SIT772 Database/IR: Inverted Indexing and Search Engine Queries
VerifiedAdded on 2023/06/15
|14
|1448
|66
Report
AI Summary
This report delves into the design and implementation of a search algorithm using both the Vector and Boolean models. It begins with the creation of an inverted index from three documents covering search engines, cloud computing, and security/privacy, detailing the steps of stop word eliminatio...
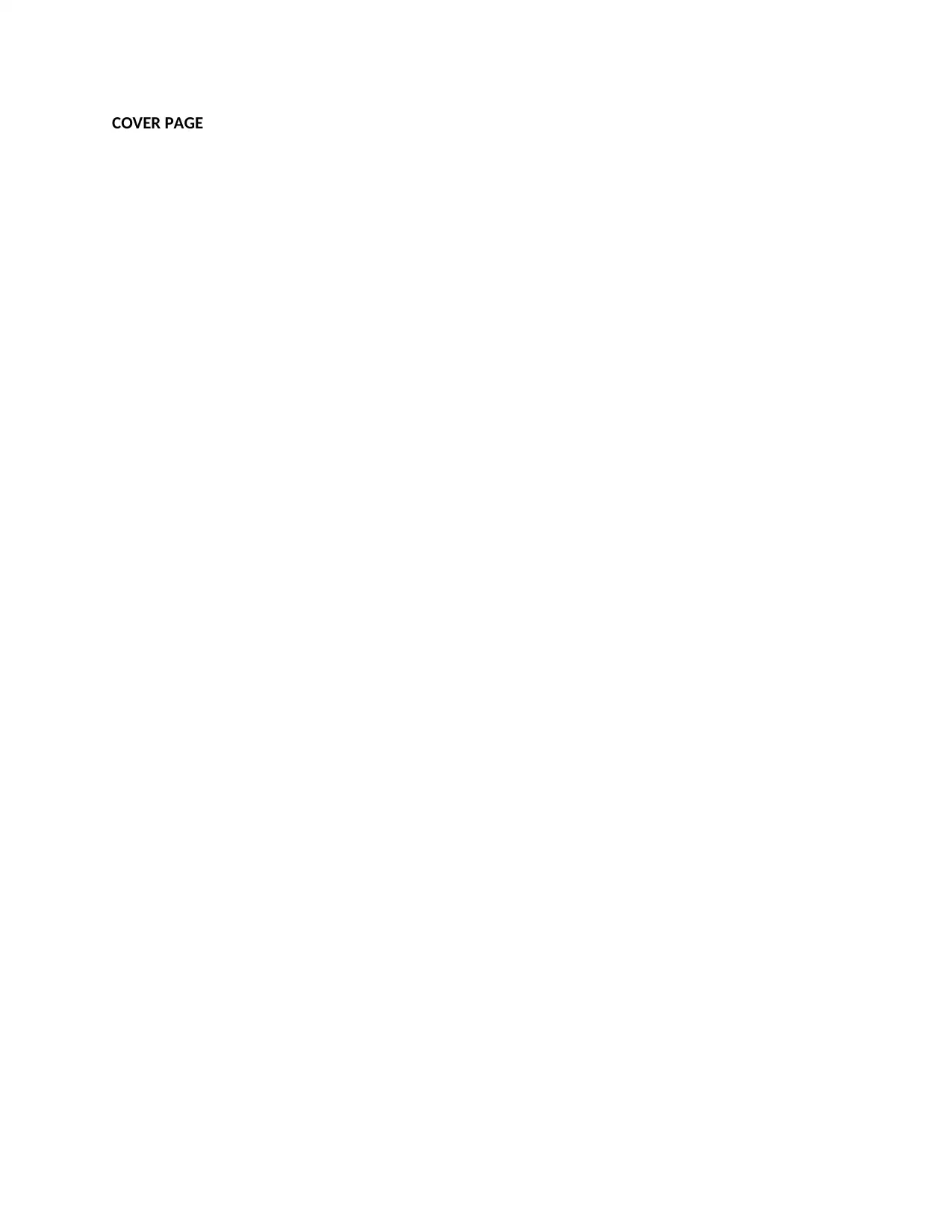
COVER PAGE
Paraphrase This Document
Need a fresh take? Get an instant paraphrase of this document with our AI Paraphraser
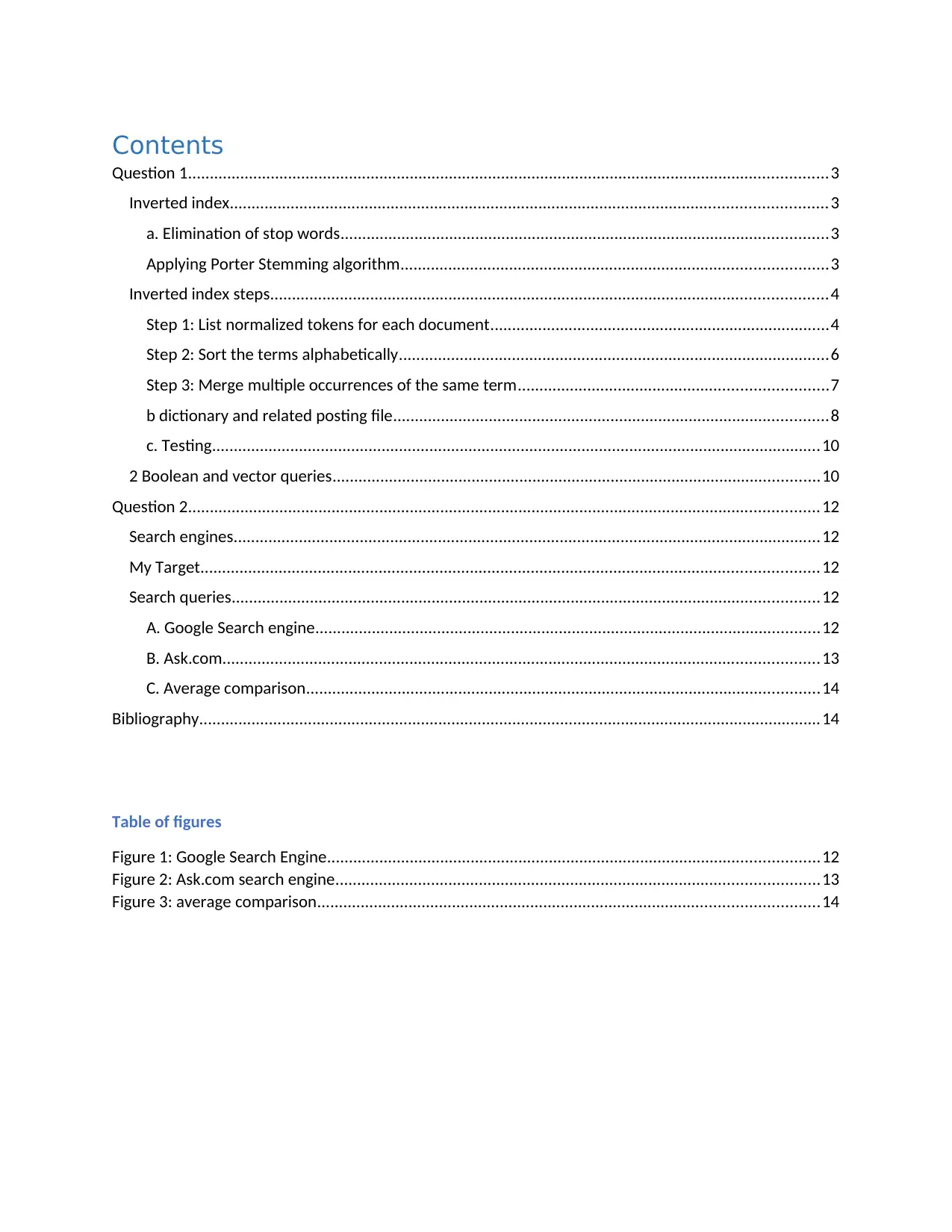
Contents
Question 1...................................................................................................................................................3
Inverted index.........................................................................................................................................3
a. Elimination of stop words................................................................................................................3
Applying Porter Stemming algorithm..................................................................................................3
Inverted index steps................................................................................................................................4
Step 1: List normalized tokens for each document..............................................................................4
Step 2: Sort the terms alphabetically...................................................................................................6
Step 3: Merge multiple occurrences of the same term.......................................................................7
b dictionary and related posting file....................................................................................................8
c. Testing............................................................................................................................................10
2 Boolean and vector queries................................................................................................................10
Question 2.................................................................................................................................................12
Search engines.......................................................................................................................................12
My Target..............................................................................................................................................12
Search queries.......................................................................................................................................12
A. Google Search engine....................................................................................................................12
B. Ask.com.........................................................................................................................................13
C. Average comparison......................................................................................................................14
Bibliography...............................................................................................................................................14
Table of figures
Figure 1: Google Search Engine.................................................................................................................12
Figure 2: Ask.com search engine...............................................................................................................13
Figure 3: average comparison...................................................................................................................14
Question 1...................................................................................................................................................3
Inverted index.........................................................................................................................................3
a. Elimination of stop words................................................................................................................3
Applying Porter Stemming algorithm..................................................................................................3
Inverted index steps................................................................................................................................4
Step 1: List normalized tokens for each document..............................................................................4
Step 2: Sort the terms alphabetically...................................................................................................6
Step 3: Merge multiple occurrences of the same term.......................................................................7
