Public Health: Statistical Analysis of Healthy Lifestyle Data Report
VerifiedAdded on 2022/12/23
|21
|4018
|78
Report
AI Summary
This report presents a statistical analysis of a healthy lifestyle intervention aimed at university students, focusing on improving health literacy and reducing weight. The study involved 81 participants, divided into intervention and control groups, with data collected on age, gender, height, smoking stat...
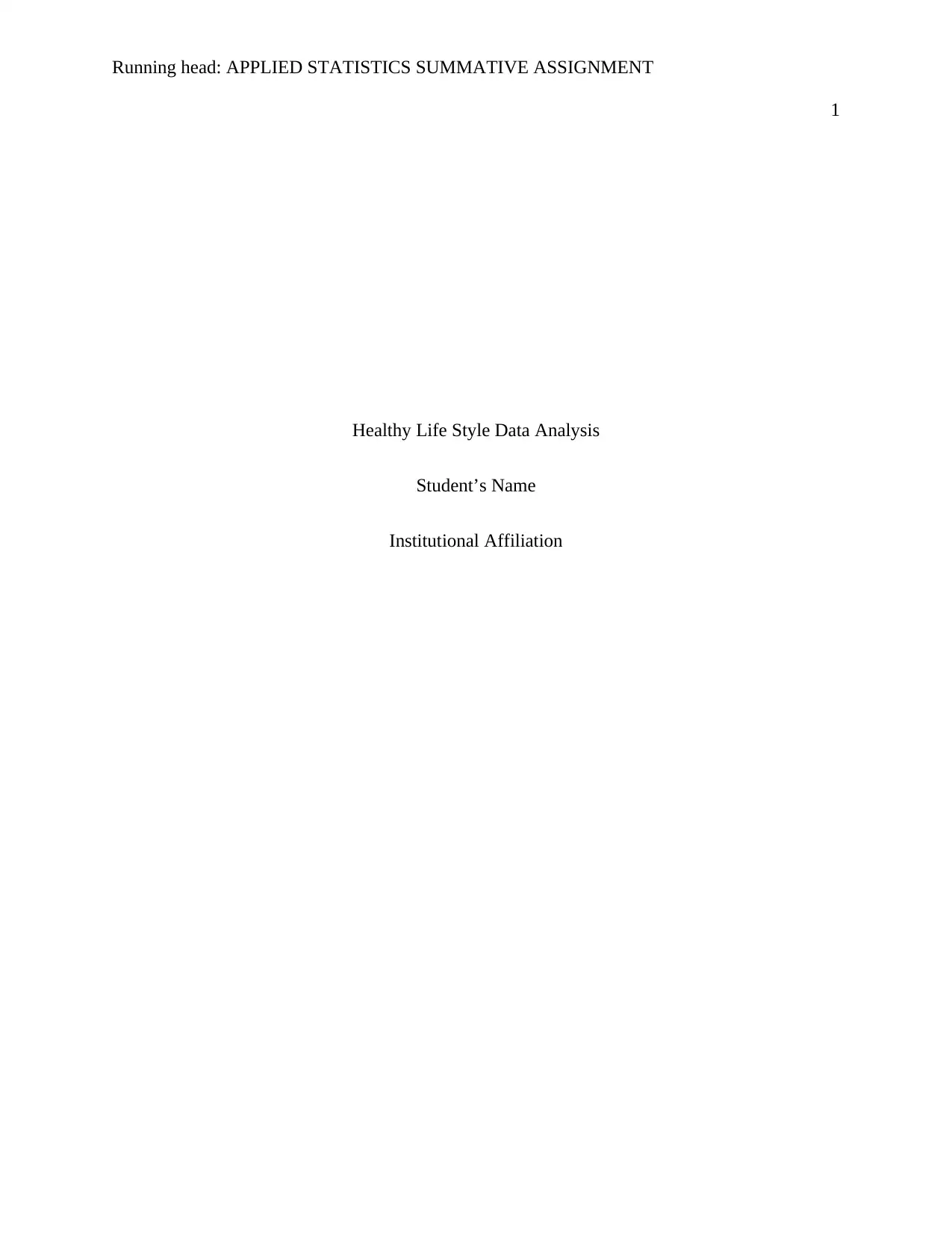
Running head: APPLIED STATISTICS SUMMATIVE ASSIGNMENT
1
Healthy Life Style Data Analysis
Student’s Name
Institutional Affiliation
1
Healthy Life Style Data Analysis
Student’s Name
Institutional Affiliation
Paraphrase This Document
Need a fresh take? Get an instant paraphrase of this document with our AI Paraphraser
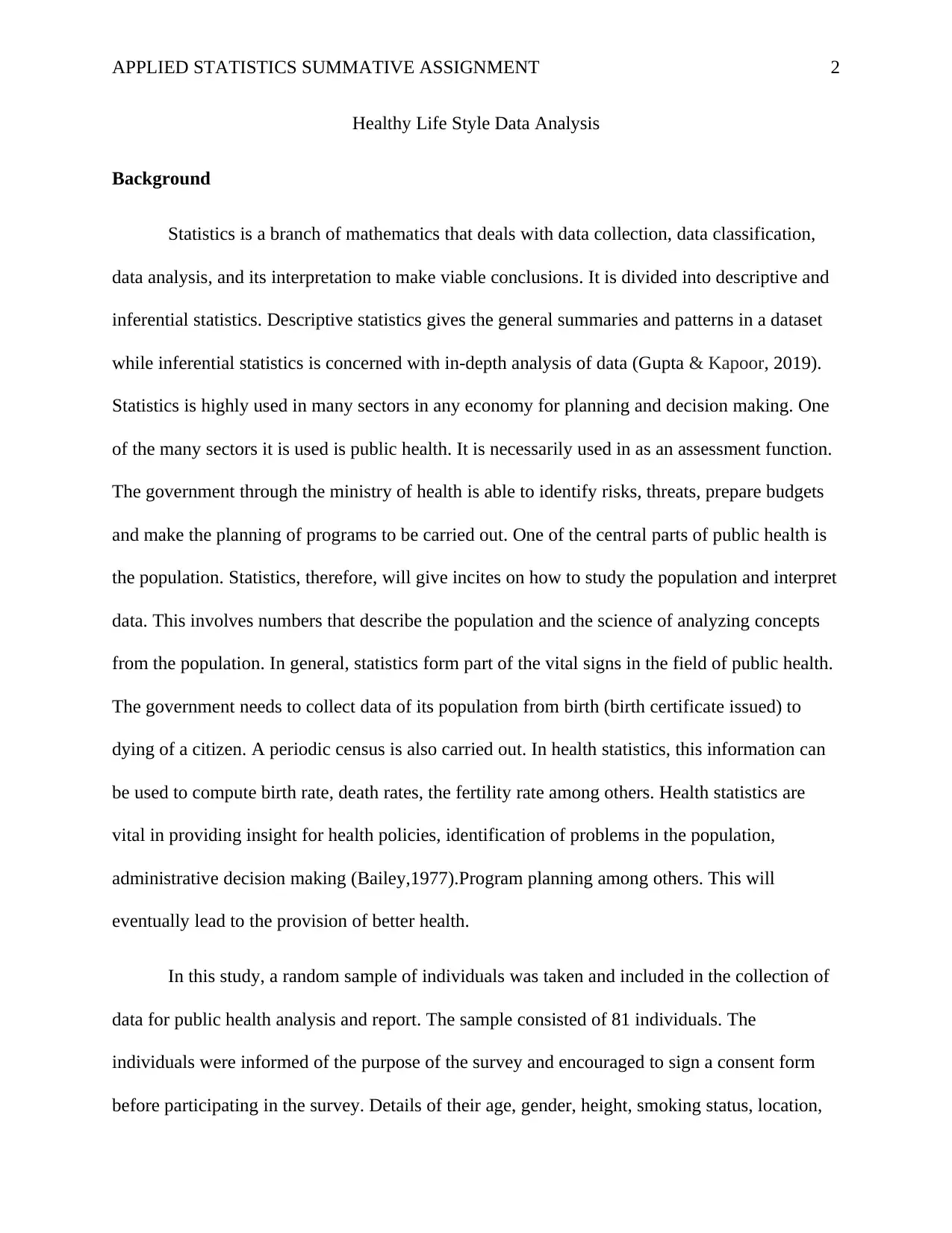
APPLIED STATISTICS SUMMATIVE ASSIGNMENT 2
Healthy Life Style Data Analysis
Background
Statistics is a branch of mathematics that deals with data collection, data classification,
data analysis, and its interpretation to make viable conclusions. It is divided into descriptive and
inferential statistics. Descriptive statistics gives the general summaries and patterns in a dataset
while inferential statistics is concerned with in-depth analysis of data (Gupta & Kapoor, 2019).
Statistics is highly used in many sectors in any economy for planning and decision making. One
of the many sectors it is used is public health. It is necessarily used in as an assessment function.
The government through the ministry of health is able to identify risks, threats, prepare budgets
and make the planning of programs to be carried out. One of the central parts of public health is
the population. Statistics, therefore, will give incites on how to study the population and interpret
data. This involves numbers that describe the population and the science of analyzing concepts
from the population. In general, statistics form part of the vital signs in the field of public health.
The government needs to collect data of its population from birth (birth certificate issued) to
dying of a citizen. A periodic census is also carried out. In health statistics, this information can
be used to compute birth rate, death rates, the fertility rate among others. Health statistics are
vital in providing insight for health policies, identification of problems in the population,
administrative decision making (Bailey,1977).Program planning among others. This will
eventually lead to the provision of better health.
In this study, a random sample of individuals was taken and included in the collection of
data for public health analysis and report. The sample consisted of 81 individuals. The
individuals were informed of the purpose of the survey and encouraged to sign a consent form
before participating in the survey. Details of their age, gender, height, smoking status, location,
Healthy Life Style Data Analysis
Background
Statistics is a branch of mathematics that deals with data collection, data classification,
data analysis, and its interpretation to make viable conclusions. It is divided into descriptive and
inferential statistics. Descriptive statistics gives the general summaries and patterns in a dataset
while inferential statistics is concerned with in-depth analysis of data (Gupta & Kapoor, 2019).
Statistics is highly used in many sectors in any economy for planning and decision making. One
of the many sectors it is used is public health. It is necessarily used in as an assessment function.
The government through the ministry of health is able to identify risks, threats, prepare budgets
and make the planning of programs to be carried out. One of the central parts of public health is
the population. Statistics, therefore, will give incites on how to study the population and interpret
data. This involves numbers that describe the population and the science of analyzing concepts
from the population. In general, statistics form part of the vital signs in the field of public health.
The government needs to collect data of its population from birth (birth certificate issued) to
dying of a citizen. A periodic census is also carried out. In health statistics, this information can
be used to compute birth rate, death rates, the fertility rate among others. Health statistics are
vital in providing insight for health policies, identification of problems in the population,
administrative decision making (Bailey,1977).Program planning among others. This will
eventually lead to the provision of better health.
In this study, a random sample of individuals was taken and included in the collection of
data for public health analysis and report. The sample consisted of 81 individuals. The
individuals were informed of the purpose of the survey and encouraged to sign a consent form
before participating in the survey. Details of their age, gender, height, smoking status, location,
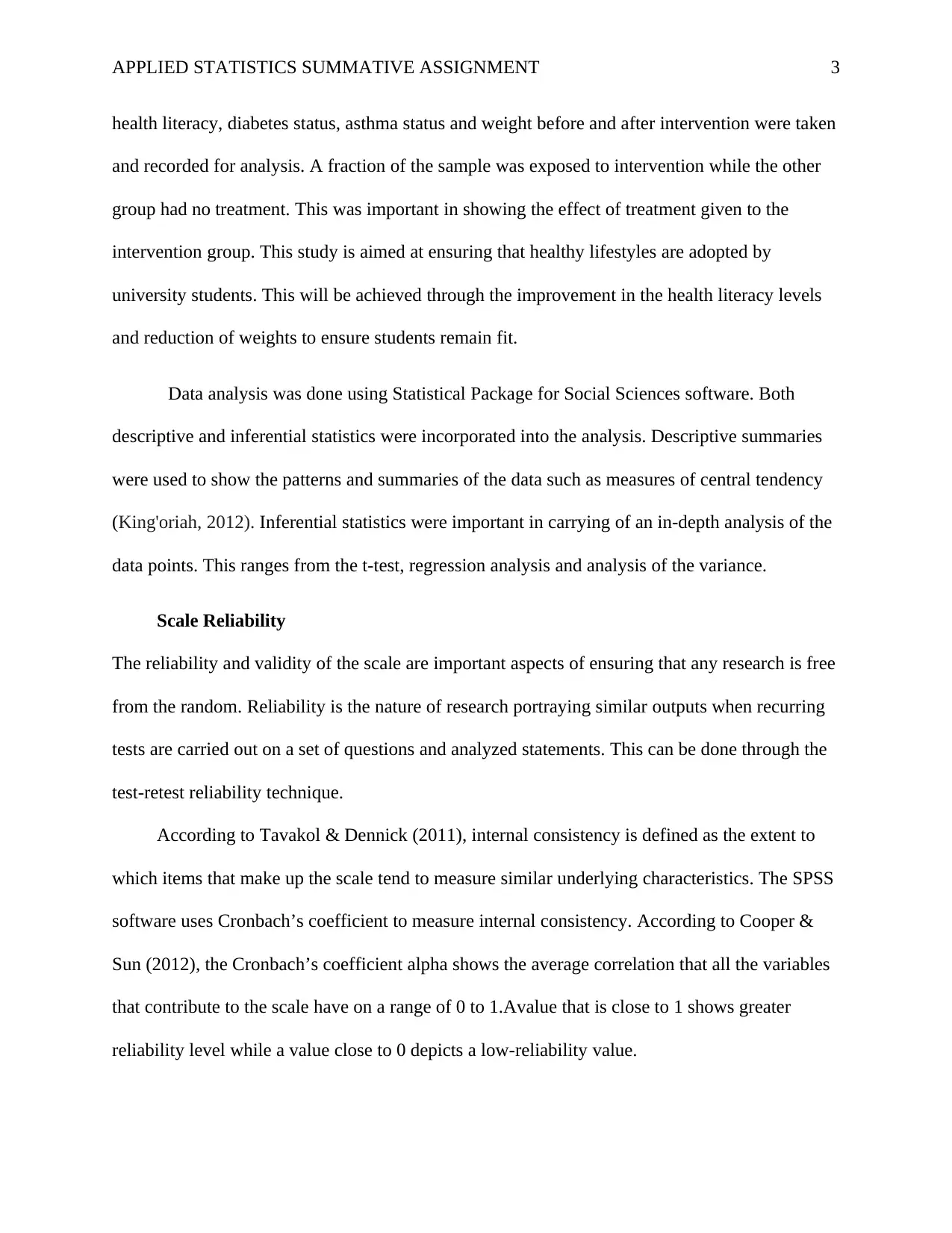
APPLIED STATISTICS SUMMATIVE ASSIGNMENT 3
health literacy, diabetes status, asthma status and weight before and after intervention were taken
and recorded for analysis. A fraction of the sample was exposed to intervention while the other
group had no treatment. This was important in showing the effect of treatment given to the
intervention group. This study is aimed at ensuring that healthy lifestyles are adopted by
university students. This will be achieved through the improvement in the health literacy levels
and reduction of weights to ensure students remain fit.
