Data Mining and Predictive Analysis
VerifiedAdded on 2022/12/16
|8
|1598
|447
AI Summary
This document provides an overview of data mining and predictive analysis techniques. It explains how data mining involves analyzing large data sets to identify patterns and generate new information. The document also discusses the use of data sets and data mining tools for flagging data that does not meet review or human intervention. Examples from public databases, such as the US government's open data website, are used to illustrate the application of data mining in different fields. The document also mentions the use of data mining techniques in the United Parcel Service (UPS) for efficient data analysis and prediction in their operations.
Contribute Materials
Your contribution can guide someone’s learning journey. Share your
documents today.
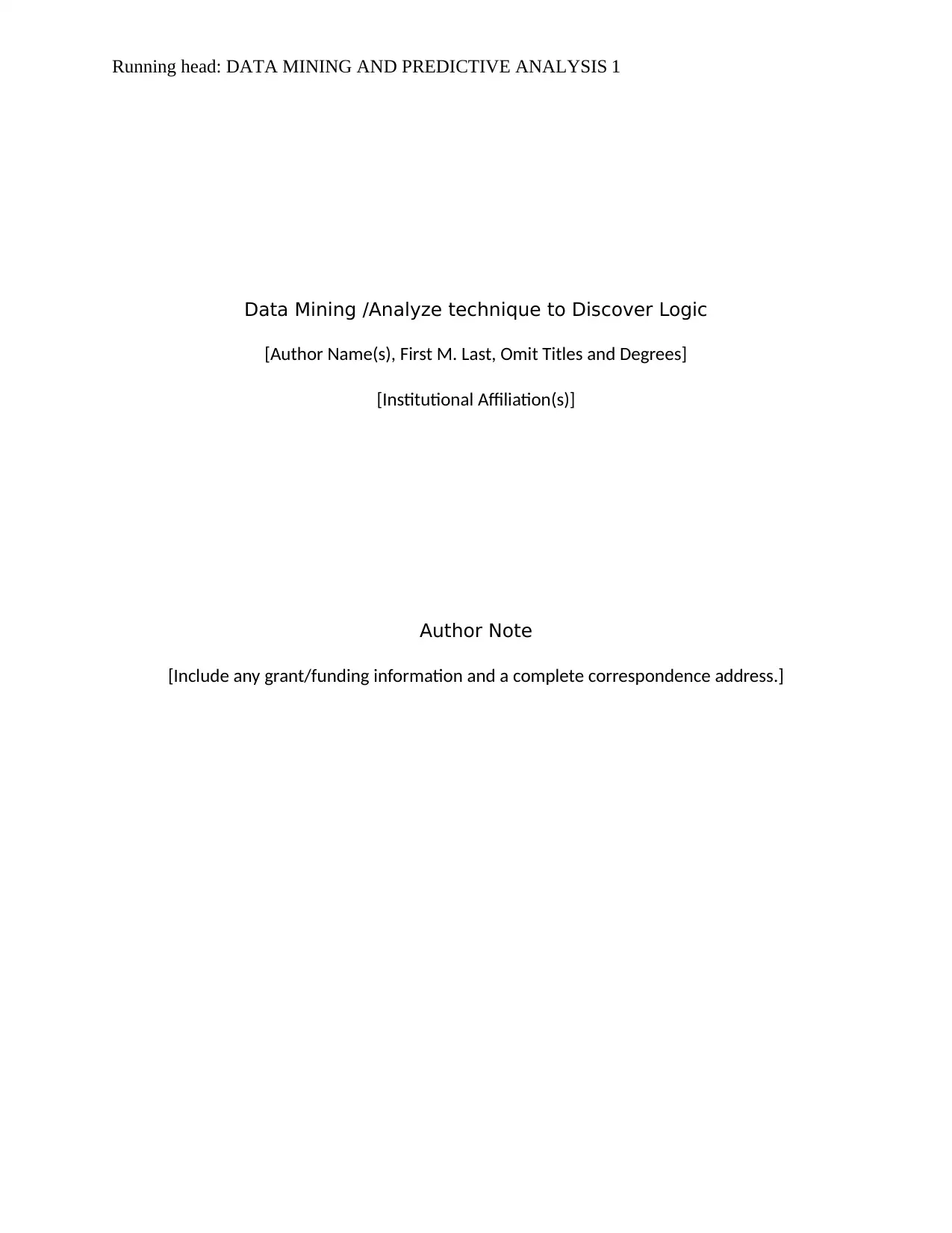
Running head: DATA MINING AND PREDICTIVE ANALYSIS 1
Data Mining /Analyze technique to Discover Logic
[Author Name(s), First M. Last, Omit Titles and Degrees]
[Institutional Affiliation(s)]
Author Note
[Include any grant/funding information and a complete correspondence address.]
Data Mining /Analyze technique to Discover Logic
[Author Name(s), First M. Last, Omit Titles and Degrees]
[Institutional Affiliation(s)]
Author Note
[Include any grant/funding information and a complete correspondence address.]
Secure Best Marks with AI Grader
Need help grading? Try our AI Grader for instant feedback on your assignments.