b dictionary and related posting file....................................................................................................8
c. Testing............................................................................................................................................10
2 Boolean and vector queries................................................................................................................10
Question 2.................................................................................................................................................12
Search engines.......................................................................................................................................12
My Target..............................................................................................................................................12
Search queries.......................................................................................................................................12
A. Google Search engine....................................................................................................................12
B. Ask.com.........................................................................................................................................13
C. Average comparison......................................................................................................................14
Bibliography...............................................................................................................................................14
Table of figures
Figure 1: Google Search Engine.................................................................................................................12
Figure 2: Ask.com search engine...............................................................................................................13
Figure 3: average comparison...................................................................................................................14
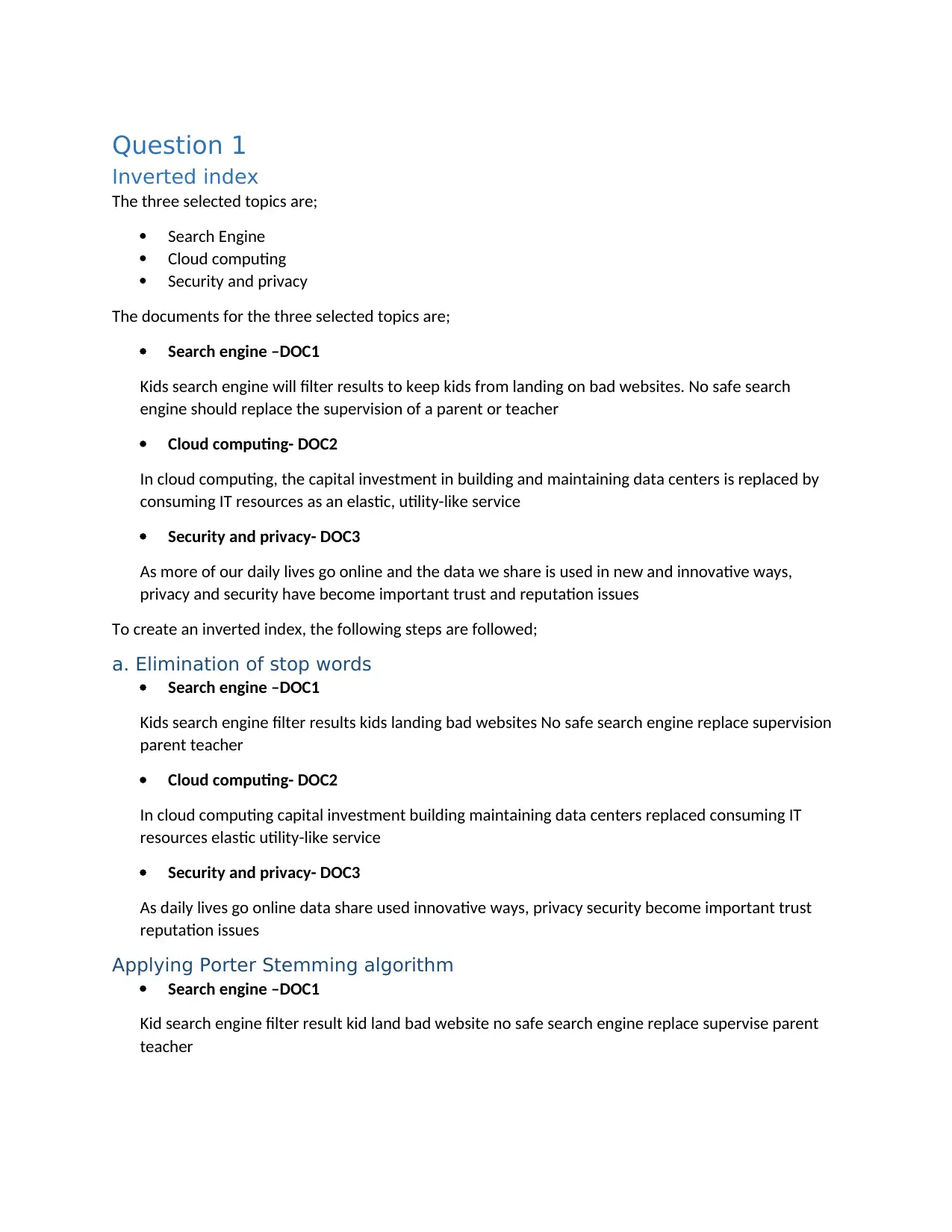
Question 1
Inverted index
The three selected topics are;
Search Engine
Cloud computing
Security and privacy
The documents for the three selected topics are;
Search engine –DOC1
Kids search engine will filter results to keep kids from landing on bad websites. No safe search
engine should replace the supervision of a parent or teacher
Cloud computing- DOC2
In cloud computing, the capital investment in building and maintaining data centers is replaced by
consuming IT resources as an elastic, utility-like service
Security and privacy- DOC3
As more of our daily lives go online and the data we share is used in new and innovative ways,
privacy and security have become important trust and reputation issues
To create an inverted index, the following steps are followed;
a. Elimination of stop words
Search engine –DOC1
Kids search engine filter results kids landing bad websites No safe search engine replace supervision
parent teacher
Cloud computing- DOC2
In cloud computing capital investment building maintaining data centers replaced consuming IT
resources elastic utility-like service
Security and privacy- DOC3
As daily lives go online data share used innovative ways, privacy security become important trust
reputation issues
Applying Porter Stemming algorithm
Search engine –DOC1
Kid search engine filter result kid land bad website no safe search engine replace supervise parent
teacher
Inverted index
The three selected topics are;
Search Engine
Cloud computing
Security and privacy
The documents for the three selected topics are;
Search engine –DOC1
Kids search engine will filter results to keep kids from landing on bad websites. No safe search
engine should replace the supervision of a parent or teacher
Cloud computing- DOC2
In cloud computing, the capital investment in building and maintaining data centers is replaced by
consuming IT resources as an elastic, utility-like service
Security and privacy- DOC3
As more of our daily lives go online and the data we share is used in new and innovative ways,
privacy and security have become important trust and reputation issues
To create an inverted index, the following steps are followed;
a. Elimination of stop words
Search engine –DOC1
Kids search engine filter results kids landing bad websites No safe search engine replace supervision
parent teacher
Cloud computing- DOC2
In cloud computing capital investment building maintaining data centers replaced consuming IT
resources elastic utility-like service
Security and privacy- DOC3
As daily lives go online data share used innovative ways, privacy security become important trust
reputation issues
Applying Porter Stemming algorithm
Search engine –DOC1
Kid search engine filter result kid land bad website no safe search engine replace supervise parent
teacher
⊘ This is a preview!⊘
Do you want full access?
Subscribe today to unlock all pages.