Data analysis was done using Statistical Package for Social Sciences software. Both
descriptive and inferential statistics were incorporated into the analysis. Descriptive summaries
were used to show the patterns and summaries of the data such as measures of central tendency
(King'oriah, 2012). Inferential statistics were important in carrying of an in-depth analysis of the
data points. This ranges from the t-test, regression analysis and analysis of the variance.
Scale Reliability
The reliability and validity of the scale are important aspects of ensuring that any research is free
from the random. Reliability is the nature of research portraying similar outputs when recurring
tests are carried out on a set of questions and analyzed statements. This can be done through the
test-retest reliability technique.
According to Tavakol & Dennick (2011), internal consistency is defined as the extent to
which items that make up the scale tend to measure similar underlying characteristics. The SPSS
software uses Cronbach’s coefficient to measure internal consistency. According to Cooper &
Sun (2012), the Cronbach’s coefficient alpha shows the average correlation that all the variables
that contribute to the scale have on a range of 0 to 1.Avalue that is close to 1 shows greater
reliability level while a value close to 0 depicts a low-reliability value.
health literacy, diabetes status, asthma status and weight before and after intervention were taken
and recorded for analysis. A fraction of the sample was exposed to intervention while the other
group had no treatment. This was important in showing the effect of treatment given to the
intervention group. This study is aimed at ensuring that healthy lifestyles are adopted by
university students. This will be achieved through the improvement in the health literacy levels
and reduction of weights to ensure students remain fit.
Data analysis was done using Statistical Package for Social Sciences software. Both
descriptive and inferential statistics were incorporated into the analysis. Descriptive summaries
were used to show the patterns and summaries of the data such as measures of central tendency
(King'oriah, 2012). Inferential statistics were important in carrying of an in-depth analysis of the
data points. This ranges from the t-test, regression analysis and analysis of the variance.
Scale Reliability
The reliability and validity of the scale are important aspects of ensuring that any research is free
from the random. Reliability is the nature of research portraying similar outputs when recurring
tests are carried out on a set of questions and analyzed statements. This can be done through the
test-retest reliability technique.
According to Tavakol & Dennick (2011), internal consistency is defined as the extent to
which items that make up the scale tend to measure similar underlying characteristics. The SPSS
software uses Cronbach’s coefficient to measure internal consistency. According to Cooper &
Sun (2012), the Cronbach’s coefficient alpha shows the average correlation that all the variables
that contribute to the scale have on a range of 0 to 1.Avalue that is close to 1 shows greater
reliability level while a value close to 0 depicts a low-reliability value.
You're viewing a preview
Unlock full access by subscribing today!
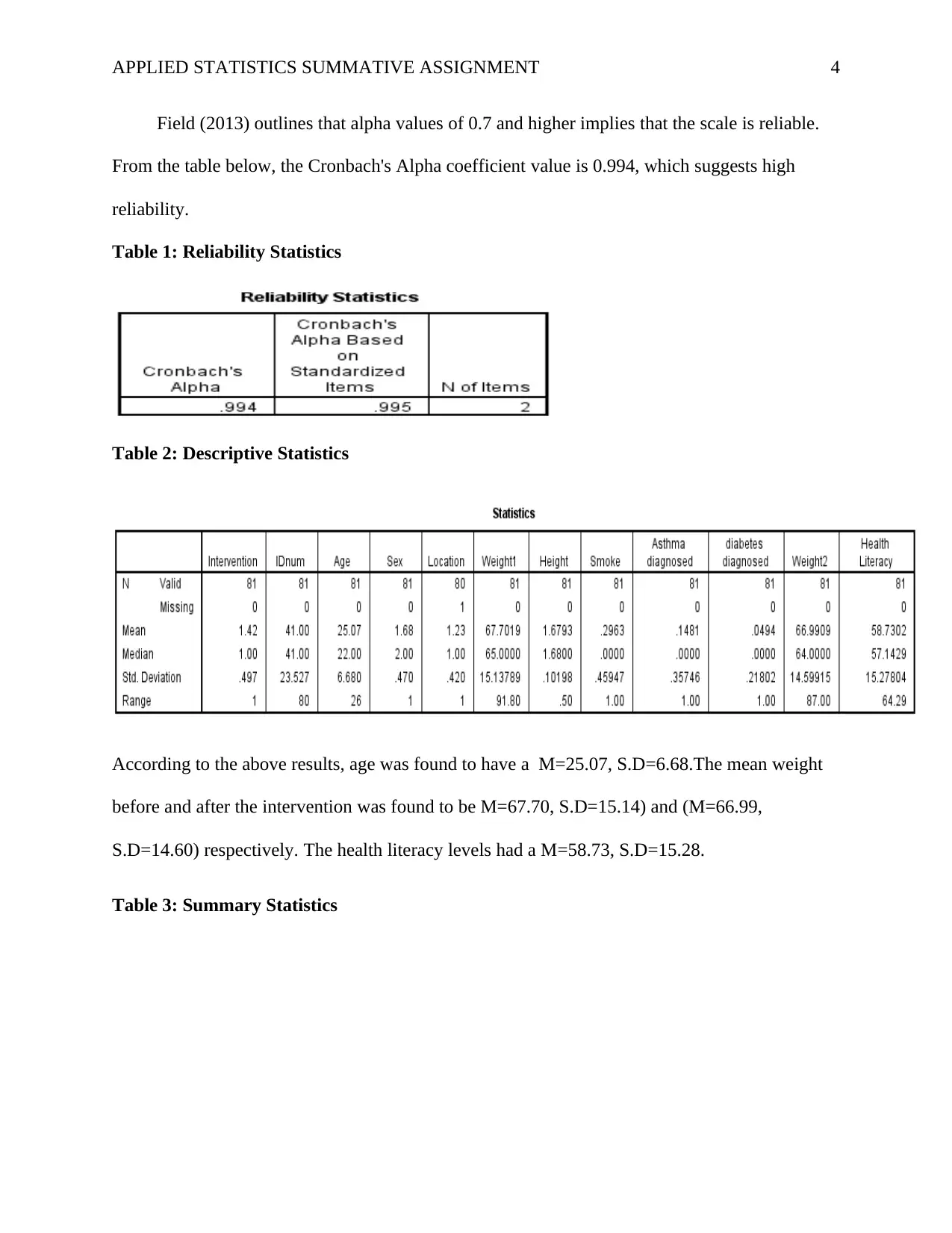
APPLIED STATISTICS SUMMATIVE ASSIGNMENT 4
Field (2013) outlines that alpha values of 0.7 and higher implies that the scale is reliable.
From the table below, the Cronbach's Alpha coefficient value is 0.994, which suggests high
reliability.
Table 1: Reliability Statistics
Table 2: Descriptive Statistics
According to the above results, age was found to have a M=25.07, S.D=6.68.The mean weight
before and after the intervention was found to be M=67.70, S.D=15.14) and (M=66.99,
S.D=14.60) respectively. The health literacy levels had a M=58.73, S.D=15.28.
Table 3: Summary Statistics
Field (2013) outlines that alpha values of 0.7 and higher implies that the scale is reliable.
From the table below, the Cronbach's Alpha coefficient value is 0.994, which suggests high
reliability.
Table 1: Reliability Statistics
Table 2: Descriptive Statistics
According to the above results, age was found to have a M=25.07, S.D=6.68.The mean weight
before and after the intervention was found to be M=67.70, S.D=15.14) and (M=66.99,
S.D=14.60) respectively. The health literacy levels had a M=58.73, S.D=15.28.
Table 3: Summary Statistics
Paraphrase This Document
Need a fresh take? Get an instant paraphrase of this document with our AI Paraphraser
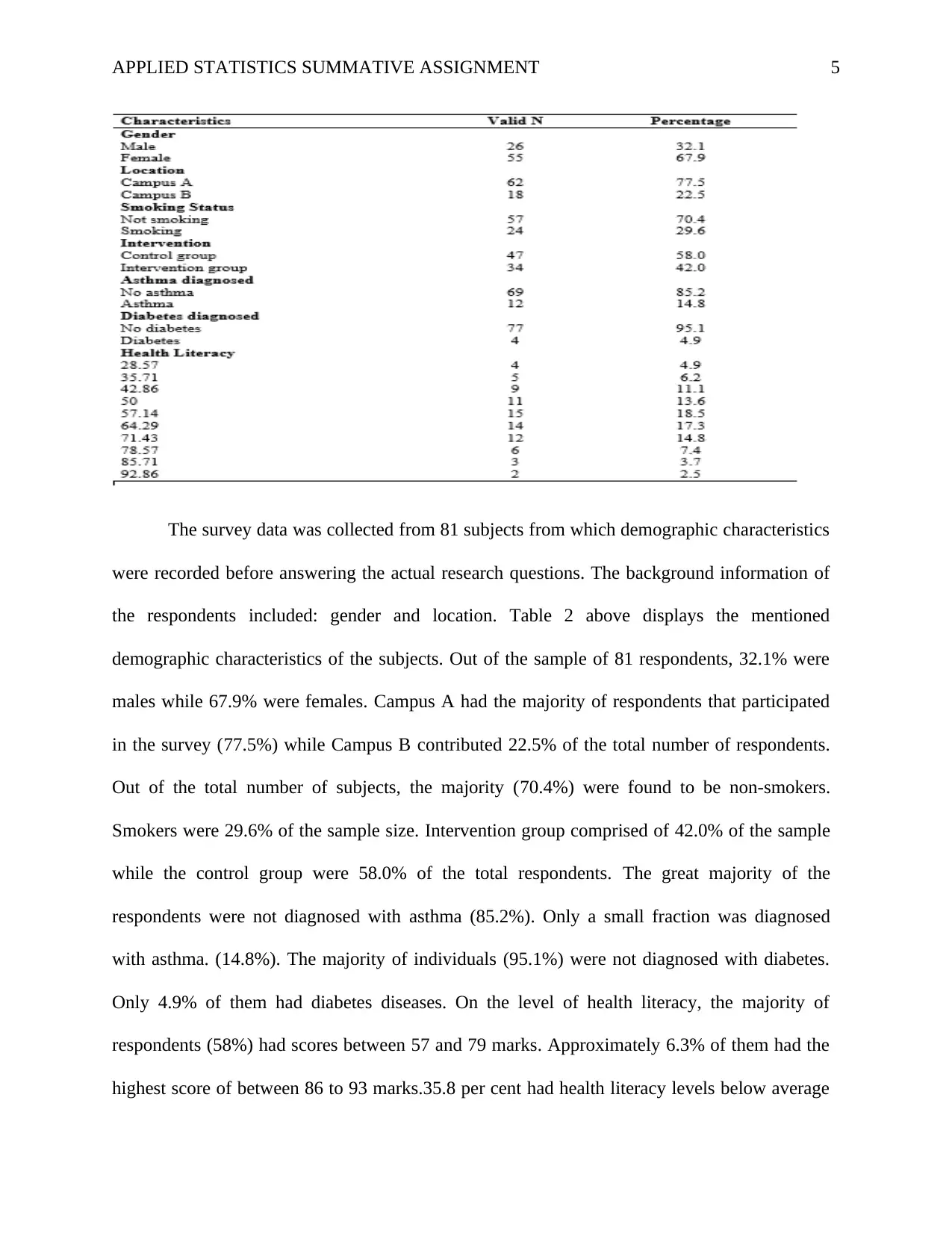
APPLIED STATISTICS SUMMATIVE ASSIGNMENT 5
The survey data was collected from 81 subjects from which demographic characteristics
were recorded before answering the actual research questions. The background information of
the respondents included: gender and location. Table 2 above displays the mentioned
demographic characteristics of the subjects. Out of the sample of 81 respondents, 32.1% were
males while 67.9% were females. Campus A had the majority of respondents that participated
in the survey (77.5%) while Campus B contributed 22.5% of the total number of respondents.
Out of the total number of subjects, the majority (70.4%) were found to be non-smokers.
Smokers were 29.6% of the sample size. Intervention group comprised of 42.0% of the sample
while the control group were 58.0% of the total respondents. The great majority of the
respondents were not diagnosed with asthma (85.2%). Only a small fraction was diagnosed
with asthma. (14.8%). The majority of individuals (95.1%) were not diagnosed with diabetes.
Only 4.9% of them had diabetes diseases. On the level of health literacy, the majority of
respondents (58%) had scores between 57 and 79 marks. Approximately 6.3% of them had the
highest score of between 86 to 93 marks.35.8 per cent had health literacy levels below average
The survey data was collected from 81 subjects from which demographic characteristics
were recorded before answering the actual research questions. The background information of
the respondents included: gender and location. Table 2 above displays the mentioned
demographic characteristics of the subjects. Out of the sample of 81 respondents, 32.1% were
males while 67.9% were females. Campus A had the majority of respondents that participated
in the survey (77.5%) while Campus B contributed 22.5% of the total number of respondents.
Out of the total number of subjects, the majority (70.4%) were found to be non-smokers.
Smokers were 29.6% of the sample size. Intervention group comprised of 42.0% of the sample
while the control group were 58.0% of the total respondents. The great majority of the
respondents were not diagnosed with asthma (85.2%). Only a small fraction was diagnosed
with asthma. (14.8%). The majority of individuals (95.1%) were not diagnosed with diabetes.
