Descriptive Statistics and Inferential Statistics: Measures of Central Tendency and Dispersion
VerifiedAdded on 2023/06/13
|19
|4575
|323
AI Summary
This study worksheet and quiz covers topics such as descriptive and inferential statistics, measures of central tendency and dispersion, nominal, ordinal, interval and ratio scales, probability, sensitivity, specificity, predictive value, efficiency, central limit theorem, type I and type II errors, paired t-test, Wilcoxon matched-pairs signed-rank test, parametric and nonparametric tests, and ANOVA. It includes a hypothetical research question and hypothesis for each type of test, as well as a critical thinking assessment of an article on ANOVA.
Contribute Materials
Your contribution can guide someone’s learning journey. Share your
documents today.
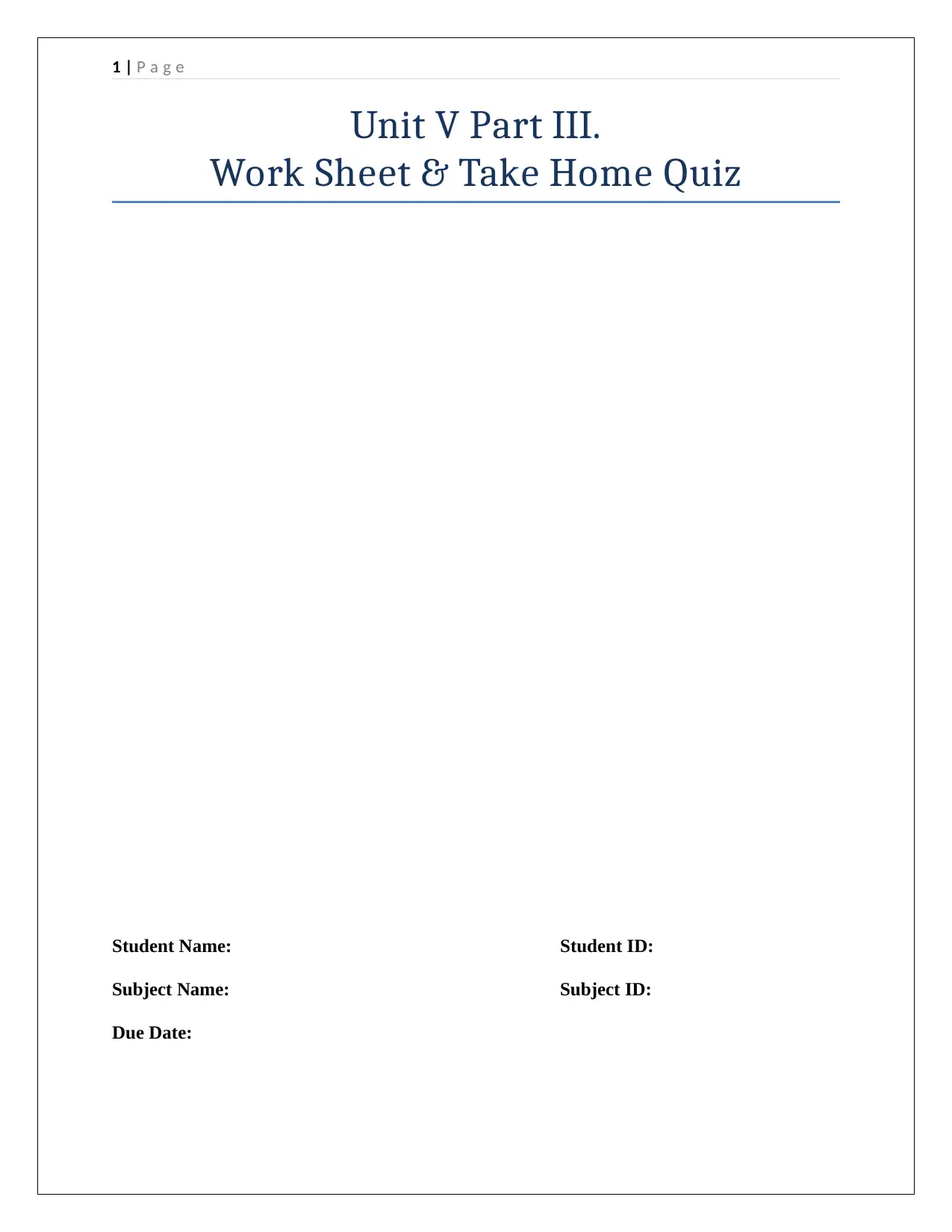
1 | P a g e
Unit V Part III.
Work Sheet & Take Home Quiz
Student Name: Student ID:
Subject Name: Subject ID:
Due Date:
Unit V Part III.
Work Sheet & Take Home Quiz
Student Name: Student ID:
Subject Name: Subject ID:
Due Date:
Secure Best Marks with AI Grader
Need help grading? Try our AI Grader for instant feedback on your assignments.
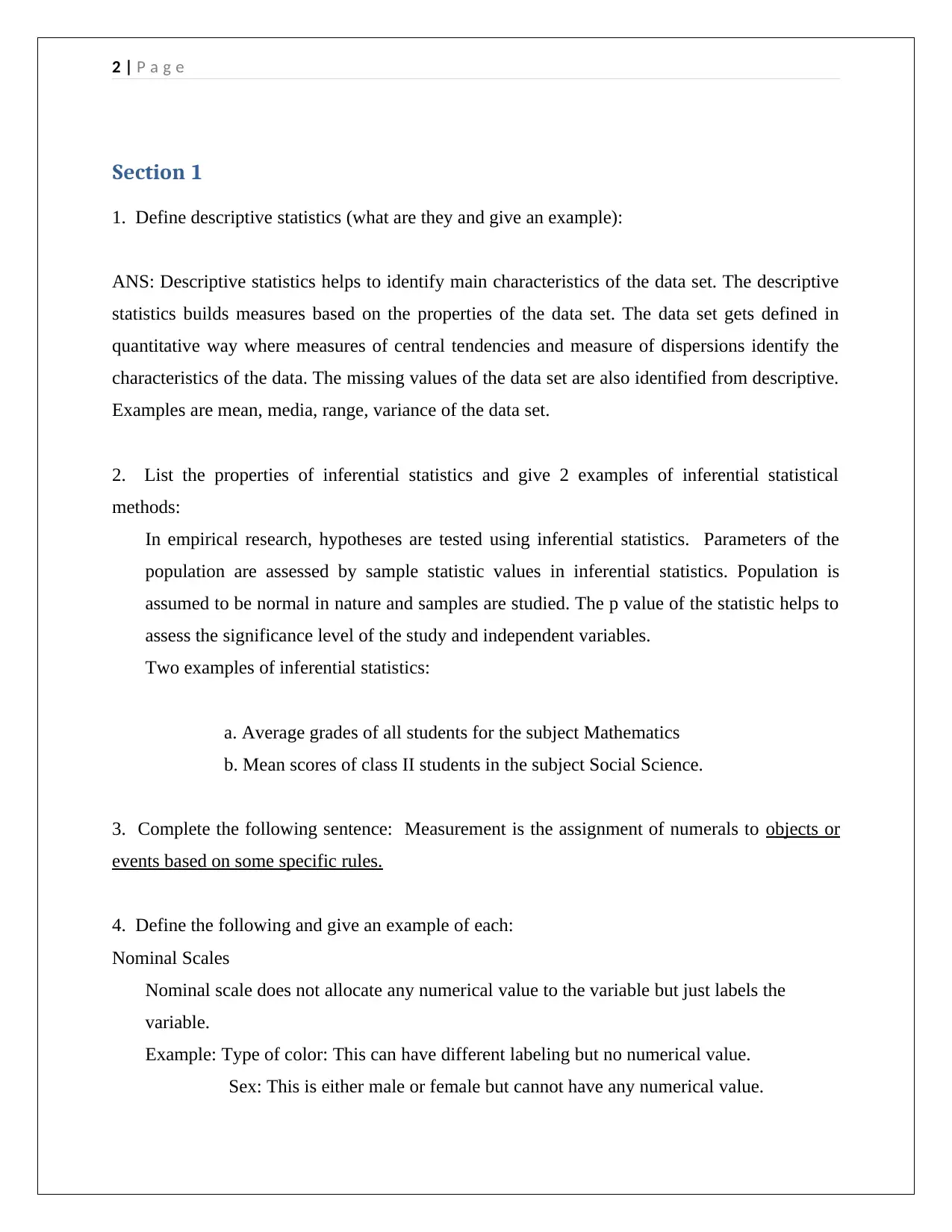
2 | P a g e
Section 1
1. Define descriptive statistics (what are they and give an example):
ANS: Descriptive statistics helps to identify main characteristics of the data set. The descriptive
statistics builds measures based on the properties of the data set. The data set gets defined in
quantitative way where measures of central tendencies and measure of dispersions identify the
characteristics of the data. The missing values of the data set are also identified from descriptive.
Examples are mean, media, range, variance of the data set.
2. List the properties of inferential statistics and give 2 examples of inferential statistical
methods:
In empirical research, hypotheses are tested using inferential statistics. Parameters of the
population are assessed by sample statistic values in inferential statistics. Population is
assumed to be normal in nature and samples are studied. The p value of the statistic helps to
assess the significance level of the study and independent variables.
Two examples of inferential statistics:
a. Average grades of all students for the subject Mathematics
b. Mean scores of class II students in the subject Social Science.
3. Complete the following sentence: Measurement is the assignment of numerals to objects or
events based on some specific rules.
4. Define the following and give an example of each:
Nominal Scales
Nominal scale does not allocate any numerical value to the variable but just labels the
variable.
Example: Type of color: This can have different labeling but no numerical value.
Sex: This is either male or female but cannot have any numerical value.
Section 1
1. Define descriptive statistics (what are they and give an example):
ANS: Descriptive statistics helps to identify main characteristics of the data set. The descriptive
statistics builds measures based on the properties of the data set. The data set gets defined in
quantitative way where measures of central tendencies and measure of dispersions identify the
characteristics of the data. The missing values of the data set are also identified from descriptive.
Examples are mean, media, range, variance of the data set.
2. List the properties of inferential statistics and give 2 examples of inferential statistical
methods:
In empirical research, hypotheses are tested using inferential statistics. Parameters of the
population are assessed by sample statistic values in inferential statistics. Population is
assumed to be normal in nature and samples are studied. The p value of the statistic helps to
assess the significance level of the study and independent variables.
Two examples of inferential statistics:
a. Average grades of all students for the subject Mathematics
b. Mean scores of class II students in the subject Social Science.
3. Complete the following sentence: Measurement is the assignment of numerals to objects or
events based on some specific rules.
4. Define the following and give an example of each:
Nominal Scales
Nominal scale does not allocate any numerical value to the variable but just labels the
variable.
Example: Type of color: This can have different labeling but no numerical value.
Sex: This is either male or female but cannot have any numerical value.
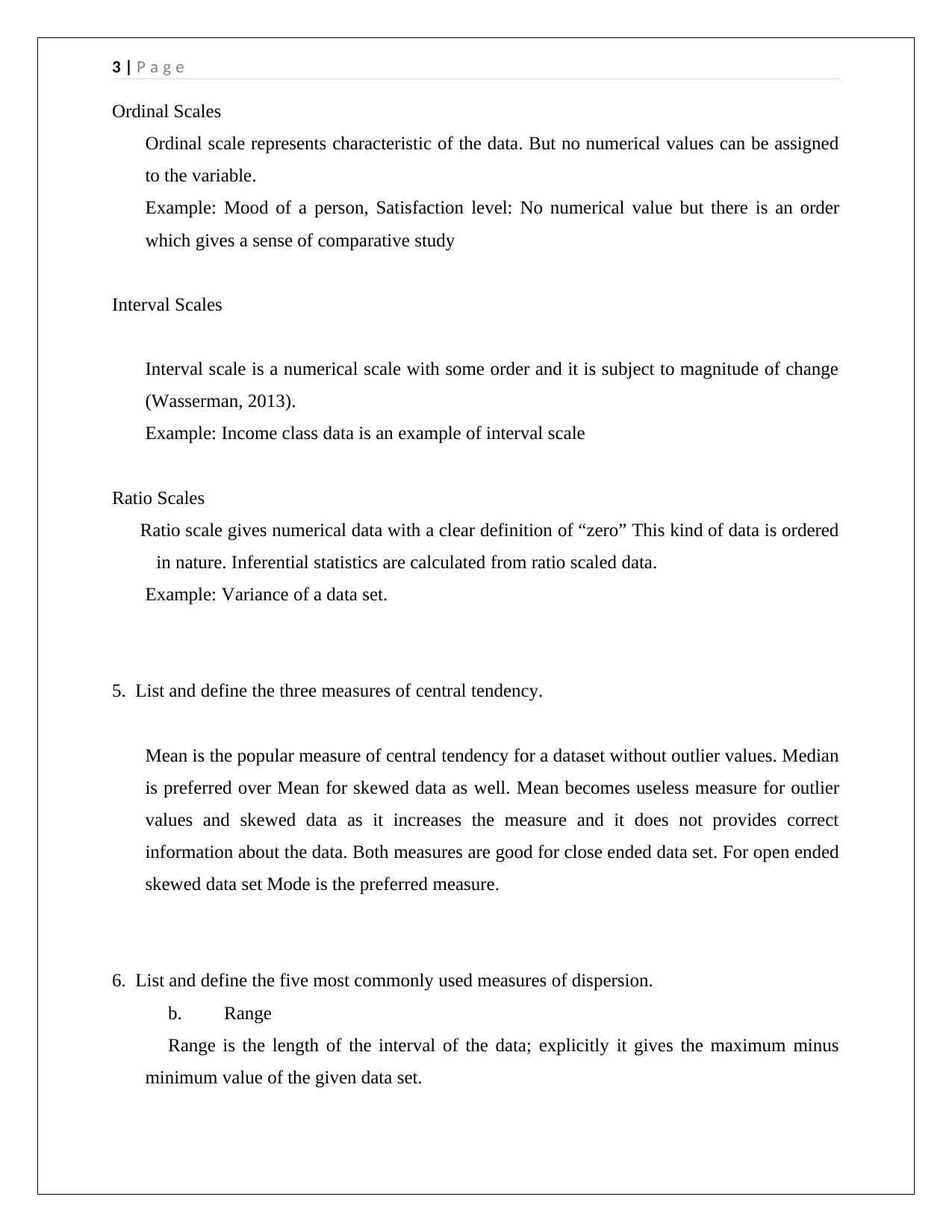
3 | P a g e
Ordinal Scales
Ordinal scale represents characteristic of the data. But no numerical values can be assigned
to the variable.
