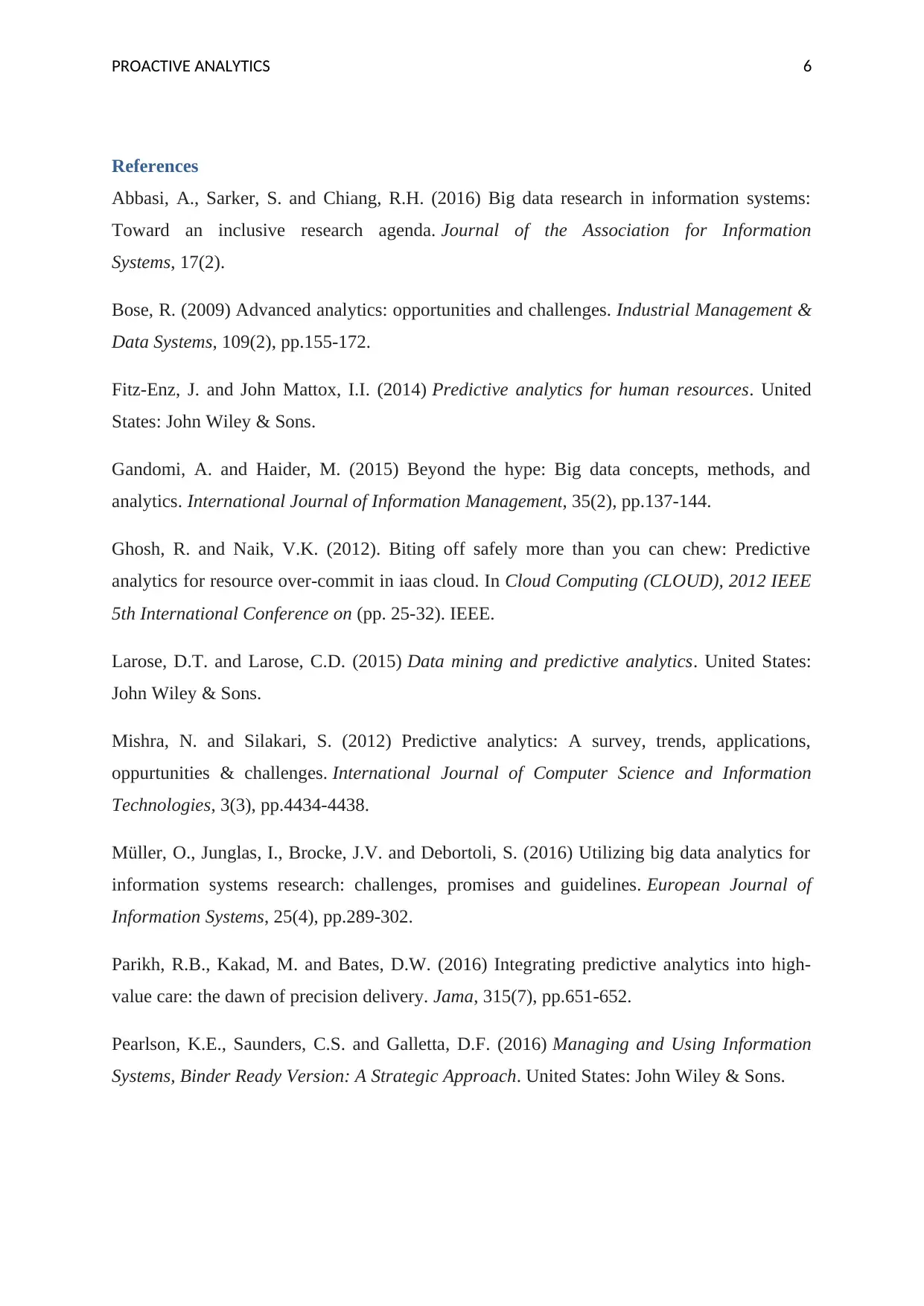
PROACTIVE ANALYTICS 6
References
Abbasi, A., Sarker, S. and Chiang, R.H. (2016) Big data research in information systems:
Toward an inclusive research agenda. Journal of the Association for Information
Systems, 17(2).
Bose, R. (2009) Advanced analytics: opportunities and challenges. Industrial Management &
Data Systems, 109(2), pp.155-172.
Fitz-Enz, J. and John Mattox, I.I. (2014) Predictive analytics for human resources. United
States: John Wiley & Sons.