Trusted by 1+ million students worldwide
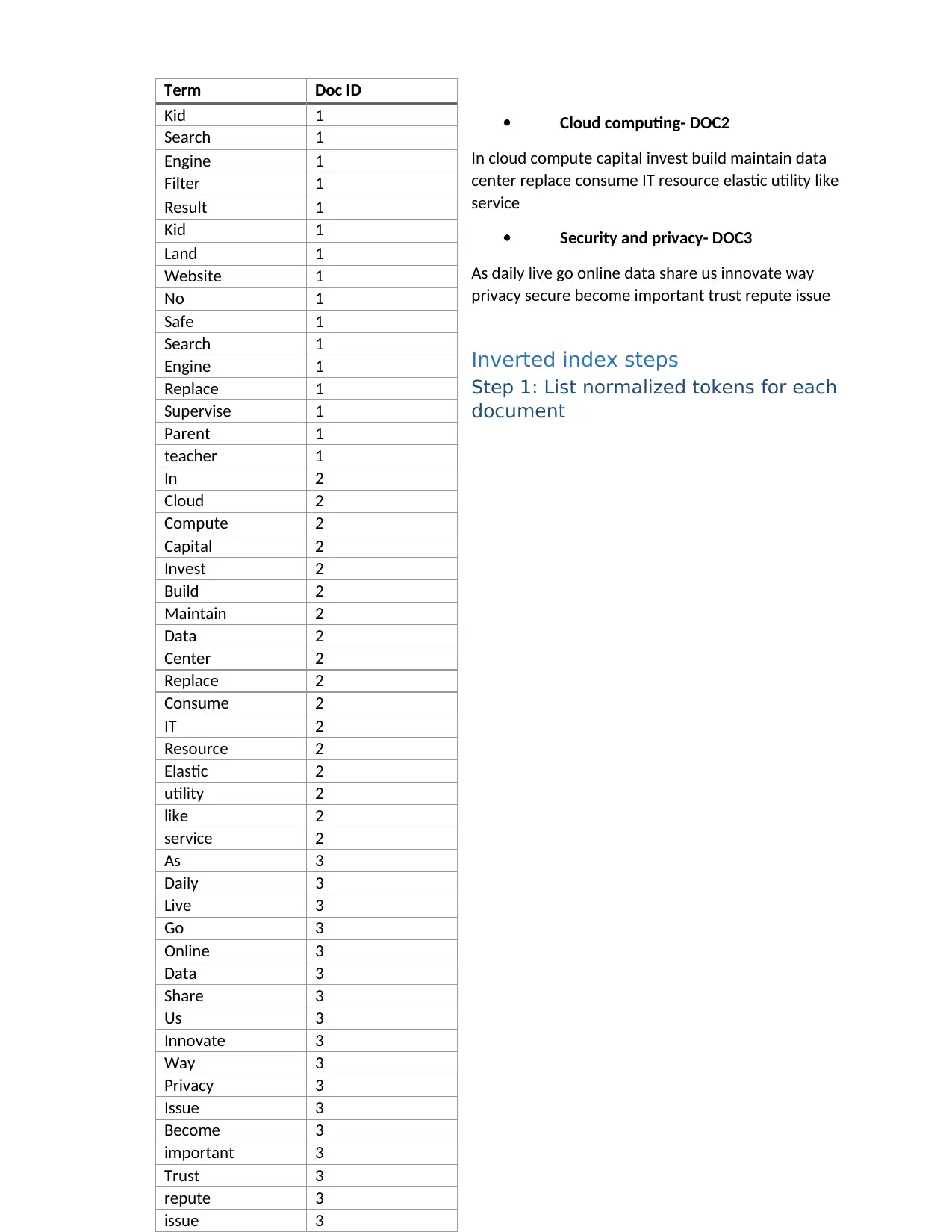
Cloud computing- DOC2
In cloud compute capital invest build maintain data
center replace consume IT resource elastic utility like
service
Security and privacy- DOC3
As daily live go online data share us innovate way
privacy secure become important trust repute issue
Inverted index steps
Step 1: List normalized tokens for each
document
Term Doc ID
Kid 1
Search 1
Engine 1
Filter 1
Result 1
Kid 1
Land 1
Website 1
No 1
Safe 1
Search 1
Engine 1
Replace 1
Supervise 1
Parent 1
teacher 1
In 2
Cloud 2
Compute 2
Capital 2
Invest 2
Build 2
Maintain 2
Data 2
Center 2
Replace 2
Consume 2
IT 2
Resource 2
Elastic 2
utility 2
like 2
service 2
As 3
Daily 3
Live 3
Go 3
Online 3
Data 3
Share 3
Us 3
Innovate 3
Way 3
Privacy 3
Issue 3
Become 3
important 3
Trust 3
repute 3
issue 3
In cloud compute capital invest build maintain data
center replace consume IT resource elastic utility like
service
Security and privacy- DOC3
As daily live go online data share us innovate way
privacy secure become important trust repute issue
Inverted index steps
Step 1: List normalized tokens for each
document
Term Doc ID
Kid 1
Search 1
Engine 1
Filter 1
Result 1
Kid 1
Land 1
Website 1
No 1
Safe 1
Search 1
Engine 1
Replace 1
Supervise 1
Parent 1
teacher 1
In 2
Cloud 2
Compute 2
Capital 2
Invest 2
Build 2
Maintain 2
Data 2
Center 2
Replace 2
Consume 2