Example: Mood of a person, Satisfaction level: No numerical value but there is an order
which gives a sense of comparative study
Interval Scales
Interval scale is a numerical scale with some order and it is subject to magnitude of change
(Wasserman, 2013).
Example: Income class data is an example of interval scale
Ratio Scales
Ratio scale gives numerical data with a clear definition of “zero” This kind of data is ordered
in nature. Inferential statistics are calculated from ratio scaled data.
Example: Variance of a data set.
5. List and define the three measures of central tendency.
Mean is the popular measure of central tendency for a dataset without outlier values. Median
is preferred over Mean for skewed data as well. Mean becomes useless measure for outlier
values and skewed data as it increases the measure and it does not provides correct
information about the data. Both measures are good for close ended data set. For open ended
skewed data set Mode is the preferred measure.
6. List and define the five most commonly used measures of dispersion.
b. Range
Range is the length of the interval of the data; explicitly it gives the maximum minus
minimum value of the given data set.
Ordinal Scales
Ordinal scale represents characteristic of the data. But no numerical values can be assigned
to the variable.
Example: Mood of a person, Satisfaction level: No numerical value but there is an order
which gives a sense of comparative study
Interval Scales
Interval scale is a numerical scale with some order and it is subject to magnitude of change
(Wasserman, 2013).
Example: Income class data is an example of interval scale
Ratio Scales
Ratio scale gives numerical data with a clear definition of “zero” This kind of data is ordered
in nature. Inferential statistics are calculated from ratio scaled data.
Example: Variance of a data set.
5. List and define the three measures of central tendency.
Mean is the popular measure of central tendency for a dataset without outlier values. Median
is preferred over Mean for skewed data as well. Mean becomes useless measure for outlier
values and skewed data as it increases the measure and it does not provides correct
information about the data. Both measures are good for close ended data set. For open ended
skewed data set Mode is the preferred measure.
6. List and define the five most commonly used measures of dispersion.
b. Range
Range is the length of the interval of the data; explicitly it gives the maximum minus
minimum value of the given data set.
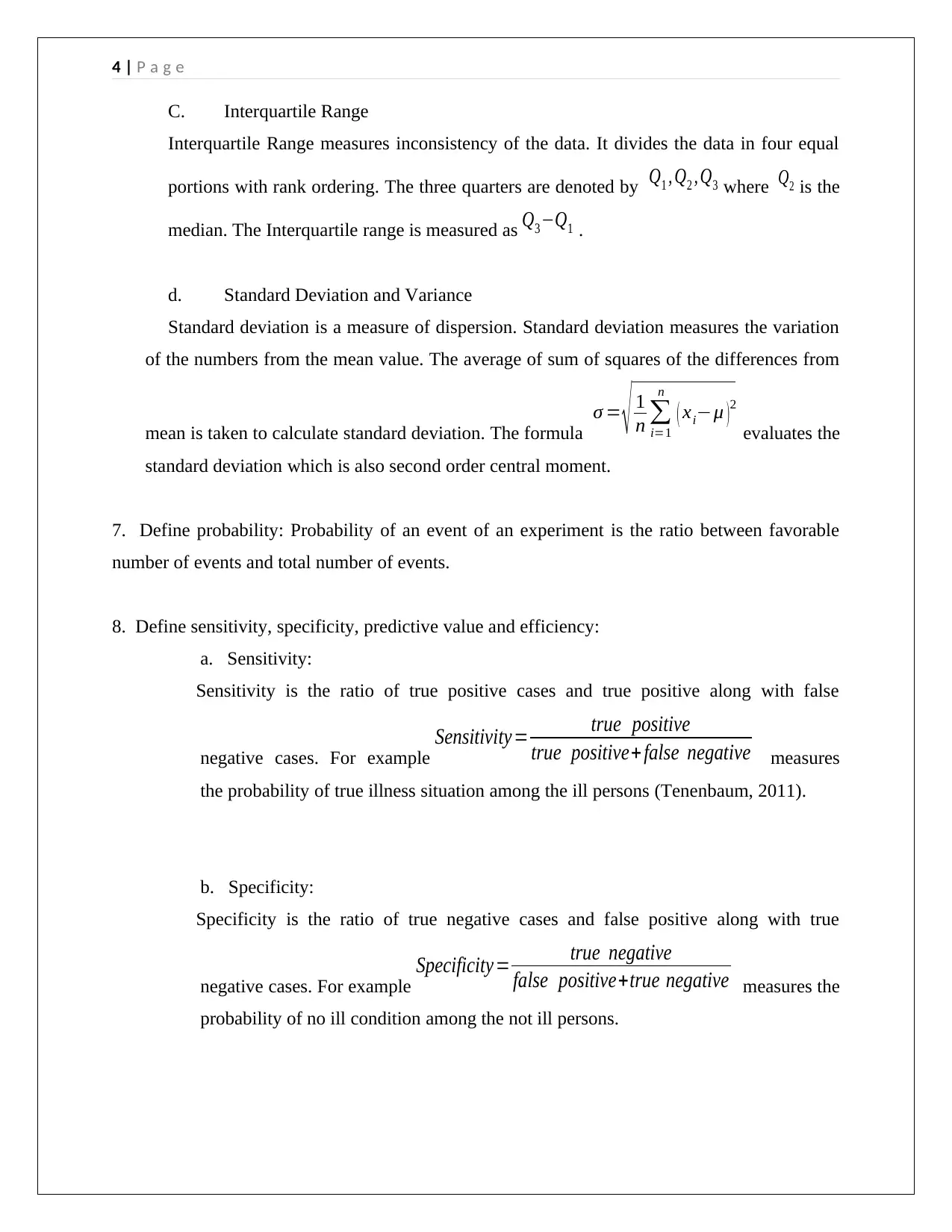
4 | P a g e
C. Interquartile Range
Interquartile Range measures inconsistency of the data. It divides the data in four equal
portions with rank ordering. The three quarters are denoted by Q1 , Q2 , Q3 where Q2 is the
median. The Interquartile range is measured as Q3−Q1 .
d. Standard Deviation and Variance
Standard deviation is a measure of dispersion. Standard deviation measures the variation
of the numbers from the mean value. The average of sum of squares of the differences from
mean is taken to calculate standard deviation. The formula
σ = √ 1
n ∑
i=1
n
( xi−μ )
2
evaluates the
standard deviation which is also second order central moment.
7. Define probability: Probability of an event of an experiment is the ratio between favorable
number of events and total number of events.
8. Define sensitivity, specificity, predictive value and efficiency:
a. Sensitivity:
Sensitivity is the ratio of true positive cases and true positive along with false
negative cases. For example
Sensitivity= true positive
true positive+ false negative measures
the probability of true illness situation among the ill persons (Tenenbaum, 2011).
b. Specificity:
Specificity is the ratio of true negative cases and false positive along with true
negative cases. For example
Specificity= true negative
false positive+true negative measures the
probability of no ill condition among the not ill persons.
C. Interquartile Range
Interquartile Range measures inconsistency of the data. It divides the data in four equal
portions with rank ordering. The three quarters are denoted by Q1 , Q2 , Q3 where Q2 is the
median. The Interquartile range is measured as Q3−Q1 .
d. Standard Deviation and Variance
Standard deviation is a measure of dispersion. Standard deviation measures the variation
of the numbers from the mean value. The average of sum of squares of the differences from
mean is taken to calculate standard deviation. The formula
σ = √ 1
n ∑
i=1
n
( xi−μ )
2
evaluates the
standard deviation which is also second order central moment.
7. Define probability: Probability of an event of an experiment is the ratio between favorable
number of events and total number of events.
8. Define sensitivity, specificity, predictive value and efficiency:
a. Sensitivity:
Sensitivity is the ratio of true positive cases and true positive along with false
negative cases. For example
Sensitivity= true positive
true positive+ false negative measures
the probability of true illness situation among the ill persons (Tenenbaum, 2011).
b. Specificity:
Specificity is the ratio of true negative cases and false positive along with true
negative cases. For example
Specificity= true negative
false positive+true negative measures the
probability of no ill condition among the not ill persons.
Secure Best Marks with AI Grader
Need help grading? Try our AI Grader for instant feedback on your assignments.
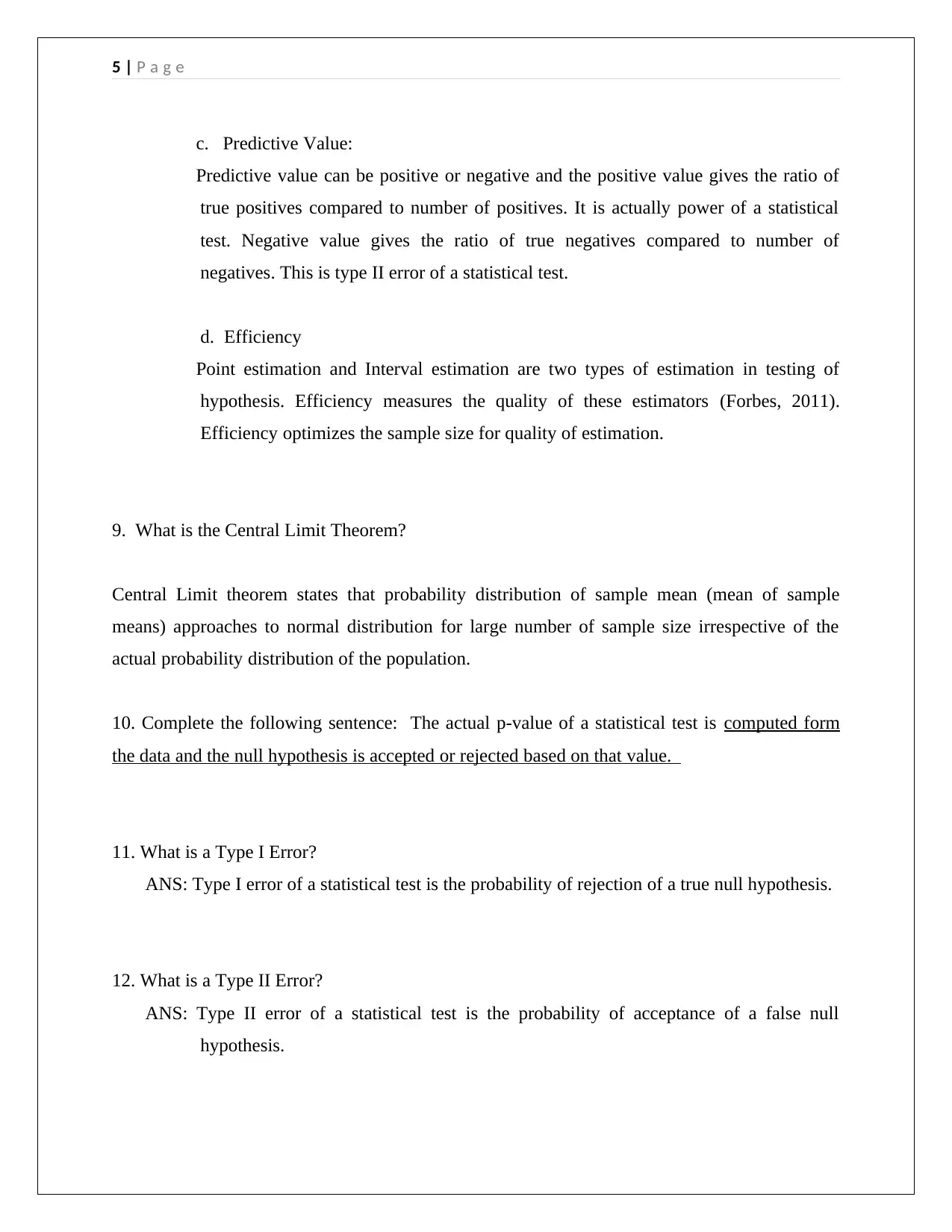
5 | P a g e
c. Predictive Value:
Predictive value can be positive or negative and the positive value gives the ratio of
true positives compared to number of positives. It is actually power of a statistical
test. Negative value gives the ratio of true negatives compared to number of
negatives. This is type II error of a statistical test.
d. Efficiency
Point estimation and Interval estimation are two types of estimation in testing of
hypothesis. Efficiency measures the quality of these estimators (Forbes, 2011).
Efficiency optimizes the sample size for quality of estimation.
9. What is the Central Limit Theorem?
Central Limit theorem states that probability distribution of sample mean (mean of sample
means) approaches to normal distribution for large number of sample size irrespective of the
actual probability distribution of the population.
10. Complete the following sentence: The actual p-value of a statistical test is computed form
the data and the null hypothesis is accepted or rejected based on that value.
11. What is a Type I Error?
ANS: Type I error of a statistical test is the probability of rejection of a true null hypothesis.
12. What is a Type II Error?
ANS: Type II error of a statistical test is the probability of acceptance of a false null
hypothesis.
c. Predictive Value:
Predictive value can be positive or negative and the positive value gives the ratio of
true positives compared to number of positives. It is actually power of a statistical
test. Negative value gives the ratio of true negatives compared to number of
negatives. This is type II error of a statistical test.
d. Efficiency
Point estimation and Interval estimation are two types of estimation in testing of
hypothesis. Efficiency measures the quality of these estimators (Forbes, 2011).
Efficiency optimizes the sample size for quality of estimation.
9. What is the Central Limit Theorem?
Central Limit theorem states that probability distribution of sample mean (mean of sample
means) approaches to normal distribution for large number of sample size irrespective of the
actual probability distribution of the population.
10. Complete the following sentence: The actual p-value of a statistical test is computed form
the data and the null hypothesis is accepted or rejected based on that value.
11. What is a Type I Error?
ANS: Type I error of a statistical test is the probability of rejection of a true null hypothesis.
12. What is a Type II Error?
ANS: Type II error of a statistical test is the probability of acceptance of a false null
hypothesis.
