S&P 500 Prediction: A Multiple Regression Model Analysis Report
VerifiedAdded on 2023/06/04
|11
|2819
|391
Report
AI Summary
This report presents a multiple regression analysis aimed at predicting the behavior of the S&P 500 using various economic indicators. The study employs two regression models, incorporating factors such as Consumer Price Index (CPI), Producer Price Index (PPI), House Price Index (HPI), interest rates, unemployment rate, and Gross Domestic Product (GDP) of the US, Spain, Germany, and China. The first model analyzes the percentage change in S&P 500 against these variables, while the second model focuses on the average annual S&P 500. The analysis reveals the relationships between these economic factors and the stock market, with key findings indicating the impact of US GDP and inverse relationships with HPI. The report provides detailed statistical outputs, including coefficients, R-squared values, and interpretations, offering insights into the predictability and influential factors of the S&P 500.

Running head: MULTIPLE REGRESSION MODEL 1
Assignment One: Multiple Regression Model Analysis
Name
Institution
Assignment One: Multiple Regression Model Analysis
Name
Institution
Paraphrase This Document
Need a fresh take? Get an instant paraphrase of this document with our AI Paraphraser

MULTIPLE REGRESSION MODEL 2
Assignment One: Multiple Regression Model Analysis
Introduction
Today’s stock market offers as many opportunities for investors to raise money as
jeopardizes to lose it because market depends on different factors, such as overall observed
country’s performance, foreign countries’ performance, and unexpected events (Friedberg,
2018). One of the most important stock market indexes is Standard & Poor's 500 (S&P 500) as it
comprises the 500 largest American companies across various industries and sectors. Many
people put their money into the market to get return on investment (Roberts, 2017). Investors ask
themselves questions like how to make money on the stock market and is there a way to predict
in some degree how the stock market will behave? There are lots and lots of variables involved
in how the stock market behaves at a specific time.
The stock market is in a way an information agency. Based on new information, whether
good or bad regarding almost everything from political issues to interest rates and inflation, the
stock market can go up or down. The market is anticipating economic occurrences pro-actively,
ignoring already occurred events that were predicted before. This way it is very hard to predict
how it is going to move in the future. As S&P 500 is considered to be the most reliable
benchmark for the overall U.S. stock market (Berger, 2017), we decided to study what factor has
the most impact on it. We created two regression models and included the economic indicators,
such as Consumer Price Index, Producer Price Index, House Price index, Interest Rate,
Unemployment Rate, and Gross Domestic Product of some countries.
First Regression Model Specifications and Data
Assignment One: Multiple Regression Model Analysis
Introduction
Today’s stock market offers as many opportunities for investors to raise money as
jeopardizes to lose it because market depends on different factors, such as overall observed
country’s performance, foreign countries’ performance, and unexpected events (Friedberg,
2018). One of the most important stock market indexes is Standard & Poor's 500 (S&P 500) as it
comprises the 500 largest American companies across various industries and sectors. Many
people put their money into the market to get return on investment (Roberts, 2017). Investors ask
themselves questions like how to make money on the stock market and is there a way to predict
in some degree how the stock market will behave? There are lots and lots of variables involved
in how the stock market behaves at a specific time.
The stock market is in a way an information agency. Based on new information, whether
good or bad regarding almost everything from political issues to interest rates and inflation, the
stock market can go up or down. The market is anticipating economic occurrences pro-actively,
ignoring already occurred events that were predicted before. This way it is very hard to predict
how it is going to move in the future. As S&P 500 is considered to be the most reliable
benchmark for the overall U.S. stock market (Berger, 2017), we decided to study what factor has
the most impact on it. We created two regression models and included the economic indicators,
such as Consumer Price Index, Producer Price Index, House Price index, Interest Rate,
Unemployment Rate, and Gross Domestic Product of some countries.