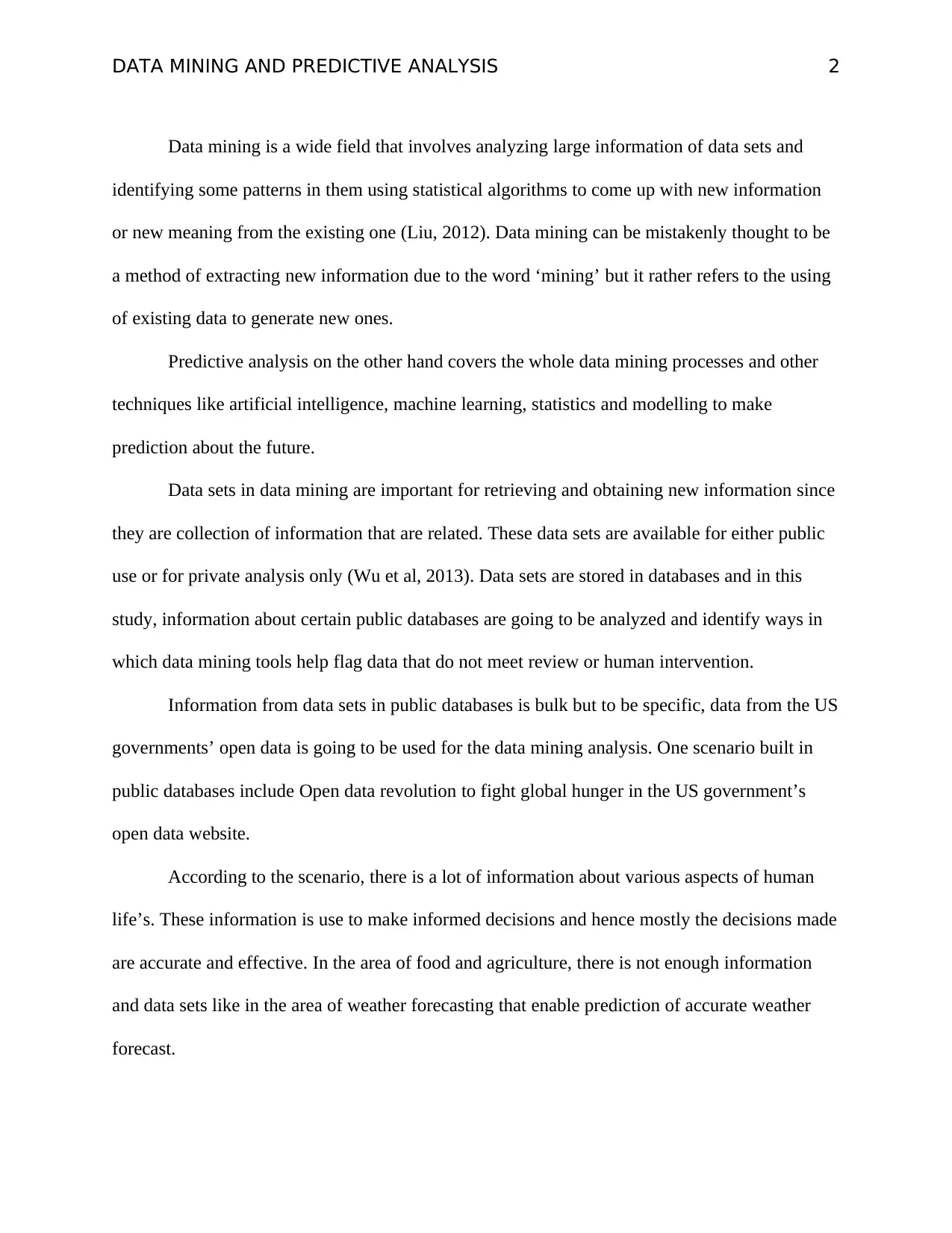
DATA MINING AND PREDICTIVE ANALYSIS 2
Data mining is a wide field that involves analyzing large information of data sets and
identifying some patterns in them using statistical algorithms to come up with new information
or new meaning from the existing one (Liu, 2012). Data mining can be mistakenly thought to be
a method of extracting new information due to the word ‘mining’ but it rather refers to the using
of existing data to generate new ones.
Predictive analysis on the other hand covers the whole data mining processes and other
techniques like artificial intelligence, machine learning, statistics and modelling to make
prediction about the future.
Data sets in data mining are important for retrieving and obtaining new information since
they are collection of information that are related. These data sets are available for either public
use or for private analysis only (Wu et al, 2013). Data sets are stored in databases and in this
study, information about certain public databases are going to be analyzed and identify ways in
which data mining tools help flag data that do not meet review or human intervention.
Information from data sets in public databases is bulk but to be specific, data from the US
governments’ open data is going to be used for the data mining analysis. One scenario built in
public databases include Open data revolution to fight global hunger in the US government’s
open data website.
According to the scenario, there is a lot of information about various aspects of human
life’s. These information is use to make informed decisions and hence mostly the decisions made
are accurate and effective. In the area of food and agriculture, there is not enough information
and data sets like in the area of weather forecasting that enable prediction of accurate weather
forecast.
Data mining is a wide field that involves analyzing large information of data sets and
identifying some patterns in them using statistical algorithms to come up with new information
or new meaning from the existing one (Liu, 2012). Data mining can be mistakenly thought to be
a method of extracting new information due to the word ‘mining’ but it rather refers to the using
of existing data to generate new ones.
Predictive analysis on the other hand covers the whole data mining processes and other
techniques like artificial intelligence, machine learning, statistics and modelling to make
prediction about the future.
Data sets in data mining are important for retrieving and obtaining new information since
they are collection of information that are related. These data sets are available for either public
use or for private analysis only (Wu et al, 2013). Data sets are stored in databases and in this
study, information about certain public databases are going to be analyzed and identify ways in
which data mining tools help flag data that do not meet review or human intervention.
Information from data sets in public databases is bulk but to be specific, data from the US
governments’ open data is going to be used for the data mining analysis. One scenario built in
public databases include Open data revolution to fight global hunger in the US government’s
open data website.
According to the scenario, there is a lot of information about various aspects of human
life’s. These information is use to make informed decisions and hence mostly the decisions made
are accurate and effective. In the area of food and agriculture, there is not enough information
and data sets like in the area of weather forecasting that enable prediction of accurate weather
forecast.
