Effect of Big Data in Banking Sector
VerifiedAdded on 2023/04/22
|88
|20410
|156
AI Summary
Contribute Materials
Your contribution can guide someone’s learning journey. Share your
documents today.
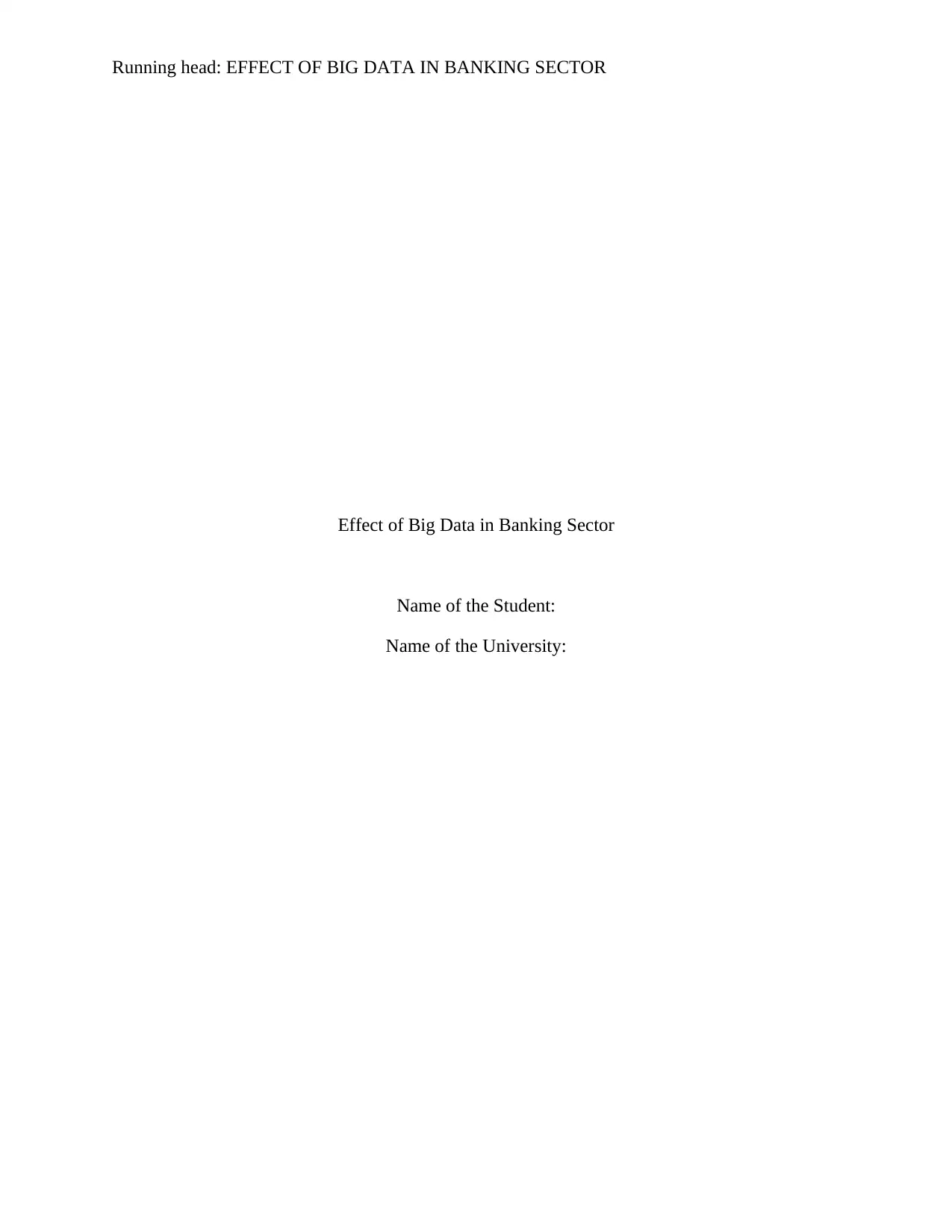
Running head: EFFECT OF BIG DATA IN BANKING SECTOR
Effect of Big Data in Banking Sector
Name of the Student:
Name of the University:
Effect of Big Data in Banking Sector
Name of the Student:
Name of the University:
Secure Best Marks with AI Grader
Need help grading? Try our AI Grader for instant feedback on your assignments.
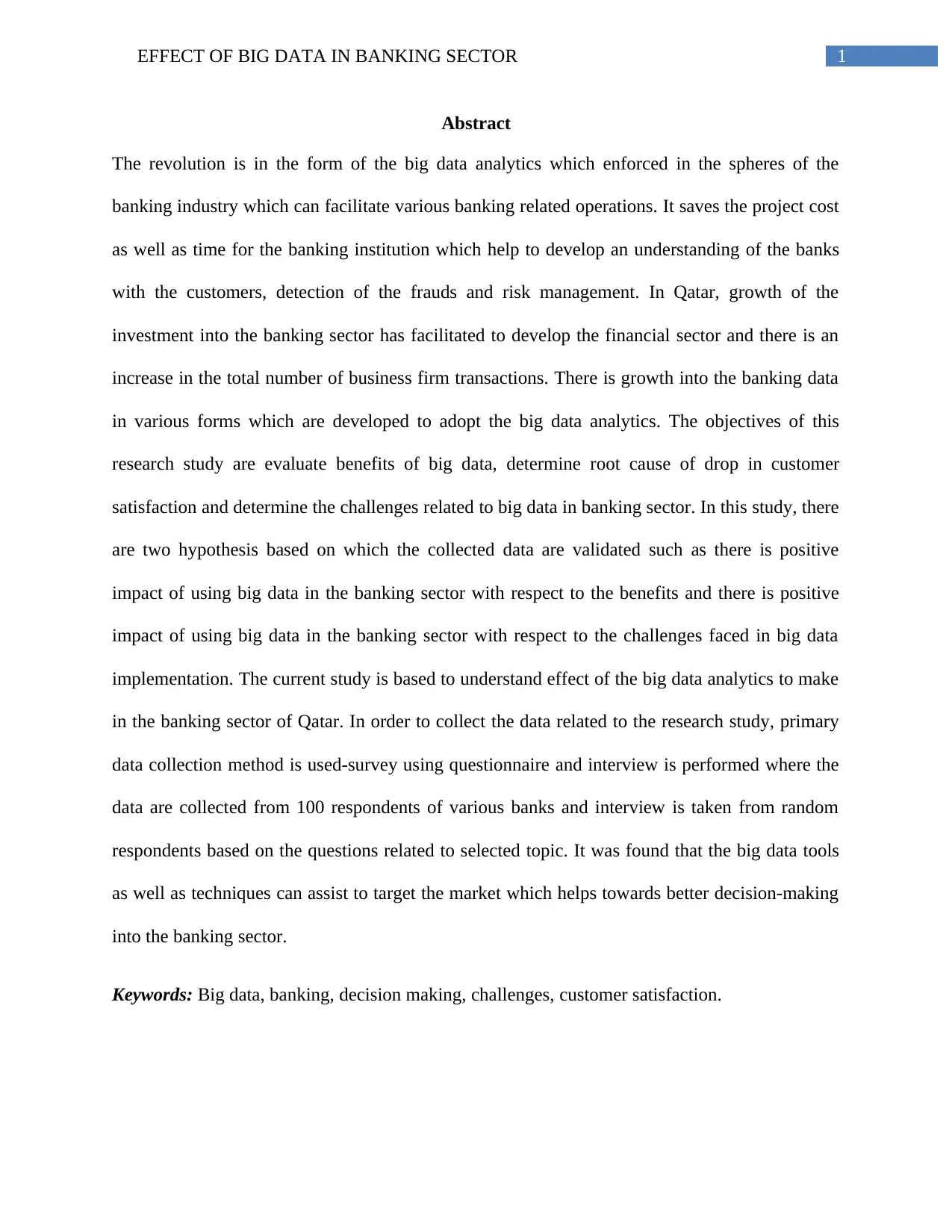
1EFFECT OF BIG DATA IN BANKING SECTOR
Abstract
The revolution is in the form of the big data analytics which enforced in the spheres of the
banking industry which can facilitate various banking related operations. It saves the project cost
as well as time for the banking institution which help to develop an understanding of the banks
with the customers, detection of the frauds and risk management. In Qatar, growth of the
investment into the banking sector has facilitated to develop the financial sector and there is an
increase in the total number of business firm transactions. There is growth into the banking data
in various forms which are developed to adopt the big data analytics. The objectives of this
research study are evaluate benefits of big data, determine root cause of drop in customer
satisfaction and determine the challenges related to big data in banking sector. In this study, there
are two hypothesis based on which the collected data are validated such as there is positive
impact of using big data in the banking sector with respect to the benefits and there is positive
impact of using big data in the banking sector with respect to the challenges faced in big data
implementation. The current study is based to understand effect of the big data analytics to make
in the banking sector of Qatar. In order to collect the data related to the research study, primary
data collection method is used-survey using questionnaire and interview is performed where the
data are collected from 100 respondents of various banks and interview is taken from random
respondents based on the questions related to selected topic. It was found that the big data tools
as well as techniques can assist to target the market which helps towards better decision-making
into the banking sector.
Keywords: Big data, banking, decision making, challenges, customer satisfaction.
Abstract
The revolution is in the form of the big data analytics which enforced in the spheres of the
banking industry which can facilitate various banking related operations. It saves the project cost
as well as time for the banking institution which help to develop an understanding of the banks
with the customers, detection of the frauds and risk management. In Qatar, growth of the
investment into the banking sector has facilitated to develop the financial sector and there is an
increase in the total number of business firm transactions. There is growth into the banking data
in various forms which are developed to adopt the big data analytics. The objectives of this
research study are evaluate benefits of big data, determine root cause of drop in customer
satisfaction and determine the challenges related to big data in banking sector. In this study, there
are two hypothesis based on which the collected data are validated such as there is positive
impact of using big data in the banking sector with respect to the benefits and there is positive
impact of using big data in the banking sector with respect to the challenges faced in big data
implementation. The current study is based to understand effect of the big data analytics to make
in the banking sector of Qatar. In order to collect the data related to the research study, primary
data collection method is used-survey using questionnaire and interview is performed where the
data are collected from 100 respondents of various banks and interview is taken from random
respondents based on the questions related to selected topic. It was found that the big data tools
as well as techniques can assist to target the market which helps towards better decision-making
into the banking sector.
Keywords: Big data, banking, decision making, challenges, customer satisfaction.
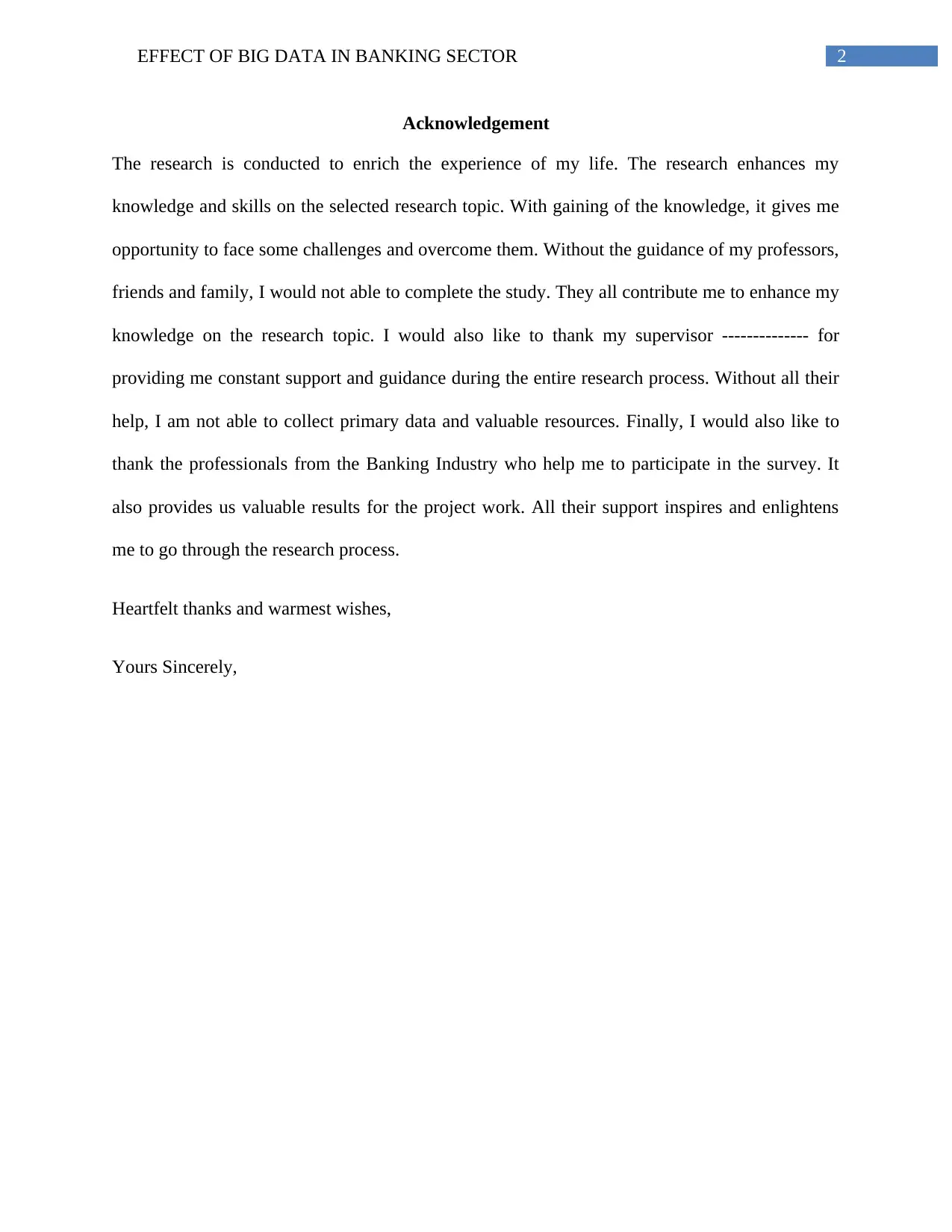
2EFFECT OF BIG DATA IN BANKING SECTOR
Acknowledgement
The research is conducted to enrich the experience of my life. The research enhances my
knowledge and skills on the selected research topic. With gaining of the knowledge, it gives me
opportunity to face some challenges and overcome them. Without the guidance of my professors,
friends and family, I would not able to complete the study. They all contribute me to enhance my
knowledge on the research topic. I would also like to thank my supervisor -------------- for
providing me constant support and guidance during the entire research process. Without all their
help, I am not able to collect primary data and valuable resources. Finally, I would also like to
thank the professionals from the Banking Industry who help me to participate in the survey. It
also provides us valuable results for the project work. All their support inspires and enlightens
me to go through the research process.
Heartfelt thanks and warmest wishes,
Yours Sincerely,
Acknowledgement
The research is conducted to enrich the experience of my life. The research enhances my
knowledge and skills on the selected research topic. With gaining of the knowledge, it gives me
opportunity to face some challenges and overcome them. Without the guidance of my professors,
friends and family, I would not able to complete the study. They all contribute me to enhance my
knowledge on the research topic. I would also like to thank my supervisor -------------- for
providing me constant support and guidance during the entire research process. Without all their
help, I am not able to collect primary data and valuable resources. Finally, I would also like to
thank the professionals from the Banking Industry who help me to participate in the survey. It
also provides us valuable results for the project work. All their support inspires and enlightens
me to go through the research process.
Heartfelt thanks and warmest wishes,
Yours Sincerely,
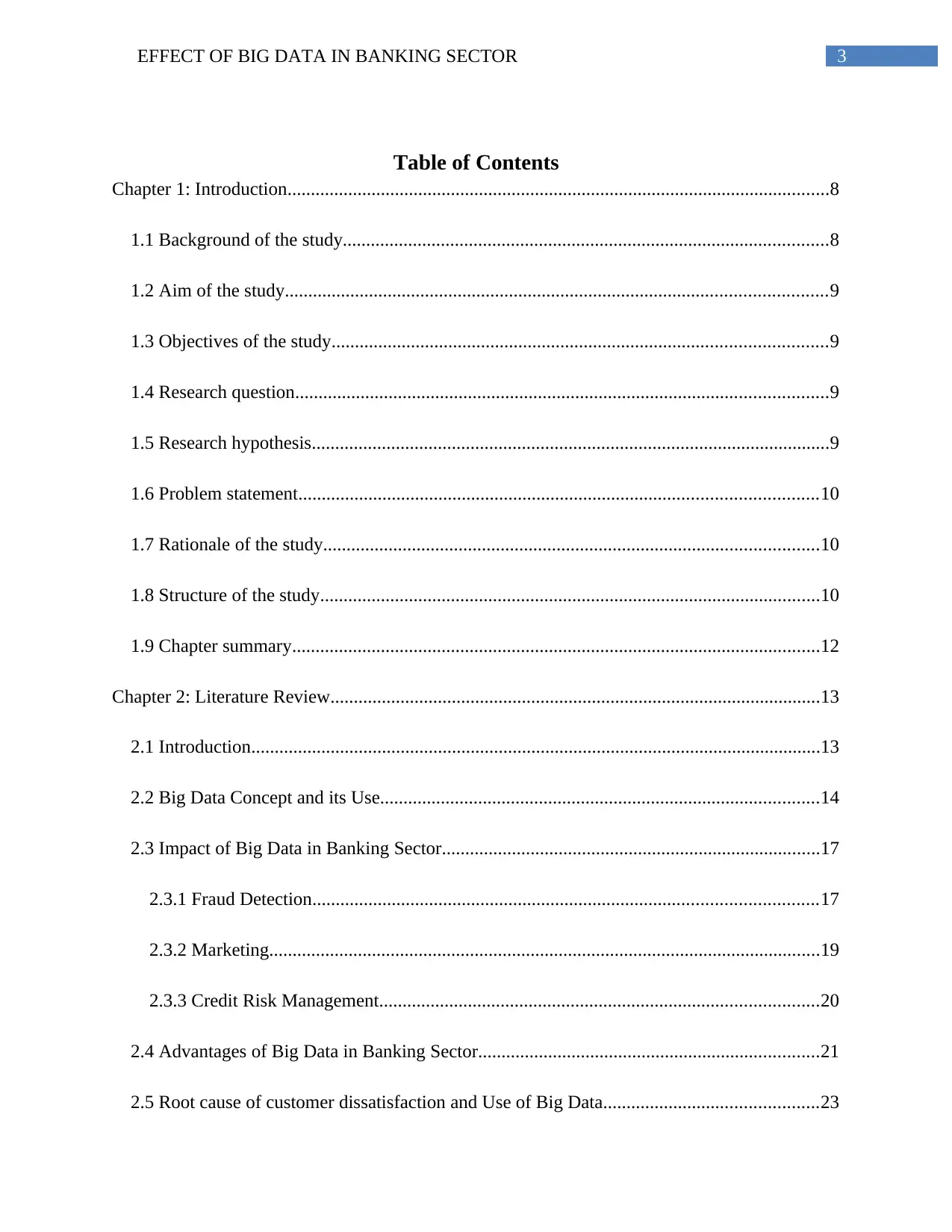
3EFFECT OF BIG DATA IN BANKING SECTOR
Table of Contents
Chapter 1: Introduction....................................................................................................................8
1.1 Background of the study........................................................................................................8
1.2 Aim of the study....................................................................................................................9
1.3 Objectives of the study..........................................................................................................9
1.4 Research question..................................................................................................................9
1.5 Research hypothesis...............................................................................................................9
1.6 Problem statement...............................................................................................................10
1.7 Rationale of the study..........................................................................................................10
1.8 Structure of the study...........................................................................................................10
1.9 Chapter summary.................................................................................................................12
Chapter 2: Literature Review.........................................................................................................13
2.1 Introduction..........................................................................................................................13
2.2 Big Data Concept and its Use..............................................................................................14
2.3 Impact of Big Data in Banking Sector.................................................................................17
2.3.1 Fraud Detection............................................................................................................17
2.3.2 Marketing......................................................................................................................19
2.3.3 Credit Risk Management..............................................................................................20
2.4 Advantages of Big Data in Banking Sector.........................................................................21
2.5 Root cause of customer dissatisfaction and Use of Big Data..............................................23
Table of Contents
Chapter 1: Introduction....................................................................................................................8
1.1 Background of the study........................................................................................................8
1.2 Aim of the study....................................................................................................................9
1.3 Objectives of the study..........................................................................................................9
1.4 Research question..................................................................................................................9
1.5 Research hypothesis...............................................................................................................9
1.6 Problem statement...............................................................................................................10
1.7 Rationale of the study..........................................................................................................10
1.8 Structure of the study...........................................................................................................10
1.9 Chapter summary.................................................................................................................12
Chapter 2: Literature Review.........................................................................................................13
2.1 Introduction..........................................................................................................................13
2.2 Big Data Concept and its Use..............................................................................................14
2.3 Impact of Big Data in Banking Sector.................................................................................17
2.3.1 Fraud Detection............................................................................................................17
2.3.2 Marketing......................................................................................................................19
2.3.3 Credit Risk Management..............................................................................................20
2.4 Advantages of Big Data in Banking Sector.........................................................................21
2.5 Root cause of customer dissatisfaction and Use of Big Data..............................................23
Paraphrase This Document
Need a fresh take? Get an instant paraphrase of this document with our AI Paraphraser
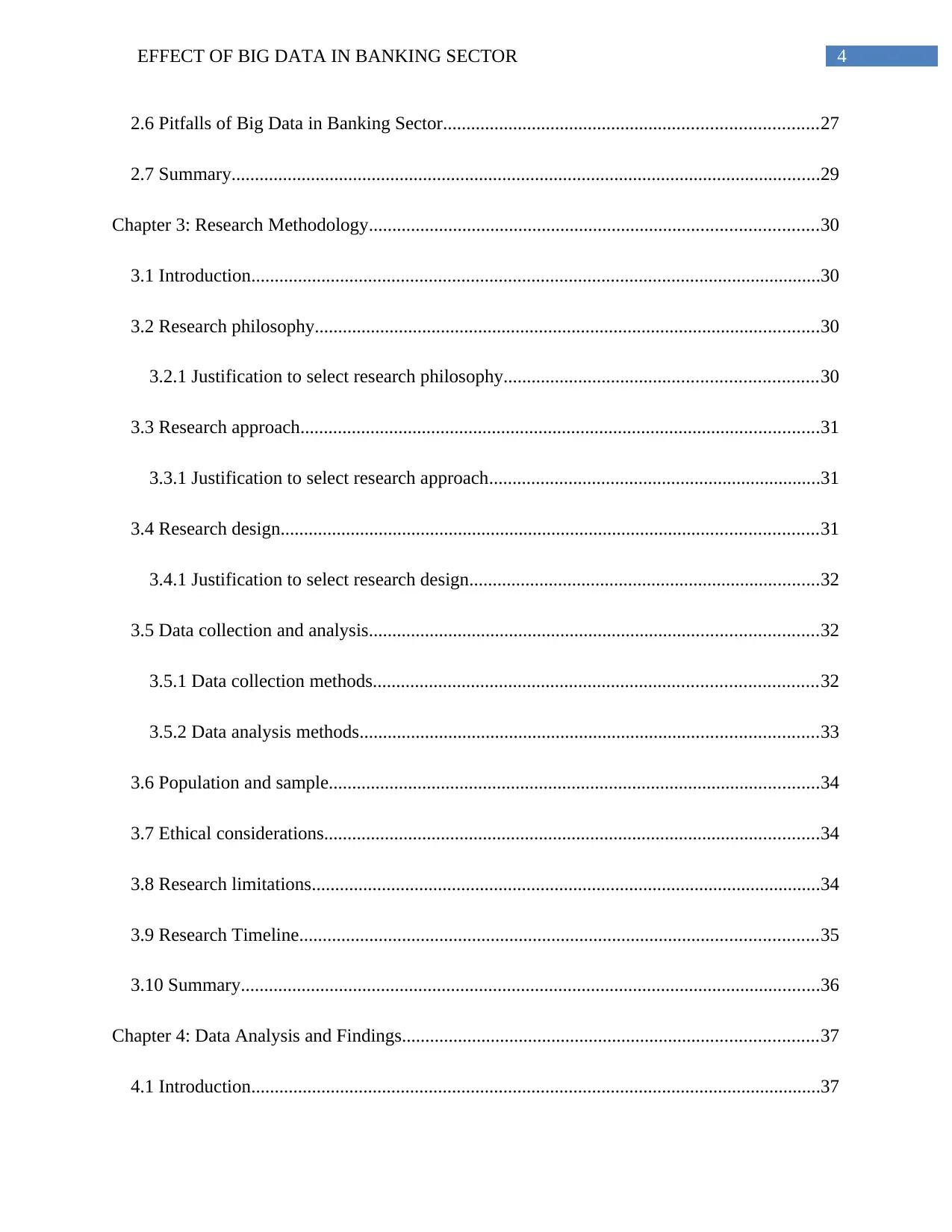
4EFFECT OF BIG DATA IN BANKING SECTOR
2.6 Pitfalls of Big Data in Banking Sector................................................................................27
2.7 Summary..............................................................................................................................29
Chapter 3: Research Methodology................................................................................................30
3.1 Introduction..........................................................................................................................30
3.2 Research philosophy............................................................................................................30
3.2.1 Justification to select research philosophy...................................................................30
3.3 Research approach...............................................................................................................31
3.3.1 Justification to select research approach.......................................................................31
3.4 Research design...................................................................................................................31
3.4.1 Justification to select research design...........................................................................32
3.5 Data collection and analysis................................................................................................32
3.5.1 Data collection methods...............................................................................................32
3.5.2 Data analysis methods..................................................................................................33
3.6 Population and sample.........................................................................................................34
3.7 Ethical considerations..........................................................................................................34
3.8 Research limitations.............................................................................................................34
3.9 Research Timeline...............................................................................................................35
3.10 Summary............................................................................................................................36
Chapter 4: Data Analysis and Findings.........................................................................................37
4.1 Introduction..........................................................................................................................37
2.6 Pitfalls of Big Data in Banking Sector................................................................................27
2.7 Summary..............................................................................................................................29
Chapter 3: Research Methodology................................................................................................30
3.1 Introduction..........................................................................................................................30
3.2 Research philosophy............................................................................................................30
3.2.1 Justification to select research philosophy...................................................................30
3.3 Research approach...............................................................................................................31
3.3.1 Justification to select research approach.......................................................................31
3.4 Research design...................................................................................................................31
3.4.1 Justification to select research design...........................................................................32
3.5 Data collection and analysis................................................................................................32
3.5.1 Data collection methods...............................................................................................32
3.5.2 Data analysis methods..................................................................................................33
3.6 Population and sample.........................................................................................................34
3.7 Ethical considerations..........................................................................................................34
3.8 Research limitations.............................................................................................................34
3.9 Research Timeline...............................................................................................................35
3.10 Summary............................................................................................................................36
Chapter 4: Data Analysis and Findings.........................................................................................37
4.1 Introduction..........................................................................................................................37
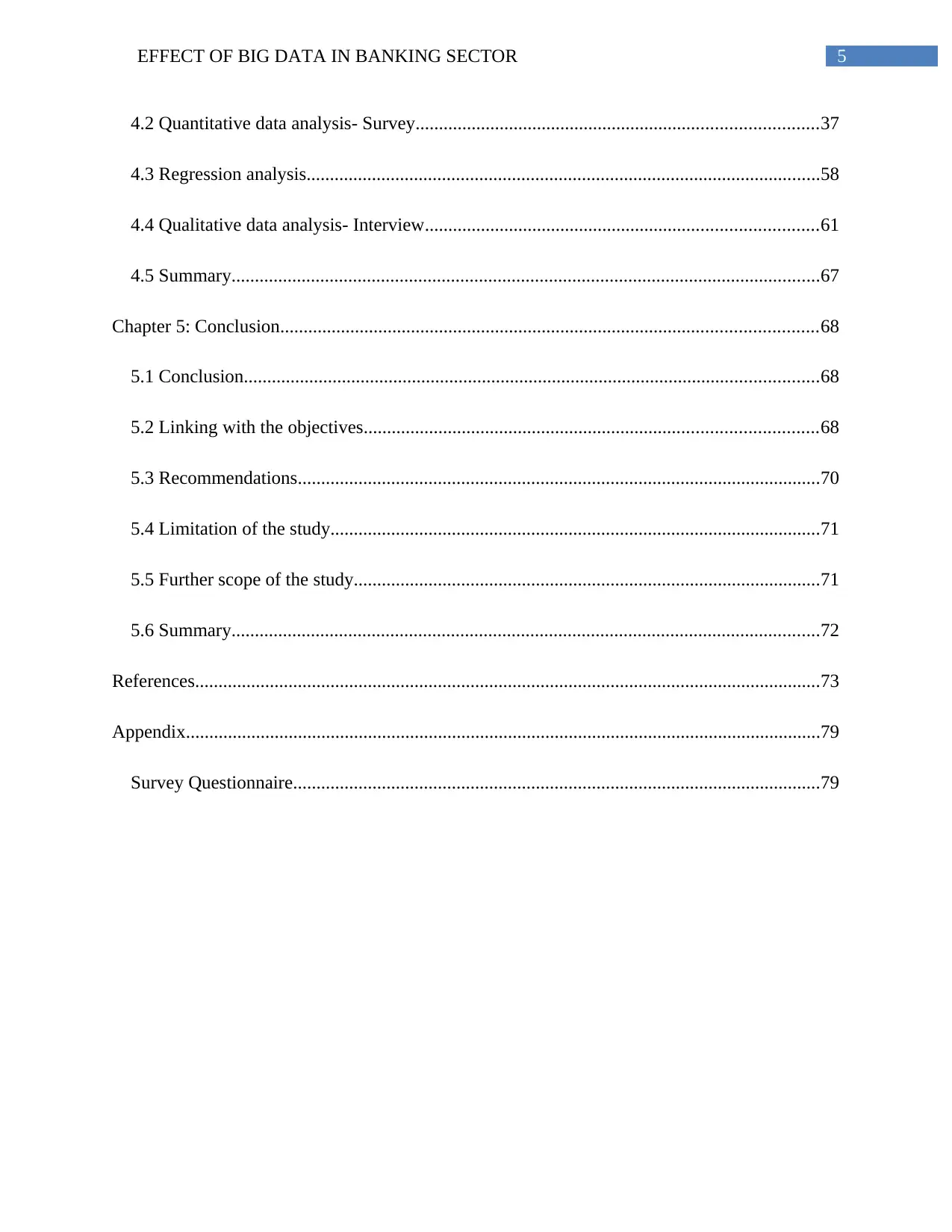
5EFFECT OF BIG DATA IN BANKING SECTOR
4.2 Quantitative data analysis- Survey......................................................................................37
4.3 Regression analysis..............................................................................................................58
4.4 Qualitative data analysis- Interview....................................................................................61
4.5 Summary..............................................................................................................................67
Chapter 5: Conclusion...................................................................................................................68
5.1 Conclusion...........................................................................................................................68
5.2 Linking with the objectives.................................................................................................68
5.3 Recommendations................................................................................................................70
5.4 Limitation of the study.........................................................................................................71
5.5 Further scope of the study....................................................................................................71
5.6 Summary..............................................................................................................................72
References......................................................................................................................................73
Appendix........................................................................................................................................79
Survey Questionnaire.................................................................................................................79
4.2 Quantitative data analysis- Survey......................................................................................37
4.3 Regression analysis..............................................................................................................58
4.4 Qualitative data analysis- Interview....................................................................................61
4.5 Summary..............................................................................................................................67
Chapter 5: Conclusion...................................................................................................................68
5.1 Conclusion...........................................................................................................................68
5.2 Linking with the objectives.................................................................................................68
5.3 Recommendations................................................................................................................70
5.4 Limitation of the study.........................................................................................................71
5.5 Further scope of the study....................................................................................................71
5.6 Summary..............................................................................................................................72
References......................................................................................................................................73
Appendix........................................................................................................................................79
Survey Questionnaire.................................................................................................................79
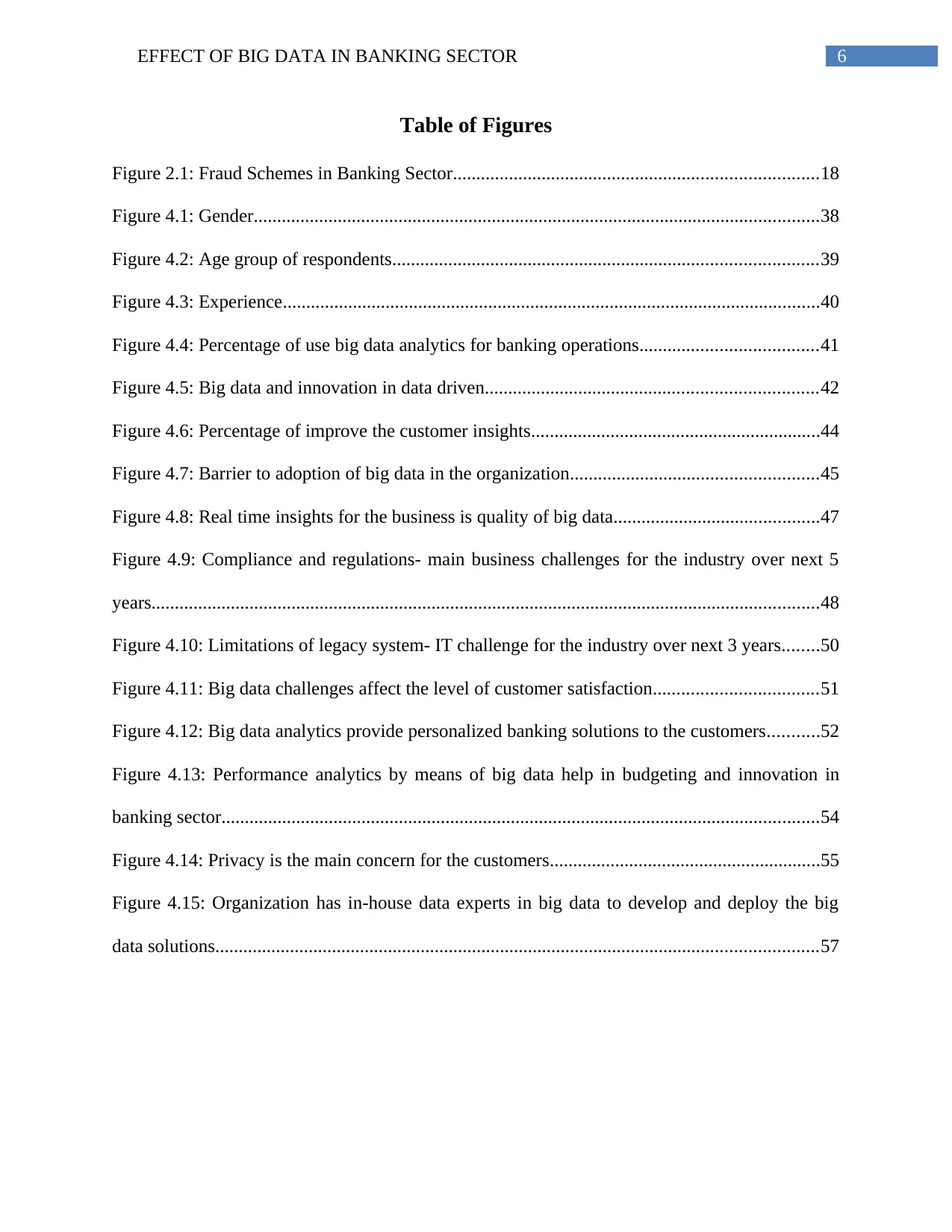
6EFFECT OF BIG DATA IN BANKING SECTOR
Table of Figures
Figure 2.1: Fraud Schemes in Banking Sector..............................................................................18
Figure 4.1: Gender.........................................................................................................................38
Figure 4.2: Age group of respondents...........................................................................................39
Figure 4.3: Experience...................................................................................................................40
Figure 4.4: Percentage of use big data analytics for banking operations......................................41
Figure 4.5: Big data and innovation in data driven.......................................................................42
Figure 4.6: Percentage of improve the customer insights..............................................................44
Figure 4.7: Barrier to adoption of big data in the organization.....................................................45
Figure 4.8: Real time insights for the business is quality of big data............................................47
Figure 4.9: Compliance and regulations- main business challenges for the industry over next 5
years...............................................................................................................................................48
Figure 4.10: Limitations of legacy system- IT challenge for the industry over next 3 years........50
Figure 4.11: Big data challenges affect the level of customer satisfaction...................................51
Figure 4.12: Big data analytics provide personalized banking solutions to the customers...........52
Figure 4.13: Performance analytics by means of big data help in budgeting and innovation in
banking sector................................................................................................................................54
Figure 4.14: Privacy is the main concern for the customers..........................................................55
Figure 4.15: Organization has in-house data experts in big data to develop and deploy the big
data solutions.................................................................................................................................57
Table of Figures
Figure 2.1: Fraud Schemes in Banking Sector..............................................................................18
Figure 4.1: Gender.........................................................................................................................38
Figure 4.2: Age group of respondents...........................................................................................39
Figure 4.3: Experience...................................................................................................................40
Figure 4.4: Percentage of use big data analytics for banking operations......................................41
Figure 4.5: Big data and innovation in data driven.......................................................................42
Figure 4.6: Percentage of improve the customer insights..............................................................44
Figure 4.7: Barrier to adoption of big data in the organization.....................................................45
Figure 4.8: Real time insights for the business is quality of big data............................................47
Figure 4.9: Compliance and regulations- main business challenges for the industry over next 5
years...............................................................................................................................................48
Figure 4.10: Limitations of legacy system- IT challenge for the industry over next 3 years........50
Figure 4.11: Big data challenges affect the level of customer satisfaction...................................51
Figure 4.12: Big data analytics provide personalized banking solutions to the customers...........52
Figure 4.13: Performance analytics by means of big data help in budgeting and innovation in
banking sector................................................................................................................................54
Figure 4.14: Privacy is the main concern for the customers..........................................................55
Figure 4.15: Organization has in-house data experts in big data to develop and deploy the big
data solutions.................................................................................................................................57
Secure Best Marks with AI Grader
Need help grading? Try our AI Grader for instant feedback on your assignments.
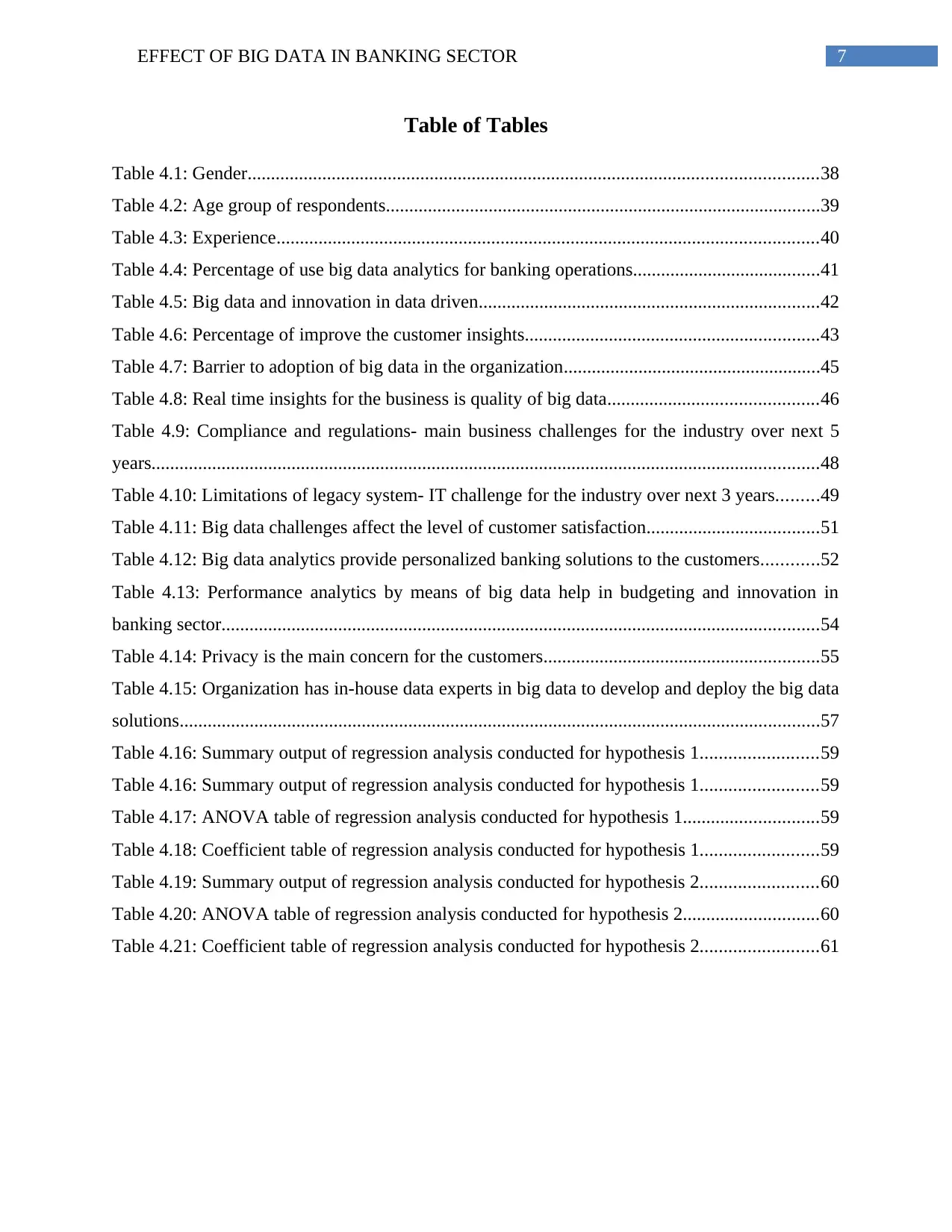
7EFFECT OF BIG DATA IN BANKING SECTOR
Table of Tables
Table 4.1: Gender..........................................................................................................................38
Table 4.2: Age group of respondents.............................................................................................39
Table 4.3: Experience....................................................................................................................40
Table 4.4: Percentage of use big data analytics for banking operations........................................41
Table 4.5: Big data and innovation in data driven.........................................................................42
Table 4.6: Percentage of improve the customer insights...............................................................43
Table 4.7: Barrier to adoption of big data in the organization.......................................................45
Table 4.8: Real time insights for the business is quality of big data.............................................46
Table 4.9: Compliance and regulations- main business challenges for the industry over next 5
years...............................................................................................................................................48
Table 4.10: Limitations of legacy system- IT challenge for the industry over next 3 years.........49
Table 4.11: Big data challenges affect the level of customer satisfaction.....................................51
Table 4.12: Big data analytics provide personalized banking solutions to the customers............52
Table 4.13: Performance analytics by means of big data help in budgeting and innovation in
banking sector................................................................................................................................54
Table 4.14: Privacy is the main concern for the customers...........................................................55
Table 4.15: Organization has in-house data experts in big data to develop and deploy the big data
solutions.........................................................................................................................................57
Table 4.16: Summary output of regression analysis conducted for hypothesis 1.........................59
Table 4.16: Summary output of regression analysis conducted for hypothesis 1.........................59
Table 4.17: ANOVA table of regression analysis conducted for hypothesis 1.............................59
Table 4.18: Coefficient table of regression analysis conducted for hypothesis 1.........................59
Table 4.19: Summary output of regression analysis conducted for hypothesis 2.........................60
Table 4.20: ANOVA table of regression analysis conducted for hypothesis 2.............................60
Table 4.21: Coefficient table of regression analysis conducted for hypothesis 2.........................61
Table of Tables
Table 4.1: Gender..........................................................................................................................38
Table 4.2: Age group of respondents.............................................................................................39
Table 4.3: Experience....................................................................................................................40
Table 4.4: Percentage of use big data analytics for banking operations........................................41
Table 4.5: Big data and innovation in data driven.........................................................................42
Table 4.6: Percentage of improve the customer insights...............................................................43
Table 4.7: Barrier to adoption of big data in the organization.......................................................45
Table 4.8: Real time insights for the business is quality of big data.............................................46
Table 4.9: Compliance and regulations- main business challenges for the industry over next 5
years...............................................................................................................................................48
Table 4.10: Limitations of legacy system- IT challenge for the industry over next 3 years.........49
Table 4.11: Big data challenges affect the level of customer satisfaction.....................................51
Table 4.12: Big data analytics provide personalized banking solutions to the customers............52
Table 4.13: Performance analytics by means of big data help in budgeting and innovation in
banking sector................................................................................................................................54
Table 4.14: Privacy is the main concern for the customers...........................................................55
Table 4.15: Organization has in-house data experts in big data to develop and deploy the big data
solutions.........................................................................................................................................57
Table 4.16: Summary output of regression analysis conducted for hypothesis 1.........................59
Table 4.16: Summary output of regression analysis conducted for hypothesis 1.........................59
Table 4.17: ANOVA table of regression analysis conducted for hypothesis 1.............................59
Table 4.18: Coefficient table of regression analysis conducted for hypothesis 1.........................59
Table 4.19: Summary output of regression analysis conducted for hypothesis 2.........................60
Table 4.20: ANOVA table of regression analysis conducted for hypothesis 2.............................60
Table 4.21: Coefficient table of regression analysis conducted for hypothesis 2.........................61
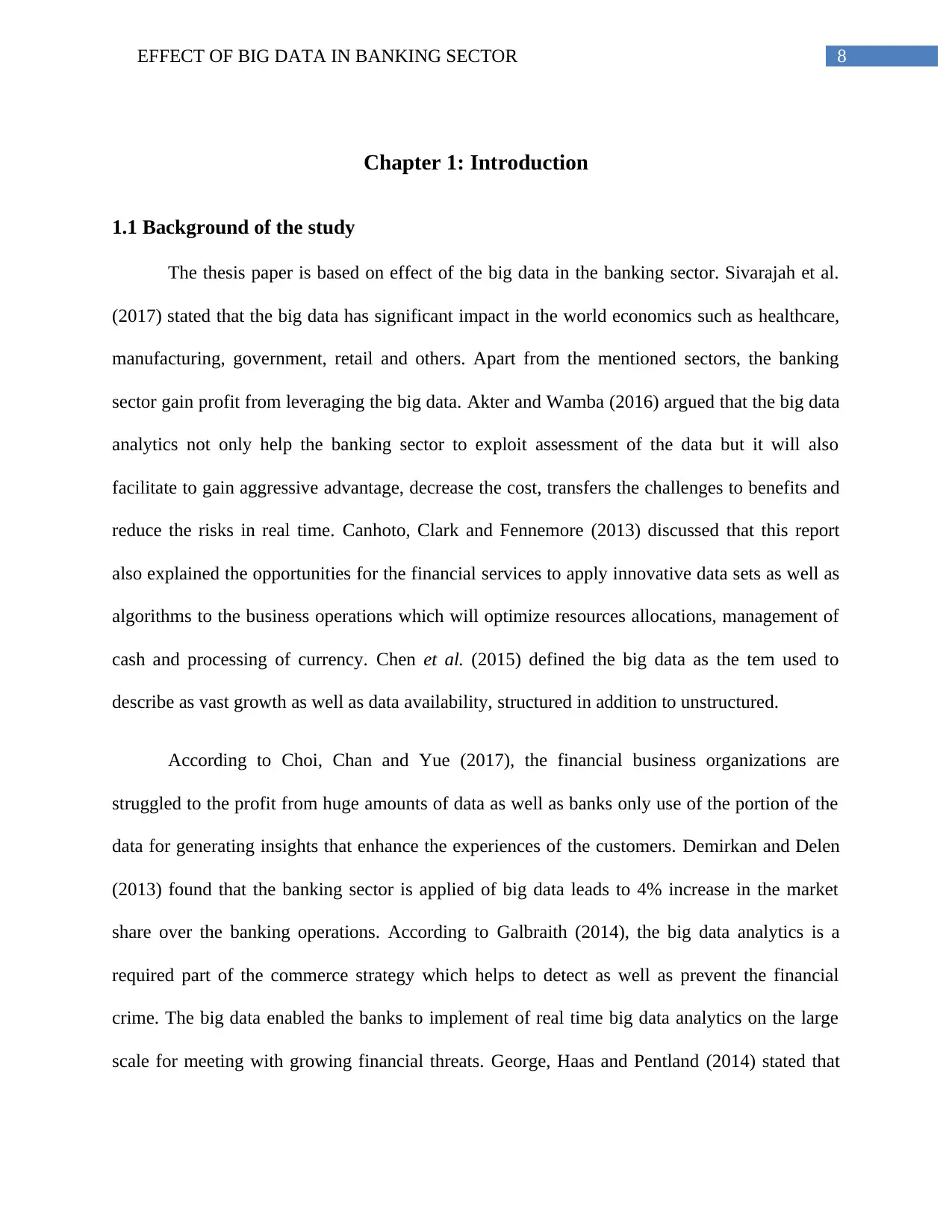
8EFFECT OF BIG DATA IN BANKING SECTOR
Chapter 1: Introduction
1.1 Background of the study
The thesis paper is based on effect of the big data in the banking sector. Sivarajah et al.