First Regression Model Specifications and Data
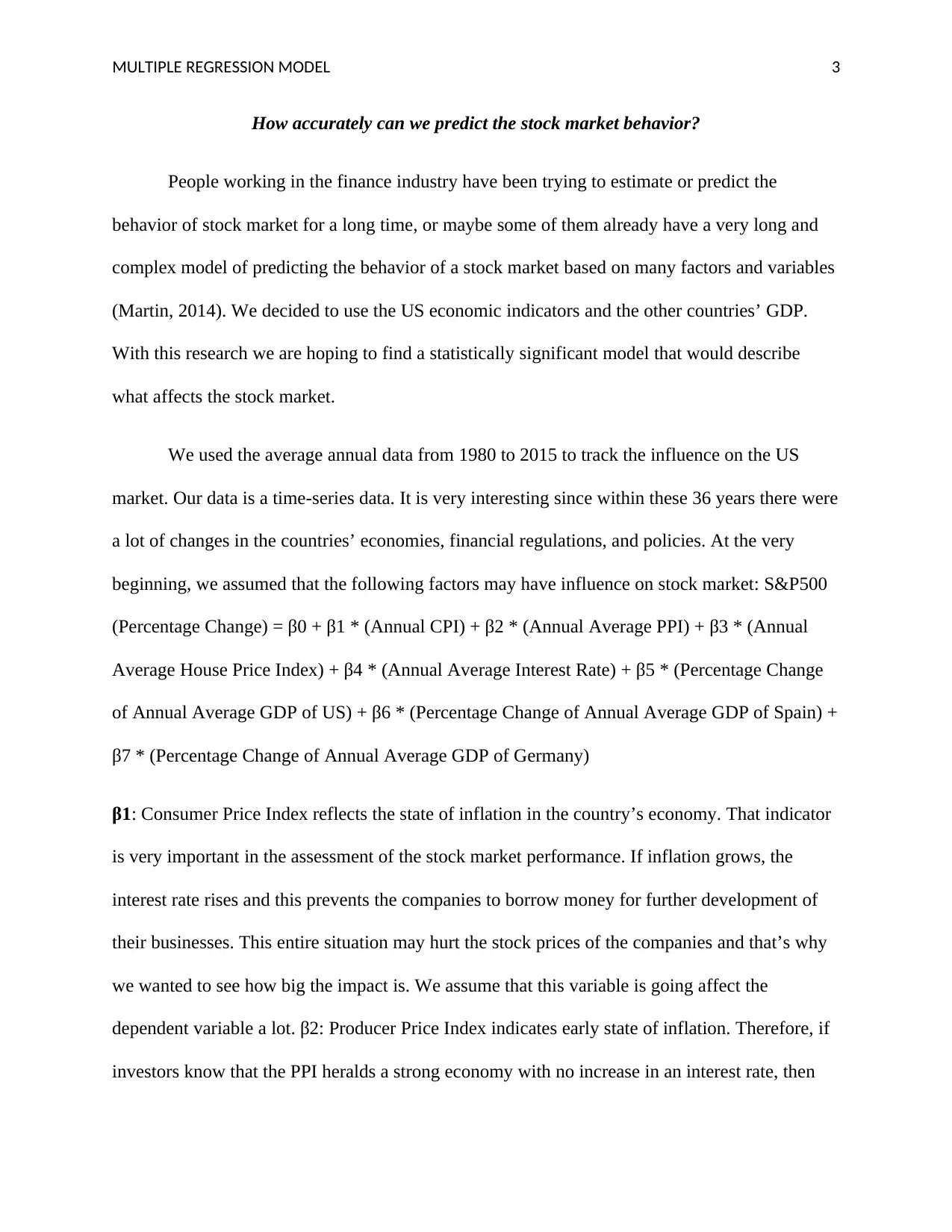
MULTIPLE REGRESSION MODEL 3
How accurately can we predict the stock market behavior?
People working in the finance industry have been trying to estimate or predict the
behavior of stock market for a long time, or maybe some of them already have a very long and
complex model of predicting the behavior of a stock market based on many factors and variables
(Martin, 2014). We decided to use the US economic indicators and the other countries’ GDP.
