Statistics Assignment: Regression Assumptions and Data Analysis
VerifiedAdded on 2021/06/14
|9
|2206
|79
Homework Assignment
AI Summary
This statistics assignment delves into the concepts of simple and multiple linear regression, exploring both descriptive and inferential assumptions. The assignment analyzes the relationship between variables, such as GPA and television viewing time, highlighting the interpretation of slope and int...
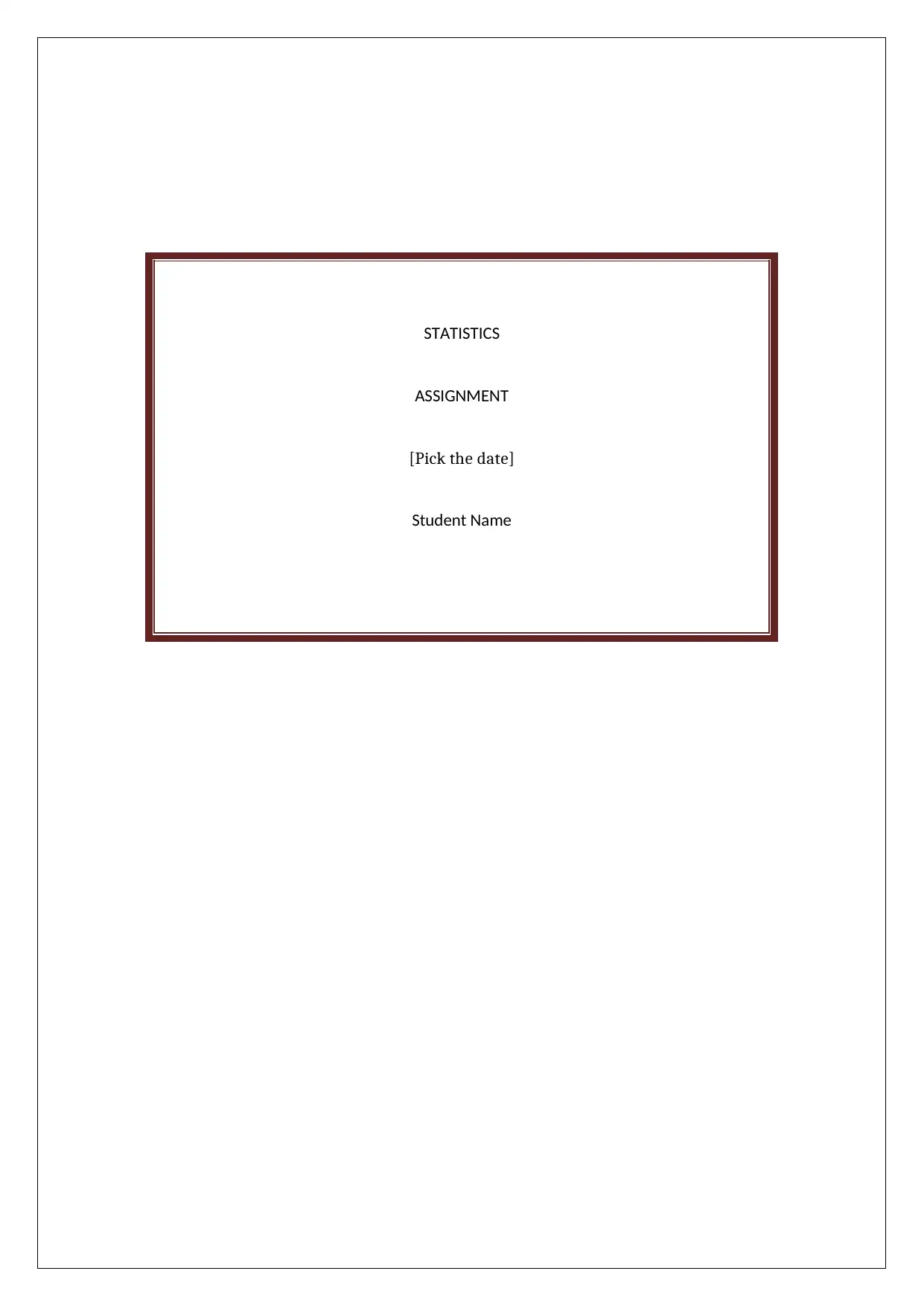
STATISTICS
ASSIGNMENT
[Pick the date]
Student Name
ASSIGNMENT
[Pick the date]
Student Name
Paraphrase This Document
Need a fresh take? Get an instant paraphrase of this document with our AI Paraphraser
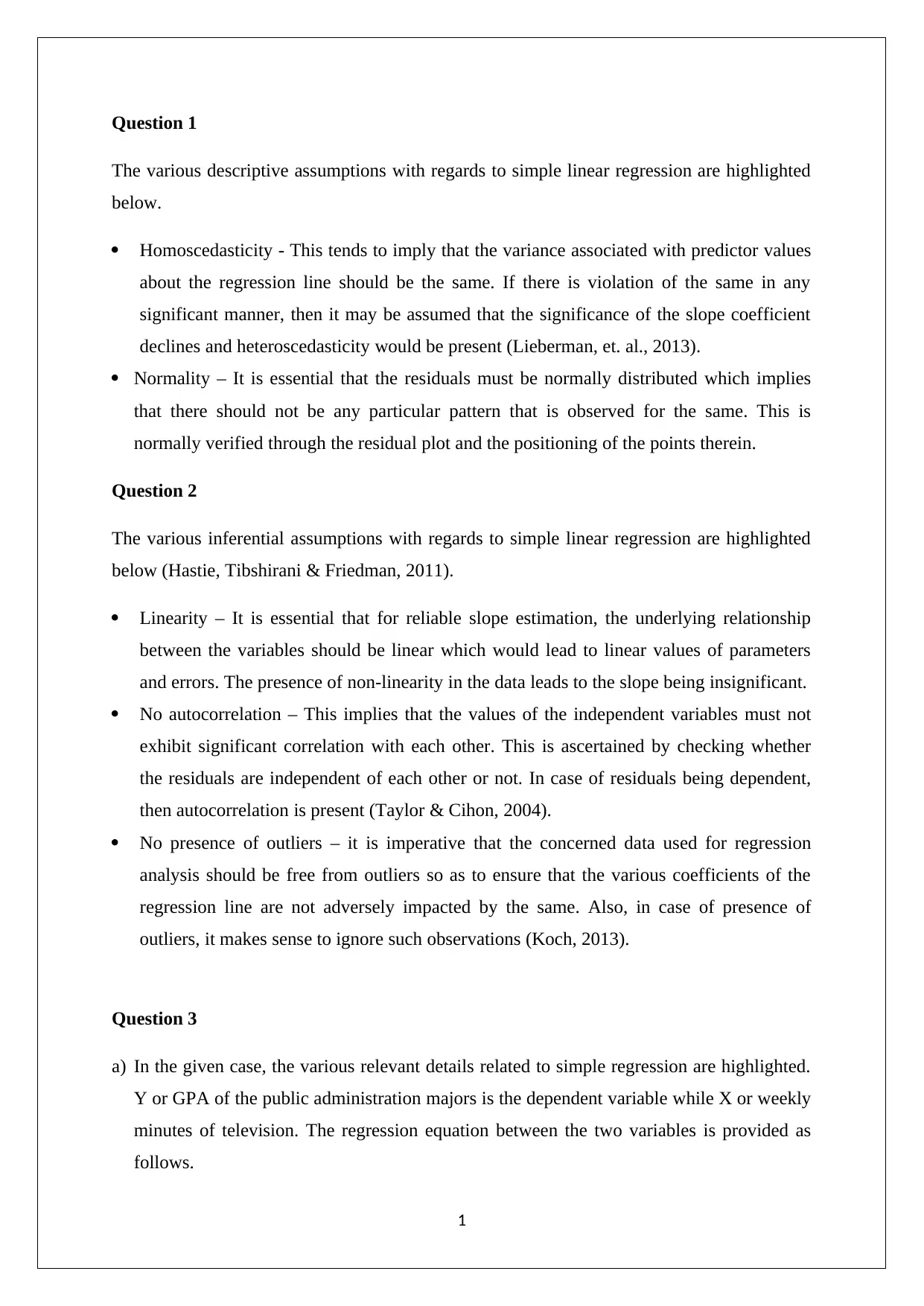
Question 1
The various descriptive assumptions with regards to simple linear regression are highlighted
below.