(2017) stated that the big data has significant impact in the world economics such as healthcare,
manufacturing, government, retail and others. Apart from the mentioned sectors, the banking
sector gain profit from leveraging the big data. Akter and Wamba (2016) argued that the big data
analytics not only help the banking sector to exploit assessment of the data but it will also
facilitate to gain aggressive advantage, decrease the cost, transfers the challenges to benefits and
reduce the risks in real time. Canhoto, Clark and Fennemore (2013) discussed that this report
also explained the opportunities for the financial services to apply innovative data sets as well as
algorithms to the business operations which will optimize resources allocations, management of
cash and processing of currency. Chen et al. (2015) defined the big data as the tem used to
describe as vast growth as well as data availability, structured in addition to unstructured.
According to Choi, Chan and Yue (2017), the financial business organizations are
struggled to the profit from huge amounts of data as well as banks only use of the portion of the
data for generating insights that enhance the experiences of the customers. Demirkan and Delen
(2013) found that the banking sector is applied of big data leads to 4% increase in the market
share over the banking operations. According to Galbraith (2014), the big data analytics is a
required part of the commerce strategy which helps to detect as well as prevent the financial
crime. The big data enabled the banks to implement of real time big data analytics on the large
scale for meeting with growing financial threats. George, Haas and Pentland (2014) stated that
Chapter 1: Introduction
1.1 Background of the study
The thesis paper is based on effect of the big data in the banking sector. Sivarajah et al.
(2017) stated that the big data has significant impact in the world economics such as healthcare,
manufacturing, government, retail and others. Apart from the mentioned sectors, the banking
sector gain profit from leveraging the big data. Akter and Wamba (2016) argued that the big data
analytics not only help the banking sector to exploit assessment of the data but it will also
facilitate to gain aggressive advantage, decrease the cost, transfers the challenges to benefits and
reduce the risks in real time. Canhoto, Clark and Fennemore (2013) discussed that this report
also explained the opportunities for the financial services to apply innovative data sets as well as
algorithms to the business operations which will optimize resources allocations, management of
cash and processing of currency. Chen et al. (2015) defined the big data as the tem used to
describe as vast growth as well as data availability, structured in addition to unstructured.
According to Choi, Chan and Yue (2017), the financial business organizations are
struggled to the profit from huge amounts of data as well as banks only use of the portion of the
data for generating insights that enhance the experiences of the customers. Demirkan and Delen
(2013) found that the banking sector is applied of big data leads to 4% increase in the market
share over the banking operations. According to Galbraith (2014), the big data analytics is a
required part of the commerce strategy which helps to detect as well as prevent the financial
crime. The big data enabled the banks to implement of real time big data analytics on the large
scale for meeting with growing financial threats. George, Haas and Pentland (2014) stated that
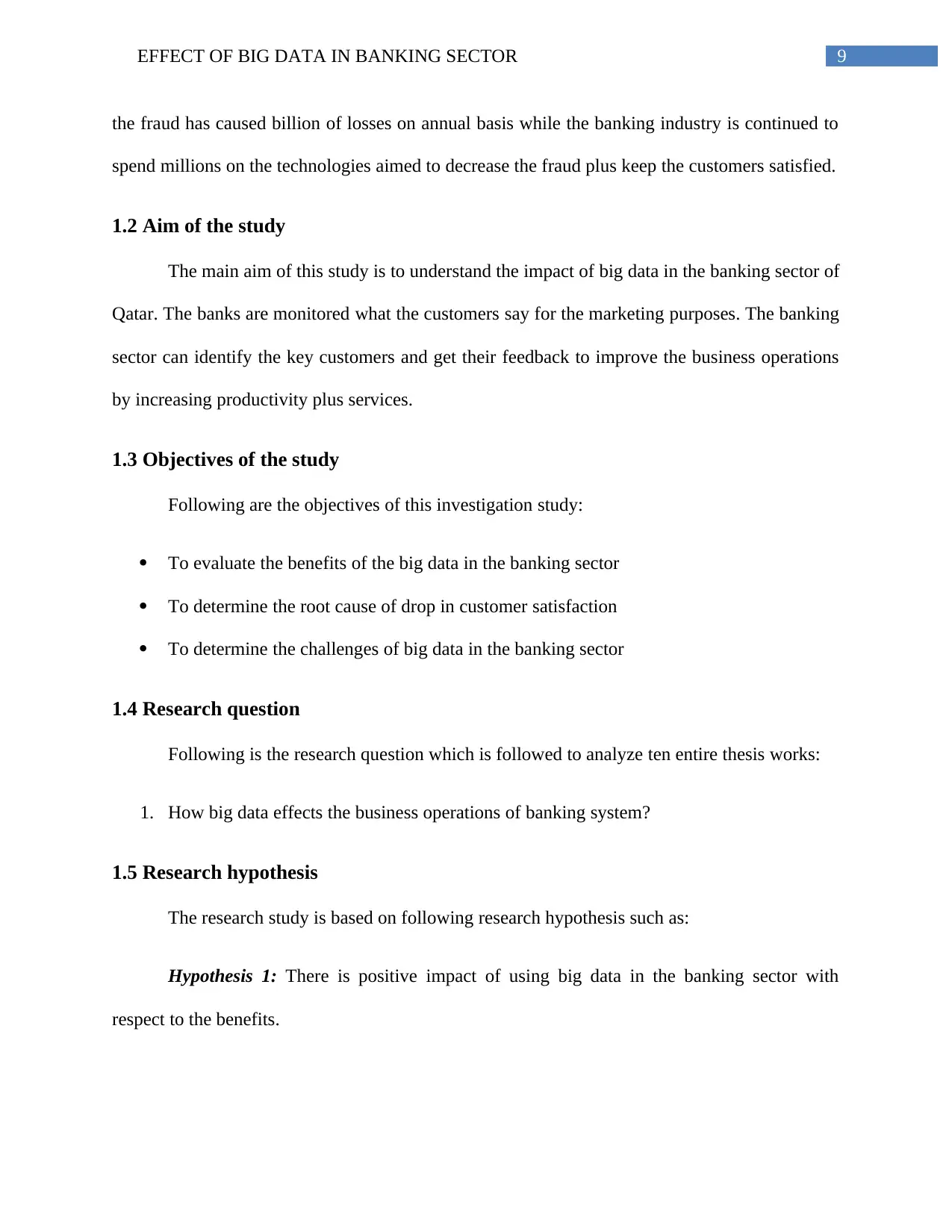
9EFFECT OF BIG DATA IN BANKING SECTOR
the fraud has caused billion of losses on annual basis while the banking industry is continued to
spend millions on the technologies aimed to decrease the fraud plus keep the customers satisfied.
1.2 Aim of the study
The main aim of this study is to understand the impact of big data in the banking sector of
Qatar. The banks are monitored what the customers say for the marketing purposes. The banking
sector can identify the key customers and get their feedback to improve the business operations
by increasing productivity plus services.
1.3 Objectives of the study
Following are the objectives of this investigation study:
To evaluate the benefits of the big data in the banking sector
To determine the root cause of drop in customer satisfaction
To determine the challenges of big data in the banking sector
1.4 Research question
Following is the research question which is followed to analyze ten entire thesis works:
1. How big data effects the business operations of banking system?
1.5 Research hypothesis
The research study is based on following research hypothesis such as:
Hypothesis 1: There is positive impact of using big data in the banking sector with
respect to the benefits.
the fraud has caused billion of losses on annual basis while the banking industry is continued to
spend millions on the technologies aimed to decrease the fraud plus keep the customers satisfied.
1.2 Aim of the study
The main aim of this study is to understand the impact of big data in the banking sector of
Qatar. The banks are monitored what the customers say for the marketing purposes. The banking
sector can identify the key customers and get their feedback to improve the business operations
by increasing productivity plus services.
1.3 Objectives of the study
Following are the objectives of this investigation study:
To evaluate the benefits of the big data in the banking sector
To determine the root cause of drop in customer satisfaction
To determine the challenges of big data in the banking sector
1.4 Research question
Following is the research question which is followed to analyze ten entire thesis works:
1. How big data effects the business operations of banking system?
1.5 Research hypothesis
The research study is based on following research hypothesis such as:
Hypothesis 1: There is positive impact of using big data in the banking sector with
respect to the benefits.
Paraphrase This Document
Need a fresh take? Get an instant paraphrase of this document with our AI Paraphraser
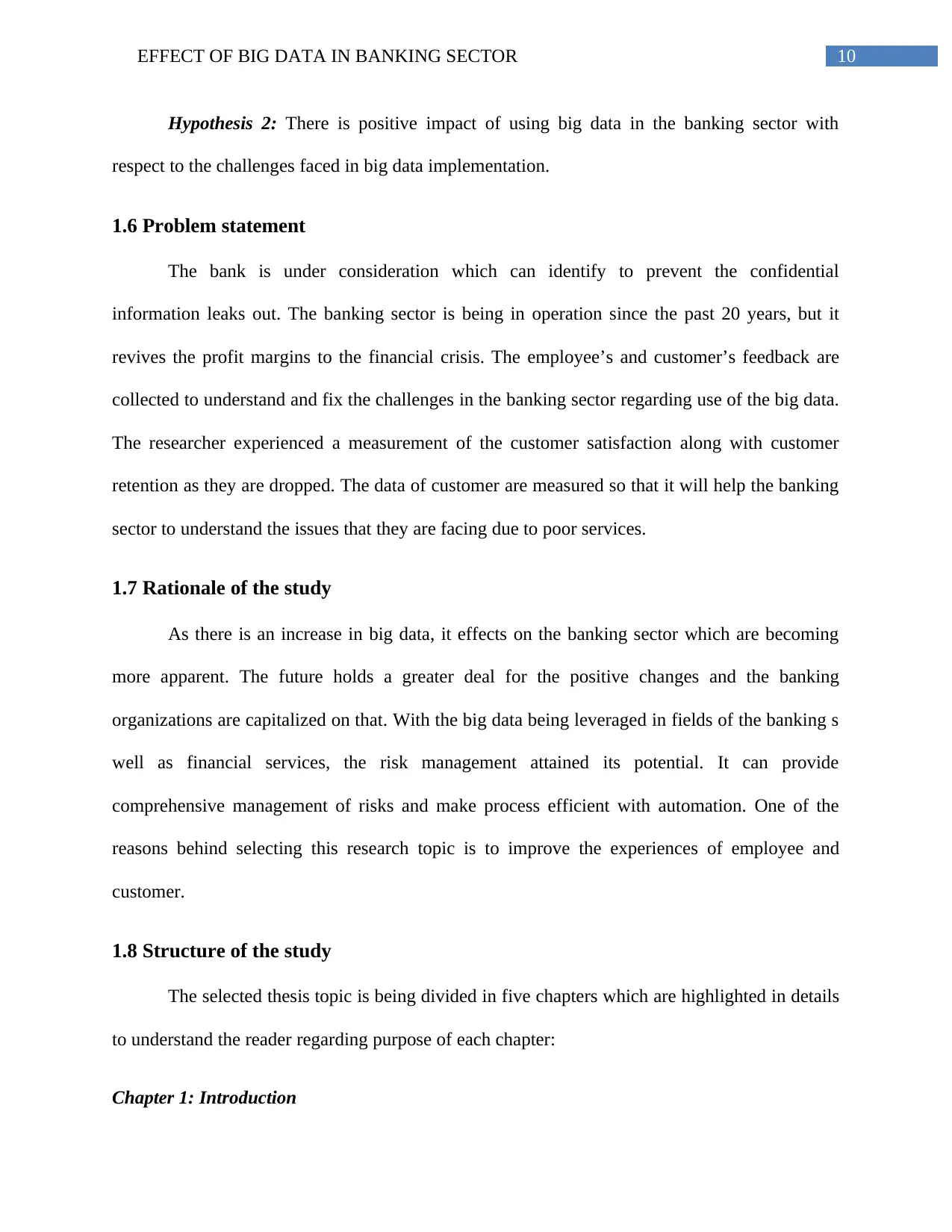
10EFFECT OF BIG DATA IN BANKING SECTOR
Hypothesis 2: There is positive impact of using big data in the banking sector with
respect to the challenges faced in big data implementation.
1.6 Problem statement
The bank is under consideration which can identify to prevent the confidential
information leaks out. The banking sector is being in operation since the past 20 years, but it
revives the profit margins to the financial crisis. The employee’s and customer’s feedback are
collected to understand and fix the challenges in the banking sector regarding use of the big data.
The researcher experienced a measurement of the customer satisfaction along with customer
retention as they are dropped. The data of customer are measured so that it will help the banking
sector to understand the issues that they are facing due to poor services.
1.7 Rationale of the study
As there is an increase in big data, it effects on the banking sector which are becoming
more apparent. The future holds a greater deal for the positive changes and the banking
organizations are capitalized on that. With the big data being leveraged in fields of the banking s
well as financial services, the risk management attained its potential. It can provide
comprehensive management of risks and make process efficient with automation. One of the
reasons behind selecting this research topic is to improve the experiences of employee and
customer.
1.8 Structure of the study
The selected thesis topic is being divided in five chapters which are highlighted in details
to understand the reader regarding purpose of each chapter:
Chapter 1: Introduction
Hypothesis 2: There is positive impact of using big data in the banking sector with
respect to the challenges faced in big data implementation.
1.6 Problem statement
The bank is under consideration which can identify to prevent the confidential
information leaks out. The banking sector is being in operation since the past 20 years, but it
revives the profit margins to the financial crisis. The employee’s and customer’s feedback are
collected to understand and fix the challenges in the banking sector regarding use of the big data.
The researcher experienced a measurement of the customer satisfaction along with customer
retention as they are dropped. The data of customer are measured so that it will help the banking
sector to understand the issues that they are facing due to poor services.
1.7 Rationale of the study
As there is an increase in big data, it effects on the banking sector which are becoming
more apparent. The future holds a greater deal for the positive changes and the banking
organizations are capitalized on that. With the big data being leveraged in fields of the banking s
well as financial services, the risk management attained its potential. It can provide
comprehensive management of risks and make process efficient with automation. One of the
reasons behind selecting this research topic is to improve the experiences of employee and
customer.
1.8 Structure of the study
The selected thesis topic is being divided in five chapters which are highlighted in details
to understand the reader regarding purpose of each chapter:
Chapter 1: Introduction
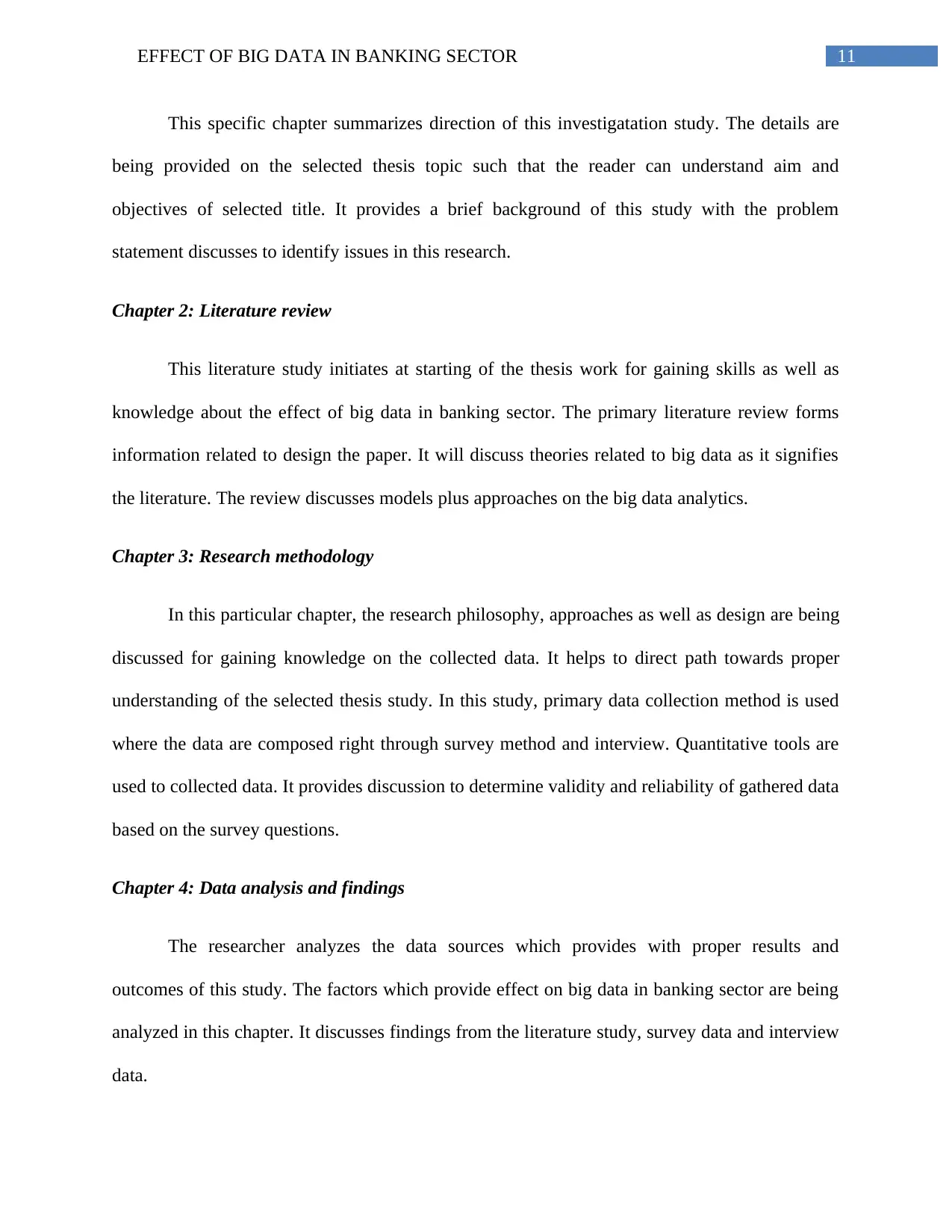
11EFFECT OF BIG DATA IN BANKING SECTOR
This specific chapter summarizes direction of this investigatation study. The details are
being provided on the selected thesis topic such that the reader can understand aim and
objectives of selected title. It provides a brief background of this study with the problem
statement discusses to identify issues in this research.
Chapter 2: Literature review
This literature study initiates at starting of the thesis work for gaining skills as well as
knowledge about the effect of big data in banking sector. The primary literature review forms
information related to design the paper. It will discuss theories related to big data as it signifies
the literature. The review discusses models plus approaches on the big data analytics.
Chapter 3: Research methodology
In this particular chapter, the research philosophy, approaches as well as design are being
discussed for gaining knowledge on the collected data. It helps to direct path towards proper
understanding of the selected thesis study. In this study, primary data collection method is used
where the data are composed right through survey method and interview. Quantitative tools are
used to collected data. It provides discussion to determine validity and reliability of gathered data
based on the survey questions.
Chapter 4: Data analysis and findings
The researcher analyzes the data sources which provides with proper results and
outcomes of this study. The factors which provide effect on big data in banking sector are being
analyzed in this chapter. It discusses findings from the literature study, survey data and interview
data.
This specific chapter summarizes direction of this investigatation study. The details are
being provided on the selected thesis topic such that the reader can understand aim and
objectives of selected title. It provides a brief background of this study with the problem
statement discusses to identify issues in this research.
Chapter 2: Literature review
This literature study initiates at starting of the thesis work for gaining skills as well as
knowledge about the effect of big data in banking sector. The primary literature review forms
information related to design the paper. It will discuss theories related to big data as it signifies
the literature. The review discusses models plus approaches on the big data analytics.
Chapter 3: Research methodology
In this particular chapter, the research philosophy, approaches as well as design are being
discussed for gaining knowledge on the collected data. It helps to direct path towards proper
understanding of the selected thesis study. In this study, primary data collection method is used
where the data are composed right through survey method and interview. Quantitative tools are
used to collected data. It provides discussion to determine validity and reliability of gathered data
based on the survey questions.
Chapter 4: Data analysis and findings
The researcher analyzes the data sources which provides with proper results and
outcomes of this study. The factors which provide effect on big data in banking sector are being
analyzed in this chapter. It discusses findings from the literature study, survey data and interview
data.
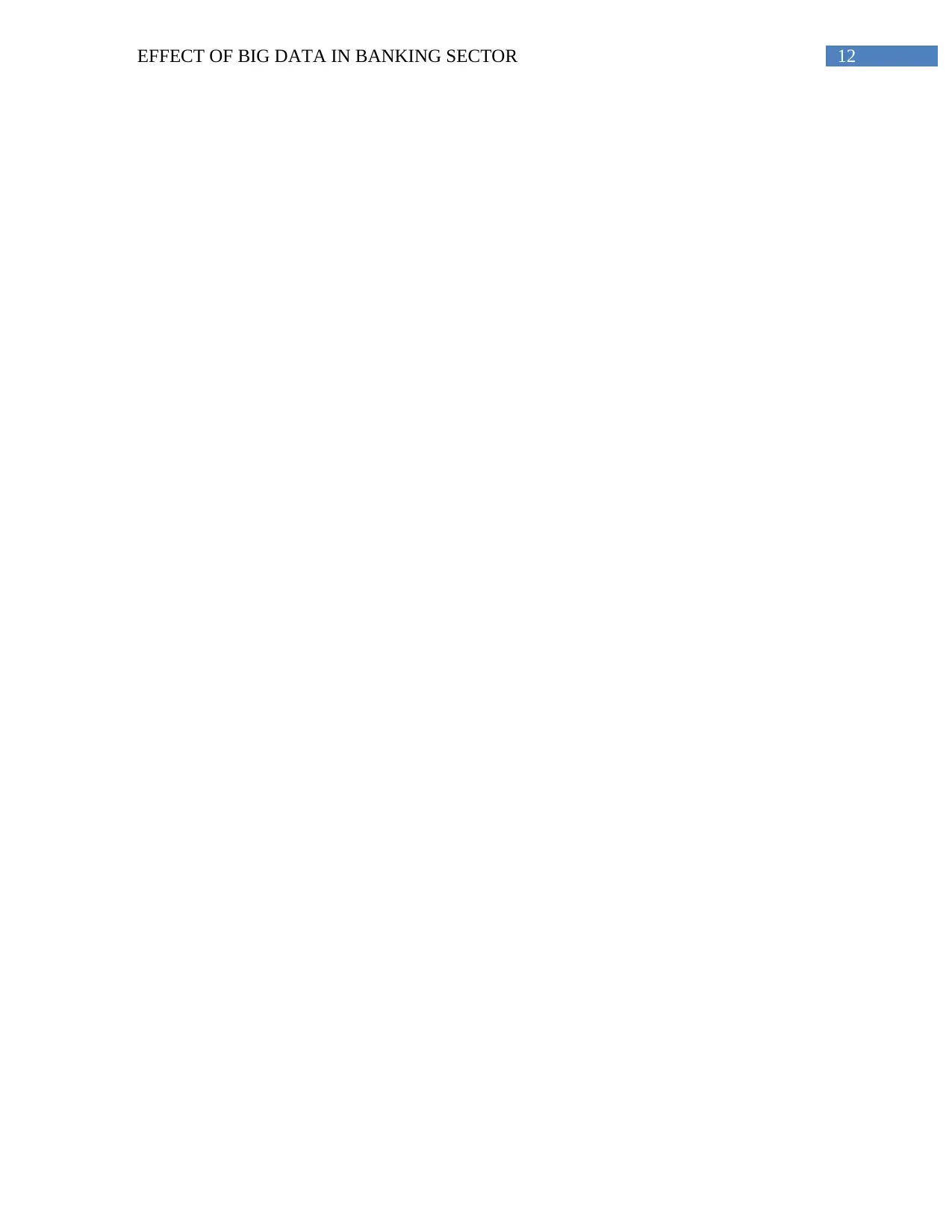
12EFFECT OF BIG DATA IN BANKING SECTOR
Secure Best Marks with AI Grader
Need help grading? Try our AI Grader for instant feedback on your assignments.
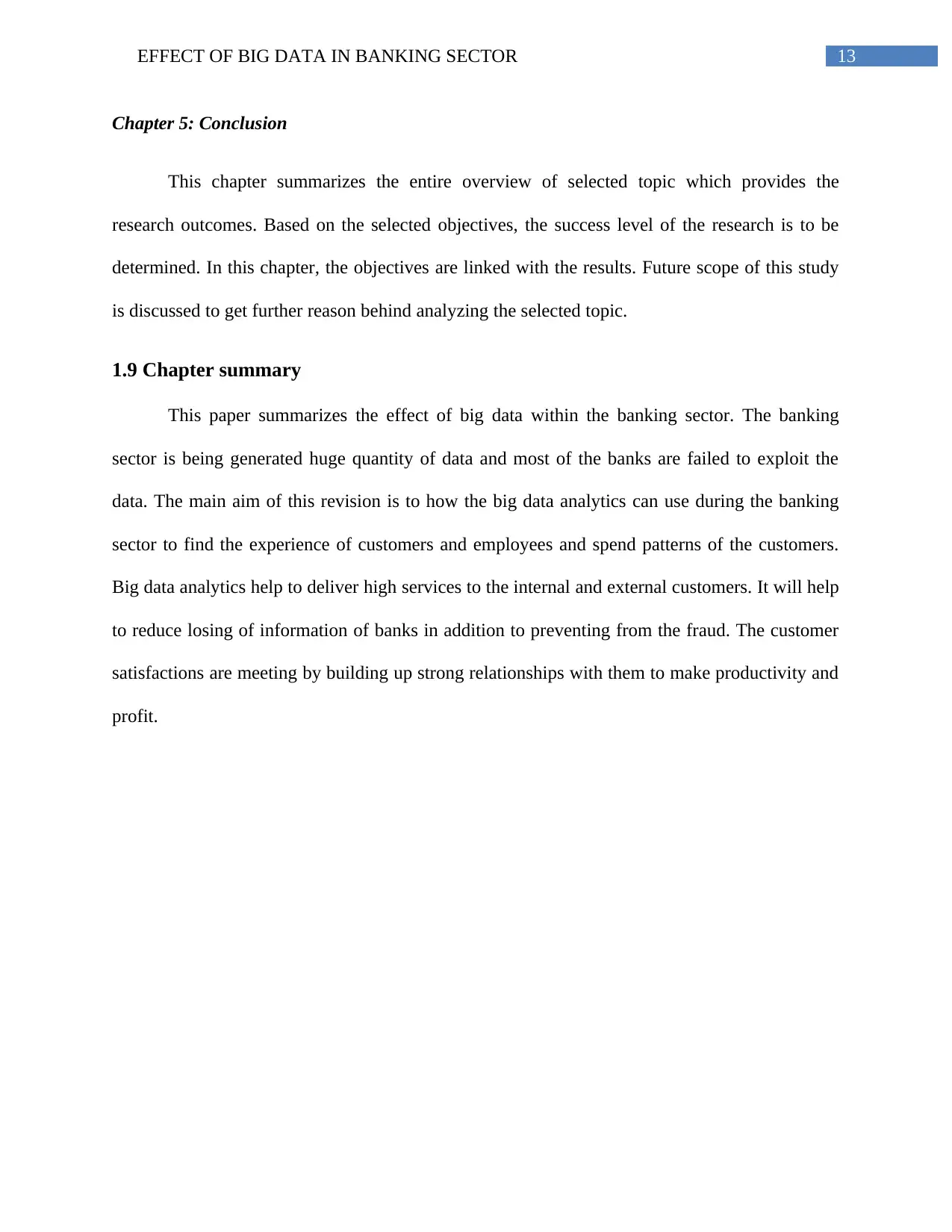
13EFFECT OF BIG DATA IN BANKING SECTOR
Chapter 5: Conclusion
This chapter summarizes the entire overview of selected topic which provides the
research outcomes. Based on the selected objectives, the success level of the research is to be
determined. In this chapter, the objectives are linked with the results. Future scope of this study
is discussed to get further reason behind analyzing the selected topic.
1.9 Chapter summary
This paper summarizes the effect of big data within the banking sector. The banking
sector is being generated huge quantity of data and most of the banks are failed to exploit the
data. The main aim of this revision is to how the big data analytics can use during the banking
sector to find the experience of customers and employees and spend patterns of the customers.
Big data analytics help to deliver high services to the internal and external customers. It will help
to reduce losing of information of banks in addition to preventing from the fraud. The customer
satisfactions are meeting by building up strong relationships with them to make productivity and
profit.
Chapter 5: Conclusion
This chapter summarizes the entire overview of selected topic which provides the
research outcomes. Based on the selected objectives, the success level of the research is to be
determined. In this chapter, the objectives are linked with the results. Future scope of this study
is discussed to get further reason behind analyzing the selected topic.
1.9 Chapter summary
This paper summarizes the effect of big data within the banking sector. The banking
sector is being generated huge quantity of data and most of the banks are failed to exploit the
data. The main aim of this revision is to how the big data analytics can use during the banking
sector to find the experience of customers and employees and spend patterns of the customers.
Big data analytics help to deliver high services to the internal and external customers. It will help
to reduce losing of information of banks in addition to preventing from the fraud. The customer
satisfactions are meeting by building up strong relationships with them to make productivity and
profit.
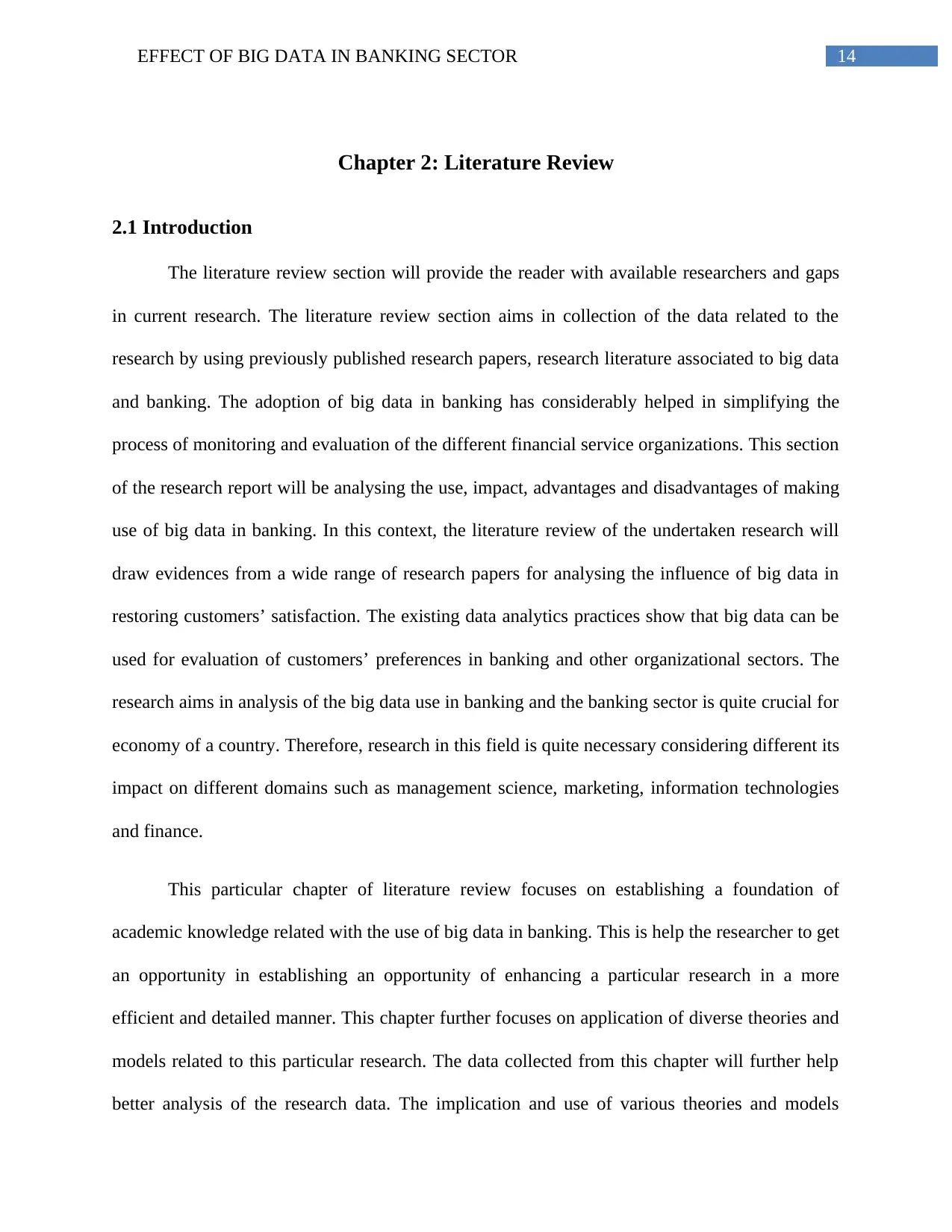
14EFFECT OF BIG DATA IN BANKING SECTOR
Chapter 2: Literature Review
2.1 Introduction
The literature review section will provide the reader with available researchers and gaps
in current research. The literature review section aims in collection of the data related to the
research by using previously published research papers, research literature associated to big data
and banking. The adoption of big data in banking has considerably helped in simplifying the
process of monitoring and evaluation of the different financial service organizations. This section
of the research report will be analysing the use, impact, advantages and disadvantages of making
use of big data in banking. In this context, the literature review of the undertaken research will
draw evidences from a wide range of research papers for analysing the influence of big data in
restoring customers’ satisfaction. The existing data analytics practices show that big data can be
used for evaluation of customers’ preferences in banking and other organizational sectors. The
research aims in analysis of the big data use in banking and the banking sector is quite crucial for
economy of a country. Therefore, research in this field is quite necessary considering different its
impact on different domains such as management science, marketing, information technologies
and finance.
This particular chapter of literature review focuses on establishing a foundation of
academic knowledge related with the use of big data in banking. This is help the researcher to get
an opportunity in establishing an opportunity of enhancing a particular research in a more
efficient and detailed manner. This chapter further focuses on application of diverse theories and
models related to this particular research. The data collected from this chapter will further help
better analysis of the research data. The implication and use of various theories and models
Chapter 2: Literature Review
2.1 Introduction
The literature review section will provide the reader with available researchers and gaps
in current research. The literature review section aims in collection of the data related to the
research by using previously published research papers, research literature associated to big data
and banking. The adoption of big data in banking has considerably helped in simplifying the
process of monitoring and evaluation of the different financial service organizations. This section
of the research report will be analysing the use, impact, advantages and disadvantages of making
use of big data in banking. In this context, the literature review of the undertaken research will
draw evidences from a wide range of research papers for analysing the influence of big data in
restoring customers’ satisfaction. The existing data analytics practices show that big data can be
used for evaluation of customers’ preferences in banking and other organizational sectors. The
research aims in analysis of the big data use in banking and the banking sector is quite crucial for
economy of a country. Therefore, research in this field is quite necessary considering different its
impact on different domains such as management science, marketing, information technologies
and finance.
This particular chapter of literature review focuses on establishing a foundation of
academic knowledge related with the use of big data in banking. This is help the researcher to get
an opportunity in establishing an opportunity of enhancing a particular research in a more
efficient and detailed manner. This chapter further focuses on application of diverse theories and
models related to this particular research. The data collected from this chapter will further help
better analysis of the research data. The implication and use of various theories and models
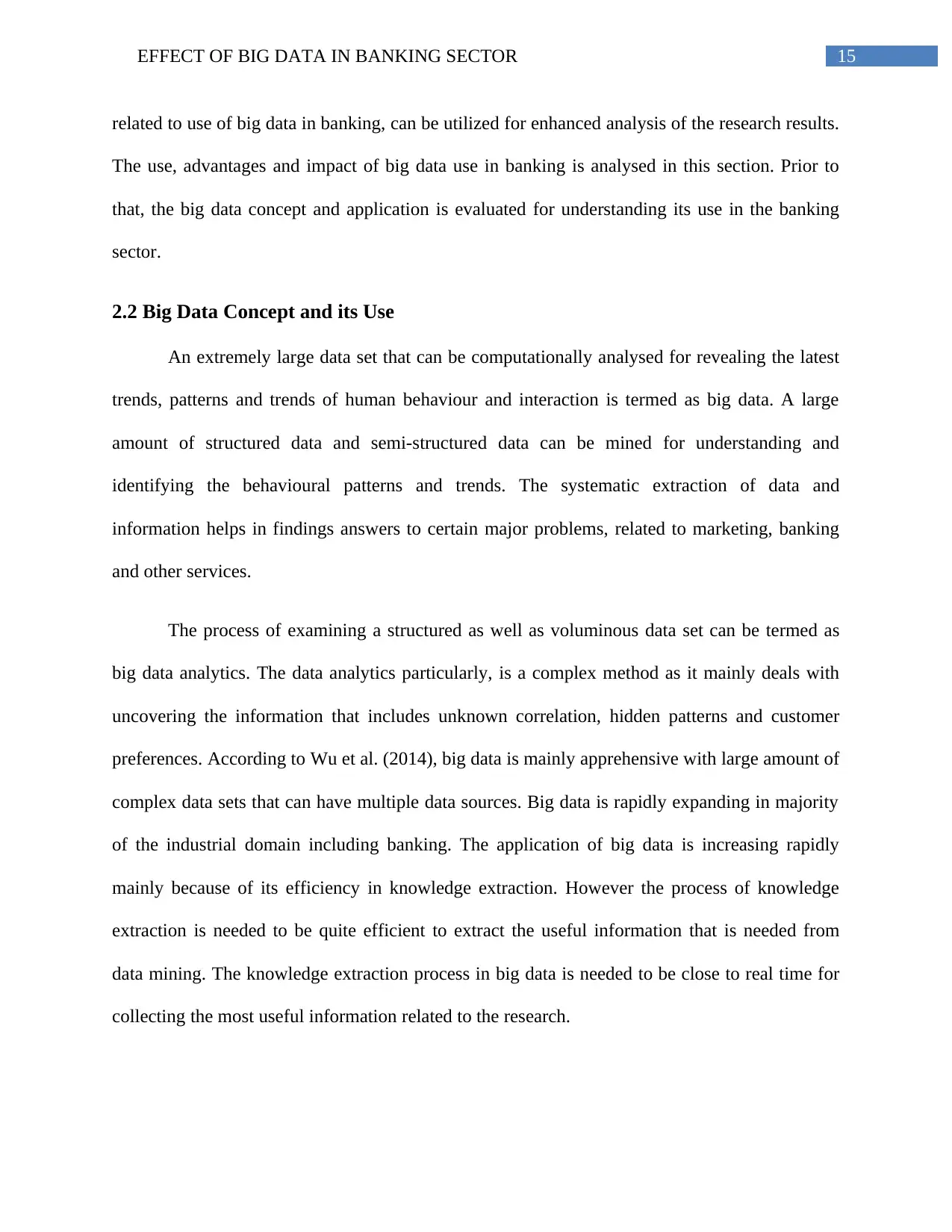
15EFFECT OF BIG DATA IN BANKING SECTOR
related to use of big data in banking, can be utilized for enhanced analysis of the research results.
The use, advantages and impact of big data use in banking is analysed in this section. Prior to
that, the big data concept and application is evaluated for understanding its use in the banking
sector.
2.2 Big Data Concept and its Use
An extremely large data set that can be computationally analysed for revealing the latest
trends, patterns and trends of human behaviour and interaction is termed as big data. A large
amount of structured data and semi-structured data can be mined for understanding and
identifying the behavioural patterns and trends. The systematic extraction of data and
information helps in findings answers to certain major problems, related to marketing, banking
and other services.
The process of examining a structured as well as voluminous data set can be termed as
big data analytics. The data analytics particularly, is a complex method as it mainly deals with
uncovering the information that includes unknown correlation, hidden patterns and customer
preferences. According to Wu et al. (2014), big data is mainly apprehensive with large amount of
complex data sets that can have multiple data sources. Big data is rapidly expanding in majority
of the industrial domain including banking. The application of big data is increasing rapidly
mainly because of its efficiency in knowledge extraction. However the process of knowledge
extraction is needed to be quite efficient to extract the useful information that is needed from
data mining. The knowledge extraction process in big data is needed to be close to real time for
collecting the most useful information related to the research.
related to use of big data in banking, can be utilized for enhanced analysis of the research results.
The use, advantages and impact of big data use in banking is analysed in this section. Prior to
that, the big data concept and application is evaluated for understanding its use in the banking
sector.
2.2 Big Data Concept and its Use
An extremely large data set that can be computationally analysed for revealing the latest
trends, patterns and trends of human behaviour and interaction is termed as big data. A large
amount of structured data and semi-structured data can be mined for understanding and
identifying the behavioural patterns and trends. The systematic extraction of data and
information helps in findings answers to certain major problems, related to marketing, banking
and other services.
The process of examining a structured as well as voluminous data set can be termed as
big data analytics. The data analytics particularly, is a complex method as it mainly deals with
uncovering the information that includes unknown correlation, hidden patterns and customer
preferences. According to Wu et al. (2014), big data is mainly apprehensive with large amount of
complex data sets that can have multiple data sources. Big data is rapidly expanding in majority
of the industrial domain including banking. The application of big data is increasing rapidly
mainly because of its efficiency in knowledge extraction. However the process of knowledge
extraction is needed to be quite efficient to extract the useful information that is needed from
data mining. The knowledge extraction process in big data is needed to be close to real time for
collecting the most useful information related to the research.
Paraphrase This Document
Need a fresh take? Get an instant paraphrase of this document with our AI Paraphraser
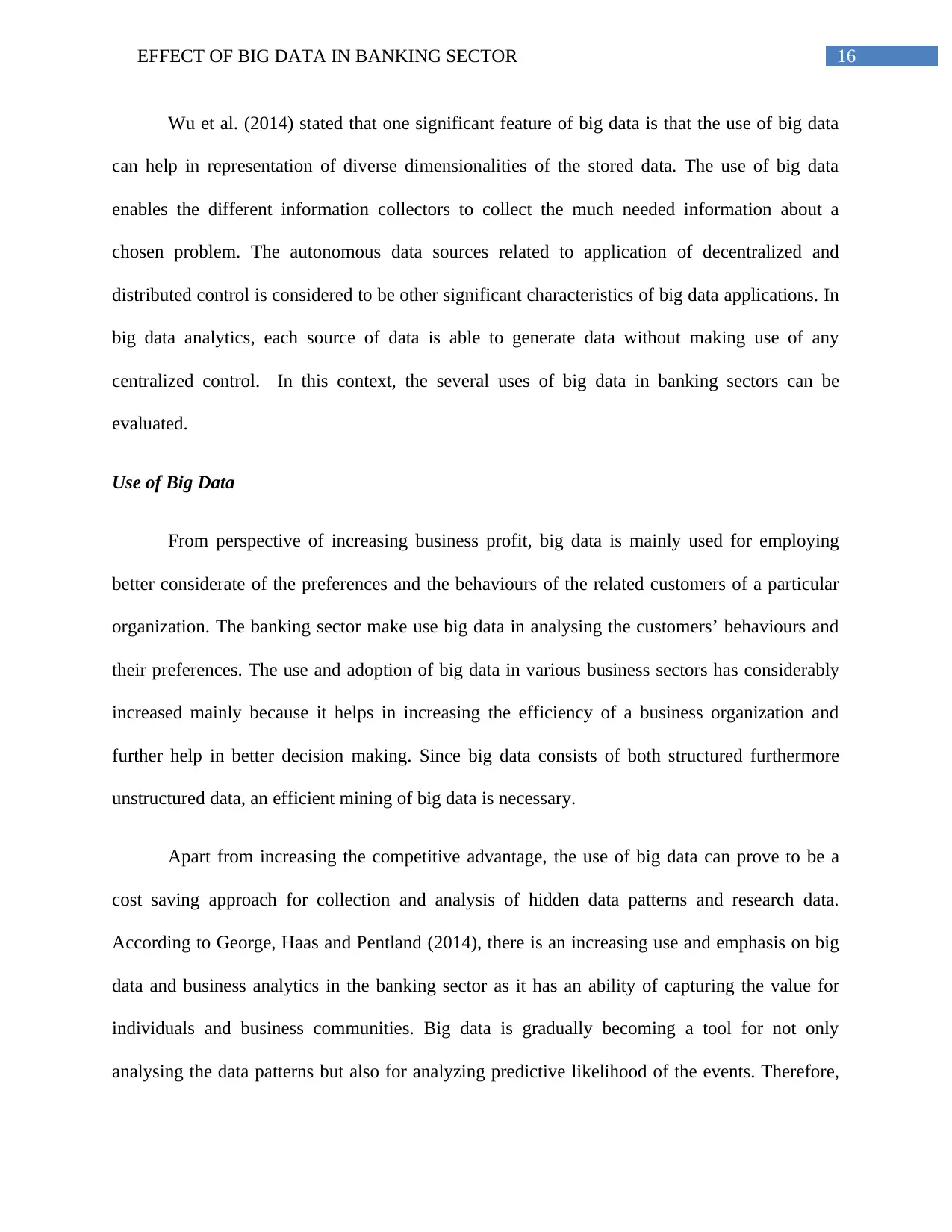
16EFFECT OF BIG DATA IN BANKING SECTOR
Wu et al. (2014) stated that one significant feature of big data is that the use of big data
can help in representation of diverse dimensionalities of the stored data. The use of big data
enables the different information collectors to collect the much needed information about a
chosen problem. The autonomous data sources related to application of decentralized and
distributed control is considered to be other significant characteristics of big data applications. In
big data analytics, each source of data is able to generate data without making use of any
centralized control. In this context, the several uses of big data in banking sectors can be
evaluated.