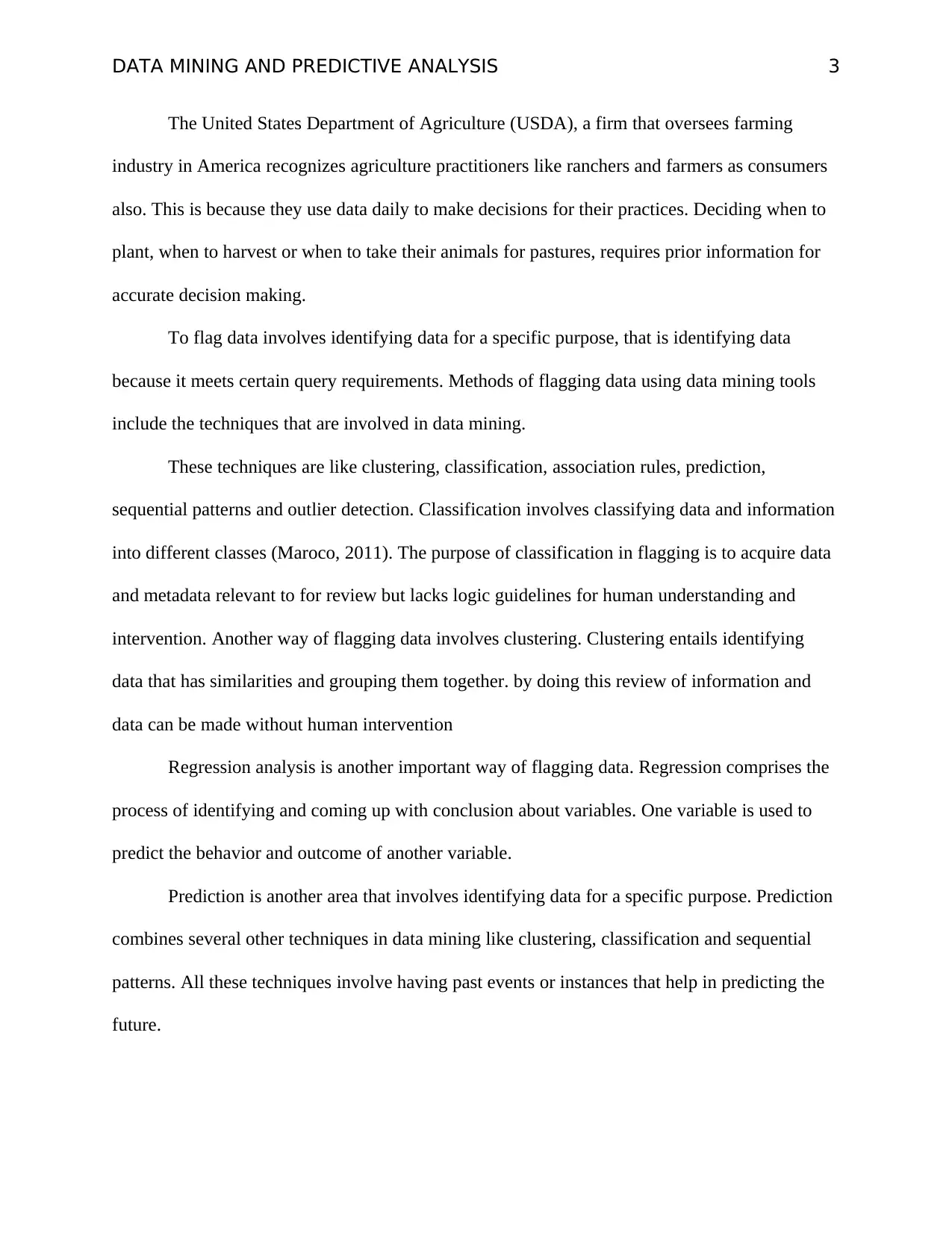
DATA MINING AND PREDICTIVE ANALYSIS 3
The United States Department of Agriculture (USDA), a firm that oversees farming
industry in America recognizes agriculture practitioners like ranchers and farmers as consumers
also. This is because they use data daily to make decisions for their practices. Deciding when to
plant, when to harvest or when to take their animals for pastures, requires prior information for
accurate decision making.
To flag data involves identifying data for a specific purpose, that is identifying data
because it meets certain query requirements. Methods of flagging data using data mining tools
include the techniques that are involved in data mining.
These techniques are like clustering, classification, association rules, prediction,
sequential patterns and outlier detection. Classification involves classifying data and information
into different classes (Maroco, 2011). The purpose of classification in flagging is to acquire data
and metadata relevant to for review but lacks logic guidelines for human understanding and
intervention. Another way of flagging data involves clustering. Clustering entails identifying
data that has similarities and grouping them together. by doing this review of information and
data can be made without human intervention
Regression analysis is another important way of flagging data. Regression comprises the
process of identifying and coming up with conclusion about variables. One variable is used to
predict the behavior and outcome of another variable.
Prediction is another area that involves identifying data for a specific purpose. Prediction
combines several other techniques in data mining like clustering, classification and sequential
patterns. All these techniques involve having past events or instances that help in predicting the
future.
The United States Department of Agriculture (USDA), a firm that oversees farming
industry in America recognizes agriculture practitioners like ranchers and farmers as consumers
also. This is because they use data daily to make decisions for their practices. Deciding when to
plant, when to harvest or when to take their animals for pastures, requires prior information for
accurate decision making.
To flag data involves identifying data for a specific purpose, that is identifying data
because it meets certain query requirements. Methods of flagging data using data mining tools
include the techniques that are involved in data mining.