Homoscedasticity - This tends to imply that the variance associated with predictor values
about the regression line should be the same. If there is violation of the same in any
significant manner, then it may be assumed that the significance of the slope coefficient
declines and heteroscedasticity would be present (Lieberman, et. al., 2013).
Normality – It is essential that the residuals must be normally distributed which implies
that there should not be any particular pattern that is observed for the same. This is
normally verified through the residual plot and the positioning of the points therein.
Question 2
The various inferential assumptions with regards to simple linear regression are highlighted
below (Hastie, Tibshirani & Friedman, 2011).
Linearity – It is essential that for reliable slope estimation, the underlying relationship
between the variables should be linear which would lead to linear values of parameters
and errors. The presence of non-linearity in the data leads to the slope being insignificant.
No autocorrelation – This implies that the values of the independent variables must not
exhibit significant correlation with each other. This is ascertained by checking whether
the residuals are independent of each other or not. In case of residuals being dependent,
then autocorrelation is present (Taylor & Cihon, 2004).
No presence of outliers – it is imperative that the concerned data used for regression
analysis should be free from outliers so as to ensure that the various coefficients of the
regression line are not adversely impacted by the same. Also, in case of presence of
outliers, it makes sense to ignore such observations (Koch, 2013).
Question 3
a) In the given case, the various relevant details related to simple regression are highlighted.
Y or GPA of the public administration majors is the dependent variable while X or weekly
minutes of television. The regression equation between the two variables is provided as
follows.
1
The various descriptive assumptions with regards to simple linear regression are highlighted
below.
Homoscedasticity - This tends to imply that the variance associated with predictor values
about the regression line should be the same. If there is violation of the same in any
significant manner, then it may be assumed that the significance of the slope coefficient
declines and heteroscedasticity would be present (Lieberman, et. al., 2013).
Normality – It is essential that the residuals must be normally distributed which implies
that there should not be any particular pattern that is observed for the same. This is
normally verified through the residual plot and the positioning of the points therein.
Question 2
The various inferential assumptions with regards to simple linear regression are highlighted
below (Hastie, Tibshirani & Friedman, 2011).
Linearity – It is essential that for reliable slope estimation, the underlying relationship
between the variables should be linear which would lead to linear values of parameters
and errors. The presence of non-linearity in the data leads to the slope being insignificant.
No autocorrelation – This implies that the values of the independent variables must not
exhibit significant correlation with each other. This is ascertained by checking whether
the residuals are independent of each other or not. In case of residuals being dependent,
then autocorrelation is present (Taylor & Cihon, 2004).
No presence of outliers – it is imperative that the concerned data used for regression
analysis should be free from outliers so as to ensure that the various coefficients of the
regression line are not adversely impacted by the same. Also, in case of presence of
outliers, it makes sense to ignore such observations (Koch, 2013).
Question 3
a) In the given case, the various relevant details related to simple regression are highlighted.
Y or GPA of the public administration majors is the dependent variable while X or weekly
minutes of television. The regression equation between the two variables is provided as
follows.
1
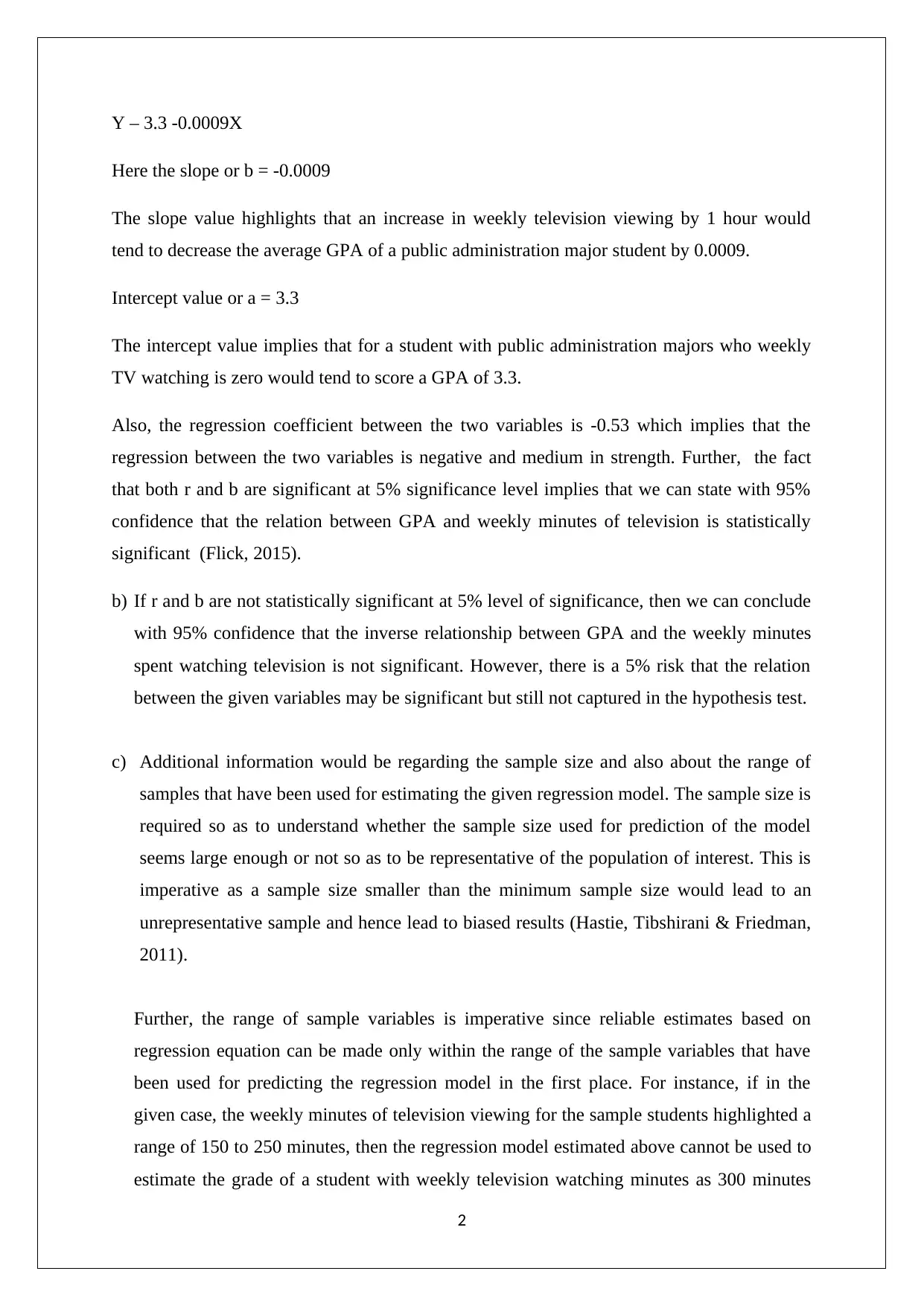
Y – 3.3 -0.0009X
Here the slope or b = -0.0009
The slope value highlights that an increase in weekly television viewing by 1 hour would
tend to decrease the average GPA of a public administration major student by 0.0009.
