AI and Energy: Exploring the Importance of Artificial Intelligence and Data Science for Power Network and Smart Grid Developments
VerifiedAdded on 2023/01/12
|11
|4992
|75
AI Summary
This article explores the importance of Artificial Intelligence (AI) and Data Science in the development of power networks and smart grids. It explains the meanings of AI and Data Science and their relevance to the energy sector. The article also discusses the difference between knowledge-driven and data-driven approaches and provides examples of techniques from each category. It further delves into the applications of intelligent systems in circuit breaker condition monitoring, diagnostics, and prediction.
Contribute Materials
Your contribution can guide someone’s learning journey. Share your
documents today.
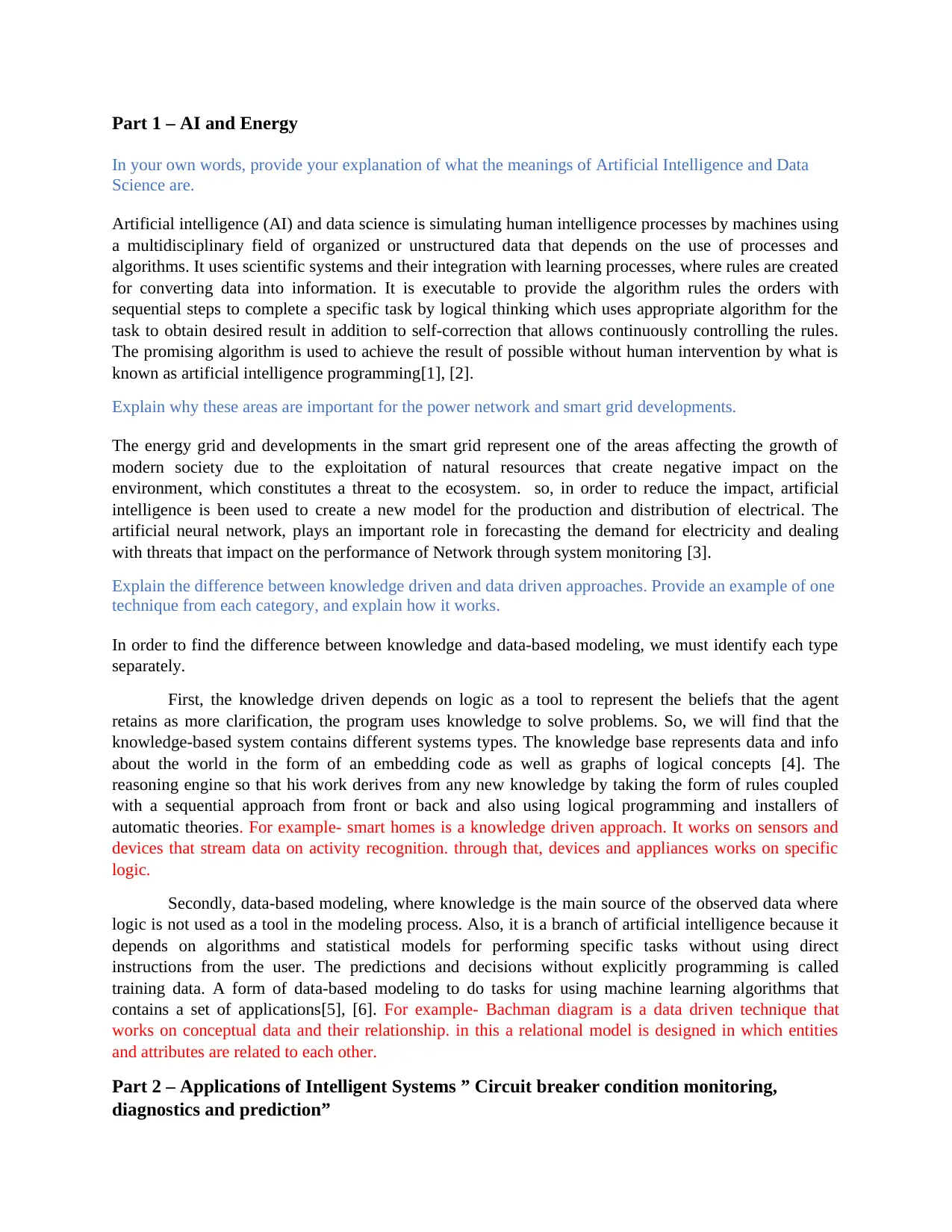
Part 1 – AI and Energy
In your own words, provide your explanation of what the meanings of Artificial Intelligence and Data
Science are.
Artificial intelligence (AI) and data science is simulating human intelligence processes by machines using
a multidisciplinary field of organized or unstructured data that depends on the use of processes and
algorithms. It uses scientific systems and their integration with learning processes, where rules are created
for converting data into information. It is executable to provide the algorithm rules the orders with
sequential steps to complete a specific task by logical thinking which uses appropriate algorithm for the
task to obtain desired result in addition to self-correction that allows continuously controlling the rules.
The promising algorithm is used to achieve the result of possible without human intervention by what is
known as artificial intelligence programming[1], [2].
Explain why these areas are important for the power network and smart grid developments.
The energy grid and developments in the smart grid represent one of the areas affecting the growth of
modern society due to the exploitation of natural resources that create negative impact on the
environment, which constitutes a threat to the ecosystem. so, in order to reduce the impact, artificial
intelligence is been used to create a new model for the production and distribution of electrical. The
artificial neural network, plays an important role in forecasting the demand for electricity and dealing
with threats that impact on the performance of Network through system monitoring [3].
Explain the difference between knowledge driven and data driven approaches. Provide an example of one
technique from each category, and explain how it works.
In order to find the difference between knowledge and data-based modeling, we must identify each type
separately.
First, the knowledge driven depends on logic as a tool to represent the beliefs that the agent
retains as more clarification, the program uses knowledge to solve problems. So, we will find that the
knowledge-based system contains different systems types. The knowledge base represents data and info
about the world in the form of an embedding code as well as graphs of logical concepts [4]. The
reasoning engine so that his work derives from any new knowledge by taking the form of rules coupled
with a sequential approach from front or back and also using logical programming and installers of
automatic theories. For example- smart homes is a knowledge driven approach. It works on sensors and
devices that stream data on activity recognition. through that, devices and appliances works on specific
logic.
Secondly, data-based modeling, where knowledge is the main source of the observed data where
logic is not used as a tool in the modeling process. Also, it is a branch of artificial intelligence because it
depends on algorithms and statistical models for performing specific tasks without using direct
instructions from the user. The predictions and decisions without explicitly programming is called
training data. A form of data-based modeling to do tasks for using machine learning algorithms that
contains a set of applications[5], [6]. For example- Bachman diagram is a data driven technique that
works on conceptual data and their relationship. in this a relational model is designed in which entities
and attributes are related to each other.
Part 2 – Applications of Intelligent Systems ” Circuit breaker condition monitoring,
diagnostics and prediction”
In your own words, provide your explanation of what the meanings of Artificial Intelligence and Data
Science are.
Artificial intelligence (AI) and data science is simulating human intelligence processes by machines using
a multidisciplinary field of organized or unstructured data that depends on the use of processes and
algorithms. It uses scientific systems and their integration with learning processes, where rules are created
for converting data into information. It is executable to provide the algorithm rules the orders with
sequential steps to complete a specific task by logical thinking which uses appropriate algorithm for the
task to obtain desired result in addition to self-correction that allows continuously controlling the rules.
The promising algorithm is used to achieve the result of possible without human intervention by what is
known as artificial intelligence programming[1], [2].
Explain why these areas are important for the power network and smart grid developments.
The energy grid and developments in the smart grid represent one of the areas affecting the growth of
modern society due to the exploitation of natural resources that create negative impact on the
environment, which constitutes a threat to the ecosystem. so, in order to reduce the impact, artificial
intelligence is been used to create a new model for the production and distribution of electrical. The
artificial neural network, plays an important role in forecasting the demand for electricity and dealing
with threats that impact on the performance of Network through system monitoring [3].
Explain the difference between knowledge driven and data driven approaches. Provide an example of one
technique from each category, and explain how it works.
In order to find the difference between knowledge and data-based modeling, we must identify each type
separately.
First, the knowledge driven depends on logic as a tool to represent the beliefs that the agent
retains as more clarification, the program uses knowledge to solve problems. So, we will find that the
knowledge-based system contains different systems types. The knowledge base represents data and info
about the world in the form of an embedding code as well as graphs of logical concepts [4]. The
reasoning engine so that his work derives from any new knowledge by taking the form of rules coupled
with a sequential approach from front or back and also using logical programming and installers of
automatic theories. For example- smart homes is a knowledge driven approach. It works on sensors and
devices that stream data on activity recognition. through that, devices and appliances works on specific
logic.
Secondly, data-based modeling, where knowledge is the main source of the observed data where
logic is not used as a tool in the modeling process. Also, it is a branch of artificial intelligence because it
depends on algorithms and statistical models for performing specific tasks without using direct
instructions from the user. The predictions and decisions without explicitly programming is called
training data. A form of data-based modeling to do tasks for using machine learning algorithms that
contains a set of applications[5], [6]. For example- Bachman diagram is a data driven technique that
works on conceptual data and their relationship. in this a relational model is designed in which entities
and attributes are related to each other.
Part 2 – Applications of Intelligent Systems ” Circuit breaker condition monitoring,
diagnostics and prediction”
Secure Best Marks with AI Grader
Need help grading? Try our AI Grader for instant feedback on your assignments.