These techniques are like clustering, classification, association rules, prediction,
sequential patterns and outlier detection. Classification involves classifying data and information
into different classes (Maroco, 2011). The purpose of classification in flagging is to acquire data
and metadata relevant to for review but lacks logic guidelines for human understanding and
intervention. Another way of flagging data involves clustering. Clustering entails identifying
data that has similarities and grouping them together. by doing this review of information and
data can be made without human intervention
Regression analysis is another important way of flagging data. Regression comprises the
process of identifying and coming up with conclusion about variables. One variable is used to
predict the behavior and outcome of another variable.
Prediction is another area that involves identifying data for a specific purpose. Prediction
combines several other techniques in data mining like clustering, classification and sequential
patterns. All these techniques involve having past events or instances that help in predicting the
future.
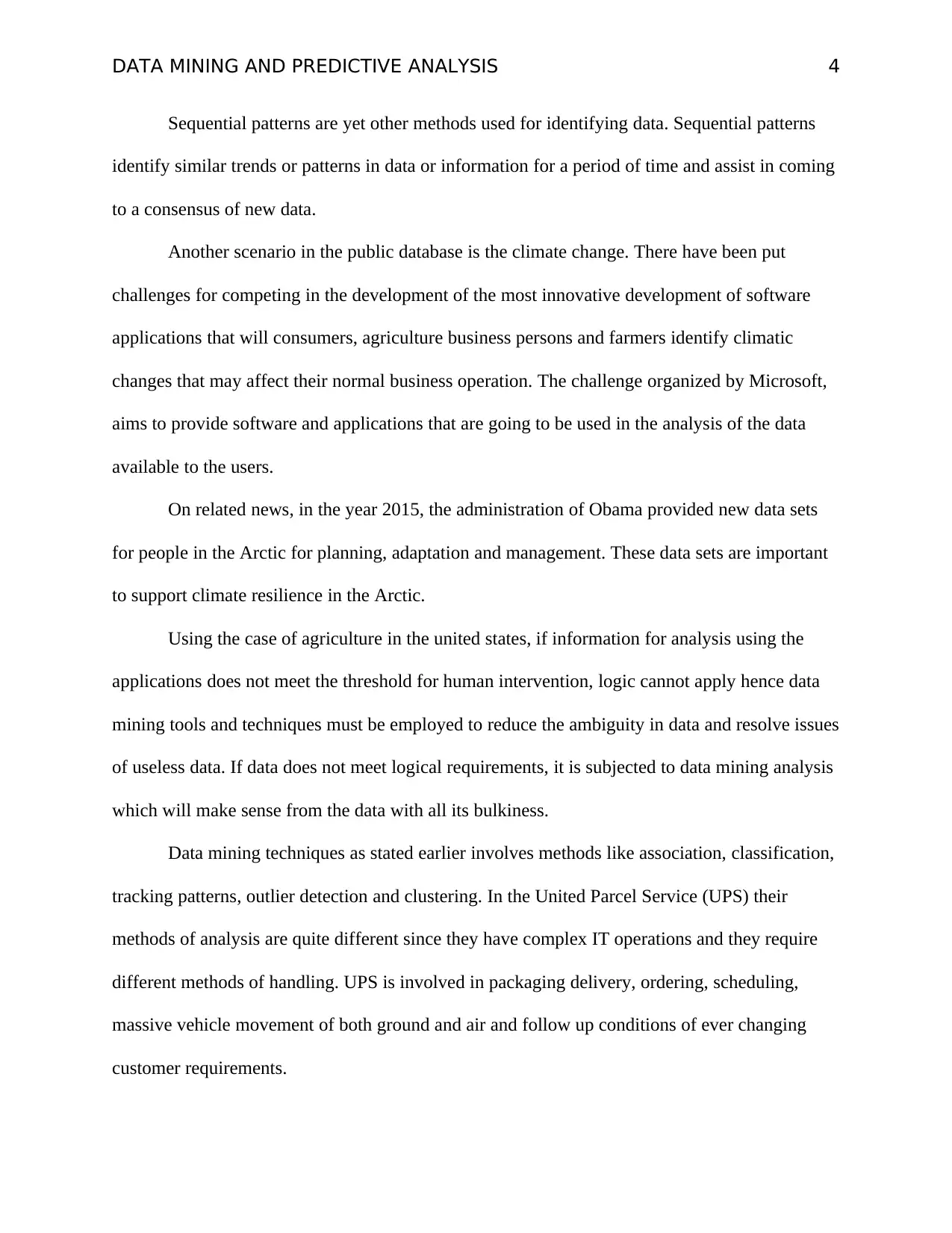
DATA MINING AND PREDICTIVE ANALYSIS 4
Sequential patterns are yet other methods used for identifying data. Sequential patterns
identify similar trends or patterns in data or information for a period of time and assist in coming
to a consensus of new data.
Another scenario in the public database is the climate change. There have been put
challenges for competing in the development of the most innovative development of software
applications that will consumers, agriculture business persons and farmers identify climatic
changes that may affect their normal business operation. The challenge organized by Microsoft,
aims to provide software and applications that are going to be used in the analysis of the data
available to the users.
On related news, in the year 2015, the administration of Obama provided new data sets
for people in the Arctic for planning, adaptation and management. These data sets are important
to support climate resilience in the Arctic.
Using the case of agriculture in the united states, if information for analysis using the
applications does not meet the threshold for human intervention, logic cannot apply hence data
mining tools and techniques must be employed to reduce the ambiguity in data and resolve issues
of useless data. If data does not meet logical requirements, it is subjected to data mining analysis
which will make sense from the data with all its bulkiness.
Data mining techniques as stated earlier involves methods like association, classification,
tracking patterns, outlier detection and clustering. In the United Parcel Service (UPS) their
methods of analysis are quite different since they have complex IT operations and they require
different methods of handling. UPS is involved in packaging delivery, ordering, scheduling,
massive vehicle movement of both ground and air and follow up conditions of ever changing
customer requirements.