Intercept value or a = 3.3
The intercept value implies that for a student with public administration majors who weekly
TV watching is zero would tend to score a GPA of 3.3.
Also, the regression coefficient between the two variables is -0.53 which implies that the
regression between the two variables is negative and medium in strength. Further, the fact
that both r and b are significant at 5% significance level implies that we can state with 95%
confidence that the relation between GPA and weekly minutes of television is statistically
significant (Flick, 2015).
b) If r and b are not statistically significant at 5% level of significance, then we can conclude
with 95% confidence that the inverse relationship between GPA and the weekly minutes
spent watching television is not significant. However, there is a 5% risk that the relation
between the given variables may be significant but still not captured in the hypothesis test.
c) Additional information would be regarding the sample size and also about the range of
samples that have been used for estimating the given regression model. The sample size is
required so as to understand whether the sample size used for prediction of the model
seems large enough or not so as to be representative of the population of interest. This is
imperative as a sample size smaller than the minimum sample size would lead to an
unrepresentative sample and hence lead to biased results (Hastie, Tibshirani & Friedman,
2011).
Further, the range of sample variables is imperative since reliable estimates based on
regression equation can be made only within the range of the sample variables that have
been used for predicting the regression model in the first place. For instance, if in the
given case, the weekly minutes of television viewing for the sample students highlighted a
range of 150 to 250 minutes, then the regression model estimated above cannot be used to
estimate the grade of a student with weekly television watching minutes as 300 minutes
2
Here the slope or b = -0.0009
The slope value highlights that an increase in weekly television viewing by 1 hour would
tend to decrease the average GPA of a public administration major student by 0.0009.
Intercept value or a = 3.3
The intercept value implies that for a student with public administration majors who weekly
TV watching is zero would tend to score a GPA of 3.3.
Also, the regression coefficient between the two variables is -0.53 which implies that the
regression between the two variables is negative and medium in strength. Further, the fact
that both r and b are significant at 5% significance level implies that we can state with 95%
confidence that the relation between GPA and weekly minutes of television is statistically
significant (Flick, 2015).
b) If r and b are not statistically significant at 5% level of significance, then we can conclude
with 95% confidence that the inverse relationship between GPA and the weekly minutes
spent watching television is not significant. However, there is a 5% risk that the relation
between the given variables may be significant but still not captured in the hypothesis test.
c) Additional information would be regarding the sample size and also about the range of
samples that have been used for estimating the given regression model. The sample size is
required so as to understand whether the sample size used for prediction of the model
seems large enough or not so as to be representative of the population of interest. This is
imperative as a sample size smaller than the minimum sample size would lead to an
unrepresentative sample and hence lead to biased results (Hastie, Tibshirani & Friedman,
2011).
Further, the range of sample variables is imperative since reliable estimates based on
regression equation can be made only within the range of the sample variables that have
been used for predicting the regression model in the first place. For instance, if in the
given case, the weekly minutes of television viewing for the sample students highlighted a
range of 150 to 250 minutes, then the regression model estimated above cannot be used to
estimate the grade of a student with weekly television watching minutes as 300 minutes
2
You're viewing a preview
Unlock full access by subscribing today!
