Box-Jenkins Approach to ARMA Models & Analysis of US CPI Data
Added on 2023-06-15
6 Pages2695 Words67 Views
1. Explain the Box-Jenkins approach to building ARMA models.
This method has been developed by Box and Jenkins to identify and estimate the model for specific
time series, which is called as Box-Jenkins method. Auto regressive integrated moving average
(ARIMA) model is known as autoregressive moving average (ARMA) is particularly used in
econometrics and time series analysis for forecasting the future Box et.al (1974), Box et.al (1973) and
Ljung and Box (1978). The method is well fitted to long and medium time series at least 50
observations. We call times time series has a sequenced though time. The sequence can be denoted by
X1, X2, X3.... Xt , where t is known as time period and X are variables. If X is determined by
mathematical rule we call it deterministic, if only future value can be predicted by probability we call
it statistical. Statistical process is known as stationary stochastic process Cramér and Leadbetter
(2013). If distribution is same for all values of t then the statistical process is stationary, which means
mean and variance is constant for all values of t. A series having trend is non-stationary because it
depends on value of t. It is important in Box-Jenkins method to make non-stationary data into a
stationary data. To check the statinarity we can apply Dickey and Fuller (1981) test of unit root.
The Box-Jenkins approach is based on iteration approach that consists of the following 3 steps.
1. Identification: we used it for plotting of the data, autocorrelation and partial correlation that
best summarizes the data.
In identification we further divide it into two parts.
Differencing: We can difference the data on the basis of unit root test, by which we can make non-
stationary data, stationary data by first or second order differencing.
Configuring AR and MA
Auto correlation function: The first step summarizes the autocorrelation and X-axis shows the lag
and the y-axis gives correlation coefficient between -1 to 1.
Partial autocorrelation function: The correlation is collected for the lag values that are not
accounted.
2. Estimation: The estimation of Box-Jenkins can be done by Maximum Likelihood technique.
This estimation is done for checking the autocorrelation of the residuals. If the autocorrelation
is small the model is fine and forecast can be generated. If the autocorrelation is large then p
and q are adjusted and re-estimated accordingly.
3. Diagnostic Checking: we evaluate the model on the bases of available data by taking
autocorrelation into consideration. Weather the model is good fit or not.
Over fitting: If the model is complex, that means the model over fits the data, we need to calculate
random noise. This problem arises in time series forecasting analysis because of negative results for
poor forecasting.
Residual errors:
By calculating residuals errors can be reduced, which means errors in model would resemble white
noise, which is Gaussian distribution with zero mean and uniform variance. We can use plots,
This method has been developed by Box and Jenkins to identify and estimate the model for specific
time series, which is called as Box-Jenkins method. Auto regressive integrated moving average
(ARIMA) model is known as autoregressive moving average (ARMA) is particularly used in
econometrics and time series analysis for forecasting the future Box et.al (1974), Box et.al (1973) and
Ljung and Box (1978). The method is well fitted to long and medium time series at least 50
observations. We call times time series has a sequenced though time. The sequence can be denoted by
X1, X2, X3.... Xt , where t is known as time period and X are variables. If X is determined by
mathematical rule we call it deterministic, if only future value can be predicted by probability we call
it statistical. Statistical process is known as stationary stochastic process Cramér and Leadbetter
(2013). If distribution is same for all values of t then the statistical process is stationary, which means
mean and variance is constant for all values of t. A series having trend is non-stationary because it
depends on value of t. It is important in Box-Jenkins method to make non-stationary data into a
stationary data. To check the statinarity we can apply Dickey and Fuller (1981) test of unit root.
The Box-Jenkins approach is based on iteration approach that consists of the following 3 steps.
1. Identification: we used it for plotting of the data, autocorrelation and partial correlation that
best summarizes the data.
In identification we further divide it into two parts.
Differencing: We can difference the data on the basis of unit root test, by which we can make non-
stationary data, stationary data by first or second order differencing.
Configuring AR and MA
Auto correlation function: The first step summarizes the autocorrelation and X-axis shows the lag
and the y-axis gives correlation coefficient between -1 to 1.
Partial autocorrelation function: The correlation is collected for the lag values that are not
accounted.