Use of Big Data
From perspective of increasing business profit, big data is mainly used for employing
better considerate of the preferences and the behaviours of the related customers of a particular
organization. The banking sector make use big data in analysing the customers’ behaviours and
their preferences. The use and adoption of big data in various business sectors has considerably
increased mainly because it helps in increasing the efficiency of a business organization and
further help in better decision making. Since big data consists of both structured furthermore
unstructured data, an efficient mining of big data is necessary.
Apart from increasing the competitive advantage, the use of big data can prove to be a
cost saving approach for collection and analysis of hidden data patterns and research data.
According to George, Haas and Pentland (2014), there is an increasing use and emphasis on big
data and business analytics in the banking sector as it has an ability of capturing the value for
individuals and business communities. Big data is gradually becoming a tool for not only
analysing the data patterns but also for analyzing predictive likelihood of the events. Therefore,
Wu et al. (2014) stated that one significant feature of big data is that the use of big data
can help in representation of diverse dimensionalities of the stored data. The use of big data
enables the different information collectors to collect the much needed information about a
chosen problem. The autonomous data sources related to application of decentralized and
distributed control is considered to be other significant characteristics of big data applications. In
big data analytics, each source of data is able to generate data without making use of any
centralized control. In this context, the several uses of big data in banking sectors can be
evaluated.
Use of Big Data
From perspective of increasing business profit, big data is mainly used for employing
better considerate of the preferences and the behaviours of the related customers of a particular
organization. The banking sector make use big data in analysing the customers’ behaviours and
their preferences. The use and adoption of big data in various business sectors has considerably
increased mainly because it helps in increasing the efficiency of a business organization and
further help in better decision making. Since big data consists of both structured furthermore
unstructured data, an efficient mining of big data is necessary.
Apart from increasing the competitive advantage, the use of big data can prove to be a
cost saving approach for collection and analysis of hidden data patterns and research data.
According to George, Haas and Pentland (2014), there is an increasing use and emphasis on big
data and business analytics in the banking sector as it has an ability of capturing the value for
individuals and business communities. Big data is gradually becoming a tool for not only
analysing the data patterns but also for analyzing predictive likelihood of the events. Therefore,
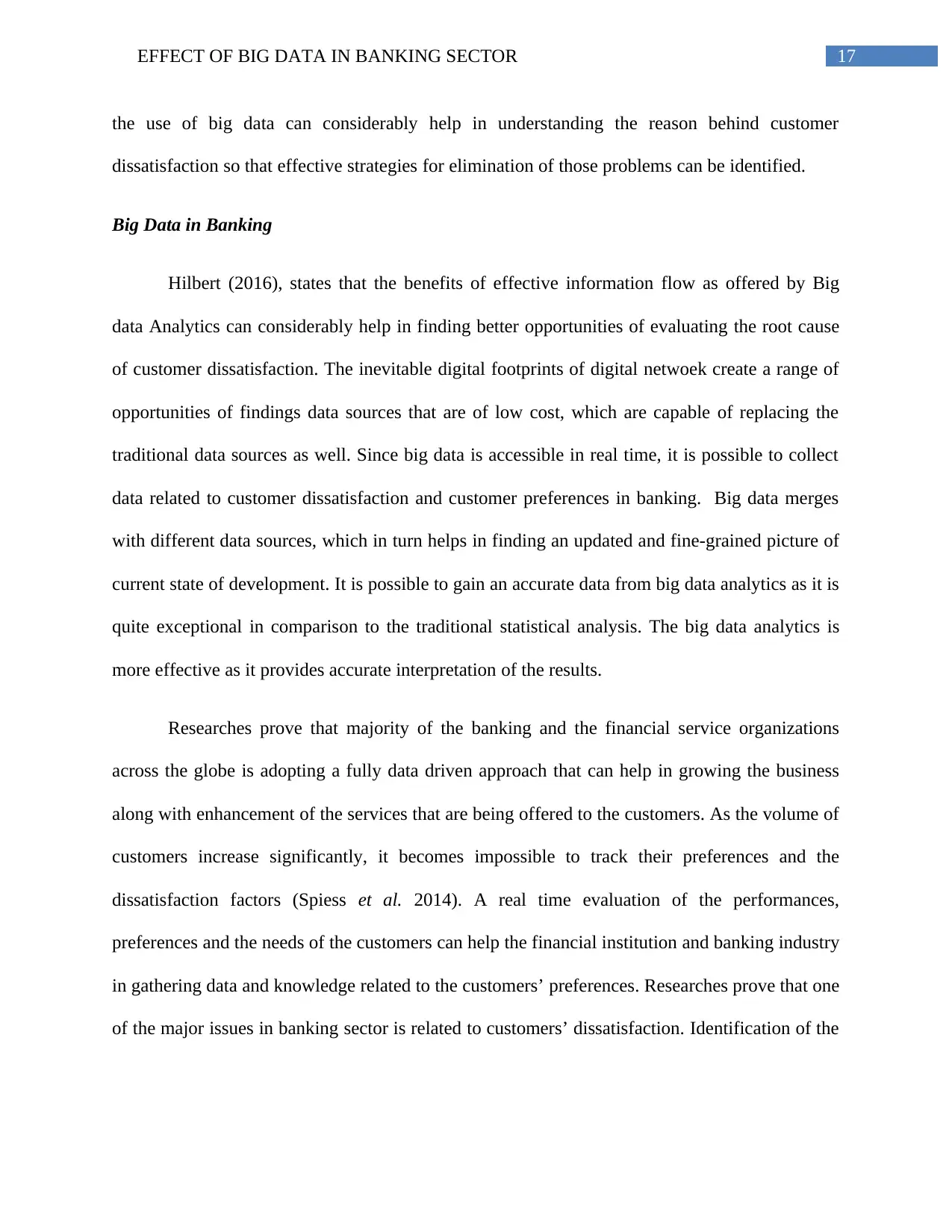
17EFFECT OF BIG DATA IN BANKING SECTOR
the use of big data can considerably help in understanding the reason behind customer
dissatisfaction so that effective strategies for elimination of those problems can be identified.
Big Data in Banking
Hilbert (2016), states that the benefits of effective information flow as offered by Big
data Analytics can considerably help in finding better opportunities of evaluating the root cause
of customer dissatisfaction. The inevitable digital footprints of digital netwoek create a range of
opportunities of findings data sources that are of low cost, which are capable of replacing the
traditional data sources as well. Since big data is accessible in real time, it is possible to collect
data related to customer dissatisfaction and customer preferences in banking. Big data merges
with different data sources, which in turn helps in finding an updated and fine-grained picture of
current state of development. It is possible to gain an accurate data from big data analytics as it is
quite exceptional in comparison to the traditional statistical analysis. The big data analytics is
more effective as it provides accurate interpretation of the results.
Researches prove that majority of the banking and the financial service organizations
across the globe is adopting a fully data driven approach that can help in growing the business
along with enhancement of the services that are being offered to the customers. As the volume of
customers increase significantly, it becomes impossible to track their preferences and the
dissatisfaction factors (Spiess et al. 2014). A real time evaluation of the performances,
preferences and the needs of the customers can help the financial institution and banking industry
in gathering data and knowledge related to the customers’ preferences. Researches prove that one
of the major issues in banking sector is related to customers’ dissatisfaction. Identification of the
the use of big data can considerably help in understanding the reason behind customer
dissatisfaction so that effective strategies for elimination of those problems can be identified.
Big Data in Banking
Hilbert (2016), states that the benefits of effective information flow as offered by Big
data Analytics can considerably help in finding better opportunities of evaluating the root cause
of customer dissatisfaction. The inevitable digital footprints of digital netwoek create a range of
opportunities of findings data sources that are of low cost, which are capable of replacing the
traditional data sources as well. Since big data is accessible in real time, it is possible to collect
data related to customer dissatisfaction and customer preferences in banking. Big data merges
with different data sources, which in turn helps in finding an updated and fine-grained picture of
current state of development. It is possible to gain an accurate data from big data analytics as it is
quite exceptional in comparison to the traditional statistical analysis. The big data analytics is
more effective as it provides accurate interpretation of the results.
Researches prove that majority of the banking and the financial service organizations
across the globe is adopting a fully data driven approach that can help in growing the business
along with enhancement of the services that are being offered to the customers. As the volume of
customers increase significantly, it becomes impossible to track their preferences and the
dissatisfaction factors (Spiess et al. 2014). A real time evaluation of the performances,
preferences and the needs of the customers can help the financial institution and banking industry
in gathering data and knowledge related to the customers’ preferences. Researches prove that one
of the major issues in banking sector is related to customers’ dissatisfaction. Identification of the
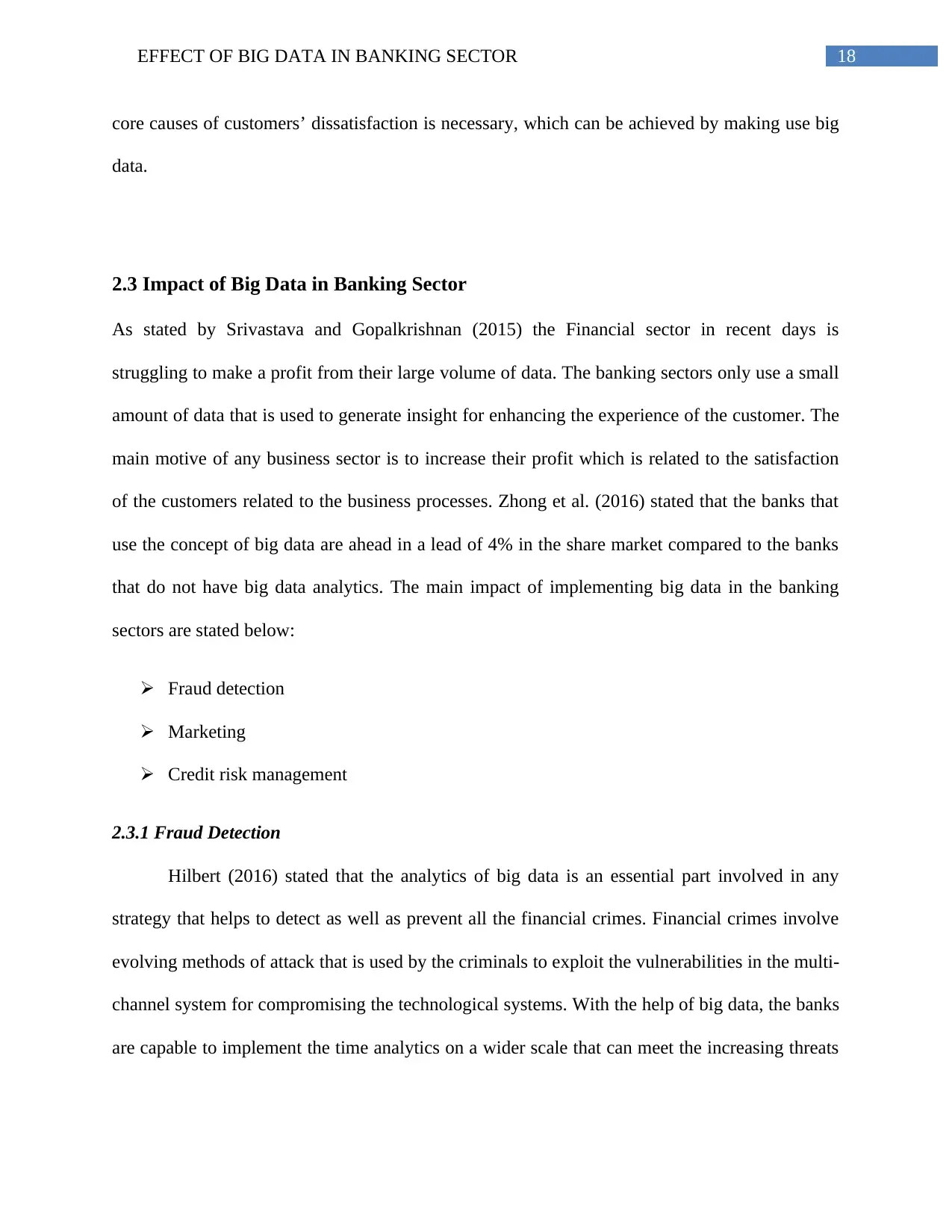
18EFFECT OF BIG DATA IN BANKING SECTOR
core causes of customers’ dissatisfaction is necessary, which can be achieved by making use big
data.
2.3 Impact of Big Data in Banking Sector
As stated by Srivastava and Gopalkrishnan (2015) the Financial sector in recent days is
struggling to make a profit from their large volume of data. The banking sectors only use a small
amount of data that is used to generate insight for enhancing the experience of the customer. The
main motive of any business sector is to increase their profit which is related to the satisfaction
of the customers related to the business processes. Zhong et al. (2016) stated that the banks that
use the concept of big data are ahead in a lead of 4% in the share market compared to the banks
that do not have big data analytics. The main impact of implementing big data in the banking
sectors are stated below:
Fraud detection
Marketing
Credit risk management
2.3.1 Fraud Detection
Hilbert (2016) stated that the analytics of big data is an essential part involved in any
strategy that helps to detect as well as prevent all the financial crimes. Financial crimes involve
evolving methods of attack that is used by the criminals to exploit the vulnerabilities in the multi-
channel system for compromising the technological systems. With the help of big data, the banks
are capable to implement the time analytics on a wider scale that can meet the increasing threats
core causes of customers’ dissatisfaction is necessary, which can be achieved by making use big
data.
2.3 Impact of Big Data in Banking Sector
As stated by Srivastava and Gopalkrishnan (2015) the Financial sector in recent days is
struggling to make a profit from their large volume of data. The banking sectors only use a small
amount of data that is used to generate insight for enhancing the experience of the customer. The
main motive of any business sector is to increase their profit which is related to the satisfaction
of the customers related to the business processes. Zhong et al. (2016) stated that the banks that
use the concept of big data are ahead in a lead of 4% in the share market compared to the banks
that do not have big data analytics. The main impact of implementing big data in the banking
sectors are stated below:
Fraud detection
Marketing
Credit risk management
2.3.1 Fraud Detection
Hilbert (2016) stated that the analytics of big data is an essential part involved in any
strategy that helps to detect as well as prevent all the financial crimes. Financial crimes involve
evolving methods of attack that is used by the criminals to exploit the vulnerabilities in the multi-
channel system for compromising the technological systems. With the help of big data, the banks
are capable to implement the time analytics on a wider scale that can meet the increasing threats
Secure Best Marks with AI Grader
Need help grading? Try our AI Grader for instant feedback on your assignments.
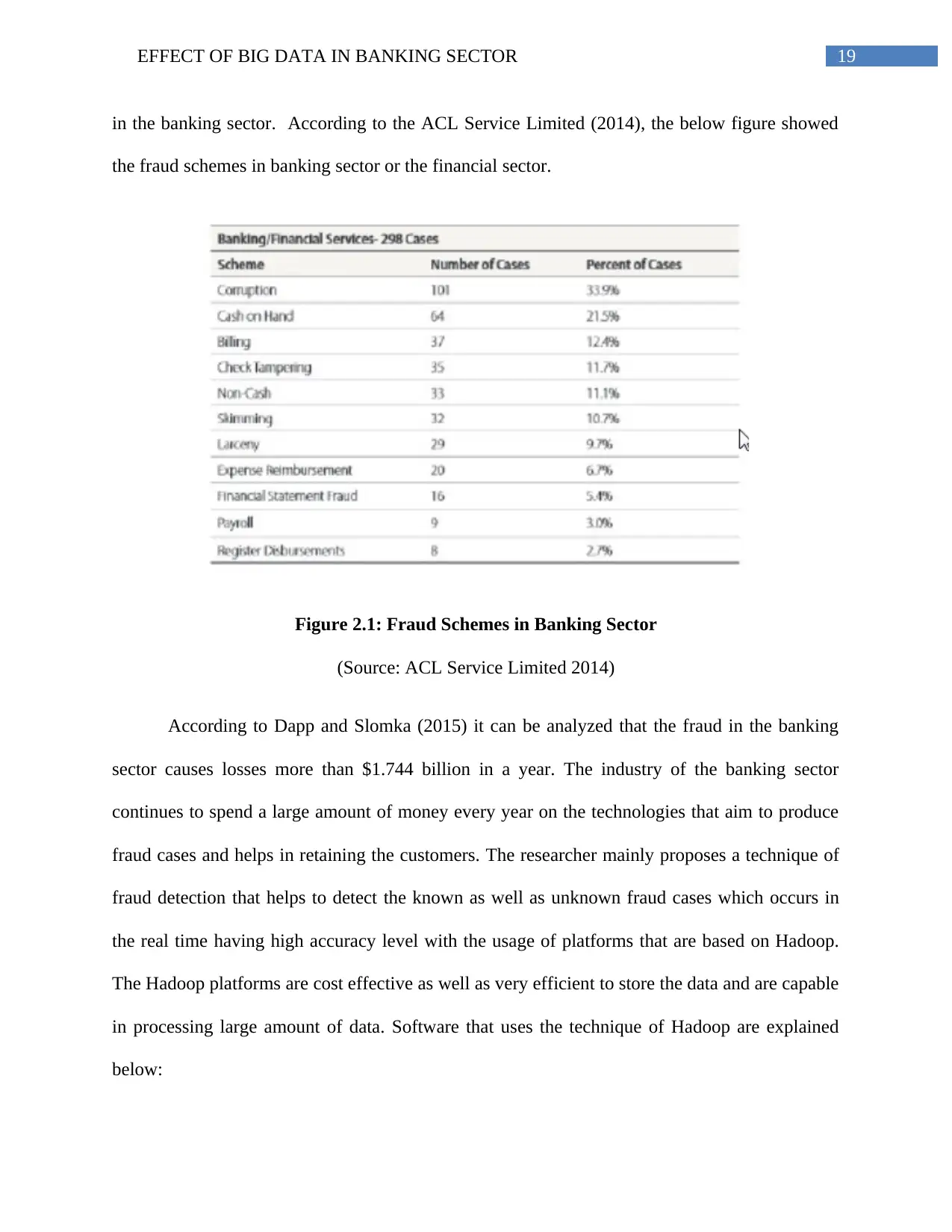
19EFFECT OF BIG DATA IN BANKING SECTOR
in the banking sector. According to the ACL Service Limited (2014), the below figure showed
the fraud schemes in banking sector or the financial sector.
Figure 2.1: Fraud Schemes in Banking Sector
(Source: ACL Service Limited 2014)
According to Dapp and Slomka (2015) it can be analyzed that the fraud in the banking
sector causes losses more than $1.744 billion in a year. The industry of the banking sector
continues to spend a large amount of money every year on the technologies that aim to produce
fraud cases and helps in retaining the customers. The researcher mainly proposes a technique of
fraud detection that helps to detect the known as well as unknown fraud cases which occurs in
the real time having high accuracy level with the usage of platforms that are based on Hadoop.
The Hadoop platforms are cost effective as well as very efficient to store the data and are capable
in processing large amount of data. Software that uses the technique of Hadoop are explained
below:
in the banking sector. According to the ACL Service Limited (2014), the below figure showed
the fraud schemes in banking sector or the financial sector.
Figure 2.1: Fraud Schemes in Banking Sector
(Source: ACL Service Limited 2014)
According to Dapp and Slomka (2015) it can be analyzed that the fraud in the banking
sector causes losses more than $1.744 billion in a year. The industry of the banking sector
continues to spend a large amount of money every year on the technologies that aim to produce
fraud cases and helps in retaining the customers. The researcher mainly proposes a technique of
fraud detection that helps to detect the known as well as unknown fraud cases which occurs in
the real time having high accuracy level with the usage of platforms that are based on Hadoop.
The Hadoop platforms are cost effective as well as very efficient to store the data and are capable
in processing large amount of data. Software that uses the technique of Hadoop are explained
below:
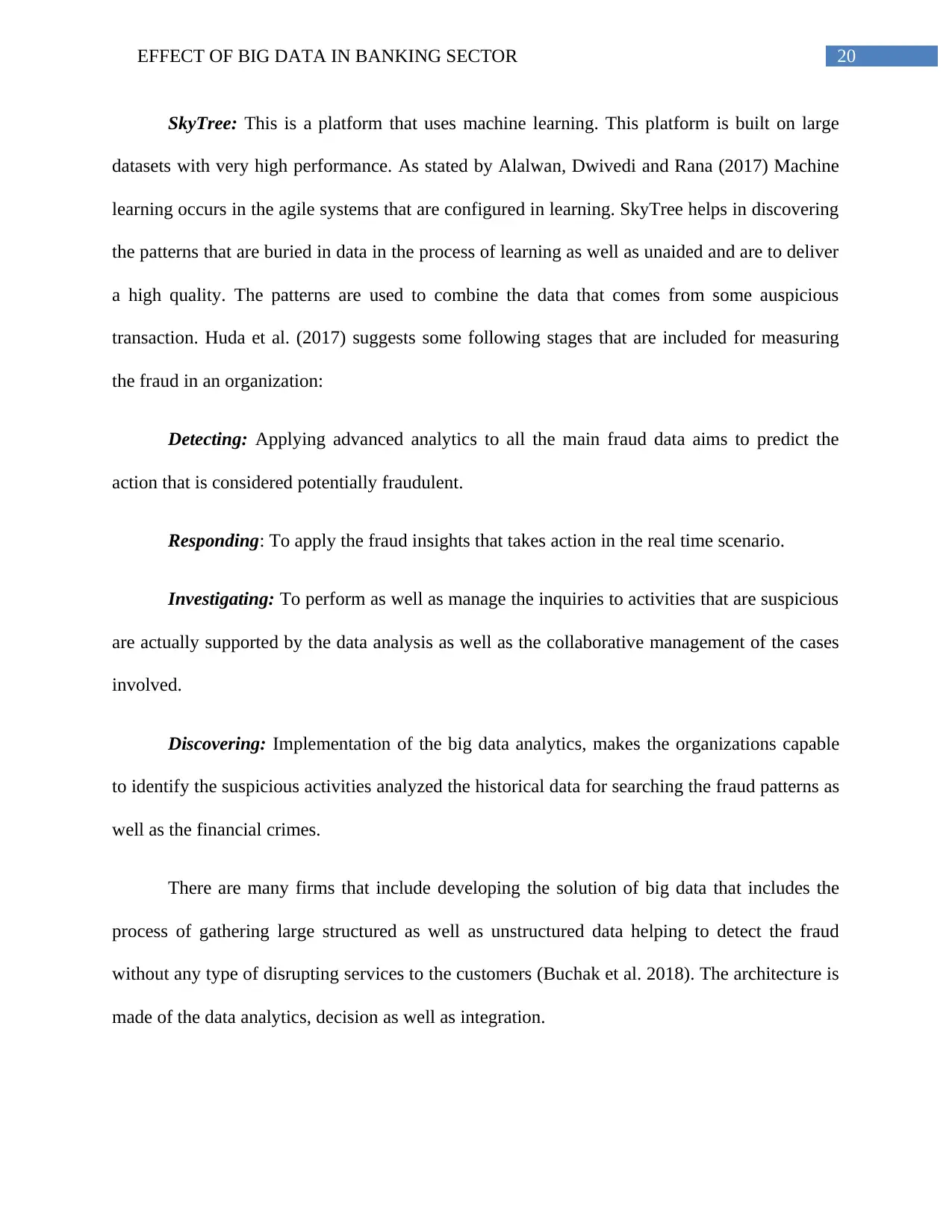
20EFFECT OF BIG DATA IN BANKING SECTOR
SkyTree: This is a platform that uses machine learning. This platform is built on large
datasets with very high performance. As stated by Alalwan, Dwivedi and Rana (2017) Machine
learning occurs in the agile systems that are configured in learning. SkyTree helps in discovering
the patterns that are buried in data in the process of learning as well as unaided and are to deliver
a high quality. The patterns are used to combine the data that comes from some auspicious
transaction. Huda et al. (2017) suggests some following stages that are included for measuring
the fraud in an organization:
Detecting: Applying advanced analytics to all the main fraud data aims to predict the
action that is considered potentially fraudulent.
Responding: To apply the fraud insights that takes action in the real time scenario.
Investigating: To perform as well as manage the inquiries to activities that are suspicious
are actually supported by the data analysis as well as the collaborative management of the cases
involved.
Discovering: Implementation of the big data analytics, makes the organizations capable
to identify the suspicious activities analyzed the historical data for searching the fraud patterns as
well as the financial crimes.
There are many firms that include developing the solution of big data that includes the
process of gathering large structured as well as unstructured data helping to detect the fraud
without any type of disrupting services to the customers (Buchak et al. 2018). The architecture is
made of the data analytics, decision as well as integration.
SkyTree: This is a platform that uses machine learning. This platform is built on large
datasets with very high performance. As stated by Alalwan, Dwivedi and Rana (2017) Machine
learning occurs in the agile systems that are configured in learning. SkyTree helps in discovering
the patterns that are buried in data in the process of learning as well as unaided and are to deliver
a high quality. The patterns are used to combine the data that comes from some auspicious
transaction. Huda et al. (2017) suggests some following stages that are included for measuring
the fraud in an organization:
Detecting: Applying advanced analytics to all the main fraud data aims to predict the
action that is considered potentially fraudulent.
Responding: To apply the fraud insights that takes action in the real time scenario.
Investigating: To perform as well as manage the inquiries to activities that are suspicious
are actually supported by the data analysis as well as the collaborative management of the cases
involved.
Discovering: Implementation of the big data analytics, makes the organizations capable
to identify the suspicious activities analyzed the historical data for searching the fraud patterns as
well as the financial crimes.
There are many firms that include developing the solution of big data that includes the
process of gathering large structured as well as unstructured data helping to detect the fraud
without any type of disrupting services to the customers (Buchak et al. 2018). The architecture is
made of the data analytics, decision as well as integration.
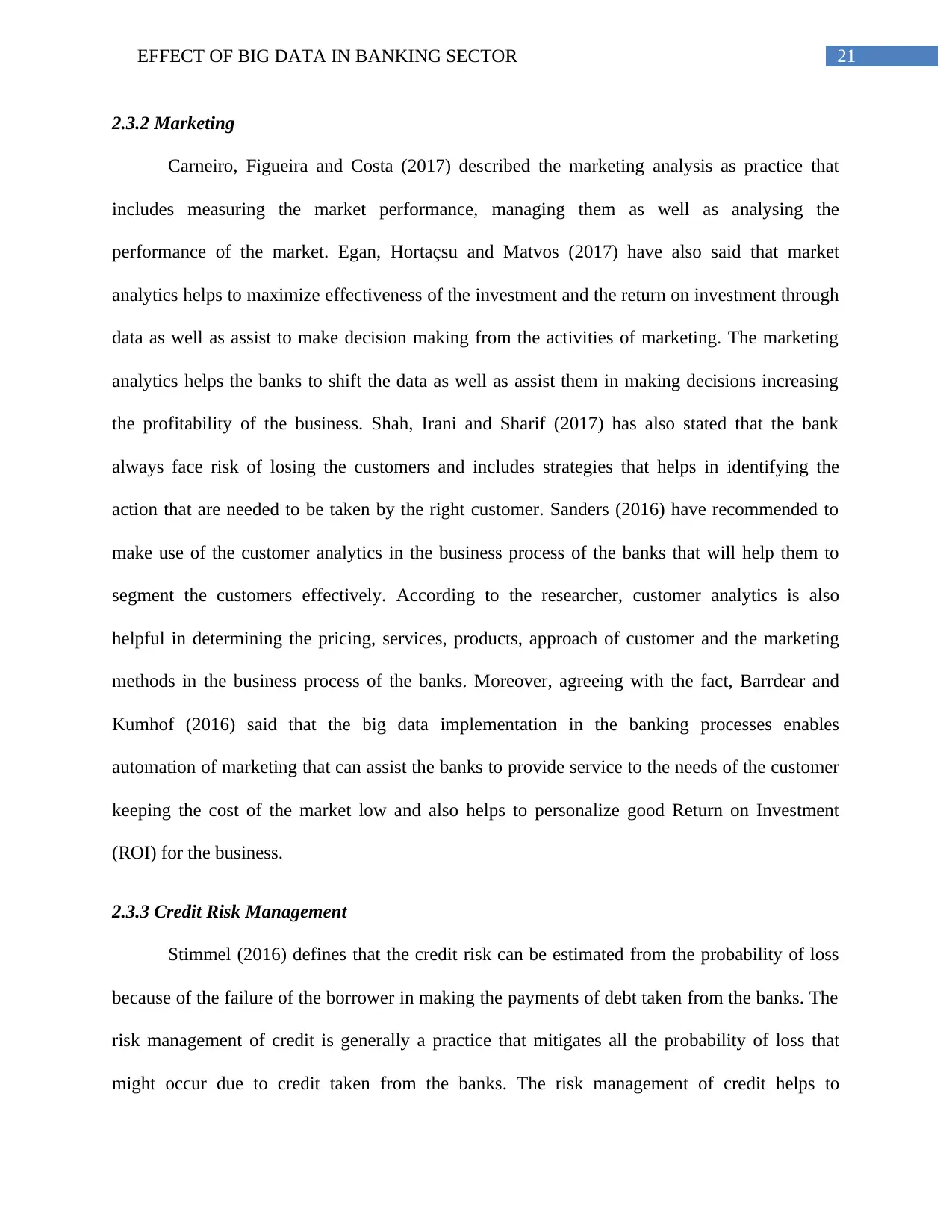
21EFFECT OF BIG DATA IN BANKING SECTOR
2.3.2 Marketing
Carneiro, Figueira and Costa (2017) described the marketing analysis as practice that
includes measuring the market performance, managing them as well as analysing the
performance of the market. Egan, Hortaçsu and Matvos (2017) have also said that market
analytics helps to maximize effectiveness of the investment and the return on investment through
data as well as assist to make decision making from the activities of marketing. The marketing
analytics helps the banks to shift the data as well as assist them in making decisions increasing
the profitability of the business. Shah, Irani and Sharif (2017) has also stated that the bank
always face risk of losing the customers and includes strategies that helps in identifying the
action that are needed to be taken by the right customer. Sanders (2016) have recommended to
make use of the customer analytics in the business process of the banks that will help them to
segment the customers effectively. According to the researcher, customer analytics is also
helpful in determining the pricing, services, products, approach of customer and the marketing
methods in the business process of the banks. Moreover, agreeing with the fact, Barrdear and
Kumhof (2016) said that the big data implementation in the banking processes enables
automation of marketing that can assist the banks to provide service to the needs of the customer
keeping the cost of the market low and also helps to personalize good Return on Investment
(ROI) for the business.
2.3.3 Credit Risk Management
Stimmel (2016) defines that the credit risk can be estimated from the probability of loss
because of the failure of the borrower in making the payments of debt taken from the banks. The
risk management of credit is generally a practice that mitigates all the probability of loss that
might occur due to credit taken from the banks. The risk management of credit helps to
2.3.2 Marketing
Carneiro, Figueira and Costa (2017) described the marketing analysis as practice that
includes measuring the market performance, managing them as well as analysing the
performance of the market. Egan, Hortaçsu and Matvos (2017) have also said that market
analytics helps to maximize effectiveness of the investment and the return on investment through
data as well as assist to make decision making from the activities of marketing. The marketing
analytics helps the banks to shift the data as well as assist them in making decisions increasing
the profitability of the business. Shah, Irani and Sharif (2017) has also stated that the bank
always face risk of losing the customers and includes strategies that helps in identifying the
action that are needed to be taken by the right customer. Sanders (2016) have recommended to
make use of the customer analytics in the business process of the banks that will help them to
segment the customers effectively. According to the researcher, customer analytics is also
helpful in determining the pricing, services, products, approach of customer and the marketing
methods in the business process of the banks. Moreover, agreeing with the fact, Barrdear and
Kumhof (2016) said that the big data implementation in the banking processes enables
automation of marketing that can assist the banks to provide service to the needs of the customer
keeping the cost of the market low and also helps to personalize good Return on Investment
(ROI) for the business.
2.3.3 Credit Risk Management
Stimmel (2016) defines that the credit risk can be estimated from the probability of loss
because of the failure of the borrower in making the payments of debt taken from the banks. The
risk management of credit is generally a practice that mitigates all the probability of loss that
might occur due to credit taken from the banks. The risk management of credit helps to
Paraphrase This Document
Need a fresh take? Get an instant paraphrase of this document with our AI Paraphraser
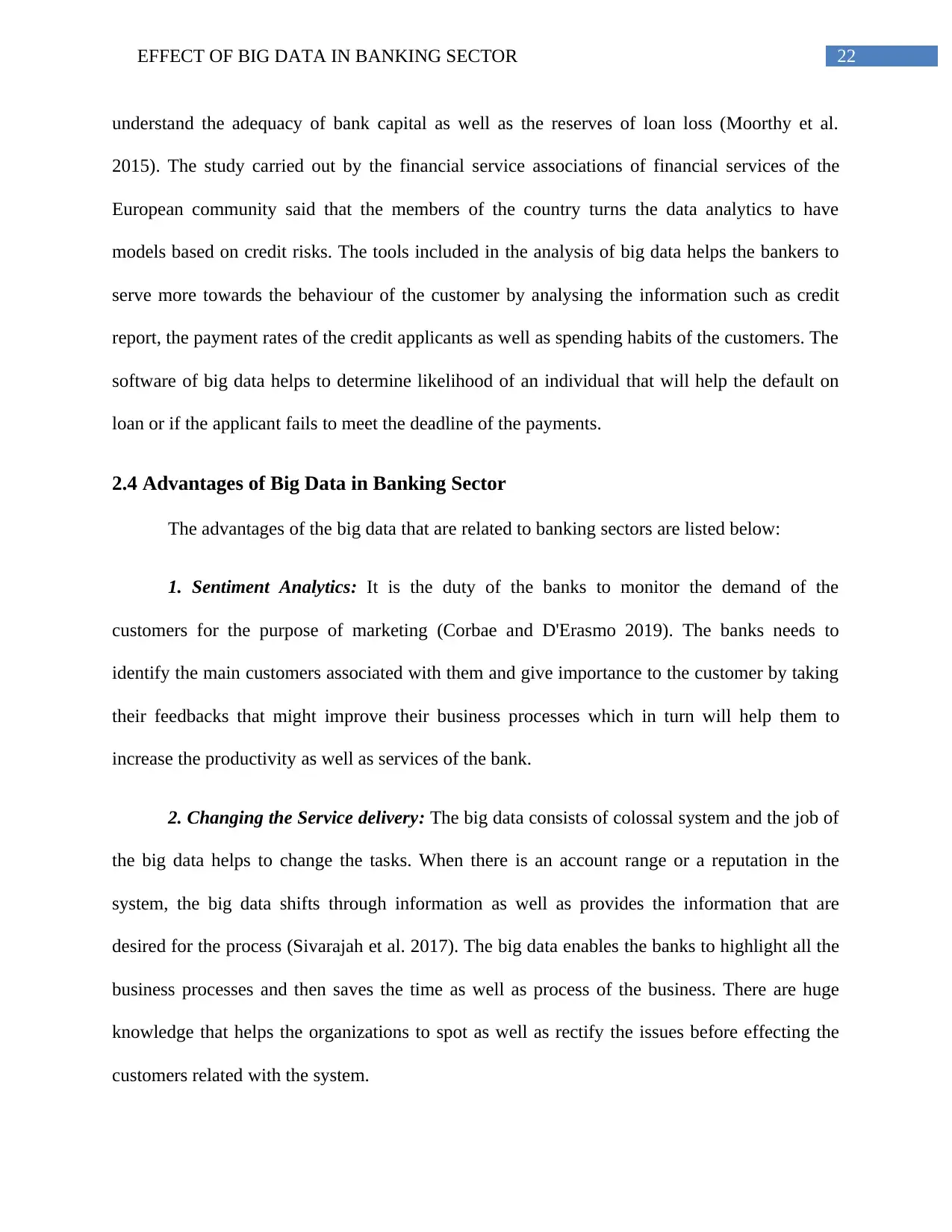
22EFFECT OF BIG DATA IN BANKING SECTOR
understand the adequacy of bank capital as well as the reserves of loan loss (Moorthy et al.
2015). The study carried out by the financial service associations of financial services of the
European community said that the members of the country turns the data analytics to have
models based on credit risks. The tools included in the analysis of big data helps the bankers to
serve more towards the behaviour of the customer by analysing the information such as credit
report, the payment rates of the credit applicants as well as spending habits of the customers. The
software of big data helps to determine likelihood of an individual that will help the default on
loan or if the applicant fails to meet the deadline of the payments.
2.4 Advantages of Big Data in Banking Sector
The advantages of the big data that are related to banking sectors are listed below:
1. Sentiment Analytics: It is the duty of the banks to monitor the demand of the
customers for the purpose of marketing (Corbae and D'Erasmo 2019). The banks needs to
identify the main customers associated with them and give importance to the customer by taking
their feedbacks that might improve their business processes which in turn will help them to
increase the productivity as well as services of the bank.
2. Changing the Service delivery: The big data consists of colossal system and the job of
the big data helps to change the tasks. When there is an account range or a reputation in the
system, the big data shifts through information as well as provides the information that are
desired for the process (Sivarajah et al. 2017). The big data enables the banks to highlight all the
business processes and then saves the time as well as process of the business. There are huge
knowledge that helps the organizations to spot as well as rectify the issues before effecting the
customers related with the system.
understand the adequacy of bank capital as well as the reserves of loan loss (Moorthy et al.
2015). The study carried out by the financial service associations of financial services of the
European community said that the members of the country turns the data analytics to have
models based on credit risks. The tools included in the analysis of big data helps the bankers to
serve more towards the behaviour of the customer by analysing the information such as credit
report, the payment rates of the credit applicants as well as spending habits of the customers. The
software of big data helps to determine likelihood of an individual that will help the default on
loan or if the applicant fails to meet the deadline of the payments.
2.4 Advantages of Big Data in Banking Sector
The advantages of the big data that are related to banking sectors are listed below:
1. Sentiment Analytics: It is the duty of the banks to monitor the demand of the
customers for the purpose of marketing (Corbae and D'Erasmo 2019). The banks needs to
identify the main customers associated with them and give importance to the customer by taking
their feedbacks that might improve their business processes which in turn will help them to
increase the productivity as well as services of the bank.
2. Changing the Service delivery: The big data consists of colossal system and the job of
the big data helps to change the tasks. When there is an account range or a reputation in the
system, the big data shifts through information as well as provides the information that are
desired for the process (Sivarajah et al. 2017). The big data enables the banks to highlight all the
business processes and then saves the time as well as process of the business. There are huge
knowledge that helps the organizations to spot as well as rectify the issues before effecting the
customers related with the system.
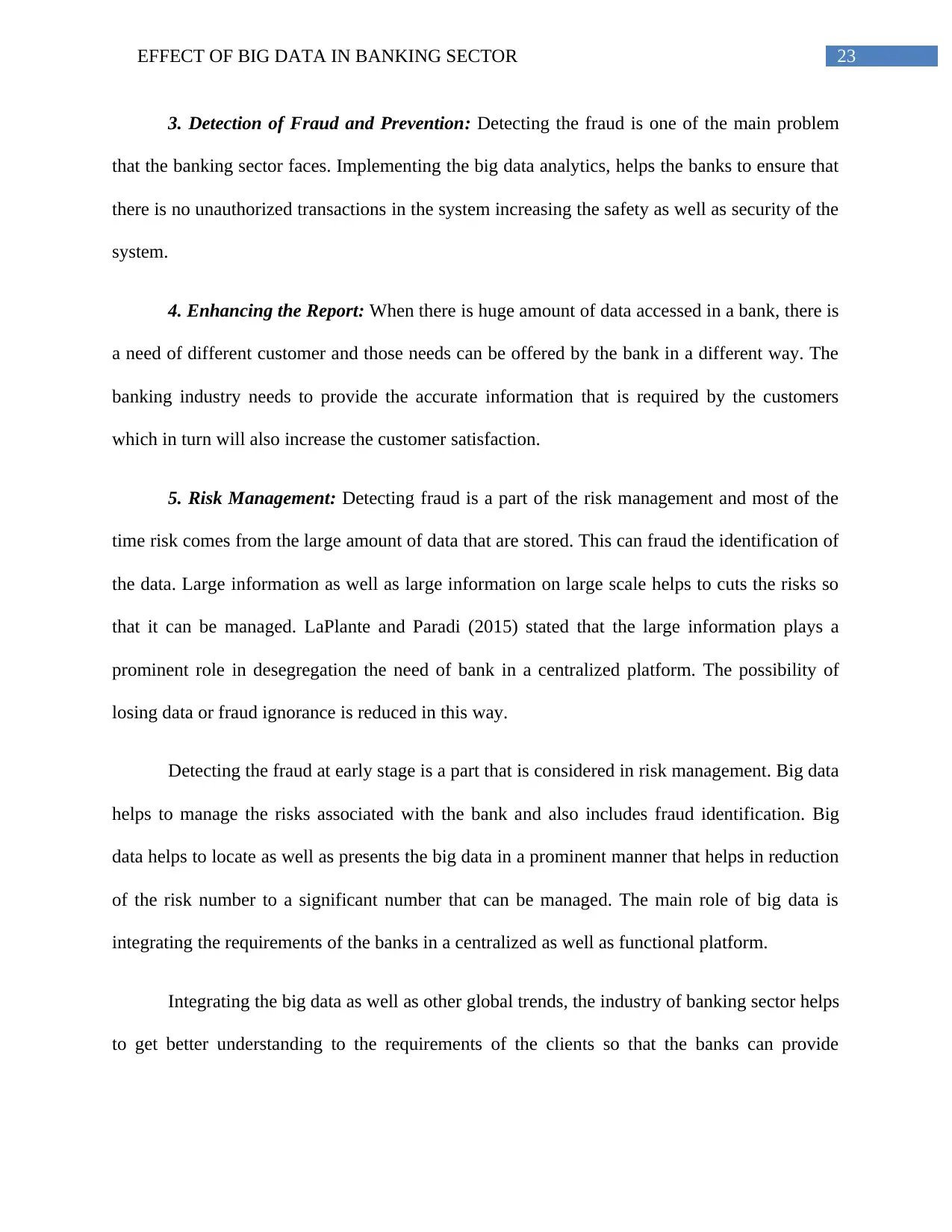
23EFFECT OF BIG DATA IN BANKING SECTOR
3. Detection of Fraud and Prevention: Detecting the fraud is one of the main problem
that the banking sector faces. Implementing the big data analytics, helps the banks to ensure that
there is no unauthorized transactions in the system increasing the safety as well as security of the
system.
4. Enhancing the Report: When there is huge amount of data accessed in a bank, there is
a need of different customer and those needs can be offered by the bank in a different way. The
banking industry needs to provide the accurate information that is required by the customers
which in turn will also increase the customer satisfaction.
5. Risk Management: Detecting fraud is a part of the risk management and most of the
time risk comes from the large amount of data that are stored. This can fraud the identification of
the data. Large information as well as large information on large scale helps to cuts the risks so
that it can be managed. LaPlante and Paradi (2015) stated that the large information plays a
prominent role in desegregation the need of bank in a centralized platform. The possibility of
losing data or fraud ignorance is reduced in this way.
Detecting the fraud at early stage is a part that is considered in risk management. Big data
helps to manage the risks associated with the bank and also includes fraud identification. Big
data helps to locate as well as presents the big data in a prominent manner that helps in reduction
of the risk number to a significant number that can be managed. The main role of big data is
integrating the requirements of the banks in a centralized as well as functional platform.
Integrating the big data as well as other global trends, the industry of banking sector helps
to get better understanding to the requirements of the clients so that the banks can provide
3. Detection of Fraud and Prevention: Detecting the fraud is one of the main problem
that the banking sector faces. Implementing the big data analytics, helps the banks to ensure that
there is no unauthorized transactions in the system increasing the safety as well as security of the
system.
4. Enhancing the Report: When there is huge amount of data accessed in a bank, there is
a need of different customer and those needs can be offered by the bank in a different way. The
banking industry needs to provide the accurate information that is required by the customers
which in turn will also increase the customer satisfaction.
5. Risk Management: Detecting fraud is a part of the risk management and most of the
time risk comes from the large amount of data that are stored. This can fraud the identification of
the data. Large information as well as large information on large scale helps to cuts the risks so
that it can be managed. LaPlante and Paradi (2015) stated that the large information plays a
prominent role in desegregation the need of bank in a centralized platform. The possibility of
losing data or fraud ignorance is reduced in this way.
Detecting the fraud at early stage is a part that is considered in risk management. Big data
helps to manage the risks associated with the bank and also includes fraud identification. Big
data helps to locate as well as presents the big data in a prominent manner that helps in reduction
of the risk number to a significant number that can be managed. The main role of big data is
integrating the requirements of the banks in a centralized as well as functional platform.