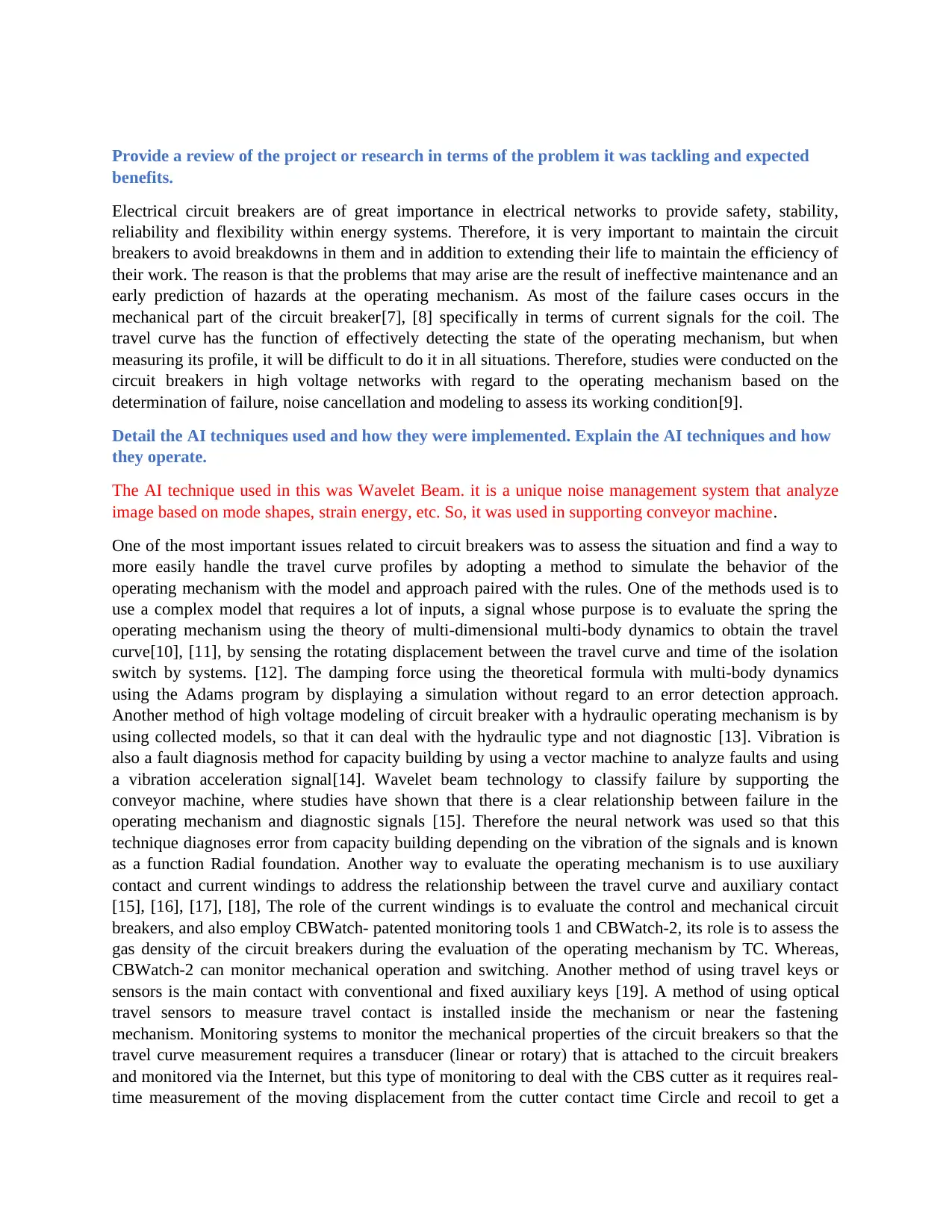
Provide a review of the project or research in terms of the problem it was tackling and expected
benefits.
Electrical circuit breakers are of great importance in electrical networks to provide safety, stability,
reliability and flexibility within energy systems. Therefore, it is very important to maintain the circuit
breakers to avoid breakdowns in them and in addition to extending their life to maintain the efficiency of
their work. The reason is that the problems that may arise are the result of ineffective maintenance and an
early prediction of hazards at the operating mechanism. As most of the failure cases occurs in the
mechanical part of the circuit breaker[7], [8] specifically in terms of current signals for the coil. The
travel curve has the function of effectively detecting the state of the operating mechanism, but when
measuring its profile, it will be difficult to do it in all situations. Therefore, studies were conducted on the
circuit breakers in high voltage networks with regard to the operating mechanism based on the
determination of failure, noise cancellation and modeling to assess its working condition[9].
Detail the AI techniques used and how they were implemented. Explain the AI techniques and how
they operate.
The AI technique used in this was Wavelet Beam. it is a unique noise management system that analyze
image based on mode shapes, strain energy, etc. So, it was used in supporting conveyor machine.
One of the most important issues related to circuit breakers was to assess the situation and find a way to
more easily handle the travel curve profiles by adopting a method to simulate the behavior of the
operating mechanism with the model and approach paired with the rules. One of the methods used is to
use a complex model that requires a lot of inputs, a signal whose purpose is to evaluate the spring the
operating mechanism using the theory of multi-dimensional multi-body dynamics to obtain the travel
curve[10], [11], by sensing the rotating displacement between the travel curve and time of the isolation
switch by systems. [12]. The damping force using the theoretical formula with multi-body dynamics
using the Adams program by displaying a simulation without regard to an error detection approach.
Another method of high voltage modeling of circuit breaker with a hydraulic operating mechanism is by
using collected models, so that it can deal with the hydraulic type and not diagnostic [13]. Vibration is
also a fault diagnosis method for capacity building by using a vector machine to analyze faults and using
a vibration acceleration signal[14]. Wavelet beam technology to classify failure by supporting the
conveyor machine, where studies have shown that there is a clear relationship between failure in the
operating mechanism and diagnostic signals [15]. Therefore the neural network was used so that this
technique diagnoses error from capacity building depending on the vibration of the signals and is known
as a function Radial foundation. Another way to evaluate the operating mechanism is to use auxiliary
contact and current windings to address the relationship between the travel curve and auxiliary contact
[15], [16], [17], [18], The role of the current windings is to evaluate the control and mechanical circuit
breakers, and also employ CBWatch- patented monitoring tools 1 and CBWatch-2, its role is to assess the
gas density of the circuit breakers during the evaluation of the operating mechanism by TC. Whereas,
CBWatch-2 can monitor mechanical operation and switching. Another method of using travel keys or
sensors is the main contact with conventional and fixed auxiliary keys [19]. A method of using optical
travel sensors to measure travel contact is installed inside the mechanism or near the fastening
mechanism. Monitoring systems to monitor the mechanical properties of the circuit breakers so that the
travel curve measurement requires a transducer (linear or rotary) that is attached to the circuit breakers
and monitored via the Internet, but this type of monitoring to deal with the CBS cutter as it requires real-
time measurement of the moving displacement from the cutter contact time Circle and recoil to get a
benefits.
Electrical circuit breakers are of great importance in electrical networks to provide safety, stability,
reliability and flexibility within energy systems. Therefore, it is very important to maintain the circuit
breakers to avoid breakdowns in them and in addition to extending their life to maintain the efficiency of
their work. The reason is that the problems that may arise are the result of ineffective maintenance and an
early prediction of hazards at the operating mechanism. As most of the failure cases occurs in the
mechanical part of the circuit breaker[7], [8] specifically in terms of current signals for the coil. The
travel curve has the function of effectively detecting the state of the operating mechanism, but when
measuring its profile, it will be difficult to do it in all situations. Therefore, studies were conducted on the
circuit breakers in high voltage networks with regard to the operating mechanism based on the
determination of failure, noise cancellation and modeling to assess its working condition[9].
Detail the AI techniques used and how they were implemented. Explain the AI techniques and how
they operate.
The AI technique used in this was Wavelet Beam. it is a unique noise management system that analyze
image based on mode shapes, strain energy, etc. So, it was used in supporting conveyor machine.
One of the most important issues related to circuit breakers was to assess the situation and find a way to
more easily handle the travel curve profiles by adopting a method to simulate the behavior of the
operating mechanism with the model and approach paired with the rules. One of the methods used is to
use a complex model that requires a lot of inputs, a signal whose purpose is to evaluate the spring the
operating mechanism using the theory of multi-dimensional multi-body dynamics to obtain the travel
curve[10], [11], by sensing the rotating displacement between the travel curve and time of the isolation
switch by systems. [12]. The damping force using the theoretical formula with multi-body dynamics
using the Adams program by displaying a simulation without regard to an error detection approach.
Another method of high voltage modeling of circuit breaker with a hydraulic operating mechanism is by
using collected models, so that it can deal with the hydraulic type and not diagnostic [13]. Vibration is
also a fault diagnosis method for capacity building by using a vector machine to analyze faults and using
a vibration acceleration signal[14]. Wavelet beam technology to classify failure by supporting the
conveyor machine, where studies have shown that there is a clear relationship between failure in the
operating mechanism and diagnostic signals [15]. Therefore the neural network was used so that this
technique diagnoses error from capacity building depending on the vibration of the signals and is known
as a function Radial foundation. Another way to evaluate the operating mechanism is to use auxiliary
contact and current windings to address the relationship between the travel curve and auxiliary contact
[15], [16], [17], [18], The role of the current windings is to evaluate the control and mechanical circuit
breakers, and also employ CBWatch- patented monitoring tools 1 and CBWatch-2, its role is to assess the
gas density of the circuit breakers during the evaluation of the operating mechanism by TC. Whereas,
CBWatch-2 can monitor mechanical operation and switching. Another method of using travel keys or
sensors is the main contact with conventional and fixed auxiliary keys [19]. A method of using optical
travel sensors to measure travel contact is installed inside the mechanism or near the fastening
mechanism. Monitoring systems to monitor the mechanical properties of the circuit breakers so that the
travel curve measurement requires a transducer (linear or rotary) that is attached to the circuit breakers
and monitored via the Internet, but this type of monitoring to deal with the CBS cutter as it requires real-
time measurement of the moving displacement from the cutter contact time Circle and recoil to get a
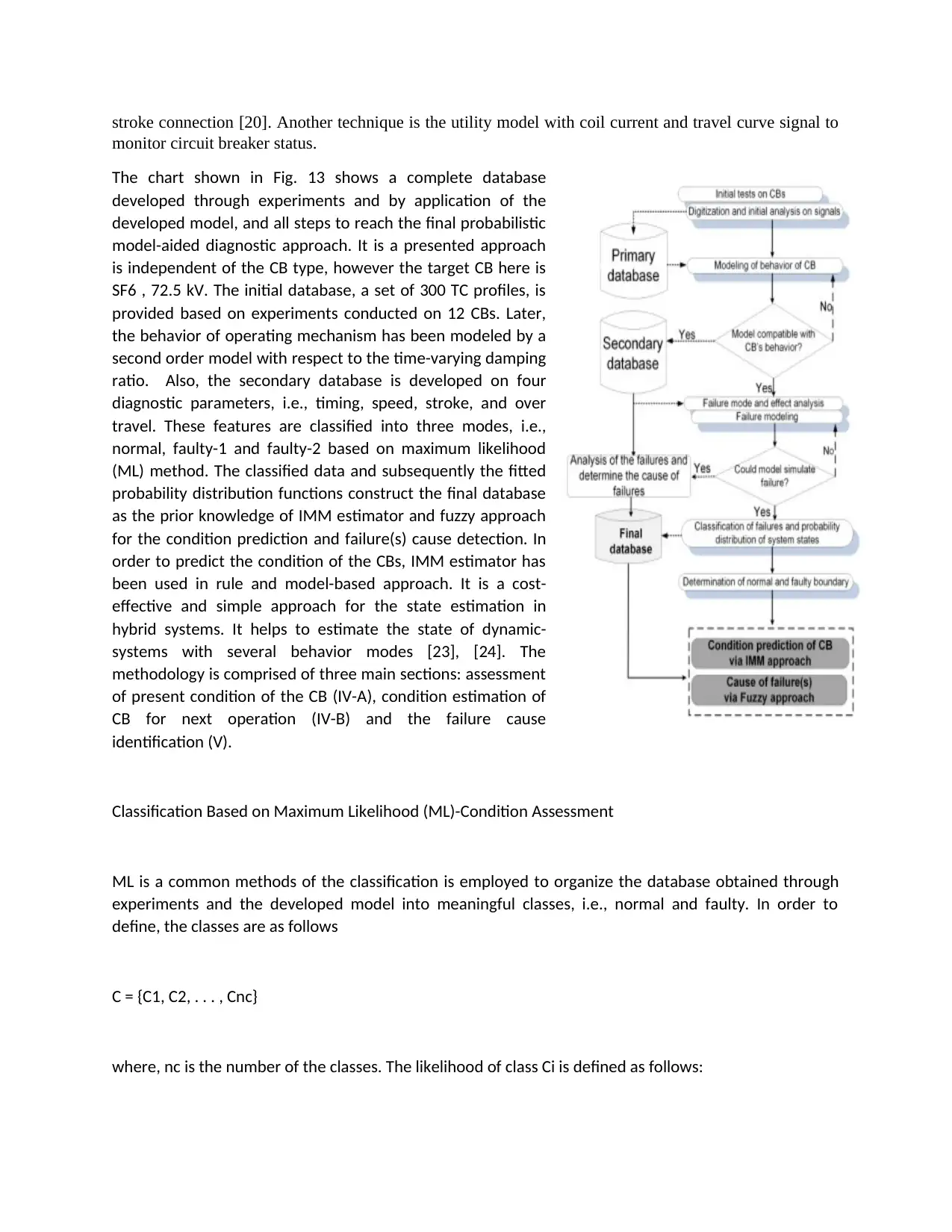
stroke connection [20]. Another technique is the utility model with coil current and travel curve signal to
monitor circuit breaker status.