Sequential patterns are yet other methods used for identifying data. Sequential patterns
identify similar trends or patterns in data or information for a period of time and assist in coming
to a consensus of new data.
Another scenario in the public database is the climate change. There have been put
challenges for competing in the development of the most innovative development of software
applications that will consumers, agriculture business persons and farmers identify climatic
changes that may affect their normal business operation. The challenge organized by Microsoft,
aims to provide software and applications that are going to be used in the analysis of the data
available to the users.
On related news, in the year 2015, the administration of Obama provided new data sets
for people in the Arctic for planning, adaptation and management. These data sets are important
to support climate resilience in the Arctic.
Using the case of agriculture in the united states, if information for analysis using the
applications does not meet the threshold for human intervention, logic cannot apply hence data
mining tools and techniques must be employed to reduce the ambiguity in data and resolve issues
of useless data. If data does not meet logical requirements, it is subjected to data mining analysis
which will make sense from the data with all its bulkiness.
Data mining techniques as stated earlier involves methods like association, classification,
tracking patterns, outlier detection and clustering. In the United Parcel Service (UPS) their
methods of analysis are quite different since they have complex IT operations and they require
different methods of handling. UPS is involved in packaging delivery, ordering, scheduling,
massive vehicle movement of both ground and air and follow up conditions of ever changing
customer requirements.
Secure Best Marks with AI Grader
Need help grading? Try our AI Grader for instant feedback on your assignments.
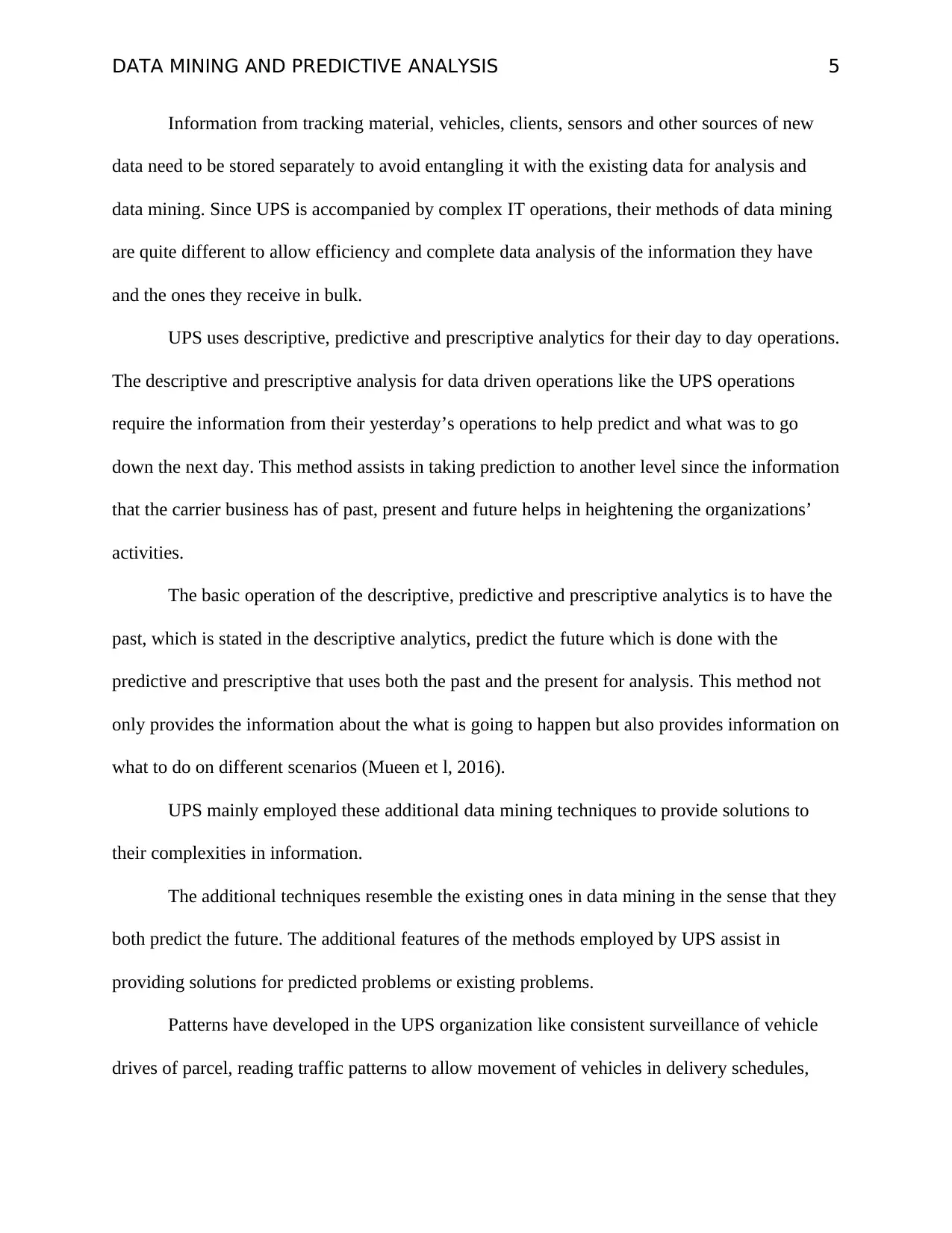
DATA MINING AND PREDICTIVE ANALYSIS 5
Information from tracking material, vehicles, clients, sensors and other sources of new
data need to be stored separately to avoid entangling it with the existing data for analysis and
data mining. Since UPS is accompanied by complex IT operations, their methods of data mining
are quite different to allow efficiency and complete data analysis of the information they have
and the ones they receive in bulk.