IT 2
Resource 2
Elastic 2
utility 2
like 2
service 2
As 3
Daily 3
Live 3
Go 3
Online 3
Data 3
Share 3
Us 3
Innovate 3
Way 3
Privacy 3
Issue 3
Become 3
important 3
Trust 3
repute 3
issue 3
Paraphrase This Document
Need a fresh take? Get an instant paraphrase of this document with our AI Paraphraser
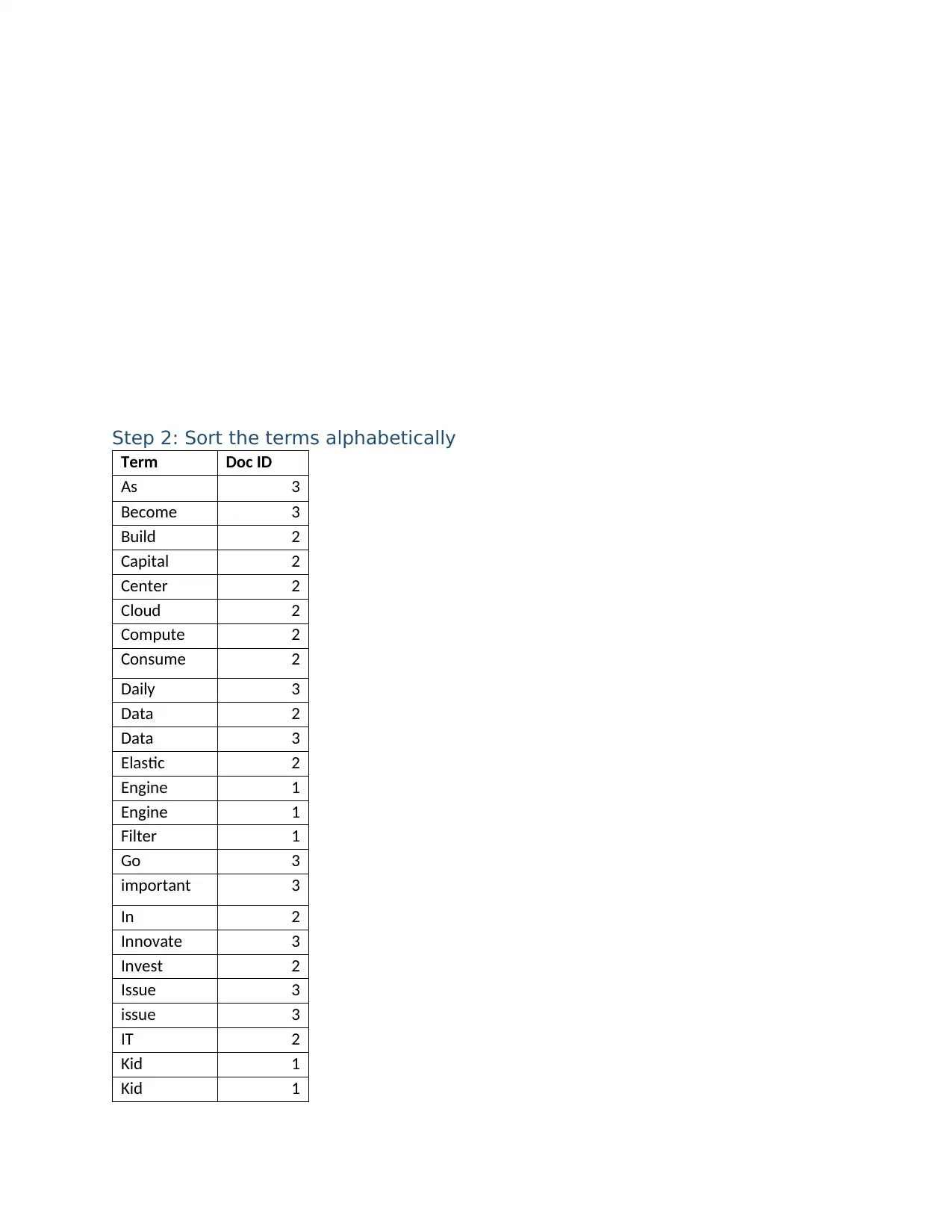
Step 2: Sort the terms alphabetically
Term Doc ID
As 3
Become 3
Build 2
Capital 2
Center 2
Cloud 2
Compute 2
Consume 2
Daily 3
Data 2
Data 3
Elastic 2
Engine 1
Engine 1
Filter 1
Go 3
important 3
In 2
Innovate 3
Invest 2
Issue 3
issue 3
IT 2
Kid 1
Kid 1
Term Doc ID
As 3
Become 3
Build 2
Capital 2
Center 2
Cloud 2
Compute 2
Consume 2
Daily 3
Data 2
Data 3
Elastic 2
Engine 1
Engine 1
Filter 1
Go 3
important 3
In 2
Innovate 3
Invest 2
Issue 3
issue 3
IT 2
Kid 1
Kid 1
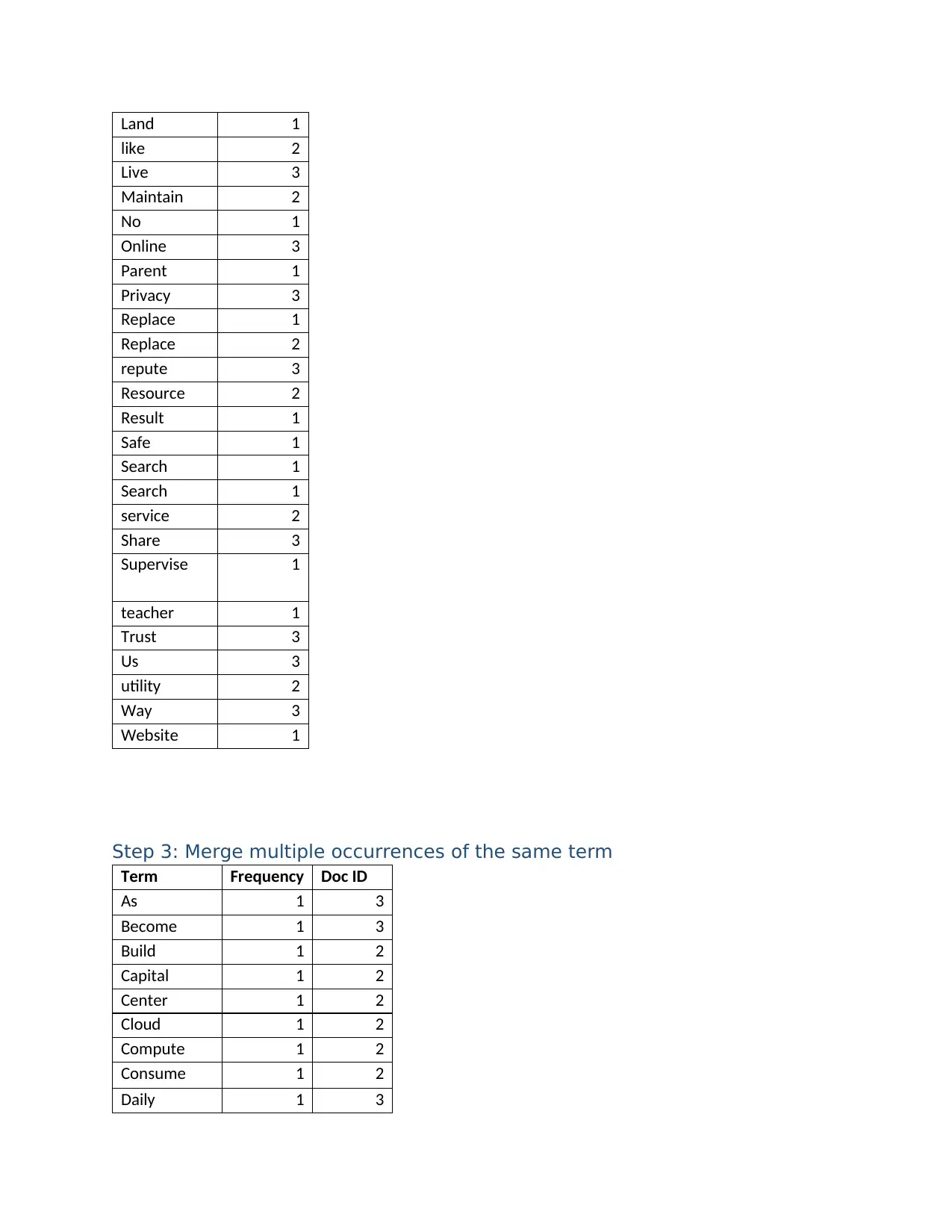
Land 1
like 2
Live 3
Maintain 2
No 1
Online 3
Parent 1
Privacy 3
Replace 1
Replace 2
repute 3
Resource 2
Result 1
Safe 1
Search 1
Search 1
service 2
Share 3
Supervise 1
teacher 1
Trust 3
Us 3
utility 2
Way 3
Website 1
Step 3: Merge multiple occurrences of the same term
Term Frequency Doc ID
As 1 3
Become 1 3
Build 1 2
Capital 1 2
Center 1 2
Cloud 1 2
Compute 1 2
Consume 1 2
Daily 1 3
like 2
Live 3
Maintain 2
No 1
Online 3
Parent 1
Privacy 3
Replace 1
Replace 2
repute 3
Resource 2
Result 1
Safe 1
Search 1
Search 1
service 2
Share 3
Supervise 1
teacher 1