With this research we are hoping to find a statistically significant model that would describe
what affects the stock market.
We used the average annual data from 1980 to 2015 to track the influence on the US
market. Our data is a time-series data. It is very interesting since within these 36 years there were
a lot of changes in the countries’ economies, financial regulations, and policies. At the very
beginning, we assumed that the following factors may have influence on stock market: S&P500
(Percentage Change) = β0 + β1 * (Annual CPI) + β2 * (Annual Average PPI) + β3 * (Annual
Average House Price Index) + β4 * (Annual Average Interest Rate) + β5 * (Percentage Change
of Annual Average GDP of US) + β6 * (Percentage Change of Annual Average GDP of Spain) +
β7 * (Percentage Change of Annual Average GDP of Germany)
β1: Consumer Price Index reflects the state of inflation in the country’s economy. That indicator
is very important in the assessment of the stock market performance. If inflation grows, the
interest rate rises and this prevents the companies to borrow money for further development of
their businesses. This entire situation may hurt the stock prices of the companies and that’s why
we wanted to see how big the impact is. We assume that this variable is going affect the
dependent variable a lot. β2: Producer Price Index indicates early state of inflation. Therefore, if
investors know that the PPI heralds a strong economy with no increase in an interest rate, then
How accurately can we predict the stock market behavior?
People working in the finance industry have been trying to estimate or predict the
behavior of stock market for a long time, or maybe some of them already have a very long and
complex model of predicting the behavior of a stock market based on many factors and variables
(Martin, 2014). We decided to use the US economic indicators and the other countries’ GDP.
With this research we are hoping to find a statistically significant model that would describe
what affects the stock market.
We used the average annual data from 1980 to 2015 to track the influence on the US
market. Our data is a time-series data. It is very interesting since within these 36 years there were
a lot of changes in the countries’ economies, financial regulations, and policies. At the very
beginning, we assumed that the following factors may have influence on stock market: S&P500
(Percentage Change) = β0 + β1 * (Annual CPI) + β2 * (Annual Average PPI) + β3 * (Annual
Average House Price Index) + β4 * (Annual Average Interest Rate) + β5 * (Percentage Change
of Annual Average GDP of US) + β6 * (Percentage Change of Annual Average GDP of Spain) +
β7 * (Percentage Change of Annual Average GDP of Germany)
β1: Consumer Price Index reflects the state of inflation in the country’s economy. That indicator
is very important in the assessment of the stock market performance. If inflation grows, the
interest rate rises and this prevents the companies to borrow money for further development of
their businesses. This entire situation may hurt the stock prices of the companies and that’s why
we wanted to see how big the impact is. We assume that this variable is going affect the
dependent variable a lot. β2: Producer Price Index indicates early state of inflation. Therefore, if
investors know that the PPI heralds a strong economy with no increase in an interest rate, then
You're viewing a preview
Unlock full access by subscribing today!

MULTIPLE REGRESSION MODEL 4
they feel confident to invest in the businesses what means increased positive activity in the
market. We assume that this variable is going to have some impact on the dependent variable
however; it is not going to be crucial.
β3: House Price Index is an analytical tool for estimating changes in the rates of mortgages. If
mortgage rates are high, then housing market is weak because demand for houses drops due to
expensive loans, therefore HPI drops (Poff & Poff, 2013). In 2008 mortgage default affected
stock market very severely because before that period house prices went down because people
couldn’t pay their mortgage payments and banks collapsed. Decrease in house prices is one of
the possible contributors to recession because the home owners lose their equity in their houses.
Considering such recession scenario, the stock market always becomes bearish. Additionally,
house market is considered more stable investment than stock market. When stock market drops,
people are willing in the houses and HPI goes up. We assume that HPI and stock market
shouldn’t move in the same direction thereby we don’t take into consideration the complex
scenario of 2008.
β4: 10-Year Treasury Constant Maturity Rate impacts on the number of issued bond and is used
as risk free rate to calculate the excess return on the investment. It also has an influence on the
stock market.