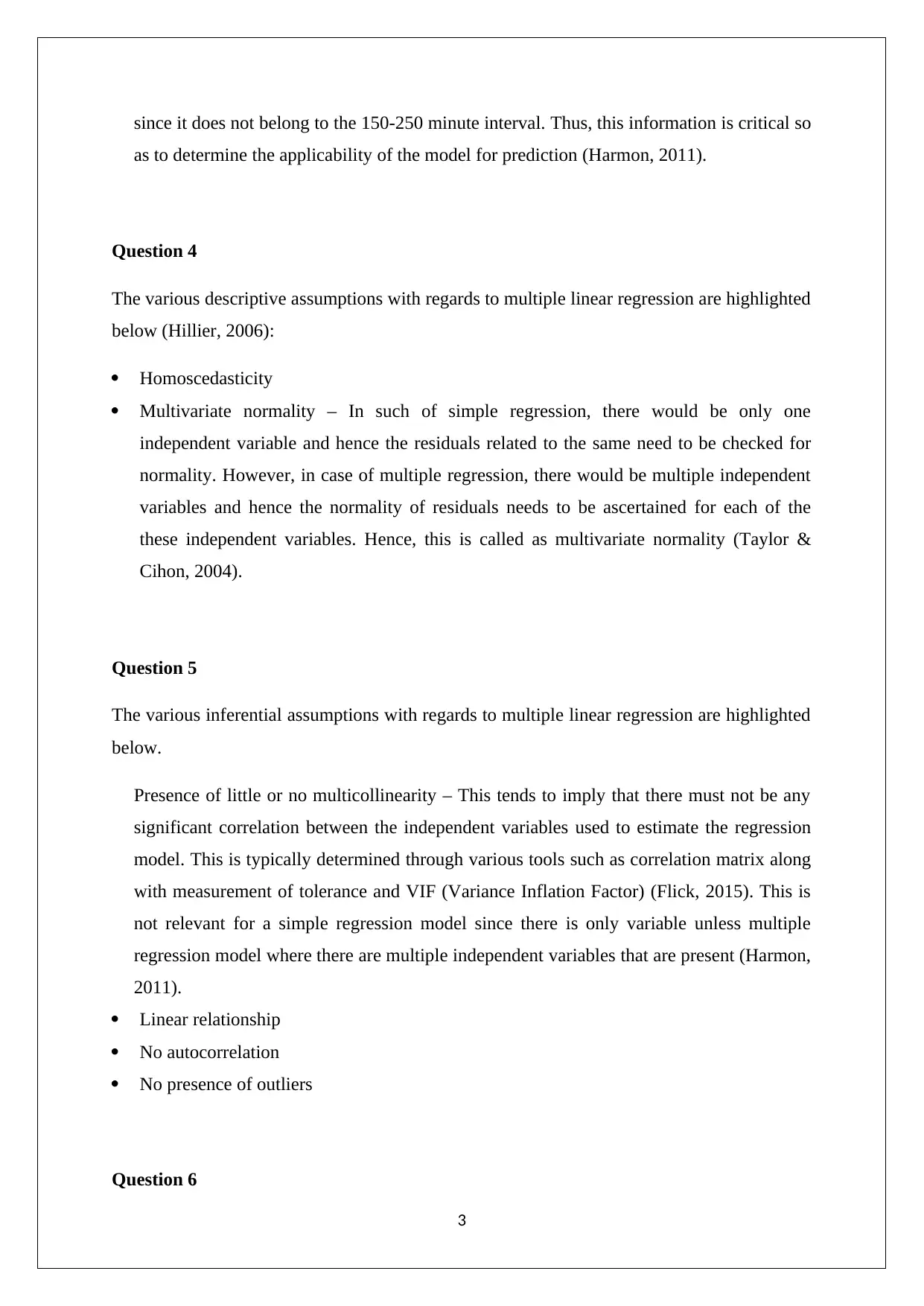
since it does not belong to the 150-250 minute interval. Thus, this information is critical so
as to determine the applicability of the model for prediction (Harmon, 2011).
Question 4
The various descriptive assumptions with regards to multiple linear regression are highlighted
below (Hillier, 2006):
Homoscedasticity
Multivariate normality – In such of simple regression, there would be only one
independent variable and hence the residuals related to the same need to be checked for
normality. However, in case of multiple regression, there would be multiple independent
variables and hence the normality of residuals needs to be ascertained for each of the
these independent variables. Hence, this is called as multivariate normality (Taylor &
Cihon, 2004).
Question 5
The various inferential assumptions with regards to multiple linear regression are highlighted
below.
Presence of little or no multicollinearity – This tends to imply that there must not be any
significant correlation between the independent variables used to estimate the regression
model. This is typically determined through various tools such as correlation matrix along
with measurement of tolerance and VIF (Variance Inflation Factor) (Flick, 2015). This is
not relevant for a simple regression model since there is only variable unless multiple
regression model where there are multiple independent variables that are present (Harmon,
2011).
Linear relationship
No autocorrelation
No presence of outliers
Question 6
3
as to determine the applicability of the model for prediction (Harmon, 2011).
Question 4
The various descriptive assumptions with regards to multiple linear regression are highlighted
below (Hillier, 2006):
Homoscedasticity
Multivariate normality – In such of simple regression, there would be only one
independent variable and hence the residuals related to the same need to be checked for
normality. However, in case of multiple regression, there would be multiple independent
variables and hence the normality of residuals needs to be ascertained for each of the
these independent variables. Hence, this is called as multivariate normality (Taylor &
Cihon, 2004).
Question 5
The various inferential assumptions with regards to multiple linear regression are highlighted
below.
Presence of little or no multicollinearity – This tends to imply that there must not be any
significant correlation between the independent variables used to estimate the regression
model. This is typically determined through various tools such as correlation matrix along
with measurement of tolerance and VIF (Variance Inflation Factor) (Flick, 2015). This is
not relevant for a simple regression model since there is only variable unless multiple
regression model where there are multiple independent variables that are present (Harmon,
2011).
Linear relationship
No autocorrelation
No presence of outliers
Question 6
3
Paraphrase This Document
Need a fresh take? Get an instant paraphrase of this document with our AI Paraphraser
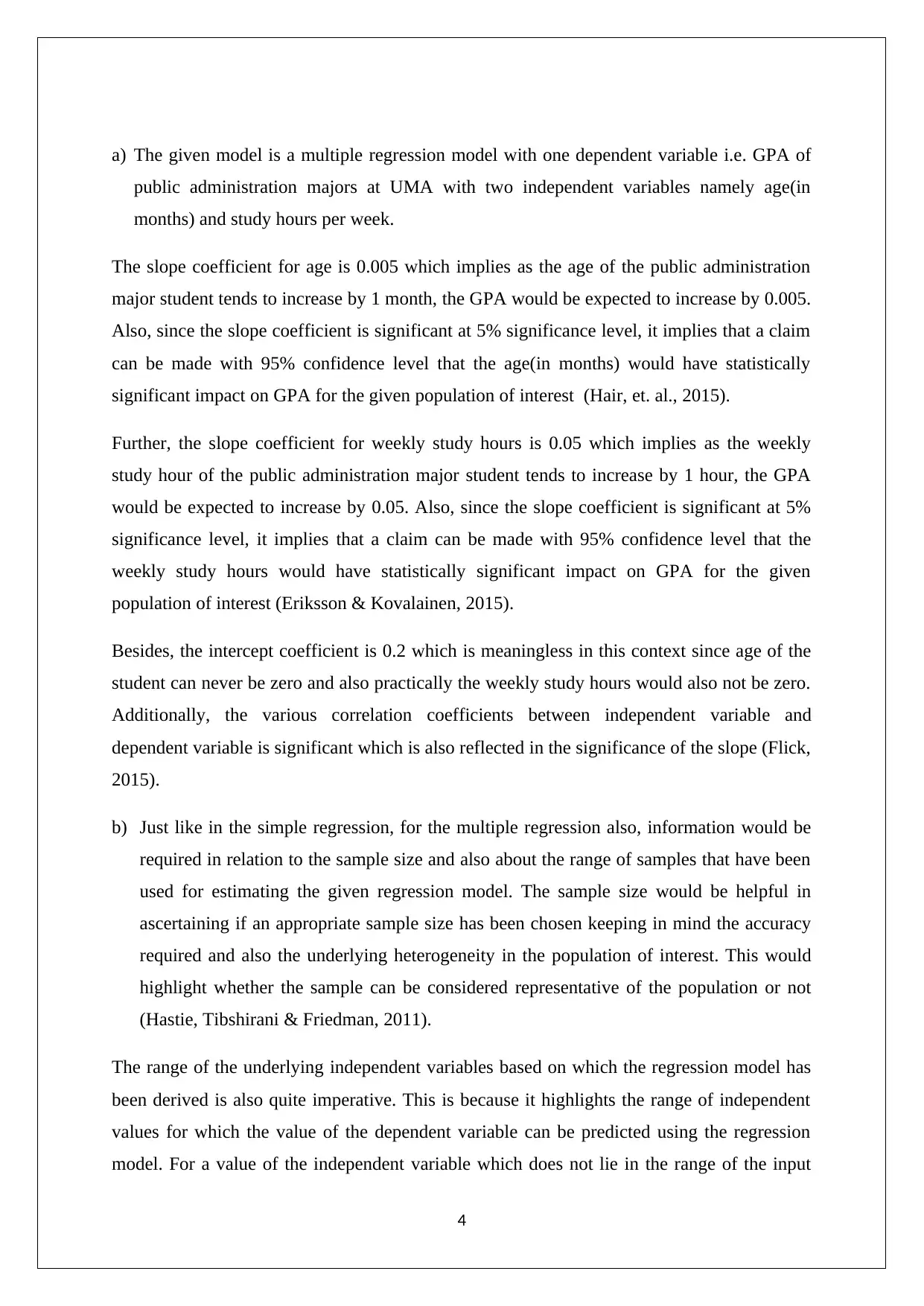
a) The given model is a multiple regression model with one dependent variable i.e. GPA of
public administration majors at UMA with two independent variables namely age(in
months) and study hours per week.