Integrating the big data as well as other global trends, the industry of banking sector helps
to get better understanding to the requirements of the clients so that the banks can provide
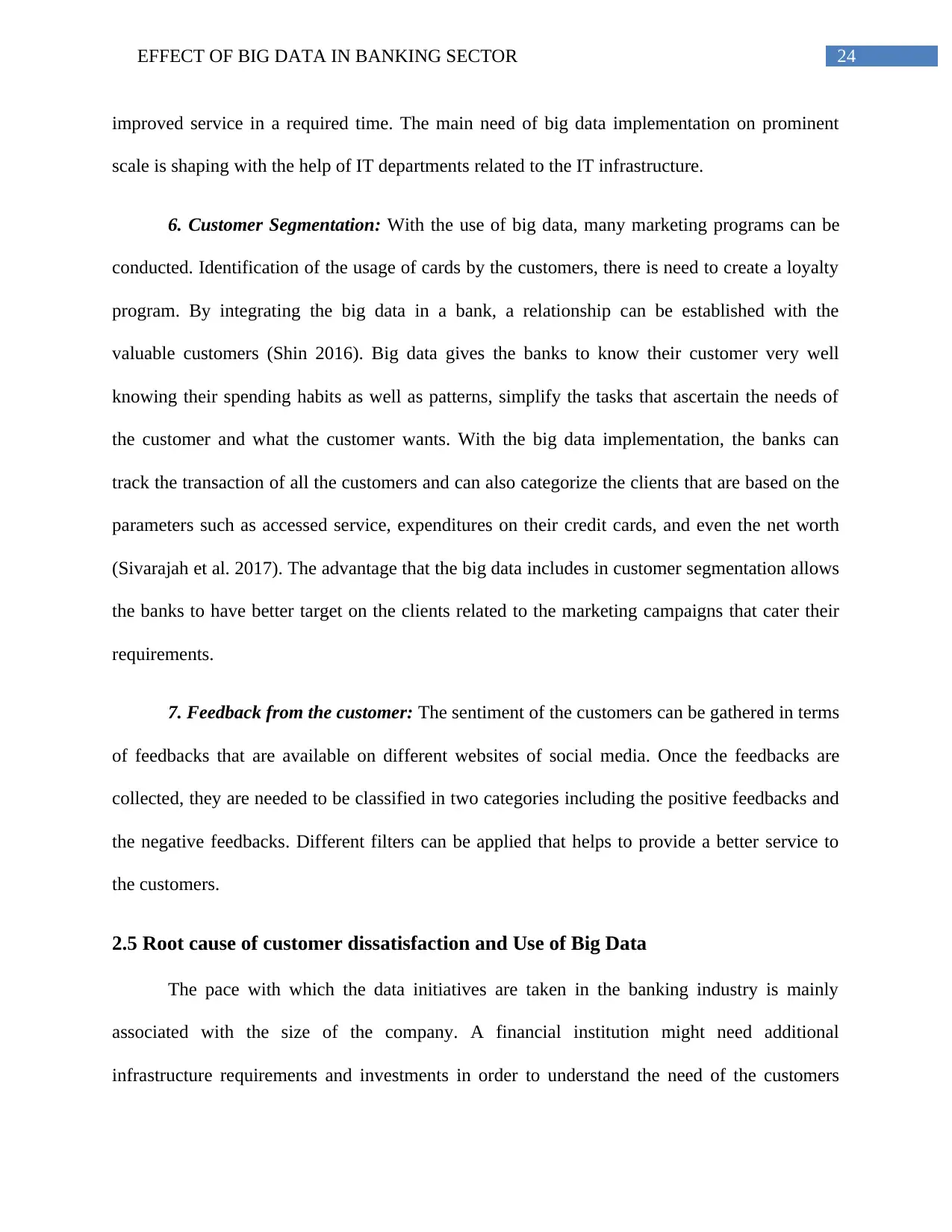
24EFFECT OF BIG DATA IN BANKING SECTOR
improved service in a required time. The main need of big data implementation on prominent
scale is shaping with the help of IT departments related to the IT infrastructure.
6. Customer Segmentation: With the use of big data, many marketing programs can be
conducted. Identification of the usage of cards by the customers, there is need to create a loyalty
program. By integrating the big data in a bank, a relationship can be established with the
valuable customers (Shin 2016). Big data gives the banks to know their customer very well
knowing their spending habits as well as patterns, simplify the tasks that ascertain the needs of
the customer and what the customer wants. With the big data implementation, the banks can
track the transaction of all the customers and can also categorize the clients that are based on the
parameters such as accessed service, expenditures on their credit cards, and even the net worth
(Sivarajah et al. 2017). The advantage that the big data includes in customer segmentation allows
the banks to have better target on the clients related to the marketing campaigns that cater their
requirements.
7. Feedback from the customer: The sentiment of the customers can be gathered in terms
of feedbacks that are available on different websites of social media. Once the feedbacks are
collected, they are needed to be classified in two categories including the positive feedbacks and
the negative feedbacks. Different filters can be applied that helps to provide a better service to
the customers.
2.5 Root cause of customer dissatisfaction and Use of Big Data
The pace with which the data initiatives are taken in the banking industry is mainly
associated with the size of the company. A financial institution might need additional
infrastructure requirements and investments in order to understand the need of the customers
improved service in a required time. The main need of big data implementation on prominent
scale is shaping with the help of IT departments related to the IT infrastructure.
6. Customer Segmentation: With the use of big data, many marketing programs can be
conducted. Identification of the usage of cards by the customers, there is need to create a loyalty
program. By integrating the big data in a bank, a relationship can be established with the
valuable customers (Shin 2016). Big data gives the banks to know their customer very well
knowing their spending habits as well as patterns, simplify the tasks that ascertain the needs of
the customer and what the customer wants. With the big data implementation, the banks can
track the transaction of all the customers and can also categorize the clients that are based on the
parameters such as accessed service, expenditures on their credit cards, and even the net worth
(Sivarajah et al. 2017). The advantage that the big data includes in customer segmentation allows
the banks to have better target on the clients related to the marketing campaigns that cater their
requirements.
7. Feedback from the customer: The sentiment of the customers can be gathered in terms
of feedbacks that are available on different websites of social media. Once the feedbacks are
collected, they are needed to be classified in two categories including the positive feedbacks and
the negative feedbacks. Different filters can be applied that helps to provide a better service to
the customers.
2.5 Root cause of customer dissatisfaction and Use of Big Data
The pace with which the data initiatives are taken in the banking industry is mainly
associated with the size of the company. A financial institution might need additional
infrastructure requirements and investments in order to understand the need of the customers
Secure Best Marks with AI Grader
Need help grading? Try our AI Grader for instant feedback on your assignments.
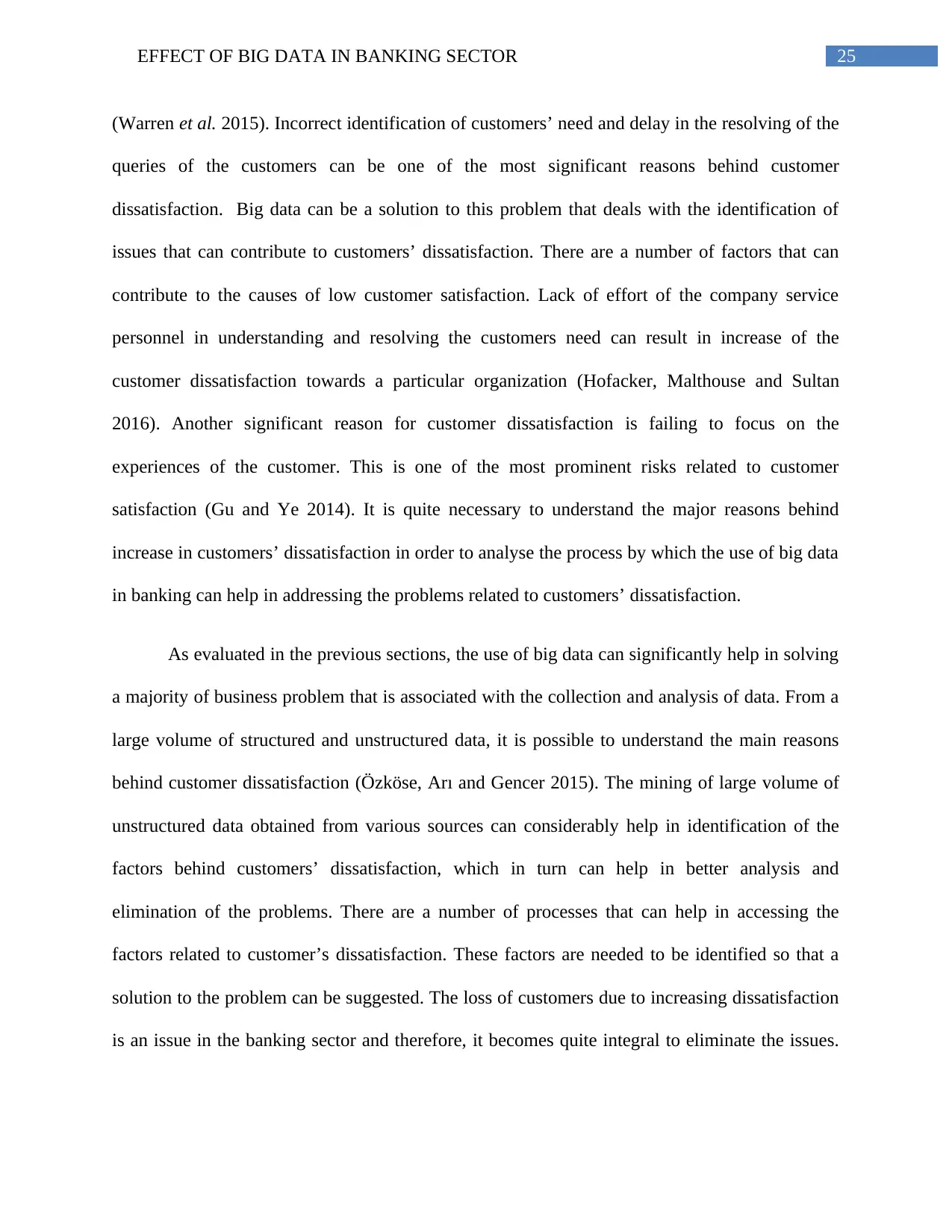
25EFFECT OF BIG DATA IN BANKING SECTOR
(Warren et al. 2015). Incorrect identification of customers’ need and delay in the resolving of the
queries of the customers can be one of the most significant reasons behind customer
dissatisfaction. Big data can be a solution to this problem that deals with the identification of
issues that can contribute to customers’ dissatisfaction. There are a number of factors that can
contribute to the causes of low customer satisfaction. Lack of effort of the company service
personnel in understanding and resolving the customers need can result in increase of the
customer dissatisfaction towards a particular organization (Hofacker, Malthouse and Sultan
2016). Another significant reason for customer dissatisfaction is failing to focus on the
experiences of the customer. This is one of the most prominent risks related to customer
satisfaction (Gu and Ye 2014). It is quite necessary to understand the major reasons behind
increase in customers’ dissatisfaction in order to analyse the process by which the use of big data
in banking can help in addressing the problems related to customers’ dissatisfaction.
As evaluated in the previous sections, the use of big data can significantly help in solving
a majority of business problem that is associated with the collection and analysis of data. From a
large volume of structured and unstructured data, it is possible to understand the main reasons
behind customer dissatisfaction (Özköse, Arı and Gencer 2015). The mining of large volume of
unstructured data obtained from various sources can considerably help in identification of the
factors behind customers’ dissatisfaction, which in turn can help in better analysis and
elimination of the problems. There are a number of processes that can help in accessing the
factors related to customer’s dissatisfaction. These factors are needed to be identified so that a
solution to the problem can be suggested. The loss of customers due to increasing dissatisfaction
is an issue in the banking sector and therefore, it becomes quite integral to eliminate the issues.
(Warren et al. 2015). Incorrect identification of customers’ need and delay in the resolving of the
queries of the customers can be one of the most significant reasons behind customer
dissatisfaction. Big data can be a solution to this problem that deals with the identification of
issues that can contribute to customers’ dissatisfaction. There are a number of factors that can
contribute to the causes of low customer satisfaction. Lack of effort of the company service
personnel in understanding and resolving the customers need can result in increase of the
customer dissatisfaction towards a particular organization (Hofacker, Malthouse and Sultan
2016). Another significant reason for customer dissatisfaction is failing to focus on the
experiences of the customer. This is one of the most prominent risks related to customer
satisfaction (Gu and Ye 2014). It is quite necessary to understand the major reasons behind
increase in customers’ dissatisfaction in order to analyse the process by which the use of big data
in banking can help in addressing the problems related to customers’ dissatisfaction.
As evaluated in the previous sections, the use of big data can significantly help in solving
a majority of business problem that is associated with the collection and analysis of data. From a
large volume of structured and unstructured data, it is possible to understand the main reasons
behind customer dissatisfaction (Özköse, Arı and Gencer 2015). The mining of large volume of
unstructured data obtained from various sources can considerably help in identification of the
factors behind customers’ dissatisfaction, which in turn can help in better analysis and
elimination of the problems. There are a number of processes that can help in accessing the
factors related to customer’s dissatisfaction. These factors are needed to be identified so that a
solution to the problem can be suggested. The loss of customers due to increasing dissatisfaction
is an issue in the banking sector and therefore, it becomes quite integral to eliminate the issues.
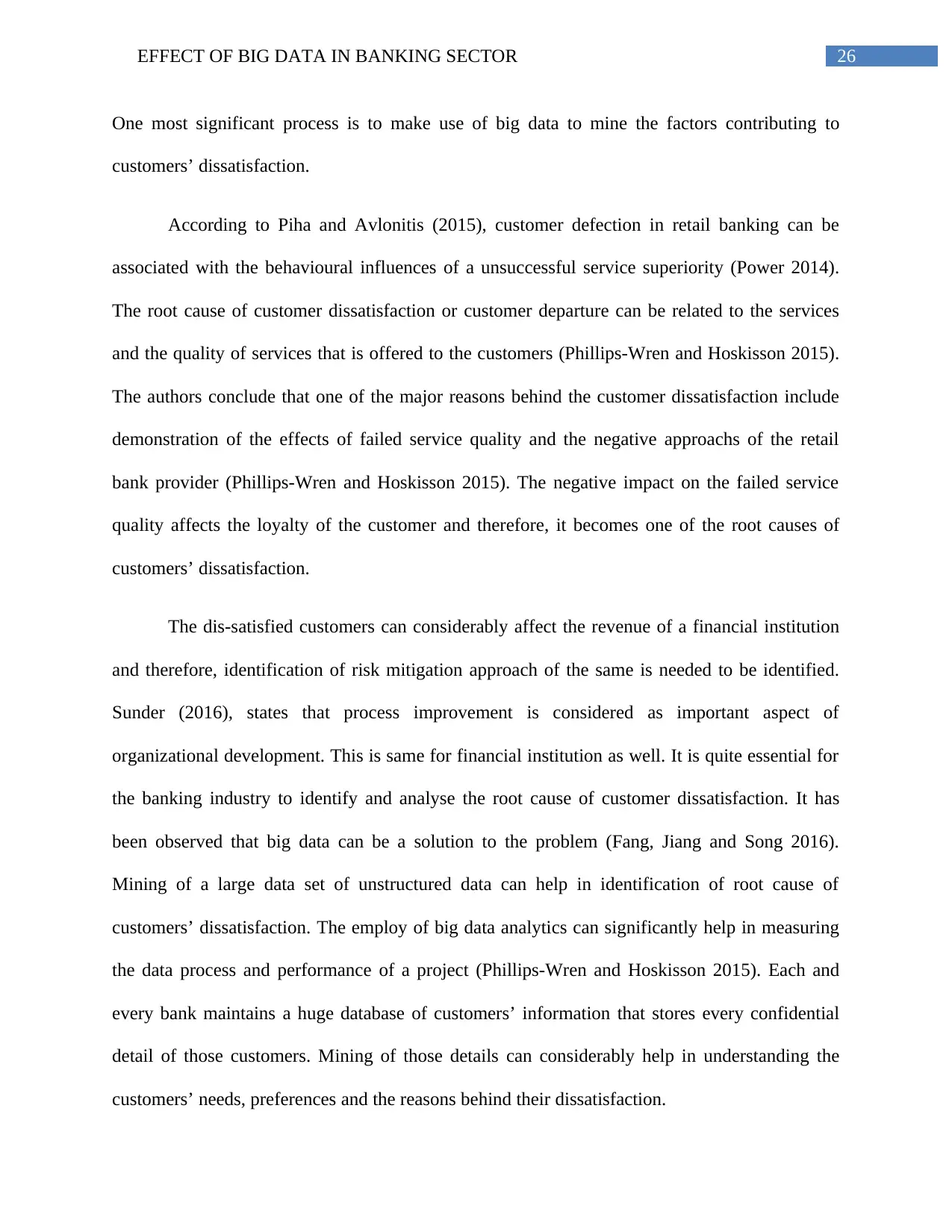
26EFFECT OF BIG DATA IN BANKING SECTOR
One most significant process is to make use of big data to mine the factors contributing to
customers’ dissatisfaction.
According to Piha and Avlonitis (2015), customer defection in retail banking can be
associated with the behavioural influences of a unsuccessful service superiority (Power 2014).
The root cause of customer dissatisfaction or customer departure can be related to the services
and the quality of services that is offered to the customers (Phillips-Wren and Hoskisson 2015).
The authors conclude that one of the major reasons behind the customer dissatisfaction include
demonstration of the effects of failed service quality and the negative approachs of the retail
bank provider (Phillips-Wren and Hoskisson 2015). The negative impact on the failed service
quality affects the loyalty of the customer and therefore, it becomes one of the root causes of
customers’ dissatisfaction.
The dis-satisfied customers can considerably affect the revenue of a financial institution
and therefore, identification of risk mitigation approach of the same is needed to be identified.
Sunder (2016), states that process improvement is considered as important aspect of
organizational development. This is same for financial institution as well. It is quite essential for
the banking industry to identify and analyse the root cause of customer dissatisfaction. It has
been observed that big data can be a solution to the problem (Fang, Jiang and Song 2016).
Mining of a large data set of unstructured data can help in identification of root cause of
customers’ dissatisfaction. The employ of big data analytics can significantly help in measuring
the data process and performance of a project (Phillips-Wren and Hoskisson 2015). Each and
every bank maintains a huge database of customers’ information that stores every confidential
detail of those customers. Mining of those details can considerably help in understanding the
customers’ needs, preferences and the reasons behind their dissatisfaction.
One most significant process is to make use of big data to mine the factors contributing to
customers’ dissatisfaction.
According to Piha and Avlonitis (2015), customer defection in retail banking can be
associated with the behavioural influences of a unsuccessful service superiority (Power 2014).
The root cause of customer dissatisfaction or customer departure can be related to the services
and the quality of services that is offered to the customers (Phillips-Wren and Hoskisson 2015).
The authors conclude that one of the major reasons behind the customer dissatisfaction include
demonstration of the effects of failed service quality and the negative approachs of the retail
bank provider (Phillips-Wren and Hoskisson 2015). The negative impact on the failed service
quality affects the loyalty of the customer and therefore, it becomes one of the root causes of
customers’ dissatisfaction.
The dis-satisfied customers can considerably affect the revenue of a financial institution
and therefore, identification of risk mitigation approach of the same is needed to be identified.
Sunder (2016), states that process improvement is considered as important aspect of
organizational development. This is same for financial institution as well. It is quite essential for
the banking industry to identify and analyse the root cause of customer dissatisfaction. It has
been observed that big data can be a solution to the problem (Fang, Jiang and Song 2016).
Mining of a large data set of unstructured data can help in identification of root cause of
customers’ dissatisfaction. The employ of big data analytics can significantly help in measuring
the data process and performance of a project (Phillips-Wren and Hoskisson 2015). Each and
every bank maintains a huge database of customers’ information that stores every confidential
detail of those customers. Mining of those details can considerably help in understanding the
customers’ needs, preferences and the reasons behind their dissatisfaction.
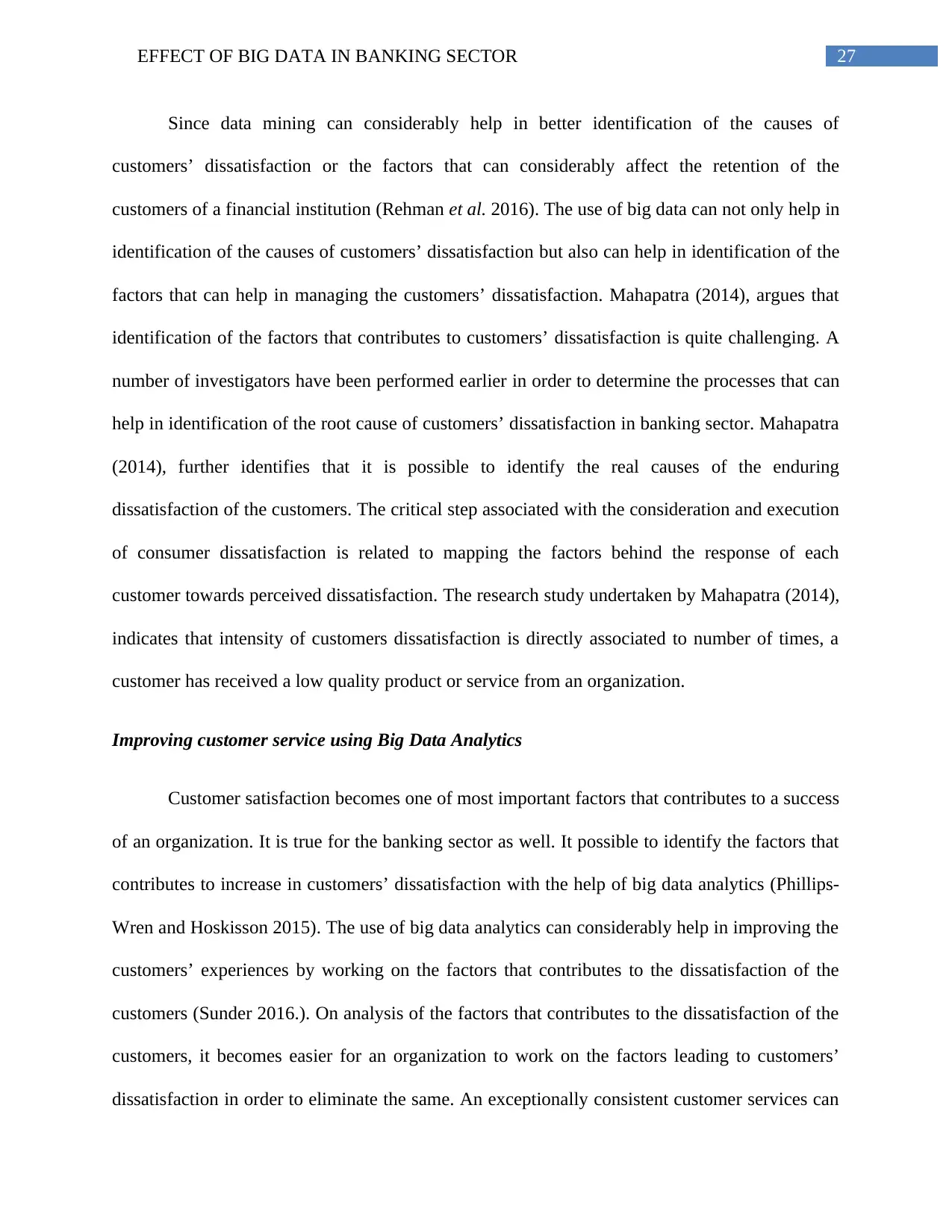
27EFFECT OF BIG DATA IN BANKING SECTOR
Since data mining can considerably help in better identification of the causes of
customers’ dissatisfaction or the factors that can considerably affect the retention of the
customers of a financial institution (Rehman et al. 2016). The use of big data can not only help in
identification of the causes of customers’ dissatisfaction but also can help in identification of the
factors that can help in managing the customers’ dissatisfaction. Mahapatra (2014), argues that
identification of the factors that contributes to customers’ dissatisfaction is quite challenging. A
number of investigators have been performed earlier in order to determine the processes that can
help in identification of the root cause of customers’ dissatisfaction in banking sector. Mahapatra
(2014), further identifies that it is possible to identify the real causes of the enduring
dissatisfaction of the customers. The critical step associated with the consideration and execution
of consumer dissatisfaction is related to mapping the factors behind the response of each
customer towards perceived dissatisfaction. The research study undertaken by Mahapatra (2014),
indicates that intensity of customers dissatisfaction is directly associated to number of times, a
customer has received a low quality product or service from an organization.
Improving customer service using Big Data Analytics
Customer satisfaction becomes one of most important factors that contributes to a success
of an organization. It is true for the banking sector as well. It possible to identify the factors that
contributes to increase in customers’ dissatisfaction with the help of big data analytics (Phillips-
Wren and Hoskisson 2015). The use of big data analytics can considerably help in improving the
customers’ experiences by working on the factors that contributes to the dissatisfaction of the
customers (Sunder 2016.). On analysis of the factors that contributes to the dissatisfaction of the
customers, it becomes easier for an organization to work on the factors leading to customers’
dissatisfaction in order to eliminate the same. An exceptionally consistent customer services can
Since data mining can considerably help in better identification of the causes of
customers’ dissatisfaction or the factors that can considerably affect the retention of the
customers of a financial institution (Rehman et al. 2016). The use of big data can not only help in
identification of the causes of customers’ dissatisfaction but also can help in identification of the
factors that can help in managing the customers’ dissatisfaction. Mahapatra (2014), argues that
identification of the factors that contributes to customers’ dissatisfaction is quite challenging. A
number of investigators have been performed earlier in order to determine the processes that can
help in identification of the root cause of customers’ dissatisfaction in banking sector. Mahapatra
(2014), further identifies that it is possible to identify the real causes of the enduring
dissatisfaction of the customers. The critical step associated with the consideration and execution
of consumer dissatisfaction is related to mapping the factors behind the response of each
customer towards perceived dissatisfaction. The research study undertaken by Mahapatra (2014),
indicates that intensity of customers dissatisfaction is directly associated to number of times, a
customer has received a low quality product or service from an organization.
Improving customer service using Big Data Analytics
Customer satisfaction becomes one of most important factors that contributes to a success
of an organization. It is true for the banking sector as well. It possible to identify the factors that
contributes to increase in customers’ dissatisfaction with the help of big data analytics (Phillips-
Wren and Hoskisson 2015). The use of big data analytics can considerably help in improving the
customers’ experiences by working on the factors that contributes to the dissatisfaction of the
customers (Sunder 2016.). On analysis of the factors that contributes to the dissatisfaction of the
customers, it becomes easier for an organization to work on the factors leading to customers’
dissatisfaction in order to eliminate the same. An exceptionally consistent customer services can
Paraphrase This Document
Need a fresh take? Get an instant paraphrase of this document with our AI Paraphraser
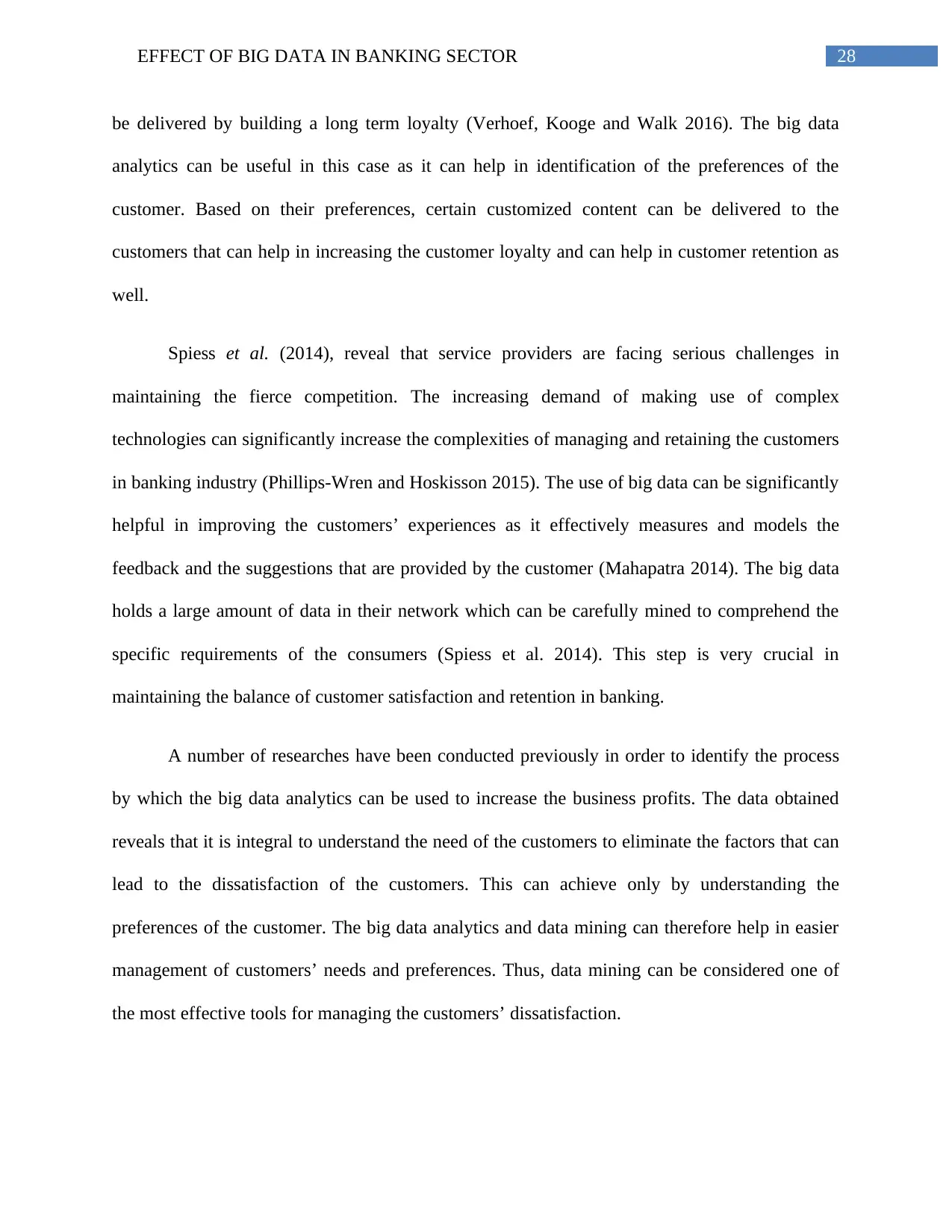
28EFFECT OF BIG DATA IN BANKING SECTOR
be delivered by building a long term loyalty (Verhoef, Kooge and Walk 2016). The big data
analytics can be useful in this case as it can help in identification of the preferences of the
customer. Based on their preferences, certain customized content can be delivered to the
customers that can help in increasing the customer loyalty and can help in customer retention as
well.
Spiess et al. (2014), reveal that service providers are facing serious challenges in
maintaining the fierce competition. The increasing demand of making use of complex
technologies can significantly increase the complexities of managing and retaining the customers
in banking industry (Phillips-Wren and Hoskisson 2015). The use of big data can be significantly
helpful in improving the customers’ experiences as it effectively measures and models the
feedback and the suggestions that are provided by the customer (Mahapatra 2014). The big data
holds a large amount of data in their network which can be carefully mined to comprehend the
specific requirements of the consumers (Spiess et al. 2014). This step is very crucial in
maintaining the balance of customer satisfaction and retention in banking.
A number of researches have been conducted previously in order to identify the process
by which the big data analytics can be used to increase the business profits. The data obtained
reveals that it is integral to understand the need of the customers to eliminate the factors that can
lead to the dissatisfaction of the customers. This can achieve only by understanding the
preferences of the customer. The big data analytics and data mining can therefore help in easier
management of customers’ needs and preferences. Thus, data mining can be considered one of
the most effective tools for managing the customers’ dissatisfaction.
be delivered by building a long term loyalty (Verhoef, Kooge and Walk 2016). The big data
analytics can be useful in this case as it can help in identification of the preferences of the
customer. Based on their preferences, certain customized content can be delivered to the
customers that can help in increasing the customer loyalty and can help in customer retention as
well.
Spiess et al. (2014), reveal that service providers are facing serious challenges in
maintaining the fierce competition. The increasing demand of making use of complex
technologies can significantly increase the complexities of managing and retaining the customers
in banking industry (Phillips-Wren and Hoskisson 2015). The use of big data can be significantly
helpful in improving the customers’ experiences as it effectively measures and models the
feedback and the suggestions that are provided by the customer (Mahapatra 2014). The big data
holds a large amount of data in their network which can be carefully mined to comprehend the
specific requirements of the consumers (Spiess et al. 2014). This step is very crucial in
maintaining the balance of customer satisfaction and retention in banking.
A number of researches have been conducted previously in order to identify the process
by which the big data analytics can be used to increase the business profits. The data obtained
reveals that it is integral to understand the need of the customers to eliminate the factors that can
lead to the dissatisfaction of the customers. This can achieve only by understanding the
preferences of the customer. The big data analytics and data mining can therefore help in easier
management of customers’ needs and preferences. Thus, data mining can be considered one of
the most effective tools for managing the customers’ dissatisfaction.
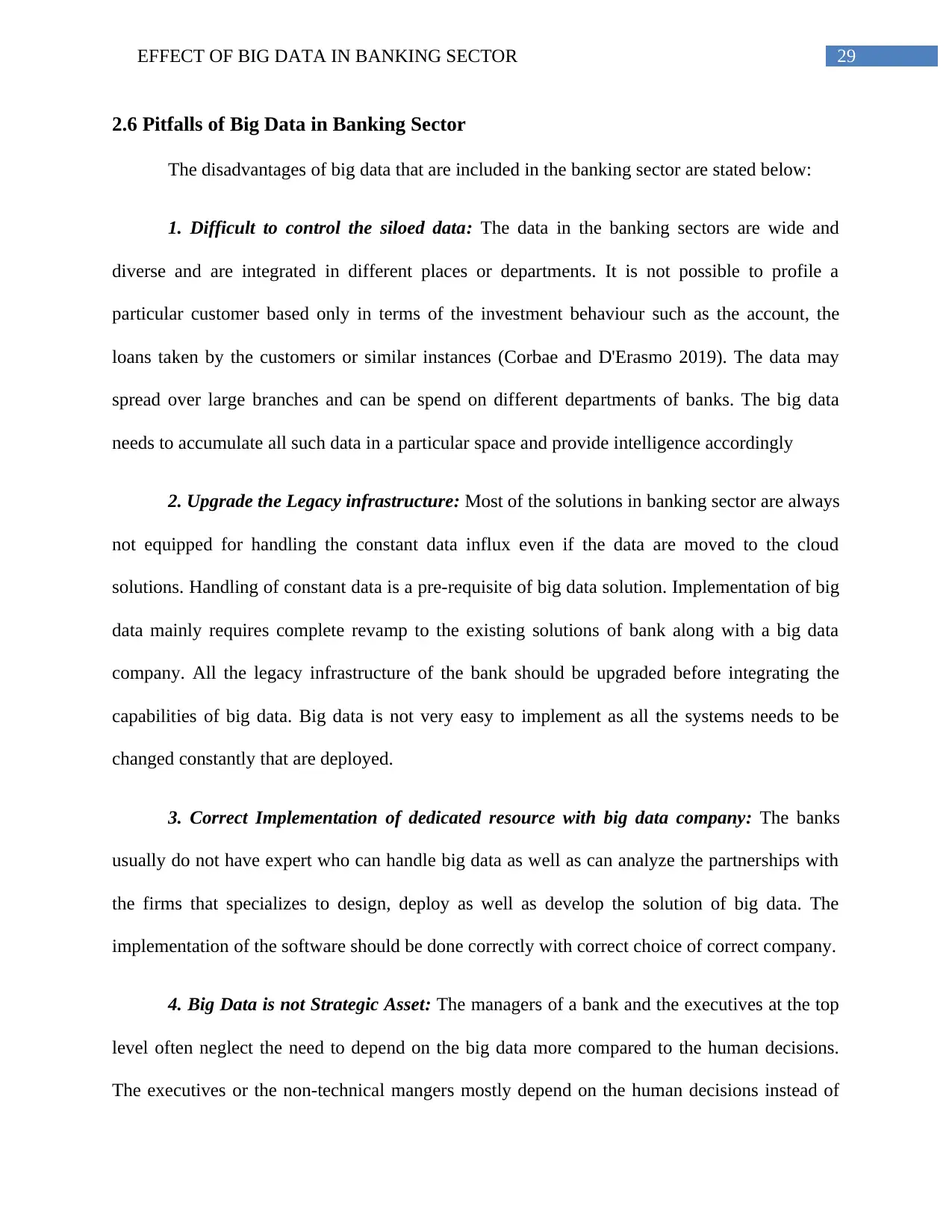
29EFFECT OF BIG DATA IN BANKING SECTOR
2.6 Pitfalls of Big Data in Banking Sector
The disadvantages of big data that are included in the banking sector are stated below:
1. Difficult to control the siloed data: The data in the banking sectors are wide and
diverse and are integrated in different places or departments. It is not possible to profile a
particular customer based only in terms of the investment behaviour such as the account, the
loans taken by the customers or similar instances (Corbae and D'Erasmo 2019). The data may
spread over large branches and can be spend on different departments of banks. The big data
needs to accumulate all such data in a particular space and provide intelligence accordingly
2. Upgrade the Legacy infrastructure: Most of the solutions in banking sector are always
not equipped for handling the constant data influx even if the data are moved to the cloud
solutions. Handling of constant data is a pre-requisite of big data solution. Implementation of big
data mainly requires complete revamp to the existing solutions of bank along with a big data
company. All the legacy infrastructure of the bank should be upgraded before integrating the
capabilities of big data. Big data is not very easy to implement as all the systems needs to be
changed constantly that are deployed.
3. Correct Implementation of dedicated resource with big data company: The banks
usually do not have expert who can handle big data as well as can analyze the partnerships with
the firms that specializes to design, deploy as well as develop the solution of big data. The
implementation of the software should be done correctly with correct choice of correct company.
4. Big Data is not Strategic Asset: The managers of a bank and the executives at the top
level often neglect the need to depend on the big data more compared to the human decisions.
The executives or the non-technical mangers mostly depend on the human decisions instead of
2.6 Pitfalls of Big Data in Banking Sector
The disadvantages of big data that are included in the banking sector are stated below:
1. Difficult to control the siloed data: The data in the banking sectors are wide and
diverse and are integrated in different places or departments. It is not possible to profile a
particular customer based only in terms of the investment behaviour such as the account, the
loans taken by the customers or similar instances (Corbae and D'Erasmo 2019). The data may
spread over large branches and can be spend on different departments of banks. The big data
needs to accumulate all such data in a particular space and provide intelligence accordingly
2. Upgrade the Legacy infrastructure: Most of the solutions in banking sector are always
not equipped for handling the constant data influx even if the data are moved to the cloud
solutions. Handling of constant data is a pre-requisite of big data solution. Implementation of big
data mainly requires complete revamp to the existing solutions of bank along with a big data
company. All the legacy infrastructure of the bank should be upgraded before integrating the
capabilities of big data. Big data is not very easy to implement as all the systems needs to be
changed constantly that are deployed.
3. Correct Implementation of dedicated resource with big data company: The banks
usually do not have expert who can handle big data as well as can analyze the partnerships with
the firms that specializes to design, deploy as well as develop the solution of big data. The
implementation of the software should be done correctly with correct choice of correct company.
4. Big Data is not Strategic Asset: The managers of a bank and the executives at the top
level often neglect the need to depend on the big data more compared to the human decisions.
The executives or the non-technical mangers mostly depend on the human decisions instead of
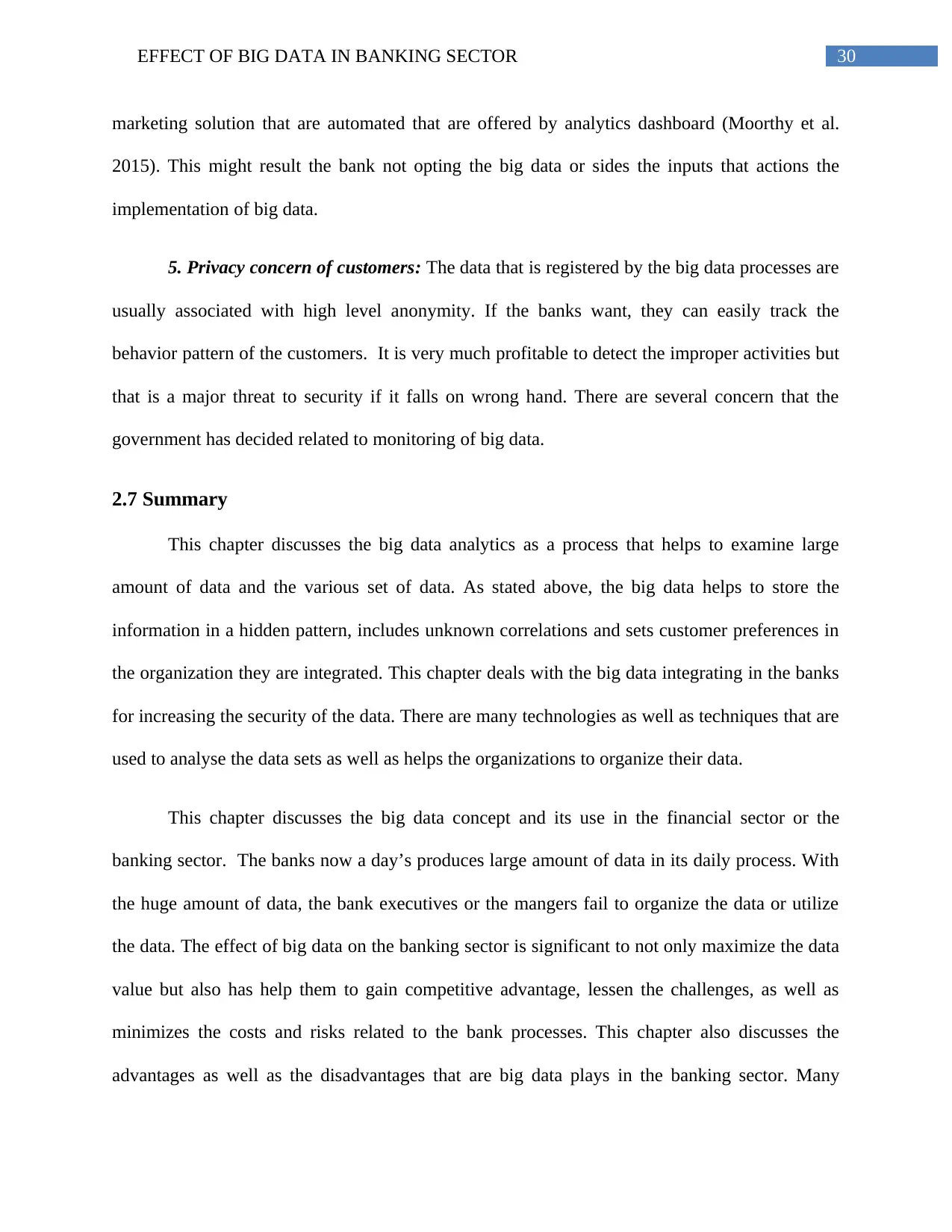
30EFFECT OF BIG DATA IN BANKING SECTOR
marketing solution that are automated that are offered by analytics dashboard (Moorthy et al.
2015). This might result the bank not opting the big data or sides the inputs that actions the
implementation of big data.
5. Privacy concern of customers: The data that is registered by the big data processes are
usually associated with high level anonymity. If the banks want, they can easily track the
behavior pattern of the customers. It is very much profitable to detect the improper activities but
that is a major threat to security if it falls on wrong hand. There are several concern that the
government has decided related to monitoring of big data.
2.7 Summary
This chapter discusses the big data analytics as a process that helps to examine large
amount of data and the various set of data. As stated above, the big data helps to store the
information in a hidden pattern, includes unknown correlations and sets customer preferences in
the organization they are integrated. This chapter deals with the big data integrating in the banks
for increasing the security of the data. There are many technologies as well as techniques that are
used to analyse the data sets as well as helps the organizations to organize their data.
This chapter discusses the big data concept and its use in the financial sector or the
banking sector. The banks now a day’s produces large amount of data in its daily process. With
the huge amount of data, the bank executives or the mangers fail to organize the data or utilize
the data. The effect of big data on the banking sector is significant to not only maximize the data
value but also has help them to gain competitive advantage, lessen the challenges, as well as
minimizes the costs and risks related to the bank processes. This chapter also discusses the
advantages as well as the disadvantages that are big data plays in the banking sector. Many
marketing solution that are automated that are offered by analytics dashboard (Moorthy et al.
2015). This might result the bank not opting the big data or sides the inputs that actions the
implementation of big data.
5. Privacy concern of customers: The data that is registered by the big data processes are
usually associated with high level anonymity. If the banks want, they can easily track the
behavior pattern of the customers. It is very much profitable to detect the improper activities but
that is a major threat to security if it falls on wrong hand. There are several concern that the
government has decided related to monitoring of big data.
2.7 Summary
This chapter discusses the big data analytics as a process that helps to examine large
amount of data and the various set of data. As stated above, the big data helps to store the
information in a hidden pattern, includes unknown correlations and sets customer preferences in
the organization they are integrated. This chapter deals with the big data integrating in the banks
for increasing the security of the data. There are many technologies as well as techniques that are
used to analyse the data sets as well as helps the organizations to organize their data.
This chapter discusses the big data concept and its use in the financial sector or the
banking sector. The banks now a day’s produces large amount of data in its daily process. With
the huge amount of data, the bank executives or the mangers fail to organize the data or utilize
the data. The effect of big data on the banking sector is significant to not only maximize the data
value but also has help them to gain competitive advantage, lessen the challenges, as well as
minimizes the costs and risks related to the bank processes. This chapter also discusses the
advantages as well as the disadvantages that are big data plays in the banking sector. Many
Secure Best Marks with AI Grader
Need help grading? Try our AI Grader for instant feedback on your assignments.