β5: Gross Domestic Product of the US is important for business profit and this can drive the
stock prices up (Poff & Poff, 2013). Investing in the stock market seems reasonable when the
economy is doing well. If the economy is growing fast then the stock market should be affected
positively, the investors are more optimistic about the future and they put more money into
they feel confident to invest in the businesses what means increased positive activity in the
market. We assume that this variable is going to have some impact on the dependent variable
however; it is not going to be crucial.
β3: House Price Index is an analytical tool for estimating changes in the rates of mortgages. If
mortgage rates are high, then housing market is weak because demand for houses drops due to
expensive loans, therefore HPI drops (Poff & Poff, 2013). In 2008 mortgage default affected
stock market very severely because before that period house prices went down because people
couldn’t pay their mortgage payments and banks collapsed. Decrease in house prices is one of
the possible contributors to recession because the home owners lose their equity in their houses.
Considering such recession scenario, the stock market always becomes bearish. Additionally,
house market is considered more stable investment than stock market. When stock market drops,
people are willing in the houses and HPI goes up. We assume that HPI and stock market
shouldn’t move in the same direction thereby we don’t take into consideration the complex
scenario of 2008.
β4: 10-Year Treasury Constant Maturity Rate impacts on the number of issued bond and is used
as risk free rate to calculate the excess return on the investment. It also has an influence on the
stock market.
β5: Gross Domestic Product of the US is important for business profit and this can drive the
stock prices up (Poff & Poff, 2013). Investing in the stock market seems reasonable when the
economy is doing well. If the economy is growing fast then the stock market should be affected
positively, the investors are more optimistic about the future and they put more money into
Paraphrase This Document
Need a fresh take? Get an instant paraphrase of this document with our AI Paraphraser

MULTIPLE REGRESSION MODEL 5
market more. This variable is crucial for the dependent one.
β6: Gross Domestic Product of Spain. Since Europe is currently in a recession, we wanted to
include the GDP of Spain, as one of the weakest economies in Europe now, to check if there is
any relationship between Spain’s economy and the US stock market performance. Very small
percentage of US investments goes to Spain (Poff & Poff, 2013). Compared to Germany, which
is the 5th country the USA invests into, Spain is the 31st country on the list. There should not be
any correlation between these two variables, so we included Spain’s GDP into our regression to
check our hypothesis.
β7: Gross Domestic Product of Germany is an indicator of Germany is the 5th largest economy
in the world and is the largest European trade and investment partner of the US. Germany is the
largest economy in Europe and almost 1/5 of GDP of the European Union is that of Germany
alone. We assume that this variable has to have an impact on the US stock market.
Second Regression Model
S&P500 (Annual Average) = β0 + β1 * (Annual CPI) + β2 * (Annual Average House Price
Index) + β3 * (Annual Average Interest Rate) + β4 * (Average Annual Unemployment Rate) +
β5 * (Annual Average GDP of US) + β6 * (Annual Average GDP of Germany) + β7 * (Annual
Average GDP of China). After we run the regression of the second model, it resulted in
improving of our model accuracy. We excluded PPI, GDP of Spain because it came out that
these variables have no impact on the US stock market. Also, we added the unemployment rate
and GDP of China because it is the largest US business partner.
Explanation of the new variables
market more. This variable is crucial for the dependent one.
β6: Gross Domestic Product of Spain. Since Europe is currently in a recession, we wanted to
include the GDP of Spain, as one of the weakest economies in Europe now, to check if there is
any relationship between Spain’s economy and the US stock market performance. Very small
percentage of US investments goes to Spain (Poff & Poff, 2013). Compared to Germany, which
is the 5th country the USA invests into, Spain is the 31st country on the list. There should not be
any correlation between these two variables, so we included Spain’s GDP into our regression to
check our hypothesis.
β7: Gross Domestic Product of Germany is an indicator of Germany is the 5th largest economy
in the world and is the largest European trade and investment partner of the US. Germany is the
largest economy in Europe and almost 1/5 of GDP of the European Union is that of Germany
alone. We assume that this variable has to have an impact on the US stock market.