The chart shown in Fig. 13 shows a complete database
developed through experiments and by application of the
developed model, and all steps to reach the final probabilistic
model-aided diagnostic approach. It is a presented approach
is independent of the CB type, however the target CB here is
SF6 , 72.5 kV. The initial database, a set of 300 TC profiles, is
provided based on experiments conducted on 12 CBs. Later,
the behavior of operating mechanism has been modeled by a
second order model with respect to the time-varying damping
ratio. Also, the secondary database is developed on four
diagnostic parameters, i.e., timing, speed, stroke, and over
travel. These features are classified into three modes, i.e.,
normal, faulty-1 and faulty-2 based on maximum likelihood
(ML) method. The classified data and subsequently the fitted
probability distribution functions construct the final database
as the prior knowledge of IMM estimator and fuzzy approach
for the condition prediction and failure(s) cause detection. In
order to predict the condition of the CBs, IMM estimator has
been used in rule and model-based approach. It is a cost-
effective and simple approach for the state estimation in
hybrid systems. It helps to estimate the state of dynamic-
systems with several behavior modes [23], [24]. The
methodology is comprised of three main sections: assessment
of present condition of the CB (IV-A), condition estimation of
CB for next operation (IV-B) and the failure cause
identification (V).
Classification Based on Maximum Likelihood (ML)-Condition Assessment
ML is a common methods of the classification is employed to organize the database obtained through
experiments and the developed model into meaningful classes, i.e., normal and faulty. In order to
define, the classes are as follows
C = {C1, C2, . . . , Cnc}
where, nc is the number of the classes. The likelihood of class Ci is defined as follows:
monitor circuit breaker status.
The chart shown in Fig. 13 shows a complete database
developed through experiments and by application of the
developed model, and all steps to reach the final probabilistic
model-aided diagnostic approach. It is a presented approach
is independent of the CB type, however the target CB here is
SF6 , 72.5 kV. The initial database, a set of 300 TC profiles, is
provided based on experiments conducted on 12 CBs. Later,
the behavior of operating mechanism has been modeled by a
second order model with respect to the time-varying damping
ratio. Also, the secondary database is developed on four
diagnostic parameters, i.e., timing, speed, stroke, and over
travel. These features are classified into three modes, i.e.,
normal, faulty-1 and faulty-2 based on maximum likelihood
(ML) method. The classified data and subsequently the fitted
probability distribution functions construct the final database
as the prior knowledge of IMM estimator and fuzzy approach
for the condition prediction and failure(s) cause detection. In
order to predict the condition of the CBs, IMM estimator has
been used in rule and model-based approach. It is a cost-
effective and simple approach for the state estimation in
hybrid systems. It helps to estimate the state of dynamic-
systems with several behavior modes [23], [24]. The
methodology is comprised of three main sections: assessment
of present condition of the CB (IV-A), condition estimation of
CB for next operation (IV-B) and the failure cause
identification (V).
Classification Based on Maximum Likelihood (ML)-Condition Assessment
ML is a common methods of the classification is employed to organize the database obtained through
experiments and the developed model into meaningful classes, i.e., normal and faulty. In order to
define, the classes are as follows
C = {C1, C2, . . . , Cnc}
where, nc is the number of the classes. The likelihood of class Ci is defined as follows:
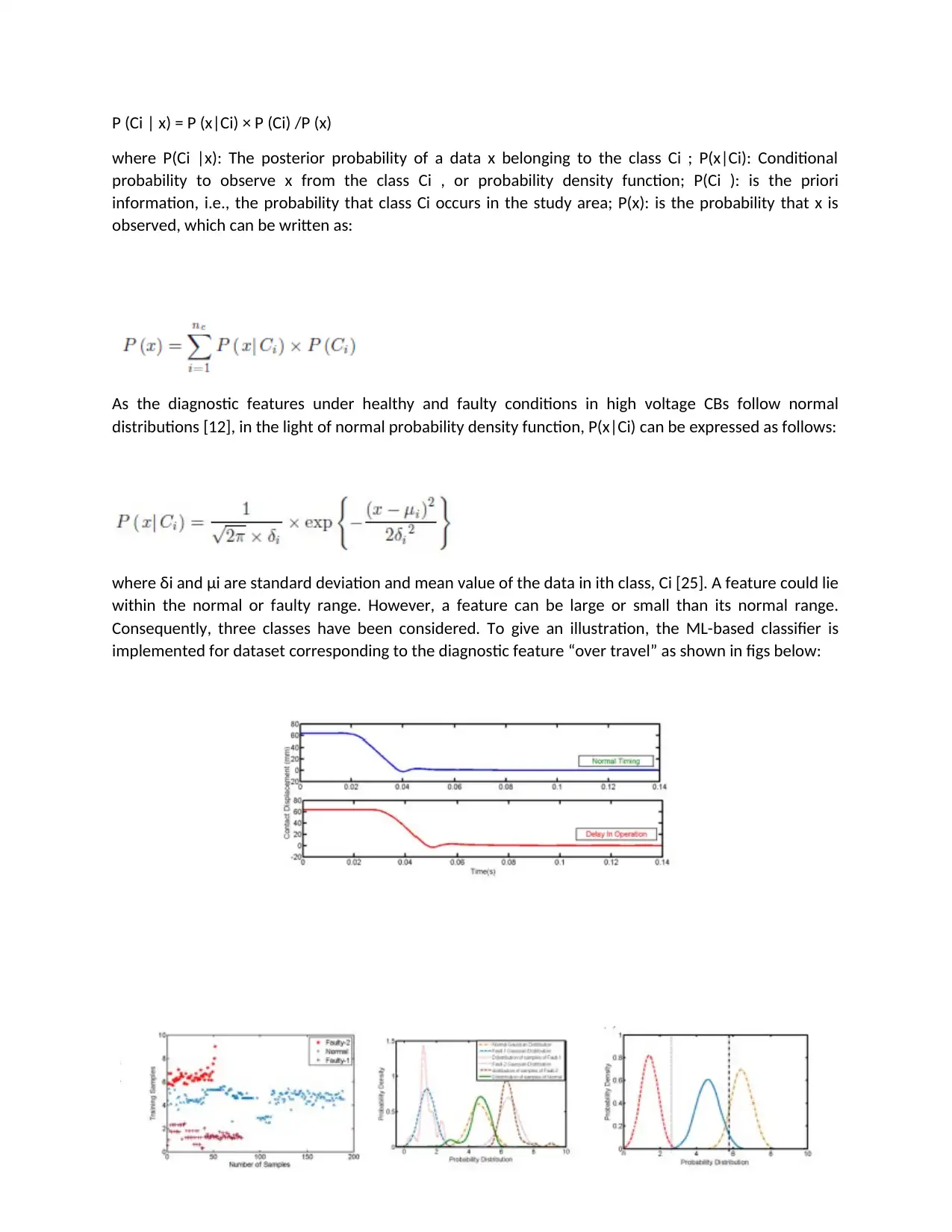
P (Ci | x) = P (x|Ci) × P (Ci) /P (x)
where P(Ci |x): The posterior probability of a data x belonging to the class Ci ; P(x|Ci): Conditional
probability to observe x from the class Ci , or probability density function; P(Ci ): is the priori
information, i.e., the probability that class Ci occurs in the study area; P(x): is the probability that x is
observed, which can be written as:
As the diagnostic features under healthy and faulty conditions in high voltage CBs follow normal
distributions [12], in the light of normal probability density function, P(x|Ci) can be expressed as follows:
where δi and μi are standard deviation and mean value of the data in ith class, Ci [25]. A feature could lie
within the normal or faulty range. However, a feature can be large or small than its normal range.
Consequently, three classes have been considered. To give an illustration, the ML-based classifier is
implemented for dataset corresponding to the diagnostic feature “over travel” as shown in figs below:
where P(Ci |x): The posterior probability of a data x belonging to the class Ci ; P(x|Ci): Conditional
probability to observe x from the class Ci , or probability density function; P(Ci ): is the priori
information, i.e., the probability that class Ci occurs in the study area; P(x): is the probability that x is
observed, which can be written as:
As the diagnostic features under healthy and faulty conditions in high voltage CBs follow normal
distributions [12], in the light of normal probability density function, P(x|Ci) can be expressed as follows:
where δi and μi are standard deviation and mean value of the data in ith class, Ci [25]. A feature could lie
within the normal or faulty range. However, a feature can be large or small than its normal range.
Consequently, three classes have been considered. To give an illustration, the ML-based classifier is
implemented for dataset corresponding to the diagnostic feature “over travel” as shown in figs below:
Secure Best Marks with AI Grader
Need help grading? Try our AI Grader for instant feedback on your assignments.