The slope coefficient for age is 0.005 which implies as the age of the public administration
major student tends to increase by 1 month, the GPA would be expected to increase by 0.005.
Also, since the slope coefficient is significant at 5% significance level, it implies that a claim
can be made with 95% confidence level that the age(in months) would have statistically
significant impact on GPA for the given population of interest (Hair, et. al., 2015).
Further, the slope coefficient for weekly study hours is 0.05 which implies as the weekly
study hour of the public administration major student tends to increase by 1 hour, the GPA
would be expected to increase by 0.05. Also, since the slope coefficient is significant at 5%
significance level, it implies that a claim can be made with 95% confidence level that the
weekly study hours would have statistically significant impact on GPA for the given
population of interest (Eriksson & Kovalainen, 2015).
Besides, the intercept coefficient is 0.2 which is meaningless in this context since age of the
student can never be zero and also practically the weekly study hours would also not be zero.
Additionally, the various correlation coefficients between independent variable and
dependent variable is significant which is also reflected in the significance of the slope (Flick,
2015).
b) Just like in the simple regression, for the multiple regression also, information would be
required in relation to the sample size and also about the range of samples that have been
used for estimating the given regression model. The sample size would be helpful in
ascertaining if an appropriate sample size has been chosen keeping in mind the accuracy
required and also the underlying heterogeneity in the population of interest. This would
highlight whether the sample can be considered representative of the population or not
(Hastie, Tibshirani & Friedman, 2011).
The range of the underlying independent variables based on which the regression model has
been derived is also quite imperative. This is because it highlights the range of independent
values for which the value of the dependent variable can be predicted using the regression
model. For a value of the independent variable which does not lie in the range of the input
4
public administration majors at UMA with two independent variables namely age(in
months) and study hours per week.
The slope coefficient for age is 0.005 which implies as the age of the public administration
major student tends to increase by 1 month, the GPA would be expected to increase by 0.005.
Also, since the slope coefficient is significant at 5% significance level, it implies that a claim
can be made with 95% confidence level that the age(in months) would have statistically
significant impact on GPA for the given population of interest (Hair, et. al., 2015).
Further, the slope coefficient for weekly study hours is 0.05 which implies as the weekly
study hour of the public administration major student tends to increase by 1 hour, the GPA
would be expected to increase by 0.05. Also, since the slope coefficient is significant at 5%
significance level, it implies that a claim can be made with 95% confidence level that the
weekly study hours would have statistically significant impact on GPA for the given
population of interest (Eriksson & Kovalainen, 2015).
Besides, the intercept coefficient is 0.2 which is meaningless in this context since age of the
student can never be zero and also practically the weekly study hours would also not be zero.
Additionally, the various correlation coefficients between independent variable and
dependent variable is significant which is also reflected in the significance of the slope (Flick,
2015).
b) Just like in the simple regression, for the multiple regression also, information would be
required in relation to the sample size and also about the range of samples that have been
used for estimating the given regression model. The sample size would be helpful in
ascertaining if an appropriate sample size has been chosen keeping in mind the accuracy
required and also the underlying heterogeneity in the population of interest. This would
highlight whether the sample can be considered representative of the population or not
(Hastie, Tibshirani & Friedman, 2011).
The range of the underlying independent variables based on which the regression model has
been derived is also quite imperative. This is because it highlights the range of independent
values for which the value of the dependent variable can be predicted using the regression
model. For a value of the independent variable which does not lie in the range of the input
4
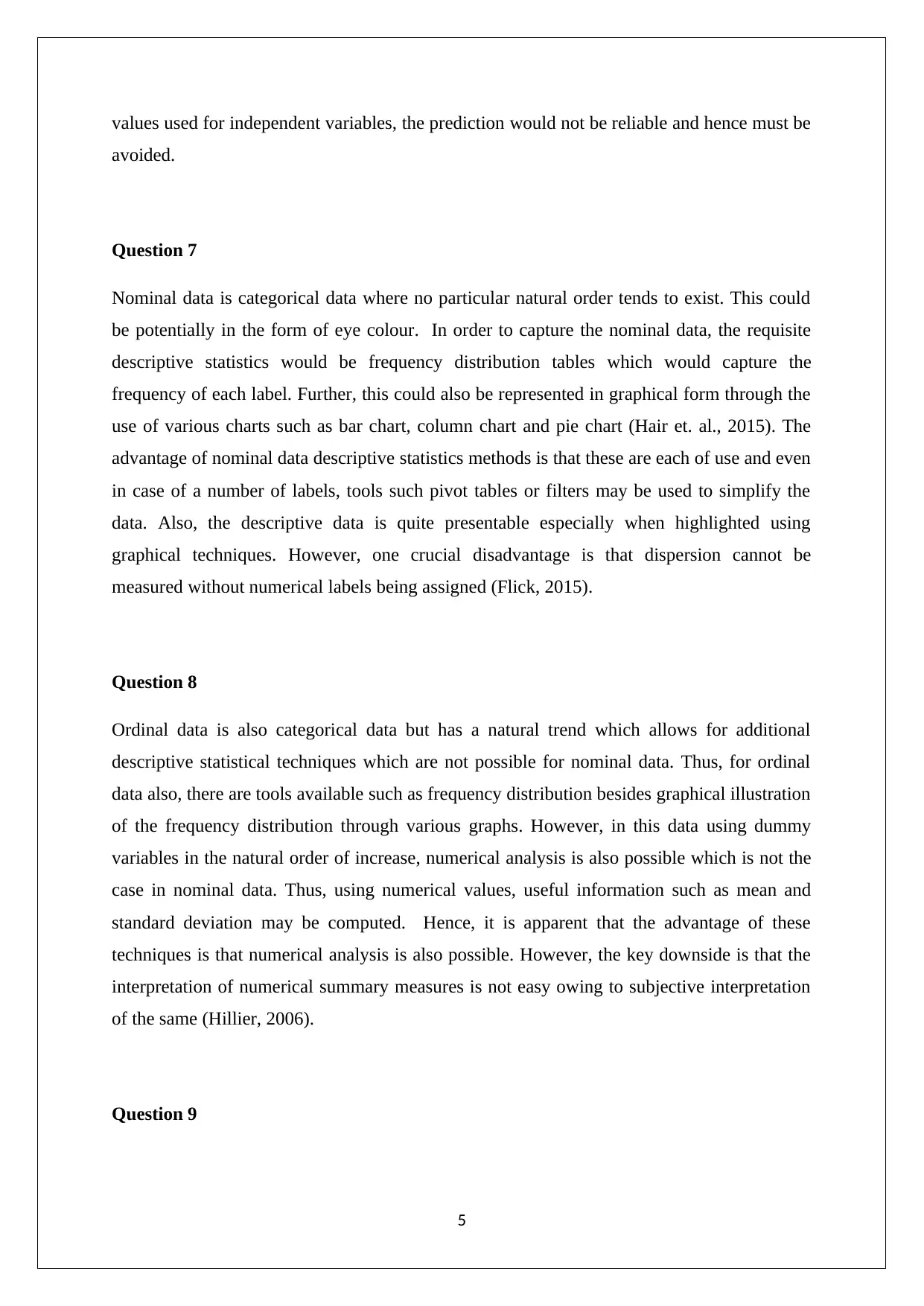
values used for independent variables, the prediction would not be reliable and hence must be
avoided.