Only 4.9% of them had diabetes diseases. On the level of health literacy, the majority of
respondents (58%) had scores between 57 and 79 marks. Approximately 6.3% of them had the
highest score of between 86 to 93 marks.35.8 per cent had health literacy levels below average
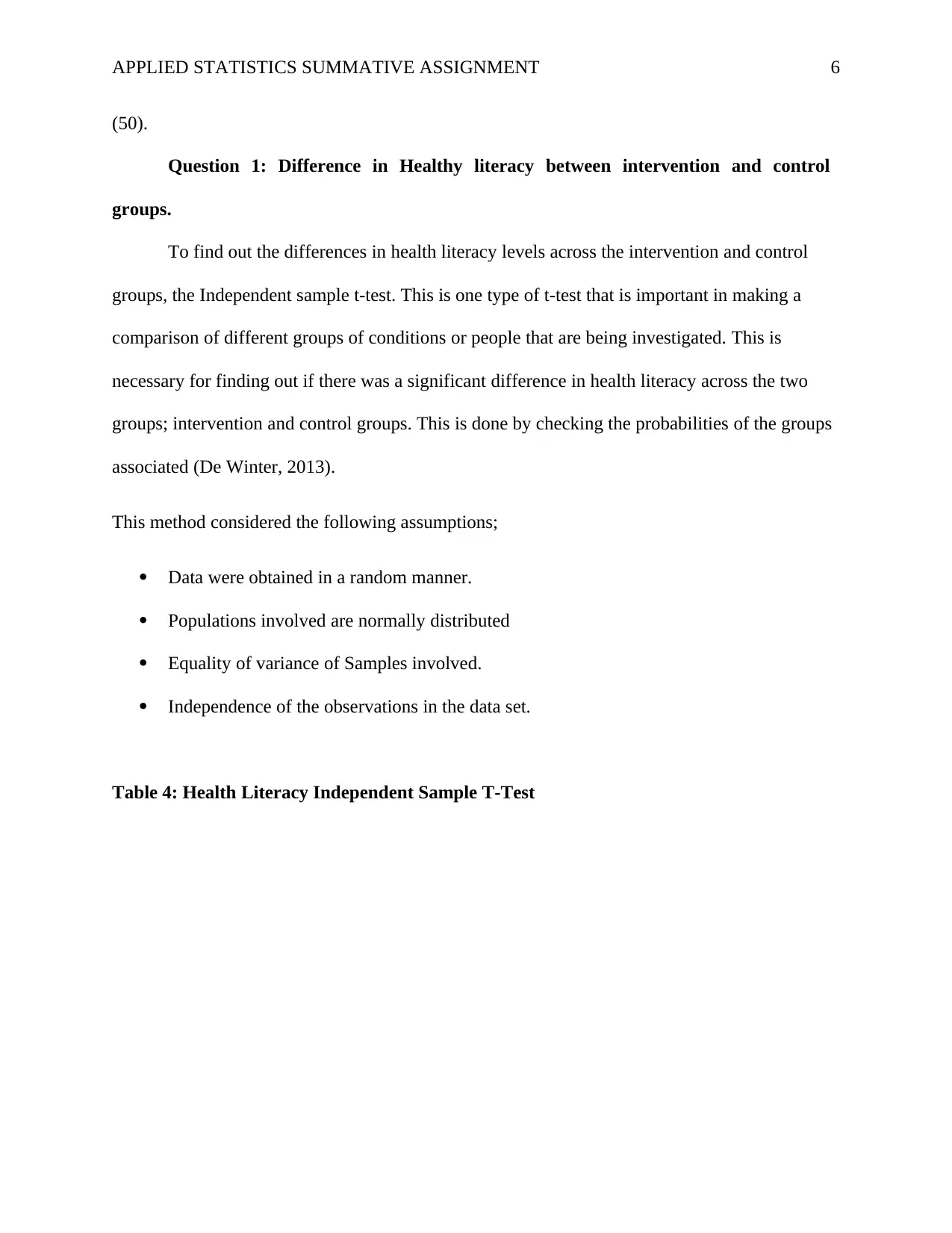
APPLIED STATISTICS SUMMATIVE ASSIGNMENT 6
(50).
Question 1: Difference in Healthy literacy between intervention and control
groups.
To find out the differences in health literacy levels across the intervention and control
groups, the Independent sample t-test. This is one type of t-test that is important in making a
comparison of different groups of conditions or people that are being investigated. This is
necessary for finding out if there was a significant difference in health literacy across the two
groups; intervention and control groups. This is done by checking the probabilities of the groups
associated (De Winter, 2013).
This method considered the following assumptions;
Data were obtained in a random manner.
Populations involved are normally distributed
Equality of variance of Samples involved.
Independence of the observations in the data set.
Table 4: Health Literacy Independent Sample T-Test
(50).
Question 1: Difference in Healthy literacy between intervention and control
groups.
To find out the differences in health literacy levels across the intervention and control
groups, the Independent sample t-test. This is one type of t-test that is important in making a
comparison of different groups of conditions or people that are being investigated. This is
necessary for finding out if there was a significant difference in health literacy across the two
groups; intervention and control groups. This is done by checking the probabilities of the groups
associated (De Winter, 2013).
This method considered the following assumptions;
Data were obtained in a random manner.
Populations involved are normally distributed
Equality of variance of Samples involved.
Independence of the observations in the data set.
Table 4: Health Literacy Independent Sample T-Test
You're viewing a preview
Unlock full access by subscribing today!
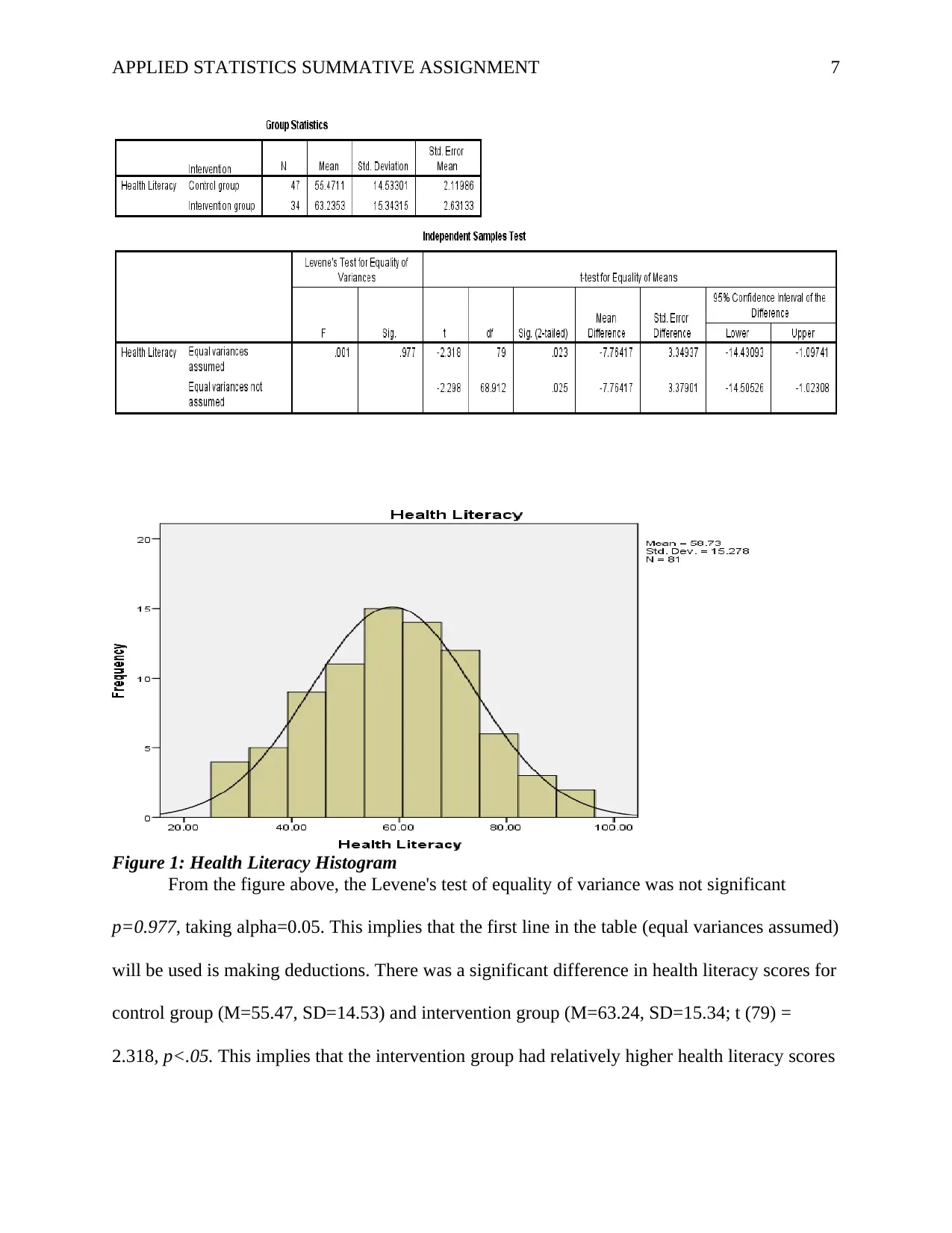
APPLIED STATISTICS SUMMATIVE ASSIGNMENT 7
Figure 1: Health Literacy Histogram
From the figure above, the Levene's test of equality of variance was not significant
p=0.977, taking alpha=0.05. This implies that the first line in the table (equal variances assumed)
will be used is making deductions. There was a significant difference in health literacy scores for
control group (M=55.47, SD=14.53) and intervention group (M=63.24, SD=15.34; t (79) =
2.318, p<.05. This implies that the intervention group had relatively higher health literacy scores
Figure 1: Health Literacy Histogram
From the figure above, the Levene's test of equality of variance was not significant
p=0.977, taking alpha=0.05. This implies that the first line in the table (equal variances assumed)
will be used is making deductions. There was a significant difference in health literacy scores for
control group (M=55.47, SD=14.53) and intervention group (M=63.24, SD=15.34; t (79) =
2.318, p<.05. This implies that the intervention group had relatively higher health literacy scores
Paraphrase This Document
Need a fresh take? Get an instant paraphrase of this document with our AI Paraphraser
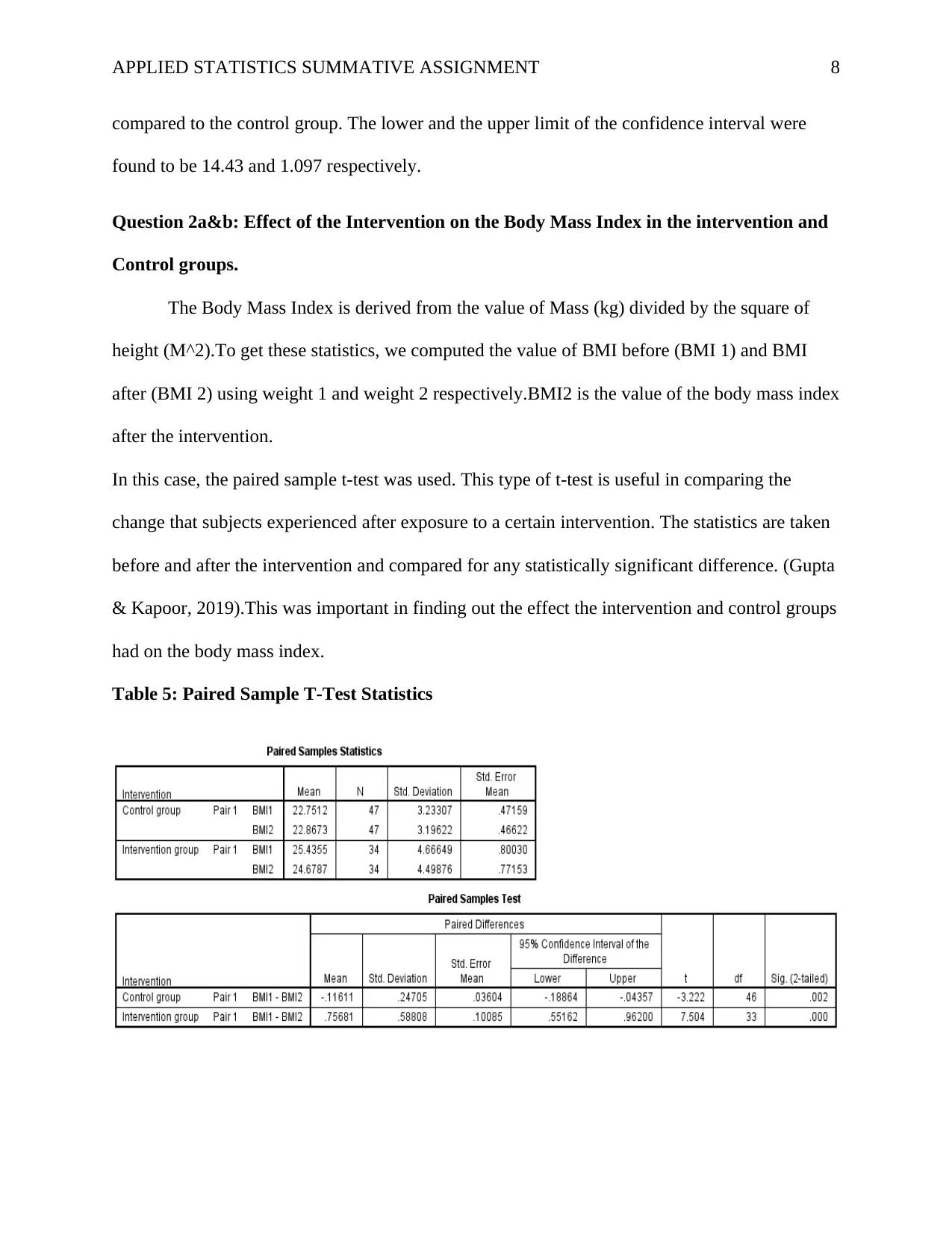
APPLIED STATISTICS SUMMATIVE ASSIGNMENT 8
compared to the control group. The lower and the upper limit of the confidence interval were
found to be 14.43 and 1.097 respectively.
Question 2a&b: Effect of the Intervention on the Body Mass Index in the intervention and
Control groups.
The Body Mass Index is derived from the value of Mass (kg) divided by the square of
height (M^2).To get these statistics, we computed the value of BMI before (BMI 1) and BMI
after (BMI 2) using weight 1 and weight 2 respectively.BMI2 is the value of the body mass index
after the intervention.
In this case, the paired sample t-test was used. This type of t-test is useful in comparing the
change that subjects experienced after exposure to a certain intervention. The statistics are taken
before and after the intervention and compared for any statistically significant difference. (Gupta
& Kapoor, 2019).This was important in finding out the effect the intervention and control groups
had on the body mass index.
Table 5: Paired Sample T-Test Statistics
compared to the control group. The lower and the upper limit of the confidence interval were
found to be 14.43 and 1.097 respectively.
Question 2a&b: Effect of the Intervention on the Body Mass Index in the intervention and
Control groups.
The Body Mass Index is derived from the value of Mass (kg) divided by the square of
height (M^2).To get these statistics, we computed the value of BMI before (BMI 1) and BMI
after (BMI 2) using weight 1 and weight 2 respectively.BMI2 is the value of the body mass index
after the intervention.
In this case, the paired sample t-test was used. This type of t-test is useful in comparing the
change that subjects experienced after exposure to a certain intervention. The statistics are taken
before and after the intervention and compared for any statistically significant difference. (Gupta
& Kapoor, 2019).This was important in finding out the effect the intervention and control groups
had on the body mass index.