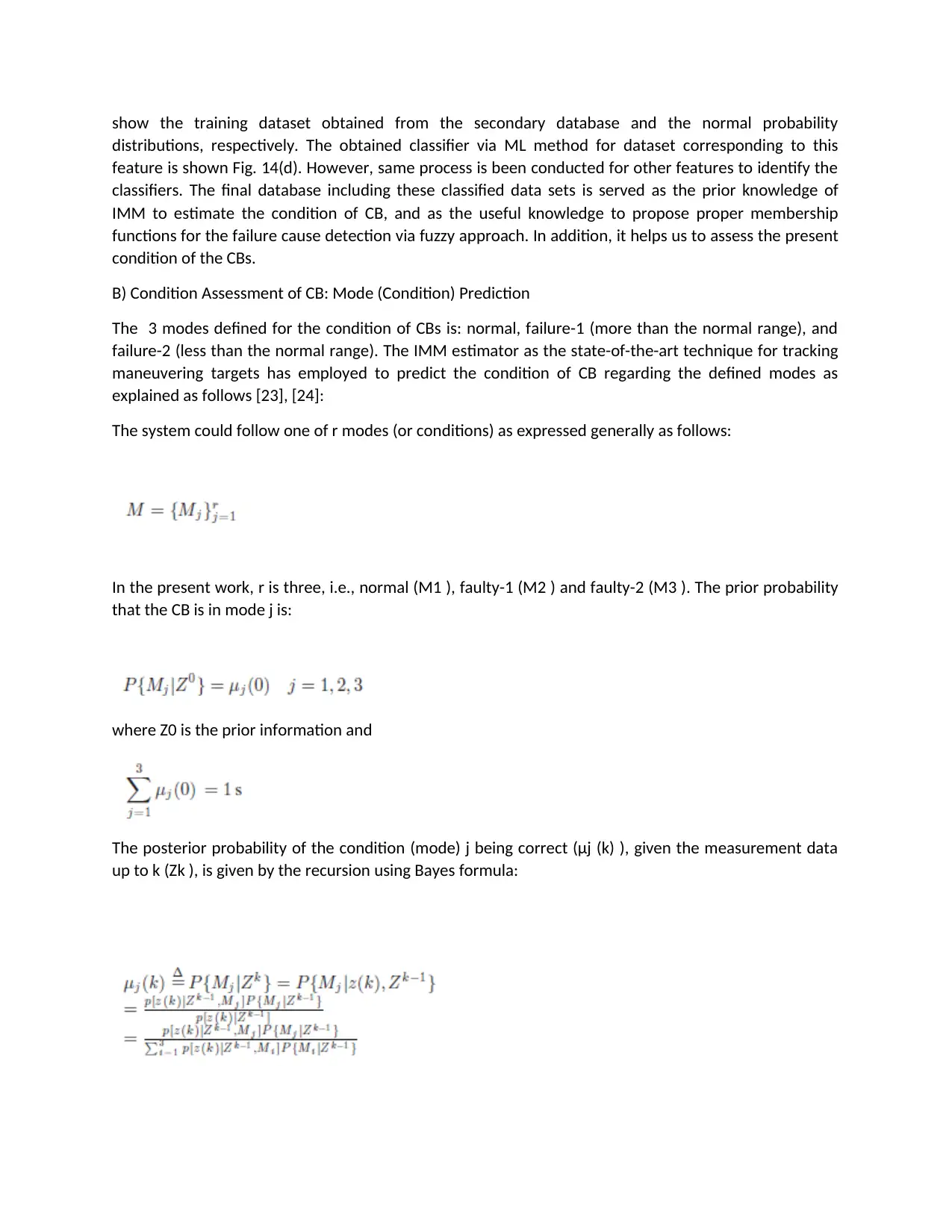
show the training dataset obtained from the secondary database and the normal probability
distributions, respectively. The obtained classifier via ML method for dataset corresponding to this
feature is shown Fig. 14(d). However, same process is been conducted for other features to identify the
classifiers. The final database including these classified data sets is served as the prior knowledge of
IMM to estimate the condition of CB, and as the useful knowledge to propose proper membership
functions for the failure cause detection via fuzzy approach. In addition, it helps us to assess the present
condition of the CBs.
B) Condition Assessment of CB: Mode (Condition) Prediction
The 3 modes defined for the condition of CBs is: normal, failure-1 (more than the normal range), and
failure-2 (less than the normal range). The IMM estimator as the state-of-the-art technique for tracking
maneuvering targets has employed to predict the condition of CB regarding the defined modes as
explained as follows [23], [24]:
The system could follow one of r modes (or conditions) as expressed generally as follows:
In the present work, r is three, i.e., normal (M1 ), faulty-1 (M2 ) and faulty-2 (M3 ). The prior probability
that the CB is in mode j is:
where Z0 is the prior information and
The posterior probability of the condition (mode) j being correct (μj (k) ), given the measurement data
up to k (Zk ), is given by the recursion using Bayes formula:
distributions, respectively. The obtained classifier via ML method for dataset corresponding to this
feature is shown Fig. 14(d). However, same process is been conducted for other features to identify the
classifiers. The final database including these classified data sets is served as the prior knowledge of
IMM to estimate the condition of CB, and as the useful knowledge to propose proper membership
functions for the failure cause detection via fuzzy approach. In addition, it helps us to assess the present
condition of the CBs.
B) Condition Assessment of CB: Mode (Condition) Prediction
The 3 modes defined for the condition of CBs is: normal, failure-1 (more than the normal range), and
failure-2 (less than the normal range). The IMM estimator as the state-of-the-art technique for tracking
maneuvering targets has employed to predict the condition of CB regarding the defined modes as
explained as follows [23], [24]:
The system could follow one of r modes (or conditions) as expressed generally as follows:
In the present work, r is three, i.e., normal (M1 ), faulty-1 (M2 ) and faulty-2 (M3 ). The prior probability
that the CB is in mode j is:
where Z0 is the prior information and
The posterior probability of the condition (mode) j being correct (μj (k) ), given the measurement data
up to k (Zk ), is given by the recursion using Bayes formula:
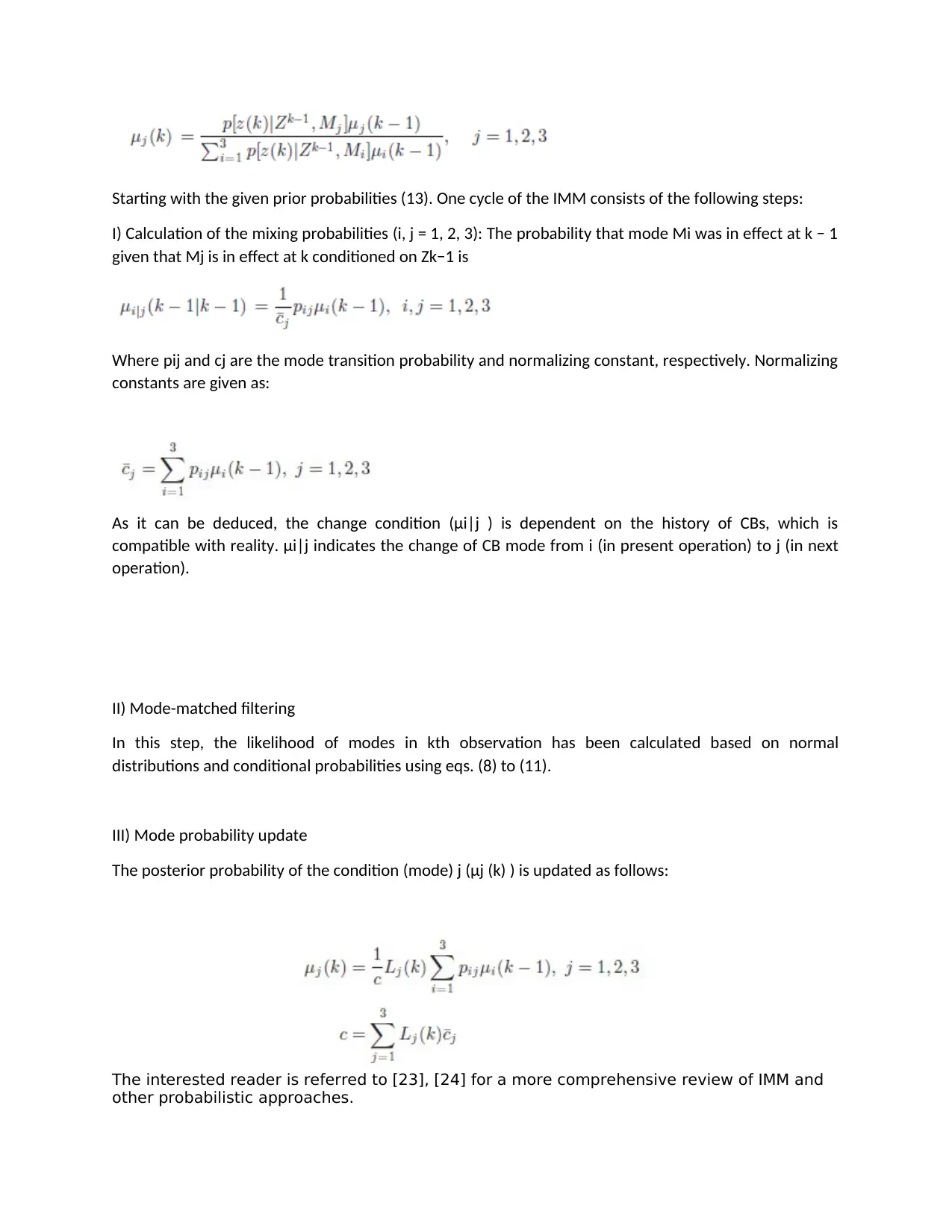
Starting with the given prior probabilities (13). One cycle of the IMM consists of the following steps:
I) Calculation of the mixing probabilities (i, j = 1, 2, 3): The probability that mode Mi was in effect at k − 1
given that Mj is in effect at k conditioned on Zk−1 is
Where pij and cj are the mode transition probability and normalizing constant, respectively. Normalizing
constants are given as:
As it can be deduced, the change condition (μi|j ) is dependent on the history of CBs, which is
compatible with reality. μi|j indicates the change of CB mode from i (in present operation) to j (in next
operation).
II) Mode-matched filtering
In this step, the likelihood of modes in kth observation has been calculated based on normal
distributions and conditional probabilities using eqs. (8) to (11).
III) Mode probability update
The posterior probability of the condition (mode) j (μj (k) ) is updated as follows:
The interested reader is referred to [23], [24] for a more comprehensive review of IMM and
other probabilistic approaches.
I) Calculation of the mixing probabilities (i, j = 1, 2, 3): The probability that mode Mi was in effect at k − 1
given that Mj is in effect at k conditioned on Zk−1 is
Where pij and cj are the mode transition probability and normalizing constant, respectively. Normalizing
constants are given as:
As it can be deduced, the change condition (μi|j ) is dependent on the history of CBs, which is
compatible with reality. μi|j indicates the change of CB mode from i (in present operation) to j (in next
operation).
II) Mode-matched filtering
In this step, the likelihood of modes in kth observation has been calculated based on normal
distributions and conditional probabilities using eqs. (8) to (11).
III) Mode probability update
The posterior probability of the condition (mode) j (μj (k) ) is updated as follows:
The interested reader is referred to [23], [24] for a more comprehensive review of IMM and
other probabilistic approaches.