UPS uses descriptive, predictive and prescriptive analytics for their day to day operations.
The descriptive and prescriptive analysis for data driven operations like the UPS operations
require the information from their yesterday’s operations to help predict and what was to go
down the next day. This method assists in taking prediction to another level since the information
that the carrier business has of past, present and future helps in heightening the organizations’
activities.
The basic operation of the descriptive, predictive and prescriptive analytics is to have the
past, which is stated in the descriptive analytics, predict the future which is done with the
predictive and prescriptive that uses both the past and the present for analysis. This method not
only provides the information about the what is going to happen but also provides information on
what to do on different scenarios (Mueen et l, 2016).
UPS mainly employed these additional data mining techniques to provide solutions to
their complexities in information.
The additional techniques resemble the existing ones in data mining in the sense that they
both predict the future. The additional features of the methods employed by UPS assist in
providing solutions for predicted problems or existing problems.
Patterns have developed in the UPS organization like consistent surveillance of vehicle
drives of parcel, reading traffic patterns to allow movement of vehicles in delivery schedules,
Information from tracking material, vehicles, clients, sensors and other sources of new
data need to be stored separately to avoid entangling it with the existing data for analysis and
data mining. Since UPS is accompanied by complex IT operations, their methods of data mining
are quite different to allow efficiency and complete data analysis of the information they have
and the ones they receive in bulk.
UPS uses descriptive, predictive and prescriptive analytics for their day to day operations.
The descriptive and prescriptive analysis for data driven operations like the UPS operations
require the information from their yesterday’s operations to help predict and what was to go
down the next day. This method assists in taking prediction to another level since the information
that the carrier business has of past, present and future helps in heightening the organizations’
activities.
The basic operation of the descriptive, predictive and prescriptive analytics is to have the
past, which is stated in the descriptive analytics, predict the future which is done with the
predictive and prescriptive that uses both the past and the present for analysis. This method not
only provides the information about the what is going to happen but also provides information on
what to do on different scenarios (Mueen et l, 2016).
UPS mainly employed these additional data mining techniques to provide solutions to
their complexities in information.
The additional techniques resemble the existing ones in data mining in the sense that they
both predict the future. The additional features of the methods employed by UPS assist in
providing solutions for predicted problems or existing problems.
Patterns have developed in the UPS organization like consistent surveillance of vehicle
drives of parcel, reading traffic patterns to allow movement of vehicles in delivery schedules,
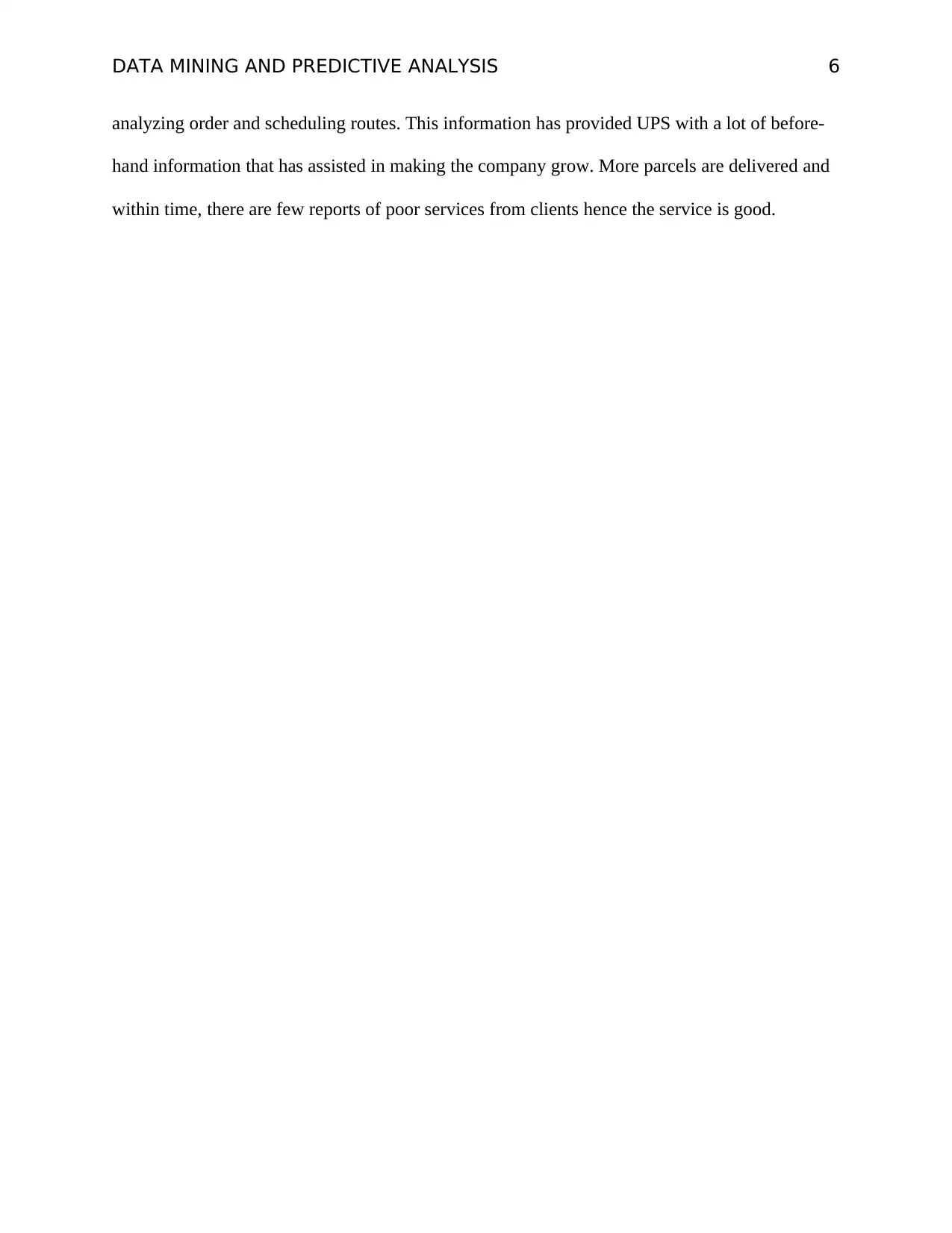
DATA MINING AND PREDICTIVE ANALYSIS 6
analyzing order and scheduling routes. This information has provided UPS with a lot of before-
hand information that has assisted in making the company grow. More parcels are delivered and
within time, there are few reports of poor services from clients hence the service is good.