Second Regression Model
S&P500 (Annual Average) = β0 + β1 * (Annual CPI) + β2 * (Annual Average House Price
Index) + β3 * (Annual Average Interest Rate) + β4 * (Average Annual Unemployment Rate) +
β5 * (Annual Average GDP of US) + β6 * (Annual Average GDP of Germany) + β7 * (Annual
Average GDP of China). After we run the regression of the second model, it resulted in
improving of our model accuracy. We excluded PPI, GDP of Spain because it came out that
these variables have no impact on the US stock market. Also, we added the unemployment rate
and GDP of China because it is the largest US business partner.
Explanation of the new variables

MULTIPLE REGRESSION MODEL 6
Unemployment Rate is one of the most important factors of the economy’s performance.
High unemployment rate decreases the buyer power of the consumers. 2/3 of the US economy is
consumer based and it influences the stock market negatively. We assume that there is a
relationship between these two variables.
Gross Domestic Product of China affects the US economy because cheap export from China
prevents inflation in the US. China is a huge buyer of the US Treasuries. It lowers the interest
rate and companies borrow money to invest in development hence, it directly affects the stock
market. We assume that GDP of China and US stock market move in the same direction,
meaning if China does well, it has money to buy US Treasuries. Additionally, the US stock
market increases because production of those US companies that is outsourced to China grows.
Data analysis and Discussion
i) First Regression Model
Multiple regression analyses were carried out to evaluate the relationship between S &
Index, and factors that we assumed influenced the stock market: Annual CPI (CPI), Annual
average PPI (PPI), Annual average House Price Index (HPI), annual average interest Rate
(IR_US), Percentage change of annual average GDP of the US (GDP-R_US), Percentage
average GDP of Spain (GDP-R_S), and the percentage change of annual average GDP of
Germany (GDP-R_G). Table 1 and table 2 below summarize key statistics found during MS
Excel multiple regression analysis.
The multiple regression model with all seven explanatory variables produced coefficients
of CPI, PPI, HPI, IR_US, GDP-R-US, GDP-R_S, and GDP-R_G as 0.002, 0.004, -0.001, 3.567,
0.005, and 0.0159, respectively. A negative coefficient indicates indirect relationship between
Unemployment Rate is one of the most important factors of the economy’s performance.
High unemployment rate decreases the buyer power of the consumers. 2/3 of the US economy is
consumer based and it influences the stock market negatively. We assume that there is a
relationship between these two variables.
Gross Domestic Product of China affects the US economy because cheap export from China
prevents inflation in the US. China is a huge buyer of the US Treasuries. It lowers the interest
rate and companies borrow money to invest in development hence, it directly affects the stock
market. We assume that GDP of China and US stock market move in the same direction,
meaning if China does well, it has money to buy US Treasuries. Additionally, the US stock
market increases because production of those US companies that is outsourced to China grows.
Data analysis and Discussion
i) First Regression Model
Multiple regression analyses were carried out to evaluate the relationship between S &
Index, and factors that we assumed influenced the stock market: Annual CPI (CPI), Annual
average PPI (PPI), Annual average House Price Index (HPI), annual average interest Rate
(IR_US), Percentage change of annual average GDP of the US (GDP-R_US), Percentage
average GDP of Spain (GDP-R_S), and the percentage change of annual average GDP of
Germany (GDP-R_G). Table 1 and table 2 below summarize key statistics found during MS
Excel multiple regression analysis.
The multiple regression model with all seven explanatory variables produced coefficients
of CPI, PPI, HPI, IR_US, GDP-R-US, GDP-R_S, and GDP-R_G as 0.002, 0.004, -0.001, 3.567,
0.005, and 0.0159, respectively. A negative coefficient indicates indirect relationship between
You're viewing a preview
Unlock full access by subscribing today!