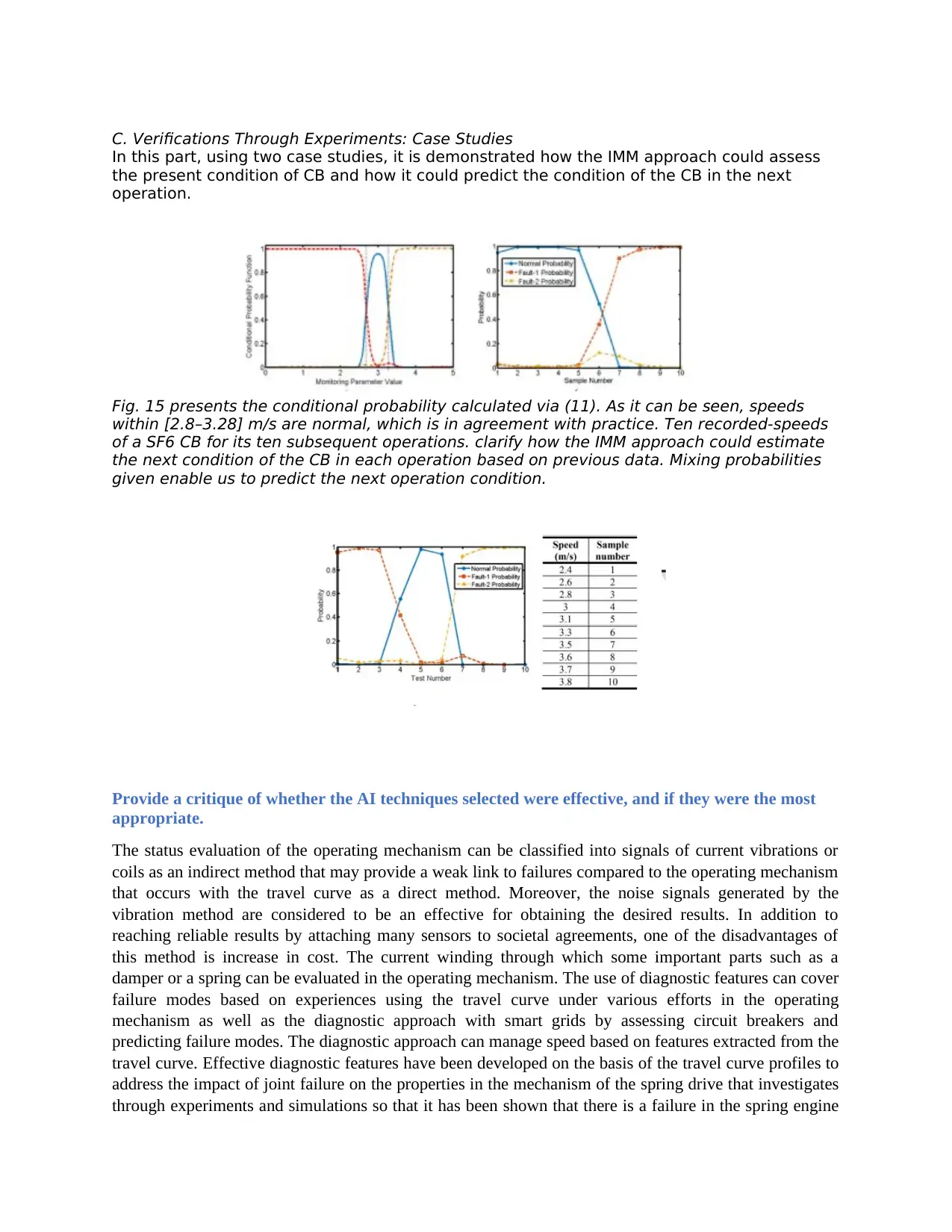
C. Verifications Through Experiments: Case Studies
In this part, using two case studies, it is demonstrated how the IMM approach could assess
the present condition of CB and how it could predict the condition of the CB in the next
operation.
Fig. 15 presents the conditional probability calculated via (11). As it can be seen, speeds
within [2.8–3.28] m/s are normal, which is in agreement with practice. Ten recorded-speeds
of a SF6 CB for its ten subsequent operations. clarify how the IMM approach could estimate
the next condition of the CB in each operation based on previous data. Mixing probabilities
given enable us to predict the next operation condition.
Provide a critique of whether the AI techniques selected were effective, and if they were the most
appropriate.
The status evaluation of the operating mechanism can be classified into signals of current vibrations or
coils as an indirect method that may provide a weak link to failures compared to the operating mechanism
that occurs with the travel curve as a direct method. Moreover, the noise signals generated by the
vibration method are considered to be an effective for obtaining the desired results. In addition to
reaching reliable results by attaching many sensors to societal agreements, one of the disadvantages of
this method is increase in cost. The current winding through which some important parts such as a
damper or a spring can be evaluated in the operating mechanism. The use of diagnostic features can cover
failure modes based on experiences using the travel curve under various efforts in the operating
mechanism as well as the diagnostic approach with smart grids by assessing circuit breakers and
predicting failure modes. The diagnostic approach can manage speed based on features extracted from the
travel curve. Effective diagnostic features have been developed on the basis of the travel curve profiles to
address the impact of joint failure on the properties in the mechanism of the spring drive that investigates
through experiments and simulations so that it has been shown that there is a failure in the spring engine
In this part, using two case studies, it is demonstrated how the IMM approach could assess
the present condition of CB and how it could predict the condition of the CB in the next
operation.
Fig. 15 presents the conditional probability calculated via (11). As it can be seen, speeds
within [2.8–3.28] m/s are normal, which is in agreement with practice. Ten recorded-speeds
of a SF6 CB for its ten subsequent operations. clarify how the IMM approach could estimate
the next condition of the CB in each operation based on previous data. Mixing probabilities
given enable us to predict the next operation condition.
Provide a critique of whether the AI techniques selected were effective, and if they were the most
appropriate.
The status evaluation of the operating mechanism can be classified into signals of current vibrations or
coils as an indirect method that may provide a weak link to failures compared to the operating mechanism
that occurs with the travel curve as a direct method. Moreover, the noise signals generated by the
vibration method are considered to be an effective for obtaining the desired results. In addition to
reaching reliable results by attaching many sensors to societal agreements, one of the disadvantages of
this method is increase in cost. The current winding through which some important parts such as a
damper or a spring can be evaluated in the operating mechanism. The use of diagnostic features can cover
failure modes based on experiences using the travel curve under various efforts in the operating
mechanism as well as the diagnostic approach with smart grids by assessing circuit breakers and
predicting failure modes. The diagnostic approach can manage speed based on features extracted from the
travel curve. Effective diagnostic features have been developed on the basis of the travel curve profiles to
address the impact of joint failure on the properties in the mechanism of the spring drive that investigates
through experiments and simulations so that it has been shown that there is a failure in the spring engine
Paraphrase This Document
Need a fresh take? Get an instant paraphrase of this document with our AI Paraphraser
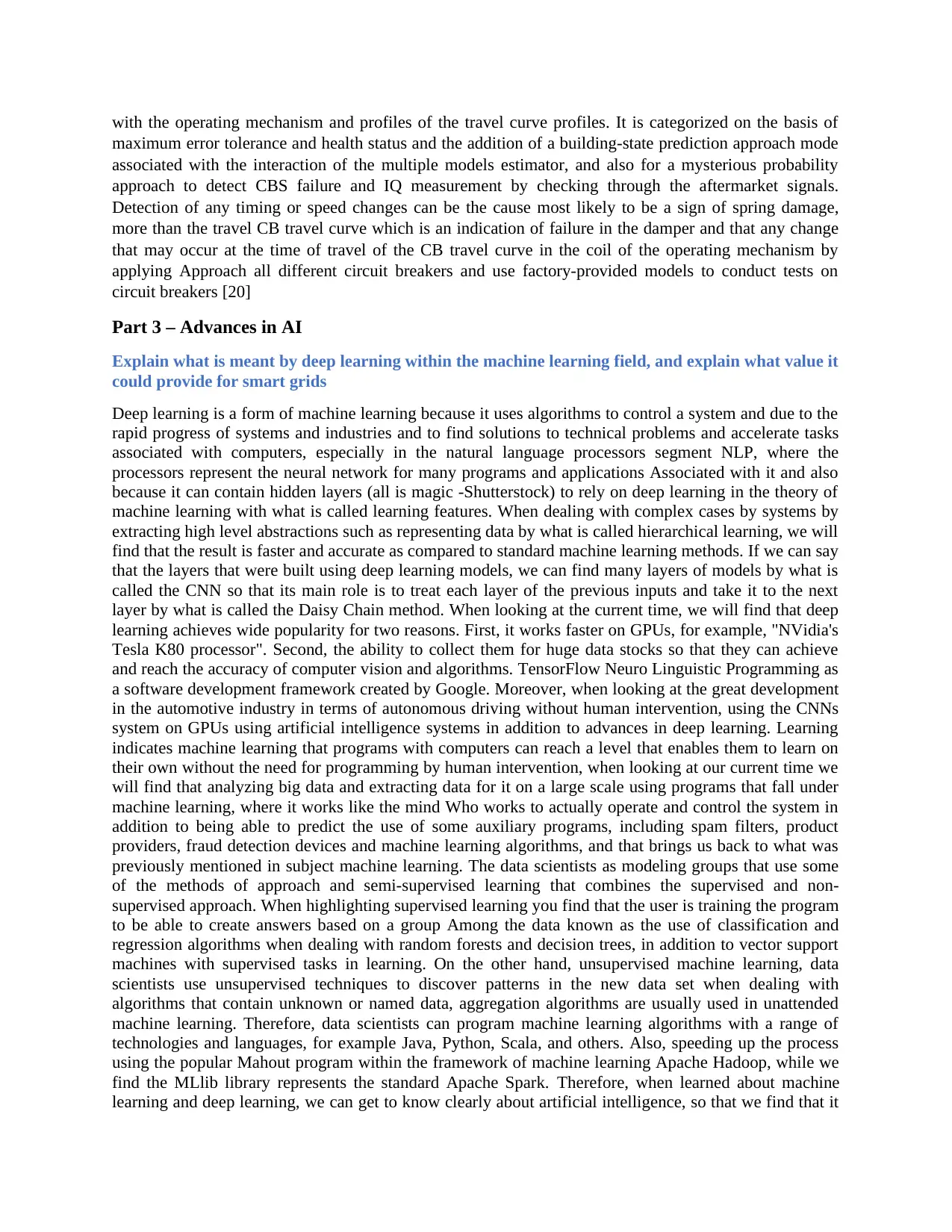
with the operating mechanism and profiles of the travel curve profiles. It is categorized on the basis of
maximum error tolerance and health status and the addition of a building-state prediction approach mode
associated with the interaction of the multiple models estimator, and also for a mysterious probability
approach to detect CBS failure and IQ measurement by checking through the aftermarket signals.