2. Estimation: The estimation of Box-Jenkins can be done by Maximum Likelihood technique.
This estimation is done for checking the autocorrelation of the residuals. If the autocorrelation
is small the model is fine and forecast can be generated. If the autocorrelation is large then p
and q are adjusted and re-estimated accordingly.
3. Diagnostic Checking: we evaluate the model on the bases of available data by taking
autocorrelation into consideration. Weather the model is good fit or not.
Over fitting: If the model is complex, that means the model over fits the data, we need to calculate
random noise. This problem arises in time series forecasting analysis because of negative results for
poor forecasting.
Residual errors:
By calculating residuals errors can be reduced, which means errors in model would resemble white
noise, which is Gaussian distribution with zero mean and uniform variance. We can use plots,
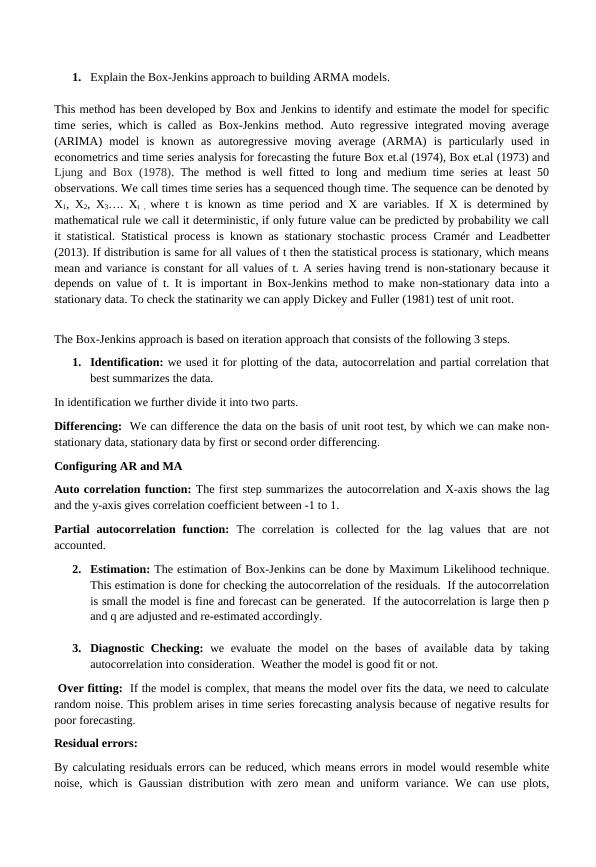
histograms and Q-Q plots. Otherwise we can check it by ACF and PACF plot of residual error time
series.
2. Open the workfile "cpi.dta". The file contains quarterly data of the U.S. Consumer Price Index
(CPI) over the period 1960Q1 to 2012 Q4. Calculate the logarithmic change of the series, i.e.:
𝛥𝑐𝑝𝑖𝑡=𝑐𝑝𝑖𝑡−𝑐𝑝𝑖𝑡−1
where 𝑐𝑝𝑖𝑡=ln(𝐶𝑃𝐼𝑡) (Note: Use the natural logarithm).
a) Use the full sample period to obtain a graph of the Δ𝑐𝑝𝑖 series. Based on the graph, does the
series appear to be stationary? Comment on the behavior of the series over time.
The logarithmic change of data for U.S. Consumer Price Index (CPI) over the period 1960Q1 to 2012
Q4 has been calculated by taking Log of variable CPI.
3.2
3.6
4.0
4.4
4.8
5.2
5.6
60 65 70 75 80 85 90 95 00 05 10
CPI1
Source: Authors Competitions
Note: The variable CPI seems stationary there is no fluctuation over the period of time.
The graph of CPI over the time period 1960Q1 to 2012 Q4 has been calculated. The graph is based on
log variables, based on graph it look the variable is stationary for all period of time. It means the data
have constant mean, variance and autocorrelation, etc. are all constant over time.
b) Read Chapter 6 in Brooks (2014) and briefly explain the terms autocorrelation function (ACF) and
partial autocorrelation function (PACF). What shape would these two functions take for a stationary
autoregressive process, a moving average process, and a combination autoregressive moving average
process?