Trust 3
Us 3
utility 2
Way 3
Website 1
Step 3: Merge multiple occurrences of the same term
Term Frequency Doc ID
As 1 3
Become 1 3
Build 1 2
Capital 1 2
Center 1 2
Cloud 1 2
Compute 1 2
Consume 1 2
Daily 1 3
⊘ This is a preview!⊘
Do you want full access?
Subscribe today to unlock all pages.

Trusted by 1+ million students worldwide
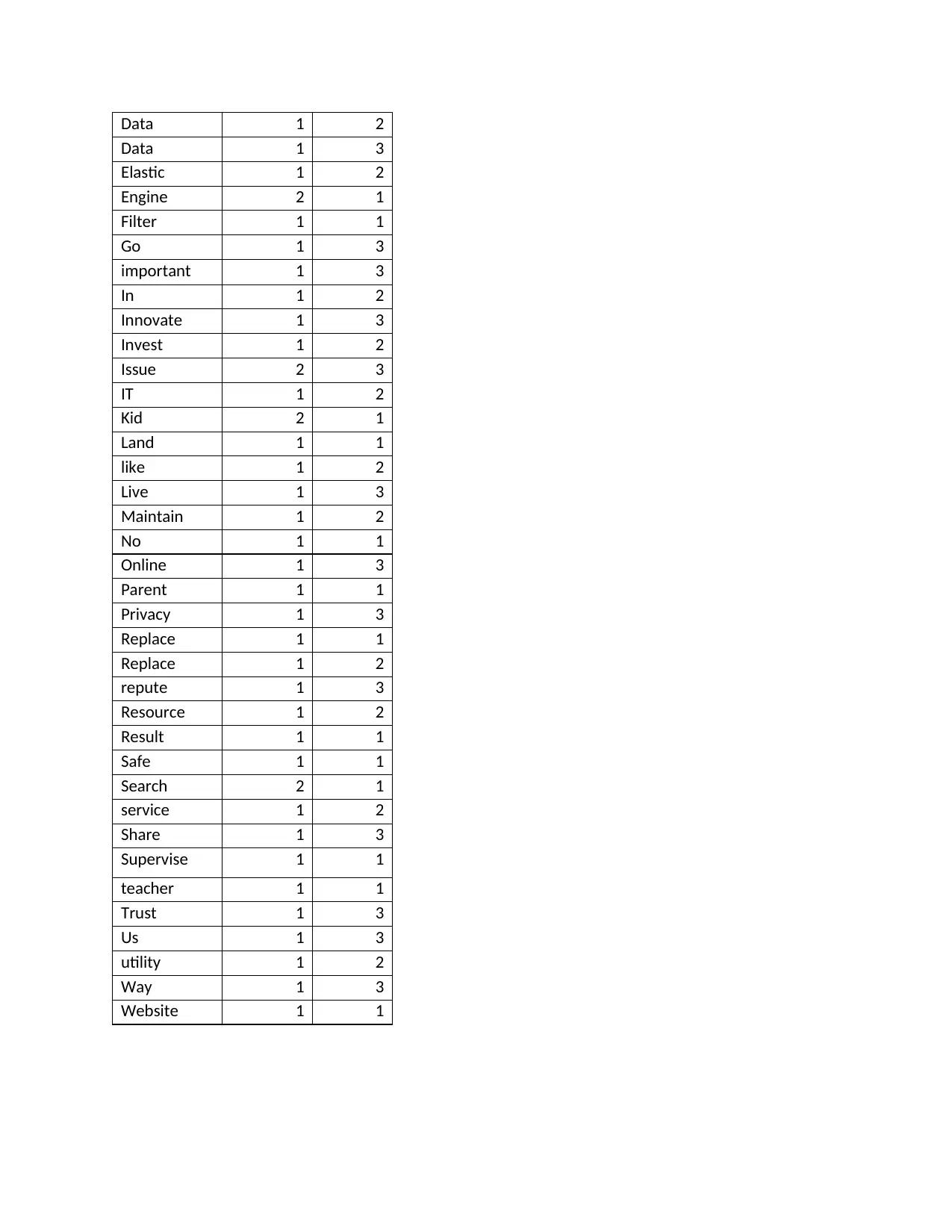
Data 1 2
Data 1 3
Elastic 1 2
Engine 2 1
Filter 1 1
Go 1 3
important 1 3
In 1 2
Innovate 1 3
Invest 1 2
Issue 2 3
IT 1 2
Kid 2 1
Land 1 1
like 1 2
Live 1 3
Maintain 1 2
No 1 1
Online 1 3
Parent 1 1
Privacy 1 3
Replace 1 1
Replace 1 2
repute 1 3
Resource 1 2
Result 1 1
Safe 1 1
Search 2 1
service 1 2
Share 1 3
Supervise 1 1
teacher 1 1
Trust 1 3
Us 1 3
utility 1 2
Way 1 3
Website 1 1
Data 1 3
Elastic 1 2
Engine 2 1
Filter 1 1
Go 1 3
important 1 3
In 1 2
Innovate 1 3
Invest 1 2
Issue 2 3
IT 1 2
Kid 2 1
Land 1 1
like 1 2
Live 1 3
Maintain 1 2
No 1 1
Online 1 3
Parent 1 1
Privacy 1 3
Replace 1 1
Replace 1 2
repute 1 3
Resource 1 2
Result 1 1
Safe 1 1
Search 2 1
service 1 2
Share 1 3
Supervise 1 1
teacher 1 1
Trust 1 3
Us 1 3
utility 1 2
Way 1 3
Website 1 1
Paraphrase This Document
Need a fresh take? Get an instant paraphrase of this document with our AI Paraphraser
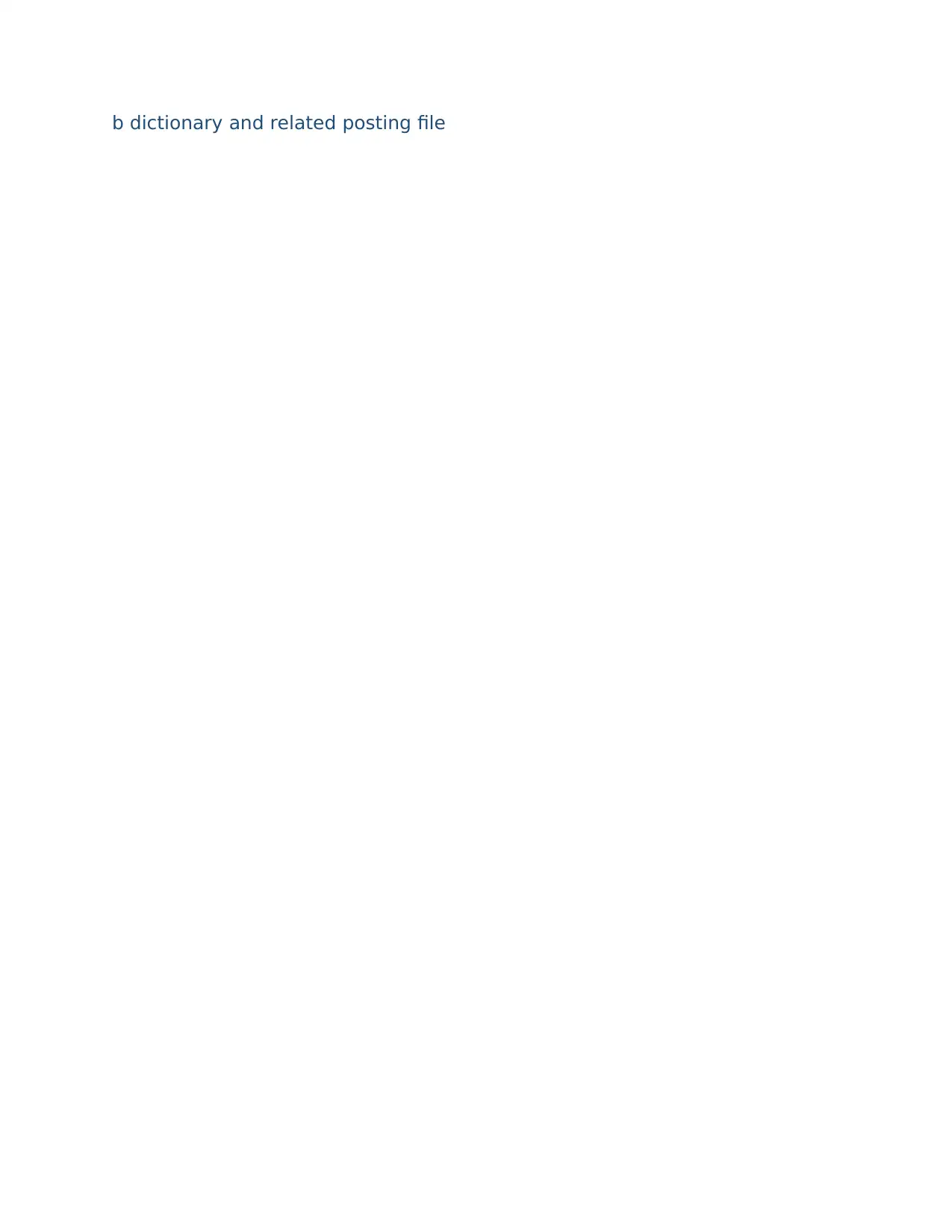
b dictionary and related posting file
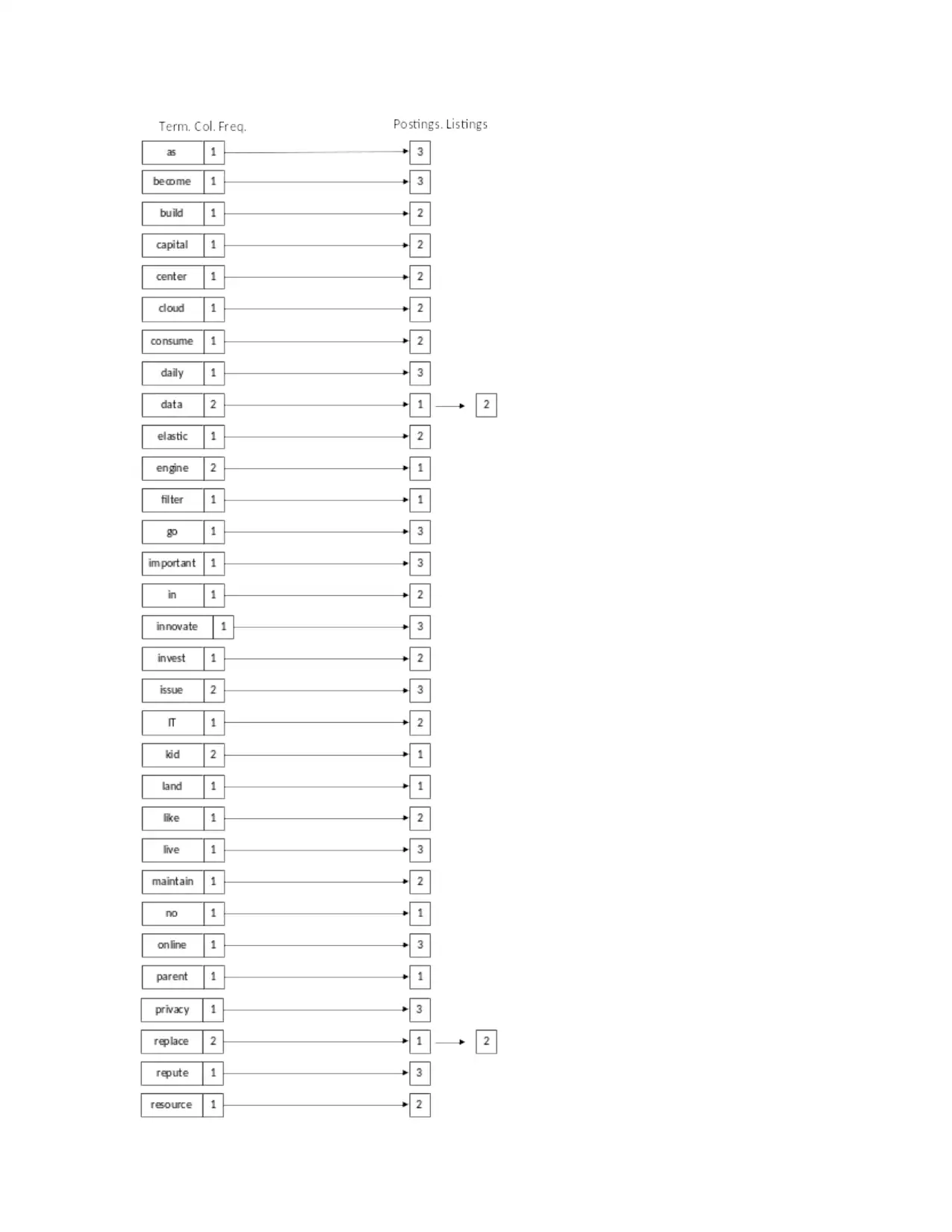
⊘ This is a preview!⊘
Do you want full access?