Gandomi, A. and Haider, M. (2015) Beyond the hype: Big data concepts, methods, and
analytics. International Journal of Information Management, 35(2), pp.137-144.
Ghosh, R. and Naik, V.K. (2012). Biting off safely more than you can chew: Predictive
analytics for resource over-commit in iaas cloud. In Cloud Computing (CLOUD), 2012 IEEE
5th International Conference on (pp. 25-32). IEEE.
Larose, D.T. and Larose, C.D. (2015) Data mining and predictive analytics. United States:
John Wiley & Sons.
Mishra, N. and Silakari, S. (2012) Predictive analytics: A survey, trends, applications,
oppurtunities & challenges. International Journal of Computer Science and Information
Technologies, 3(3), pp.4434-4438.
Müller, O., Junglas, I., Brocke, J.V. and Debortoli, S. (2016) Utilizing big data analytics for
information systems research: challenges, promises and guidelines. European Journal of
Information Systems, 25(4), pp.289-302.
Parikh, R.B., Kakad, M. and Bates, D.W. (2016) Integrating predictive analytics into high-
value care: the dawn of precision delivery. Jama, 315(7), pp.651-652.
Pearlson, K.E., Saunders, C.S. and Galletta, D.F. (2016) Managing and Using Information
Systems, Binder Ready Version: A Strategic Approach. United States: John Wiley & Sons.
References
Abbasi, A., Sarker, S. and Chiang, R.H. (2016) Big data research in information systems:
Toward an inclusive research agenda. Journal of the Association for Information
Systems, 17(2).
Bose, R. (2009) Advanced analytics: opportunities and challenges. Industrial Management &
Data Systems, 109(2), pp.155-172.
Fitz-Enz, J. and John Mattox, I.I. (2014) Predictive analytics for human resources. United
States: John Wiley & Sons.
Gandomi, A. and Haider, M. (2015) Beyond the hype: Big data concepts, methods, and
analytics. International Journal of Information Management, 35(2), pp.137-144.
Ghosh, R. and Naik, V.K. (2012). Biting off safely more than you can chew: Predictive
analytics for resource over-commit in iaas cloud. In Cloud Computing (CLOUD), 2012 IEEE
5th International Conference on (pp. 25-32). IEEE.
Larose, D.T. and Larose, C.D. (2015) Data mining and predictive analytics. United States:
John Wiley & Sons.
Mishra, N. and Silakari, S. (2012) Predictive analytics: A survey, trends, applications,
oppurtunities & challenges. International Journal of Computer Science and Information
Technologies, 3(3), pp.4434-4438.
Müller, O., Junglas, I., Brocke, J.V. and Debortoli, S. (2016) Utilizing big data analytics for