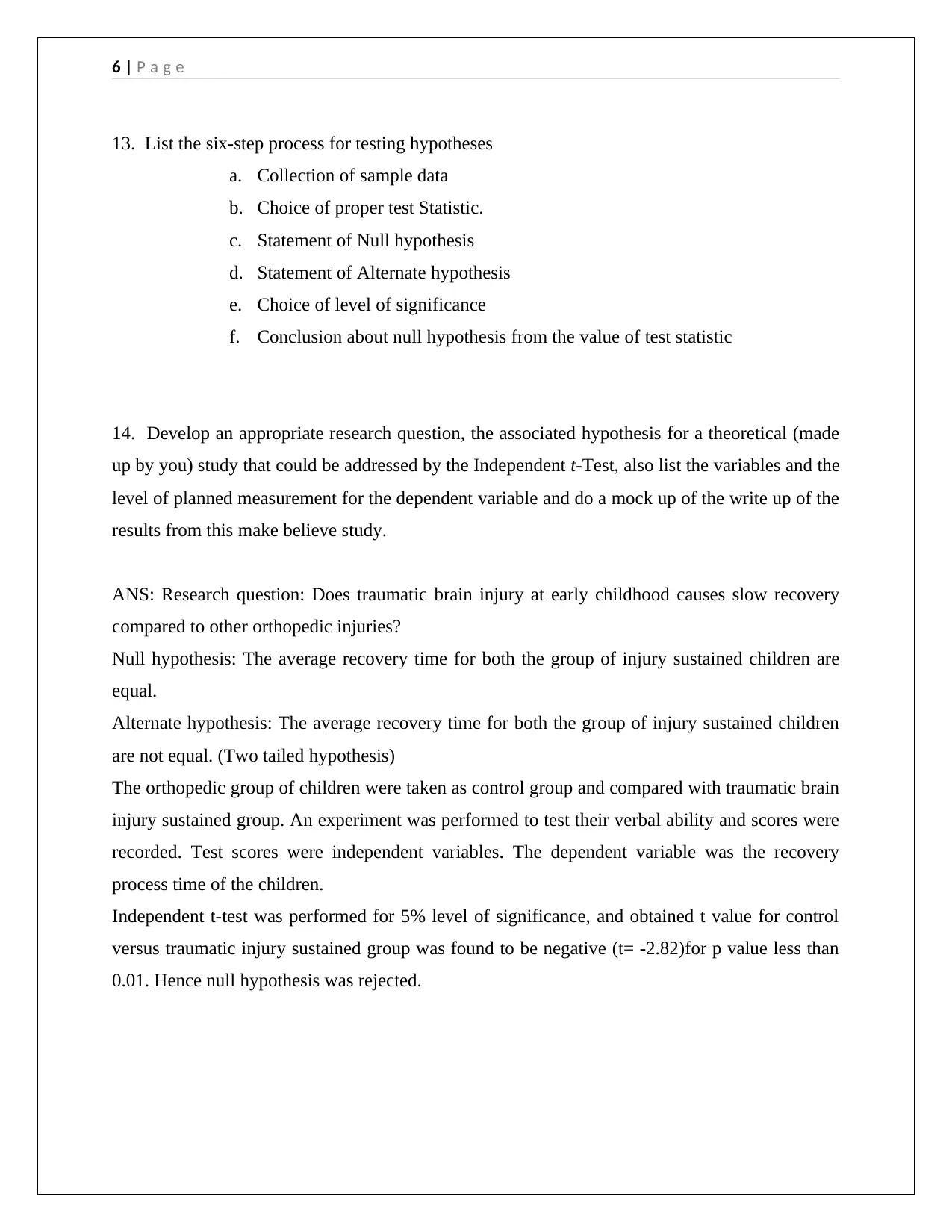
6 | P a g e
13. List the six-step process for testing hypotheses
a. Collection of sample data
b. Choice of proper test Statistic.
c. Statement of Null hypothesis
d. Statement of Alternate hypothesis
e. Choice of level of significance
f. Conclusion about null hypothesis from the value of test statistic
14. Develop an appropriate research question, the associated hypothesis for a theoretical (made
up by you) study that could be addressed by the Independent t-Test, also list the variables and the
level of planned measurement for the dependent variable and do a mock up of the write up of the
results from this make believe study.
ANS: Research question: Does traumatic brain injury at early childhood causes slow recovery
compared to other orthopedic injuries?
Null hypothesis: The average recovery time for both the group of injury sustained children are
equal.
Alternate hypothesis: The average recovery time for both the group of injury sustained children
are not equal. (Two tailed hypothesis)
The orthopedic group of children were taken as control group and compared with traumatic brain
injury sustained group. An experiment was performed to test their verbal ability and scores were
recorded. Test scores were independent variables. The dependent variable was the recovery
process time of the children.
Independent t-test was performed for 5% level of significance, and obtained t value for control
versus traumatic injury sustained group was found to be negative (t= -2.82)for p value less than
0.01. Hence null hypothesis was rejected.
13. List the six-step process for testing hypotheses
a. Collection of sample data
b. Choice of proper test Statistic.
c. Statement of Null hypothesis
d. Statement of Alternate hypothesis
e. Choice of level of significance
f. Conclusion about null hypothesis from the value of test statistic
14. Develop an appropriate research question, the associated hypothesis for a theoretical (made
up by you) study that could be addressed by the Independent t-Test, also list the variables and the
level of planned measurement for the dependent variable and do a mock up of the write up of the
results from this make believe study.
ANS: Research question: Does traumatic brain injury at early childhood causes slow recovery
compared to other orthopedic injuries?
Null hypothesis: The average recovery time for both the group of injury sustained children are
equal.
Alternate hypothesis: The average recovery time for both the group of injury sustained children
are not equal. (Two tailed hypothesis)
The orthopedic group of children were taken as control group and compared with traumatic brain
injury sustained group. An experiment was performed to test their verbal ability and scores were
recorded. Test scores were independent variables. The dependent variable was the recovery
process time of the children.
Independent t-test was performed for 5% level of significance, and obtained t value for control
versus traumatic injury sustained group was found to be negative (t= -2.82)for p value less than
0.01. Hence null hypothesis was rejected.
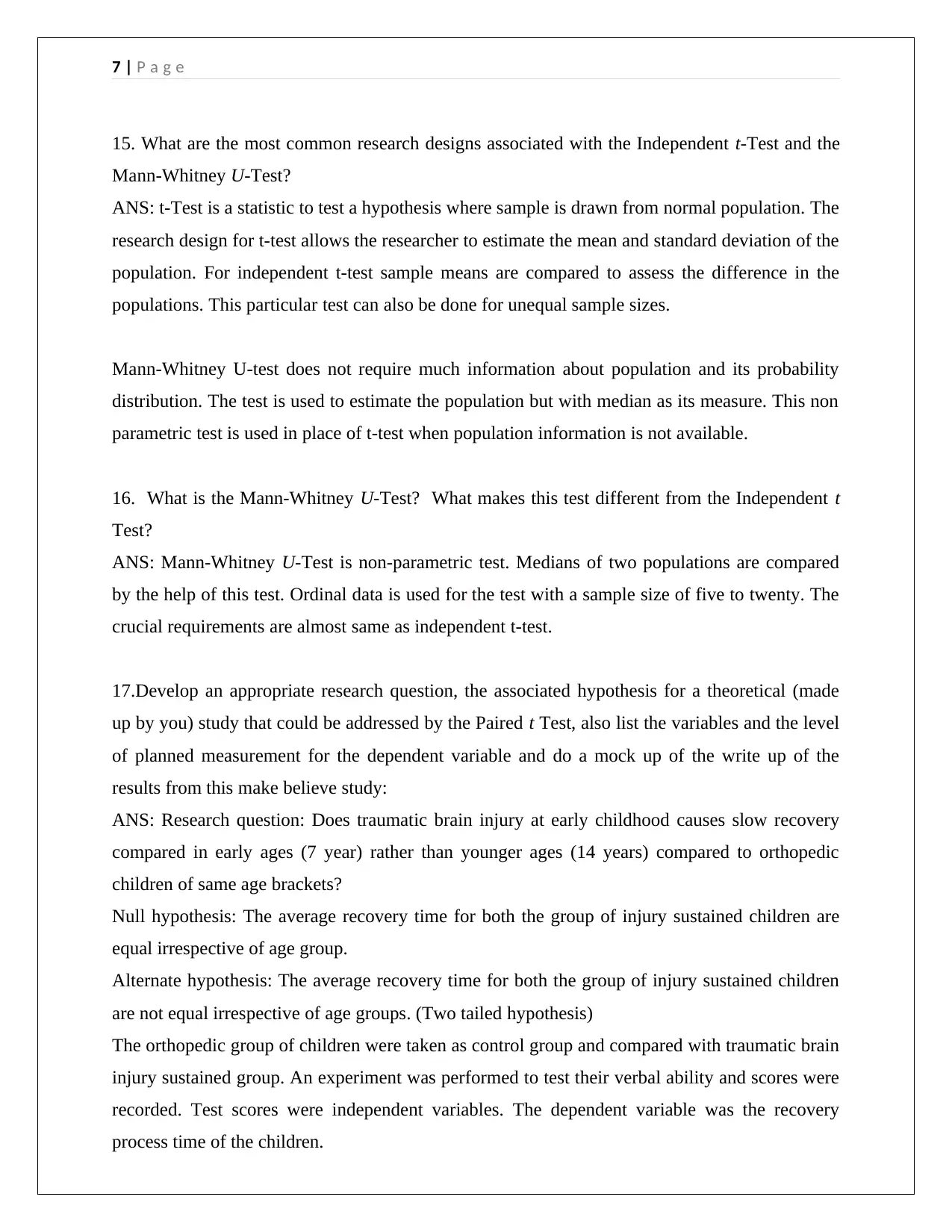
7 | P a g e
15. What are the most common research designs associated with the Independent t-Test and the
Mann-Whitney U-Test?
ANS: t-Test is a statistic to test a hypothesis where sample is drawn from normal population. The
research design for t-test allows the researcher to estimate the mean and standard deviation of the
population. For independent t-test sample means are compared to assess the difference in the
populations. This particular test can also be done for unequal sample sizes.
Mann-Whitney U-test does not require much information about population and its probability
distribution. The test is used to estimate the population but with median as its measure. This non
parametric test is used in place of t-test when population information is not available.
16. What is the Mann-Whitney U-Test? What makes this test different from the Independent t
Test?
ANS: Mann-Whitney U-Test is non-parametric test. Medians of two populations are compared
by the help of this test. Ordinal data is used for the test with a sample size of five to twenty. The
crucial requirements are almost same as independent t-test.
17.Develop an appropriate research question, the associated hypothesis for a theoretical (made
up by you) study that could be addressed by the Paired t Test, also list the variables and the level
of planned measurement for the dependent variable and do a mock up of the write up of the
results from this make believe study:
ANS: Research question: Does traumatic brain injury at early childhood causes slow recovery
compared in early ages (7 year) rather than younger ages (14 years) compared to orthopedic
children of same age brackets?
Null hypothesis: The average recovery time for both the group of injury sustained children are
equal irrespective of age group.
Alternate hypothesis: The average recovery time for both the group of injury sustained children
are not equal irrespective of age groups. (Two tailed hypothesis)
The orthopedic group of children were taken as control group and compared with traumatic brain
injury sustained group. An experiment was performed to test their verbal ability and scores were
recorded. Test scores were independent variables. The dependent variable was the recovery
process time of the children.
15. What are the most common research designs associated with the Independent t-Test and the
Mann-Whitney U-Test?
ANS: t-Test is a statistic to test a hypothesis where sample is drawn from normal population. The
research design for t-test allows the researcher to estimate the mean and standard deviation of the
population. For independent t-test sample means are compared to assess the difference in the
populations. This particular test can also be done for unequal sample sizes.
Mann-Whitney U-test does not require much information about population and its probability
distribution. The test is used to estimate the population but with median as its measure. This non
parametric test is used in place of t-test when population information is not available.
16. What is the Mann-Whitney U-Test? What makes this test different from the Independent t
Test?
ANS: Mann-Whitney U-Test is non-parametric test. Medians of two populations are compared
by the help of this test. Ordinal data is used for the test with a sample size of five to twenty. The
crucial requirements are almost same as independent t-test.
17.Develop an appropriate research question, the associated hypothesis for a theoretical (made
up by you) study that could be addressed by the Paired t Test, also list the variables and the level
of planned measurement for the dependent variable and do a mock up of the write up of the
results from this make believe study:
ANS: Research question: Does traumatic brain injury at early childhood causes slow recovery
compared in early ages (7 year) rather than younger ages (14 years) compared to orthopedic
children of same age brackets?
Null hypothesis: The average recovery time for both the group of injury sustained children are
equal irrespective of age group.
Alternate hypothesis: The average recovery time for both the group of injury sustained children
are not equal irrespective of age groups. (Two tailed hypothesis)
The orthopedic group of children were taken as control group and compared with traumatic brain
injury sustained group. An experiment was performed to test their verbal ability and scores were
recorded. Test scores were independent variables. The dependent variable was the recovery
process time of the children.