analyzing order and scheduling routes. This information has provided UPS with a lot of before-
hand information that has assisted in making the company grow. More parcels are delivered and
within time, there are few reports of poor services from clients hence the service is good.
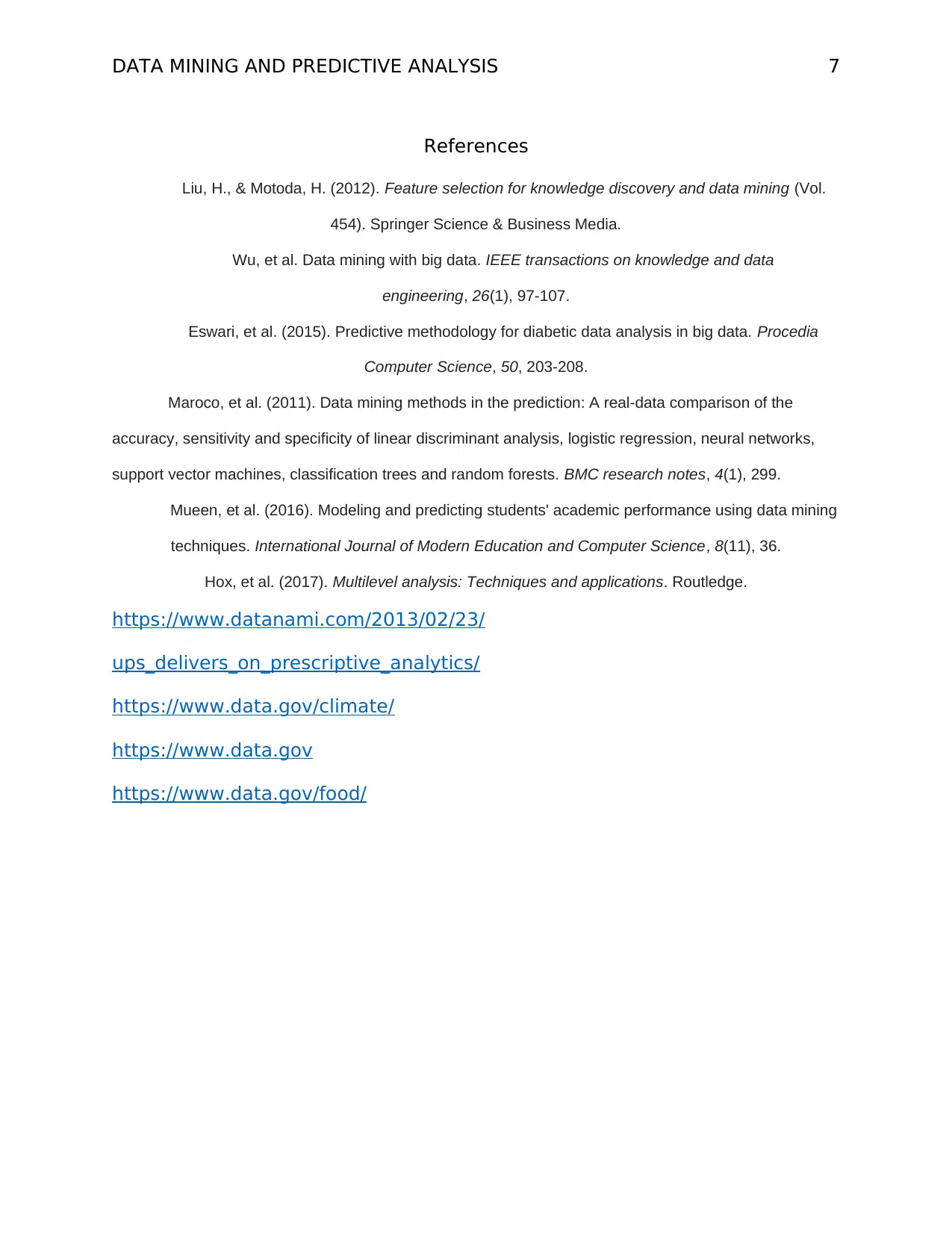
DATA MINING AND PREDICTIVE ANALYSIS 7
References
Liu, H., & Motoda, H. (2012). Feature selection for knowledge discovery and data mining (Vol.
454). Springer Science & Business Media.
Wu, et al. Data mining with big data. IEEE transactions on knowledge and data
engineering, 26(1), 97-107.
Eswari, et al. (2015). Predictive methodology for diabetic data analysis in big data. Procedia
Computer Science, 50, 203-208.
Maroco, et al. (2011). Data mining methods in the prediction: A real-data comparison of the
accuracy, sensitivity and specificity of linear discriminant analysis, logistic regression, neural networks,
support vector machines, classification trees and random forests. BMC research notes, 4(1), 299.
Mueen, et al. (2016). Modeling and predicting students' academic performance using data mining
techniques. International Journal of Modern Education and Computer Science, 8(11), 36.