information systems research: challenges, promises and guidelines. European Journal of
Information Systems, 25(4), pp.289-302.
Parikh, R.B., Kakad, M. and Bates, D.W. (2016) Integrating predictive analytics into high-
value care: the dawn of precision delivery. Jama, 315(7), pp.651-652.
Pearlson, K.E., Saunders, C.S. and Galletta, D.F. (2016) Managing and Using Information
Systems, Binder Ready Version: A Strategic Approach. United States: John Wiley & Sons.
Paraphrase This Document
Need a fresh take? Get an instant paraphrase of this document with our AI Paraphraser
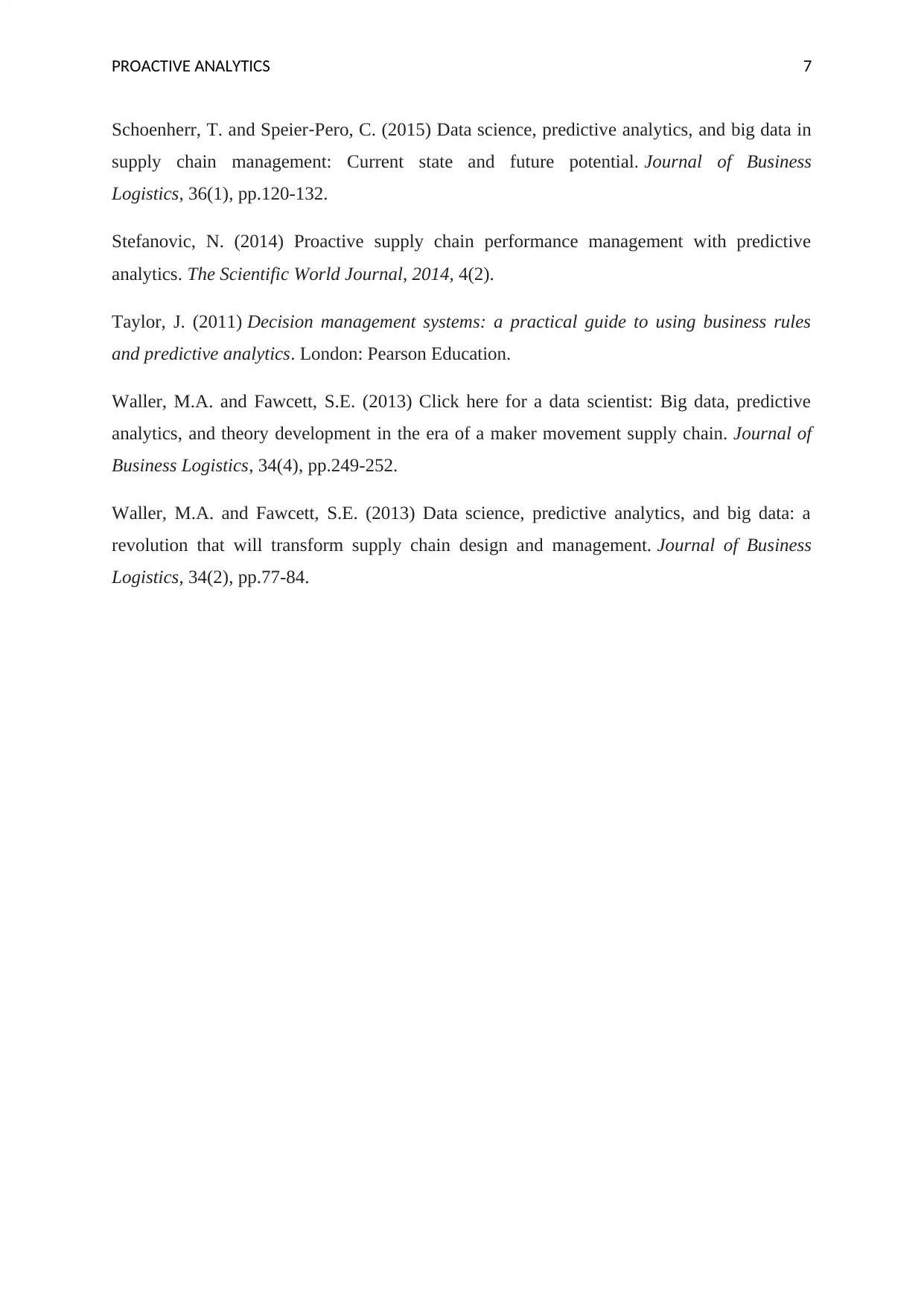
PROACTIVE ANALYTICS 7
Schoenherr, T. and Speier‐Pero, C. (2015) Data science, predictive analytics, and big data in
supply chain management: Current state and future potential. Journal of Business
Logistics, 36(1), pp.120-132.
Stefanovic, N. (2014) Proactive supply chain performance management with predictive
analytics. The Scientific World Journal, 2014, 4(2).
Taylor, J. (2011) Decision management systems: a practical guide to using business rules
and predictive analytics. London: Pearson Education.
Waller, M.A. and Fawcett, S.E. (2013) Click here for a data scientist: Big data, predictive
analytics, and theory development in the era of a maker movement supply chain. Journal of
Business Logistics, 34(4), pp.249-252.
Waller, M.A. and Fawcett, S.E. (2013) Data science, predictive analytics, and big data: a
revolution that will transform supply chain design and management. Journal of Business
Logistics, 34(2), pp.77-84.
Schoenherr, T. and Speier‐Pero, C. (2015) Data science, predictive analytics, and big data in
supply chain management: Current state and future potential. Journal of Business
Logistics, 36(1), pp.120-132.
Stefanovic, N. (2014) Proactive supply chain performance management with predictive
analytics. The Scientific World Journal, 2014, 4(2).
Taylor, J. (2011) Decision management systems: a practical guide to using business rules
and predictive analytics. London: Pearson Education.
Waller, M.A. and Fawcett, S.E. (2013) Click here for a data scientist: Big data, predictive
analytics, and theory development in the era of a maker movement supply chain. Journal of
Business Logistics, 34(4), pp.249-252.
Waller, M.A. and Fawcett, S.E. (2013) Data science, predictive analytics, and big data: a
revolution that will transform supply chain design and management. Journal of Business
Logistics, 34(2), pp.77-84.
1 out of 8