Paraphrase This Document
Need a fresh take? Get an instant paraphrase of this document with our AI Paraphraser
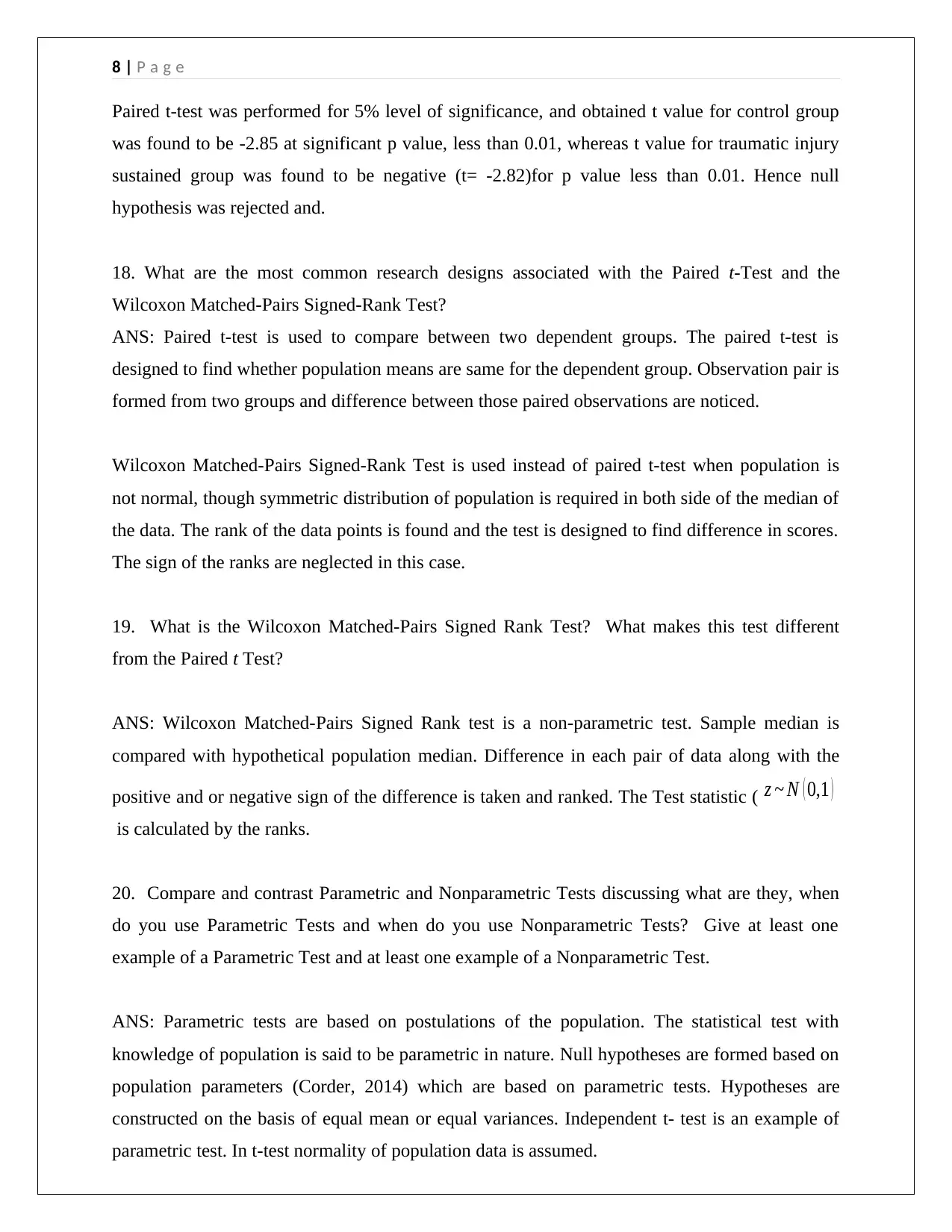
8 | P a g e
Paired t-test was performed for 5% level of significance, and obtained t value for control group
was found to be -2.85 at significant p value, less than 0.01, whereas t value for traumatic injury
sustained group was found to be negative (t= -2.82)for p value less than 0.01. Hence null
hypothesis was rejected and.
18. What are the most common research designs associated with the Paired t-Test and the
Wilcoxon Matched-Pairs Signed-Rank Test?
ANS: Paired t-test is used to compare between two dependent groups. The paired t-test is
designed to find whether population means are same for the dependent group. Observation pair is
formed from two groups and difference between those paired observations are noticed.
Wilcoxon Matched-Pairs Signed-Rank Test is used instead of paired t-test when population is
not normal, though symmetric distribution of population is required in both side of the median of
the data. The rank of the data points is found and the test is designed to find difference in scores.
The sign of the ranks are neglected in this case.
19. What is the Wilcoxon Matched-Pairs Signed Rank Test? What makes this test different
from the Paired t Test?
ANS: Wilcoxon Matched-Pairs Signed Rank test is a non-parametric test. Sample median is
compared with hypothetical population median. Difference in each pair of data along with the
positive and or negative sign of the difference is taken and ranked. The Test statistic ( z ~ N ( 0,1 )
is calculated by the ranks.
20. Compare and contrast Parametric and Nonparametric Tests discussing what are they, when
do you use Parametric Tests and when do you use Nonparametric Tests? Give at least one
example of a Parametric Test and at least one example of a Nonparametric Test.
ANS: Parametric tests are based on postulations of the population. The statistical test with
knowledge of population is said to be parametric in nature. Null hypotheses are formed based on
population parameters (Corder, 2014) which are based on parametric tests. Hypotheses are
constructed on the basis of equal mean or equal variances. Independent t- test is an example of
parametric test. In t-test normality of population data is assumed.
Paired t-test was performed for 5% level of significance, and obtained t value for control group
was found to be -2.85 at significant p value, less than 0.01, whereas t value for traumatic injury
sustained group was found to be negative (t= -2.82)for p value less than 0.01. Hence null
hypothesis was rejected and.
18. What are the most common research designs associated with the Paired t-Test and the
Wilcoxon Matched-Pairs Signed-Rank Test?
ANS: Paired t-test is used to compare between two dependent groups. The paired t-test is
designed to find whether population means are same for the dependent group. Observation pair is
formed from two groups and difference between those paired observations are noticed.
Wilcoxon Matched-Pairs Signed-Rank Test is used instead of paired t-test when population is
not normal, though symmetric distribution of population is required in both side of the median of
the data. The rank of the data points is found and the test is designed to find difference in scores.
The sign of the ranks are neglected in this case.
19. What is the Wilcoxon Matched-Pairs Signed Rank Test? What makes this test different
from the Paired t Test?
ANS: Wilcoxon Matched-Pairs Signed Rank test is a non-parametric test. Sample median is
compared with hypothetical population median. Difference in each pair of data along with the
positive and or negative sign of the difference is taken and ranked. The Test statistic ( z ~ N ( 0,1 )
is calculated by the ranks.
20. Compare and contrast Parametric and Nonparametric Tests discussing what are they, when
do you use Parametric Tests and when do you use Nonparametric Tests? Give at least one
example of a Parametric Test and at least one example of a Nonparametric Test.
ANS: Parametric tests are based on postulations of the population. The statistical test with
knowledge of population is said to be parametric in nature. Null hypotheses are formed based on
population parameters (Corder, 2014) which are based on parametric tests. Hypotheses are
constructed on the basis of equal mean or equal variances. Independent t- test is an example of
parametric test. In t-test normality of population data is assumed.
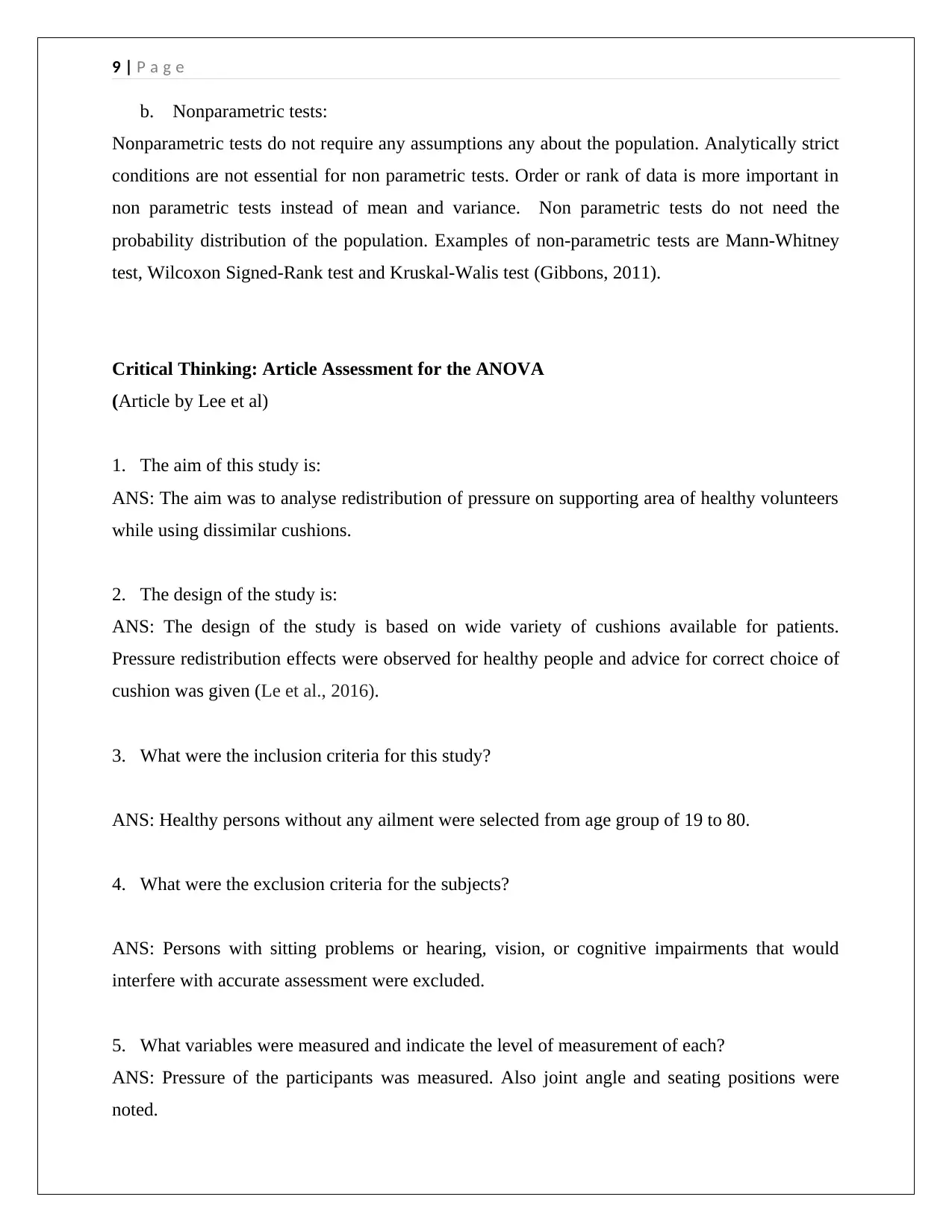
9 | P a g e
b. Nonparametric tests:
Nonparametric tests do not require any assumptions any about the population. Analytically strict
conditions are not essential for non parametric tests. Order or rank of data is more important in
non parametric tests instead of mean and variance. Non parametric tests do not need the
probability distribution of the population. Examples of non-parametric tests are Mann-Whitney
test, Wilcoxon Signed-Rank test and Kruskal-Walis test (Gibbons, 2011).
Critical Thinking: Article Assessment for the ANOVA
(Article by Lee et al)
1. The aim of this study is:
ANS: The aim was to analyse redistribution of pressure on supporting area of healthy volunteers
while using dissimilar cushions.
2. The design of the study is:
ANS: The design of the study is based on wide variety of cushions available for patients.
Pressure redistribution effects were observed for healthy people and advice for correct choice of
cushion was given (Le et al., 2016).
3. What were the inclusion criteria for this study?
ANS: Healthy persons without any ailment were selected from age group of 19 to 80.
4. What were the exclusion criteria for the subjects?
ANS: Persons with sitting problems or hearing, vision, or cognitive impairments that would
interfere with accurate assessment were excluded.
5. What variables were measured and indicate the level of measurement of each?
ANS: Pressure of the participants was measured. Also joint angle and seating positions were
noted.
b. Nonparametric tests:
Nonparametric tests do not require any assumptions any about the population. Analytically strict
conditions are not essential for non parametric tests. Order or rank of data is more important in
non parametric tests instead of mean and variance. Non parametric tests do not need the
probability distribution of the population. Examples of non-parametric tests are Mann-Whitney
test, Wilcoxon Signed-Rank test and Kruskal-Walis test (Gibbons, 2011).
Critical Thinking: Article Assessment for the ANOVA
(Article by Lee et al)
1. The aim of this study is:
ANS: The aim was to analyse redistribution of pressure on supporting area of healthy volunteers
while using dissimilar cushions.
2. The design of the study is:
ANS: The design of the study is based on wide variety of cushions available for patients.
Pressure redistribution effects were observed for healthy people and advice for correct choice of
cushion was given (Le et al., 2016).
3. What were the inclusion criteria for this study?
ANS: Healthy persons without any ailment were selected from age group of 19 to 80.
4. What were the exclusion criteria for the subjects?
ANS: Persons with sitting problems or hearing, vision, or cognitive impairments that would
interfere with accurate assessment were excluded.
5. What variables were measured and indicate the level of measurement of each?
ANS: Pressure of the participants was measured. Also joint angle and seating positions were
noted.
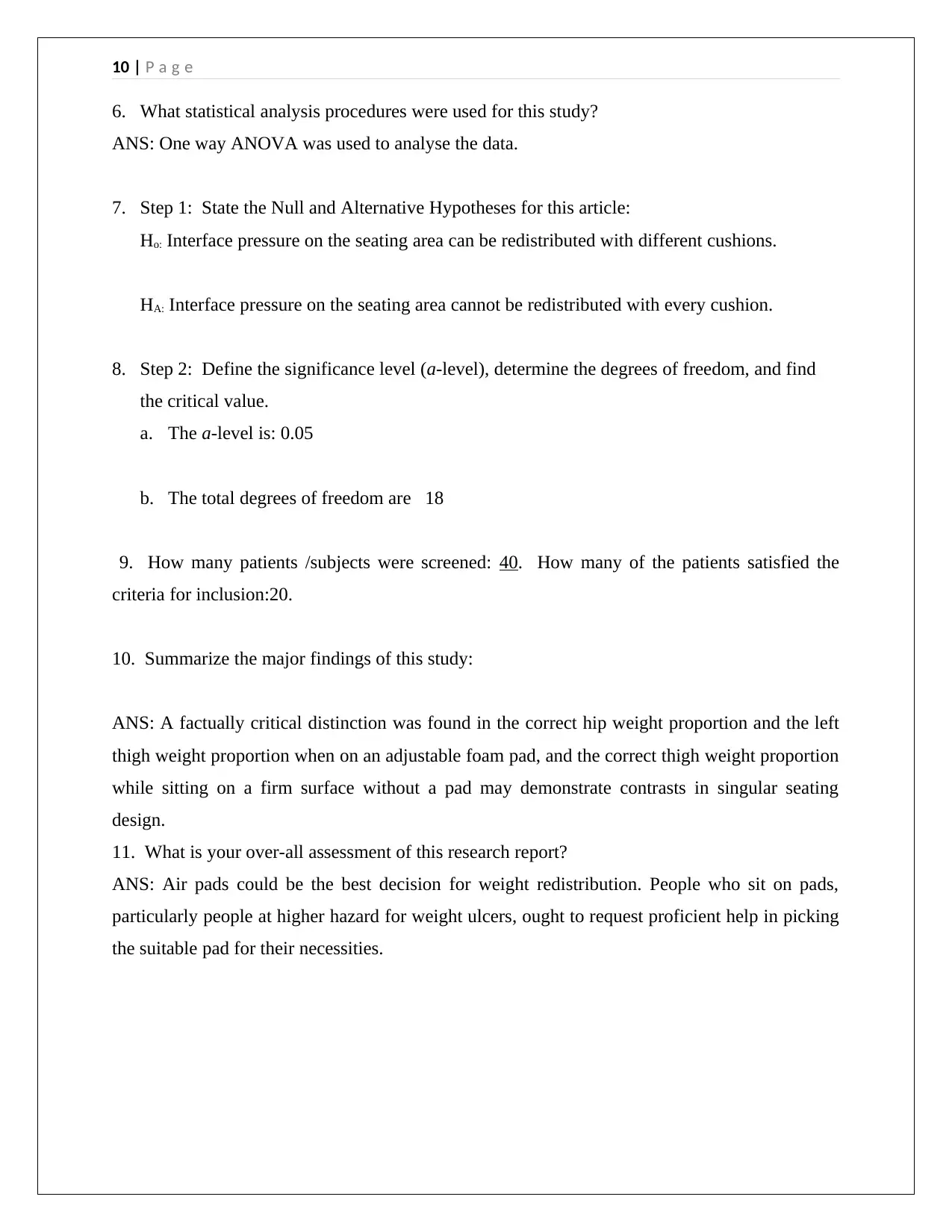
10 | P a g e
6. What statistical analysis procedures were used for this study?
ANS: One way ANOVA was used to analyse the data.