Subscribe today to unlock all pages.

Trusted by 1+ million students worldwide
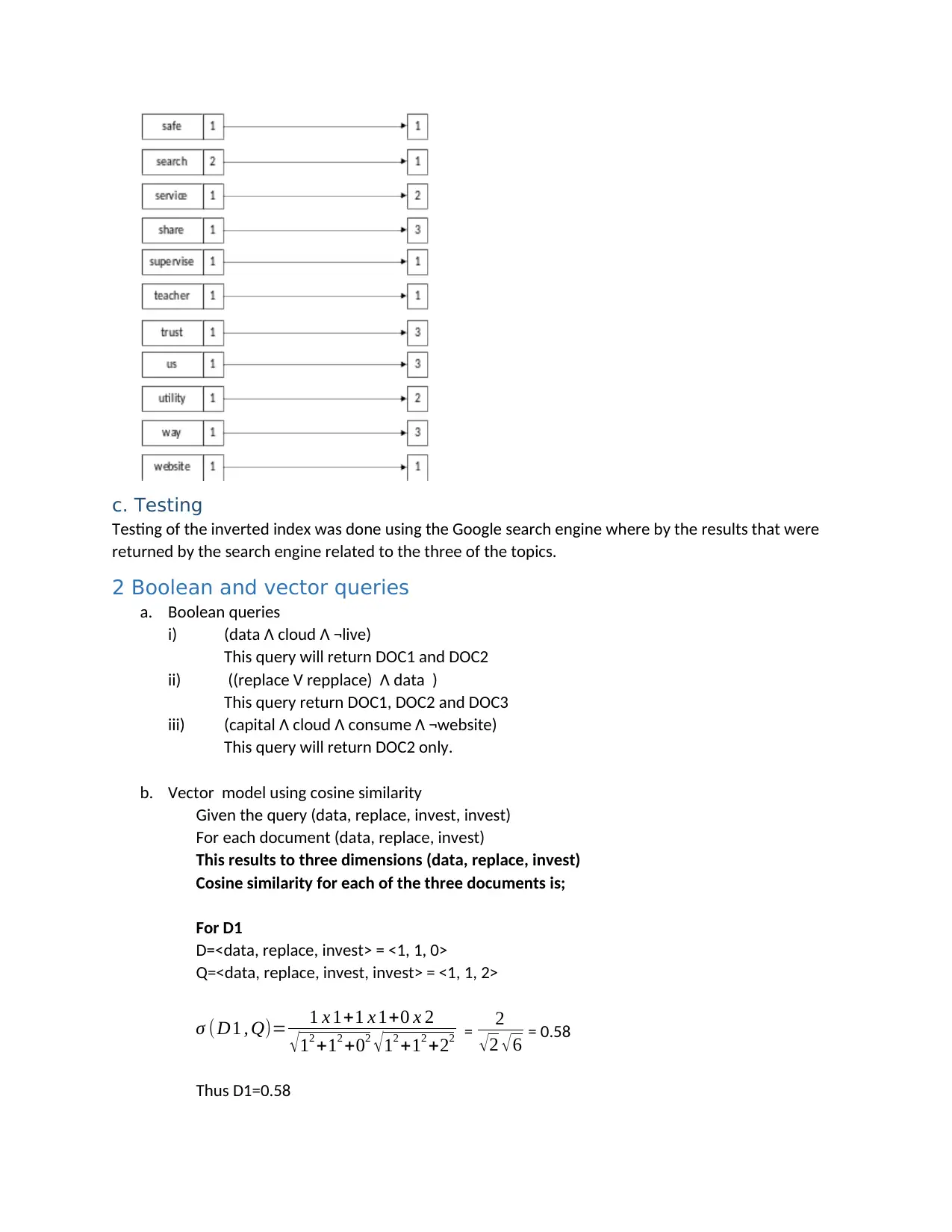
c. Testing
Testing of the inverted index was done using the Google search engine where by the results that were
returned by the search engine related to the three of the topics.
2 Boolean and vector queries
a. Boolean queries
i) (data Ʌ cloud Ʌ ¬live)
This query will return DOC1 and DOC2
ii) ((replace V repplace) Ʌ data )
This query return DOC1, DOC2 and DOC3
iii) (capital Ʌ cloud Ʌ consume Ʌ ¬website)
This query will return DOC2 only.
b. Vector model using cosine similarity
Given the query (data, replace, invest, invest)
For each document (data, replace, invest)
This results to three dimensions (data, replace, invest)
Cosine similarity for each of the three documents is;
For D1
D=<data, replace, invest> = <1, 1, 0>
Q=<data, replace, invest, invest> = <1, 1, 2>
σ ( D1 , Q)= 1 x 1+1 x 1+0 x 2
√ 12 +12 +02 √ 12 +12 +22 = 2
√2 √6 = 0.58
Thus D1=0.58
Testing of the inverted index was done using the Google search engine where by the results that were
returned by the search engine related to the three of the topics.
2 Boolean and vector queries
a. Boolean queries
i) (data Ʌ cloud Ʌ ¬live)
This query will return DOC1 and DOC2
ii) ((replace V repplace) Ʌ data )
This query return DOC1, DOC2 and DOC3
iii) (capital Ʌ cloud Ʌ consume Ʌ ¬website)
This query will return DOC2 only.