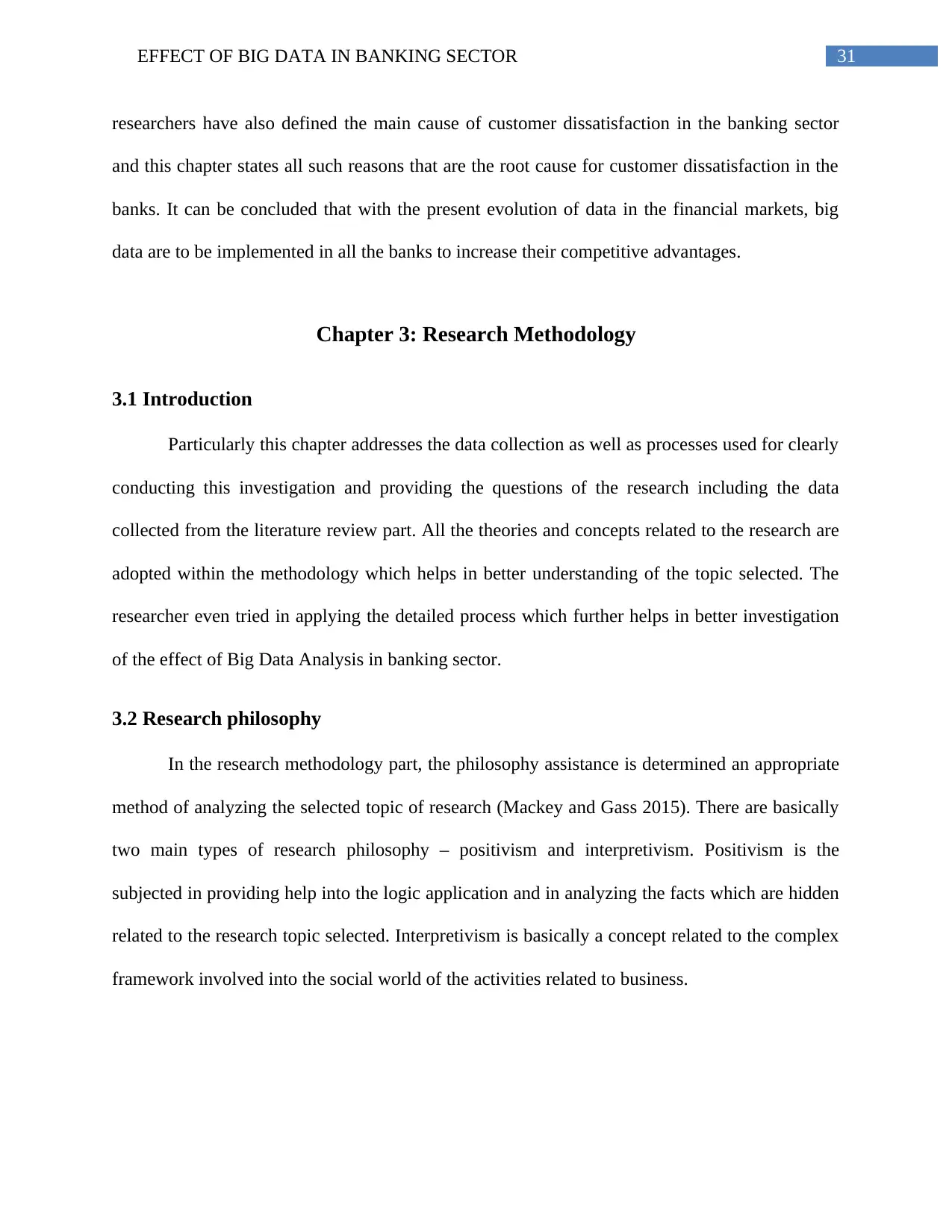
31EFFECT OF BIG DATA IN BANKING SECTOR
researchers have also defined the main cause of customer dissatisfaction in the banking sector
and this chapter states all such reasons that are the root cause for customer dissatisfaction in the
banks. It can be concluded that with the present evolution of data in the financial markets, big
data are to be implemented in all the banks to increase their competitive advantages.
Chapter 3: Research Methodology
3.1 Introduction
Particularly this chapter addresses the data collection as well as processes used for clearly
conducting this investigation and providing the questions of the research including the data
collected from the literature review part. All the theories and concepts related to the research are
adopted within the methodology which helps in better understanding of the topic selected. The
researcher even tried in applying the detailed process which further helps in better investigation
of the effect of Big Data Analysis in banking sector.
3.2 Research philosophy
In the research methodology part, the philosophy assistance is determined an appropriate
method of analyzing the selected topic of research (Mackey and Gass 2015). There are basically
two main types of research philosophy – positivism and interpretivism. Positivism is the
subjected in providing help into the logic application and in analyzing the facts which are hidden
related to the research topic selected. Interpretivism is basically a concept related to the complex
framework involved into the social world of the activities related to business.
researchers have also defined the main cause of customer dissatisfaction in the banking sector
and this chapter states all such reasons that are the root cause for customer dissatisfaction in the
banks. It can be concluded that with the present evolution of data in the financial markets, big
data are to be implemented in all the banks to increase their competitive advantages.
Chapter 3: Research Methodology
3.1 Introduction
Particularly this chapter addresses the data collection as well as processes used for clearly
conducting this investigation and providing the questions of the research including the data
collected from the literature review part. All the theories and concepts related to the research are
adopted within the methodology which helps in better understanding of the topic selected. The
researcher even tried in applying the detailed process which further helps in better investigation
of the effect of Big Data Analysis in banking sector.
3.2 Research philosophy
In the research methodology part, the philosophy assistance is determined an appropriate
method of analyzing the selected topic of research (Mackey and Gass 2015). There are basically
two main types of research philosophy – positivism and interpretivism. Positivism is the
subjected in providing help into the logic application and in analyzing the facts which are hidden
related to the research topic selected. Interpretivism is basically a concept related to the complex
framework involved into the social world of the activities related to business.
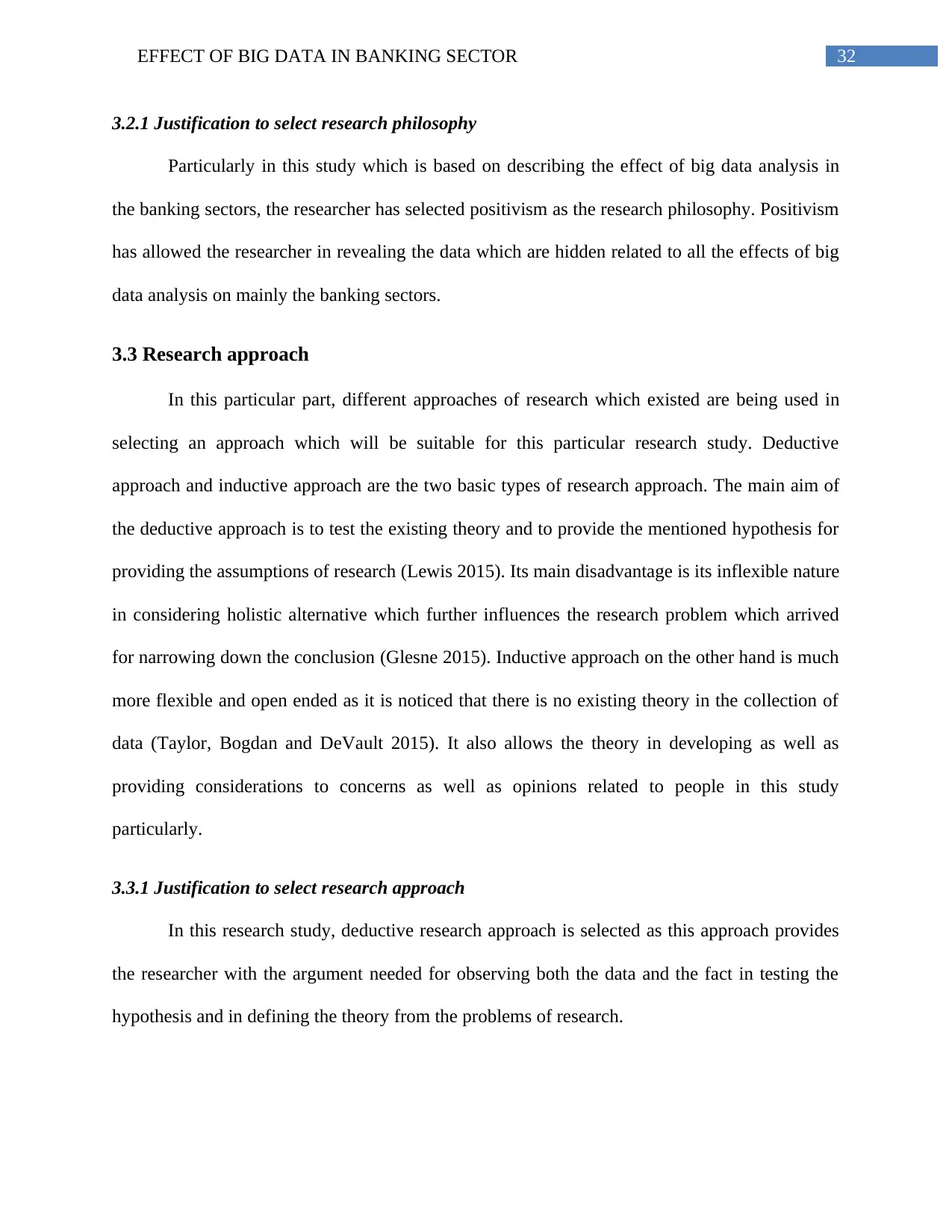
32EFFECT OF BIG DATA IN BANKING SECTOR
3.2.1 Justification to select research philosophy
Particularly in this study which is based on describing the effect of big data analysis in
the banking sectors, the researcher has selected positivism as the research philosophy. Positivism
has allowed the researcher in revealing the data which are hidden related to all the effects of big
data analysis on mainly the banking sectors.
3.3 Research approach
In this particular part, different approaches of research which existed are being used in
selecting an approach which will be suitable for this particular research study. Deductive
approach and inductive approach are the two basic types of research approach. The main aim of
the deductive approach is to test the existing theory and to provide the mentioned hypothesis for
providing the assumptions of research (Lewis 2015). Its main disadvantage is its inflexible nature
in considering holistic alternative which further influences the research problem which arrived
for narrowing down the conclusion (Glesne 2015). Inductive approach on the other hand is much
more flexible and open ended as it is noticed that there is no existing theory in the collection of
data (Taylor, Bogdan and DeVault 2015). It also allows the theory in developing as well as
providing considerations to concerns as well as opinions related to people in this study
particularly.
3.3.1 Justification to select research approach
In this research study, deductive research approach is selected as this approach provides
the researcher with the argument needed for observing both the data and the fact in testing the
hypothesis and in defining the theory from the problems of research.
3.2.1 Justification to select research philosophy
Particularly in this study which is based on describing the effect of big data analysis in
the banking sectors, the researcher has selected positivism as the research philosophy. Positivism
has allowed the researcher in revealing the data which are hidden related to all the effects of big
data analysis on mainly the banking sectors.
3.3 Research approach
In this particular part, different approaches of research which existed are being used in
selecting an approach which will be suitable for this particular research study. Deductive
approach and inductive approach are the two basic types of research approach. The main aim of
the deductive approach is to test the existing theory and to provide the mentioned hypothesis for
providing the assumptions of research (Lewis 2015). Its main disadvantage is its inflexible nature
in considering holistic alternative which further influences the research problem which arrived
for narrowing down the conclusion (Glesne 2015). Inductive approach on the other hand is much
more flexible and open ended as it is noticed that there is no existing theory in the collection of
data (Taylor, Bogdan and DeVault 2015). It also allows the theory in developing as well as
providing considerations to concerns as well as opinions related to people in this study
particularly.
3.3.1 Justification to select research approach
In this research study, deductive research approach is selected as this approach provides
the researcher with the argument needed for observing both the data and the fact in testing the
hypothesis and in defining the theory from the problems of research.
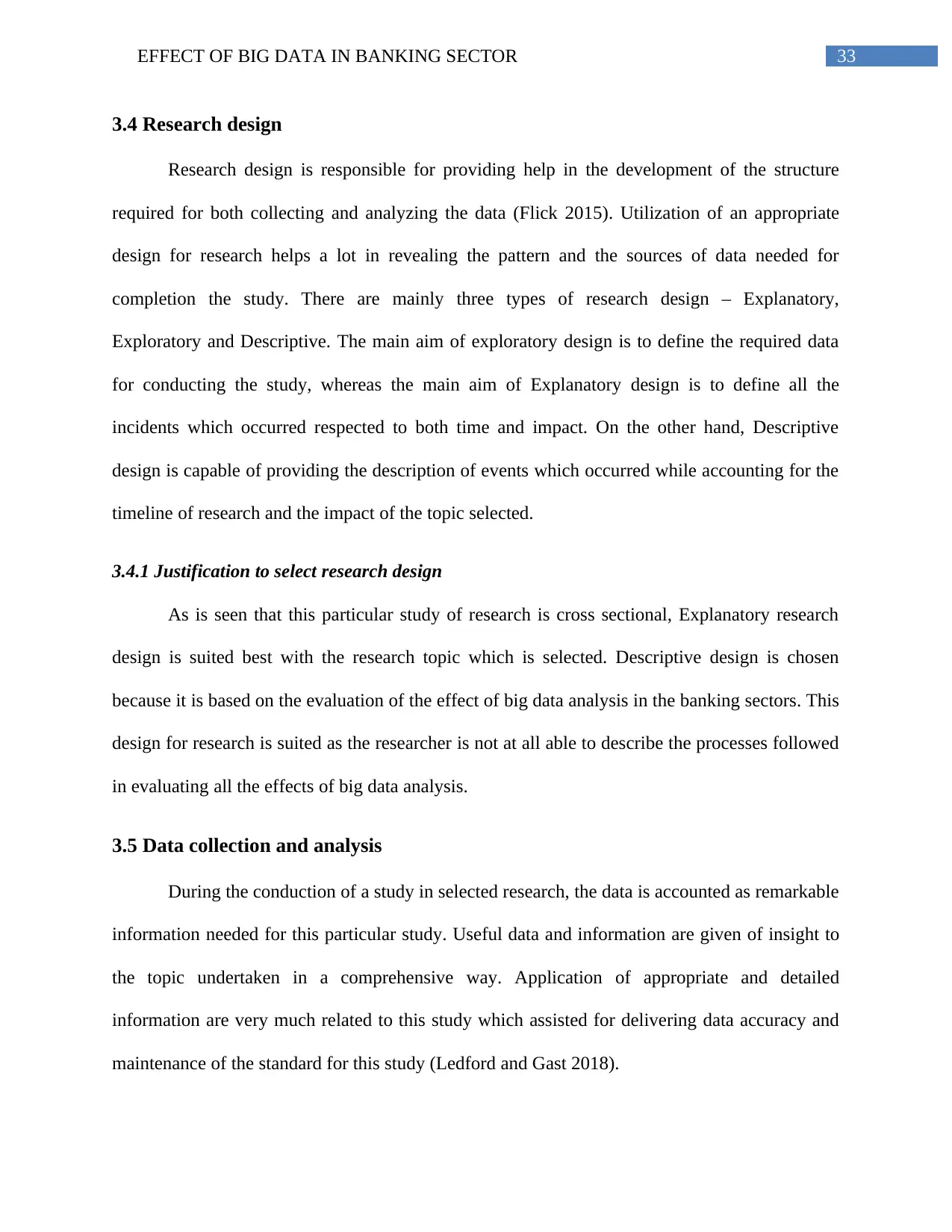
33EFFECT OF BIG DATA IN BANKING SECTOR
3.4 Research design
Research design is responsible for providing help in the development of the structure
required for both collecting and analyzing the data (Flick 2015). Utilization of an appropriate
design for research helps a lot in revealing the pattern and the sources of data needed for
completion the study. There are mainly three types of research design – Explanatory,
Exploratory and Descriptive. The main aim of exploratory design is to define the required data
for conducting the study, whereas the main aim of Explanatory design is to define all the
incidents which occurred respected to both time and impact. On the other hand, Descriptive
design is capable of providing the description of events which occurred while accounting for the
timeline of research and the impact of the topic selected.
3.4.1 Justification to select research design
As is seen that this particular study of research is cross sectional, Explanatory research
design is suited best with the research topic which is selected. Descriptive design is chosen
because it is based on the evaluation of the effect of big data analysis in the banking sectors. This
design for research is suited as the researcher is not at all able to describe the processes followed
in evaluating all the effects of big data analysis.
3.5 Data collection and analysis
During the conduction of a study in selected research, the data is accounted as remarkable
information needed for this particular study. Useful data and information are given of insight to
the topic undertaken in a comprehensive way. Application of appropriate and detailed
information are very much related to this study which assisted for delivering data accuracy and
maintenance of the standard for this study (Ledford and Gast 2018).
3.4 Research design
Research design is responsible for providing help in the development of the structure
required for both collecting and analyzing the data (Flick 2015). Utilization of an appropriate
design for research helps a lot in revealing the pattern and the sources of data needed for
completion the study. There are mainly three types of research design – Explanatory,
Exploratory and Descriptive. The main aim of exploratory design is to define the required data
for conducting the study, whereas the main aim of Explanatory design is to define all the
incidents which occurred respected to both time and impact. On the other hand, Descriptive
design is capable of providing the description of events which occurred while accounting for the
timeline of research and the impact of the topic selected.
3.4.1 Justification to select research design
As is seen that this particular study of research is cross sectional, Explanatory research
design is suited best with the research topic which is selected. Descriptive design is chosen
because it is based on the evaluation of the effect of big data analysis in the banking sectors. This
design for research is suited as the researcher is not at all able to describe the processes followed
in evaluating all the effects of big data analysis.
3.5 Data collection and analysis
During the conduction of a study in selected research, the data is accounted as remarkable
information needed for this particular study. Useful data and information are given of insight to
the topic undertaken in a comprehensive way. Application of appropriate and detailed
information are very much related to this study which assisted for delivering data accuracy and
maintenance of the standard for this study (Ledford and Gast 2018).
Paraphrase This Document
Need a fresh take? Get an instant paraphrase of this document with our AI Paraphraser
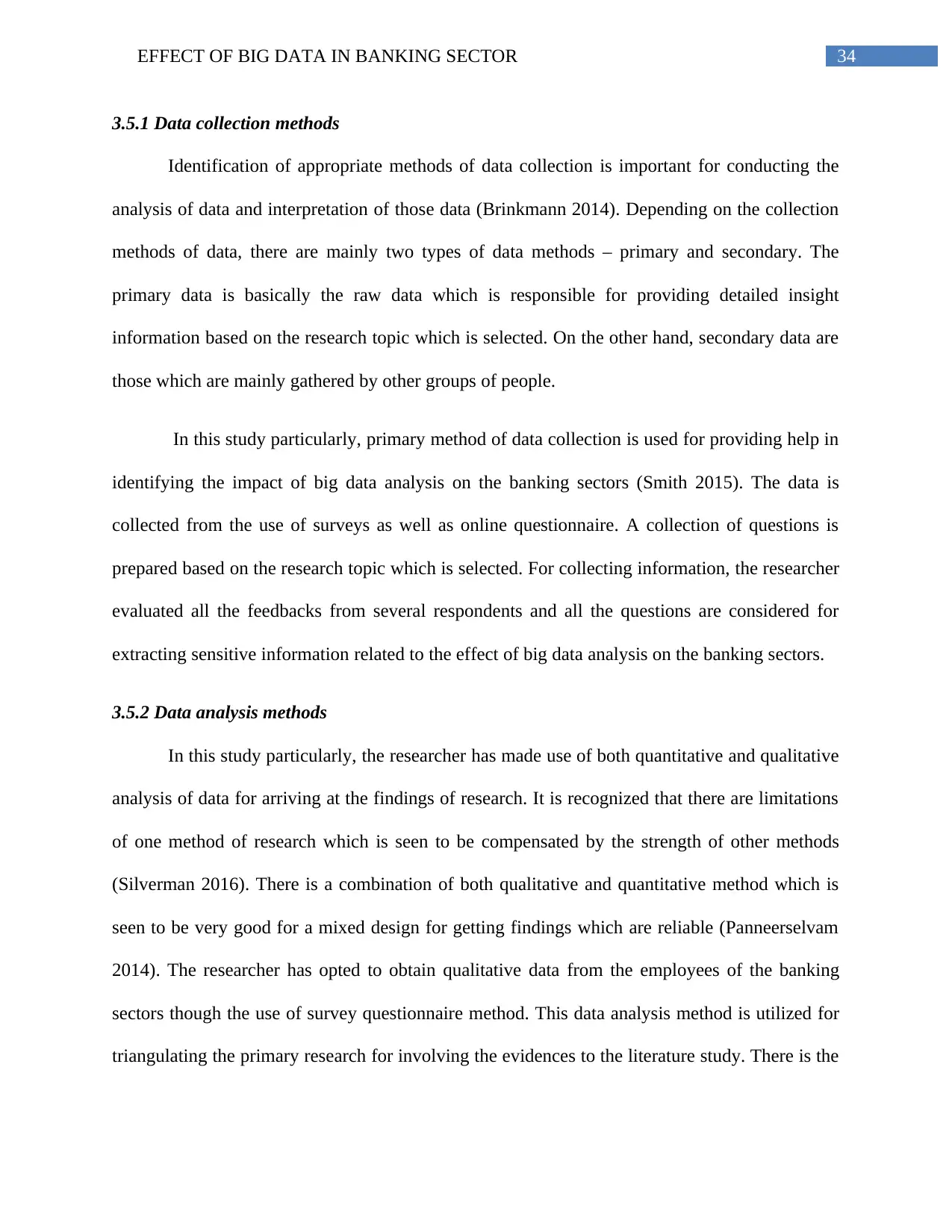
34EFFECT OF BIG DATA IN BANKING SECTOR
3.5.1 Data collection methods
Identification of appropriate methods of data collection is important for conducting the
analysis of data and interpretation of those data (Brinkmann 2014). Depending on the collection
methods of data, there are mainly two types of data methods – primary and secondary. The
primary data is basically the raw data which is responsible for providing detailed insight
information based on the research topic which is selected. On the other hand, secondary data are
those which are mainly gathered by other groups of people.
In this study particularly, primary method of data collection is used for providing help in
identifying the impact of big data analysis on the banking sectors (Smith 2015). The data is
collected from the use of surveys as well as online questionnaire. A collection of questions is
prepared based on the research topic which is selected. For collecting information, the researcher
evaluated all the feedbacks from several respondents and all the questions are considered for
extracting sensitive information related to the effect of big data analysis on the banking sectors.
3.5.2 Data analysis methods
In this study particularly, the researcher has made use of both quantitative and qualitative
analysis of data for arriving at the findings of research. It is recognized that there are limitations
of one method of research which is seen to be compensated by the strength of other methods
(Silverman 2016). There is a combination of both qualitative and quantitative method which is
seen to be very good for a mixed design for getting findings which are reliable (Panneerselvam
2014). The researcher has opted to obtain qualitative data from the employees of the banking
sectors though the use of survey questionnaire method. This data analysis method is utilized for
triangulating the primary research for involving the evidences to the literature study. There is the
3.5.1 Data collection methods
Identification of appropriate methods of data collection is important for conducting the
analysis of data and interpretation of those data (Brinkmann 2014). Depending on the collection
methods of data, there are mainly two types of data methods – primary and secondary. The
primary data is basically the raw data which is responsible for providing detailed insight
information based on the research topic which is selected. On the other hand, secondary data are
those which are mainly gathered by other groups of people.
In this study particularly, primary method of data collection is used for providing help in
identifying the impact of big data analysis on the banking sectors (Smith 2015). The data is
collected from the use of surveys as well as online questionnaire. A collection of questions is
prepared based on the research topic which is selected. For collecting information, the researcher
evaluated all the feedbacks from several respondents and all the questions are considered for
extracting sensitive information related to the effect of big data analysis on the banking sectors.
3.5.2 Data analysis methods
In this study particularly, the researcher has made use of both quantitative and qualitative
analysis of data for arriving at the findings of research. It is recognized that there are limitations
of one method of research which is seen to be compensated by the strength of other methods
(Silverman 2016). There is a combination of both qualitative and quantitative method which is
seen to be very good for a mixed design for getting findings which are reliable (Panneerselvam
2014). The researcher has opted to obtain qualitative data from the employees of the banking
sectors though the use of survey questionnaire method. This data analysis method is utilized for
triangulating the primary research for involving the evidences to the literature study. There is the
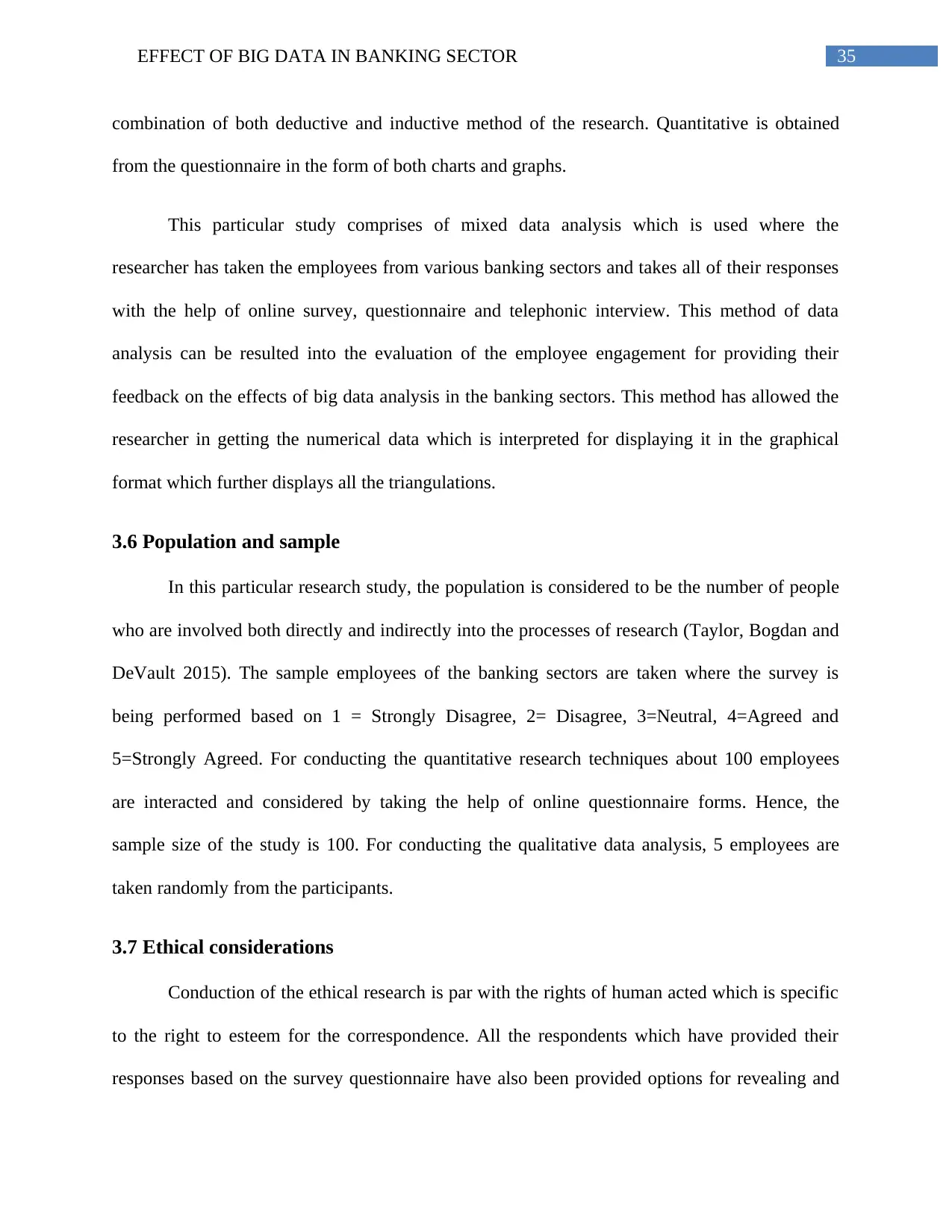
35EFFECT OF BIG DATA IN BANKING SECTOR
combination of both deductive and inductive method of the research. Quantitative is obtained
from the questionnaire in the form of both charts and graphs.
This particular study comprises of mixed data analysis which is used where the
researcher has taken the employees from various banking sectors and takes all of their responses
with the help of online survey, questionnaire and telephonic interview. This method of data
analysis can be resulted into the evaluation of the employee engagement for providing their
feedback on the effects of big data analysis in the banking sectors. This method has allowed the
researcher in getting the numerical data which is interpreted for displaying it in the graphical
format which further displays all the triangulations.
3.6 Population and sample
In this particular research study, the population is considered to be the number of people
who are involved both directly and indirectly into the processes of research (Taylor, Bogdan and
DeVault 2015). The sample employees of the banking sectors are taken where the survey is
being performed based on 1 = Strongly Disagree, 2= Disagree, 3=Neutral, 4=Agreed and
5=Strongly Agreed. For conducting the quantitative research techniques about 100 employees
are interacted and considered by taking the help of online questionnaire forms. Hence, the
sample size of the study is 100. For conducting the qualitative data analysis, 5 employees are
taken randomly from the participants.
3.7 Ethical considerations
Conduction of the ethical research is par with the rights of human acted which is specific
to the right to esteem for the correspondence. All the respondents which have provided their
responses based on the survey questionnaire have also been provided options for revealing and
combination of both deductive and inductive method of the research. Quantitative is obtained
from the questionnaire in the form of both charts and graphs.
This particular study comprises of mixed data analysis which is used where the
researcher has taken the employees from various banking sectors and takes all of their responses
with the help of online survey, questionnaire and telephonic interview. This method of data
analysis can be resulted into the evaluation of the employee engagement for providing their
feedback on the effects of big data analysis in the banking sectors. This method has allowed the
researcher in getting the numerical data which is interpreted for displaying it in the graphical
format which further displays all the triangulations.
3.6 Population and sample
In this particular research study, the population is considered to be the number of people
who are involved both directly and indirectly into the processes of research (Taylor, Bogdan and
DeVault 2015). The sample employees of the banking sectors are taken where the survey is
being performed based on 1 = Strongly Disagree, 2= Disagree, 3=Neutral, 4=Agreed and
5=Strongly Agreed. For conducting the quantitative research techniques about 100 employees
are interacted and considered by taking the help of online questionnaire forms. Hence, the
sample size of the study is 100. For conducting the qualitative data analysis, 5 employees are
taken randomly from the participants.
3.7 Ethical considerations
Conduction of the ethical research is par with the rights of human acted which is specific
to the right to esteem for the correspondence. All the respondents which have provided their
responses based on the survey questionnaire have also been provided options for revealing and
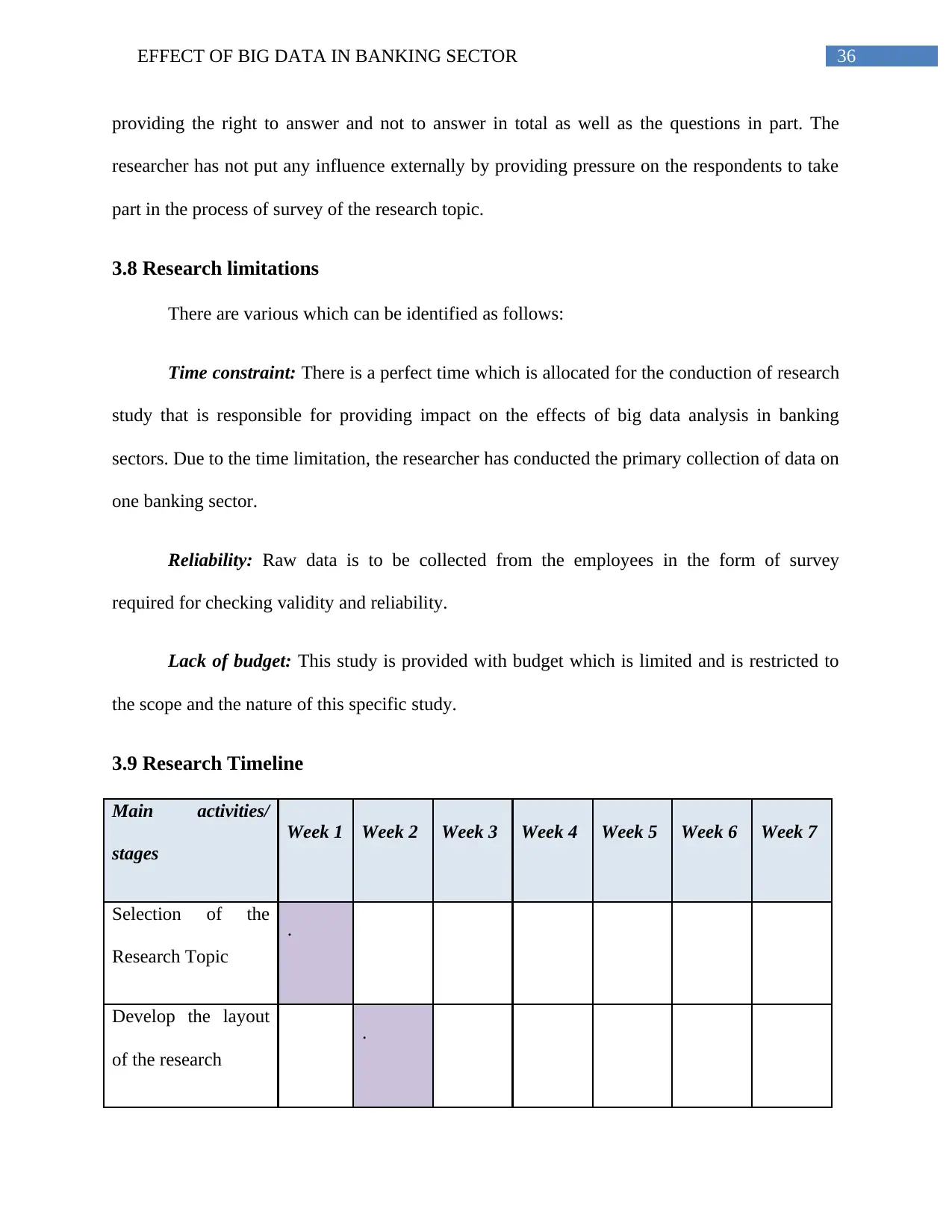
36EFFECT OF BIG DATA IN BANKING SECTOR
providing the right to answer and not to answer in total as well as the questions in part. The
researcher has not put any influence externally by providing pressure on the respondents to take
part in the process of survey of the research topic.
3.8 Research limitations
There are various which can be identified as follows:
Time constraint: There is a perfect time which is allocated for the conduction of research
study that is responsible for providing impact on the effects of big data analysis in banking
sectors. Due to the time limitation, the researcher has conducted the primary collection of data on
one banking sector.
Reliability: Raw data is to be collected from the employees in the form of survey
required for checking validity and reliability.
Lack of budget: This study is provided with budget which is limited and is restricted to
the scope and the nature of this specific study.
3.9 Research Timeline
Main activities/
stages
Week 1 Week 2 Week 3 Week 4 Week 5 Week 6 Week 7
Selection of the
Research Topic
·
Develop the layout
of the research
·
providing the right to answer and not to answer in total as well as the questions in part. The
researcher has not put any influence externally by providing pressure on the respondents to take
part in the process of survey of the research topic.
3.8 Research limitations
There are various which can be identified as follows:
Time constraint: There is a perfect time which is allocated for the conduction of research
study that is responsible for providing impact on the effects of big data analysis in banking
sectors. Due to the time limitation, the researcher has conducted the primary collection of data on
one banking sector.
Reliability: Raw data is to be collected from the employees in the form of survey
required for checking validity and reliability.
Lack of budget: This study is provided with budget which is limited and is restricted to
the scope and the nature of this specific study.
3.9 Research Timeline
Main activities/
stages
Week 1 Week 2 Week 3 Week 4 Week 5 Week 6 Week 7
Selection of the
Research Topic
·
Develop the layout
of the research
·
Secure Best Marks with AI Grader
Need help grading? Try our AI Grader for instant feedback on your assignments.
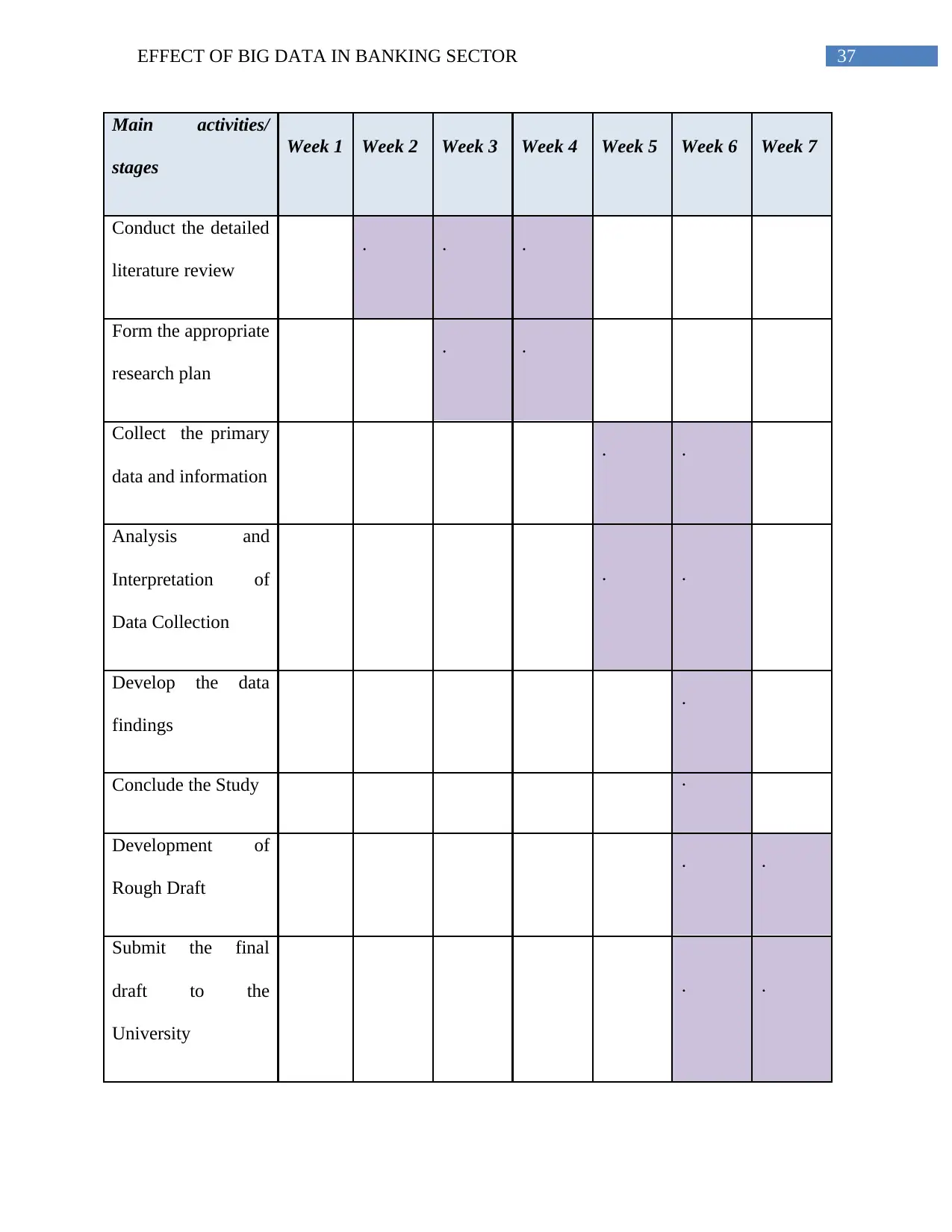
37EFFECT OF BIG DATA IN BANKING SECTOR
Main activities/
stages
Week 1 Week 2 Week 3 Week 4 Week 5 Week 6 Week 7
Conduct the detailed
literature review
· · ·
Form the appropriate
research plan
· ·
Collect the primary
data and information
· ·
Analysis and
Interpretation of
Data Collection
· ·
Develop the data
findings
·
Conclude the Study ·
Development of
Rough Draft
· ·
Submit the final
draft to the
University
· ·
Main activities/
stages
Week 1 Week 2 Week 3 Week 4 Week 5 Week 6 Week 7
Conduct the detailed
literature review
· · ·
Form the appropriate
research plan
· ·
Collect the primary
data and information
· ·
Analysis and
Interpretation of
Data Collection
· ·
Develop the data
findings
·
Conclude the Study ·
Development of
Rough Draft
· ·
Submit the final
draft to the
University
· ·
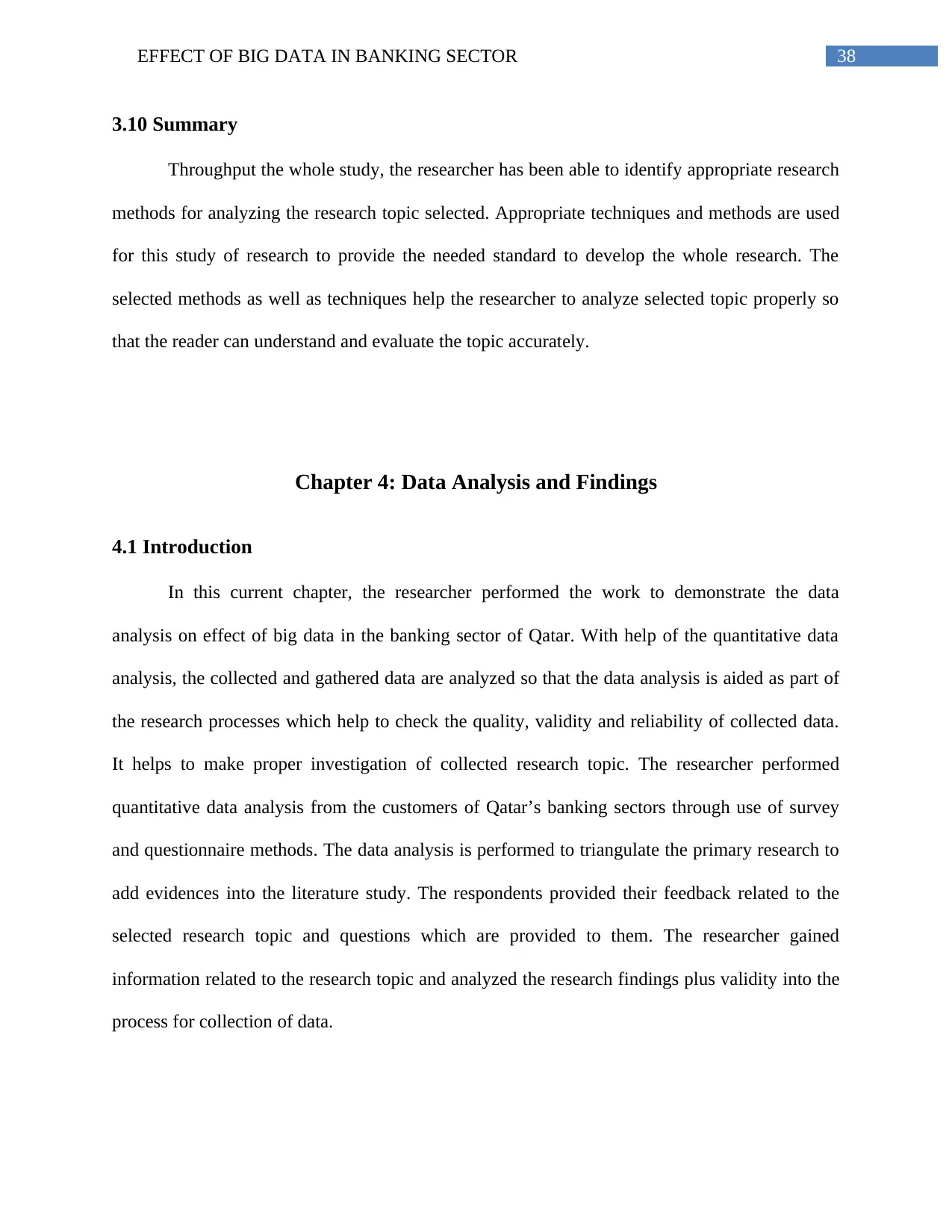
38EFFECT OF BIG DATA IN BANKING SECTOR
3.10 Summary
Throughput the whole study, the researcher has been able to identify appropriate research
methods for analyzing the research topic selected. Appropriate techniques and methods are used
for this study of research to provide the needed standard to develop the whole research. The
selected methods as well as techniques help the researcher to analyze selected topic properly so
that the reader can understand and evaluate the topic accurately.
Chapter 4: Data Analysis and Findings
4.1 Introduction
In this current chapter, the researcher performed the work to demonstrate the data
analysis on effect of big data in the banking sector of Qatar. With help of the quantitative data
analysis, the collected and gathered data are analyzed so that the data analysis is aided as part of
the research processes which help to check the quality, validity and reliability of collected data.
It helps to make proper investigation of collected research topic. The researcher performed
quantitative data analysis from the customers of Qatar’s banking sectors through use of survey
and questionnaire methods. The data analysis is performed to triangulate the primary research to
add evidences into the literature study. The respondents provided their feedback related to the
selected research topic and questions which are provided to them. The researcher gained
information related to the research topic and analyzed the research findings plus validity into the
process for collection of data.
3.10 Summary
Throughput the whole study, the researcher has been able to identify appropriate research
methods for analyzing the research topic selected. Appropriate techniques and methods are used
for this study of research to provide the needed standard to develop the whole research. The
selected methods as well as techniques help the researcher to analyze selected topic properly so
that the reader can understand and evaluate the topic accurately.
Chapter 4: Data Analysis and Findings
4.1 Introduction
In this current chapter, the researcher performed the work to demonstrate the data
analysis on effect of big data in the banking sector of Qatar. With help of the quantitative data
analysis, the collected and gathered data are analyzed so that the data analysis is aided as part of
the research processes which help to check the quality, validity and reliability of collected data.
It helps to make proper investigation of collected research topic. The researcher performed
quantitative data analysis from the customers of Qatar’s banking sectors through use of survey
and questionnaire methods. The data analysis is performed to triangulate the primary research to
add evidences into the literature study. The respondents provided their feedback related to the
selected research topic and questions which are provided to them. The researcher gained
information related to the research topic and analyzed the research findings plus validity into the
process for collection of data.