Autocorrelation function (ACF) is based on AR (1) model which predicts the present value of a times
series by taking immediate values of time Wu and Wei (1989). ACF means series is weakly stationary
series. Which means autocorrelation for any participant lag is same. It means mean, variance,
covariance and correlation between Xt is same for all t.
Partial Autocorrelation function (PACF) is known as conditional correlation. In which we consider two
variables under the assumption by taking value of other set of variables.
series.
2. Open the workfile "cpi.dta". The file contains quarterly data of the U.S. Consumer Price Index
(CPI) over the period 1960Q1 to 2012 Q4. Calculate the logarithmic change of the series, i.e.:
𝛥𝑐𝑝𝑖𝑡=𝑐𝑝𝑖𝑡−𝑐𝑝𝑖𝑡−1
where 𝑐𝑝𝑖𝑡=ln(𝐶𝑃𝐼𝑡) (Note: Use the natural logarithm).
a) Use the full sample period to obtain a graph of the Δ𝑐𝑝𝑖 series. Based on the graph, does the
series appear to be stationary? Comment on the behavior of the series over time.
The logarithmic change of data for U.S. Consumer Price Index (CPI) over the period 1960Q1 to 2012
Q4 has been calculated by taking Log of variable CPI.
3.2
3.6
4.0
4.4
4.8
5.2
5.6
60 65 70 75 80 85 90 95 00 05 10
CPI1
Source: Authors Competitions
Note: The variable CPI seems stationary there is no fluctuation over the period of time.
The graph of CPI over the time period 1960Q1 to 2012 Q4 has been calculated. The graph is based on
log variables, based on graph it look the variable is stationary for all period of time. It means the data
have constant mean, variance and autocorrelation, etc. are all constant over time.
b) Read Chapter 6 in Brooks (2014) and briefly explain the terms autocorrelation function (ACF) and
partial autocorrelation function (PACF). What shape would these two functions take for a stationary
autoregressive process, a moving average process, and a combination autoregressive moving average
process?
Autocorrelation function (ACF) is based on AR (1) model which predicts the present value of a times
series by taking immediate values of time Wu and Wei (1989). ACF means series is weakly stationary
series. Which means autocorrelation for any participant lag is same. It means mean, variance,
covariance and correlation between Xt is same for all t.
Partial Autocorrelation function (PACF) is known as conditional correlation. In which we consider two
variables under the assumption by taking value of other set of variables.
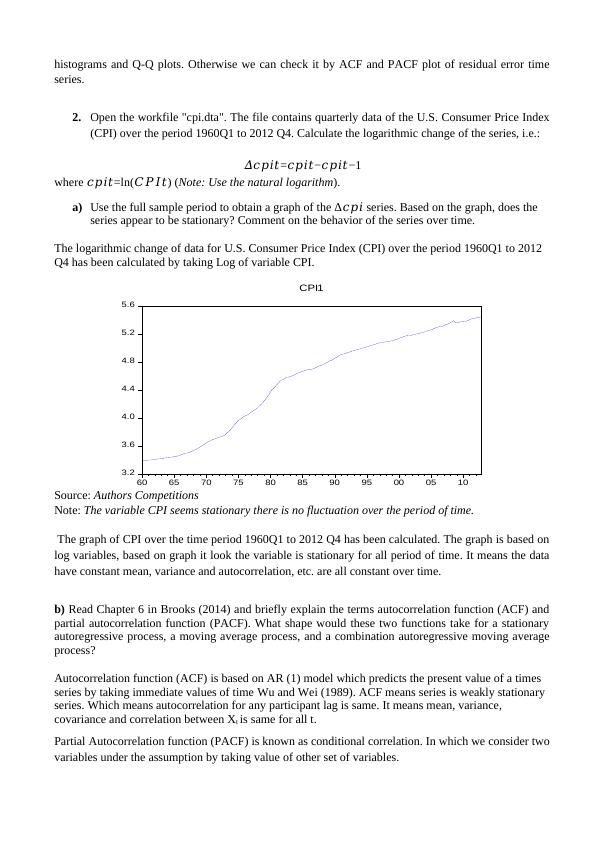
We can consider a regression equation in which y is response variable x1, x2, x3 are predictive
variables. Partial correlation between y and x3 is correlation between determined by y and x3 are related
to x1 and x2. Partial correlation can be done by correlating residuals. One is predicting from y to x1 and
x2and second from x3 to x1 and x2. We have done partial correlation from y to x3 predicted by x1 and x2.
c) Obtain the ACF and PACF for Δ𝑐𝑝𝑖 in Stata (specify the number of autocorrelations to be 12)
using data from 1960Q1 to 2009Q4 (Note that this is not the full sample). Discuss the significance of
the ACF coefficients. Based on your answer to Question 2(b), try to identify the tentative models that
you would want to estimate.