Question 7
Nominal data is categorical data where no particular natural order tends to exist. This could
be potentially in the form of eye colour. In order to capture the nominal data, the requisite
descriptive statistics would be frequency distribution tables which would capture the
frequency of each label. Further, this could also be represented in graphical form through the
use of various charts such as bar chart, column chart and pie chart (Hair et. al., 2015). The
advantage of nominal data descriptive statistics methods is that these are each of use and even
in case of a number of labels, tools such pivot tables or filters may be used to simplify the
data. Also, the descriptive data is quite presentable especially when highlighted using
graphical techniques. However, one crucial disadvantage is that dispersion cannot be
measured without numerical labels being assigned (Flick, 2015).
Question 8
Ordinal data is also categorical data but has a natural trend which allows for additional
descriptive statistical techniques which are not possible for nominal data. Thus, for ordinal
data also, there are tools available such as frequency distribution besides graphical illustration
of the frequency distribution through various graphs. However, in this data using dummy
variables in the natural order of increase, numerical analysis is also possible which is not the
case in nominal data. Thus, using numerical values, useful information such as mean and
standard deviation may be computed. Hence, it is apparent that the advantage of these
techniques is that numerical analysis is also possible. However, the key downside is that the
interpretation of numerical summary measures is not easy owing to subjective interpretation
of the same (Hillier, 2006).
Question 9
5
avoided.
Question 7
Nominal data is categorical data where no particular natural order tends to exist. This could
be potentially in the form of eye colour. In order to capture the nominal data, the requisite
descriptive statistics would be frequency distribution tables which would capture the
frequency of each label. Further, this could also be represented in graphical form through the
use of various charts such as bar chart, column chart and pie chart (Hair et. al., 2015). The
advantage of nominal data descriptive statistics methods is that these are each of use and even
in case of a number of labels, tools such pivot tables or filters may be used to simplify the
data. Also, the descriptive data is quite presentable especially when highlighted using
graphical techniques. However, one crucial disadvantage is that dispersion cannot be
measured without numerical labels being assigned (Flick, 2015).
Question 8
Ordinal data is also categorical data but has a natural trend which allows for additional
descriptive statistical techniques which are not possible for nominal data. Thus, for ordinal
data also, there are tools available such as frequency distribution besides graphical illustration
of the frequency distribution through various graphs. However, in this data using dummy
variables in the natural order of increase, numerical analysis is also possible which is not the
case in nominal data. Thus, using numerical values, useful information such as mean and
standard deviation may be computed. Hence, it is apparent that the advantage of these
techniques is that numerical analysis is also possible. However, the key downside is that the
interpretation of numerical summary measures is not easy owing to subjective interpretation
of the same (Hillier, 2006).
Question 9
5
You're viewing a preview
Unlock full access by subscribing today!
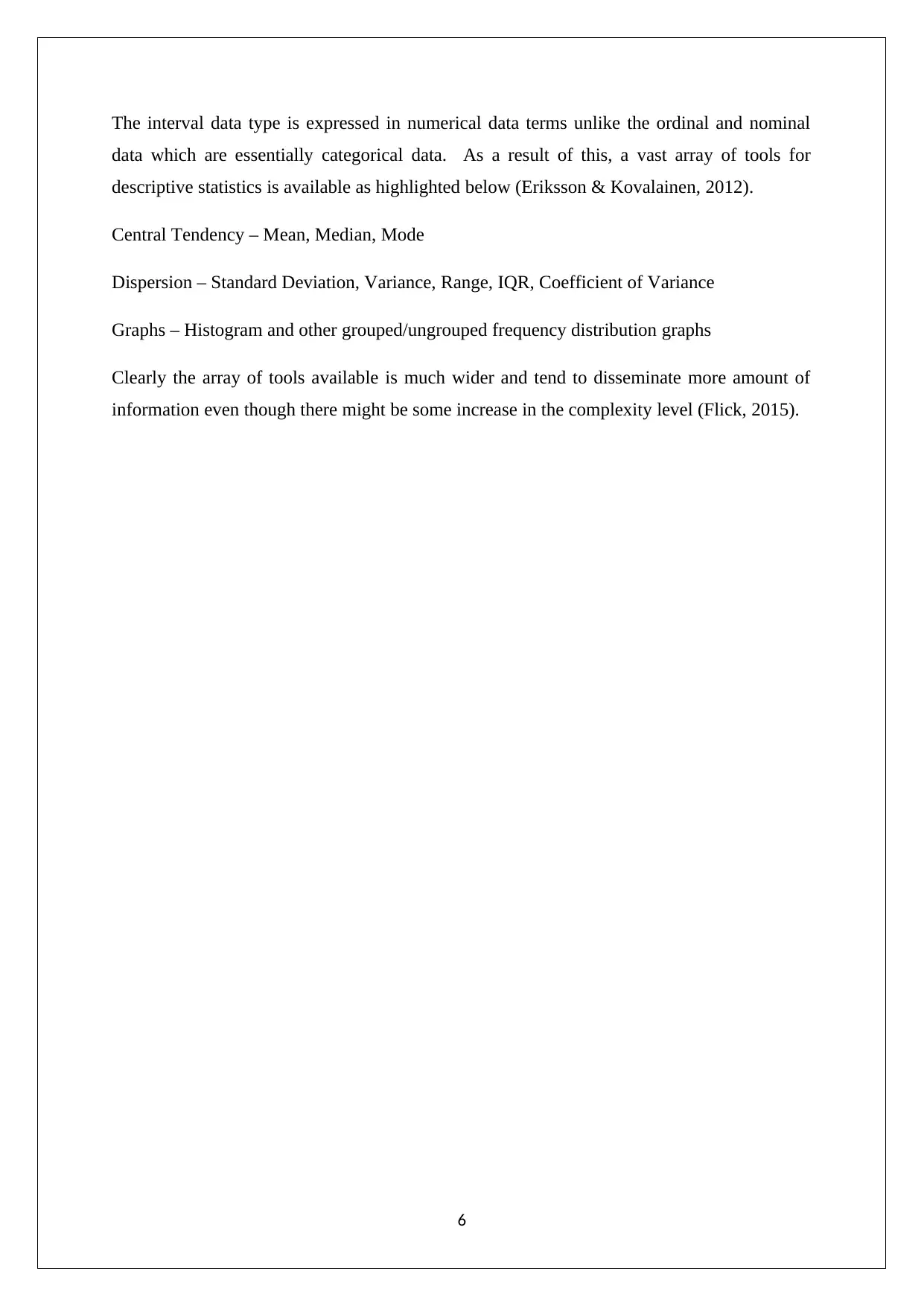
The interval data type is expressed in numerical data terms unlike the ordinal and nominal
data which are essentially categorical data. As a result of this, a vast array of tools for
descriptive statistics is available as highlighted below (Eriksson & Kovalainen, 2012).