Table 5: Paired Sample T-Test Statistics
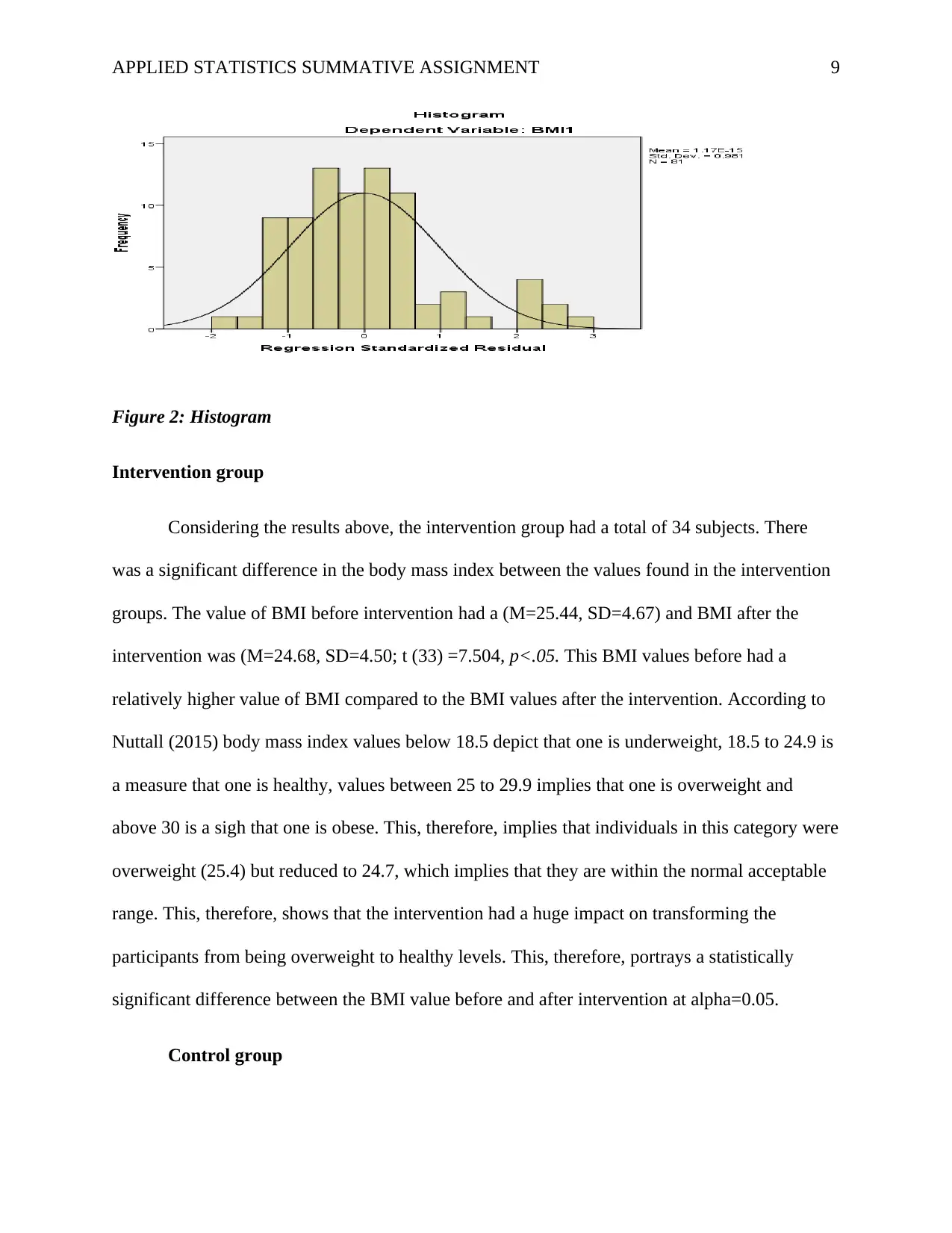
APPLIED STATISTICS SUMMATIVE ASSIGNMENT 9
Figure 2: Histogram
Intervention group
Considering the results above, the intervention group had a total of 34 subjects. There
was a significant difference in the body mass index between the values found in the intervention
groups. The value of BMI before intervention had a (M=25.44, SD=4.67) and BMI after the
intervention was (M=24.68, SD=4.50; t (33) =7.504, p<.05. This BMI values before had a
relatively higher value of BMI compared to the BMI values after the intervention. According to
Nuttall (2015) body mass index values below 18.5 depict that one is underweight, 18.5 to 24.9 is
a measure that one is healthy, values between 25 to 29.9 implies that one is overweight and
above 30 is a sigh that one is obese. This, therefore, implies that individuals in this category were
overweight (25.4) but reduced to 24.7, which implies that they are within the normal acceptable
range. This, therefore, shows that the intervention had a huge impact on transforming the
participants from being overweight to healthy levels. This, therefore, portrays a statistically
significant difference between the BMI value before and after intervention at alpha=0.05.
Control group
Figure 2: Histogram
Intervention group
Considering the results above, the intervention group had a total of 34 subjects. There
was a significant difference in the body mass index between the values found in the intervention
groups. The value of BMI before intervention had a (M=25.44, SD=4.67) and BMI after the
intervention was (M=24.68, SD=4.50; t (33) =7.504, p<.05. This BMI values before had a
relatively higher value of BMI compared to the BMI values after the intervention. According to
Nuttall (2015) body mass index values below 18.5 depict that one is underweight, 18.5 to 24.9 is
a measure that one is healthy, values between 25 to 29.9 implies that one is overweight and
above 30 is a sigh that one is obese. This, therefore, implies that individuals in this category were
overweight (25.4) but reduced to 24.7, which implies that they are within the normal acceptable
range. This, therefore, shows that the intervention had a huge impact on transforming the
participants from being overweight to healthy levels. This, therefore, portrays a statistically
significant difference between the BMI value before and after intervention at alpha=0.05.
Control group
You're viewing a preview
Unlock full access by subscribing today!
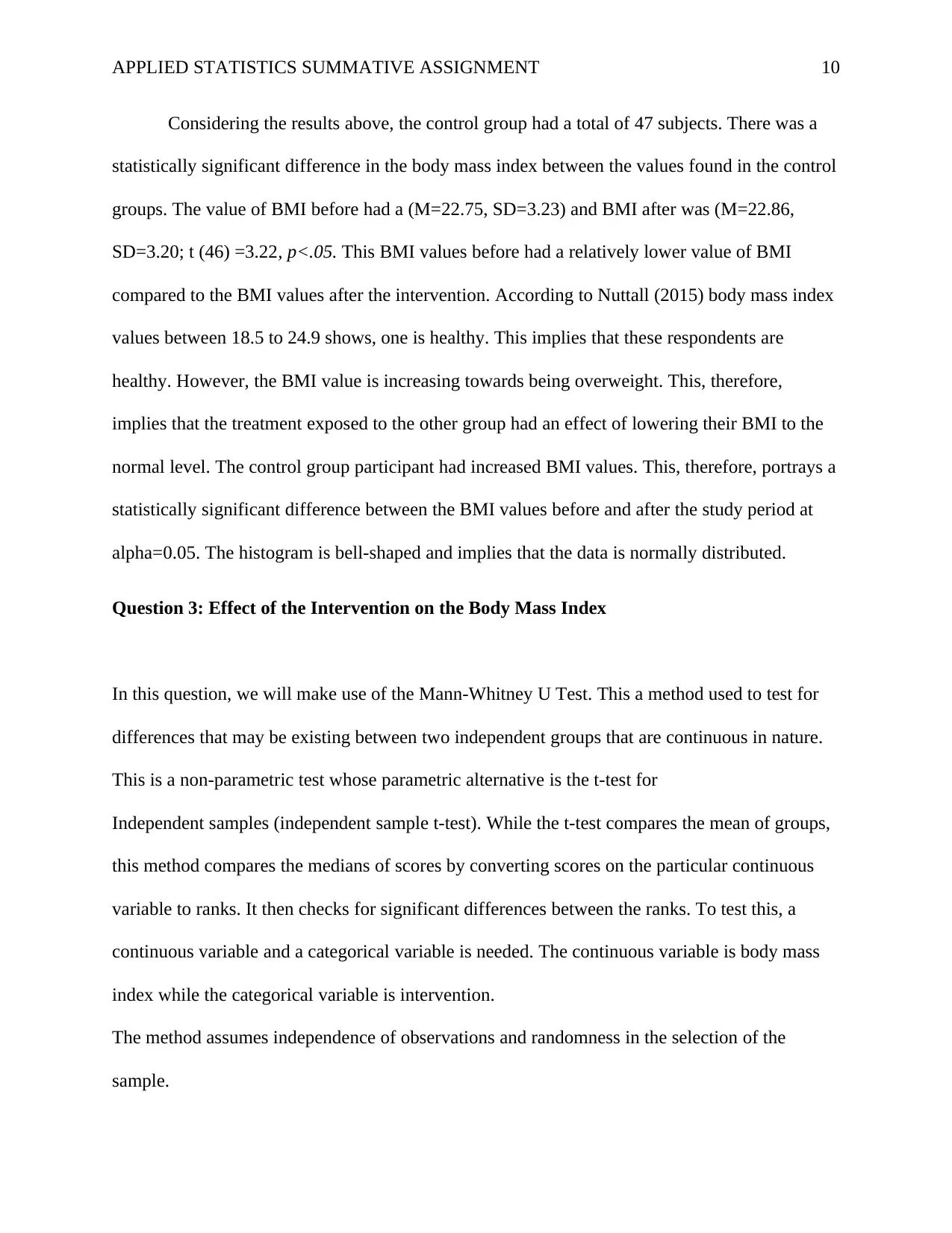
APPLIED STATISTICS SUMMATIVE ASSIGNMENT 10
Considering the results above, the control group had a total of 47 subjects. There was a
statistically significant difference in the body mass index between the values found in the control
groups. The value of BMI before had a (M=22.75, SD=3.23) and BMI after was (M=22.86,
SD=3.20; t (46) =3.22, p<.05. This BMI values before had a relatively lower value of BMI
compared to the BMI values after the intervention. According to Nuttall (2015) body mass index
values between 18.5 to 24.9 shows, one is healthy. This implies that these respondents are
healthy. However, the BMI value is increasing towards being overweight. This, therefore,
implies that the treatment exposed to the other group had an effect of lowering their BMI to the
normal level. The control group participant had increased BMI values. This, therefore, portrays a
statistically significant difference between the BMI values before and after the study period at
alpha=0.05. The histogram is bell-shaped and implies that the data is normally distributed.
Question 3: Effect of the Intervention on the Body Mass Index
In this question, we will make use of the Mann-Whitney U Test. This a method used to test for
differences that may be existing between two independent groups that are continuous in nature.
This is a non-parametric test whose parametric alternative is the t-test for
Independent samples (independent sample t-test). While the t-test compares the mean of groups,
this method compares the medians of scores by converting scores on the particular continuous
variable to ranks. It then checks for significant differences between the ranks. To test this, a
continuous variable and a categorical variable is needed. The continuous variable is body mass
index while the categorical variable is intervention.
The method assumes independence of observations and randomness in the selection of the
sample.
Considering the results above, the control group had a total of 47 subjects. There was a
statistically significant difference in the body mass index between the values found in the control
groups. The value of BMI before had a (M=22.75, SD=3.23) and BMI after was (M=22.86,
SD=3.20; t (46) =3.22, p<.05. This BMI values before had a relatively lower value of BMI
compared to the BMI values after the intervention. According to Nuttall (2015) body mass index
values between 18.5 to 24.9 shows, one is healthy. This implies that these respondents are
healthy. However, the BMI value is increasing towards being overweight. This, therefore,
implies that the treatment exposed to the other group had an effect of lowering their BMI to the
normal level. The control group participant had increased BMI values. This, therefore, portrays a
statistically significant difference between the BMI values before and after the study period at
alpha=0.05. The histogram is bell-shaped and implies that the data is normally distributed.
Question 3: Effect of the Intervention on the Body Mass Index
In this question, we will make use of the Mann-Whitney U Test. This a method used to test for
differences that may be existing between two independent groups that are continuous in nature.
This is a non-parametric test whose parametric alternative is the t-test for
Independent samples (independent sample t-test). While the t-test compares the mean of groups,
this method compares the medians of scores by converting scores on the particular continuous
variable to ranks. It then checks for significant differences between the ranks. To test this, a
continuous variable and a categorical variable is needed. The continuous variable is body mass
index while the categorical variable is intervention.
The method assumes independence of observations and randomness in the selection of the
sample.