Detection of any timing or speed changes can be the cause most likely to be a sign of spring damage,
more than the travel CB travel curve which is an indication of failure in the damper and that any change
that may occur at the time of travel of the CB travel curve in the coil of the operating mechanism by
applying Approach all different circuit breakers and use factory-provided models to conduct tests on
circuit breakers [20]
Part 3 – Advances in AI
Explain what is meant by deep learning within the machine learning field, and explain what value it
could provide for smart grids
Deep learning is a form of machine learning because it uses algorithms to control a system and due to the
rapid progress of systems and industries and to find solutions to technical problems and accelerate tasks
associated with computers, especially in the natural language processors segment NLP, where the
processors represent the neural network for many programs and applications Associated with it and also
because it can contain hidden layers (all is magic -Shutterstock) to rely on deep learning in the theory of
machine learning with what is called learning features. When dealing with complex cases by systems by
extracting high level abstractions such as representing data by what is called hierarchical learning, we will
find that the result is faster and accurate as compared to standard machine learning methods. If we can say
that the layers that were built using deep learning models, we can find many layers of models by what is
called the CNN so that its main role is to treat each layer of the previous inputs and take it to the next
layer by what is called the Daisy Chain method. When looking at the current time, we will find that deep
learning achieves wide popularity for two reasons. First, it works faster on GPUs, for example, "NVidia's
Tesla K80 processor". Second, the ability to collect them for huge data stocks so that they can achieve
and reach the accuracy of computer vision and algorithms. TensorFlow Neuro Linguistic Programming as
a software development framework created by Google. Moreover, when looking at the great development
in the automotive industry in terms of autonomous driving without human intervention, using the CNNs
system on GPUs using artificial intelligence systems in addition to advances in deep learning. Learning
indicates machine learning that programs with computers can reach a level that enables them to learn on
their own without the need for programming by human intervention, when looking at our current time we
will find that analyzing big data and extracting data for it on a large scale using programs that fall under
machine learning, where it works like the mind Who works to actually operate and control the system in
addition to being able to predict the use of some auxiliary programs, including spam filters, product
providers, fraud detection devices and machine learning algorithms, and that brings us back to what was
previously mentioned in subject machine learning. The data scientists as modeling groups that use some
of the methods of approach and semi-supervised learning that combines the supervised and non-
supervised approach. When highlighting supervised learning you find that the user is training the program
to be able to create answers based on a group Among the data known as the use of classification and
regression algorithms when dealing with random forests and decision trees, in addition to vector support
machines with supervised tasks in learning. On the other hand, unsupervised machine learning, data
scientists use unsupervised techniques to discover patterns in the new data set when dealing with
algorithms that contain unknown or named data, aggregation algorithms are usually used in unattended
machine learning. Therefore, data scientists can program machine learning algorithms with a range of
technologies and languages, for example Java, Python, Scala, and others. Also, speeding up the process
using the popular Mahout program within the framework of machine learning Apache Hadoop, while we
find the MLlib library represents the standard Apache Spark. Therefore, when learned about machine
learning and deep learning, we can get to know clearly about artificial intelligence, so that we find that it
maximum error tolerance and health status and the addition of a building-state prediction approach mode
associated with the interaction of the multiple models estimator, and also for a mysterious probability
approach to detect CBS failure and IQ measurement by checking through the aftermarket signals.
Detection of any timing or speed changes can be the cause most likely to be a sign of spring damage,
more than the travel CB travel curve which is an indication of failure in the damper and that any change
that may occur at the time of travel of the CB travel curve in the coil of the operating mechanism by
applying Approach all different circuit breakers and use factory-provided models to conduct tests on
circuit breakers [20]
Part 3 – Advances in AI
Explain what is meant by deep learning within the machine learning field, and explain what value it
could provide for smart grids
Deep learning is a form of machine learning because it uses algorithms to control a system and due to the
rapid progress of systems and industries and to find solutions to technical problems and accelerate tasks
associated with computers, especially in the natural language processors segment NLP, where the
processors represent the neural network for many programs and applications Associated with it and also
because it can contain hidden layers (all is magic -Shutterstock) to rely on deep learning in the theory of
machine learning with what is called learning features. When dealing with complex cases by systems by
extracting high level abstractions such as representing data by what is called hierarchical learning, we will
find that the result is faster and accurate as compared to standard machine learning methods. If we can say
that the layers that were built using deep learning models, we can find many layers of models by what is
called the CNN so that its main role is to treat each layer of the previous inputs and take it to the next
layer by what is called the Daisy Chain method. When looking at the current time, we will find that deep
learning achieves wide popularity for two reasons. First, it works faster on GPUs, for example, "NVidia's
Tesla K80 processor". Second, the ability to collect them for huge data stocks so that they can achieve
and reach the accuracy of computer vision and algorithms. TensorFlow Neuro Linguistic Programming as
a software development framework created by Google. Moreover, when looking at the great development
in the automotive industry in terms of autonomous driving without human intervention, using the CNNs
system on GPUs using artificial intelligence systems in addition to advances in deep learning. Learning
indicates machine learning that programs with computers can reach a level that enables them to learn on
their own without the need for programming by human intervention, when looking at our current time we
will find that analyzing big data and extracting data for it on a large scale using programs that fall under
machine learning, where it works like the mind Who works to actually operate and control the system in
addition to being able to predict the use of some auxiliary programs, including spam filters, product
providers, fraud detection devices and machine learning algorithms, and that brings us back to what was
previously mentioned in subject machine learning. The data scientists as modeling groups that use some
of the methods of approach and semi-supervised learning that combines the supervised and non-
supervised approach. When highlighting supervised learning you find that the user is training the program
to be able to create answers based on a group Among the data known as the use of classification and
regression algorithms when dealing with random forests and decision trees, in addition to vector support
machines with supervised tasks in learning. On the other hand, unsupervised machine learning, data
scientists use unsupervised techniques to discover patterns in the new data set when dealing with
algorithms that contain unknown or named data, aggregation algorithms are usually used in unattended
machine learning. Therefore, data scientists can program machine learning algorithms with a range of
technologies and languages, for example Java, Python, Scala, and others. Also, speeding up the process
using the popular Mahout program within the framework of machine learning Apache Hadoop, while we
find the MLlib library represents the standard Apache Spark. Therefore, when learned about machine
learning and deep learning, we can get to know clearly about artificial intelligence, so that we find that it
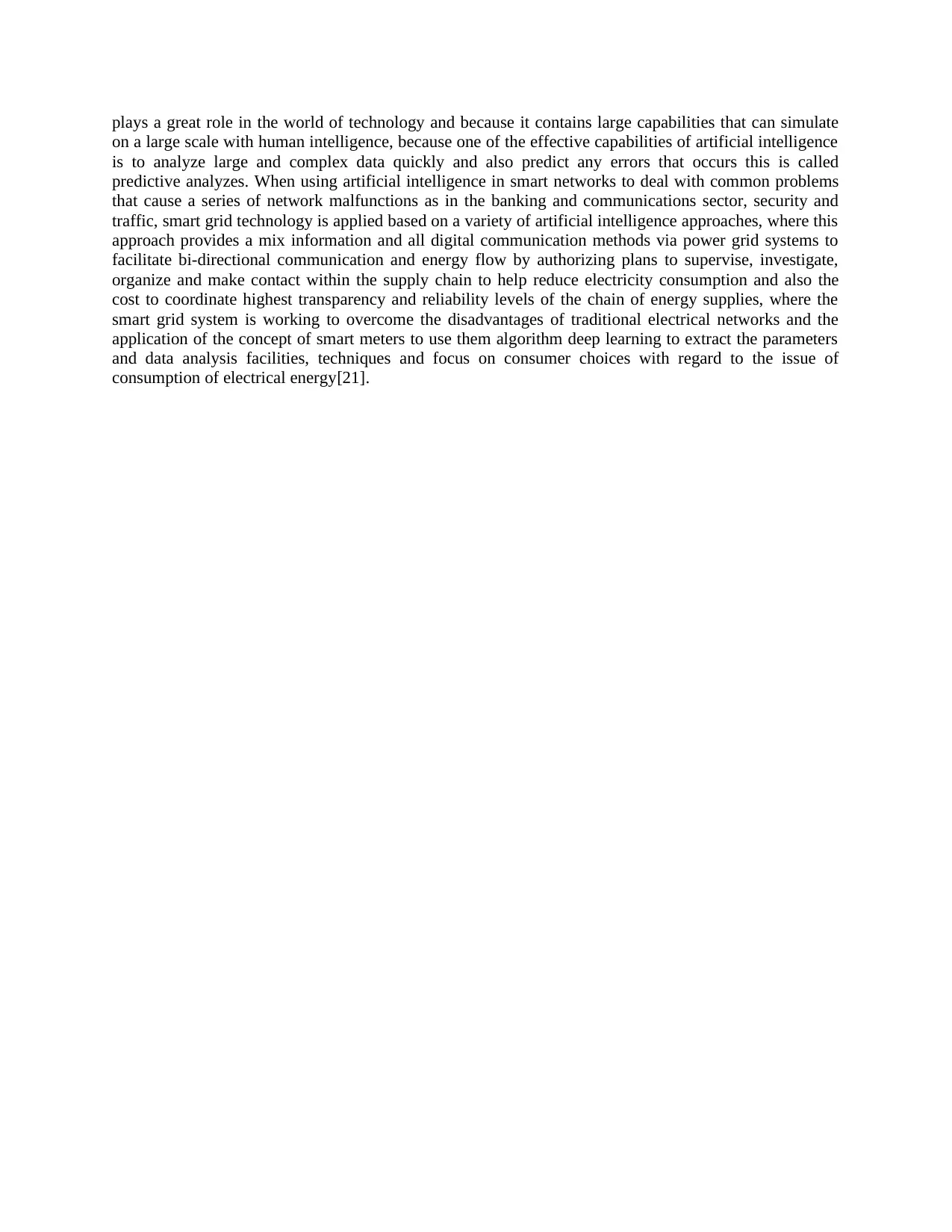
plays a great role in the world of technology and because it contains large capabilities that can simulate
on a large scale with human intelligence, because one of the effective capabilities of artificial intelligence
is to analyze large and complex data quickly and also predict any errors that occurs this is called
predictive analyzes. When using artificial intelligence in smart networks to deal with common problems
that cause a series of network malfunctions as in the banking and communications sector, security and
traffic, smart grid technology is applied based on a variety of artificial intelligence approaches, where this
approach provides a mix information and all digital communication methods via power grid systems to
facilitate bi-directional communication and energy flow by authorizing plans to supervise, investigate,
organize and make contact within the supply chain to help reduce electricity consumption and also the
cost to coordinate highest transparency and reliability levels of the chain of energy supplies, where the
smart grid system is working to overcome the disadvantages of traditional electrical networks and the
application of the concept of smart meters to use them algorithm deep learning to extract the parameters
and data analysis facilities, techniques and focus on consumer choices with regard to the issue of
consumption of electrical energy[21].
on a large scale with human intelligence, because one of the effective capabilities of artificial intelligence
is to analyze large and complex data quickly and also predict any errors that occurs this is called
predictive analyzes. When using artificial intelligence in smart networks to deal with common problems
that cause a series of network malfunctions as in the banking and communications sector, security and
traffic, smart grid technology is applied based on a variety of artificial intelligence approaches, where this
approach provides a mix information and all digital communication methods via power grid systems to
facilitate bi-directional communication and energy flow by authorizing plans to supervise, investigate,
organize and make contact within the supply chain to help reduce electricity consumption and also the
cost to coordinate highest transparency and reliability levels of the chain of energy supplies, where the
smart grid system is working to overcome the disadvantages of traditional electrical networks and the
application of the concept of smart meters to use them algorithm deep learning to extract the parameters
and data analysis facilities, techniques and focus on consumer choices with regard to the issue of
consumption of electrical energy[21].