Table1: Results of ACF and PACF
Autocorrelation Partial Correlation AC PAC Q-Stat Prob
.|*** | .|*** | 1 0.461 0.461 45.499 0.000
.|** | .|. | 2 0.241 0.036 57.989 0.000
.|** | .|* | 3 0.270 0.186 73.769 0.000
.|* | .|. | 4 0.199 0.008 82.389 0.000
.|** | .|** | 5 0.298 0.232 101.79 0.000
.|** | .|* | 6 0.304 0.078 122.11 0.000
.|* | .|. | 7 0.194 -0.006 130.40 0.000
.|* | .|. | 8 0.207 0.062 139.85 0.000
.|* | *|. | 9 0.113 -0.091 142.68 0.000
.|* | .|. | 10 0.107 0.026 145.25 0.000
.|* | .|. | 11 0.157 0.013 150.81 0.000
.|* | .|* | 12 0.180 0.088 158.11 0.000
The results if ACF shows that there is no trend in the data, because of fluctuation from 0.199 at lag 4
to 0.304 at lag 6. We can estimate simple liner regression model and if we can include some more
variables in our study we can go for short-run and long-run dynamics and causality analysis. The study
has a short coming that we are using one a single variables for our study. We need to include more
independent variables to find exact relationship.
d) Estimate all ARMA models from order (0,0) to (3,3) for Δ𝑐𝑝𝑖 over the sample period 1960Q1 to
2009Q4. From your estimations which is the preferred model order? Explain why? (You do not need to
report coefficient estimates for all the estimated models; just report the value of the AIC and SBIC
criteria in a Table).
We have estimated the model on the basis of Akaike information criterion (AIC) and Schwarz criterion
(SBIC) information criteria. For the estimation of model we need to find out AIC and SBIC lag length
Teräsvirta and Mellin (1986) and Burnham and Anderson (2004). We first take the lag order 0,0 and
the 3,3. The table below gives the results of information criteria. Based on the below table 2, we can
choose (2,3) (0,0) is a best model because the lower the value of Akaike of information criteria the
better the model. The value of AIC (2.178715) is lowest. In table 2 there is a comparison when we take
lag order 0,0 and 3,3 which model is best, based on the results model 3,3 is best because of AIC and
SBIC value is lower.
Table 1: AIC and SBIC based on lag order (0,0)
Model AIC BIC HQ
(0,0) (0,0) 2.573096 2.604973 2.585983
variables. Partial correlation between y and x3 is correlation between determined by y and x3 are related
to x1 and x2. Partial correlation can be done by correlating residuals. One is predicting from y to x1 and
x2and second from x3 to x1 and x2. We have done partial correlation from y to x3 predicted by x1 and x2.
c) Obtain the ACF and PACF for Δ𝑐𝑝𝑖 in Stata (specify the number of autocorrelations to be 12)
using data from 1960Q1 to 2009Q4 (Note that this is not the full sample). Discuss the significance of
the ACF coefficients. Based on your answer to Question 2(b), try to identify the tentative models that
you would want to estimate.