Paraphrase This Document
Need a fresh take? Get an instant paraphrase of this document with our AI Paraphraser
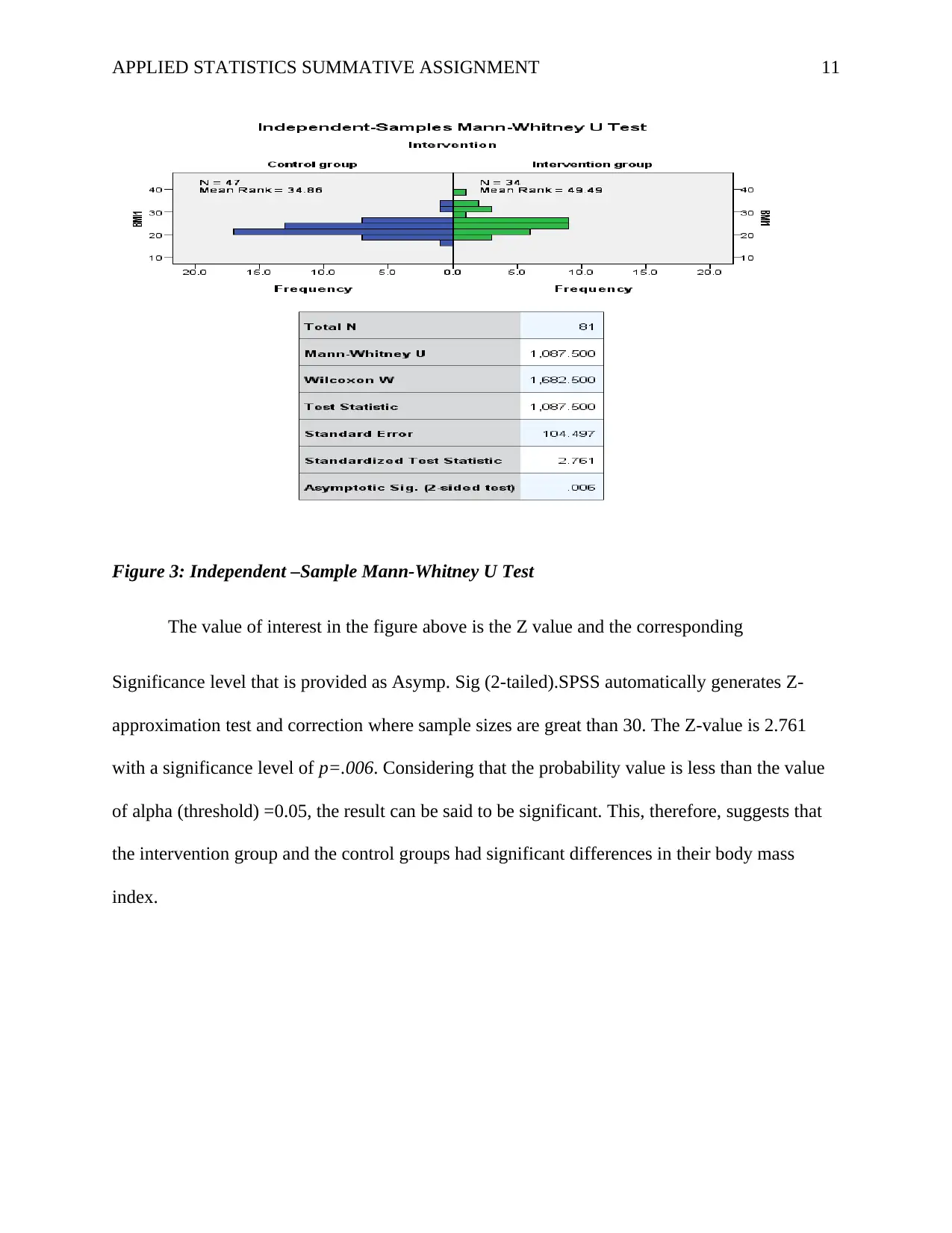
APPLIED STATISTICS SUMMATIVE ASSIGNMENT 11
Figure 3: Independent –Sample Mann-Whitney U Test
The value of interest in the figure above is the Z value and the corresponding
Significance level that is provided as Asymp. Sig (2-tailed).SPSS automatically generates Z-
approximation test and correction where sample sizes are great than 30. The Z-value is 2.761
with a significance level of p=.006. Considering that the probability value is less than the value
of alpha (threshold) =0.05, the result can be said to be significant. This, therefore, suggests that
the intervention group and the control groups had significant differences in their body mass
index.
Figure 3: Independent –Sample Mann-Whitney U Test
The value of interest in the figure above is the Z value and the corresponding
Significance level that is provided as Asymp. Sig (2-tailed).SPSS automatically generates Z-
approximation test and correction where sample sizes are great than 30. The Z-value is 2.761
with a significance level of p=.006. Considering that the probability value is less than the value
of alpha (threshold) =0.05, the result can be said to be significant. This, therefore, suggests that
the intervention group and the control groups had significant differences in their body mass
index.
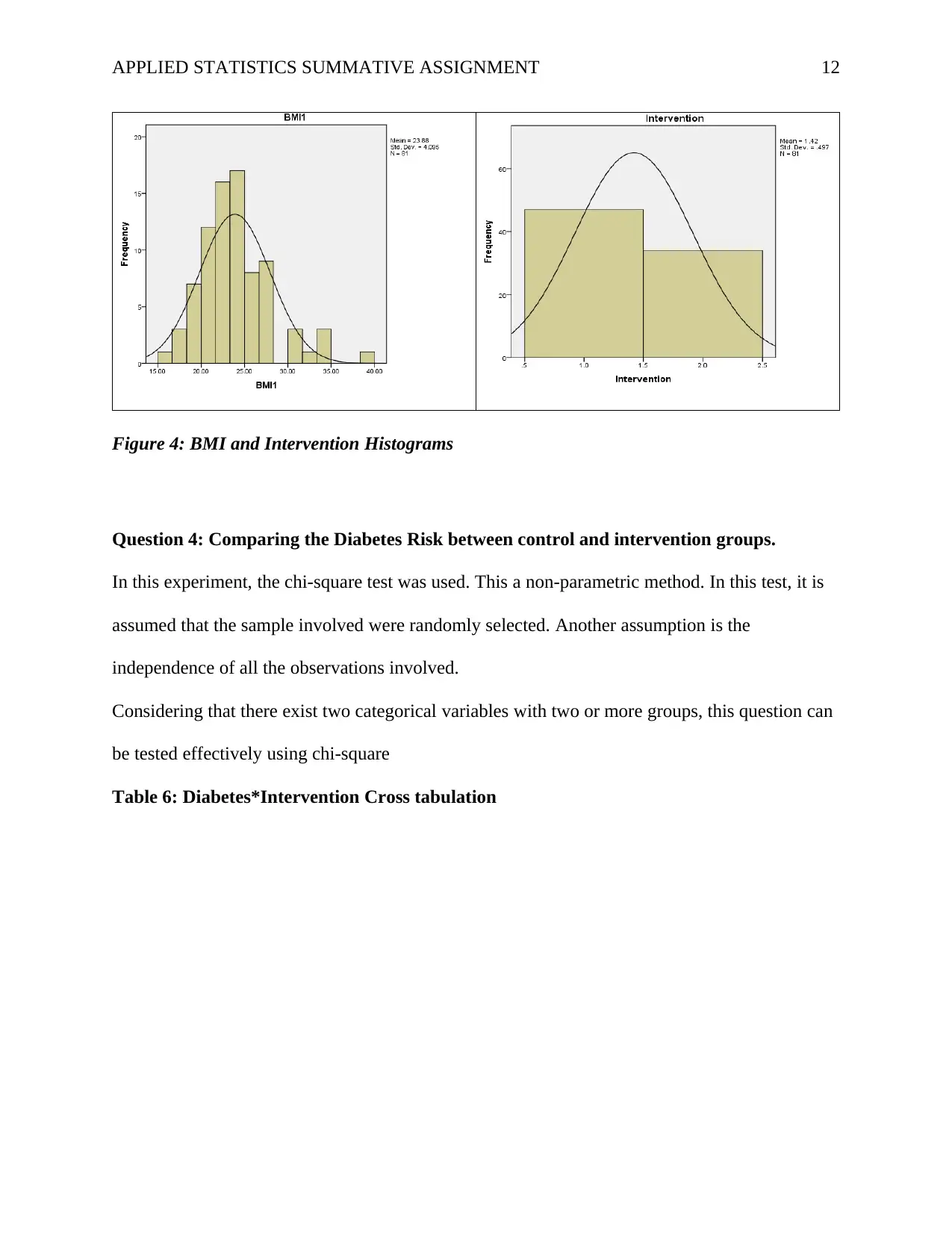
APPLIED STATISTICS SUMMATIVE ASSIGNMENT 12
Figure 4: BMI and Intervention Histograms
Question 4: Comparing the Diabetes Risk between control and intervention groups.
In this experiment, the chi-square test was used. This a non-parametric method. In this test, it is
assumed that the sample involved were randomly selected. Another assumption is the
independence of all the observations involved.
Considering that there exist two categorical variables with two or more groups, this question can
be tested effectively using chi-square
Table 6: Diabetes*Intervention Cross tabulation
Figure 4: BMI and Intervention Histograms
Question 4: Comparing the Diabetes Risk between control and intervention groups.
In this experiment, the chi-square test was used. This a non-parametric method. In this test, it is
assumed that the sample involved were randomly selected. Another assumption is the
independence of all the observations involved.
Considering that there exist two categorical variables with two or more groups, this question can
be tested effectively using chi-square
Table 6: Diabetes*Intervention Cross tabulation
You're viewing a preview
Unlock full access by subscribing today!
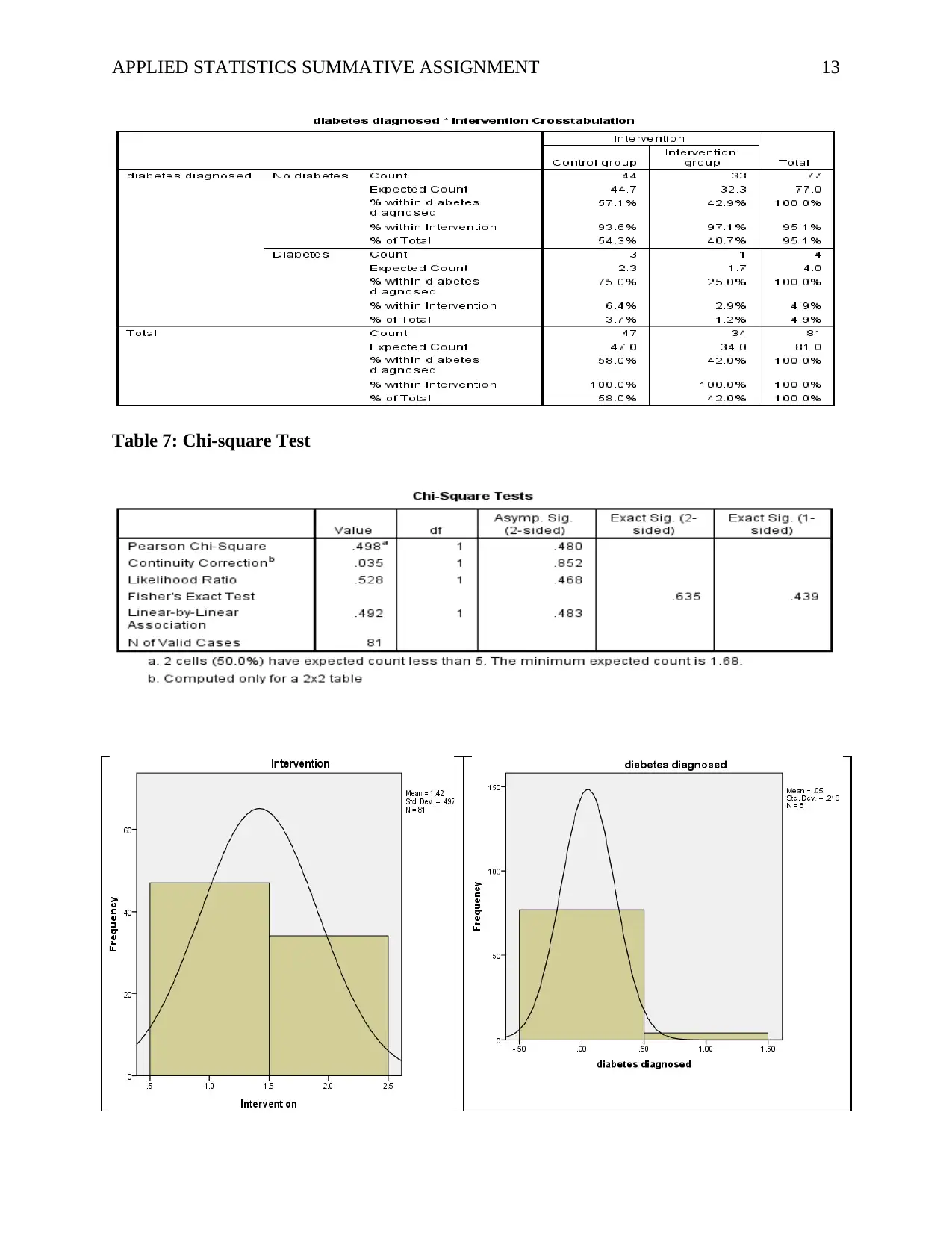
APPLIED STATISTICS SUMMATIVE ASSIGNMENT 13
Table 7: Chi-square Test
Table 7: Chi-square Test
Paraphrase This Document
Need a fresh take? Get an instant paraphrase of this document with our AI Paraphraser
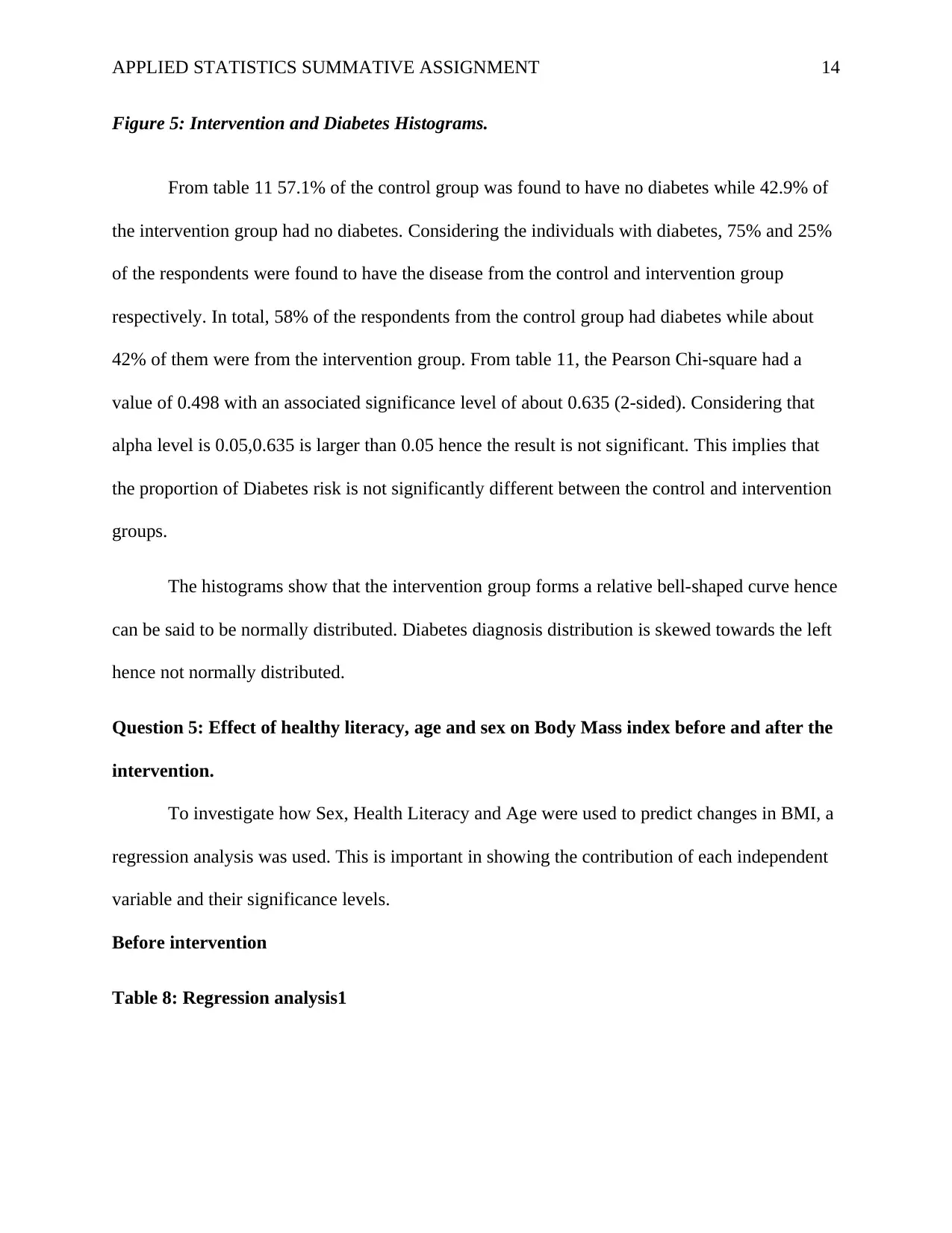
APPLIED STATISTICS SUMMATIVE ASSIGNMENT 14
Figure 5: Intervention and Diabetes Histograms.