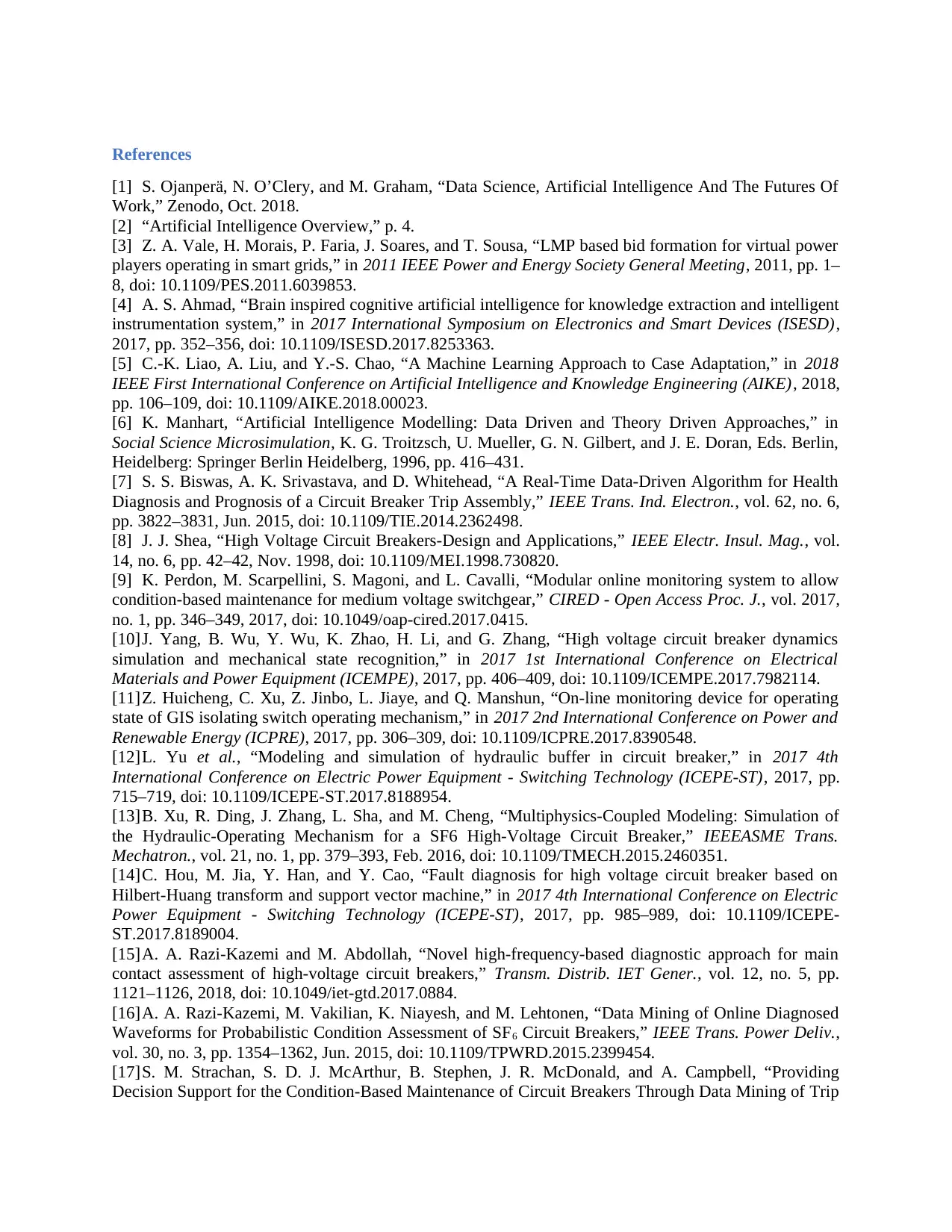
References
[1] S. Ojanperä, N. O’Clery, and M. Graham, “Data Science, Artificial Intelligence And The Futures Of
Work,” Zenodo, Oct. 2018.
[2] “Artificial Intelligence Overview,” p. 4.
[3] Z. A. Vale, H. Morais, P. Faria, J. Soares, and T. Sousa, “LMP based bid formation for virtual power
players operating in smart grids,” in 2011 IEEE Power and Energy Society General Meeting, 2011, pp. 1–
8, doi: 10.1109/PES.2011.6039853.
[4] A. S. Ahmad, “Brain inspired cognitive artificial intelligence for knowledge extraction and intelligent
instrumentation system,” in 2017 International Symposium on Electronics and Smart Devices (ISESD),
2017, pp. 352–356, doi: 10.1109/ISESD.2017.8253363.
[5] C.-K. Liao, A. Liu, and Y.-S. Chao, “A Machine Learning Approach to Case Adaptation,” in 2018
IEEE First International Conference on Artificial Intelligence and Knowledge Engineering (AIKE), 2018,
pp. 106–109, doi: 10.1109/AIKE.2018.00023.
[6] K. Manhart, “Artificial Intelligence Modelling: Data Driven and Theory Driven Approaches,” in
Social Science Microsimulation, K. G. Troitzsch, U. Mueller, G. N. Gilbert, and J. E. Doran, Eds. Berlin,
Heidelberg: Springer Berlin Heidelberg, 1996, pp. 416–431.
[7] S. S. Biswas, A. K. Srivastava, and D. Whitehead, “A Real-Time Data-Driven Algorithm for Health
Diagnosis and Prognosis of a Circuit Breaker Trip Assembly,” IEEE Trans. Ind. Electron., vol. 62, no. 6,
pp. 3822–3831, Jun. 2015, doi: 10.1109/TIE.2014.2362498.
[8] J. J. Shea, “High Voltage Circuit Breakers-Design and Applications,” IEEE Electr. Insul. Mag., vol.
14, no. 6, pp. 42–42, Nov. 1998, doi: 10.1109/MEI.1998.730820.
[9] K. Perdon, M. Scarpellini, S. Magoni, and L. Cavalli, “Modular online monitoring system to allow
condition-based maintenance for medium voltage switchgear,” CIRED - Open Access Proc. J., vol. 2017,
no. 1, pp. 346–349, 2017, doi: 10.1049/oap-cired.2017.0415.
[10]J. Yang, B. Wu, Y. Wu, K. Zhao, H. Li, and G. Zhang, “High voltage circuit breaker dynamics
simulation and mechanical state recognition,” in 2017 1st International Conference on Electrical
Materials and Power Equipment (ICEMPE), 2017, pp. 406–409, doi: 10.1109/ICEMPE.2017.7982114.
[11]Z. Huicheng, C. Xu, Z. Jinbo, L. Jiaye, and Q. Manshun, “On-line monitoring device for operating
state of GIS isolating switch operating mechanism,” in 2017 2nd International Conference on Power and
Renewable Energy (ICPRE), 2017, pp. 306–309, doi: 10.1109/ICPRE.2017.8390548.
[12]L. Yu et al., “Modeling and simulation of hydraulic buffer in circuit breaker,” in 2017 4th
International Conference on Electric Power Equipment - Switching Technology (ICEPE-ST), 2017, pp.
715–719, doi: 10.1109/ICEPE-ST.2017.8188954.
[13]B. Xu, R. Ding, J. Zhang, L. Sha, and M. Cheng, “Multiphysics-Coupled Modeling: Simulation of
the Hydraulic-Operating Mechanism for a SF6 High-Voltage Circuit Breaker,” IEEEASME Trans.
Mechatron., vol. 21, no. 1, pp. 379–393, Feb. 2016, doi: 10.1109/TMECH.2015.2460351.
[14]C. Hou, M. Jia, Y. Han, and Y. Cao, “Fault diagnosis for high voltage circuit breaker based on
Hilbert-Huang transform and support vector machine,” in 2017 4th International Conference on Electric
Power Equipment - Switching Technology (ICEPE-ST), 2017, pp. 985–989, doi: 10.1109/ICEPE-
ST.2017.8189004.
[15]A. A. Razi-Kazemi and M. Abdollah, “Novel high-frequency-based diagnostic approach for main
contact assessment of high-voltage circuit breakers,” Transm. Distrib. IET Gener., vol. 12, no. 5, pp.
1121–1126, 2018, doi: 10.1049/iet-gtd.2017.0884.
[16]A. A. Razi-Kazemi, M. Vakilian, K. Niayesh, and M. Lehtonen, “Data Mining of Online Diagnosed
Waveforms for Probabilistic Condition Assessment of SF6 Circuit Breakers,” IEEE Trans. Power Deliv.,
vol. 30, no. 3, pp. 1354–1362, Jun. 2015, doi: 10.1109/TPWRD.2015.2399454.
[17]S. M. Strachan, S. D. J. McArthur, B. Stephen, J. R. McDonald, and A. Campbell, “Providing
Decision Support for the Condition-Based Maintenance of Circuit Breakers Through Data Mining of Trip
[1] S. Ojanperä, N. O’Clery, and M. Graham, “Data Science, Artificial Intelligence And The Futures Of
Work,” Zenodo, Oct. 2018.
[2] “Artificial Intelligence Overview,” p. 4.
[3] Z. A. Vale, H. Morais, P. Faria, J. Soares, and T. Sousa, “LMP based bid formation for virtual power
players operating in smart grids,” in 2011 IEEE Power and Energy Society General Meeting, 2011, pp. 1–
8, doi: 10.1109/PES.2011.6039853.
[4] A. S. Ahmad, “Brain inspired cognitive artificial intelligence for knowledge extraction and intelligent
instrumentation system,” in 2017 International Symposium on Electronics and Smart Devices (ISESD),
2017, pp. 352–356, doi: 10.1109/ISESD.2017.8253363.
[5] C.-K. Liao, A. Liu, and Y.-S. Chao, “A Machine Learning Approach to Case Adaptation,” in 2018
IEEE First International Conference on Artificial Intelligence and Knowledge Engineering (AIKE), 2018,
pp. 106–109, doi: 10.1109/AIKE.2018.00023.
[6] K. Manhart, “Artificial Intelligence Modelling: Data Driven and Theory Driven Approaches,” in
Social Science Microsimulation, K. G. Troitzsch, U. Mueller, G. N. Gilbert, and J. E. Doran, Eds. Berlin,
Heidelberg: Springer Berlin Heidelberg, 1996, pp. 416–431.