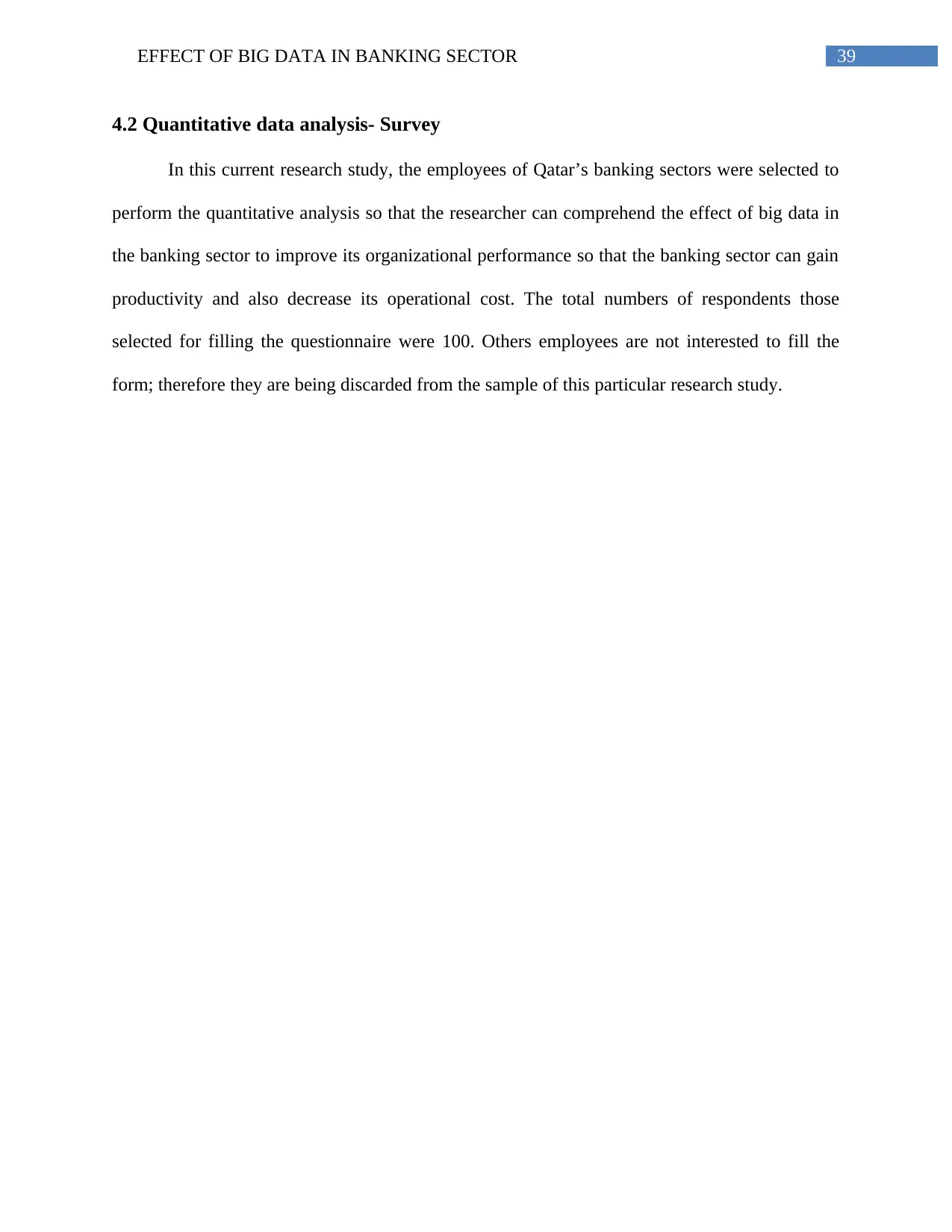
39EFFECT OF BIG DATA IN BANKING SECTOR
4.2 Quantitative data analysis- Survey
In this current research study, the employees of Qatar’s banking sectors were selected to
perform the quantitative analysis so that the researcher can comprehend the effect of big data in
the banking sector to improve its organizational performance so that the banking sector can gain
productivity and also decrease its operational cost. The total numbers of respondents those
selected for filling the questionnaire were 100. Others employees are not interested to fill the
form; therefore they are being discarded from the sample of this particular research study.
4.2 Quantitative data analysis- Survey
In this current research study, the employees of Qatar’s banking sectors were selected to
perform the quantitative analysis so that the researcher can comprehend the effect of big data in
the banking sector to improve its organizational performance so that the banking sector can gain
productivity and also decrease its operational cost. The total numbers of respondents those
selected for filling the questionnaire were 100. Others employees are not interested to fill the
form; therefore they are being discarded from the sample of this particular research study.
Paraphrase This Document
Need a fresh take? Get an instant paraphrase of this document with our AI Paraphraser
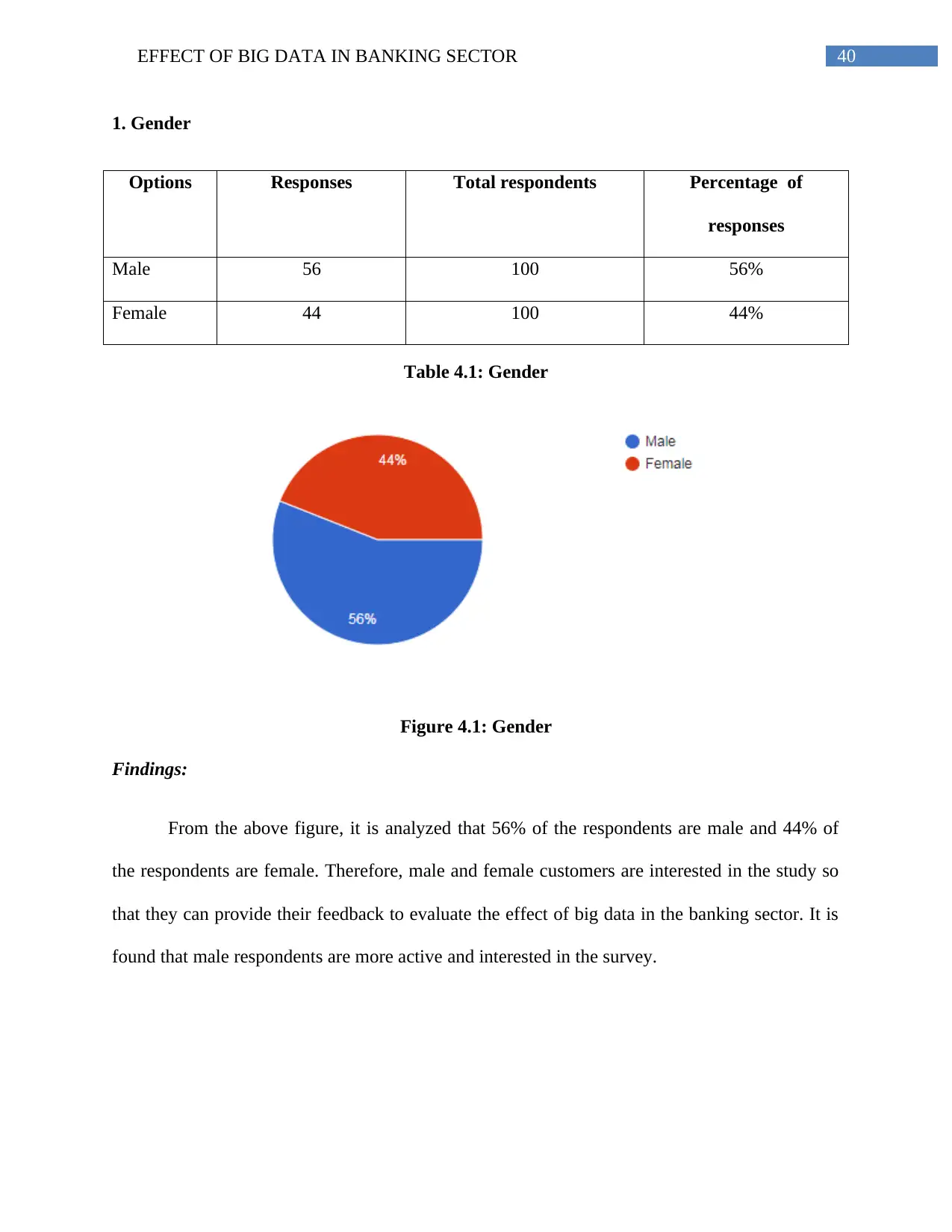
40EFFECT OF BIG DATA IN BANKING SECTOR
1. Gender
Options Responses Total respondents Percentage of
responses
Male 56 100 56%
Female 44 100 44%
Table 4.1: Gender
Figure 4.1: Gender
Findings:
From the above figure, it is analyzed that 56% of the respondents are male and 44% of
the respondents are female. Therefore, male and female customers are interested in the study so
that they can provide their feedback to evaluate the effect of big data in the banking sector. It is
found that male respondents are more active and interested in the survey.
1. Gender
Options Responses Total respondents Percentage of
responses
Male 56 100 56%
Female 44 100 44%
Table 4.1: Gender
Figure 4.1: Gender
Findings:
From the above figure, it is analyzed that 56% of the respondents are male and 44% of
the respondents are female. Therefore, male and female customers are interested in the study so
that they can provide their feedback to evaluate the effect of big data in the banking sector. It is
found that male respondents are more active and interested in the survey.
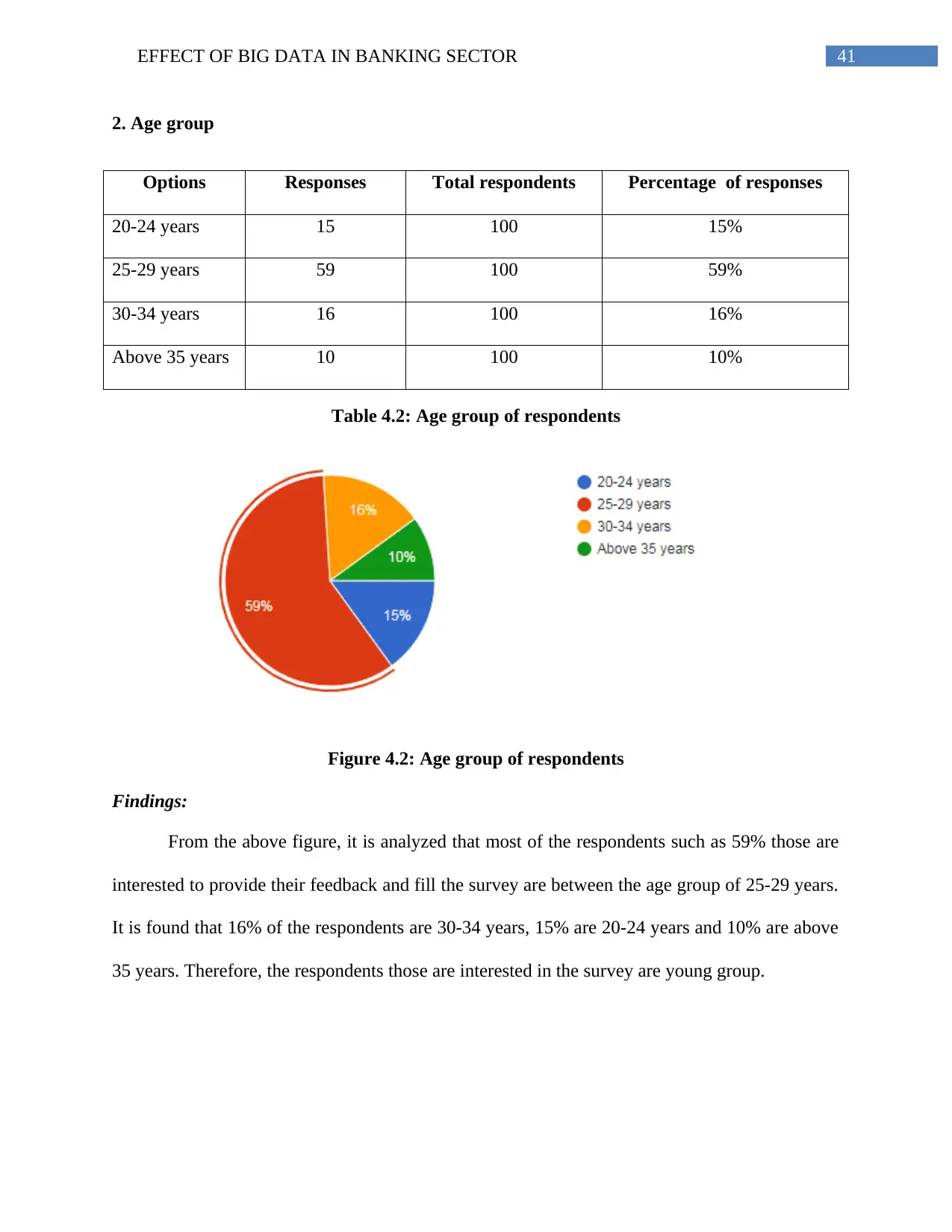
41EFFECT OF BIG DATA IN BANKING SECTOR
2. Age group
Options Responses Total respondents Percentage of responses
20-24 years 15 100 15%
25-29 years 59 100 59%
30-34 years 16 100 16%
Above 35 years 10 100 10%
Table 4.2: Age group of respondents
Figure 4.2: Age group of respondents
Findings:
From the above figure, it is analyzed that most of the respondents such as 59% those are
interested to provide their feedback and fill the survey are between the age group of 25-29 years.
It is found that 16% of the respondents are 30-34 years, 15% are 20-24 years and 10% are above
35 years. Therefore, the respondents those are interested in the survey are young group.
2. Age group
Options Responses Total respondents Percentage of responses
20-24 years 15 100 15%
25-29 years 59 100 59%
30-34 years 16 100 16%
Above 35 years 10 100 10%
Table 4.2: Age group of respondents
Figure 4.2: Age group of respondents
Findings:
From the above figure, it is analyzed that most of the respondents such as 59% those are
interested to provide their feedback and fill the survey are between the age group of 25-29 years.
It is found that 16% of the respondents are 30-34 years, 15% are 20-24 years and 10% are above
35 years. Therefore, the respondents those are interested in the survey are young group.
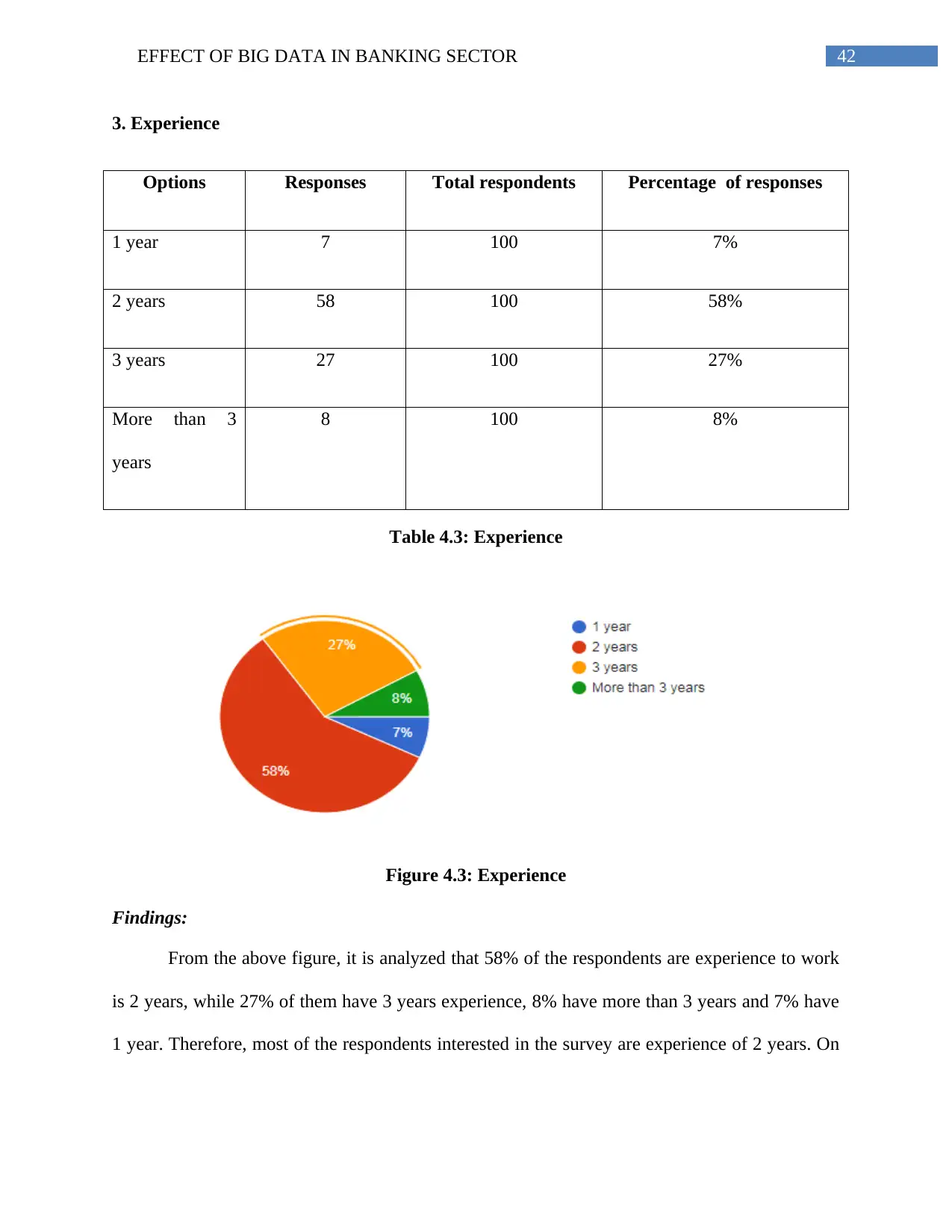
42EFFECT OF BIG DATA IN BANKING SECTOR
3. Experience
Options Responses Total respondents Percentage of responses
1 year 7 100 7%
2 years 58 100 58%
3 years 27 100 27%
More than 3
years
8 100 8%
Table 4.3: Experience
Figure 4.3: Experience
Findings:
From the above figure, it is analyzed that 58% of the respondents are experience to work
is 2 years, while 27% of them have 3 years experience, 8% have more than 3 years and 7% have
1 year. Therefore, most of the respondents interested in the survey are experience of 2 years. On
3. Experience
Options Responses Total respondents Percentage of responses
1 year 7 100 7%
2 years 58 100 58%
3 years 27 100 27%
More than 3
years
8 100 8%
Table 4.3: Experience
Figure 4.3: Experience
Findings:
From the above figure, it is analyzed that 58% of the respondents are experience to work
is 2 years, while 27% of them have 3 years experience, 8% have more than 3 years and 7% have
1 year. Therefore, most of the respondents interested in the survey are experience of 2 years. On
Secure Best Marks with AI Grader
Need help grading? Try our AI Grader for instant feedback on your assignments.
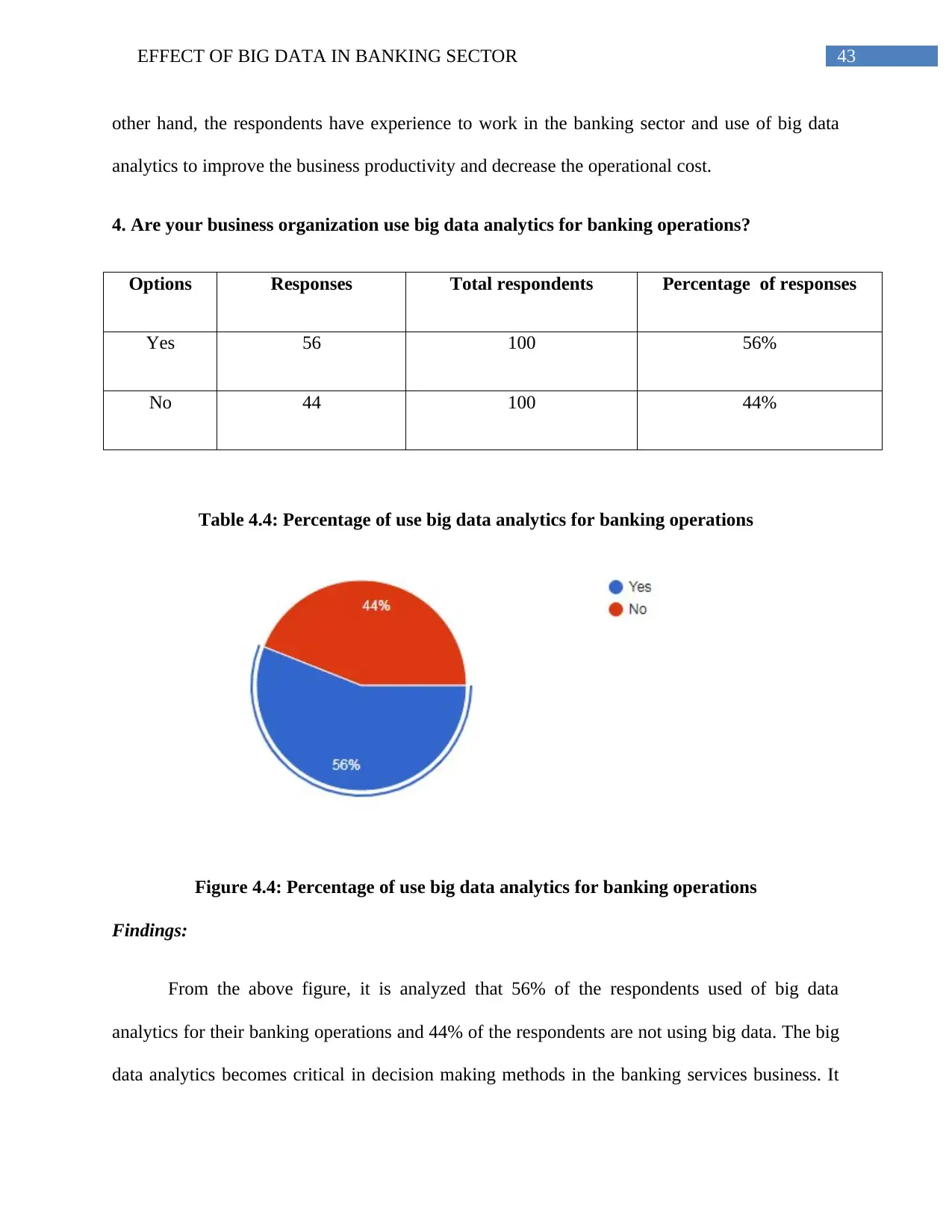
43EFFECT OF BIG DATA IN BANKING SECTOR
other hand, the respondents have experience to work in the banking sector and use of big data
analytics to improve the business productivity and decrease the operational cost.
4. Are your business organization use big data analytics for banking operations?
Options Responses Total respondents Percentage of responses
Yes 56 100 56%
No 44 100 44%
Table 4.4: Percentage of use big data analytics for banking operations
Figure 4.4: Percentage of use big data analytics for banking operations
Findings:
From the above figure, it is analyzed that 56% of the respondents used of big data
analytics for their banking operations and 44% of the respondents are not using big data. The big
data analytics becomes critical in decision making methods in the banking services business. It
other hand, the respondents have experience to work in the banking sector and use of big data
analytics to improve the business productivity and decrease the operational cost.
4. Are your business organization use big data analytics for banking operations?
Options Responses Total respondents Percentage of responses
Yes 56 100 56%
No 44 100 44%
Table 4.4: Percentage of use big data analytics for banking operations
Figure 4.4: Percentage of use big data analytics for banking operations
Findings:
From the above figure, it is analyzed that 56% of the respondents used of big data
analytics for their banking operations and 44% of the respondents are not using big data. The big
data analytics becomes critical in decision making methods in the banking services business. It
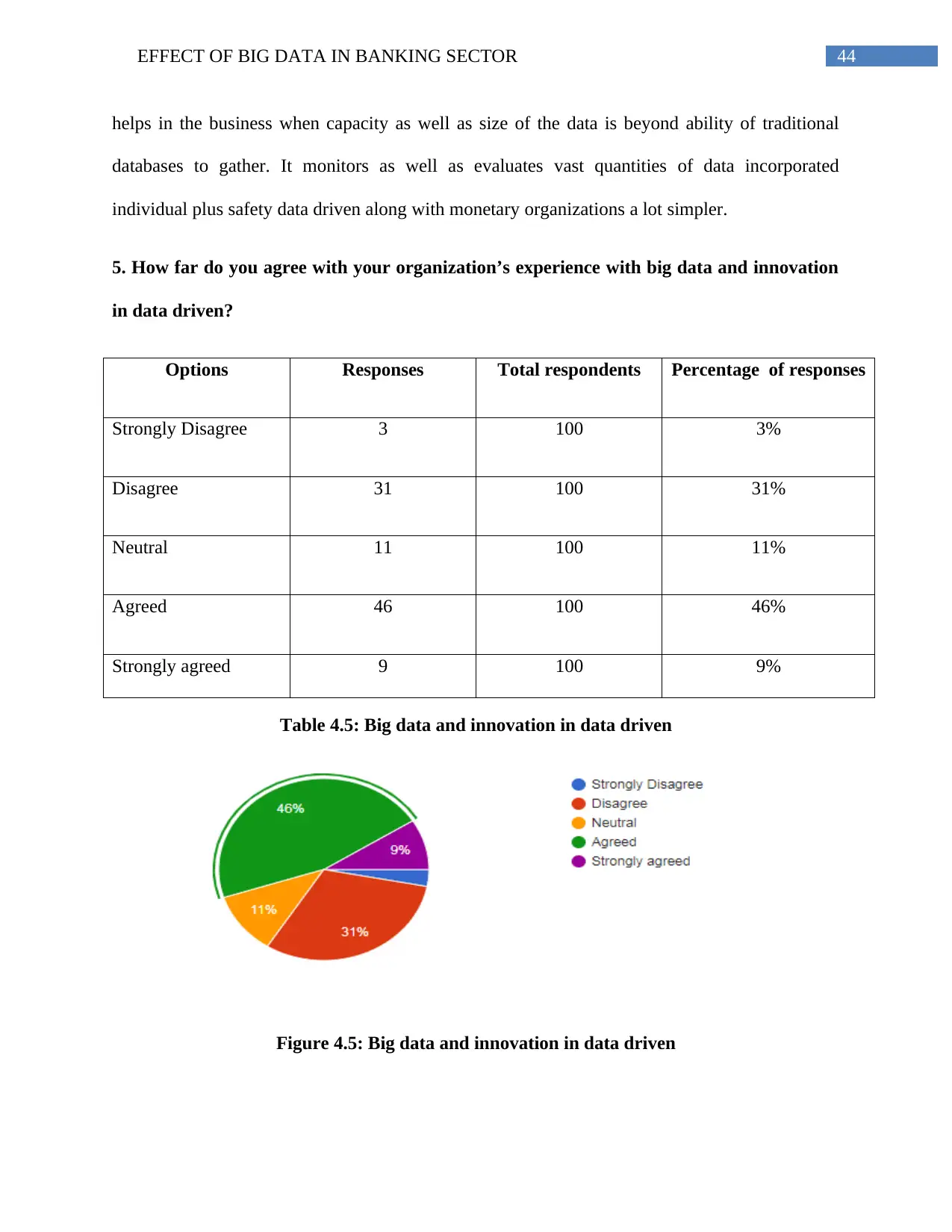
44EFFECT OF BIG DATA IN BANKING SECTOR
helps in the business when capacity as well as size of the data is beyond ability of traditional
databases to gather. It monitors as well as evaluates vast quantities of data incorporated
individual plus safety data driven along with monetary organizations a lot simpler.
5. How far do you agree with your organization’s experience with big data and innovation
in data driven?
Options Responses Total respondents Percentage of responses
Strongly Disagree 3 100 3%
Disagree 31 100 31%
Neutral 11 100 11%
Agreed 46 100 46%
Strongly agreed 9 100 9%
Table 4.5: Big data and innovation in data driven
Figure 4.5: Big data and innovation in data driven
helps in the business when capacity as well as size of the data is beyond ability of traditional
databases to gather. It monitors as well as evaluates vast quantities of data incorporated
individual plus safety data driven along with monetary organizations a lot simpler.
5. How far do you agree with your organization’s experience with big data and innovation
in data driven?
Options Responses Total respondents Percentage of responses
Strongly Disagree 3 100 3%
Disagree 31 100 31%
Neutral 11 100 11%
Agreed 46 100 46%
Strongly agreed 9 100 9%
Table 4.5: Big data and innovation in data driven
Figure 4.5: Big data and innovation in data driven
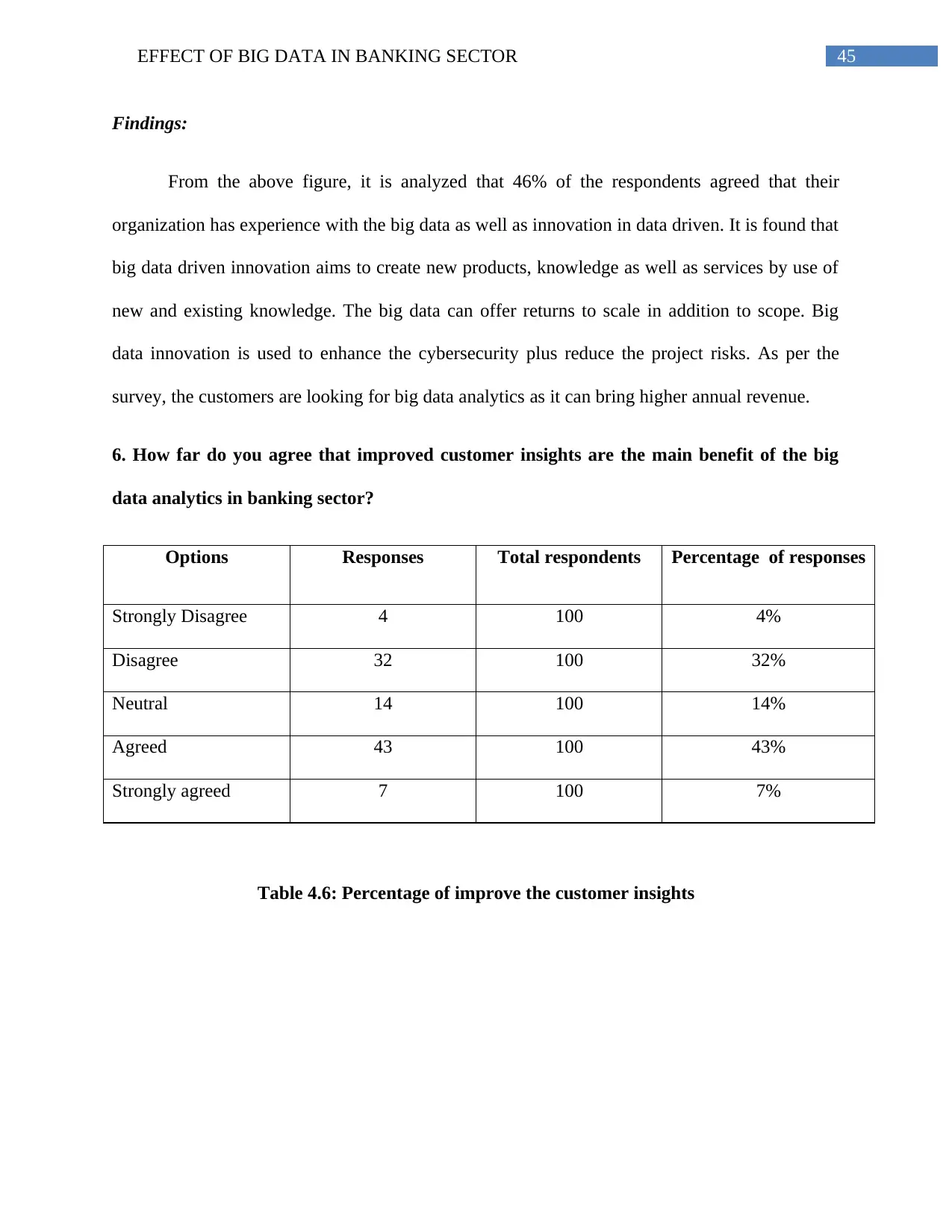
45EFFECT OF BIG DATA IN BANKING SECTOR
Findings:
From the above figure, it is analyzed that 46% of the respondents agreed that their
organization has experience with the big data as well as innovation in data driven. It is found that
big data driven innovation aims to create new products, knowledge as well as services by use of
new and existing knowledge. The big data can offer returns to scale in addition to scope. Big
data innovation is used to enhance the cybersecurity plus reduce the project risks. As per the
survey, the customers are looking for big data analytics as it can bring higher annual revenue.
6. How far do you agree that improved customer insights are the main benefit of the big
data analytics in banking sector?
Options Responses Total respondents Percentage of responses
Strongly Disagree 4 100 4%
Disagree 32 100 32%
Neutral 14 100 14%
Agreed 43 100 43%
Strongly agreed 7 100 7%
Table 4.6: Percentage of improve the customer insights
Findings:
From the above figure, it is analyzed that 46% of the respondents agreed that their
organization has experience with the big data as well as innovation in data driven. It is found that
big data driven innovation aims to create new products, knowledge as well as services by use of
new and existing knowledge. The big data can offer returns to scale in addition to scope. Big
data innovation is used to enhance the cybersecurity plus reduce the project risks. As per the
survey, the customers are looking for big data analytics as it can bring higher annual revenue.
6. How far do you agree that improved customer insights are the main benefit of the big
data analytics in banking sector?
Options Responses Total respondents Percentage of responses
Strongly Disagree 4 100 4%
Disagree 32 100 32%
Neutral 14 100 14%
Agreed 43 100 43%
Strongly agreed 7 100 7%
Table 4.6: Percentage of improve the customer insights
Paraphrase This Document
Need a fresh take? Get an instant paraphrase of this document with our AI Paraphraser
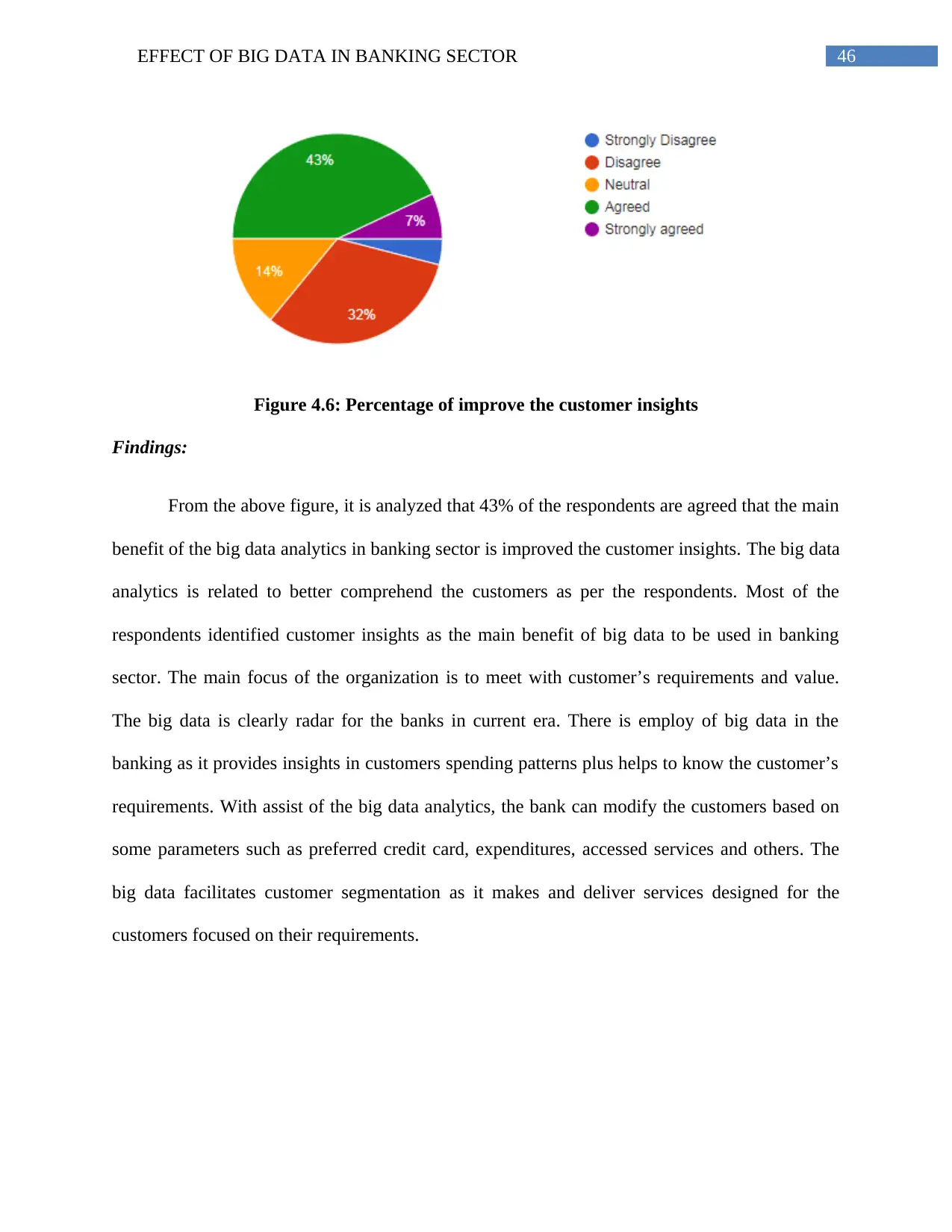
46EFFECT OF BIG DATA IN BANKING SECTOR
Figure 4.6: Percentage of improve the customer insights
Findings:
From the above figure, it is analyzed that 43% of the respondents are agreed that the main
benefit of the big data analytics in banking sector is improved the customer insights. The big data
analytics is related to better comprehend the customers as per the respondents. Most of the
respondents identified customer insights as the main benefit of big data to be used in banking
sector. The main focus of the organization is to meet with customer’s requirements and value.
The big data is clearly radar for the banks in current era. There is employ of big data in the
banking as it provides insights in customers spending patterns plus helps to know the customer’s
requirements. With assist of the big data analytics, the bank can modify the customers based on
some parameters such as preferred credit card, expenditures, accessed services and others. The
big data facilitates customer segmentation as it makes and deliver services designed for the
customers focused on their requirements.
Figure 4.6: Percentage of improve the customer insights
Findings:
From the above figure, it is analyzed that 43% of the respondents are agreed that the main
benefit of the big data analytics in banking sector is improved the customer insights. The big data
analytics is related to better comprehend the customers as per the respondents. Most of the
respondents identified customer insights as the main benefit of big data to be used in banking
sector. The main focus of the organization is to meet with customer’s requirements and value.
The big data is clearly radar for the banks in current era. There is employ of big data in the
banking as it provides insights in customers spending patterns plus helps to know the customer’s
requirements. With assist of the big data analytics, the bank can modify the customers based on
some parameters such as preferred credit card, expenditures, accessed services and others. The
big data facilitates customer segmentation as it makes and deliver services designed for the
customers focused on their requirements.
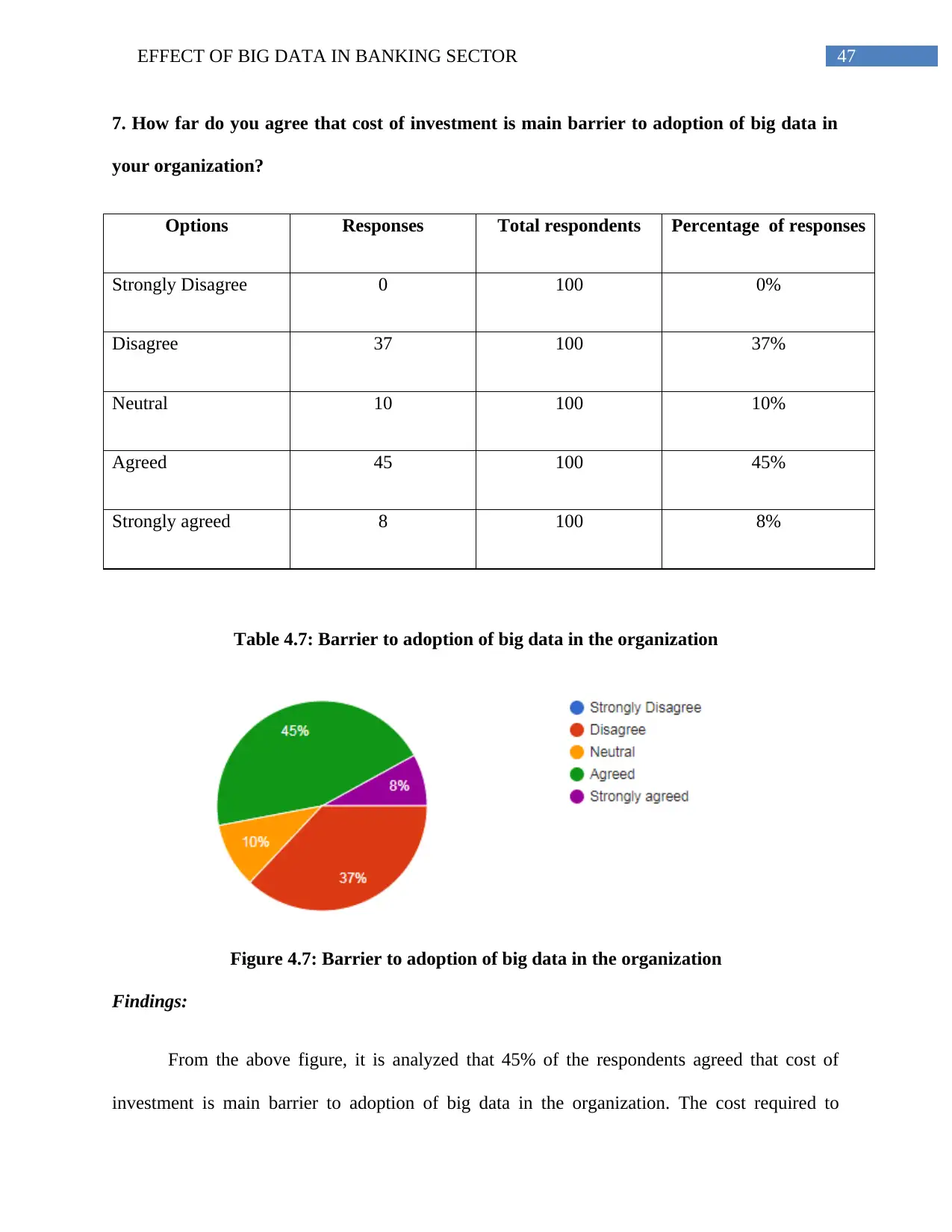
47EFFECT OF BIG DATA IN BANKING SECTOR
7. How far do you agree that cost of investment is main barrier to adoption of big data in
your organization?
Options Responses Total respondents Percentage of responses
Strongly Disagree 0 100 0%
Disagree 37 100 37%
Neutral 10 100 10%
Agreed 45 100 45%
Strongly agreed 8 100 8%
Table 4.7: Barrier to adoption of big data in the organization
Figure 4.7: Barrier to adoption of big data in the organization
Findings:
From the above figure, it is analyzed that 45% of the respondents agreed that cost of
investment is main barrier to adoption of big data in the organization. The cost required to
7. How far do you agree that cost of investment is main barrier to adoption of big data in
your organization?
Options Responses Total respondents Percentage of responses
Strongly Disagree 0 100 0%
Disagree 37 100 37%
Neutral 10 100 10%
Agreed 45 100 45%
Strongly agreed 8 100 8%
Table 4.7: Barrier to adoption of big data in the organization
Figure 4.7: Barrier to adoption of big data in the organization
Findings:
From the above figure, it is analyzed that 45% of the respondents agreed that cost of
investment is main barrier to adoption of big data in the organization. The cost required to
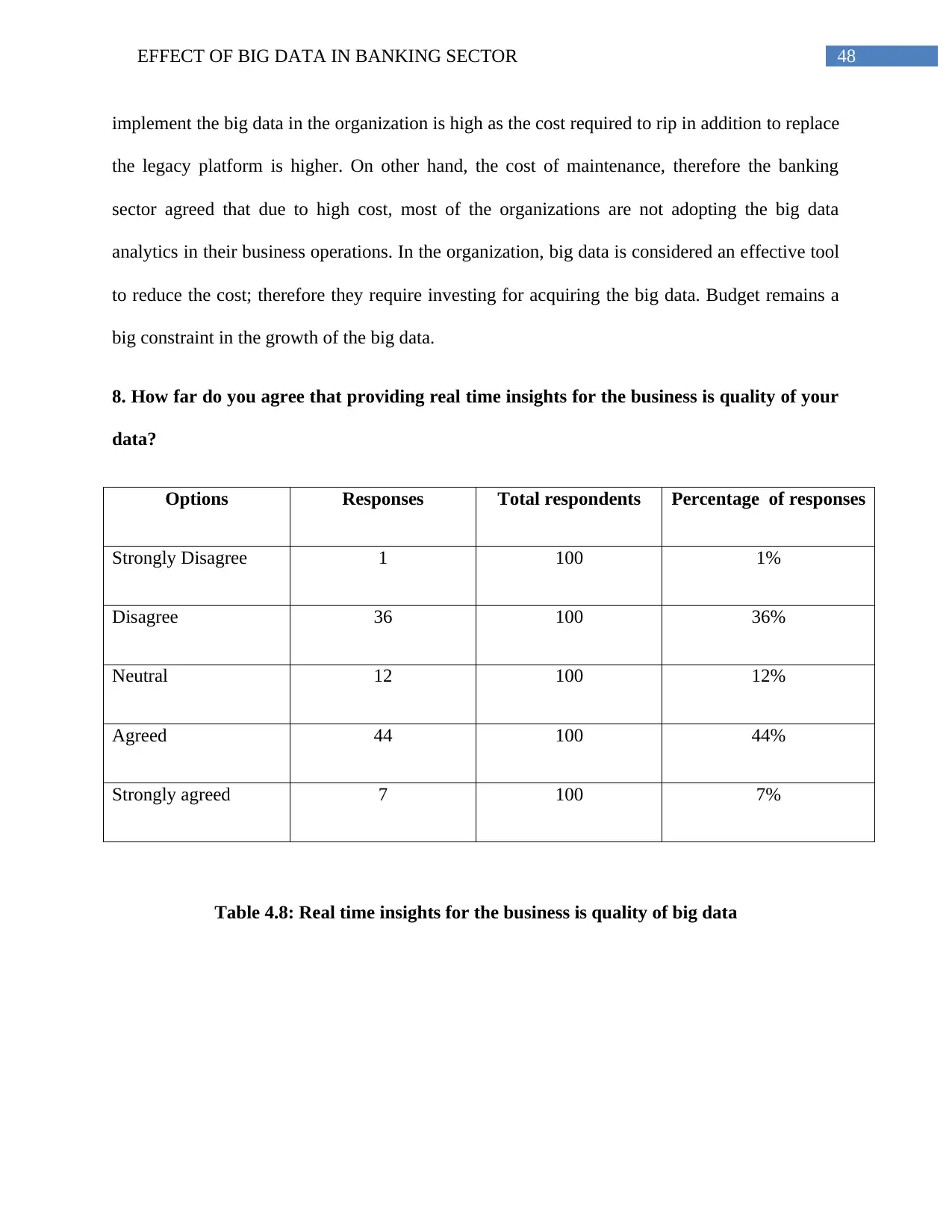
48EFFECT OF BIG DATA IN BANKING SECTOR
implement the big data in the organization is high as the cost required to rip in addition to replace
the legacy platform is higher. On other hand, the cost of maintenance, therefore the banking
sector agreed that due to high cost, most of the organizations are not adopting the big data
analytics in their business operations. In the organization, big data is considered an effective tool
to reduce the cost; therefore they require investing for acquiring the big data. Budget remains a
big constraint in the growth of the big data.
8. How far do you agree that providing real time insights for the business is quality of your
data?
Options Responses Total respondents Percentage of responses
Strongly Disagree 1 100 1%
Disagree 36 100 36%
Neutral 12 100 12%
Agreed 44 100 44%
Strongly agreed 7 100 7%
Table 4.8: Real time insights for the business is quality of big data
implement the big data in the organization is high as the cost required to rip in addition to replace
the legacy platform is higher. On other hand, the cost of maintenance, therefore the banking
sector agreed that due to high cost, most of the organizations are not adopting the big data
analytics in their business operations. In the organization, big data is considered an effective tool
to reduce the cost; therefore they require investing for acquiring the big data. Budget remains a
big constraint in the growth of the big data.
8. How far do you agree that providing real time insights for the business is quality of your
data?