Central Tendency – Mean, Median, Mode
Dispersion – Standard Deviation, Variance, Range, IQR, Coefficient of Variance
Graphs – Histogram and other grouped/ungrouped frequency distribution graphs
Clearly the array of tools available is much wider and tend to disseminate more amount of
information even though there might be some increase in the complexity level (Flick, 2015).
6
data which are essentially categorical data. As a result of this, a vast array of tools for
descriptive statistics is available as highlighted below (Eriksson & Kovalainen, 2012).
Central Tendency – Mean, Median, Mode
Dispersion – Standard Deviation, Variance, Range, IQR, Coefficient of Variance
Graphs – Histogram and other grouped/ungrouped frequency distribution graphs
Clearly the array of tools available is much wider and tend to disseminate more amount of
information even though there might be some increase in the complexity level (Flick, 2015).
6
Paraphrase This Document
Need a fresh take? Get an instant paraphrase of this document with our AI Paraphraser
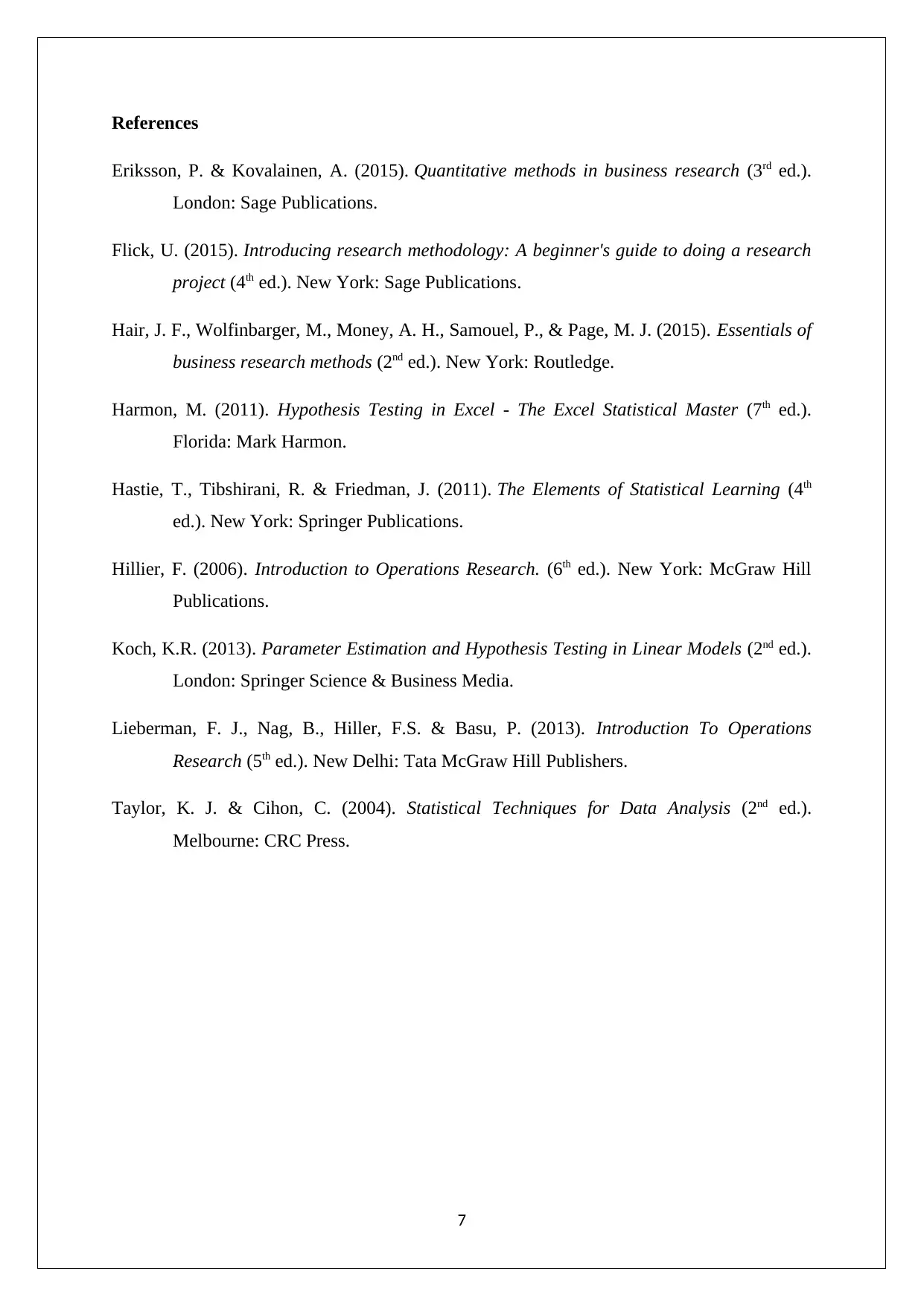
References
Eriksson, P. & Kovalainen, A. (2015). Quantitative methods in business research (3rd ed.).