From table 11 57.1% of the control group was found to have no diabetes while 42.9% of
the intervention group had no diabetes. Considering the individuals with diabetes, 75% and 25%
of the respondents were found to have the disease from the control and intervention group
respectively. In total, 58% of the respondents from the control group had diabetes while about
42% of them were from the intervention group. From table 11, the Pearson Chi-square had a
value of 0.498 with an associated significance level of about 0.635 (2-sided). Considering that
alpha level is 0.05,0.635 is larger than 0.05 hence the result is not significant. This implies that
the proportion of Diabetes risk is not significantly different between the control and intervention
groups.
The histograms show that the intervention group forms a relative bell-shaped curve hence
can be said to be normally distributed. Diabetes diagnosis distribution is skewed towards the left
hence not normally distributed.
Question 5: Effect of healthy literacy, age and sex on Body Mass index before and after the
intervention.
To investigate how Sex, Health Literacy and Age were used to predict changes in BMI, a
regression analysis was used. This is important in showing the contribution of each independent
variable and their significance levels.
Before intervention
Table 8: Regression analysis1
Figure 5: Intervention and Diabetes Histograms.
From table 11 57.1% of the control group was found to have no diabetes while 42.9% of
the intervention group had no diabetes. Considering the individuals with diabetes, 75% and 25%
of the respondents were found to have the disease from the control and intervention group
respectively. In total, 58% of the respondents from the control group had diabetes while about
42% of them were from the intervention group. From table 11, the Pearson Chi-square had a
value of 0.498 with an associated significance level of about 0.635 (2-sided). Considering that
alpha level is 0.05,0.635 is larger than 0.05 hence the result is not significant. This implies that
the proportion of Diabetes risk is not significantly different between the control and intervention
groups.
The histograms show that the intervention group forms a relative bell-shaped curve hence
can be said to be normally distributed. Diabetes diagnosis distribution is skewed towards the left
hence not normally distributed.
Question 5: Effect of healthy literacy, age and sex on Body Mass index before and after the
intervention.
To investigate how Sex, Health Literacy and Age were used to predict changes in BMI, a
regression analysis was used. This is important in showing the contribution of each independent
variable and their significance levels.
Before intervention
Table 8: Regression analysis1
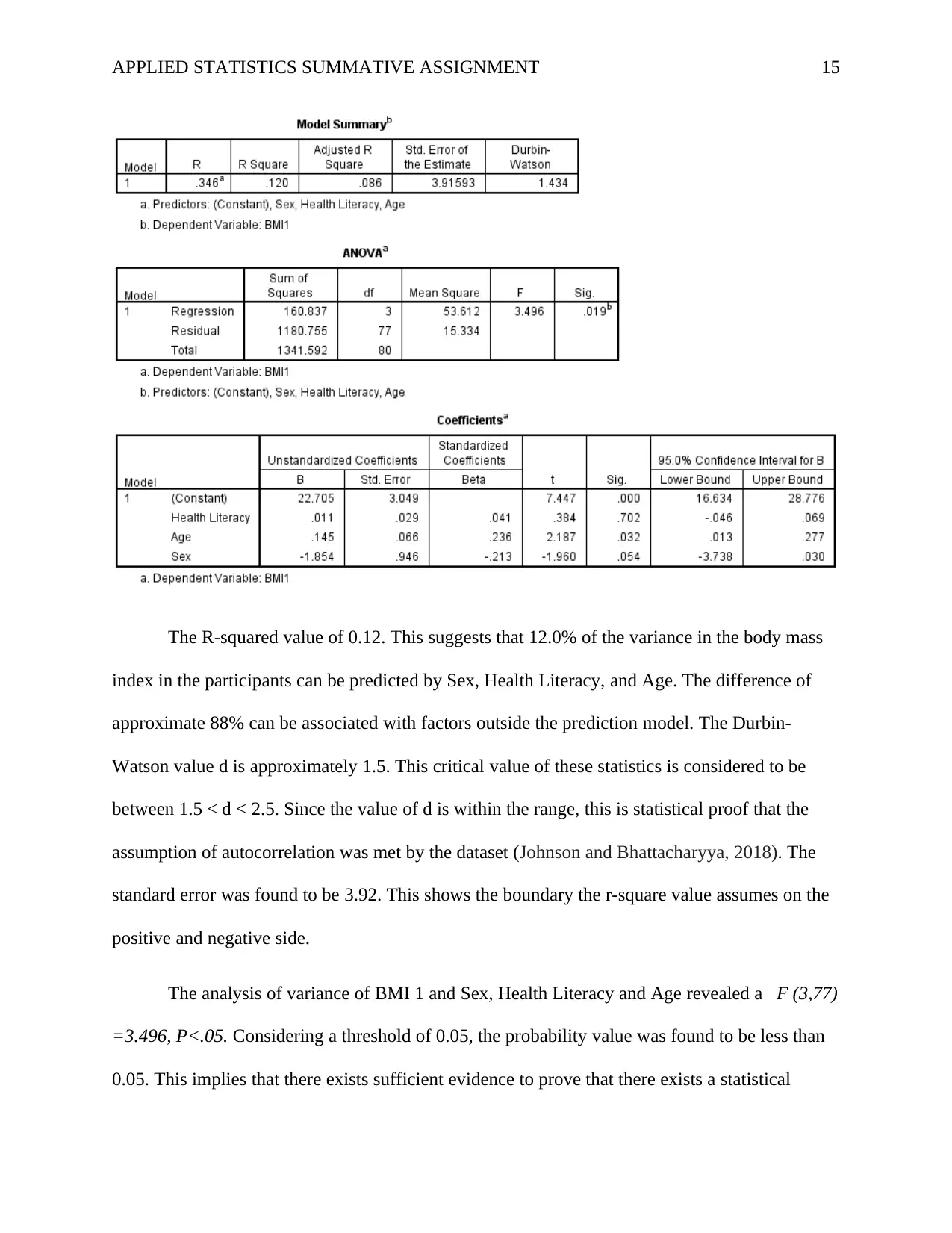
APPLIED STATISTICS SUMMATIVE ASSIGNMENT 15
The R-squared value of 0.12. This suggests that 12.0% of the variance in the body mass
index in the participants can be predicted by Sex, Health Literacy, and Age. The difference of
approximate 88% can be associated with factors outside the prediction model. The Durbin-
Watson value d is approximately 1.5. This critical value of these statistics is considered to be
between 1.5 < d < 2.5. Since the value of d is within the range, this is statistical proof that the
assumption of autocorrelation was met by the dataset (Johnson and Bhattacharyya, 2018). The
standard error was found to be 3.92. This shows the boundary the r-square value assumes on the
positive and negative side.
The analysis of variance of BMI 1 and Sex, Health Literacy and Age revealed a F (3,77)
=3.496, P<.05. Considering a threshold of 0.05, the probability value was found to be less than
0.05. This implies that there exists sufficient evidence to prove that there exists a statistical
The R-squared value of 0.12. This suggests that 12.0% of the variance in the body mass
index in the participants can be predicted by Sex, Health Literacy, and Age. The difference of
approximate 88% can be associated with factors outside the prediction model. The Durbin-
Watson value d is approximately 1.5. This critical value of these statistics is considered to be
between 1.5 < d < 2.5. Since the value of d is within the range, this is statistical proof that the
assumption of autocorrelation was met by the dataset (Johnson and Bhattacharyya, 2018). The
standard error was found to be 3.92. This shows the boundary the r-square value assumes on the
positive and negative side.
The analysis of variance of BMI 1 and Sex, Health Literacy and Age revealed a F (3,77)
=3.496, P<.05. Considering a threshold of 0.05, the probability value was found to be less than
0.05. This implies that there exists sufficient evidence to prove that there exists a statistical
You're viewing a preview
Unlock full access by subscribing today!
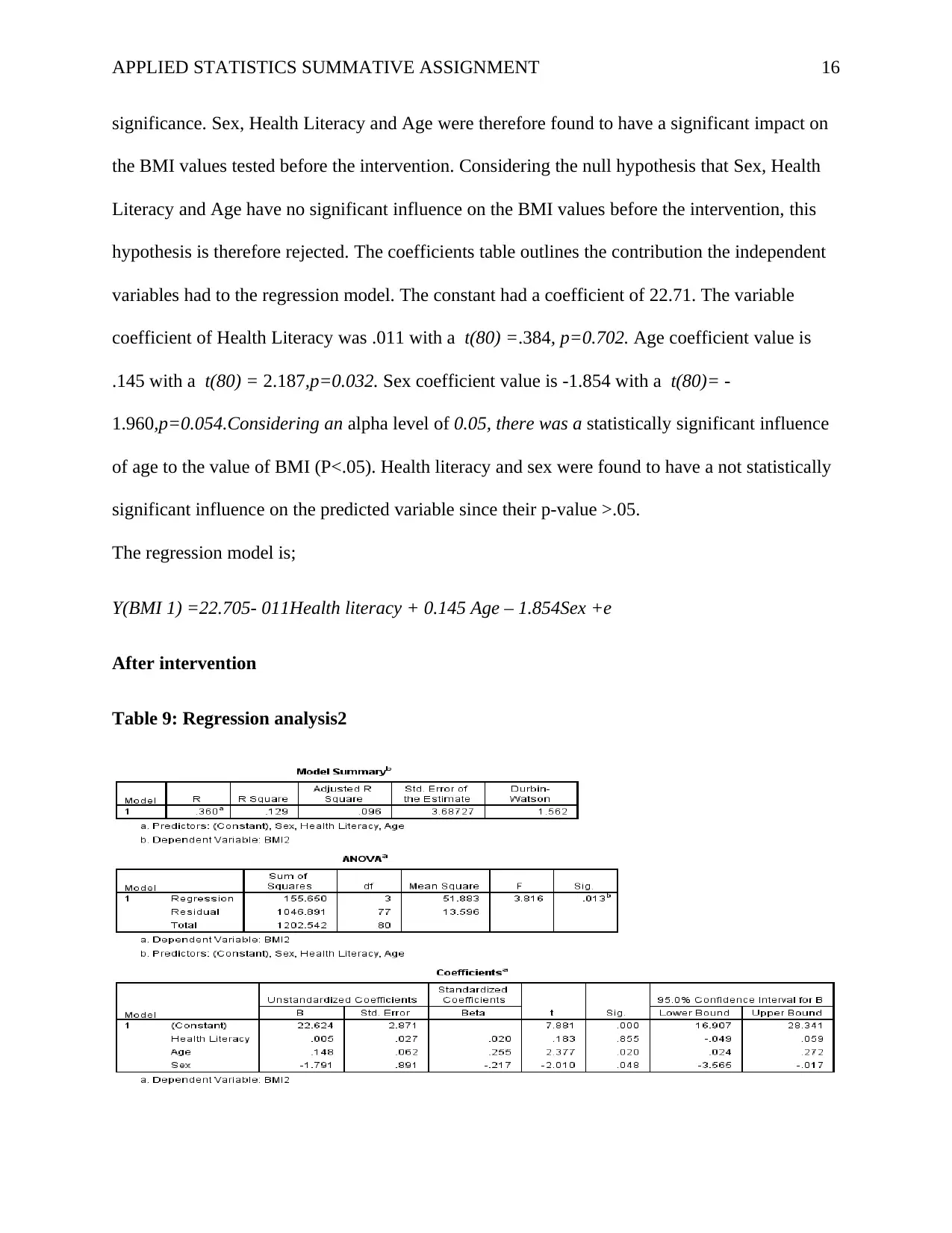
APPLIED STATISTICS SUMMATIVE ASSIGNMENT 16
significance. Sex, Health Literacy and Age were therefore found to have a significant impact on
the BMI values tested before the intervention. Considering the null hypothesis that Sex, Health
Literacy and Age have no significant influence on the BMI values before the intervention, this
hypothesis is therefore rejected. The coefficients table outlines the contribution the independent
variables had to the regression model. The constant had a coefficient of 22.71. The variable
coefficient of Health Literacy was .011 with a t(80) =.384, p=0.702. Age coefficient value is
.145 with a t(80) = 2.187,p=0.032. Sex coefficient value is -1.854 with a t(80)= -
1.960,p=0.054.Considering an alpha level of 0.05, there was a statistically significant influence
of age to the value of BMI (P<.05). Health literacy and sex were found to have a not statistically
significant influence on the predicted variable since their p-value >.05.
The regression model is;
Y(BMI 1) =22.705- 011Health literacy + 0.145 Age – 1.854Sex +e
After intervention
Table 9: Regression analysis2
significance. Sex, Health Literacy and Age were therefore found to have a significant impact on
the BMI values tested before the intervention. Considering the null hypothesis that Sex, Health
Literacy and Age have no significant influence on the BMI values before the intervention, this
hypothesis is therefore rejected. The coefficients table outlines the contribution the independent
variables had to the regression model. The constant had a coefficient of 22.71. The variable
coefficient of Health Literacy was .011 with a t(80) =.384, p=0.702. Age coefficient value is
.145 with a t(80) = 2.187,p=0.032. Sex coefficient value is -1.854 with a t(80)= -
1.960,p=0.054.Considering an alpha level of 0.05, there was a statistically significant influence
of age to the value of BMI (P<.05). Health literacy and sex were found to have a not statistically
significant influence on the predicted variable since their p-value >.05.