b. Vector model using cosine similarity
Given the query (data, replace, invest, invest)
For each document (data, replace, invest)
This results to three dimensions (data, replace, invest)
Cosine similarity for each of the three documents is;
For D1
D=<data, replace, invest> = <1, 1, 0>
Q=<data, replace, invest, invest> = <1, 1, 2>
σ ( D1 , Q)= 1 x 1+1 x 1+0 x 2
√ 12 +12 +02 √ 12 +12 +22 = 2
√2 √6 = 0.58
Thus D1=0.58
Paraphrase This Document
Need a fresh take? Get an instant paraphrase of this document with our AI Paraphraser
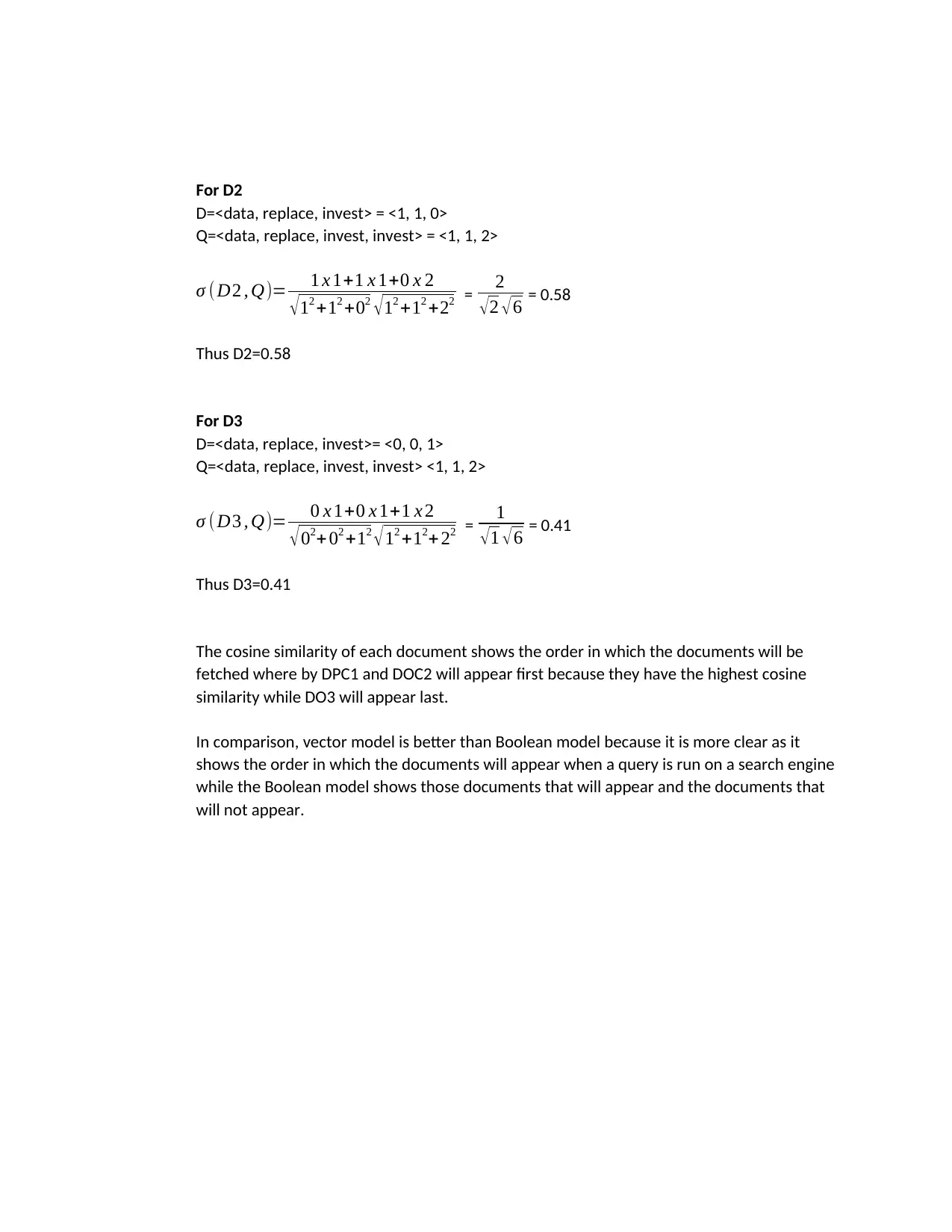
For D2
D=<data, replace, invest> = <1, 1, 0>
Q=<data, replace, invest, invest> = <1, 1, 2>
σ ( D2 , Q)= 1 x 1+1 x 1+0 x 2
√12 +12 +02 √12 +12 +22 = 2
√ 2 √ 6 = 0.58
Thus D2=0.58
For D3
D=<data, replace, invest>= <0, 0, 1>
Q=<data, replace, invest, invest> <1, 1, 2>
σ ( D3 , Q)= 0 x 1+0 x 1+1 x 2
√02+ 02 +12 √12 +12+ 22 = 1
√1 √6 = 0.41
Thus D3=0.41
The cosine similarity of each document shows the order in which the documents will be
fetched where by DPC1 and DOC2 will appear first because they have the highest cosine
similarity while DO3 will appear last.
In comparison, vector model is better than Boolean model because it is more clear as it
shows the order in which the documents will appear when a query is run on a search engine
while the Boolean model shows those documents that will appear and the documents that
will not appear.
D=<data, replace, invest> = <1, 1, 0>
Q=<data, replace, invest, invest> = <1, 1, 2>
σ ( D2 , Q)= 1 x 1+1 x 1+0 x 2
√12 +12 +02 √12 +12 +22 = 2
√ 2 √ 6 = 0.58
Thus D2=0.58
For D3
D=<data, replace, invest>= <0, 0, 1>
Q=<data, replace, invest, invest> <1, 1, 2>
σ ( D3 , Q)= 0 x 1+0 x 1+1 x 2
√02+ 02 +12 √12 +12+ 22 = 1
√1 √6 = 0.41
Thus D3=0.41
The cosine similarity of each document shows the order in which the documents will be
fetched where by DPC1 and DOC2 will appear first because they have the highest cosine
similarity while DO3 will appear last.
In comparison, vector model is better than Boolean model because it is more clear as it
shows the order in which the documents will appear when a query is run on a search engine
while the Boolean model shows those documents that will appear and the documents that
will not appear.
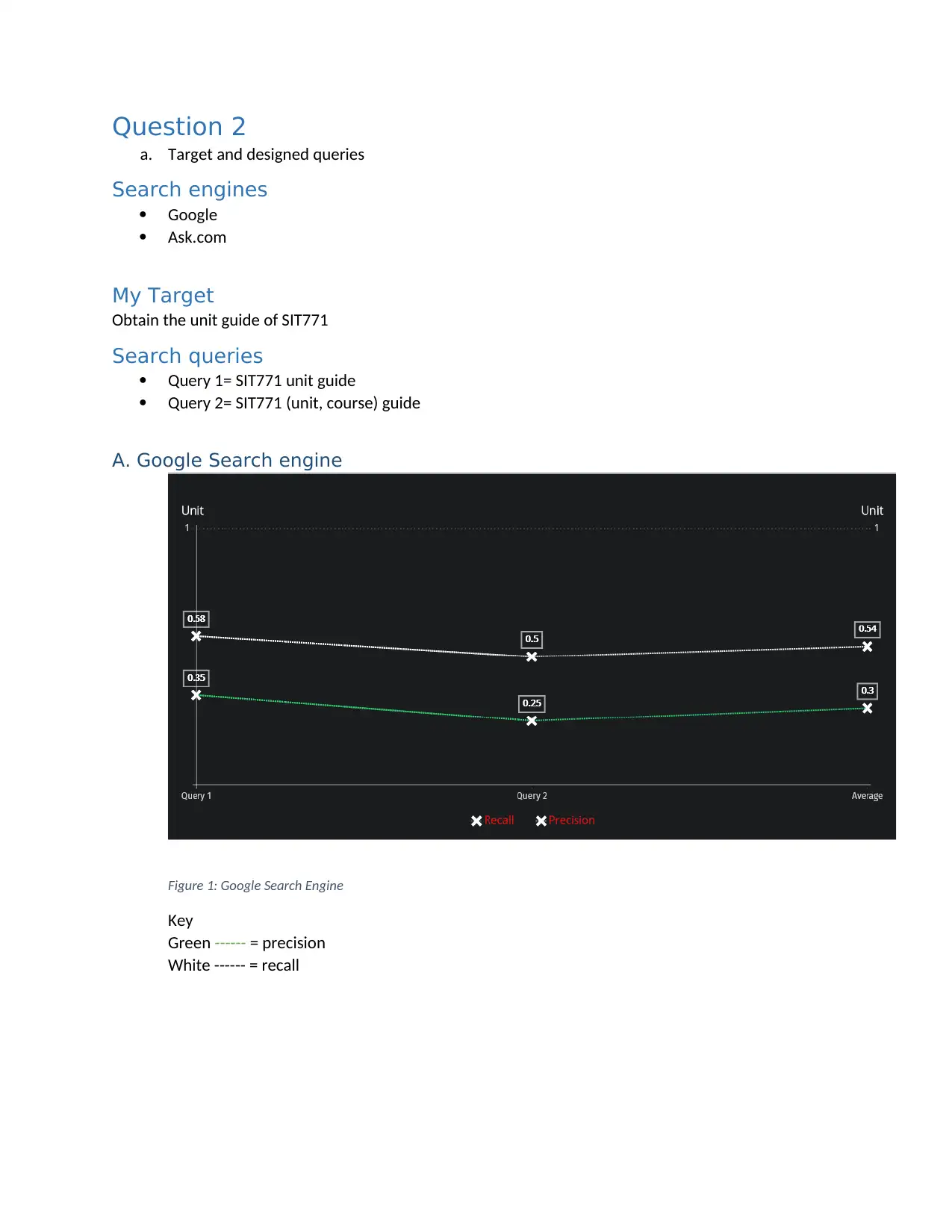
Question 2
a. Target and designed queries
Search engines
Google
Ask.com
My Target
Obtain the unit guide of SIT771
Search queries
Query 1= SIT771 unit guide
Query 2= SIT771 (unit, course) guide
A. Google Search engine
Figure 1: Google Search Engine
Key
Green ------ = precision
White ------ = recall
a. Target and designed queries
Search engines
Ask.com
My Target
Obtain the unit guide of SIT771
Search queries
Query 1= SIT771 unit guide
Query 2= SIT771 (unit, course) guide
A. Google Search engine
Figure 1: Google Search Engine
Key
Green ------ = precision
White ------ = recall
⊘ This is a preview!⊘
Do you want full access?
Subscribe today to unlock all pages.