Table1: Results of ACF and PACF
Autocorrelation Partial Correlation AC PAC Q-Stat Prob
.|*** | .|*** | 1 0.461 0.461 45.499 0.000
.|** | .|. | 2 0.241 0.036 57.989 0.000
.|** | .|* | 3 0.270 0.186 73.769 0.000
.|* | .|. | 4 0.199 0.008 82.389 0.000
.|** | .|** | 5 0.298 0.232 101.79 0.000
.|** | .|* | 6 0.304 0.078 122.11 0.000
.|* | .|. | 7 0.194 -0.006 130.40 0.000
.|* | .|. | 8 0.207 0.062 139.85 0.000
.|* | *|. | 9 0.113 -0.091 142.68 0.000
.|* | .|. | 10 0.107 0.026 145.25 0.000
.|* | .|. | 11 0.157 0.013 150.81 0.000
.|* | .|* | 12 0.180 0.088 158.11 0.000
The results if ACF shows that there is no trend in the data, because of fluctuation from 0.199 at lag 4
to 0.304 at lag 6. We can estimate simple liner regression model and if we can include some more
variables in our study we can go for short-run and long-run dynamics and causality analysis. The study
has a short coming that we are using one a single variables for our study. We need to include more
independent variables to find exact relationship.
d) Estimate all ARMA models from order (0,0) to (3,3) for Δ𝑐𝑝𝑖 over the sample period 1960Q1 to
2009Q4. From your estimations which is the preferred model order? Explain why? (You do not need to
report coefficient estimates for all the estimated models; just report the value of the AIC and SBIC
criteria in a Table).
We have estimated the model on the basis of Akaike information criterion (AIC) and Schwarz criterion
(SBIC) information criteria. For the estimation of model we need to find out AIC and SBIC lag length
Teräsvirta and Mellin (1986) and Burnham and Anderson (2004). We first take the lag order 0,0 and
the 3,3. The table below gives the results of information criteria. Based on the below table 2, we can
choose (2,3) (0,0) is a best model because the lower the value of Akaike of information criteria the
better the model. The value of AIC (2.178715) is lowest. In table 2 there is a comparison when we take
lag order 0,0 and 3,3 which model is best, based on the results model 3,3 is best because of AIC and
SBIC value is lower.
Table 1: AIC and SBIC based on lag order (0,0)
Model AIC BIC HQ
(0,0) (0,0) 2.573096 2.604973 2.585983
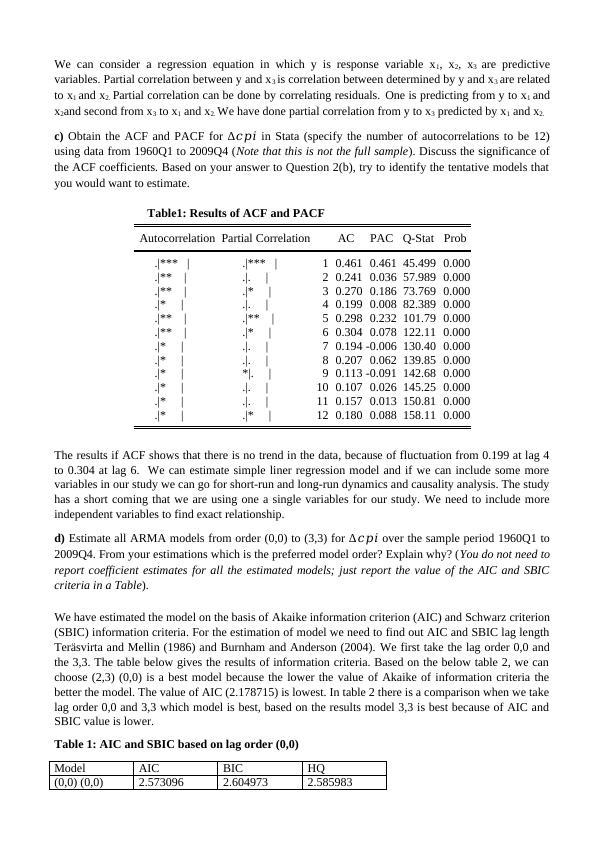
End of preview
Want to access all the pages? Upload your documents or become a member.
Related Documents
Financial Investment Analysis: Box-Jenkins Methodology, VAR Models, and Impulse Function Graphslg...
|22
|2630
|251
Financial Econometrics: ACF, PACF, Model Selection, Forecastinglg...
|14
|1955
|219
International Review of Financial Analysislg...
|12
|3242
|27
Forecasting Time Series Assignment 2022lg...
|12
|1735
|7
Problem 1.. In the dataset of “production.txt”, it has lg...
|2
|457
|269
Analysis of Time Series Data and Logit Model for House Priceslg...
|10
|982
|289