The regression model is;
Y(BMI 1) =22.705- 011Health literacy + 0.145 Age – 1.854Sex +e
After intervention
Table 9: Regression analysis2
Paraphrase This Document
Need a fresh take? Get an instant paraphrase of this document with our AI Paraphraser
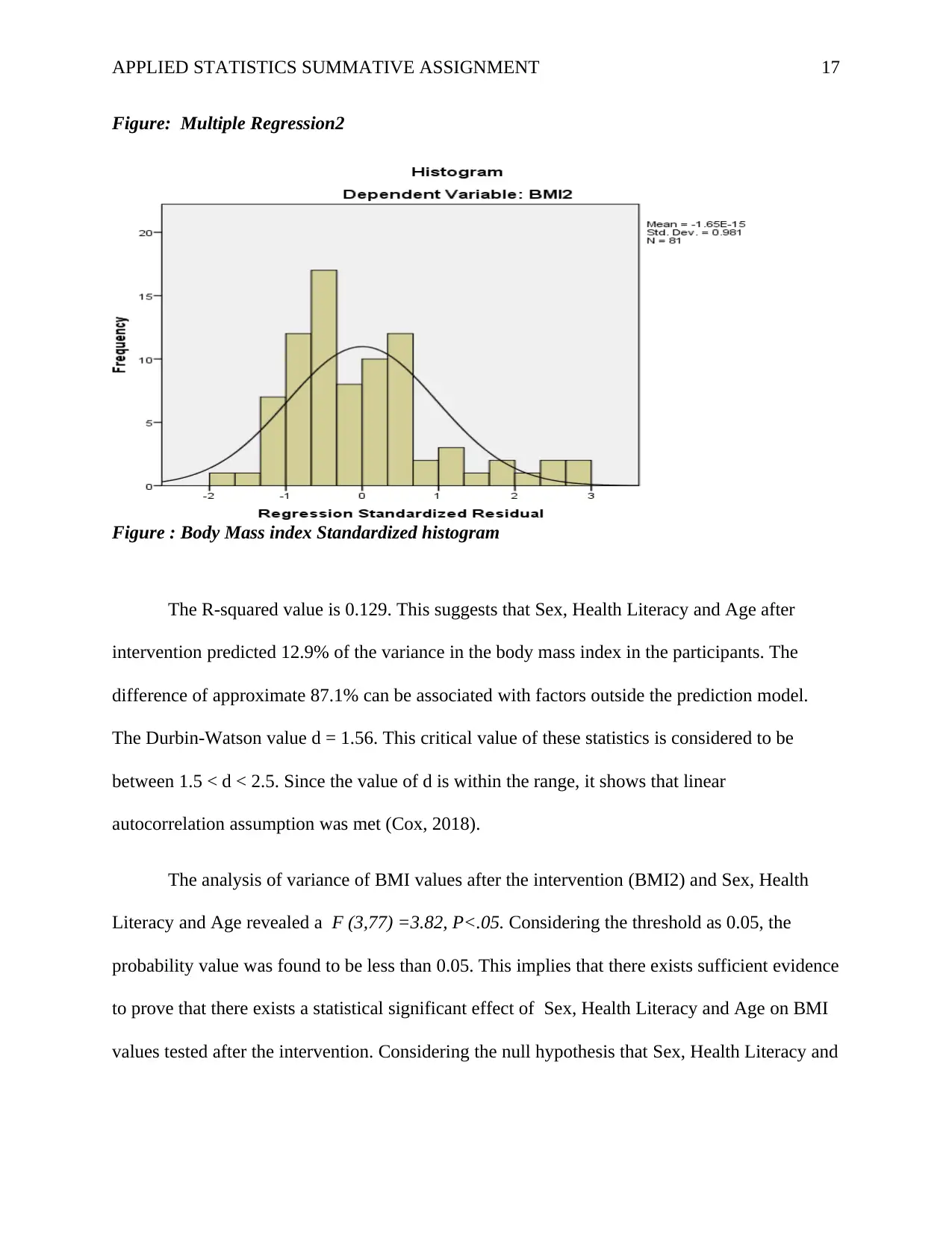
APPLIED STATISTICS SUMMATIVE ASSIGNMENT 17
Figure: Multiple Regression2
Figure : Body Mass index Standardized histogram
The R-squared value is 0.129. This suggests that Sex, Health Literacy and Age after
intervention predicted 12.9% of the variance in the body mass index in the participants. The
difference of approximate 87.1% can be associated with factors outside the prediction model.
The Durbin-Watson value d = 1.56. This critical value of these statistics is considered to be
between 1.5 < d < 2.5. Since the value of d is within the range, it shows that linear
autocorrelation assumption was met (Cox, 2018).
The analysis of variance of BMI values after the intervention (BMI2) and Sex, Health
Literacy and Age revealed a F (3,77) =3.82, P<.05. Considering the threshold as 0.05, the
probability value was found to be less than 0.05. This implies that there exists sufficient evidence
to prove that there exists a statistical significant effect of Sex, Health Literacy and Age on BMI
values tested after the intervention. Considering the null hypothesis that Sex, Health Literacy and
Figure: Multiple Regression2
Figure : Body Mass index Standardized histogram
The R-squared value is 0.129. This suggests that Sex, Health Literacy and Age after
intervention predicted 12.9% of the variance in the body mass index in the participants. The
difference of approximate 87.1% can be associated with factors outside the prediction model.
The Durbin-Watson value d = 1.56. This critical value of these statistics is considered to be
between 1.5 < d < 2.5. Since the value of d is within the range, it shows that linear
autocorrelation assumption was met (Cox, 2018).
The analysis of variance of BMI values after the intervention (BMI2) and Sex, Health
Literacy and Age revealed a F (3,77) =3.82, P<.05. Considering the threshold as 0.05, the
probability value was found to be less than 0.05. This implies that there exists sufficient evidence
to prove that there exists a statistical significant effect of Sex, Health Literacy and Age on BMI
values tested after the intervention. Considering the null hypothesis that Sex, Health Literacy and
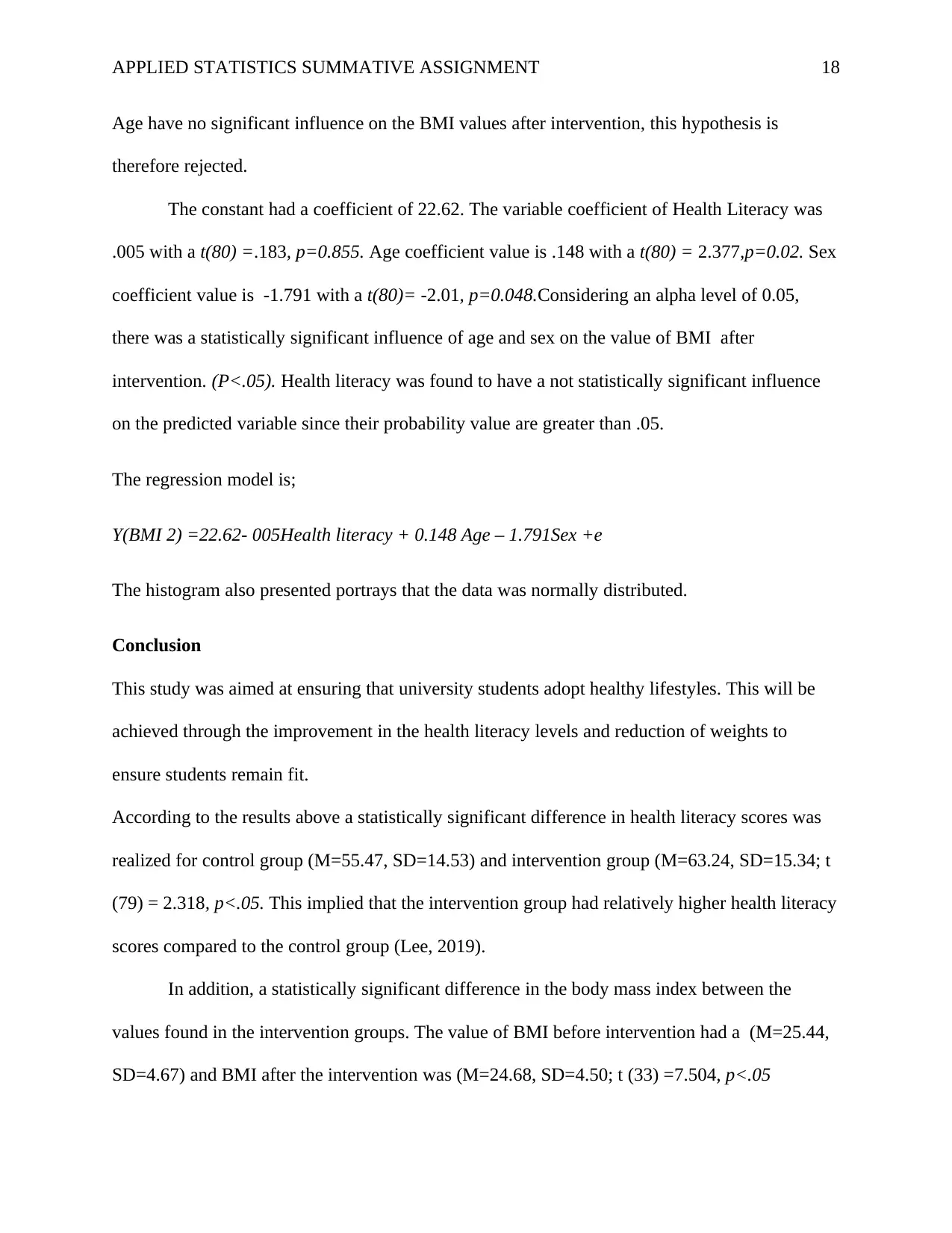
APPLIED STATISTICS SUMMATIVE ASSIGNMENT 18
Age have no significant influence on the BMI values after intervention, this hypothesis is
therefore rejected.
The constant had a coefficient of 22.62. The variable coefficient of Health Literacy was
.005 with a t(80) =.183, p=0.855. Age coefficient value is .148 with a t(80) = 2.377,p=0.02. Sex
coefficient value is -1.791 with a t(80)= -2.01, p=0.048.Considering an alpha level of 0.05,
there was a statistically significant influence of age and sex on the value of BMI after
intervention. (P<.05). Health literacy was found to have a not statistically significant influence
on the predicted variable since their probability value are greater than .05.
The regression model is;
Y(BMI 2) =22.62- 005Health literacy + 0.148 Age – 1.791Sex +e
The histogram also presented portrays that the data was normally distributed.
Conclusion
This study was aimed at ensuring that university students adopt healthy lifestyles. This will be
achieved through the improvement in the health literacy levels and reduction of weights to
ensure students remain fit.
According to the results above a statistically significant difference in health literacy scores was
realized for control group (M=55.47, SD=14.53) and intervention group (M=63.24, SD=15.34; t
(79) = 2.318, p<.05. This implied that the intervention group had relatively higher health literacy
scores compared to the control group (Lee, 2019).
In addition, a statistically significant difference in the body mass index between the
values found in the intervention groups. The value of BMI before intervention had a (M=25.44,
SD=4.67) and BMI after the intervention was (M=24.68, SD=4.50; t (33) =7.504, p<.05
Age have no significant influence on the BMI values after intervention, this hypothesis is
therefore rejected.
The constant had a coefficient of 22.62. The variable coefficient of Health Literacy was
.005 with a t(80) =.183, p=0.855. Age coefficient value is .148 with a t(80) = 2.377,p=0.02. Sex
coefficient value is -1.791 with a t(80)= -2.01, p=0.048.Considering an alpha level of 0.05,
there was a statistically significant influence of age and sex on the value of BMI after
intervention. (P<.05). Health literacy was found to have a not statistically significant influence
on the predicted variable since their probability value are greater than .05.
The regression model is;
Y(BMI 2) =22.62- 005Health literacy + 0.148 Age – 1.791Sex +e
The histogram also presented portrays that the data was normally distributed.
Conclusion
This study was aimed at ensuring that university students adopt healthy lifestyles. This will be
achieved through the improvement in the health literacy levels and reduction of weights to
ensure students remain fit.
According to the results above a statistically significant difference in health literacy scores was
realized for control group (M=55.47, SD=14.53) and intervention group (M=63.24, SD=15.34; t
(79) = 2.318, p<.05. This implied that the intervention group had relatively higher health literacy
scores compared to the control group (Lee, 2019).
In addition, a statistically significant difference in the body mass index between the
values found in the intervention groups. The value of BMI before intervention had a (M=25.44,
SD=4.67) and BMI after the intervention was (M=24.68, SD=4.50; t (33) =7.504, p<.05
You're viewing a preview
Unlock full access by subscribing today!
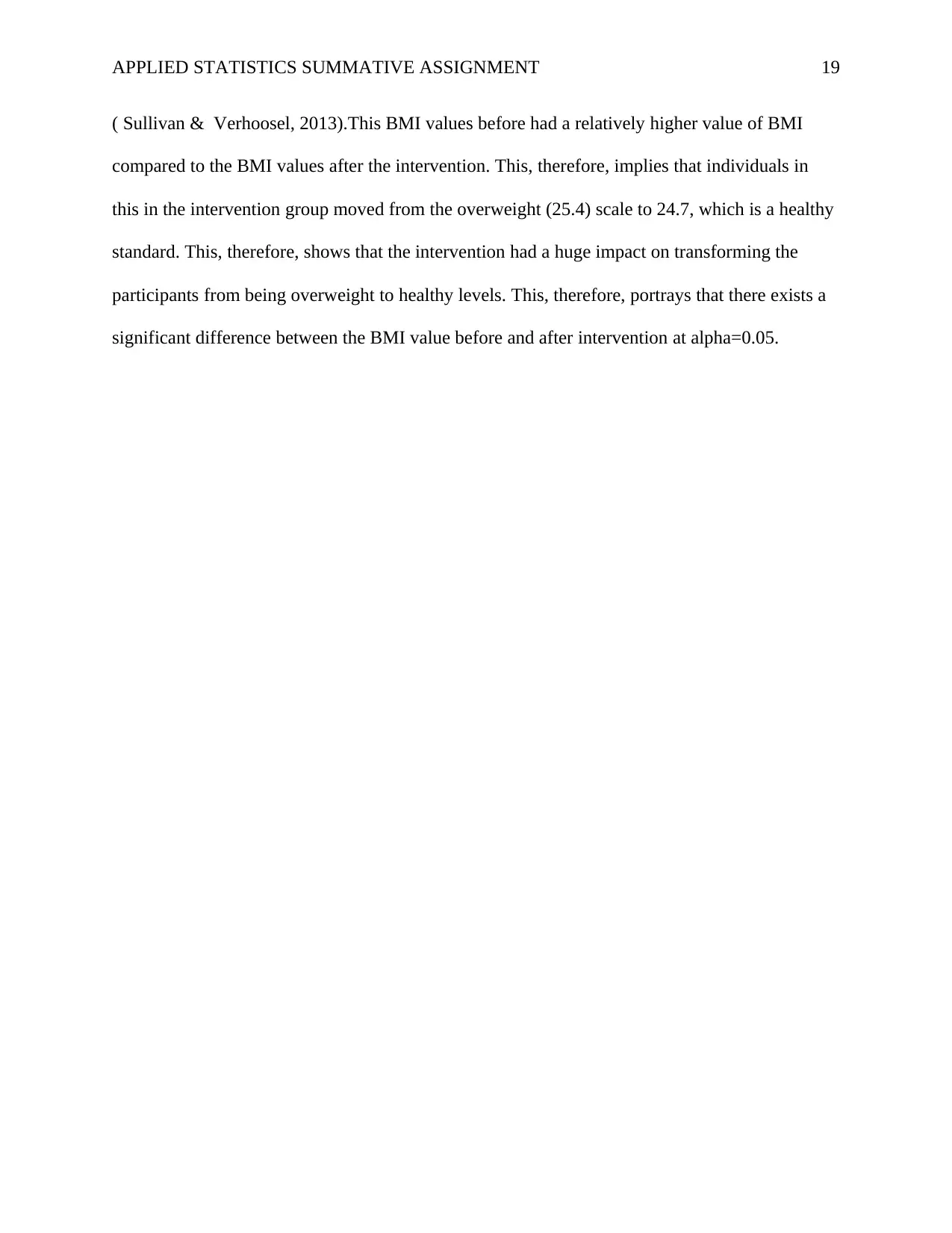
APPLIED STATISTICS SUMMATIVE ASSIGNMENT 19
( Sullivan & Verhoosel, 2013).This BMI values before had a relatively higher value of BMI
compared to the BMI values after the intervention. This, therefore, implies that individuals in
this in the intervention group moved from the overweight (25.4) scale to 24.7, which is a healthy
standard. This, therefore, shows that the intervention had a huge impact on transforming the
participants from being overweight to healthy levels. This, therefore, portrays that there exists a
significant difference between the BMI value before and after intervention at alpha=0.05.