7. Step 1: State the Null and Alternative Hypotheses for this article:
Ho: Interface pressure on the seating area can be redistributed with different cushions.
HA: Interface pressure on the seating area cannot be redistributed with every cushion.
8. Step 2: Define the significance level (a-level), determine the degrees of freedom, and find
the critical value.
a. The a-level is: 0.05
b. The total degrees of freedom are 18
9. How many patients /subjects were screened: 40. How many of the patients satisfied the
criteria for inclusion:20.
10. Summarize the major findings of this study:
ANS: A factually critical distinction was found in the correct hip weight proportion and the left
thigh weight proportion when on an adjustable foam pad, and the correct thigh weight proportion
while sitting on a firm surface without a pad may demonstrate contrasts in singular seating
design.
11. What is your over-all assessment of this research report?
ANS: Air pads could be the best decision for weight redistribution. People who sit on pads,
particularly people at higher hazard for weight ulcers, ought to request proficient help in picking
the suitable pad for their necessities.
6. What statistical analysis procedures were used for this study?
ANS: One way ANOVA was used to analyse the data.
7. Step 1: State the Null and Alternative Hypotheses for this article:
Ho: Interface pressure on the seating area can be redistributed with different cushions.
HA: Interface pressure on the seating area cannot be redistributed with every cushion.
8. Step 2: Define the significance level (a-level), determine the degrees of freedom, and find
the critical value.
a. The a-level is: 0.05
b. The total degrees of freedom are 18
9. How many patients /subjects were screened: 40. How many of the patients satisfied the
criteria for inclusion:20.
10. Summarize the major findings of this study:
ANS: A factually critical distinction was found in the correct hip weight proportion and the left
thigh weight proportion when on an adjustable foam pad, and the correct thigh weight proportion
while sitting on a firm surface without a pad may demonstrate contrasts in singular seating
design.
11. What is your over-all assessment of this research report?
ANS: Air pads could be the best decision for weight redistribution. People who sit on pads,
particularly people at higher hazard for weight ulcers, ought to request proficient help in picking
the suitable pad for their necessities.
Secure Best Marks with AI Grader
Need help grading? Try our AI Grader for instant feedback on your assignments.
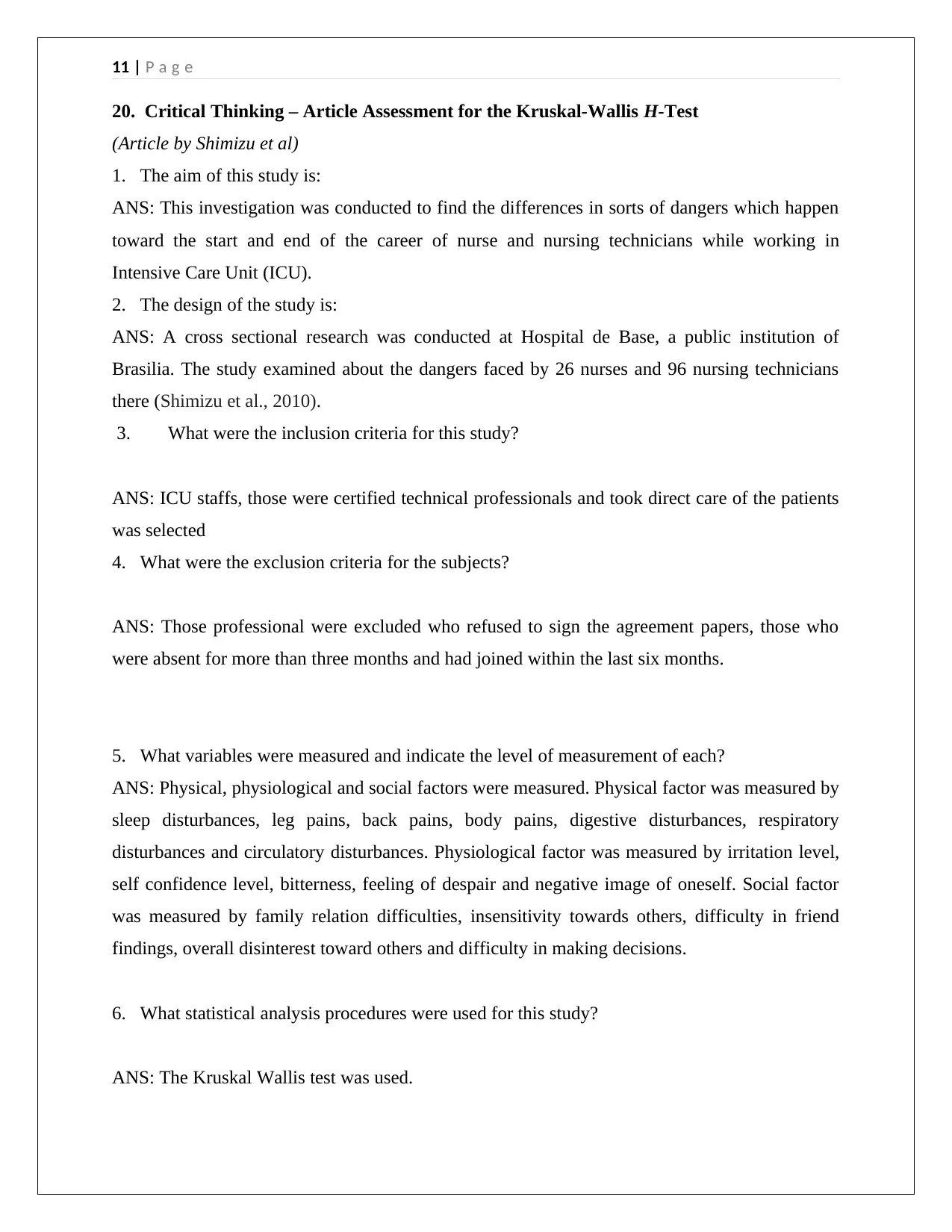
11 | P a g e
20. Critical Thinking – Article Assessment for the Kruskal-Wallis H-Test
(Article by Shimizu et al)
1. The aim of this study is:
ANS: This investigation was conducted to find the differences in sorts of dangers which happen
toward the start and end of the career of nurse and nursing technicians while working in
Intensive Care Unit (ICU).
2. The design of the study is:
ANS: A cross sectional research was conducted at Hospital de Base, a public institution of
Brasilia. The study examined about the dangers faced by 26 nurses and 96 nursing technicians
there (Shimizu et al., 2010).
3. What were the inclusion criteria for this study?
ANS: ICU staffs, those were certified technical professionals and took direct care of the patients
was selected
4. What were the exclusion criteria for the subjects?
ANS: Those professional were excluded who refused to sign the agreement papers, those who
were absent for more than three months and had joined within the last six months.
5. What variables were measured and indicate the level of measurement of each?
ANS: Physical, physiological and social factors were measured. Physical factor was measured by
sleep disturbances, leg pains, back pains, body pains, digestive disturbances, respiratory
disturbances and circulatory disturbances. Physiological factor was measured by irritation level,
self confidence level, bitterness, feeling of despair and negative image of oneself. Social factor
was measured by family relation difficulties, insensitivity towards others, difficulty in friend
findings, overall disinterest toward others and difficulty in making decisions.
6. What statistical analysis procedures were used for this study?
ANS: The Kruskal Wallis test was used.
20. Critical Thinking – Article Assessment for the Kruskal-Wallis H-Test
(Article by Shimizu et al)
1. The aim of this study is:
ANS: This investigation was conducted to find the differences in sorts of dangers which happen
toward the start and end of the career of nurse and nursing technicians while working in
Intensive Care Unit (ICU).
2. The design of the study is:
ANS: A cross sectional research was conducted at Hospital de Base, a public institution of
Brasilia. The study examined about the dangers faced by 26 nurses and 96 nursing technicians
there (Shimizu et al., 2010).
3. What were the inclusion criteria for this study?
ANS: ICU staffs, those were certified technical professionals and took direct care of the patients
was selected
4. What were the exclusion criteria for the subjects?
ANS: Those professional were excluded who refused to sign the agreement papers, those who
were absent for more than three months and had joined within the last six months.
5. What variables were measured and indicate the level of measurement of each?
ANS: Physical, physiological and social factors were measured. Physical factor was measured by
sleep disturbances, leg pains, back pains, body pains, digestive disturbances, respiratory
disturbances and circulatory disturbances. Physiological factor was measured by irritation level,
self confidence level, bitterness, feeling of despair and negative image of oneself. Social factor
was measured by family relation difficulties, insensitivity towards others, difficulty in friend
findings, overall disinterest toward others and difficulty in making decisions.
6. What statistical analysis procedures were used for this study?
ANS: The Kruskal Wallis test was used.
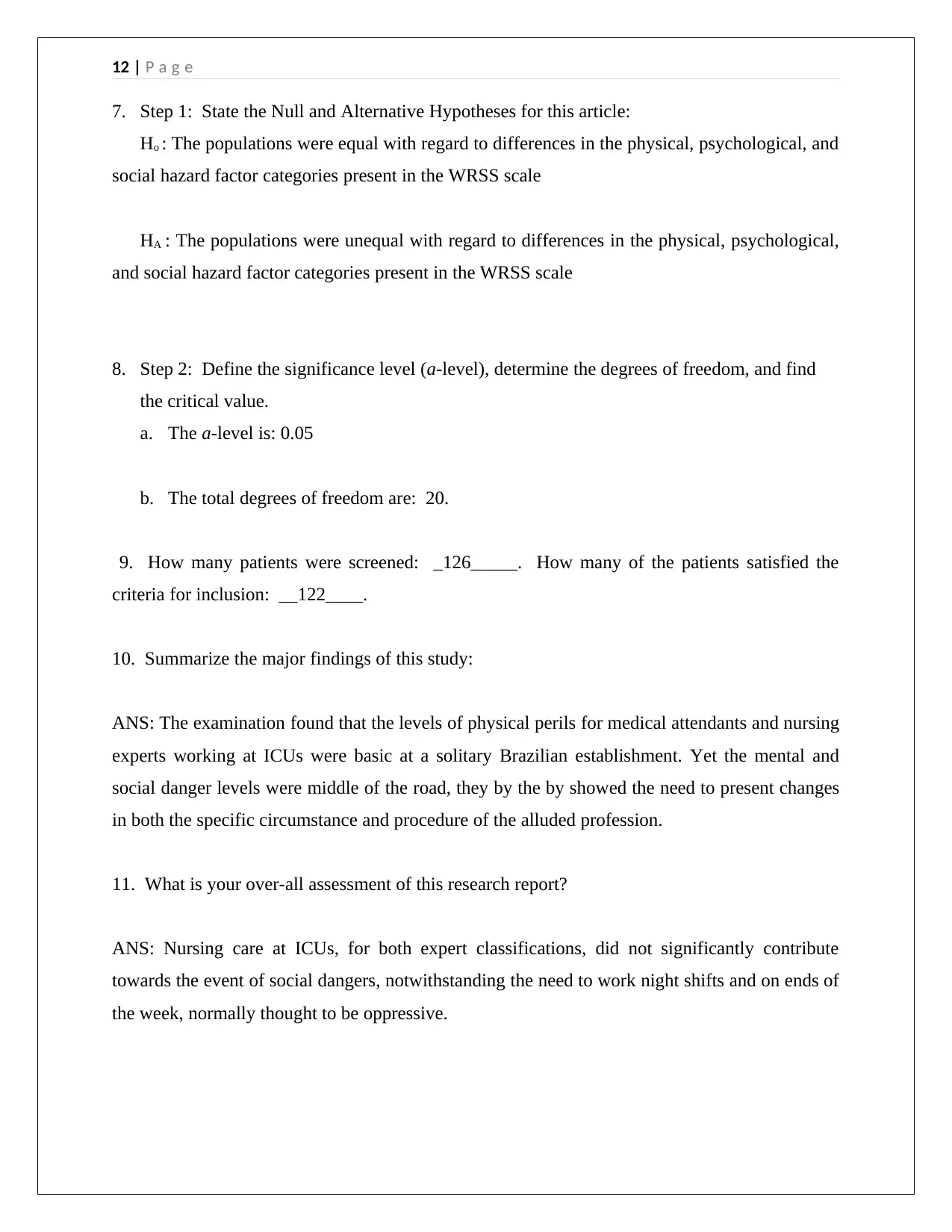
12 | P a g e
7. Step 1: State the Null and Alternative Hypotheses for this article:
Ho : The populations were equal with regard to differences in the physical, psychological, and
social hazard factor categories present in the WRSS scale
HA : The populations were unequal with regard to differences in the physical, psychological,
and social hazard factor categories present in the WRSS scale
8. Step 2: Define the significance level (a-level), determine the degrees of freedom, and find
the critical value.
a. The a-level is: 0.05
b. The total degrees of freedom are: 20.