Options Responses Total respondents Percentage of responses
Strongly Disagree 1 100 1%
Disagree 36 100 36%
Neutral 12 100 12%
Agreed 44 100 44%
Strongly agreed 7 100 7%
Table 4.8: Real time insights for the business is quality of big data
Secure Best Marks with AI Grader
Need help grading? Try our AI Grader for instant feedback on your assignments.
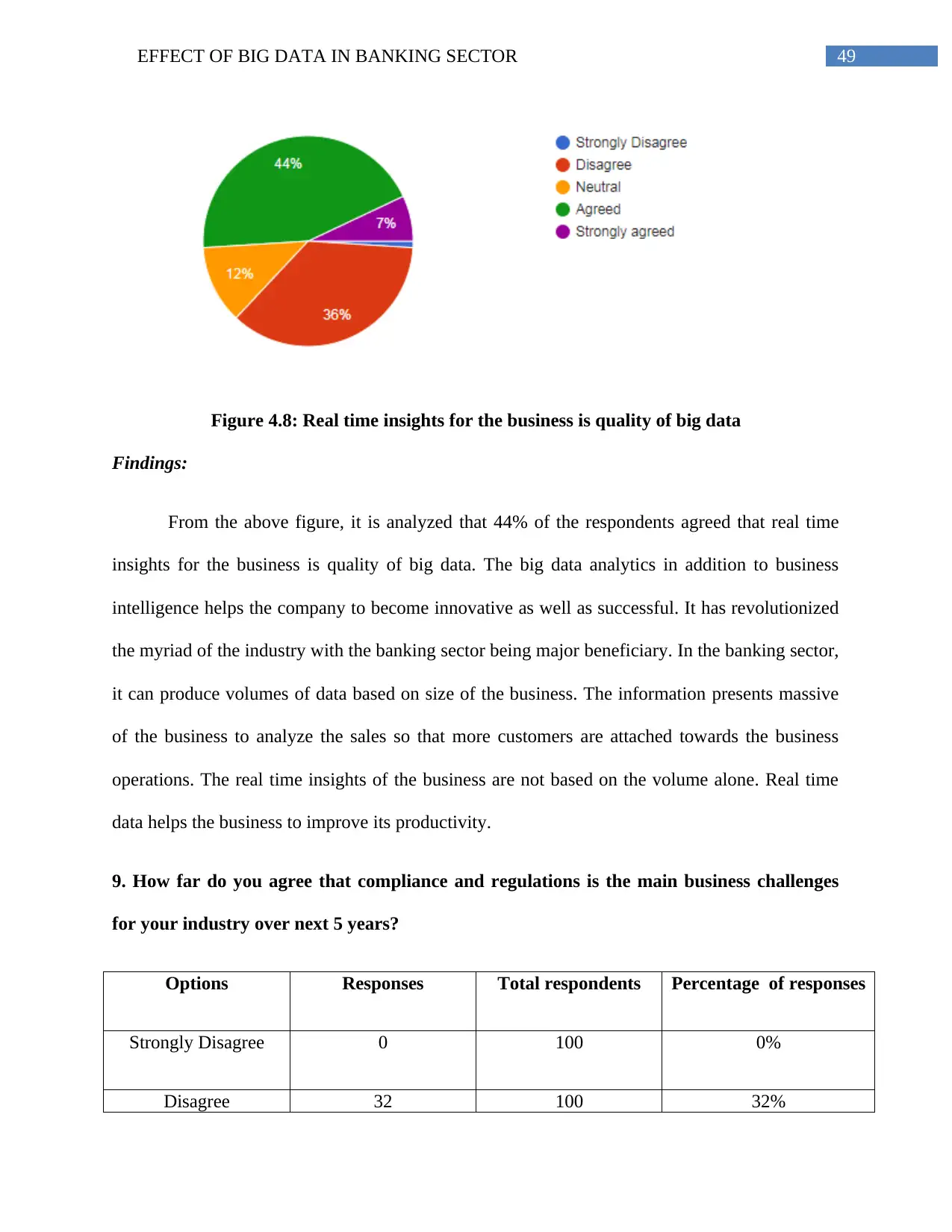
49EFFECT OF BIG DATA IN BANKING SECTOR
Figure 4.8: Real time insights for the business is quality of big data
Findings:
From the above figure, it is analyzed that 44% of the respondents agreed that real time
insights for the business is quality of big data. The big data analytics in addition to business
intelligence helps the company to become innovative as well as successful. It has revolutionized
the myriad of the industry with the banking sector being major beneficiary. In the banking sector,
it can produce volumes of data based on size of the business. The information presents massive
of the business to analyze the sales so that more customers are attached towards the business
operations. The real time insights of the business are not based on the volume alone. Real time
data helps the business to improve its productivity.
9. How far do you agree that compliance and regulations is the main business challenges
for your industry over next 5 years?
Options Responses Total respondents Percentage of responses
Strongly Disagree 0 100 0%
Disagree 32 100 32%
Figure 4.8: Real time insights for the business is quality of big data
Findings:
From the above figure, it is analyzed that 44% of the respondents agreed that real time
insights for the business is quality of big data. The big data analytics in addition to business
intelligence helps the company to become innovative as well as successful. It has revolutionized
the myriad of the industry with the banking sector being major beneficiary. In the banking sector,
it can produce volumes of data based on size of the business. The information presents massive
of the business to analyze the sales so that more customers are attached towards the business
operations. The real time insights of the business are not based on the volume alone. Real time
data helps the business to improve its productivity.
9. How far do you agree that compliance and regulations is the main business challenges
for your industry over next 5 years?
Options Responses Total respondents Percentage of responses
Strongly Disagree 0 100 0%
Disagree 32 100 32%
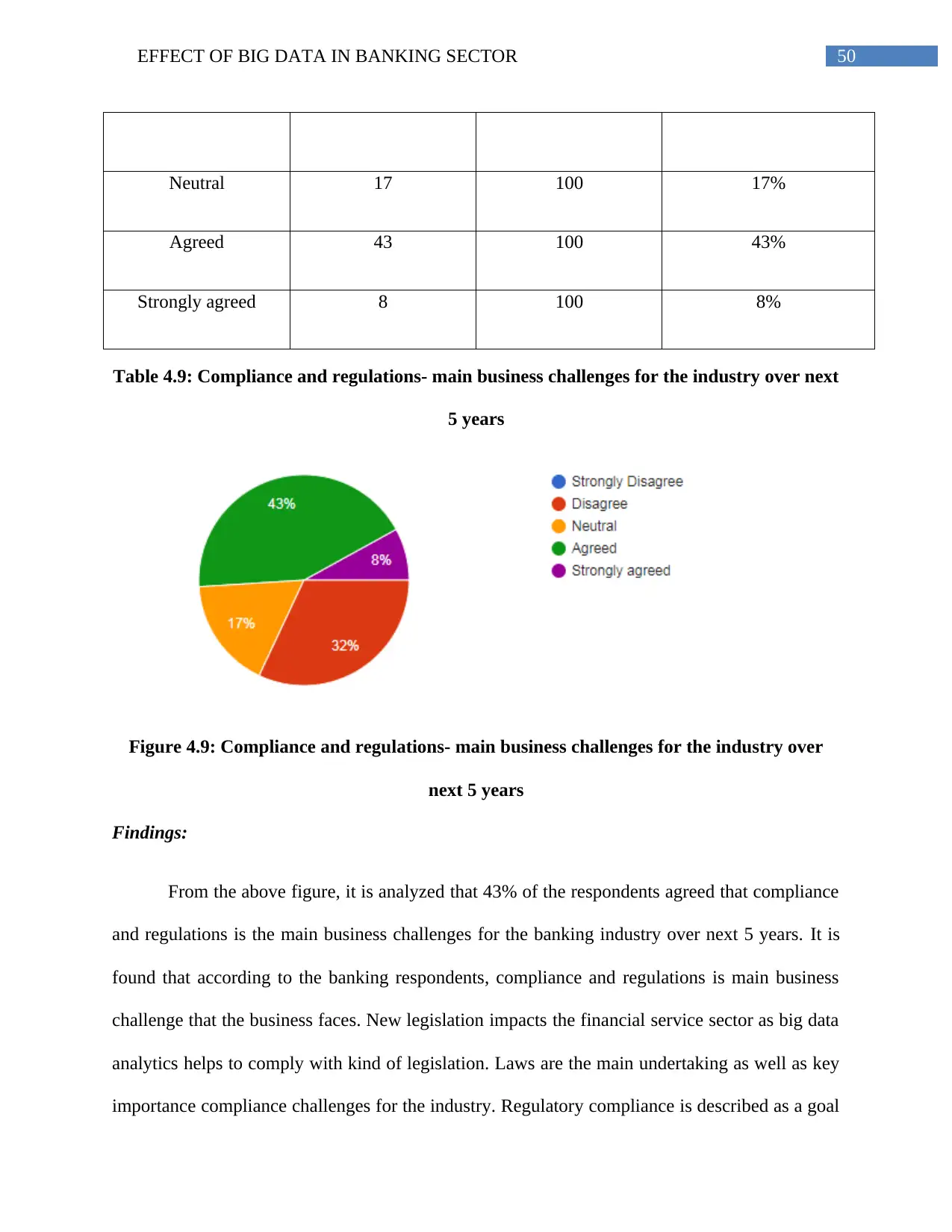
50EFFECT OF BIG DATA IN BANKING SECTOR
Neutral 17 100 17%
Agreed 43 100 43%
Strongly agreed 8 100 8%
Table 4.9: Compliance and regulations- main business challenges for the industry over next
5 years
Figure 4.9: Compliance and regulations- main business challenges for the industry over
next 5 years
Findings:
From the above figure, it is analyzed that 43% of the respondents agreed that compliance
and regulations is the main business challenges for the banking industry over next 5 years. It is
found that according to the banking respondents, compliance and regulations is main business
challenge that the business faces. New legislation impacts the financial service sector as big data
analytics helps to comply with kind of legislation. Laws are the main undertaking as well as key
importance compliance challenges for the industry. Regulatory compliance is described as a goal
Neutral 17 100 17%
Agreed 43 100 43%
Strongly agreed 8 100 8%
Table 4.9: Compliance and regulations- main business challenges for the industry over next
5 years
Figure 4.9: Compliance and regulations- main business challenges for the industry over
next 5 years
Findings:
From the above figure, it is analyzed that 43% of the respondents agreed that compliance
and regulations is the main business challenges for the banking industry over next 5 years. It is
found that according to the banking respondents, compliance and regulations is main business
challenge that the business faces. New legislation impacts the financial service sector as big data
analytics helps to comply with kind of legislation. Laws are the main undertaking as well as key
importance compliance challenges for the industry. Regulatory compliance is described as a goal
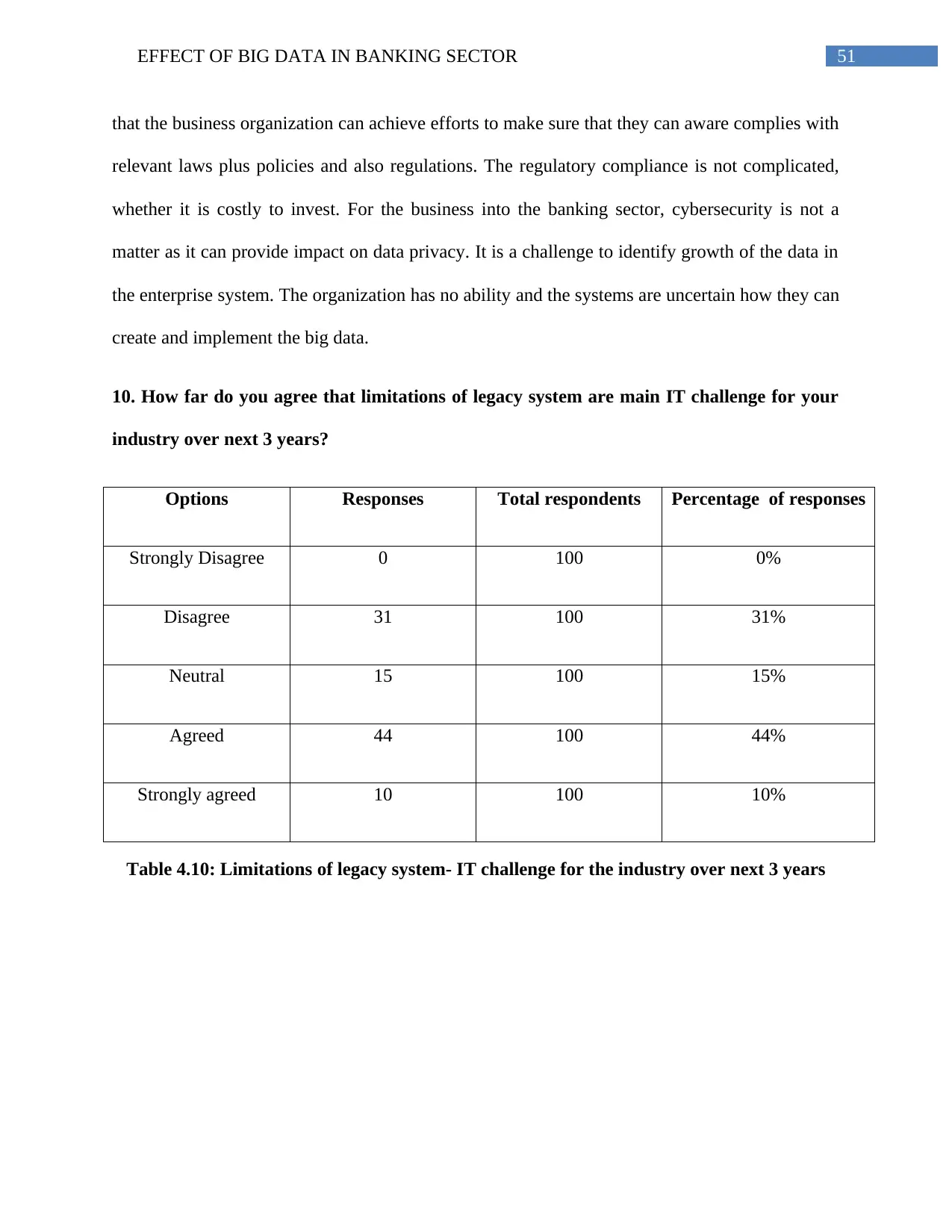
51EFFECT OF BIG DATA IN BANKING SECTOR
that the business organization can achieve efforts to make sure that they can aware complies with
relevant laws plus policies and also regulations. The regulatory compliance is not complicated,
whether it is costly to invest. For the business into the banking sector, cybersecurity is not a
matter as it can provide impact on data privacy. It is a challenge to identify growth of the data in
the enterprise system. The organization has no ability and the systems are uncertain how they can
create and implement the big data.
10. How far do you agree that limitations of legacy system are main IT challenge for your
industry over next 3 years?
Options Responses Total respondents Percentage of responses
Strongly Disagree 0 100 0%
Disagree 31 100 31%
Neutral 15 100 15%
Agreed 44 100 44%
Strongly agreed 10 100 10%
Table 4.10: Limitations of legacy system- IT challenge for the industry over next 3 years
that the business organization can achieve efforts to make sure that they can aware complies with
relevant laws plus policies and also regulations. The regulatory compliance is not complicated,
whether it is costly to invest. For the business into the banking sector, cybersecurity is not a
matter as it can provide impact on data privacy. It is a challenge to identify growth of the data in
the enterprise system. The organization has no ability and the systems are uncertain how they can
create and implement the big data.
10. How far do you agree that limitations of legacy system are main IT challenge for your
industry over next 3 years?
Options Responses Total respondents Percentage of responses
Strongly Disagree 0 100 0%
Disagree 31 100 31%
Neutral 15 100 15%
Agreed 44 100 44%
Strongly agreed 10 100 10%
Table 4.10: Limitations of legacy system- IT challenge for the industry over next 3 years
Paraphrase This Document
Need a fresh take? Get an instant paraphrase of this document with our AI Paraphraser
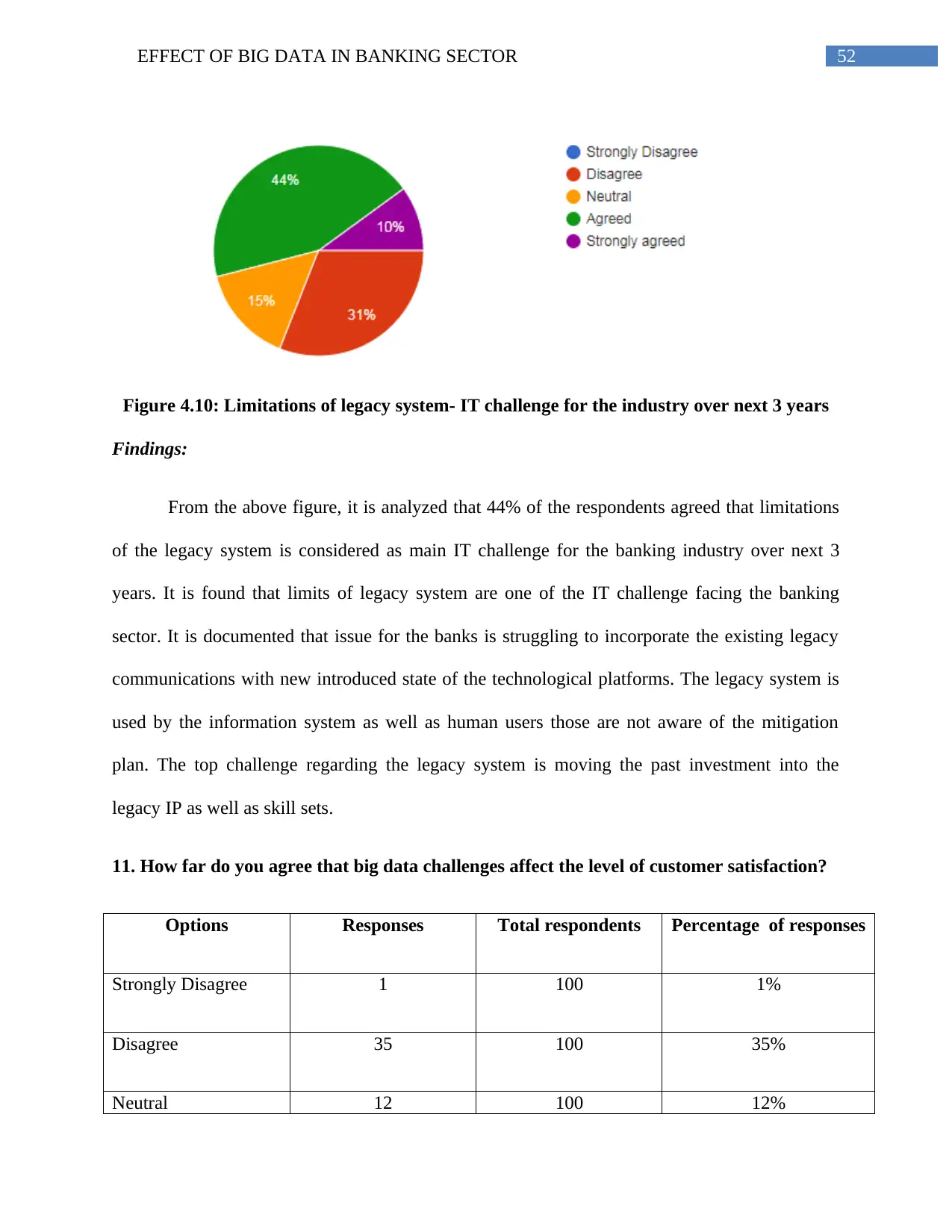
52EFFECT OF BIG DATA IN BANKING SECTOR
Figure 4.10: Limitations of legacy system- IT challenge for the industry over next 3 years
Findings:
From the above figure, it is analyzed that 44% of the respondents agreed that limitations
of the legacy system is considered as main IT challenge for the banking industry over next 3
years. It is found that limits of legacy system are one of the IT challenge facing the banking
sector. It is documented that issue for the banks is struggling to incorporate the existing legacy
communications with new introduced state of the technological platforms. The legacy system is
used by the information system as well as human users those are not aware of the mitigation
plan. The top challenge regarding the legacy system is moving the past investment into the
legacy IP as well as skill sets.
11. How far do you agree that big data challenges affect the level of customer satisfaction?
Options Responses Total respondents Percentage of responses
Strongly Disagree 1 100 1%
Disagree 35 100 35%
Neutral 12 100 12%
Figure 4.10: Limitations of legacy system- IT challenge for the industry over next 3 years
Findings:
From the above figure, it is analyzed that 44% of the respondents agreed that limitations
of the legacy system is considered as main IT challenge for the banking industry over next 3
years. It is found that limits of legacy system are one of the IT challenge facing the banking
sector. It is documented that issue for the banks is struggling to incorporate the existing legacy
communications with new introduced state of the technological platforms. The legacy system is
used by the information system as well as human users those are not aware of the mitigation
plan. The top challenge regarding the legacy system is moving the past investment into the
legacy IP as well as skill sets.
11. How far do you agree that big data challenges affect the level of customer satisfaction?
Options Responses Total respondents Percentage of responses
Strongly Disagree 1 100 1%
Disagree 35 100 35%
Neutral 12 100 12%
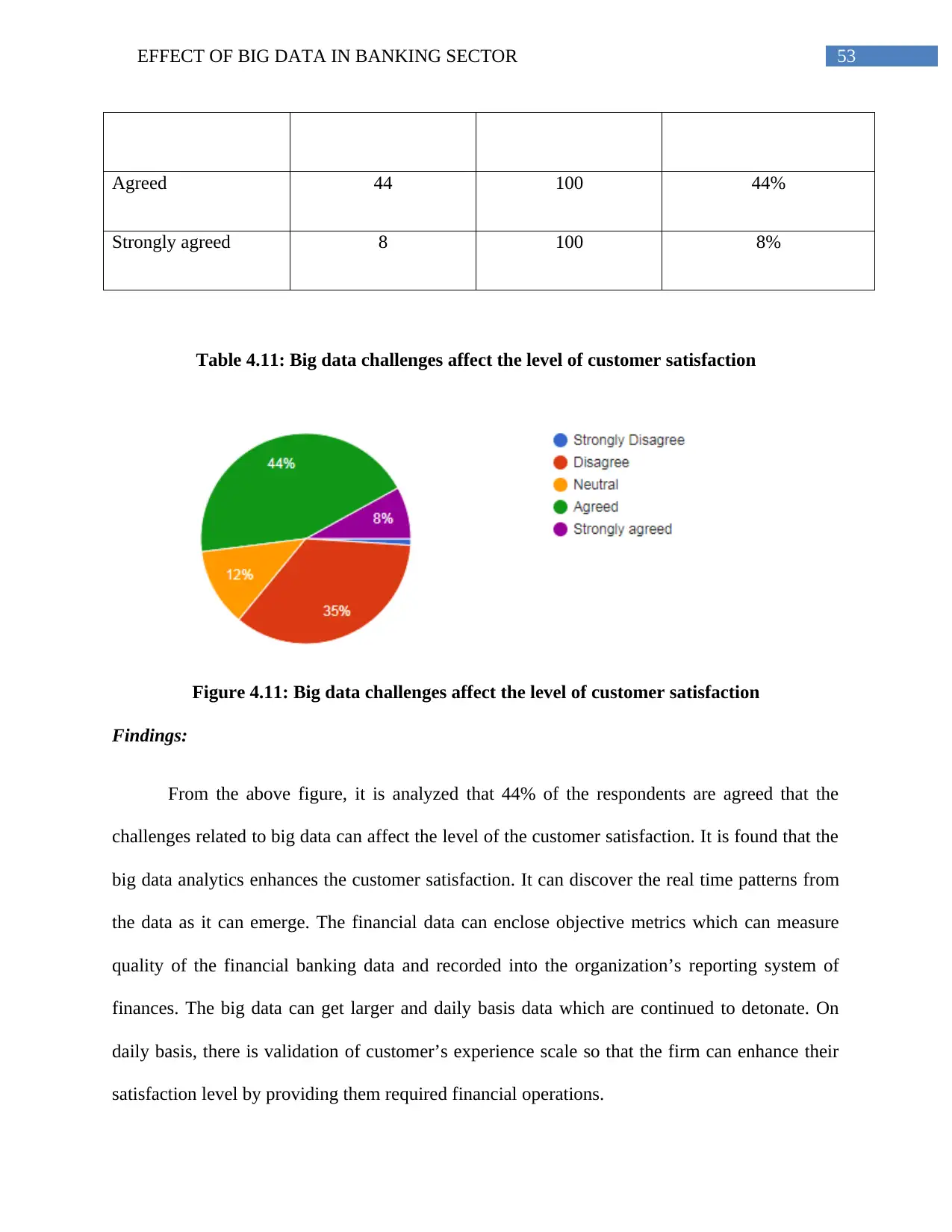
53EFFECT OF BIG DATA IN BANKING SECTOR
Agreed 44 100 44%
Strongly agreed 8 100 8%
Table 4.11: Big data challenges affect the level of customer satisfaction
Figure 4.11: Big data challenges affect the level of customer satisfaction
Findings:
From the above figure, it is analyzed that 44% of the respondents are agreed that the
challenges related to big data can affect the level of the customer satisfaction. It is found that the
big data analytics enhances the customer satisfaction. It can discover the real time patterns from
the data as it can emerge. The financial data can enclose objective metrics which can measure
quality of the financial banking data and recorded into the organization’s reporting system of
finances. The big data can get larger and daily basis data which are continued to detonate. On
daily basis, there is validation of customer’s experience scale so that the firm can enhance their
satisfaction level by providing them required financial operations.
Agreed 44 100 44%
Strongly agreed 8 100 8%
Table 4.11: Big data challenges affect the level of customer satisfaction
Figure 4.11: Big data challenges affect the level of customer satisfaction
Findings:
From the above figure, it is analyzed that 44% of the respondents are agreed that the
challenges related to big data can affect the level of the customer satisfaction. It is found that the
big data analytics enhances the customer satisfaction. It can discover the real time patterns from
the data as it can emerge. The financial data can enclose objective metrics which can measure
quality of the financial banking data and recorded into the organization’s reporting system of
finances. The big data can get larger and daily basis data which are continued to detonate. On
daily basis, there is validation of customer’s experience scale so that the firm can enhance their
satisfaction level by providing them required financial operations.
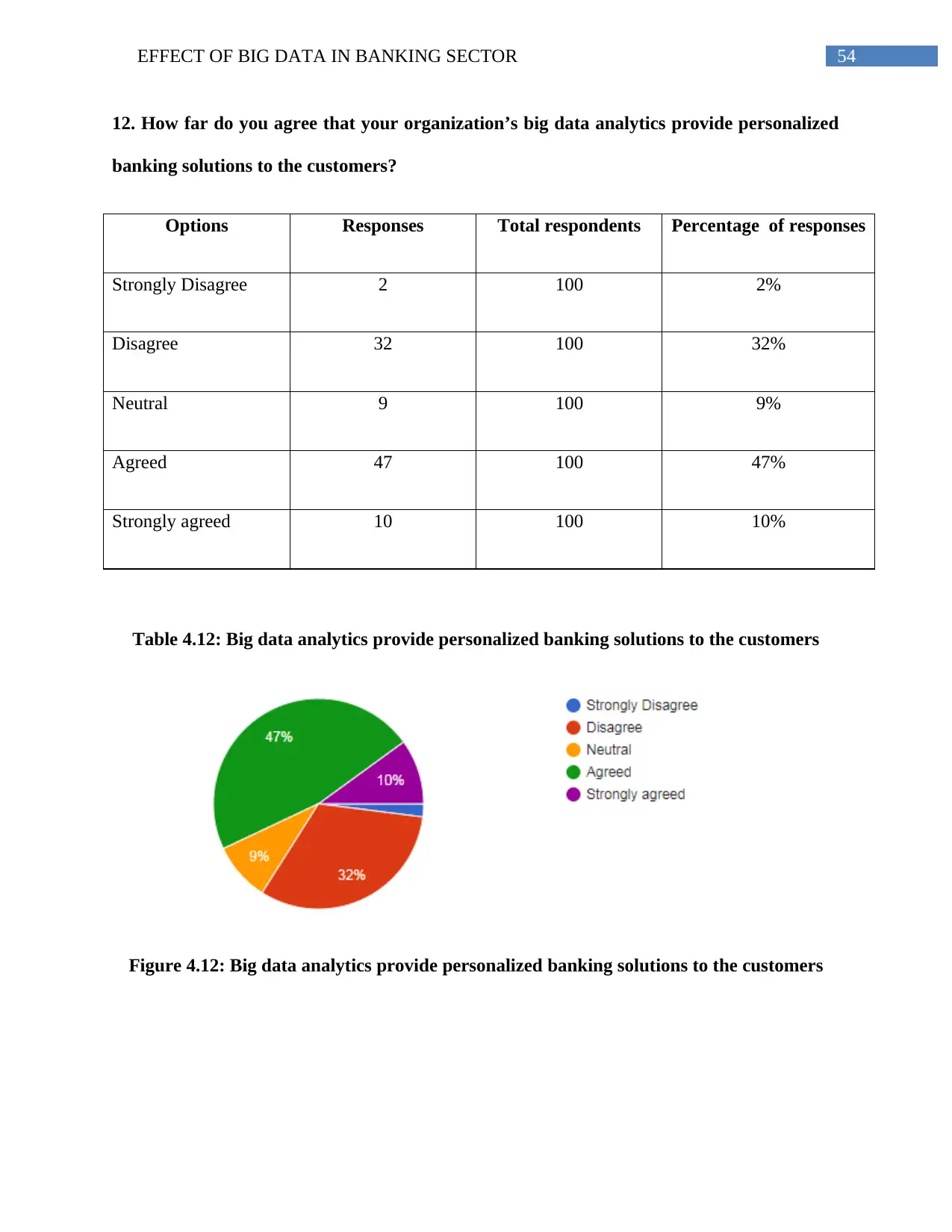
54EFFECT OF BIG DATA IN BANKING SECTOR
12. How far do you agree that your organization’s big data analytics provide personalized
banking solutions to the customers?
Options Responses Total respondents Percentage of responses
Strongly Disagree 2 100 2%
Disagree 32 100 32%
Neutral 9 100 9%
Agreed 47 100 47%
Strongly agreed 10 100 10%
Table 4.12: Big data analytics provide personalized banking solutions to the customers
Figure 4.12: Big data analytics provide personalized banking solutions to the customers
12. How far do you agree that your organization’s big data analytics provide personalized
banking solutions to the customers?
Options Responses Total respondents Percentage of responses
Strongly Disagree 2 100 2%
Disagree 32 100 32%
Neutral 9 100 9%
Agreed 47 100 47%
Strongly agreed 10 100 10%
Table 4.12: Big data analytics provide personalized banking solutions to the customers
Figure 4.12: Big data analytics provide personalized banking solutions to the customers
Secure Best Marks with AI Grader
Need help grading? Try our AI Grader for instant feedback on your assignments.
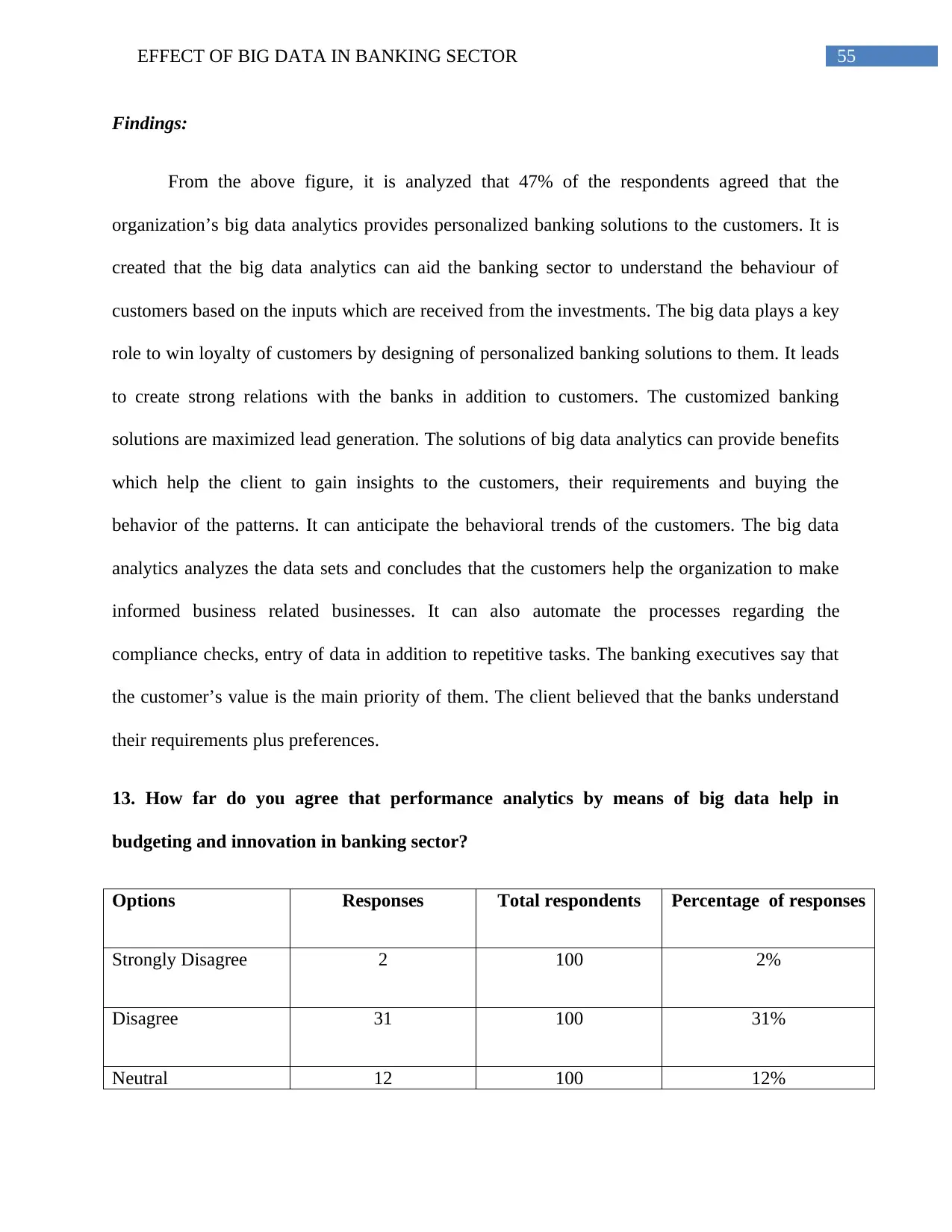
55EFFECT OF BIG DATA IN BANKING SECTOR
Findings:
From the above figure, it is analyzed that 47% of the respondents agreed that the
organization’s big data analytics provides personalized banking solutions to the customers. It is
created that the big data analytics can aid the banking sector to understand the behaviour of
customers based on the inputs which are received from the investments. The big data plays a key
role to win loyalty of customers by designing of personalized banking solutions to them. It leads
to create strong relations with the banks in addition to customers. The customized banking
solutions are maximized lead generation. The solutions of big data analytics can provide benefits
which help the client to gain insights to the customers, their requirements and buying the
behavior of the patterns. It can anticipate the behavioral trends of the customers. The big data
analytics analyzes the data sets and concludes that the customers help the organization to make
informed business related businesses. It can also automate the processes regarding the
compliance checks, entry of data in addition to repetitive tasks. The banking executives say that
the customer’s value is the main priority of them. The client believed that the banks understand
their requirements plus preferences.
13. How far do you agree that performance analytics by means of big data help in
budgeting and innovation in banking sector?
Options Responses Total respondents Percentage of responses
Strongly Disagree 2 100 2%
Disagree 31 100 31%
Neutral 12 100 12%
Findings:
From the above figure, it is analyzed that 47% of the respondents agreed that the
organization’s big data analytics provides personalized banking solutions to the customers. It is
created that the big data analytics can aid the banking sector to understand the behaviour of
customers based on the inputs which are received from the investments. The big data plays a key
role to win loyalty of customers by designing of personalized banking solutions to them. It leads
to create strong relations with the banks in addition to customers. The customized banking
solutions are maximized lead generation. The solutions of big data analytics can provide benefits
which help the client to gain insights to the customers, their requirements and buying the
behavior of the patterns. It can anticipate the behavioral trends of the customers. The big data
analytics analyzes the data sets and concludes that the customers help the organization to make
informed business related businesses. It can also automate the processes regarding the
compliance checks, entry of data in addition to repetitive tasks. The banking executives say that
the customer’s value is the main priority of them. The client believed that the banks understand
their requirements plus preferences.
13. How far do you agree that performance analytics by means of big data help in
budgeting and innovation in banking sector?
Options Responses Total respondents Percentage of responses
Strongly Disagree 2 100 2%
Disagree 31 100 31%
Neutral 12 100 12%
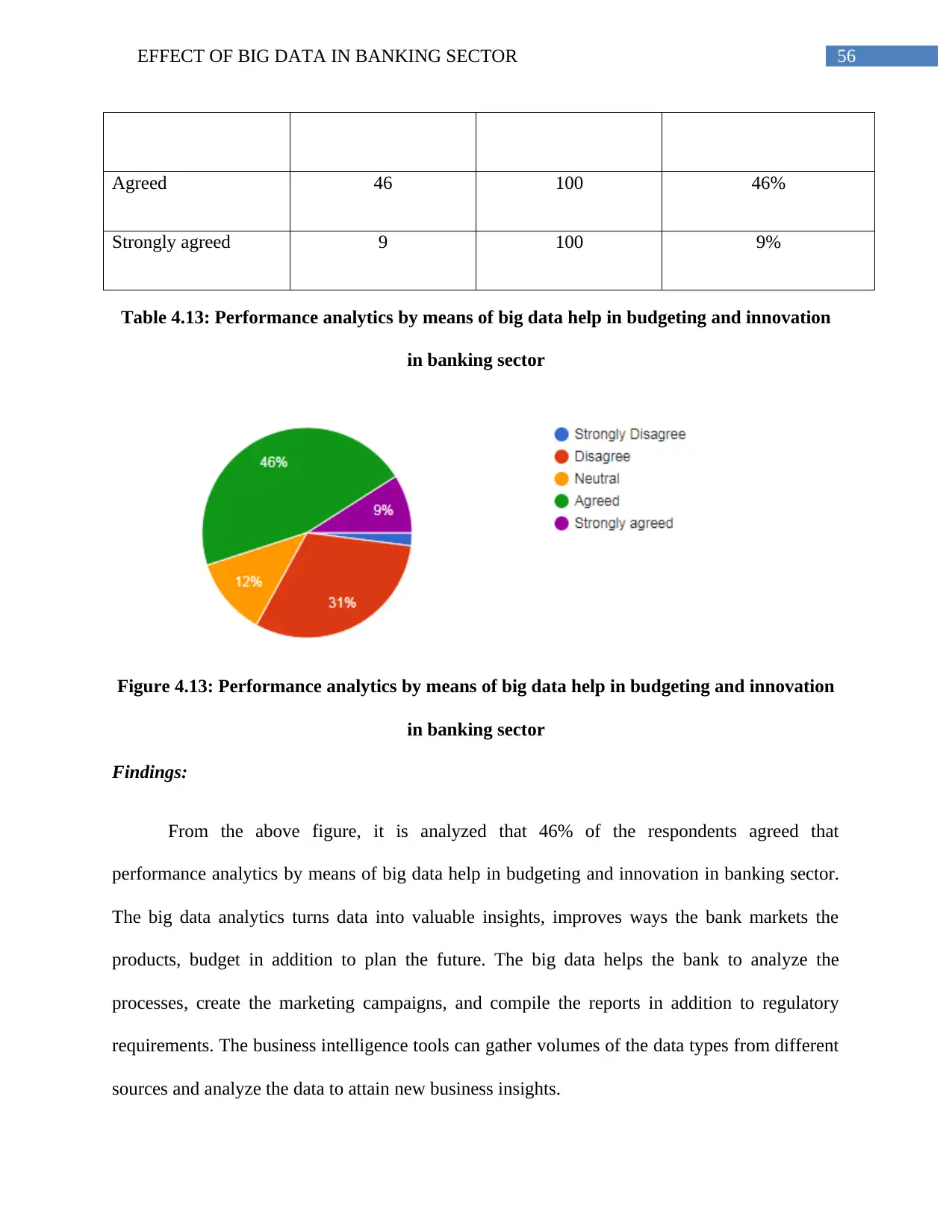
56EFFECT OF BIG DATA IN BANKING SECTOR
Agreed 46 100 46%
Strongly agreed 9 100 9%
Table 4.13: Performance analytics by means of big data help in budgeting and innovation
in banking sector
Figure 4.13: Performance analytics by means of big data help in budgeting and innovation
in banking sector
Findings:
From the above figure, it is analyzed that 46% of the respondents agreed that
performance analytics by means of big data help in budgeting and innovation in banking sector.
The big data analytics turns data into valuable insights, improves ways the bank markets the
products, budget in addition to plan the future. The big data helps the bank to analyze the
processes, create the marketing campaigns, and compile the reports in addition to regulatory
requirements. The business intelligence tools can gather volumes of the data types from different
sources and analyze the data to attain new business insights.
Agreed 46 100 46%
Strongly agreed 9 100 9%
Table 4.13: Performance analytics by means of big data help in budgeting and innovation
in banking sector
Figure 4.13: Performance analytics by means of big data help in budgeting and innovation
in banking sector
Findings:
From the above figure, it is analyzed that 46% of the respondents agreed that
performance analytics by means of big data help in budgeting and innovation in banking sector.
The big data analytics turns data into valuable insights, improves ways the bank markets the
products, budget in addition to plan the future. The big data helps the bank to analyze the
processes, create the marketing campaigns, and compile the reports in addition to regulatory
requirements. The business intelligence tools can gather volumes of the data types from different
sources and analyze the data to attain new business insights.
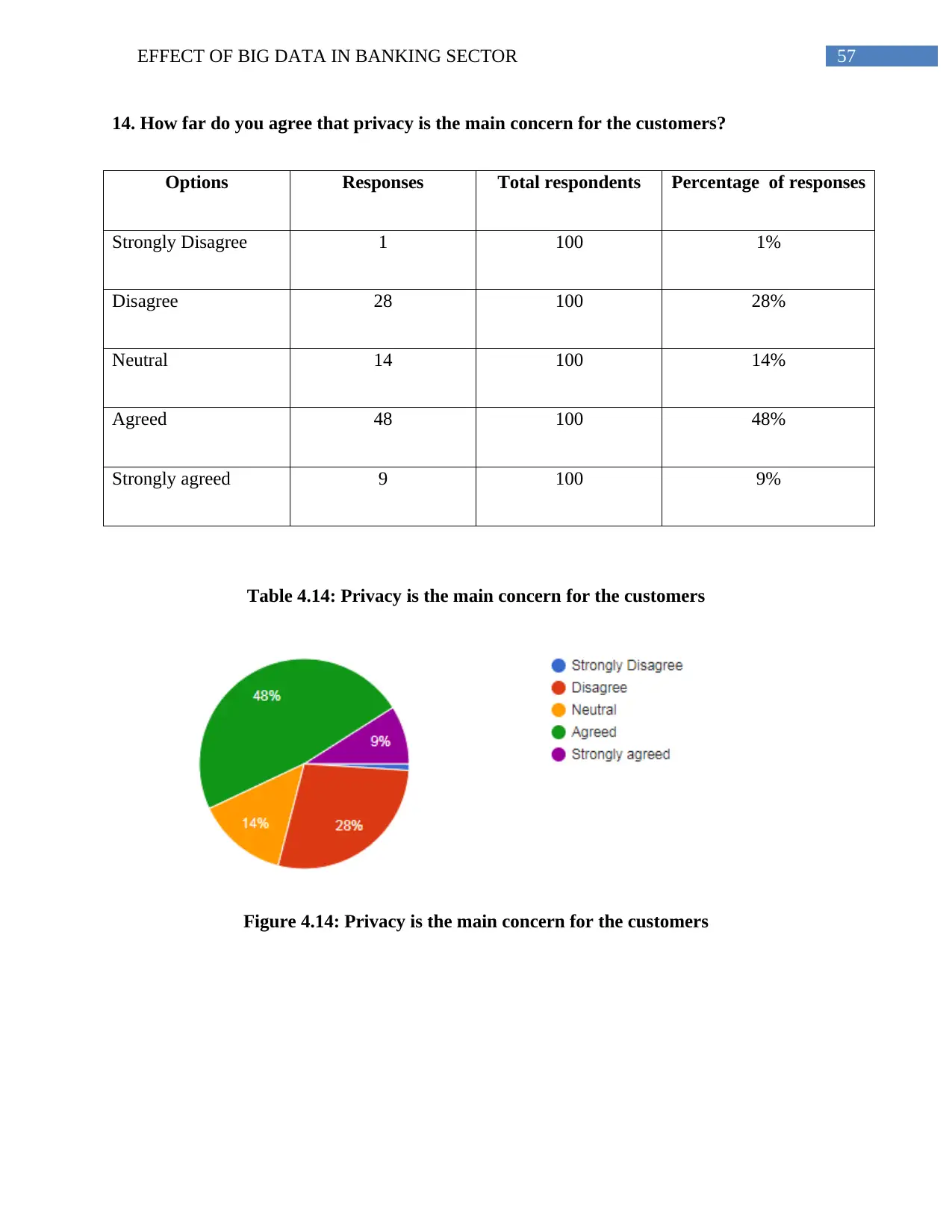
57EFFECT OF BIG DATA IN BANKING SECTOR
14. How far do you agree that privacy is the main concern for the customers?
Options Responses Total respondents Percentage of responses
Strongly Disagree 1 100 1%
Disagree 28 100 28%
Neutral 14 100 14%
Agreed 48 100 48%
Strongly agreed 9 100 9%
Table 4.14: Privacy is the main concern for the customers
Figure 4.14: Privacy is the main concern for the customers
14. How far do you agree that privacy is the main concern for the customers?
Options Responses Total respondents Percentage of responses
Strongly Disagree 1 100 1%
Disagree 28 100 28%
Neutral 14 100 14%
Agreed 48 100 48%
Strongly agreed 9 100 9%
Table 4.14: Privacy is the main concern for the customers
Figure 4.14: Privacy is the main concern for the customers
Paraphrase This Document
Need a fresh take? Get an instant paraphrase of this document with our AI Paraphraser
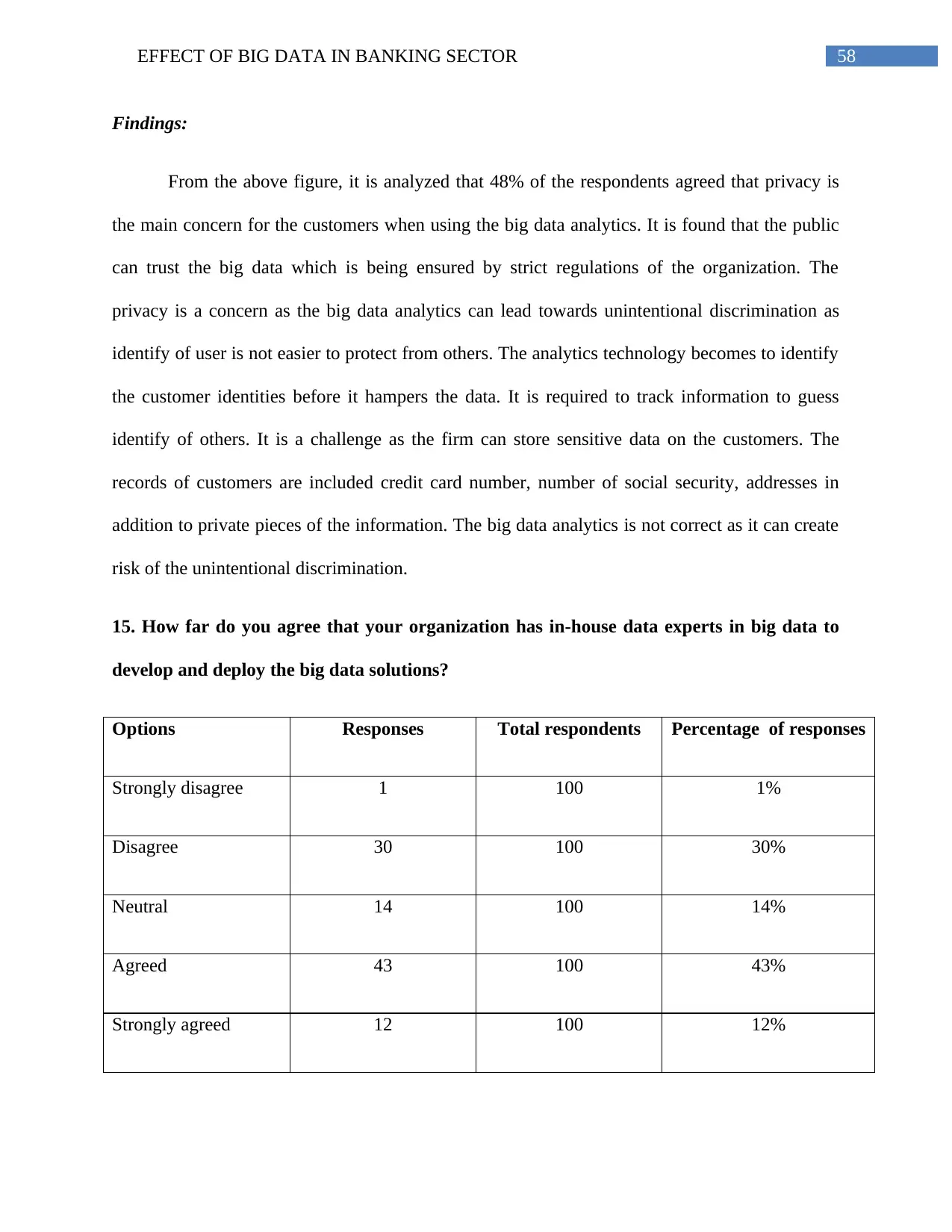
58EFFECT OF BIG DATA IN BANKING SECTOR
Findings:
From the above figure, it is analyzed that 48% of the respondents agreed that privacy is
the main concern for the customers when using the big data analytics. It is found that the public
can trust the big data which is being ensured by strict regulations of the organization. The
privacy is a concern as the big data analytics can lead towards unintentional discrimination as
identify of user is not easier to protect from others. The analytics technology becomes to identify
the customer identities before it hampers the data. It is required to track information to guess
identify of others. It is a challenge as the firm can store sensitive data on the customers. The
records of customers are included credit card number, number of social security, addresses in
addition to private pieces of the information. The big data analytics is not correct as it can create
risk of the unintentional discrimination.