( Sullivan & Verhoosel, 2013).This BMI values before had a relatively higher value of BMI
compared to the BMI values after the intervention. This, therefore, implies that individuals in
this in the intervention group moved from the overweight (25.4) scale to 24.7, which is a healthy
standard. This, therefore, shows that the intervention had a huge impact on transforming the
participants from being overweight to healthy levels. This, therefore, portrays that there exists a
significant difference between the BMI value before and after intervention at alpha=0.05.
Paraphrase This Document
Need a fresh take? Get an instant paraphrase of this document with our AI Paraphraser
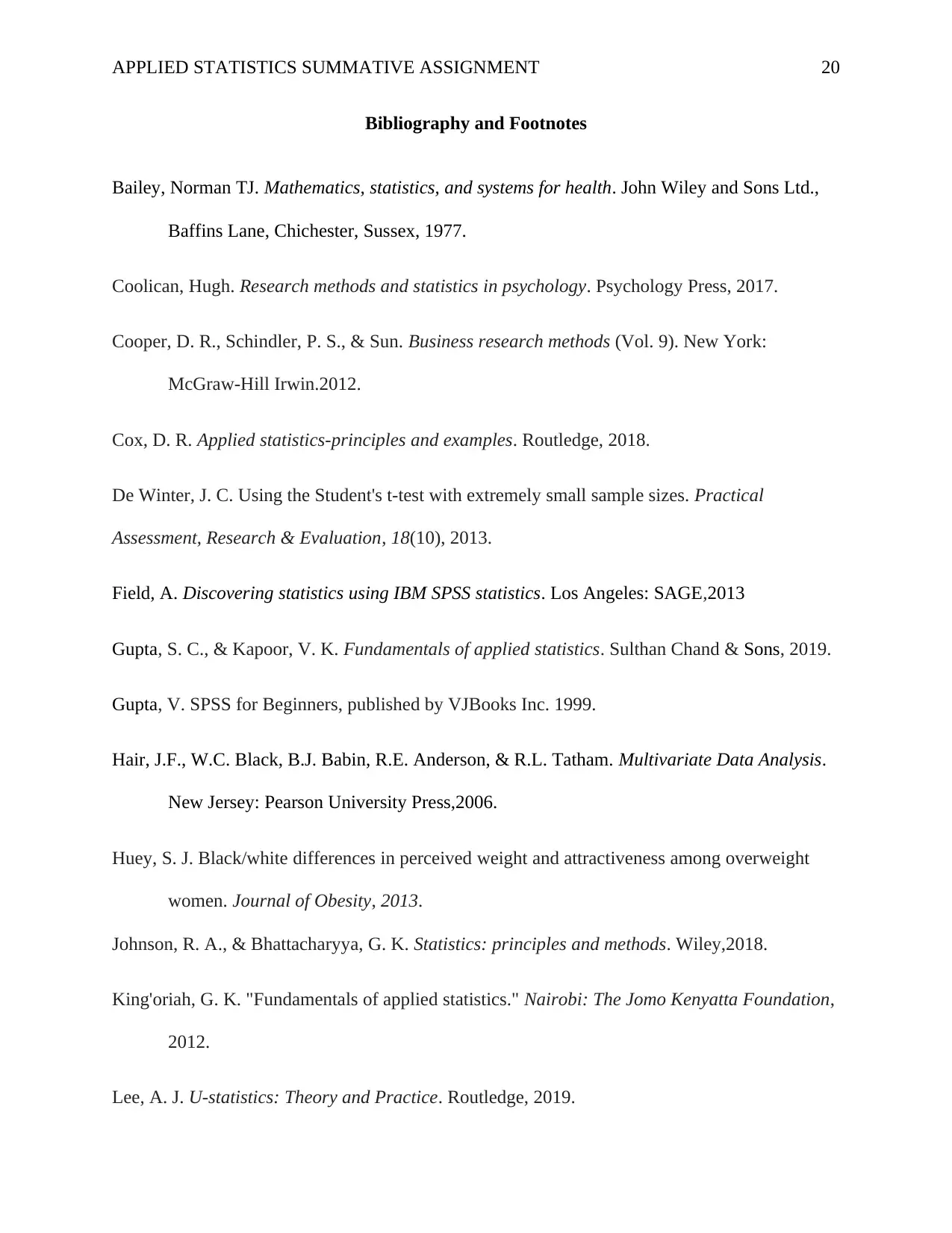
APPLIED STATISTICS SUMMATIVE ASSIGNMENT 20
Bibliography and Footnotes
Bailey, Norman TJ. Mathematics, statistics, and systems for health. John Wiley and Sons Ltd.,
Baffins Lane, Chichester, Sussex, 1977.
Coolican, Hugh. Research methods and statistics in psychology. Psychology Press, 2017.
Cooper, D. R., Schindler, P. S., & Sun. Business research methods (Vol. 9). New York:
McGraw-Hill Irwin.2012.
Cox, D. R. Applied statistics-principles and examples. Routledge, 2018.
De Winter, J. C. Using the Student's t-test with extremely small sample sizes. Practical
Assessment, Research & Evaluation, 18(10), 2013.
Field, A. Discovering statistics using IBM SPSS statistics. Los Angeles: SAGE,2013
Gupta, S. C., & Kapoor, V. K. Fundamentals of applied statistics. Sulthan Chand & Sons, 2019.
Gupta, V. SPSS for Beginners, published by VJBooks Inc. 1999.
Hair, J.F., W.C. Black, B.J. Babin, R.E. Anderson, & R.L. Tatham. Multivariate Data Analysis.
New Jersey: Pearson University Press,2006.
Huey, S. J. Black/white differences in perceived weight and attractiveness among overweight
women. Journal of Obesity, 2013.
Johnson, R. A., & Bhattacharyya, G. K. Statistics: principles and methods. Wiley,2018.
King'oriah, G. K. "Fundamentals of applied statistics." Nairobi: The Jomo Kenyatta Foundation,
2012.
Lee, A. J. U-statistics: Theory and Practice. Routledge, 2019.
Bibliography and Footnotes
Bailey, Norman TJ. Mathematics, statistics, and systems for health. John Wiley and Sons Ltd.,
Baffins Lane, Chichester, Sussex, 1977.
Coolican, Hugh. Research methods and statistics in psychology. Psychology Press, 2017.
Cooper, D. R., Schindler, P. S., & Sun. Business research methods (Vol. 9). New York:
McGraw-Hill Irwin.2012.
Cox, D. R. Applied statistics-principles and examples. Routledge, 2018.
De Winter, J. C. Using the Student's t-test with extremely small sample sizes. Practical
Assessment, Research & Evaluation, 18(10), 2013.
Field, A. Discovering statistics using IBM SPSS statistics. Los Angeles: SAGE,2013
Gupta, S. C., & Kapoor, V. K. Fundamentals of applied statistics. Sulthan Chand & Sons, 2019.
Gupta, V. SPSS for Beginners, published by VJBooks Inc. 1999.
Hair, J.F., W.C. Black, B.J. Babin, R.E. Anderson, & R.L. Tatham. Multivariate Data Analysis.
New Jersey: Pearson University Press,2006.
Huey, S. J. Black/white differences in perceived weight and attractiveness among overweight
women. Journal of Obesity, 2013.
Johnson, R. A., & Bhattacharyya, G. K. Statistics: principles and methods. Wiley,2018.
King'oriah, G. K. "Fundamentals of applied statistics." Nairobi: The Jomo Kenyatta Foundation,
2012.
Lee, A. J. U-statistics: Theory and Practice. Routledge, 2019.
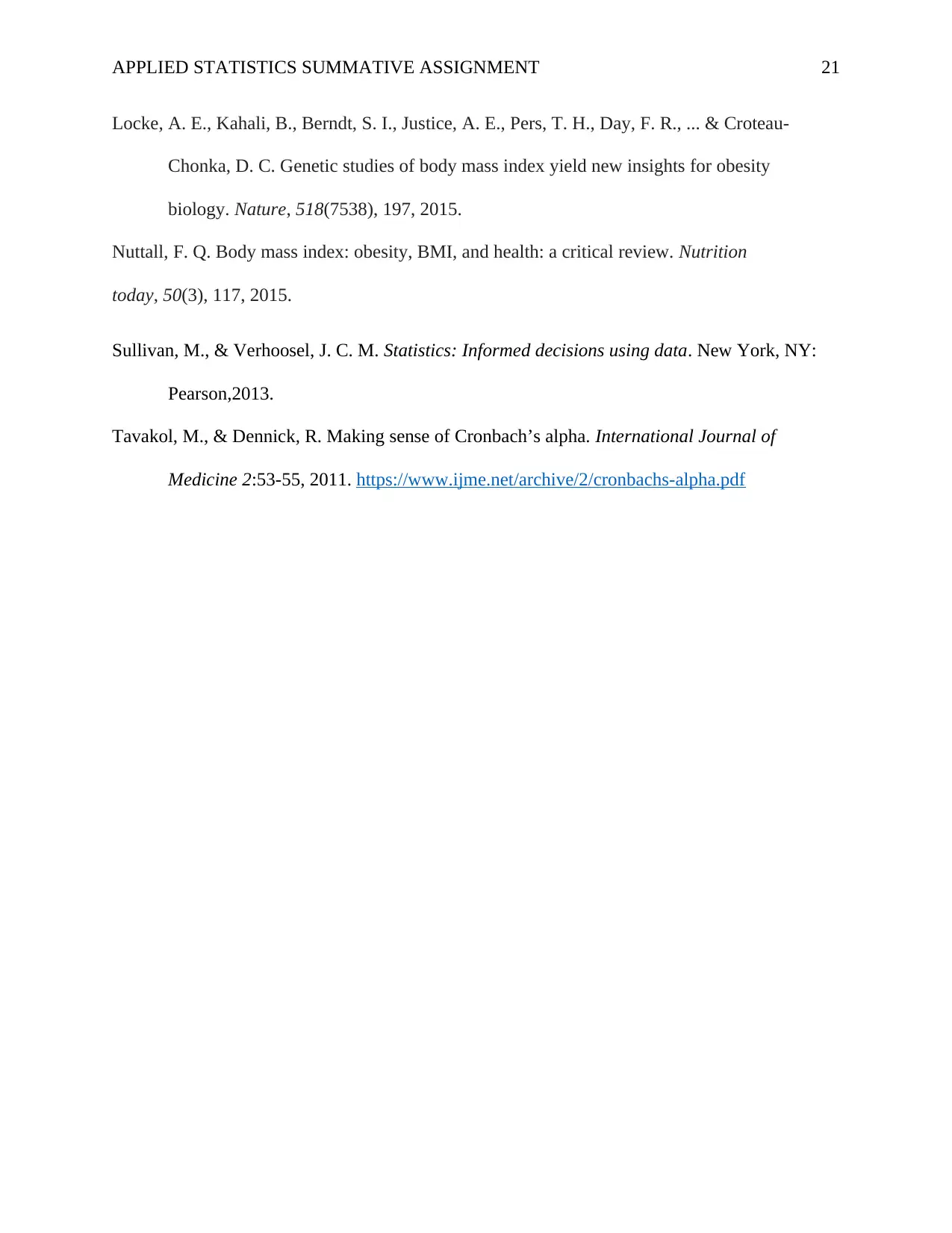
APPLIED STATISTICS SUMMATIVE ASSIGNMENT 21
Locke, A. E., Kahali, B., Berndt, S. I., Justice, A. E., Pers, T. H., Day, F. R., ... & Croteau-
Chonka, D. C. Genetic studies of body mass index yield new insights for obesity
biology. Nature, 518(7538), 197, 2015.
Nuttall, F. Q. Body mass index: obesity, BMI, and health: a critical review. Nutrition
today, 50(3), 117, 2015.
Sullivan, M., & Verhoosel, J. C. M. Statistics: Informed decisions using data. New York, NY:
Pearson,2013.
Tavakol, M., & Dennick, R. Making sense of Cronbach’s alpha. International Journal of
Medicine 2:53-55, 2011. https://www.ijme.net/archive/2/cronbachs-alpha.pdf
Locke, A. E., Kahali, B., Berndt, S. I., Justice, A. E., Pers, T. H., Day, F. R., ... & Croteau-
Chonka, D. C. Genetic studies of body mass index yield new insights for obesity
biology. Nature, 518(7538), 197, 2015.
Nuttall, F. Q. Body mass index: obesity, BMI, and health: a critical review. Nutrition
today, 50(3), 117, 2015.
Sullivan, M., & Verhoosel, J. C. M. Statistics: Informed decisions using data. New York, NY:
Pearson,2013.
Tavakol, M., & Dennick, R. Making sense of Cronbach’s alpha. International Journal of
Medicine 2:53-55, 2011. https://www.ijme.net/archive/2/cronbachs-alpha.pdf
You're viewing a preview
Unlock full access by subscribing today!
1 out of 21
Related Documents

Your All-in-One AI-Powered Toolkit for Academic Success.
+13062052269
info@desklib.com
Available 24*7 on WhatsApp / Email
Unlock your academic potential
© 2024 | Zucol Services PVT LTD | All rights reserved.