9. How many patients were screened: _126_____. How many of the patients satisfied the
criteria for inclusion: __122____.
10. Summarize the major findings of this study:
ANS: The examination found that the levels of physical perils for medical attendants and nursing
experts working at ICUs were basic at a solitary Brazilian establishment. Yet the mental and
social danger levels were middle of the road, they by the by showed the need to present changes
in both the specific circumstance and procedure of the alluded profession.
11. What is your over-all assessment of this research report?
ANS: Nursing care at ICUs, for both expert classifications, did not significantly contribute
towards the event of social dangers, notwithstanding the need to work night shifts and on ends of
the week, normally thought to be oppressive.
7. Step 1: State the Null and Alternative Hypotheses for this article:
Ho : The populations were equal with regard to differences in the physical, psychological, and
social hazard factor categories present in the WRSS scale
HA : The populations were unequal with regard to differences in the physical, psychological,
and social hazard factor categories present in the WRSS scale
8. Step 2: Define the significance level (a-level), determine the degrees of freedom, and find
the critical value.
a. The a-level is: 0.05
b. The total degrees of freedom are: 20.
9. How many patients were screened: _126_____. How many of the patients satisfied the
criteria for inclusion: __122____.
10. Summarize the major findings of this study:
ANS: The examination found that the levels of physical perils for medical attendants and nursing
experts working at ICUs were basic at a solitary Brazilian establishment. Yet the mental and
social danger levels were middle of the road, they by the by showed the need to present changes
in both the specific circumstance and procedure of the alluded profession.
11. What is your over-all assessment of this research report?
ANS: Nursing care at ICUs, for both expert classifications, did not significantly contribute
towards the event of social dangers, notwithstanding the need to work night shifts and on ends of
the week, normally thought to be oppressive.
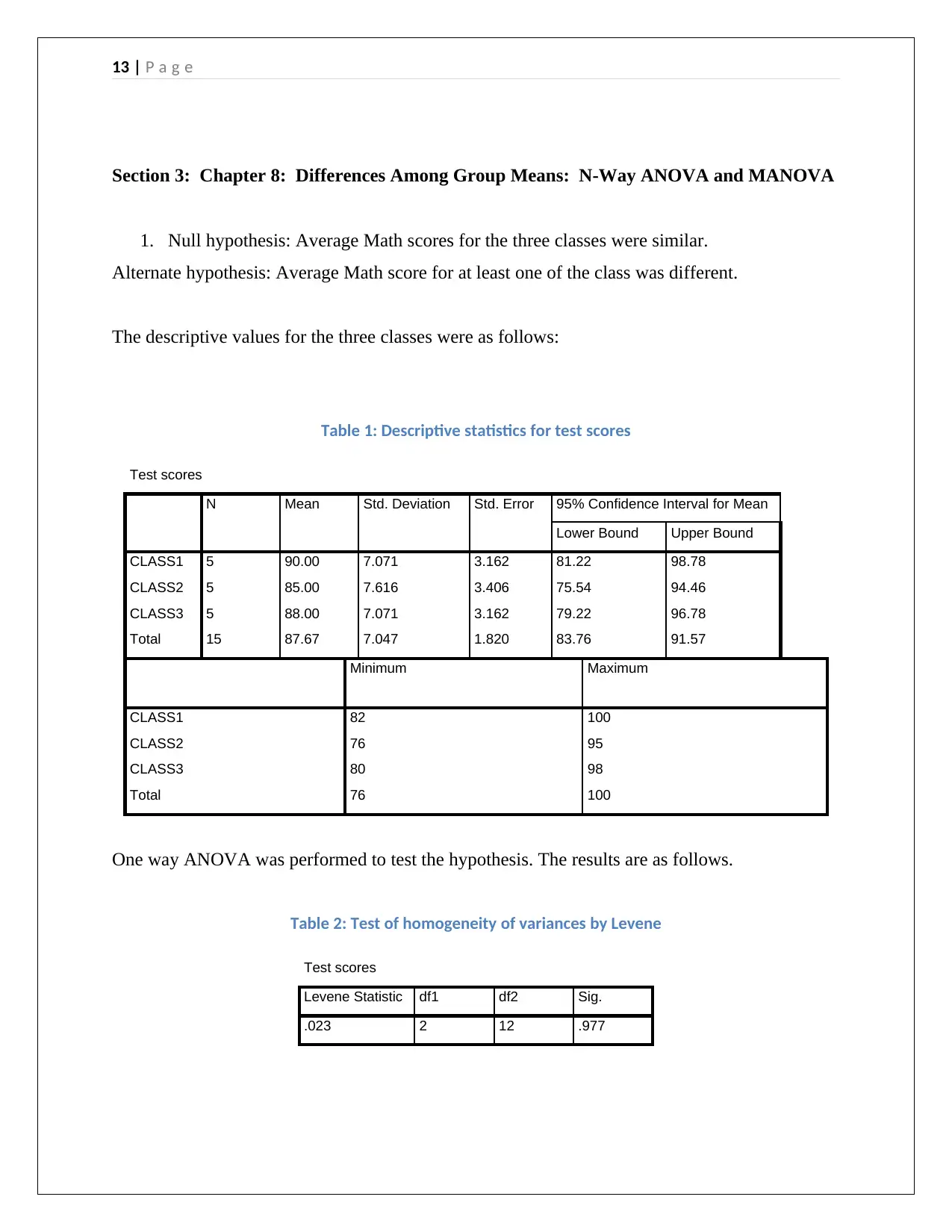
13 | P a g e
Section 3: Chapter 8: Differences Among Group Means: N-Way ANOVA and MANOVA
1. Null hypothesis: Average Math scores for the three classes were similar.
Alternate hypothesis: Average Math score for at least one of the class was different.
The descriptive values for the three classes were as follows:
Table 1: Descriptive statistics for test scores
Test scores
N Mean Std. Deviation Std. Error 95% Confidence Interval for Mean
Lower Bound Upper Bound
CLASS1 5 90.00 7.071 3.162 81.22 98.78
CLASS2 5 85.00 7.616 3.406 75.54 94.46
CLASS3 5 88.00 7.071 3.162 79.22 96.78
Total 15 87.67 7.047 1.820 83.76 91.57
Minimum Maximum
CLASS1 82 100
CLASS2 76 95
CLASS3 80 98
Total 76 100
One way ANOVA was performed to test the hypothesis. The results are as follows.
Table 2: Test of homogeneity of variances by Levene
Test scores
Levene Statistic df1 df2 Sig.
.023 2 12 .977
Section 3: Chapter 8: Differences Among Group Means: N-Way ANOVA and MANOVA
1. Null hypothesis: Average Math scores for the three classes were similar.
Alternate hypothesis: Average Math score for at least one of the class was different.
The descriptive values for the three classes were as follows:
Table 1: Descriptive statistics for test scores
Test scores
N Mean Std. Deviation Std. Error 95% Confidence Interval for Mean
Lower Bound Upper Bound
CLASS1 5 90.00 7.071 3.162 81.22 98.78
CLASS2 5 85.00 7.616 3.406 75.54 94.46
CLASS3 5 88.00 7.071 3.162 79.22 96.78
Total 15 87.67 7.047 1.820 83.76 91.57
Minimum Maximum
CLASS1 82 100
CLASS2 76 95
CLASS3 80 98
Total 76 100
One way ANOVA was performed to test the hypothesis. The results are as follows.
Table 2: Test of homogeneity of variances by Levene
Test scores
Levene Statistic df1 df2 Sig.
.023 2 12 .977
Paraphrase This Document
Need a fresh take? Get an instant paraphrase of this document with our AI Paraphraser
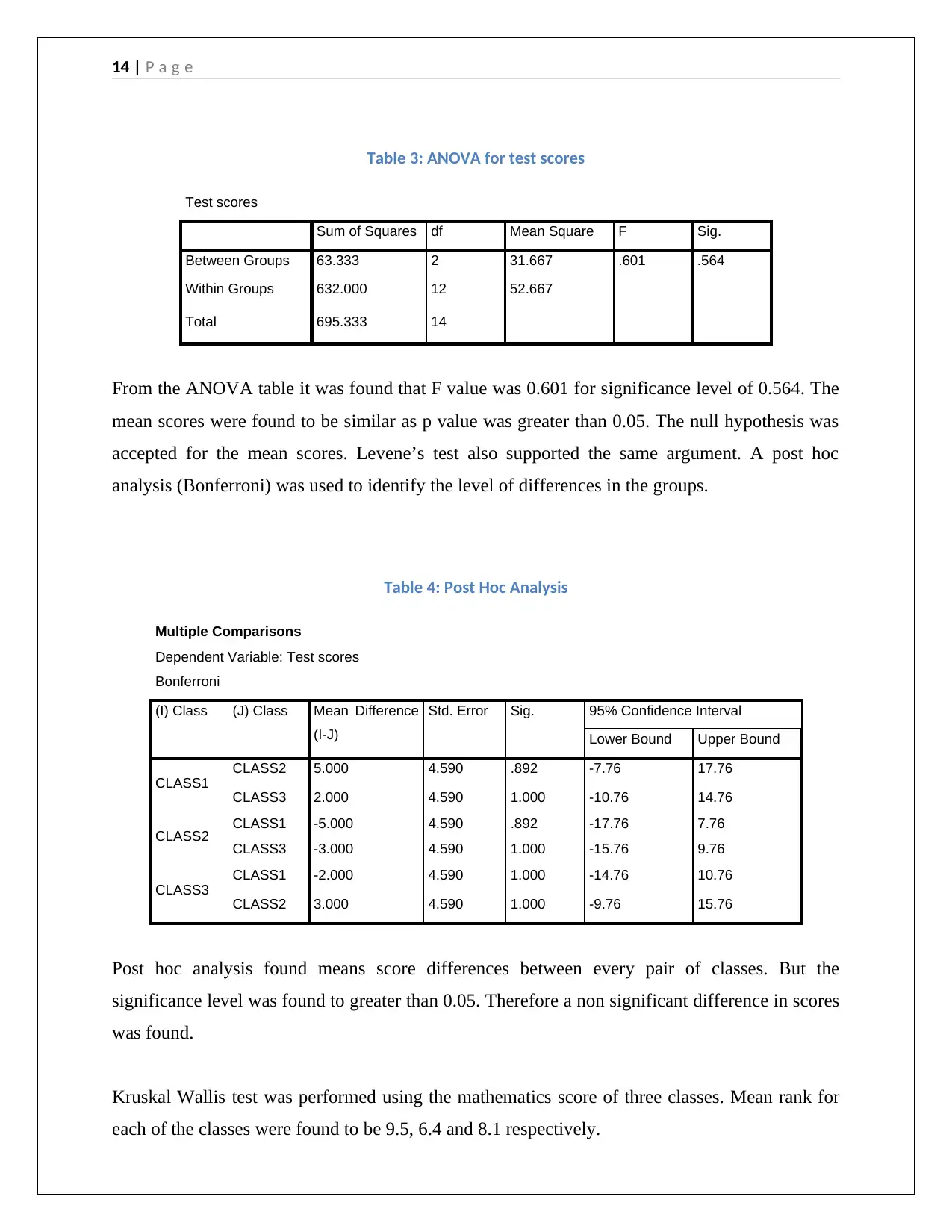
14 | P a g e
Table 3: ANOVA for test scores
Test scores
Sum of Squares df Mean Square F Sig.
Between Groups 63.333 2 31.667 .601 .564
Within Groups 632.000 12 52.667
Total 695.333 14
From the ANOVA table it was found that F value was 0.601 for significance level of 0.564. The
mean scores were found to be similar as p value was greater than 0.05. The null hypothesis was
accepted for the mean scores. Levene’s test also supported the same argument. A post hoc
analysis (Bonferroni) was used to identify the level of differences in the groups.
Table 4: Post Hoc Analysis
Multiple Comparisons
Dependent Variable: Test scores
Bonferroni
(I) Class (J) Class Mean Difference
(I-J)
Std. Error Sig. 95% Confidence Interval
Lower Bound Upper Bound
CLASS1
CLASS2 5.000 4.590 .892 -7.76 17.76
CLASS3 2.000 4.590 1.000 -10.76 14.76
CLASS2 CLASS1 -5.000 4.590 .892 -17.76 7.76
CLASS3 -3.000 4.590 1.000 -15.76 9.76
CLASS3
CLASS1 -2.000 4.590 1.000 -14.76 10.76
CLASS2 3.000 4.590 1.000 -9.76 15.76
Post hoc analysis found means score differences between every pair of classes. But the
significance level was found to greater than 0.05. Therefore a non significant difference in scores
was found.
Kruskal Wallis test was performed using the mathematics score of three classes. Mean rank for
each of the classes were found to be 9.5, 6.4 and 8.1 respectively.
Table 3: ANOVA for test scores
Test scores
Sum of Squares df Mean Square F Sig.
Between Groups 63.333 2 31.667 .601 .564
Within Groups 632.000 12 52.667
Total 695.333 14
From the ANOVA table it was found that F value was 0.601 for significance level of 0.564. The
mean scores were found to be similar as p value was greater than 0.05. The null hypothesis was
accepted for the mean scores. Levene’s test also supported the same argument. A post hoc
analysis (Bonferroni) was used to identify the level of differences in the groups.
Table 4: Post Hoc Analysis
Multiple Comparisons
Dependent Variable: Test scores
Bonferroni
(I) Class (J) Class Mean Difference
(I-J)
Std. Error Sig. 95% Confidence Interval
Lower Bound Upper Bound
CLASS1
CLASS2 5.000 4.590 .892 -7.76 17.76
CLASS3 2.000 4.590 1.000 -10.76 14.76
CLASS2 CLASS1 -5.000 4.590 .892 -17.76 7.76
CLASS3 -3.000 4.590 1.000 -15.76 9.76
CLASS3
CLASS1 -2.000 4.590 1.000 -14.76 10.76
CLASS2 3.000 4.590 1.000 -9.76 15.76
Post hoc analysis found means score differences between every pair of classes. But the
significance level was found to greater than 0.05. Therefore a non significant difference in scores
was found.
Kruskal Wallis test was performed using the mathematics score of three classes. Mean rank for
each of the classes were found to be 9.5, 6.4 and 8.1 respectively.