MULTIPLE REGRESSION MODEL 7
the predictor and the response variable (Montgomery, Peck, & Vining, 2010). Consequently,
judging from the nature of the coefficients of the predictors, it can be noted the annual House
Price Index is the only variable that has an inverse relationship with S& P average percentage
change in price. However, the sign of the coefficients is not a satisfactory evidence of the impact
of the predictors on the response variable (Montgomery, 2013). Accordingly, we have to
evaluate other factors that affect the relationship. At this point, it is possible to have a rough
estimate of the multiple linear regression function will look like. Factoring the coefficients in
table 1 in the above regression model function, we have:
S∧P 500 ( Percentage C h ange )
¿−0.057+0.002∗CPI +0.004 PPI −0.001 HPI +0.01 IR−RUS
+3.567 GDP−RUS +0.005 GDP−RS−0.0159 RG
βi Value
Intercept -0.570565784
CPI 0.002095352
PPI 0.004103526
HPI -0.001299457
IR_US 0.010369749
GDP-R_US 3.566738211
GDP-R_S 0.005194637
GDP-R_G 0.015878802
Table 1: Coefficients of Predictors from Regression Model 1
Regression
the predictor and the response variable (Montgomery, Peck, & Vining, 2010). Consequently,
judging from the nature of the coefficients of the predictors, it can be noted the annual House
Price Index is the only variable that has an inverse relationship with S& P average percentage
change in price. However, the sign of the coefficients is not a satisfactory evidence of the impact
of the predictors on the response variable (Montgomery, 2013). Accordingly, we have to
evaluate other factors that affect the relationship. At this point, it is possible to have a rough
estimate of the multiple linear regression function will look like. Factoring the coefficients in
table 1 in the above regression model function, we have:
S∧P 500 ( Percentage C h ange )
¿−0.057+0.002∗CPI +0.004 PPI −0.001 HPI +0.01 IR−RUS
+3.567 GDP−RUS +0.005 GDP−RS−0.0159 RG
βi Value
Intercept -0.570565784
CPI 0.002095352
PPI 0.004103526
HPI -0.001299457
IR_US 0.010369749
GDP-R_US 3.566738211
GDP-R_S 0.005194637
GDP-R_G 0.015878802
Table 1: Coefficients of Predictors from Regression Model 1
Regression
Paraphrase This Document
Need a fresh take? Get an instant paraphrase of this document with our AI Paraphraser

MULTIPLE REGRESSION MODEL 8
Statistics
Multiple R 0.667159679
R Square 0.445102037
Adjusted R
Square 0.306377546
Standard Error 0.116213565
Observations 36
Table 2: Key Regression Statistics-1
From table 2 above, the value of Multiple R was 0.667. The value of Multiple R is the
correlation coefficient. It explains the strength of the linear relationship between response and
explanatory variables (Smith & Draper, 2012). A value of 0.667 tells us that there is a relatively
strong linear relationship between S & P percentage change in prices and the factors suspect its
prices. R Square is t h e coefficient of determination. Given that R square of 0.445 was found, it
means that on average, and assuming that all the predictors are significant, 44.5% of the variation
of S & P 500 values around the mean are explained by the above predictors. Simply put, 44.5%
(value of R Square) indicates that 44.5% of the values fit the model.
ii) Second Regression Model
Table 3 below shows coefficients of the variables from the second regression model. Unlike
the first regression model, two predictors, again judging by the nature of the coefficients,
have an inverse relationship with S & P 500 index. These are CPI, and HPI. One common
occurrence between the first and the second models HPI; the coefficient of HPI is negative in
Statistics
Multiple R 0.667159679
R Square 0.445102037
Adjusted R
Square 0.306377546
Standard Error 0.116213565
Observations 36
Table 2: Key Regression Statistics-1
From table 2 above, the value of Multiple R was 0.667. The value of Multiple R is the
correlation coefficient. It explains the strength of the linear relationship between response and
explanatory variables (Smith & Draper, 2012). A value of 0.667 tells us that there is a relatively
strong linear relationship between S & P percentage change in prices and the factors suspect its
prices. R Square is t h e coefficient of determination. Given that R square of 0.445 was found, it
means that on average, and assuming that all the predictors are significant, 44.5% of the variation
of S & P 500 values around the mean are explained by the above predictors. Simply put, 44.5%
(value of R Square) indicates that 44.5% of the values fit the model.