Trusted by 1+ million students worldwide
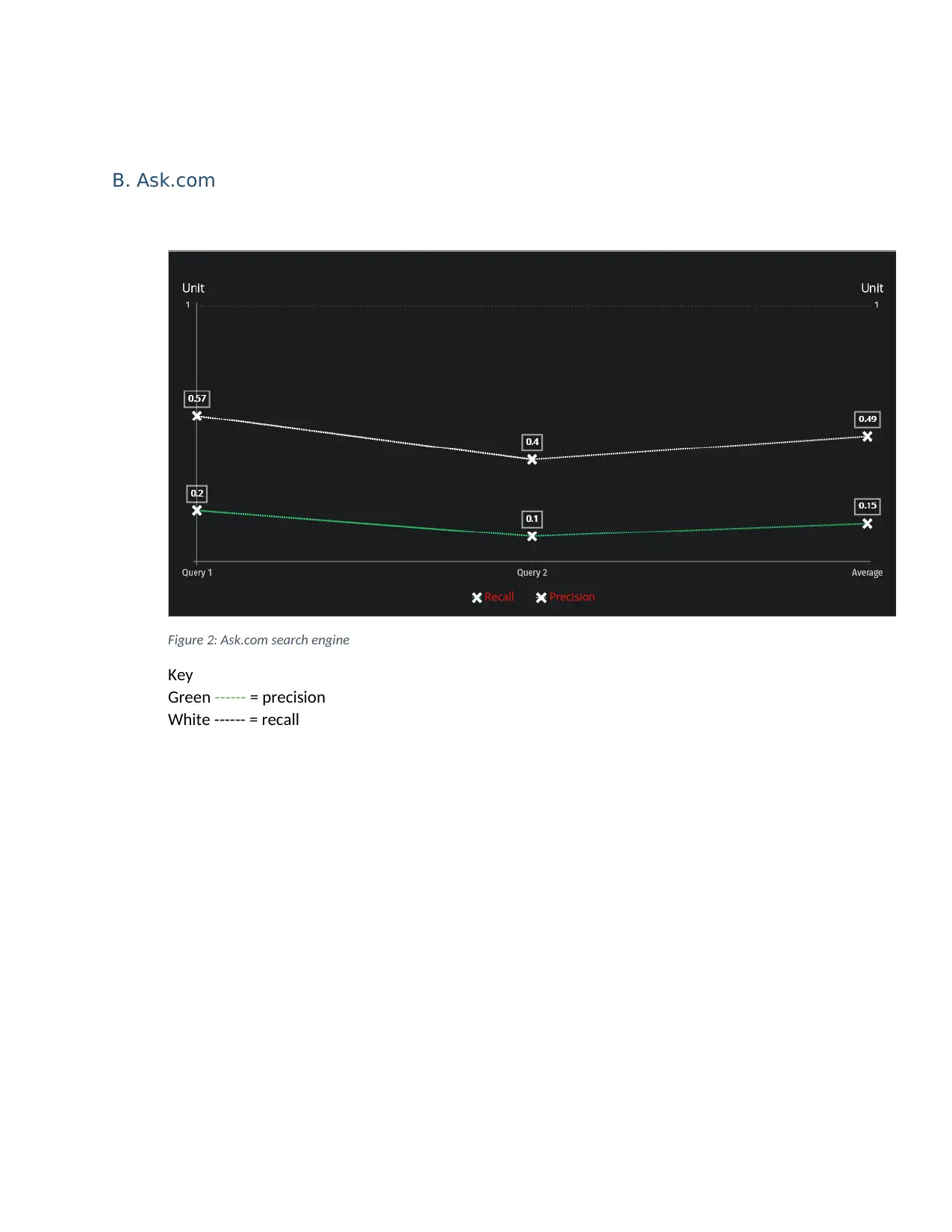
B. Ask.com
Figure 2: Ask.com search engine
Key
Green ------ = precision
White ------ = recall
Figure 2: Ask.com search engine
Key
Green ------ = precision
White ------ = recall
Paraphrase This Document
Need a fresh take? Get an instant paraphrase of this document with our AI Paraphraser
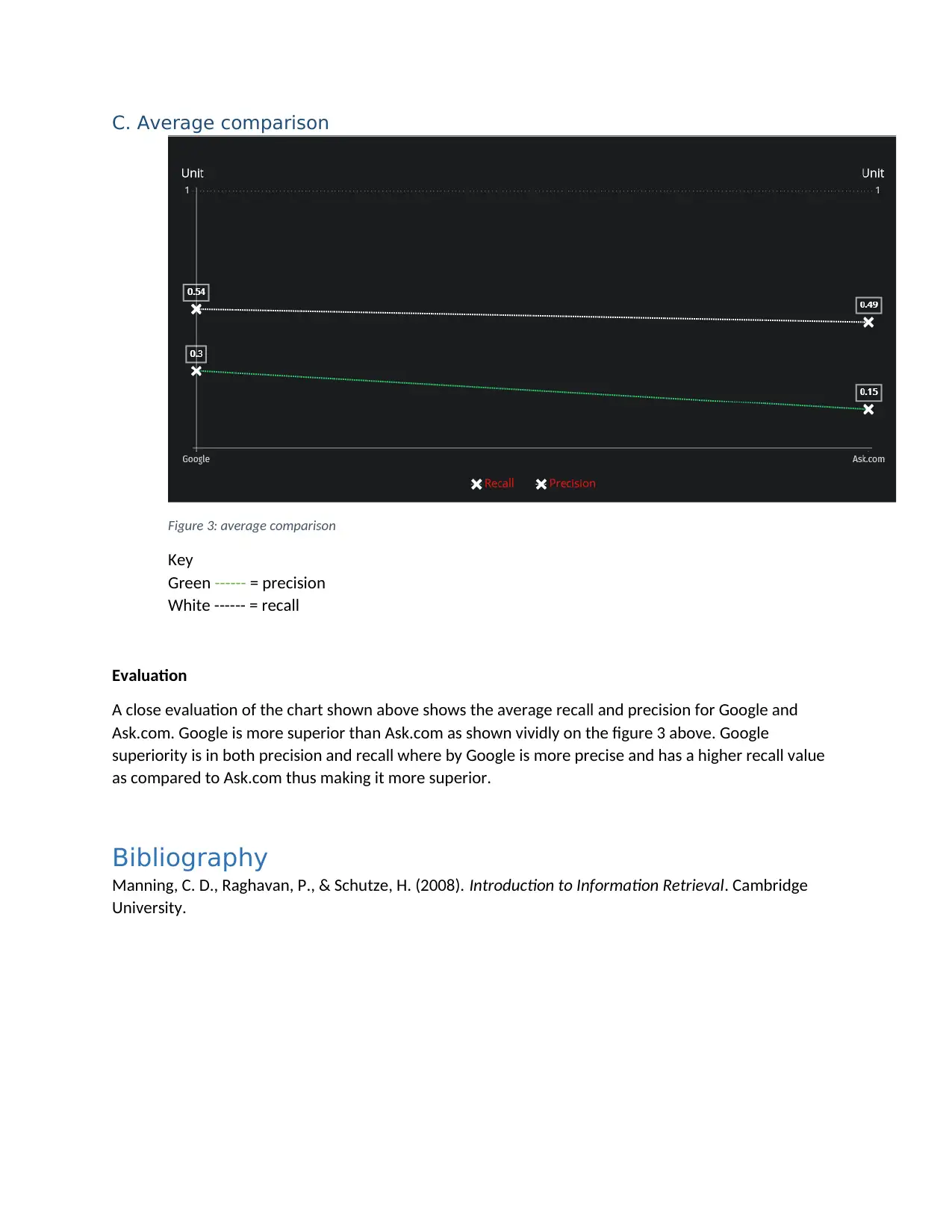
C. Average comparison
Figure 3: average comparison
Key
Green ------ = precision
White ------ = recall
Evaluation
A close evaluation of the chart shown above shows the average recall and precision for Google and
Ask.com. Google is more superior than Ask.com as shown vividly on the figure 3 above. Google
superiority is in both precision and recall where by Google is more precise and has a higher recall value
as compared to Ask.com thus making it more superior.
Bibliography
Manning, C. D., Raghavan, P., & Schutze, H. (2008). Introduction to Information Retrieval. Cambridge
University.
Figure 3: average comparison
Key
Green ------ = precision
White ------ = recall
Evaluation
A close evaluation of the chart shown above shows the average recall and precision for Google and
Ask.com. Google is more superior than Ask.com as shown vividly on the figure 3 above. Google
superiority is in both precision and recall where by Google is more precise and has a higher recall value
as compared to Ask.com thus making it more superior.
Bibliography
Manning, C. D., Raghavan, P., & Schutze, H. (2008). Introduction to Information Retrieval. Cambridge
University.
1 out of 14
Related Documents

Your All-in-One AI-Powered Toolkit for Academic Success.
+13062052269
info@desklib.com
Available 24*7 on WhatsApp / Email
Unlock your academic potential
© 2024 | Zucol Services PVT LTD | All rights reserved.