[7] S. S. Biswas, A. K. Srivastava, and D. Whitehead, “A Real-Time Data-Driven Algorithm for Health
Diagnosis and Prognosis of a Circuit Breaker Trip Assembly,” IEEE Trans. Ind. Electron., vol. 62, no. 6,
pp. 3822–3831, Jun. 2015, doi: 10.1109/TIE.2014.2362498.
[8] J. J. Shea, “High Voltage Circuit Breakers-Design and Applications,” IEEE Electr. Insul. Mag., vol.
14, no. 6, pp. 42–42, Nov. 1998, doi: 10.1109/MEI.1998.730820.
[9] K. Perdon, M. Scarpellini, S. Magoni, and L. Cavalli, “Modular online monitoring system to allow
condition-based maintenance for medium voltage switchgear,” CIRED - Open Access Proc. J., vol. 2017,
no. 1, pp. 346–349, 2017, doi: 10.1049/oap-cired.2017.0415.
[10]J. Yang, B. Wu, Y. Wu, K. Zhao, H. Li, and G. Zhang, “High voltage circuit breaker dynamics
simulation and mechanical state recognition,” in 2017 1st International Conference on Electrical
Materials and Power Equipment (ICEMPE), 2017, pp. 406–409, doi: 10.1109/ICEMPE.2017.7982114.
[11]Z. Huicheng, C. Xu, Z. Jinbo, L. Jiaye, and Q. Manshun, “On-line monitoring device for operating
state of GIS isolating switch operating mechanism,” in 2017 2nd International Conference on Power and
Renewable Energy (ICPRE), 2017, pp. 306–309, doi: 10.1109/ICPRE.2017.8390548.
[12]L. Yu et al., “Modeling and simulation of hydraulic buffer in circuit breaker,” in 2017 4th
International Conference on Electric Power Equipment - Switching Technology (ICEPE-ST), 2017, pp.
715–719, doi: 10.1109/ICEPE-ST.2017.8188954.
[13]B. Xu, R. Ding, J. Zhang, L. Sha, and M. Cheng, “Multiphysics-Coupled Modeling: Simulation of
the Hydraulic-Operating Mechanism for a SF6 High-Voltage Circuit Breaker,” IEEEASME Trans.
Mechatron., vol. 21, no. 1, pp. 379–393, Feb. 2016, doi: 10.1109/TMECH.2015.2460351.
[14]C. Hou, M. Jia, Y. Han, and Y. Cao, “Fault diagnosis for high voltage circuit breaker based on
Hilbert-Huang transform and support vector machine,” in 2017 4th International Conference on Electric
Power Equipment - Switching Technology (ICEPE-ST), 2017, pp. 985–989, doi: 10.1109/ICEPE-
ST.2017.8189004.
[15]A. A. Razi-Kazemi and M. Abdollah, “Novel high-frequency-based diagnostic approach for main
contact assessment of high-voltage circuit breakers,” Transm. Distrib. IET Gener., vol. 12, no. 5, pp.
1121–1126, 2018, doi: 10.1049/iet-gtd.2017.0884.
[16]A. A. Razi-Kazemi, M. Vakilian, K. Niayesh, and M. Lehtonen, “Data Mining of Online Diagnosed
Waveforms for Probabilistic Condition Assessment of SF6 Circuit Breakers,” IEEE Trans. Power Deliv.,
vol. 30, no. 3, pp. 1354–1362, Jun. 2015, doi: 10.1109/TPWRD.2015.2399454.
[17]S. M. Strachan, S. D. J. McArthur, B. Stephen, J. R. McDonald, and A. Campbell, “Providing
Decision Support for the Condition-Based Maintenance of Circuit Breakers Through Data Mining of Trip
Secure Best Marks with AI Grader
Need help grading? Try our AI Grader for instant feedback on your assignments.
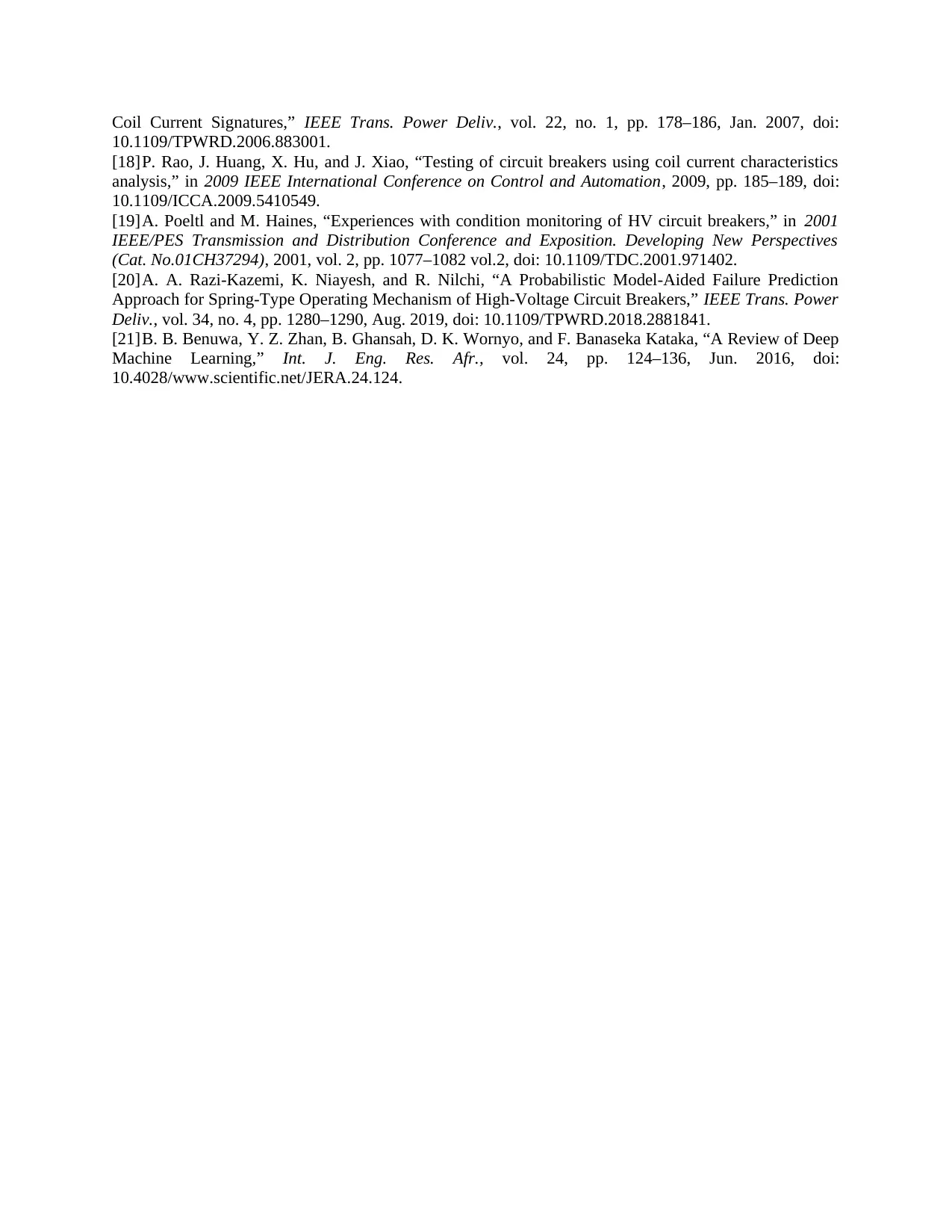
Coil Current Signatures,” IEEE Trans. Power Deliv., vol. 22, no. 1, pp. 178–186, Jan. 2007, doi:
10.1109/TPWRD.2006.883001.
[18]P. Rao, J. Huang, X. Hu, and J. Xiao, “Testing of circuit breakers using coil current characteristics
analysis,” in 2009 IEEE International Conference on Control and Automation, 2009, pp. 185–189, doi:
10.1109/ICCA.2009.5410549.
[19]A. Poeltl and M. Haines, “Experiences with condition monitoring of HV circuit breakers,” in 2001
IEEE/PES Transmission and Distribution Conference and Exposition. Developing New Perspectives
(Cat. No.01CH37294), 2001, vol. 2, pp. 1077–1082 vol.2, doi: 10.1109/TDC.2001.971402.
[20]A. A. Razi-Kazemi, K. Niayesh, and R. Nilchi, “A Probabilistic Model-Aided Failure Prediction
Approach for Spring-Type Operating Mechanism of High-Voltage Circuit Breakers,” IEEE Trans. Power
Deliv., vol. 34, no. 4, pp. 1280–1290, Aug. 2019, doi: 10.1109/TPWRD.2018.2881841.
[21]B. B. Benuwa, Y. Z. Zhan, B. Ghansah, D. K. Wornyo, and F. Banaseka Kataka, “A Review of Deep
Machine Learning,” Int. J. Eng. Res. Afr., vol. 24, pp. 124–136, Jun. 2016, doi:
10.4028/www.scientific.net/JERA.24.124.
10.1109/TPWRD.2006.883001.
[18]P. Rao, J. Huang, X. Hu, and J. Xiao, “Testing of circuit breakers using coil current characteristics
analysis,” in 2009 IEEE International Conference on Control and Automation, 2009, pp. 185–189, doi:
10.1109/ICCA.2009.5410549.
[19]A. Poeltl and M. Haines, “Experiences with condition monitoring of HV circuit breakers,” in 2001
IEEE/PES Transmission and Distribution Conference and Exposition. Developing New Perspectives
(Cat. No.01CH37294), 2001, vol. 2, pp. 1077–1082 vol.2, doi: 10.1109/TDC.2001.971402.
[20]A. A. Razi-Kazemi, K. Niayesh, and R. Nilchi, “A Probabilistic Model-Aided Failure Prediction
Approach for Spring-Type Operating Mechanism of High-Voltage Circuit Breakers,” IEEE Trans. Power
Deliv., vol. 34, no. 4, pp. 1280–1290, Aug. 2019, doi: 10.1109/TPWRD.2018.2881841.
[21]B. B. Benuwa, Y. Z. Zhan, B. Ghansah, D. K. Wornyo, and F. Banaseka Kataka, “A Review of Deep
Machine Learning,” Int. J. Eng. Res. Afr., vol. 24, pp. 124–136, Jun. 2016, doi:
10.4028/www.scientific.net/JERA.24.124.
1 out of 11
Related Documents
![[object Object]](/_next/image/?url=%2F_next%2Fstatic%2Fmedia%2Flogo.6d15ce61.png&w=640&q=75)
Your All-in-One AI-Powered Toolkit for Academic Success.
+13062052269
info@desklib.com
Available 24*7 on WhatsApp / Email
Unlock your academic potential
© 2024 | Zucol Services PVT LTD | All rights reserved.