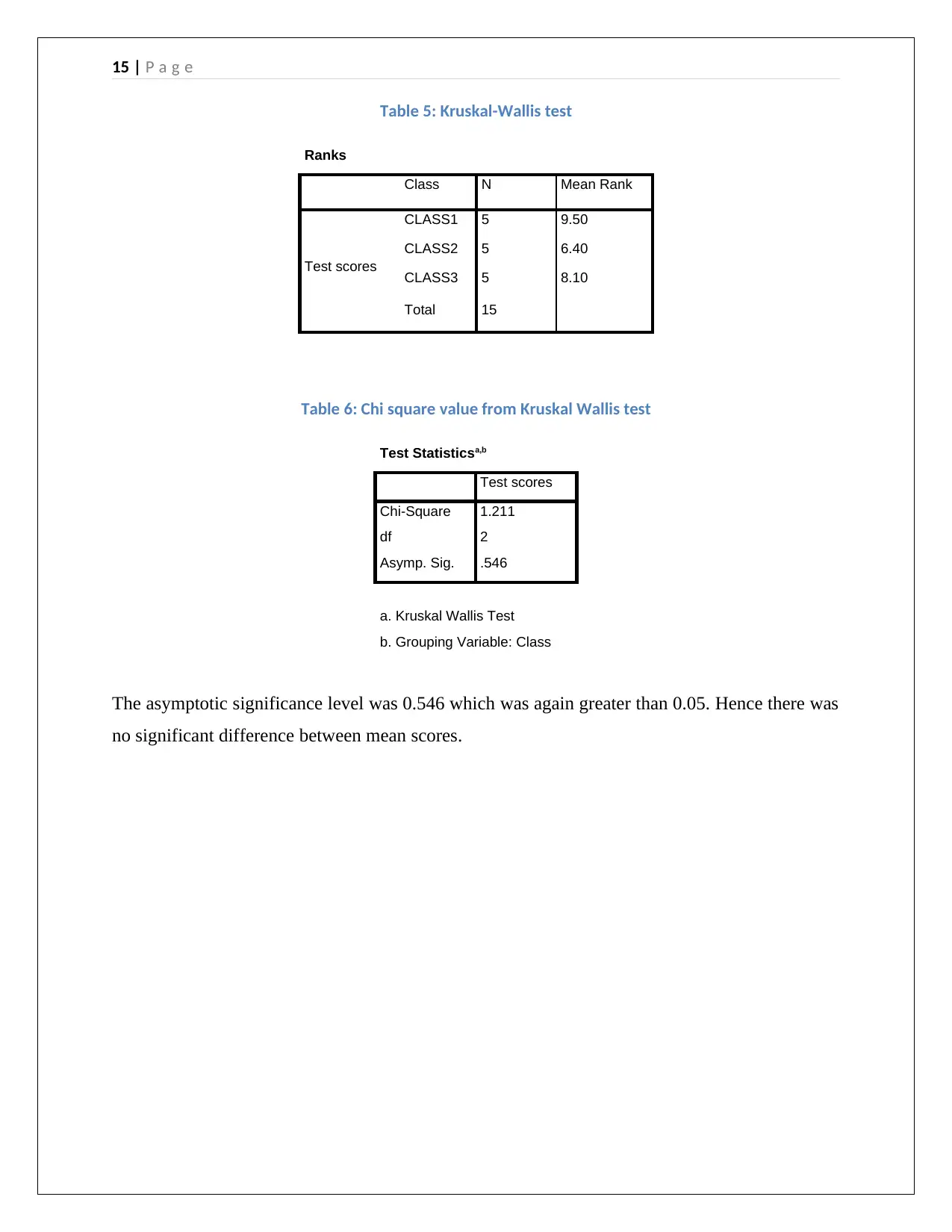
15 | P a g e
Table 5: Kruskal-Wallis test
Ranks
Class N Mean Rank
Test scores
CLASS1 5 9.50
CLASS2 5 6.40
CLASS3 5 8.10
Total 15
Table 6: Chi square value from Kruskal Wallis test
Test Statisticsa,b
Test scores
Chi-Square 1.211
df 2
Asymp. Sig. .546
a. Kruskal Wallis Test
b. Grouping Variable: Class
The asymptotic significance level was 0.546 which was again greater than 0.05. Hence there was
no significant difference between mean scores.
Table 5: Kruskal-Wallis test
Ranks
Class N Mean Rank
Test scores
CLASS1 5 9.50
CLASS2 5 6.40
CLASS3 5 8.10
Total 15
Table 6: Chi square value from Kruskal Wallis test
Test Statisticsa,b
Test scores
Chi-Square 1.211
df 2
Asymp. Sig. .546
a. Kruskal Wallis Test
b. Grouping Variable: Class
The asymptotic significance level was 0.546 which was again greater than 0.05. Hence there was
no significant difference between mean scores.
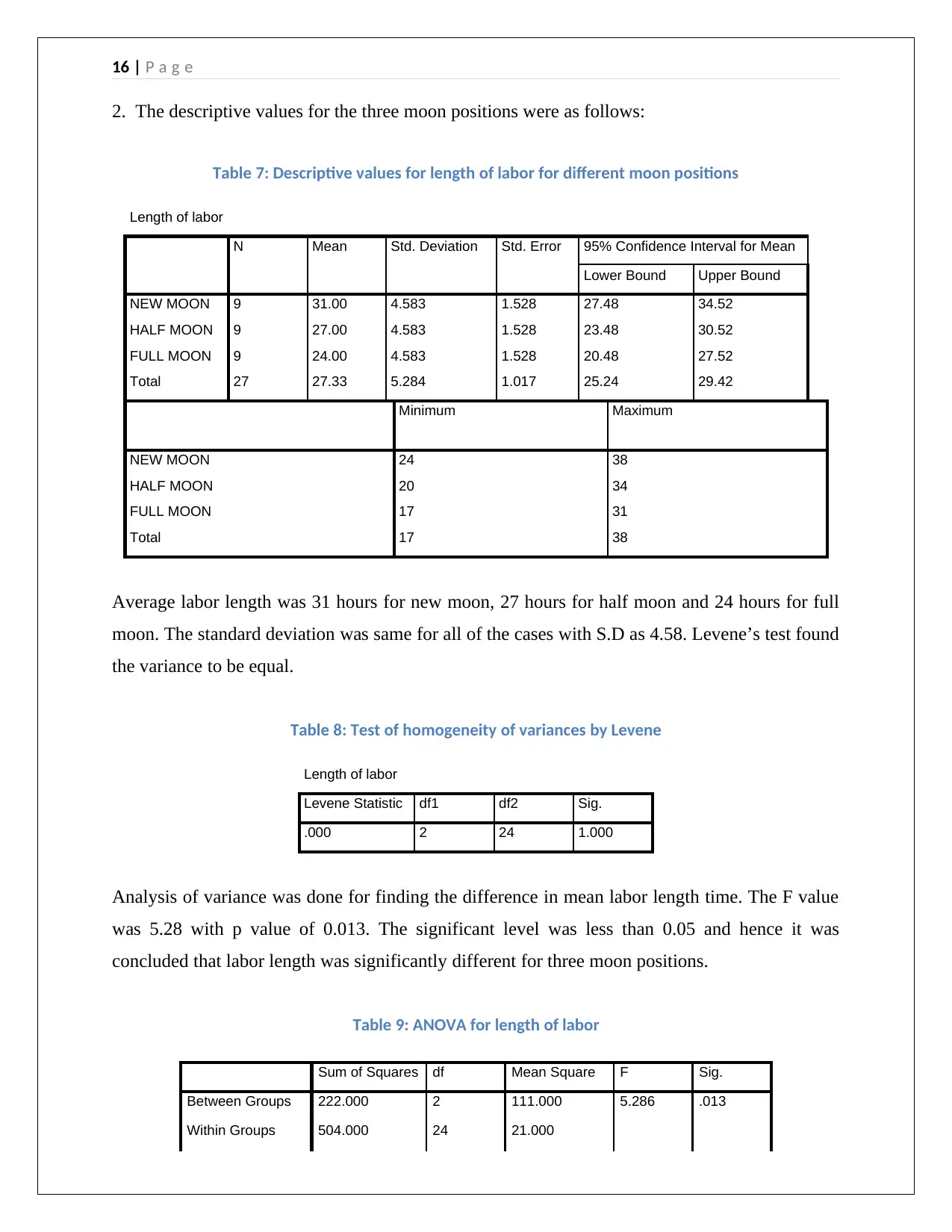
16 | P a g e
2. The descriptive values for the three moon positions were as follows:
Table 7: Descriptive values for length of labor for different moon positions
Length of labor
N Mean Std. Deviation Std. Error 95% Confidence Interval for Mean
Lower Bound Upper Bound
NEW MOON 9 31.00 4.583 1.528 27.48 34.52
HALF MOON 9 27.00 4.583 1.528 23.48 30.52
FULL MOON 9 24.00 4.583 1.528 20.48 27.52
Total 27 27.33 5.284 1.017 25.24 29.42
Minimum Maximum
NEW MOON 24 38
HALF MOON 20 34
FULL MOON 17 31
Total 17 38
Average labor length was 31 hours for new moon, 27 hours for half moon and 24 hours for full
moon. The standard deviation was same for all of the cases with S.D as 4.58. Levene’s test found
the variance to be equal.
Table 8: Test of homogeneity of variances by Levene
Length of labor
Levene Statistic df1 df2 Sig.
.000 2 24 1.000
Analysis of variance was done for finding the difference in mean labor length time. The F value
was 5.28 with p value of 0.013. The significant level was less than 0.05 and hence it was
concluded that labor length was significantly different for three moon positions.
Table 9: ANOVA for length of labor
Sum of Squares df Mean Square F Sig.
Between Groups 222.000 2 111.000 5.286 .013
Within Groups 504.000 24 21.000
2. The descriptive values for the three moon positions were as follows:
Table 7: Descriptive values for length of labor for different moon positions
Length of labor
N Mean Std. Deviation Std. Error 95% Confidence Interval for Mean
Lower Bound Upper Bound
NEW MOON 9 31.00 4.583 1.528 27.48 34.52
HALF MOON 9 27.00 4.583 1.528 23.48 30.52
FULL MOON 9 24.00 4.583 1.528 20.48 27.52
Total 27 27.33 5.284 1.017 25.24 29.42
Minimum Maximum
NEW MOON 24 38
HALF MOON 20 34
FULL MOON 17 31
Total 17 38
Average labor length was 31 hours for new moon, 27 hours for half moon and 24 hours for full
moon. The standard deviation was same for all of the cases with S.D as 4.58. Levene’s test found
the variance to be equal.
Table 8: Test of homogeneity of variances by Levene
Length of labor
Levene Statistic df1 df2 Sig.
.000 2 24 1.000
Analysis of variance was done for finding the difference in mean labor length time. The F value
was 5.28 with p value of 0.013. The significant level was less than 0.05 and hence it was
concluded that labor length was significantly different for three moon positions.
Table 9: ANOVA for length of labor
Sum of Squares df Mean Square F Sig.
Between Groups 222.000 2 111.000 5.286 .013
Within Groups 504.000 24 21.000
Secure Best Marks with AI Grader
Need help grading? Try our AI Grader for instant feedback on your assignments.
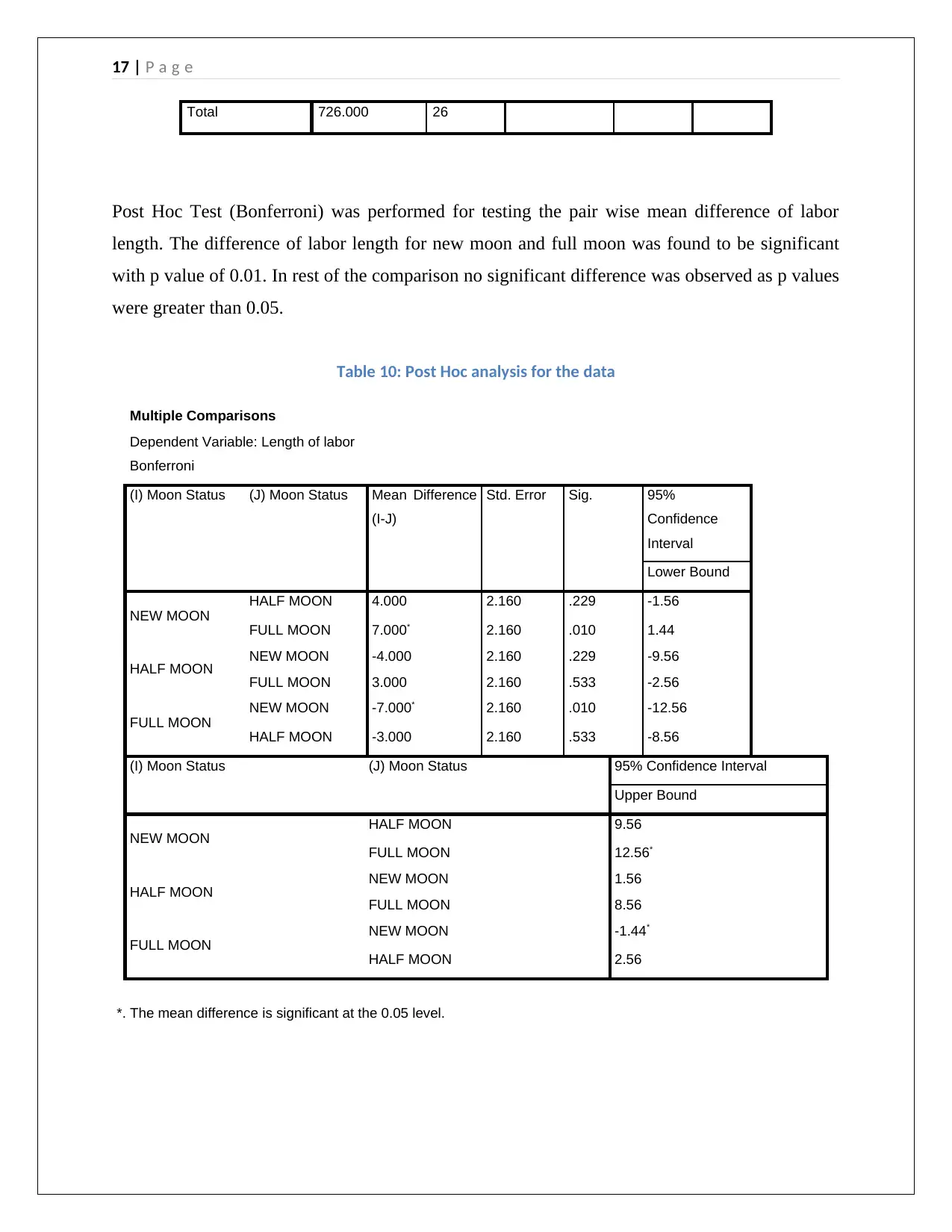
17 | P a g e
Total 726.000 26
Post Hoc Test (Bonferroni) was performed for testing the pair wise mean difference of labor
length. The difference of labor length for new moon and full moon was found to be significant
with p value of 0.01. In rest of the comparison no significant difference was observed as p values
were greater than 0.05.