ii) Second Regression Model
Table 3 below shows coefficients of the variables from the second regression model. Unlike
the first regression model, two predictors, again judging by the nature of the coefficients,
have an inverse relationship with S & P 500 index. These are CPI, and HPI. One common
occurrence between the first and the second models HPI; the coefficient of HPI is negative in

MULTIPLE REGRESSION MODEL 9
both case. This may imply that it has an inverse relationship with response variable. That is,
when the value of S & P 500 increases, the value of HPI decreases, and vice versa.
Beta Value
Intercept 1180.901781
CPI -68.41837729
HPI -8.287699927
IR_US 59.42334256
UnR_US 3693.87536
GDP_US 2.78602E-07
GDP_G 1.60727E-09
GDP_C -1.13818E-10
Table 3: Coefficients from Regression Model 2
Regression
Statistics
Multiple R
0.98779194
2
R Square
0.97573292
1
Adjusted R
Square
0.96966615
1
Standard Error 97.4915342
Observations 36
Table 4: key statistics from regression Model 2
both case. This may imply that it has an inverse relationship with response variable. That is,
when the value of S & P 500 increases, the value of HPI decreases, and vice versa.
Beta Value
Intercept 1180.901781
CPI -68.41837729
HPI -8.287699927
IR_US 59.42334256
UnR_US 3693.87536
GDP_US 2.78602E-07
GDP_G 1.60727E-09
GDP_C -1.13818E-10
Table 3: Coefficients from Regression Model 2
Regression
Statistics
Multiple R
0.98779194
2
R Square
0.97573292
1
Adjusted R
Square
0.96966615
1
Standard Error 97.4915342
Observations 36
Table 4: key statistics from regression Model 2
You're viewing a preview
Unlock full access by subscribing today!

MULTIPLE REGRESSION MODEL 10
The output of the second regression analysis had a Multiple R of 0.988 and R square of 0.976.
Apparently, the second model shows a stronger relationship between response and predictor
variables. It is also important to note that the second model, due to the value of Q square,
demonstrates that there is a 97.57 % of of S & P 500 are explained by CPI, HPI, and average
annual rate of interest in the US, Unemployment Rate, and the real GDP of the United States,
Germany, and China
Conclusion
In summary, from the above regression analysis, it can be concluded that annual CPI,
annual average House price Index, Annual average interest rate, average annual unemployment
rate, annual average GDP of US, annual average GDP of Germany, and Annual average GDP of
China have significant influence on the market price of the stock. Consequently, it is important
to evaluate, and comprehend the direction of the influence. From the above result, it can be noted
that apart from HPI, the remaining predictors have a positive impact on the market price index.
Recommendations
We recommend that the following be studied as way of making the model better, and more
reliable:
i) Significance test be carried out on all predictors in the response variables.
ii) Further study be carried out on behavior of HPI in relationship to the market price
index to ascertain the nature of its effect in the market.
iii) A regression analysis involving all the predictors highlighted in this paper be
analyzed in a single regression mode, and separately with S & P 500 to ascertain their
joint and individual impact on the stock market prices.
The output of the second regression analysis had a Multiple R of 0.988 and R square of 0.976.
Apparently, the second model shows a stronger relationship between response and predictor
variables. It is also important to note that the second model, due to the value of Q square,
demonstrates that there is a 97.57 % of of S & P 500 are explained by CPI, HPI, and average
annual rate of interest in the US, Unemployment Rate, and the real GDP of the United States,
Germany, and China
Conclusion
In summary, from the above regression analysis, it can be concluded that annual CPI,
annual average House price Index, Annual average interest rate, average annual unemployment
rate, annual average GDP of US, annual average GDP of Germany, and Annual average GDP of
China have significant influence on the market price of the stock. Consequently, it is important
to evaluate, and comprehend the direction of the influence. From the above result, it can be noted
that apart from HPI, the remaining predictors have a positive impact on the market price index.