London: Sage Publications.
Flick, U. (2015). Introducing research methodology: A beginner's guide to doing a research
project (4th ed.). New York: Sage Publications.
Hair, J. F., Wolfinbarger, M., Money, A. H., Samouel, P., & Page, M. J. (2015). Essentials of
business research methods (2nd ed.). New York: Routledge.
Harmon, M. (2011). Hypothesis Testing in Excel - The Excel Statistical Master (7th ed.).
Florida: Mark Harmon.
Hastie, T., Tibshirani, R. & Friedman, J. (2011). The Elements of Statistical Learning (4th
ed.). New York: Springer Publications.
Hillier, F. (2006). Introduction to Operations Research. (6th ed.). New York: McGraw Hill
Publications.
Koch, K.R. (2013). Parameter Estimation and Hypothesis Testing in Linear Models (2nd ed.).
London: Springer Science & Business Media.
Lieberman, F. J., Nag, B., Hiller, F.S. & Basu, P. (2013). Introduction To Operations
Research (5th ed.). New Delhi: Tata McGraw Hill Publishers.
Taylor, K. J. & Cihon, C. (2004). Statistical Techniques for Data Analysis (2nd ed.).
Melbourne: CRC Press.
7
Eriksson, P. & Kovalainen, A. (2015). Quantitative methods in business research (3rd ed.).
London: Sage Publications.
Flick, U. (2015). Introducing research methodology: A beginner's guide to doing a research
project (4th ed.). New York: Sage Publications.
Hair, J. F., Wolfinbarger, M., Money, A. H., Samouel, P., & Page, M. J. (2015). Essentials of
business research methods (2nd ed.). New York: Routledge.
Harmon, M. (2011). Hypothesis Testing in Excel - The Excel Statistical Master (7th ed.).
Florida: Mark Harmon.
Hastie, T., Tibshirani, R. & Friedman, J. (2011). The Elements of Statistical Learning (4th
ed.). New York: Springer Publications.
Hillier, F. (2006). Introduction to Operations Research. (6th ed.). New York: McGraw Hill
Publications.
Koch, K.R. (2013). Parameter Estimation and Hypothesis Testing in Linear Models (2nd ed.).
London: Springer Science & Business Media.
Lieberman, F. J., Nag, B., Hiller, F.S. & Basu, P. (2013). Introduction To Operations
Research (5th ed.). New Delhi: Tata McGraw Hill Publishers.
Taylor, K. J. & Cihon, C. (2004). Statistical Techniques for Data Analysis (2nd ed.).
Melbourne: CRC Press.
7
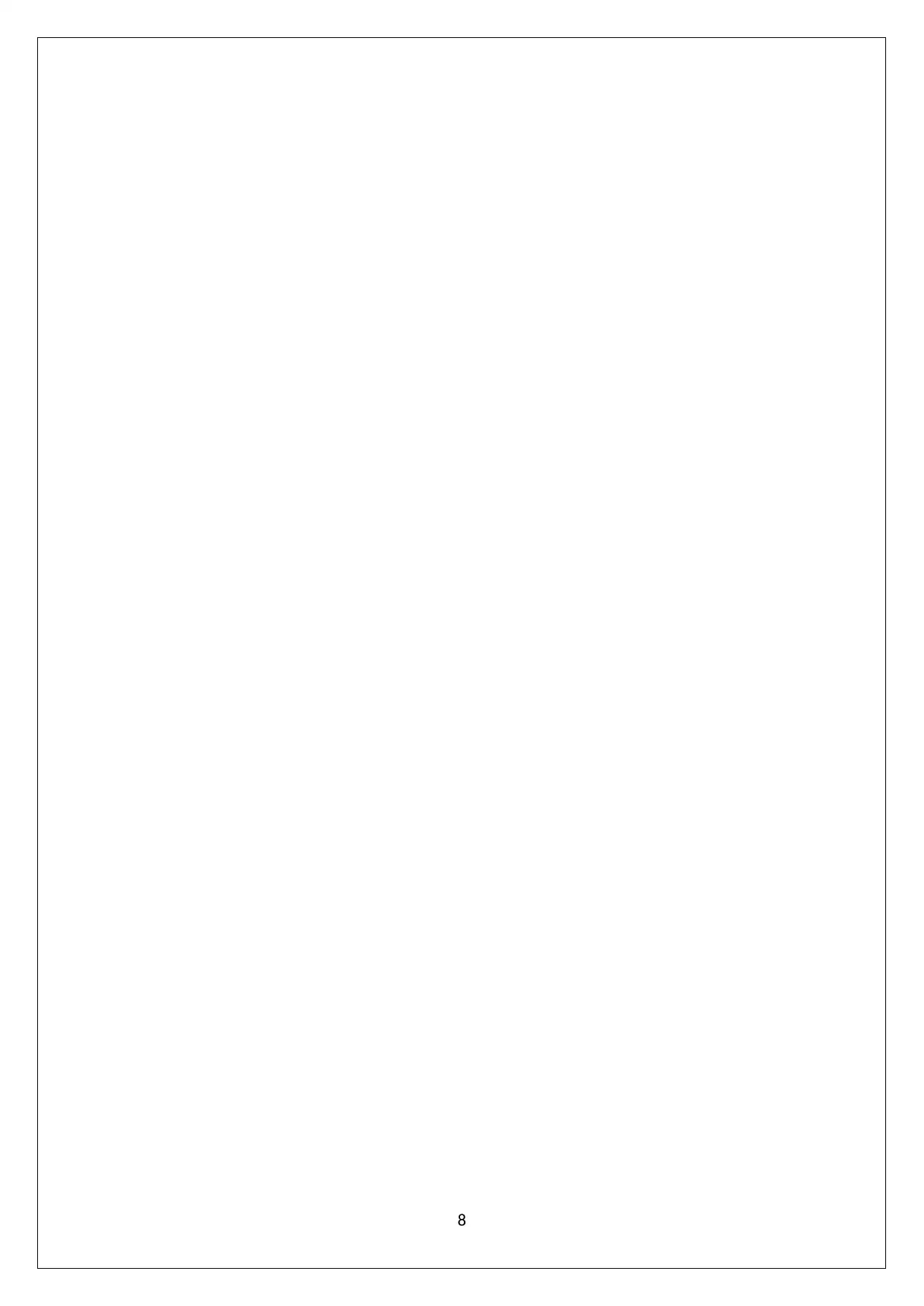
8
You're viewing a preview
Unlock full access by subscribing today!
1 out of 9
Related Documents

Your All-in-One AI-Powered Toolkit for Academic Success.
+13062052269
info@desklib.com
Available 24*7 on WhatsApp / Email
Unlock your academic potential
© 2024 | Zucol Services PVT LTD | All rights reserved.