Table 10: Post Hoc analysis for the data
Multiple Comparisons
Dependent Variable: Length of labor
Bonferroni
(I) Moon Status (J) Moon Status Mean Difference
(I-J)
Std. Error Sig. 95%
Confidence
Interval
Lower Bound
NEW MOON
HALF MOON 4.000 2.160 .229 -1.56
FULL MOON 7.000* 2.160 .010 1.44
HALF MOON NEW MOON -4.000 2.160 .229 -9.56
FULL MOON 3.000 2.160 .533 -2.56
FULL MOON
NEW MOON -7.000* 2.160 .010 -12.56
HALF MOON -3.000 2.160 .533 -8.56
(I) Moon Status (J) Moon Status 95% Confidence Interval
Upper Bound
NEW MOON
HALF MOON 9.56
FULL MOON 12.56*
HALF MOON NEW MOON 1.56
FULL MOON 8.56
FULL MOON
NEW MOON -1.44*
HALF MOON 2.56
*. The mean difference is significant at the 0.05 level.
Total 726.000 26
Post Hoc Test (Bonferroni) was performed for testing the pair wise mean difference of labor
length. The difference of labor length for new moon and full moon was found to be significant
with p value of 0.01. In rest of the comparison no significant difference was observed as p values
were greater than 0.05.
Table 10: Post Hoc analysis for the data
Multiple Comparisons
Dependent Variable: Length of labor
Bonferroni
(I) Moon Status (J) Moon Status Mean Difference
(I-J)
Std. Error Sig. 95%
Confidence
Interval
Lower Bound
NEW MOON
HALF MOON 4.000 2.160 .229 -1.56
FULL MOON 7.000* 2.160 .010 1.44
HALF MOON NEW MOON -4.000 2.160 .229 -9.56
FULL MOON 3.000 2.160 .533 -2.56
FULL MOON
NEW MOON -7.000* 2.160 .010 -12.56
HALF MOON -3.000 2.160 .533 -8.56
(I) Moon Status (J) Moon Status 95% Confidence Interval
Upper Bound
NEW MOON
HALF MOON 9.56
FULL MOON 12.56*
HALF MOON NEW MOON 1.56
FULL MOON 8.56
FULL MOON
NEW MOON -1.44*
HALF MOON 2.56
*. The mean difference is significant at the 0.05 level.
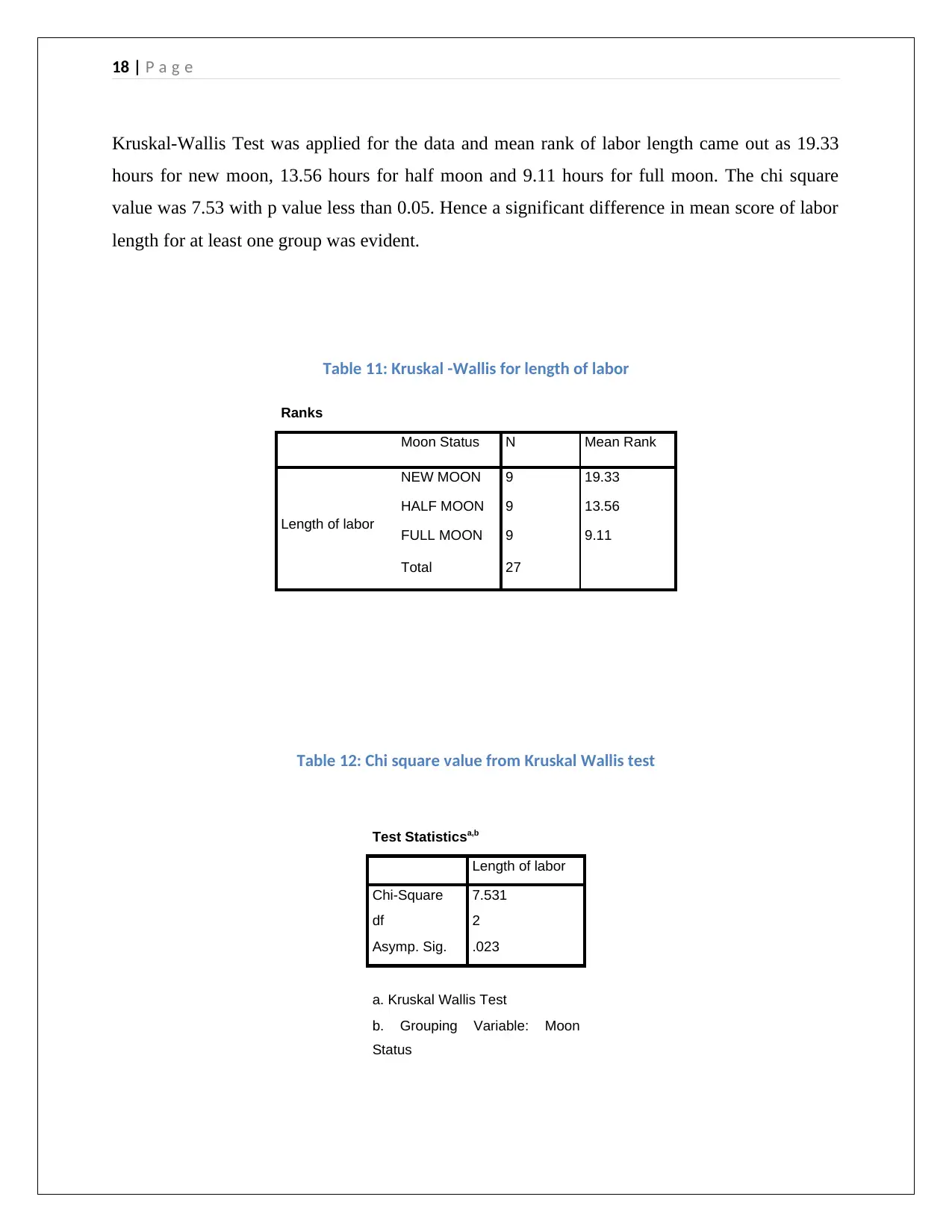
18 | P a g e
Kruskal-Wallis Test was applied for the data and mean rank of labor length came out as 19.33
hours for new moon, 13.56 hours for half moon and 9.11 hours for full moon. The chi square
value was 7.53 with p value less than 0.05. Hence a significant difference in mean score of labor
length for at least one group was evident.
Table 11: Kruskal -Wallis for length of labor
Ranks
Moon Status N Mean Rank
Length of labor
NEW MOON 9 19.33
HALF MOON 9 13.56
FULL MOON 9 9.11
Total 27
Table 12: Chi square value from Kruskal Wallis test
Test Statisticsa,b
Length of labor
Chi-Square 7.531
df 2
Asymp. Sig. .023
a. Kruskal Wallis Test
b. Grouping Variable: Moon
Status
Kruskal-Wallis Test was applied for the data and mean rank of labor length came out as 19.33
hours for new moon, 13.56 hours for half moon and 9.11 hours for full moon. The chi square
value was 7.53 with p value less than 0.05. Hence a significant difference in mean score of labor
length for at least one group was evident.
Table 11: Kruskal -Wallis for length of labor
Ranks
Moon Status N Mean Rank
Length of labor
NEW MOON 9 19.33
HALF MOON 9 13.56
FULL MOON 9 9.11
Total 27
Table 12: Chi square value from Kruskal Wallis test
Test Statisticsa,b
Length of labor
Chi-Square 7.531
df 2
Asymp. Sig. .023
a. Kruskal Wallis Test
b. Grouping Variable: Moon
Status
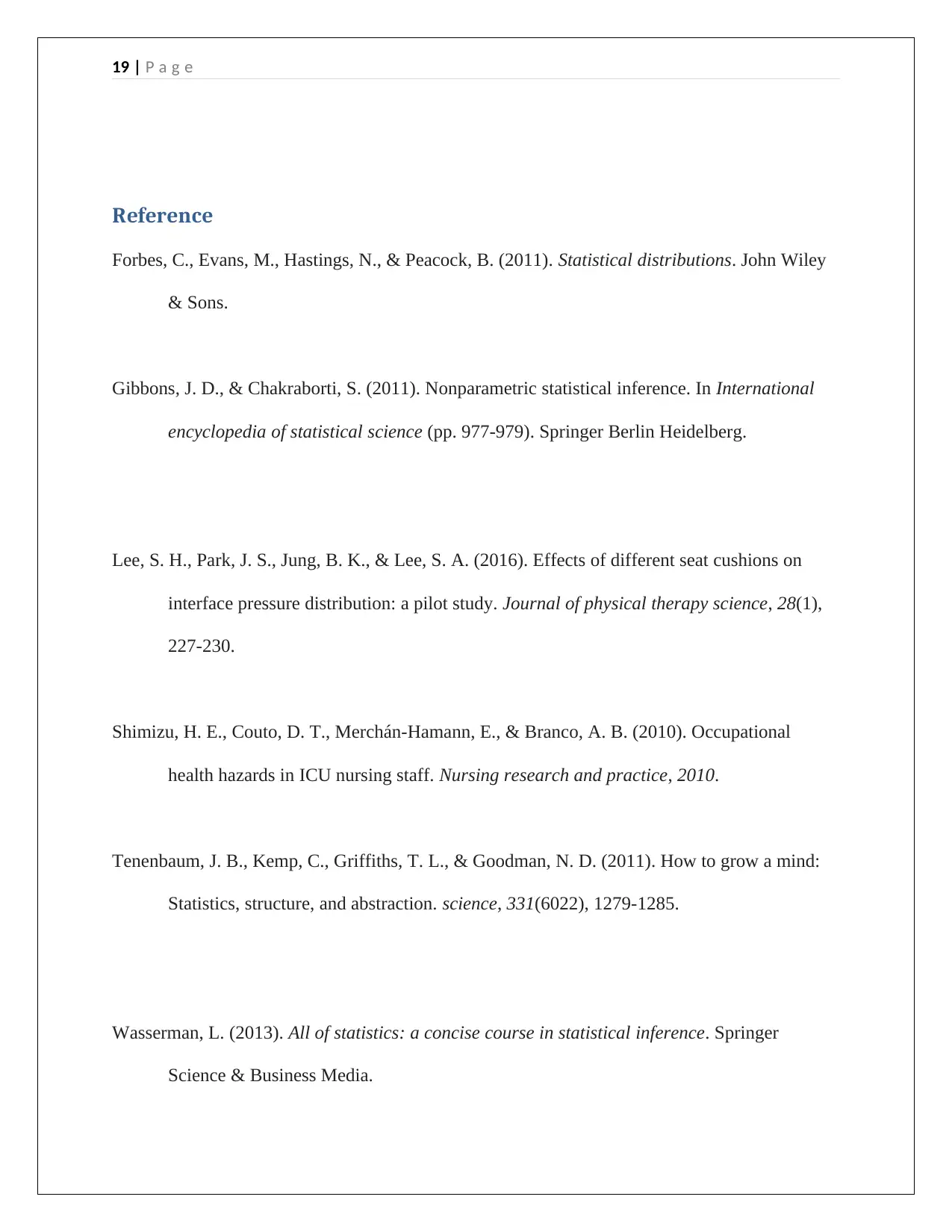
19 | P a g e
Reference
Forbes, C., Evans, M., Hastings, N., & Peacock, B. (2011). Statistical distributions. John Wiley
& Sons.
Gibbons, J. D., & Chakraborti, S. (2011). Nonparametric statistical inference. In International
encyclopedia of statistical science (pp. 977-979). Springer Berlin Heidelberg.
Lee, S. H., Park, J. S., Jung, B. K., & Lee, S. A. (2016). Effects of different seat cushions on
interface pressure distribution: a pilot study. Journal of physical therapy science, 28(1),
227-230.
Shimizu, H. E., Couto, D. T., Merchán-Hamann, E., & Branco, A. B. (2010). Occupational
health hazards in ICU nursing staff. Nursing research and practice, 2010.
Tenenbaum, J. B., Kemp, C., Griffiths, T. L., & Goodman, N. D. (2011). How to grow a mind:
Statistics, structure, and abstraction. science, 331(6022), 1279-1285.
Wasserman, L. (2013). All of statistics: a concise course in statistical inference. Springer
Science & Business Media.
Reference
Forbes, C., Evans, M., Hastings, N., & Peacock, B. (2011). Statistical distributions. John Wiley
& Sons.
Gibbons, J. D., & Chakraborti, S. (2011). Nonparametric statistical inference. In International
encyclopedia of statistical science (pp. 977-979). Springer Berlin Heidelberg.
Lee, S. H., Park, J. S., Jung, B. K., & Lee, S. A. (2016). Effects of different seat cushions on
interface pressure distribution: a pilot study. Journal of physical therapy science, 28(1),
227-230.
Shimizu, H. E., Couto, D. T., Merchán-Hamann, E., & Branco, A. B. (2010). Occupational
health hazards in ICU nursing staff. Nursing research and practice, 2010.
Tenenbaum, J. B., Kemp, C., Griffiths, T. L., & Goodman, N. D. (2011). How to grow a mind:
Statistics, structure, and abstraction. science, 331(6022), 1279-1285.
Wasserman, L. (2013). All of statistics: a concise course in statistical inference. Springer
Science & Business Media.
1 out of 19
![[object Object]](/_next/image/?url=%2F_next%2Fstatic%2Fmedia%2Flogo.6d15ce61.png&w=640&q=75)
Your All-in-One AI-Powered Toolkit for Academic Success.
+13062052269
info@desklib.com
Available 24*7 on WhatsApp / Email
Unlock your academic potential
© 2024 | Zucol Services PVT LTD | All rights reserved.