Recommendations
We recommend that the following be studied as way of making the model better, and more
reliable:
i) Significance test be carried out on all predictors in the response variables.
ii) Further study be carried out on behavior of HPI in relationship to the market price
index to ascertain the nature of its effect in the market.
iii) A regression analysis involving all the predictors highlighted in this paper be
analyzed in a single regression mode, and separately with S & P 500 to ascertain their
joint and individual impact on the stock market prices.
Paraphrase This Document
Need a fresh take? Get an instant paraphrase of this document with our AI Paraphraser

MULTIPLE REGRESSION MODEL 11
References
Berger, R. (2017, June 1). Total U.S. Stock Market Vs. The S&P 500 Index - An Investor's Guide.
Retrieved from Forbes: https://www.forbes.com/sites/robertberger/2017/06/01/total-u-s-
stock-market-vs-the-sp-500-index-an-investors-guide/#611775262fd0
Friedberg, B. (2018, May 10). Why People Lose Money in the Market. Retrieved from The
Balance: https://www.thebalance.com/why-people-lose-money-in-the-market-4144737
Martin, R. (2014, August 28). Why the S&P 500 is a better gauge than the Dow. Retrieved from
Money Watch: https://www.cbsnews.com/news/dow-jones-industrial-average-sp-500-
which-is-better/
Montgomery, D. (2013(b)). Introduction to Linear Regression Analysis, Fifth Edition Set. New
York: Wiley.
Montgomery, D., Peck, E., & Vining, G. (2010). Introduction to Linear Regression Analysis.
New Yrok: Wiley-Interscience.
Poff, J., & Poff, R. (2013). Stock Market and Financial Investing. Amazon Digital Services.
Roberts, L. (2017, July 26). Opinion: If the stock market can make you rich, why are so many
Americans poor? Retrieved from Neustar: https://www.marketwatch.com/story/if-the-
stock-market-can-make-you-rich-why-are-so-many-americans-poor-2017-07-26
Smith, H., & Draper, N. (2012). Applied Regression Analysis. New Yrok: Wiley-Interscience.
References
Berger, R. (2017, June 1). Total U.S. Stock Market Vs. The S&P 500 Index - An Investor's Guide.
Retrieved from Forbes: https://www.forbes.com/sites/robertberger/2017/06/01/total-u-s-
stock-market-vs-the-sp-500-index-an-investors-guide/#611775262fd0
Friedberg, B. (2018, May 10). Why People Lose Money in the Market. Retrieved from The
Balance: https://www.thebalance.com/why-people-lose-money-in-the-market-4144737
Martin, R. (2014, August 28). Why the S&P 500 is a better gauge than the Dow. Retrieved from
Money Watch: https://www.cbsnews.com/news/dow-jones-industrial-average-sp-500-
which-is-better/
Montgomery, D. (2013(b)). Introduction to Linear Regression Analysis, Fifth Edition Set. New
York: Wiley.
Montgomery, D., Peck, E., & Vining, G. (2010). Introduction to Linear Regression Analysis.
New Yrok: Wiley-Interscience.
Poff, J., & Poff, R. (2013). Stock Market and Financial Investing. Amazon Digital Services.
Roberts, L. (2017, July 26). Opinion: If the stock market can make you rich, why are so many
Americans poor? Retrieved from Neustar: https://www.marketwatch.com/story/if-the-
stock-market-can-make-you-rich-why-are-so-many-americans-poor-2017-07-26
Smith, H., & Draper, N. (2012). Applied Regression Analysis. New Yrok: Wiley-Interscience.
1 out of 11
Related Documents

Your All-in-One AI-Powered Toolkit for Academic Success.
+13062052269
info@desklib.com
Available 24*7 on WhatsApp / Email
Unlock your academic potential
© 2024 | Zucol Services PVT LTD | All rights reserved.