Hox, et al. (2017). Multilevel analysis: Techniques and applications. Routledge.
https://www.datanami.com/2013/02/23/
ups_delivers_on_prescriptive_analytics/
https://www.data.gov/climate/
https://www.data.gov
https://www.data.gov/food/
References
Liu, H., & Motoda, H. (2012). Feature selection for knowledge discovery and data mining (Vol.
454). Springer Science & Business Media.
Wu, et al. Data mining with big data. IEEE transactions on knowledge and data
engineering, 26(1), 97-107.
Eswari, et al. (2015). Predictive methodology for diabetic data analysis in big data. Procedia
Computer Science, 50, 203-208.
Maroco, et al. (2011). Data mining methods in the prediction: A real-data comparison of the
accuracy, sensitivity and specificity of linear discriminant analysis, logistic regression, neural networks,
support vector machines, classification trees and random forests. BMC research notes, 4(1), 299.
Mueen, et al. (2016). Modeling and predicting students' academic performance using data mining
techniques. International Journal of Modern Education and Computer Science, 8(11), 36.
Hox, et al. (2017). Multilevel analysis: Techniques and applications. Routledge.
https://www.datanami.com/2013/02/23/
ups_delivers_on_prescriptive_analytics/
https://www.data.gov/climate/
https://www.data.gov
https://www.data.gov/food/
Paraphrase This Document
Need a fresh take? Get an instant paraphrase of this document with our AI Paraphraser
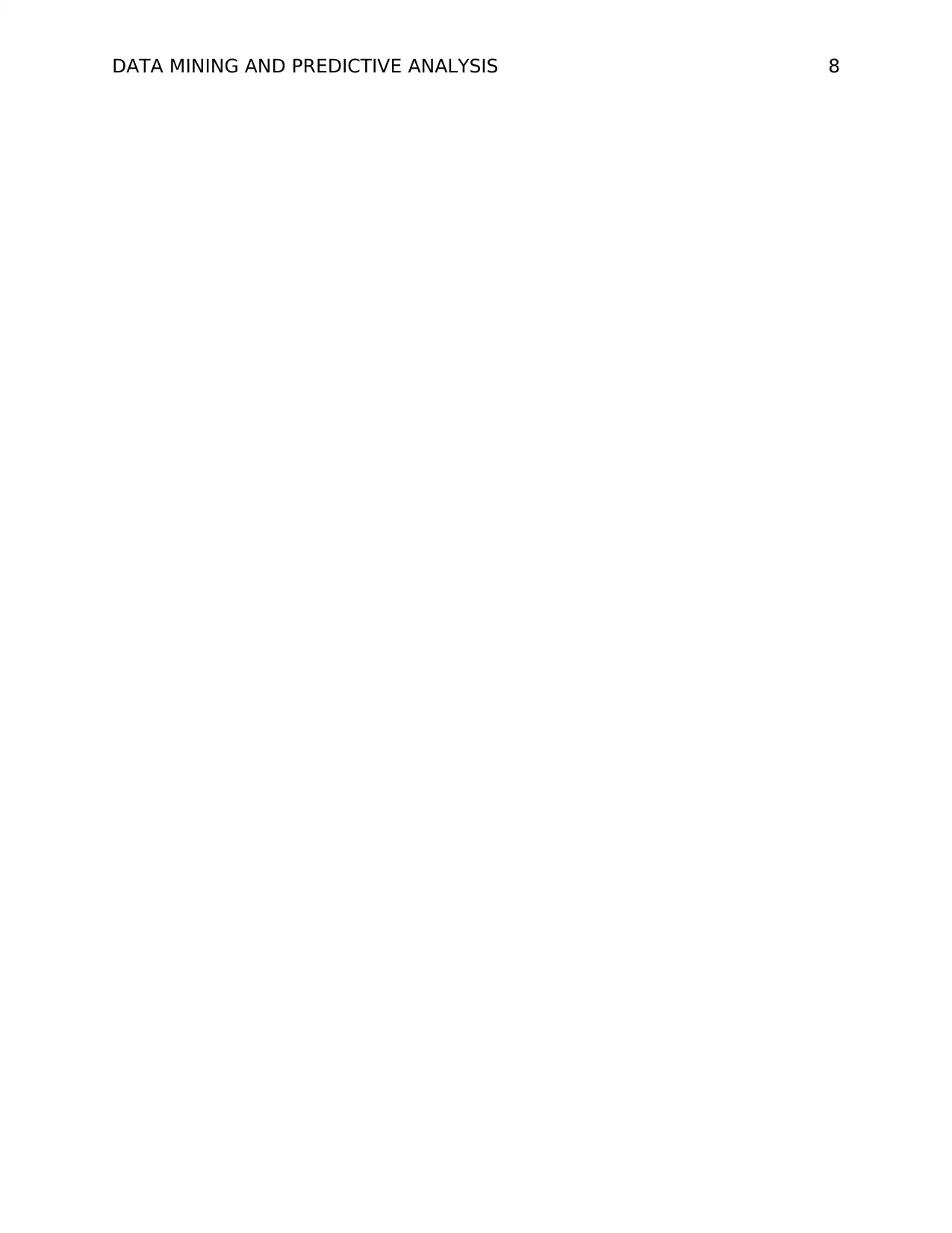
DATA MINING AND PREDICTIVE ANALYSIS 8
1 out of 8
Related Documents
![[object Object]](/_next/image/?url=%2F_next%2Fstatic%2Fmedia%2Flogo.6d15ce61.png&w=640&q=75)
Your All-in-One AI-Powered Toolkit for Academic Success.
+13062052269
info@desklib.com
Available 24*7 on WhatsApp / Email
Unlock your academic potential
© 2024 | Zucol Services PVT LTD | All rights reserved.