15. How far do you agree that your organization has in-house data experts in big data to
develop and deploy the big data solutions?
Options Responses Total respondents Percentage of responses
Strongly disagree 1 100 1%
Disagree 30 100 30%
Neutral 14 100 14%
Agreed 43 100 43%
Strongly agreed 12 100 12%
Findings:
From the above figure, it is analyzed that 48% of the respondents agreed that privacy is
the main concern for the customers when using the big data analytics. It is found that the public
can trust the big data which is being ensured by strict regulations of the organization. The
privacy is a concern as the big data analytics can lead towards unintentional discrimination as
identify of user is not easier to protect from others. The analytics technology becomes to identify
the customer identities before it hampers the data. It is required to track information to guess
identify of others. It is a challenge as the firm can store sensitive data on the customers. The
records of customers are included credit card number, number of social security, addresses in
addition to private pieces of the information. The big data analytics is not correct as it can create
risk of the unintentional discrimination.
15. How far do you agree that your organization has in-house data experts in big data to
develop and deploy the big data solutions?
Options Responses Total respondents Percentage of responses
Strongly disagree 1 100 1%
Disagree 30 100 30%
Neutral 14 100 14%
Agreed 43 100 43%
Strongly agreed 12 100 12%
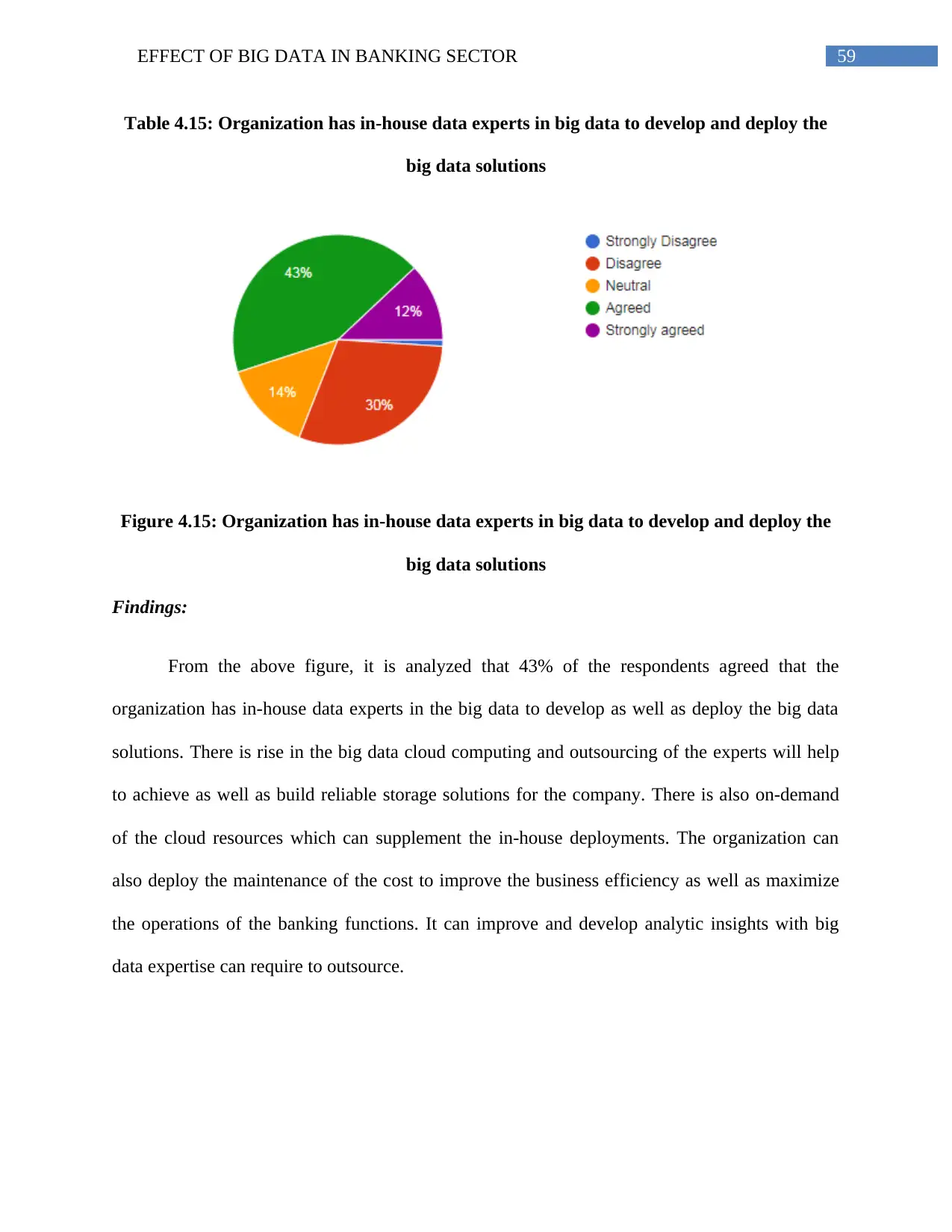
59EFFECT OF BIG DATA IN BANKING SECTOR
Table 4.15: Organization has in-house data experts in big data to develop and deploy the
big data solutions
Figure 4.15: Organization has in-house data experts in big data to develop and deploy the
big data solutions
Findings:
From the above figure, it is analyzed that 43% of the respondents agreed that the
organization has in-house data experts in the big data to develop as well as deploy the big data
solutions. There is rise in the big data cloud computing and outsourcing of the experts will help
to achieve as well as build reliable storage solutions for the company. There is also on-demand
of the cloud resources which can supplement the in-house deployments. The organization can
also deploy the maintenance of the cost to improve the business efficiency as well as maximize
the operations of the banking functions. It can improve and develop analytic insights with big
data expertise can require to outsource.
Table 4.15: Organization has in-house data experts in big data to develop and deploy the
big data solutions
Figure 4.15: Organization has in-house data experts in big data to develop and deploy the
big data solutions
Findings:
From the above figure, it is analyzed that 43% of the respondents agreed that the
organization has in-house data experts in the big data to develop as well as deploy the big data
solutions. There is rise in the big data cloud computing and outsourcing of the experts will help
to achieve as well as build reliable storage solutions for the company. There is also on-demand
of the cloud resources which can supplement the in-house deployments. The organization can
also deploy the maintenance of the cost to improve the business efficiency as well as maximize
the operations of the banking functions. It can improve and develop analytic insights with big
data expertise can require to outsource.
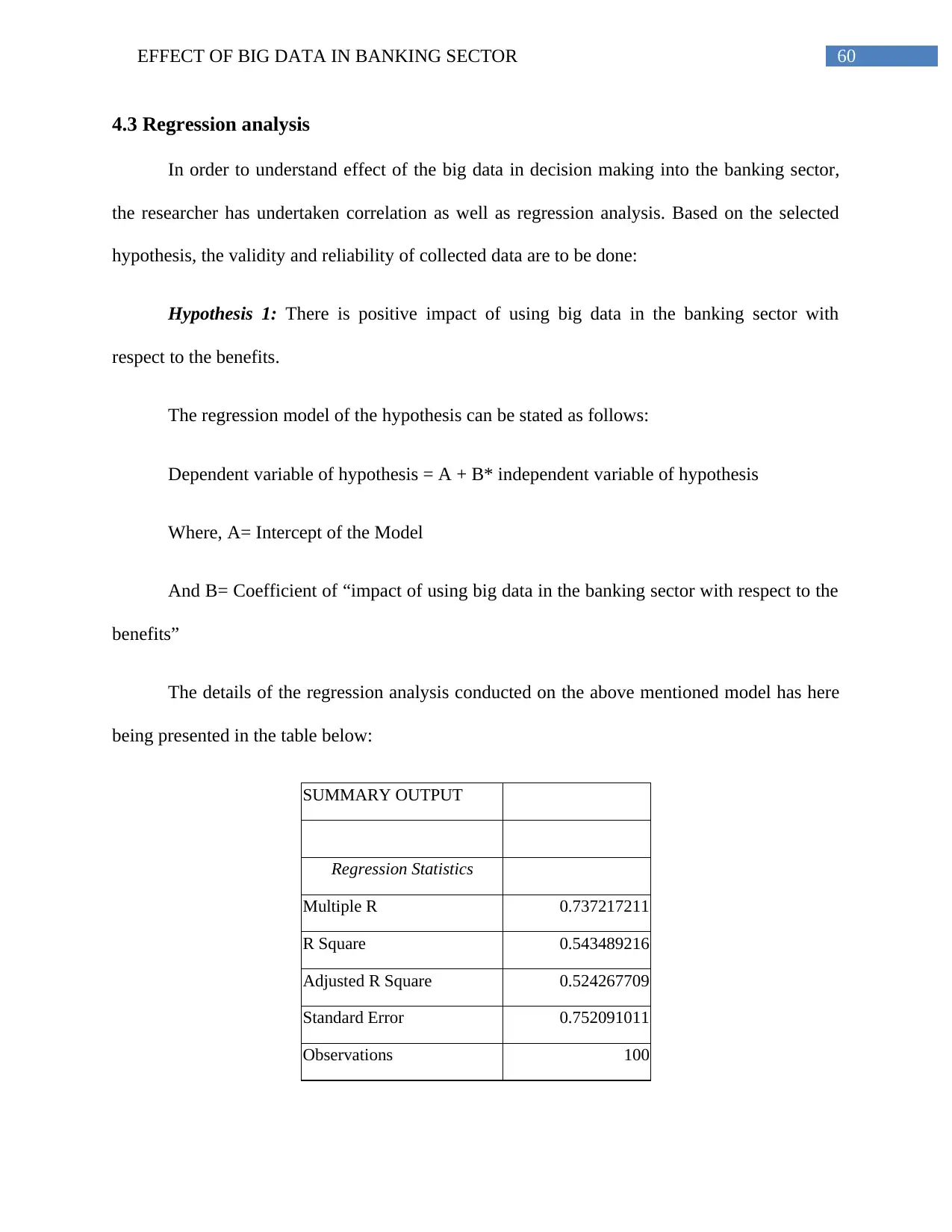
60EFFECT OF BIG DATA IN BANKING SECTOR
4.3 Regression analysis
In order to understand effect of the big data in decision making into the banking sector,
the researcher has undertaken correlation as well as regression analysis. Based on the selected
hypothesis, the validity and reliability of collected data are to be done:
Hypothesis 1: There is positive impact of using big data in the banking sector with
respect to the benefits.
The regression model of the hypothesis can be stated as follows:
Dependent variable of hypothesis = A + B* independent variable of hypothesis
Where, A= Intercept of the Model
And B= Coefficient of “impact of using big data in the banking sector with respect to the
benefits”
The details of the regression analysis conducted on the above mentioned model has here
being presented in the table below:
SUMMARY OUTPUT
Regression Statistics
Multiple R 0.737217211
R Square 0.543489216
Adjusted R Square 0.524267709
Standard Error 0.752091011
Observations 100
4.3 Regression analysis
In order to understand effect of the big data in decision making into the banking sector,
the researcher has undertaken correlation as well as regression analysis. Based on the selected
hypothesis, the validity and reliability of collected data are to be done:
Hypothesis 1: There is positive impact of using big data in the banking sector with
respect to the benefits.
The regression model of the hypothesis can be stated as follows:
Dependent variable of hypothesis = A + B* independent variable of hypothesis
Where, A= Intercept of the Model
And B= Coefficient of “impact of using big data in the banking sector with respect to the
benefits”
The details of the regression analysis conducted on the above mentioned model has here
being presented in the table below:
SUMMARY OUTPUT
Regression Statistics
Multiple R 0.737217211
R Square 0.543489216
Adjusted R Square 0.524267709
Standard Error 0.752091011
Observations 100
Secure Best Marks with AI Grader
Need help grading? Try our AI Grader for instant feedback on your assignments.
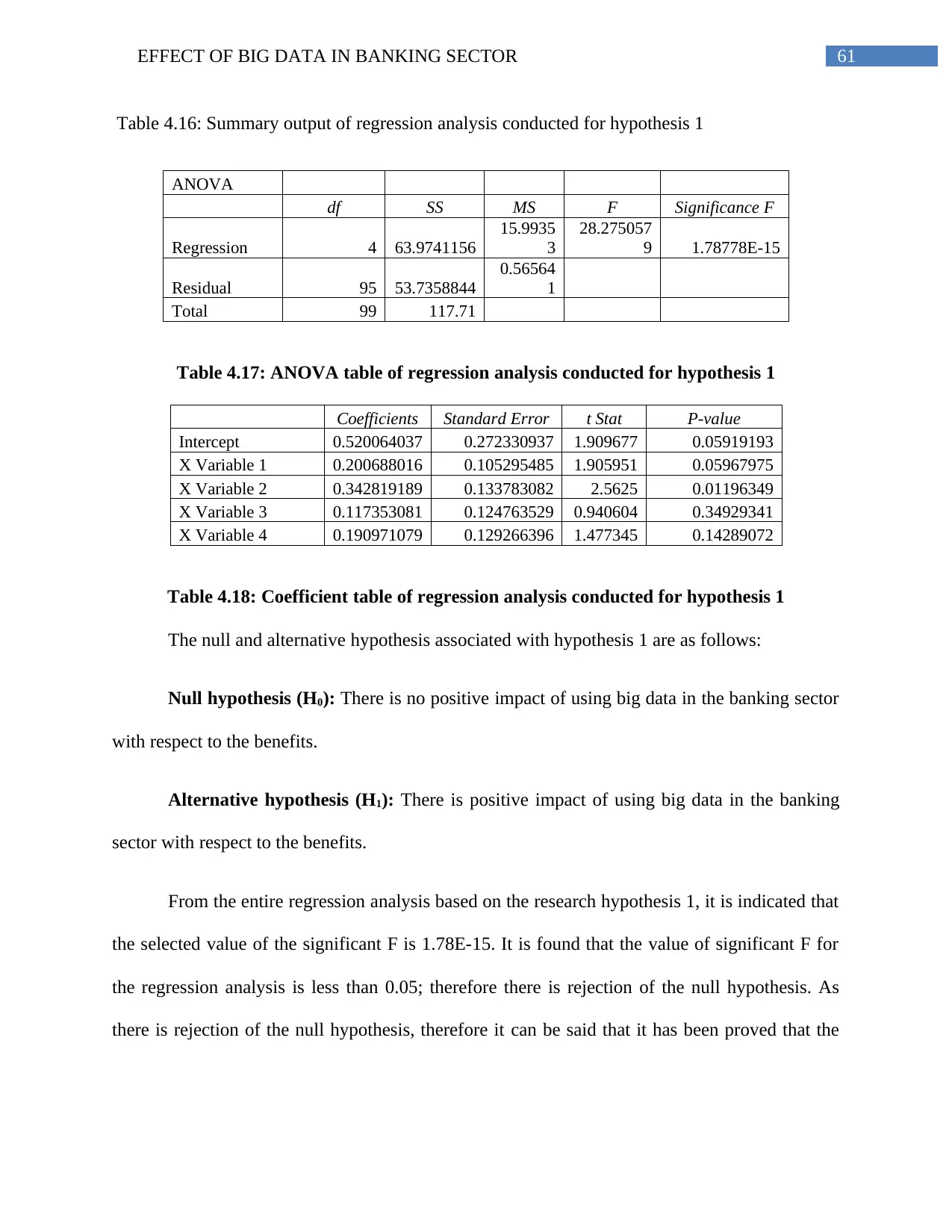
61EFFECT OF BIG DATA IN BANKING SECTOR
Table 4.16: Summary output of regression analysis conducted for hypothesis 1
ANOVA
df SS MS F Significance F
Regression 4 63.9741156
15.9935
3
28.275057
9 1.78778E-15
Residual 95 53.7358844
0.56564
1
Total 99 117.71
Table 4.17: ANOVA table of regression analysis conducted for hypothesis 1
Coefficients Standard Error t Stat P-value
Intercept 0.520064037 0.272330937 1.909677 0.05919193
X Variable 1 0.200688016 0.105295485 1.905951 0.05967975
X Variable 2 0.342819189 0.133783082 2.5625 0.01196349
X Variable 3 0.117353081 0.124763529 0.940604 0.34929341
X Variable 4 0.190971079 0.129266396 1.477345 0.14289072
Table 4.18: Coefficient table of regression analysis conducted for hypothesis 1
The null and alternative hypothesis associated with hypothesis 1 are as follows:
Null hypothesis (H0): There is no positive impact of using big data in the banking sector
with respect to the benefits.
Alternative hypothesis (H1): There is positive impact of using big data in the banking
sector with respect to the benefits.
From the entire regression analysis based on the research hypothesis 1, it is indicated that
the selected value of the significant F is 1.78E-15. It is found that the value of significant F for
the regression analysis is less than 0.05; therefore there is rejection of the null hypothesis. As
there is rejection of the null hypothesis, therefore it can be said that it has been proved that the
Table 4.16: Summary output of regression analysis conducted for hypothesis 1
ANOVA
df SS MS F Significance F
Regression 4 63.9741156
15.9935
3
28.275057
9 1.78778E-15
Residual 95 53.7358844
0.56564
1
Total 99 117.71
Table 4.17: ANOVA table of regression analysis conducted for hypothesis 1
Coefficients Standard Error t Stat P-value
Intercept 0.520064037 0.272330937 1.909677 0.05919193
X Variable 1 0.200688016 0.105295485 1.905951 0.05967975
X Variable 2 0.342819189 0.133783082 2.5625 0.01196349
X Variable 3 0.117353081 0.124763529 0.940604 0.34929341
X Variable 4 0.190971079 0.129266396 1.477345 0.14289072
Table 4.18: Coefficient table of regression analysis conducted for hypothesis 1
The null and alternative hypothesis associated with hypothesis 1 are as follows:
Null hypothesis (H0): There is no positive impact of using big data in the banking sector
with respect to the benefits.
Alternative hypothesis (H1): There is positive impact of using big data in the banking
sector with respect to the benefits.
From the entire regression analysis based on the research hypothesis 1, it is indicated that
the selected value of the significant F is 1.78E-15. It is found that the value of significant F for
the regression analysis is less than 0.05; therefore there is rejection of the null hypothesis. As
there is rejection of the null hypothesis, therefore it can be said that it has been proved that the
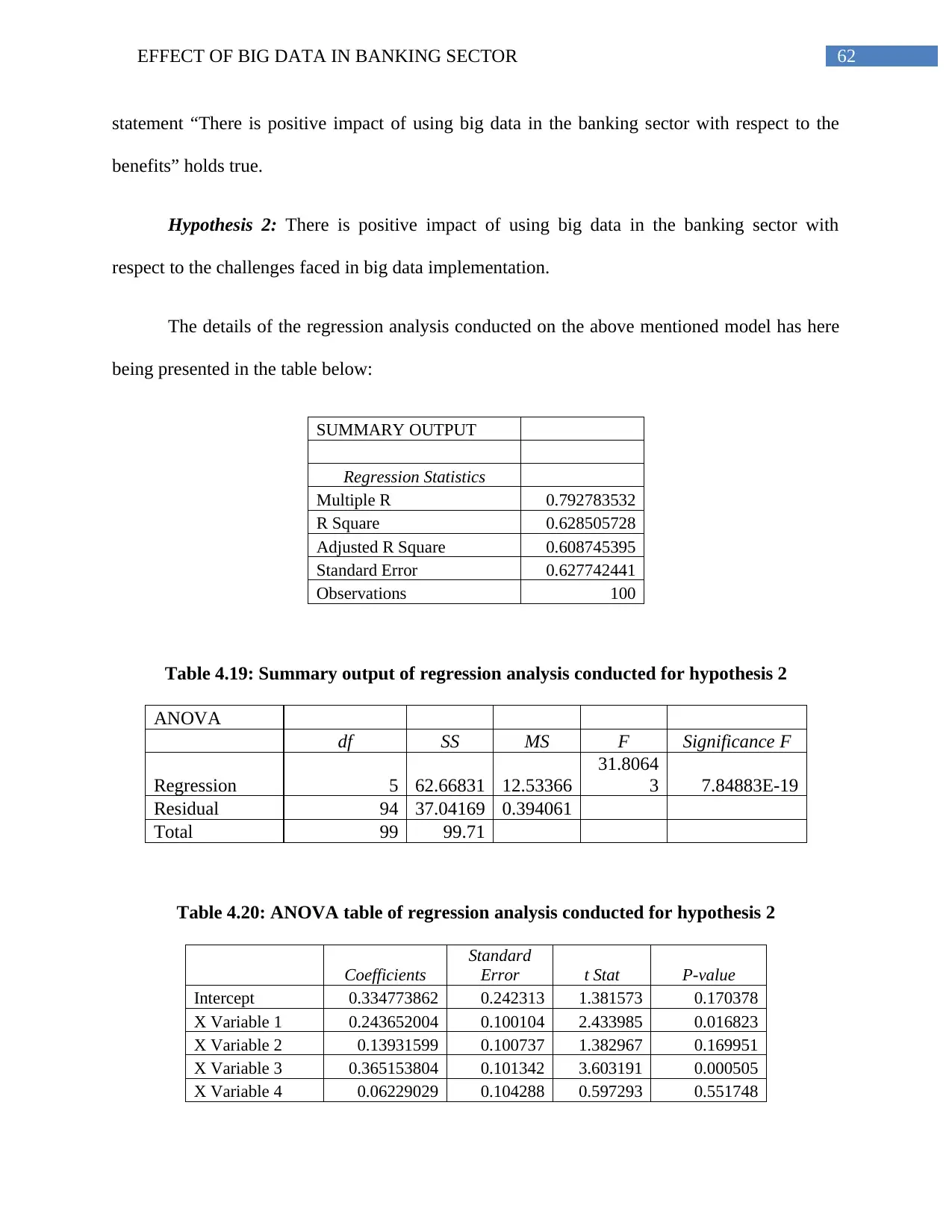
62EFFECT OF BIG DATA IN BANKING SECTOR
statement “There is positive impact of using big data in the banking sector with respect to the
benefits” holds true.
Hypothesis 2: There is positive impact of using big data in the banking sector with
respect to the challenges faced in big data implementation.
The details of the regression analysis conducted on the above mentioned model has here
being presented in the table below:
SUMMARY OUTPUT
Regression Statistics
Multiple R 0.792783532
R Square 0.628505728
Adjusted R Square 0.608745395
Standard Error 0.627742441
Observations 100
Table 4.19: Summary output of regression analysis conducted for hypothesis 2
ANOVA
df SS MS F Significance F
Regression 5 62.66831 12.53366
31.8064
3 7.84883E-19
Residual 94 37.04169 0.394061
Total 99 99.71
Table 4.20: ANOVA table of regression analysis conducted for hypothesis 2
Coefficients
Standard
Error t Stat P-value
Intercept 0.334773862 0.242313 1.381573 0.170378
X Variable 1 0.243652004 0.100104 2.433985 0.016823
X Variable 2 0.13931599 0.100737 1.382967 0.169951
X Variable 3 0.365153804 0.101342 3.603191 0.000505
X Variable 4 0.06229029 0.104288 0.597293 0.551748
statement “There is positive impact of using big data in the banking sector with respect to the
benefits” holds true.
Hypothesis 2: There is positive impact of using big data in the banking sector with
respect to the challenges faced in big data implementation.
The details of the regression analysis conducted on the above mentioned model has here
being presented in the table below:
SUMMARY OUTPUT
Regression Statistics
Multiple R 0.792783532
R Square 0.628505728
Adjusted R Square 0.608745395
Standard Error 0.627742441
Observations 100
Table 4.19: Summary output of regression analysis conducted for hypothesis 2
ANOVA
df SS MS F Significance F
Regression 5 62.66831 12.53366
31.8064
3 7.84883E-19
Residual 94 37.04169 0.394061
Total 99 99.71
Table 4.20: ANOVA table of regression analysis conducted for hypothesis 2
Coefficients
Standard
Error t Stat P-value
Intercept 0.334773862 0.242313 1.381573 0.170378
X Variable 1 0.243652004 0.100104 2.433985 0.016823
X Variable 2 0.13931599 0.100737 1.382967 0.169951
X Variable 3 0.365153804 0.101342 3.603191 0.000505
X Variable 4 0.06229029 0.104288 0.597293 0.551748
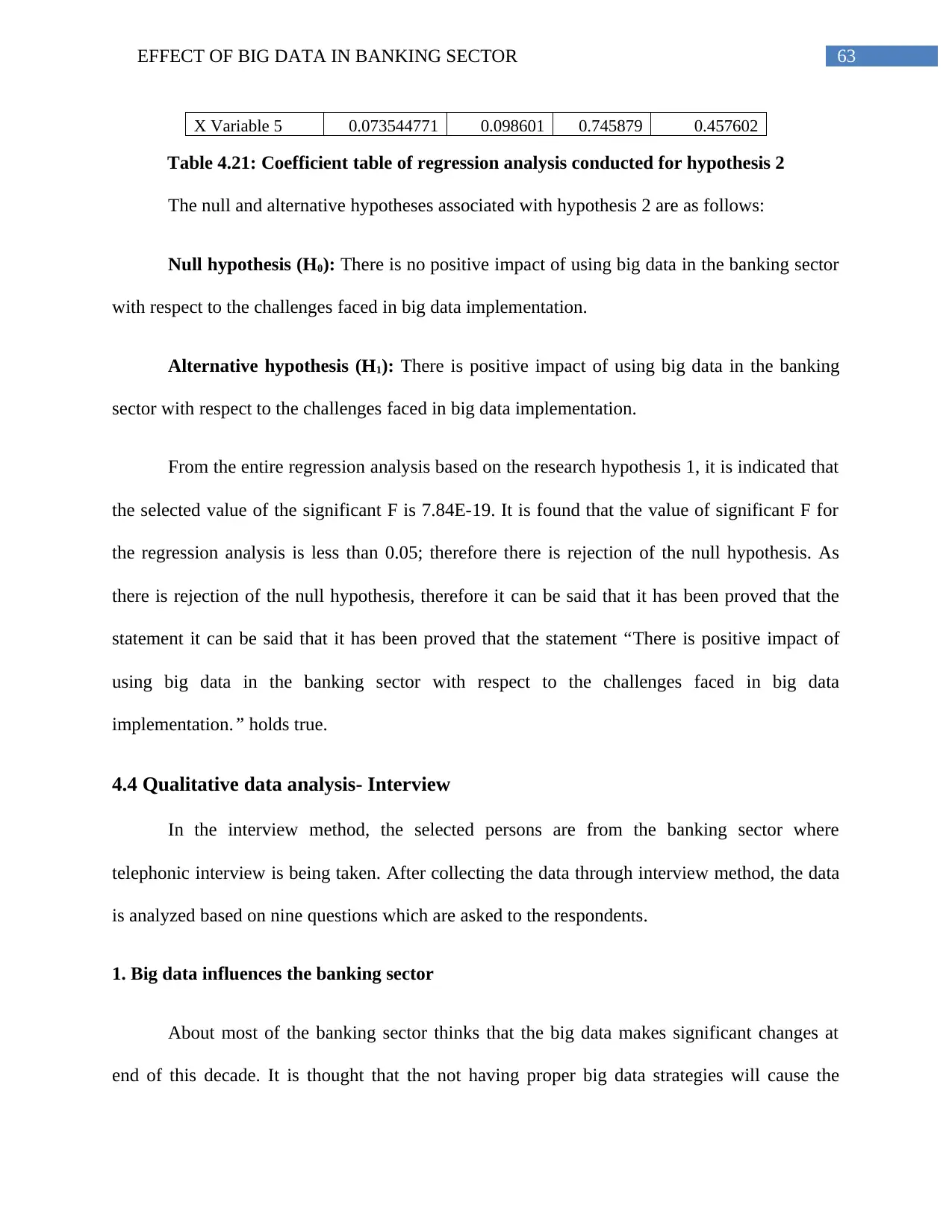
63EFFECT OF BIG DATA IN BANKING SECTOR
X Variable 5 0.073544771 0.098601 0.745879 0.457602
Table 4.21: Coefficient table of regression analysis conducted for hypothesis 2
The null and alternative hypotheses associated with hypothesis 2 are as follows:
Null hypothesis (H0): There is no positive impact of using big data in the banking sector
with respect to the challenges faced in big data implementation.
Alternative hypothesis (H1): There is positive impact of using big data in the banking
sector with respect to the challenges faced in big data implementation.
From the entire regression analysis based on the research hypothesis 1, it is indicated that
the selected value of the significant F is 7.84E-19. It is found that the value of significant F for
the regression analysis is less than 0.05; therefore there is rejection of the null hypothesis. As
there is rejection of the null hypothesis, therefore it can be said that it has been proved that the
statement it can be said that it has been proved that the statement “There is positive impact of
using big data in the banking sector with respect to the challenges faced in big data
implementation.” holds true.
4.4 Qualitative data analysis- Interview
In the interview method, the selected persons are from the banking sector where
telephonic interview is being taken. After collecting the data through interview method, the data
is analyzed based on nine questions which are asked to the respondents.
1. Big data influences the banking sector
About most of the banking sector thinks that the big data makes significant changes at
end of this decade. It is thought that the not having proper big data strategies will cause the
X Variable 5 0.073544771 0.098601 0.745879 0.457602
Table 4.21: Coefficient table of regression analysis conducted for hypothesis 2
The null and alternative hypotheses associated with hypothesis 2 are as follows:
Null hypothesis (H0): There is no positive impact of using big data in the banking sector
with respect to the challenges faced in big data implementation.
Alternative hypothesis (H1): There is positive impact of using big data in the banking
sector with respect to the challenges faced in big data implementation.
From the entire regression analysis based on the research hypothesis 1, it is indicated that
the selected value of the significant F is 7.84E-19. It is found that the value of significant F for
the regression analysis is less than 0.05; therefore there is rejection of the null hypothesis. As
there is rejection of the null hypothesis, therefore it can be said that it has been proved that the
statement it can be said that it has been proved that the statement “There is positive impact of
using big data in the banking sector with respect to the challenges faced in big data
implementation.” holds true.
4.4 Qualitative data analysis- Interview
In the interview method, the selected persons are from the banking sector where
telephonic interview is being taken. After collecting the data through interview method, the data
is analyzed based on nine questions which are asked to the respondents.
1. Big data influences the banking sector
About most of the banking sector thinks that the big data makes significant changes at
end of this decade. It is thought that the not having proper big data strategies will cause the
Paraphrase This Document
Need a fresh take? Get an instant paraphrase of this document with our AI Paraphraser
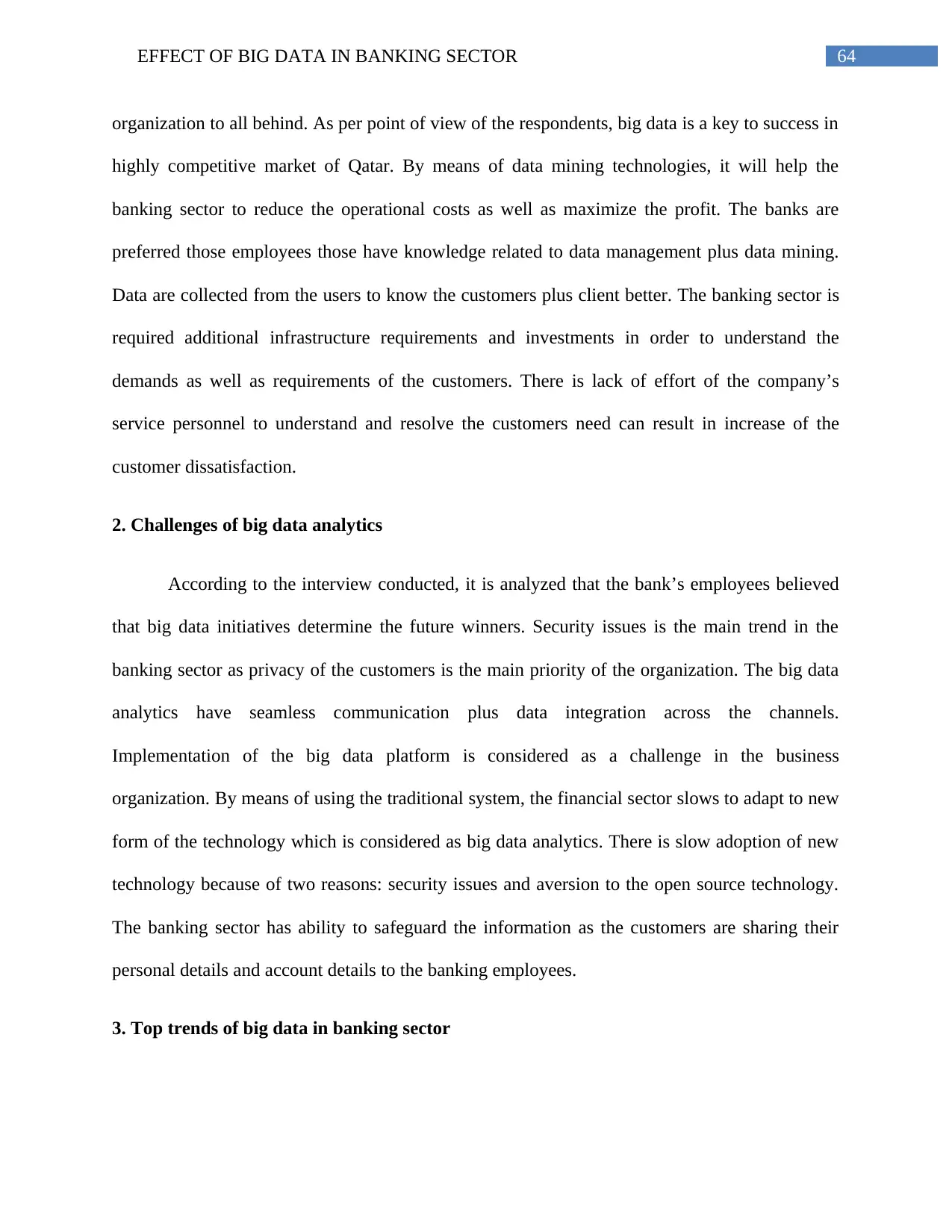
64EFFECT OF BIG DATA IN BANKING SECTOR
organization to all behind. As per point of view of the respondents, big data is a key to success in
highly competitive market of Qatar. By means of data mining technologies, it will help the
banking sector to reduce the operational costs as well as maximize the profit. The banks are
preferred those employees those have knowledge related to data management plus data mining.
Data are collected from the users to know the customers plus client better. The banking sector is
required additional infrastructure requirements and investments in order to understand the
demands as well as requirements of the customers. There is lack of effort of the company’s
service personnel to understand and resolve the customers need can result in increase of the
customer dissatisfaction.
2. Challenges of big data analytics
According to the interview conducted, it is analyzed that the bank’s employees believed
that big data initiatives determine the future winners. Security issues is the main trend in the
banking sector as privacy of the customers is the main priority of the organization. The big data
analytics have seamless communication plus data integration across the channels.
Implementation of the big data platform is considered as a challenge in the business
organization. By means of using the traditional system, the financial sector slows to adapt to new
form of the technology which is considered as big data analytics. There is slow adoption of new
technology because of two reasons: security issues and aversion to the open source technology.
The banking sector has ability to safeguard the information as the customers are sharing their
personal details and account details to the banking employees.
3. Top trends of big data in banking sector
organization to all behind. As per point of view of the respondents, big data is a key to success in
highly competitive market of Qatar. By means of data mining technologies, it will help the
banking sector to reduce the operational costs as well as maximize the profit. The banks are
preferred those employees those have knowledge related to data management plus data mining.
Data are collected from the users to know the customers plus client better. The banking sector is
required additional infrastructure requirements and investments in order to understand the
demands as well as requirements of the customers. There is lack of effort of the company’s
service personnel to understand and resolve the customers need can result in increase of the
customer dissatisfaction.
2. Challenges of big data analytics
According to the interview conducted, it is analyzed that the bank’s employees believed
that big data initiatives determine the future winners. Security issues is the main trend in the
banking sector as privacy of the customers is the main priority of the organization. The big data
analytics have seamless communication plus data integration across the channels.
Implementation of the big data platform is considered as a challenge in the business
organization. By means of using the traditional system, the financial sector slows to adapt to new
form of the technology which is considered as big data analytics. There is slow adoption of new
technology because of two reasons: security issues and aversion to the open source technology.
The banking sector has ability to safeguard the information as the customers are sharing their
personal details and account details to the banking employees.
3. Top trends of big data in banking sector
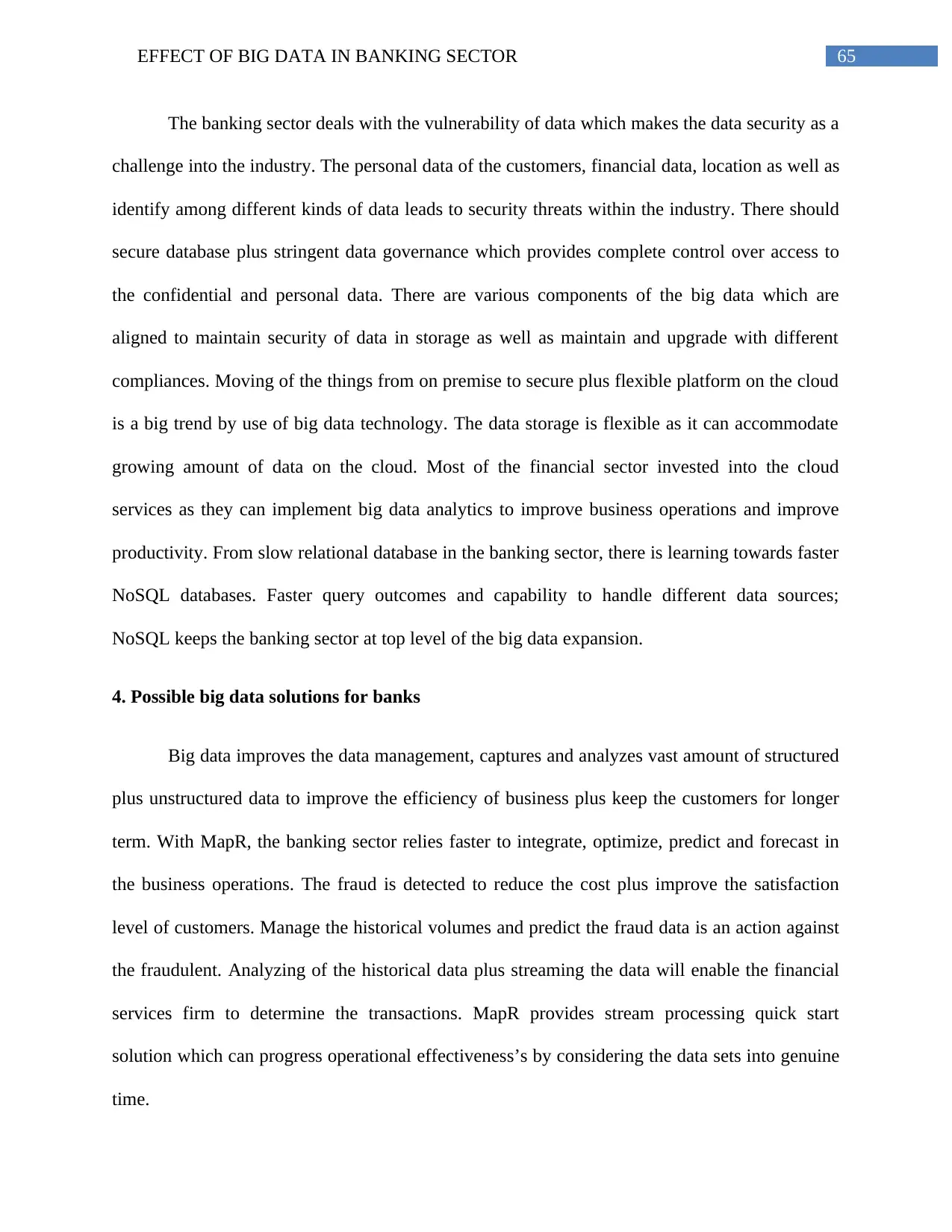
65EFFECT OF BIG DATA IN BANKING SECTOR
The banking sector deals with the vulnerability of data which makes the data security as a
challenge into the industry. The personal data of the customers, financial data, location as well as
identify among different kinds of data leads to security threats within the industry. There should
secure database plus stringent data governance which provides complete control over access to
the confidential and personal data. There are various components of the big data which are
aligned to maintain security of data in storage as well as maintain and upgrade with different
compliances. Moving of the things from on premise to secure plus flexible platform on the cloud
is a big trend by use of big data technology. The data storage is flexible as it can accommodate
growing amount of data on the cloud. Most of the financial sector invested into the cloud
services as they can implement big data analytics to improve business operations and improve
productivity. From slow relational database in the banking sector, there is learning towards faster
NoSQL databases. Faster query outcomes and capability to handle different data sources;
NoSQL keeps the banking sector at top level of the big data expansion.
4. Possible big data solutions for banks
Big data improves the data management, captures and analyzes vast amount of structured
plus unstructured data to improve the efficiency of business plus keep the customers for longer
term. With MapR, the banking sector relies faster to integrate, optimize, predict and forecast in
the business operations. The fraud is detected to reduce the cost plus improve the satisfaction
level of customers. Manage the historical volumes and predict the fraud data is an action against
the fraudulent. Analyzing of the historical data plus streaming the data will enable the financial
services firm to determine the transactions. MapR provides stream processing quick start
solution which can progress operational effectiveness’s by considering the data sets into genuine
time.
The banking sector deals with the vulnerability of data which makes the data security as a
challenge into the industry. The personal data of the customers, financial data, location as well as
identify among different kinds of data leads to security threats within the industry. There should
secure database plus stringent data governance which provides complete control over access to
the confidential and personal data. There are various components of the big data which are
aligned to maintain security of data in storage as well as maintain and upgrade with different
compliances. Moving of the things from on premise to secure plus flexible platform on the cloud
is a big trend by use of big data technology. The data storage is flexible as it can accommodate
growing amount of data on the cloud. Most of the financial sector invested into the cloud
services as they can implement big data analytics to improve business operations and improve
productivity. From slow relational database in the banking sector, there is learning towards faster
NoSQL databases. Faster query outcomes and capability to handle different data sources;
NoSQL keeps the banking sector at top level of the big data expansion.
4. Possible big data solutions for banks
Big data improves the data management, captures and analyzes vast amount of structured
plus unstructured data to improve the efficiency of business plus keep the customers for longer
term. With MapR, the banking sector relies faster to integrate, optimize, predict and forecast in
the business operations. The fraud is detected to reduce the cost plus improve the satisfaction
level of customers. Manage the historical volumes and predict the fraud data is an action against
the fraudulent. Analyzing of the historical data plus streaming the data will enable the financial
services firm to determine the transactions. MapR provides stream processing quick start
solution which can progress operational effectiveness’s by considering the data sets into genuine
time.
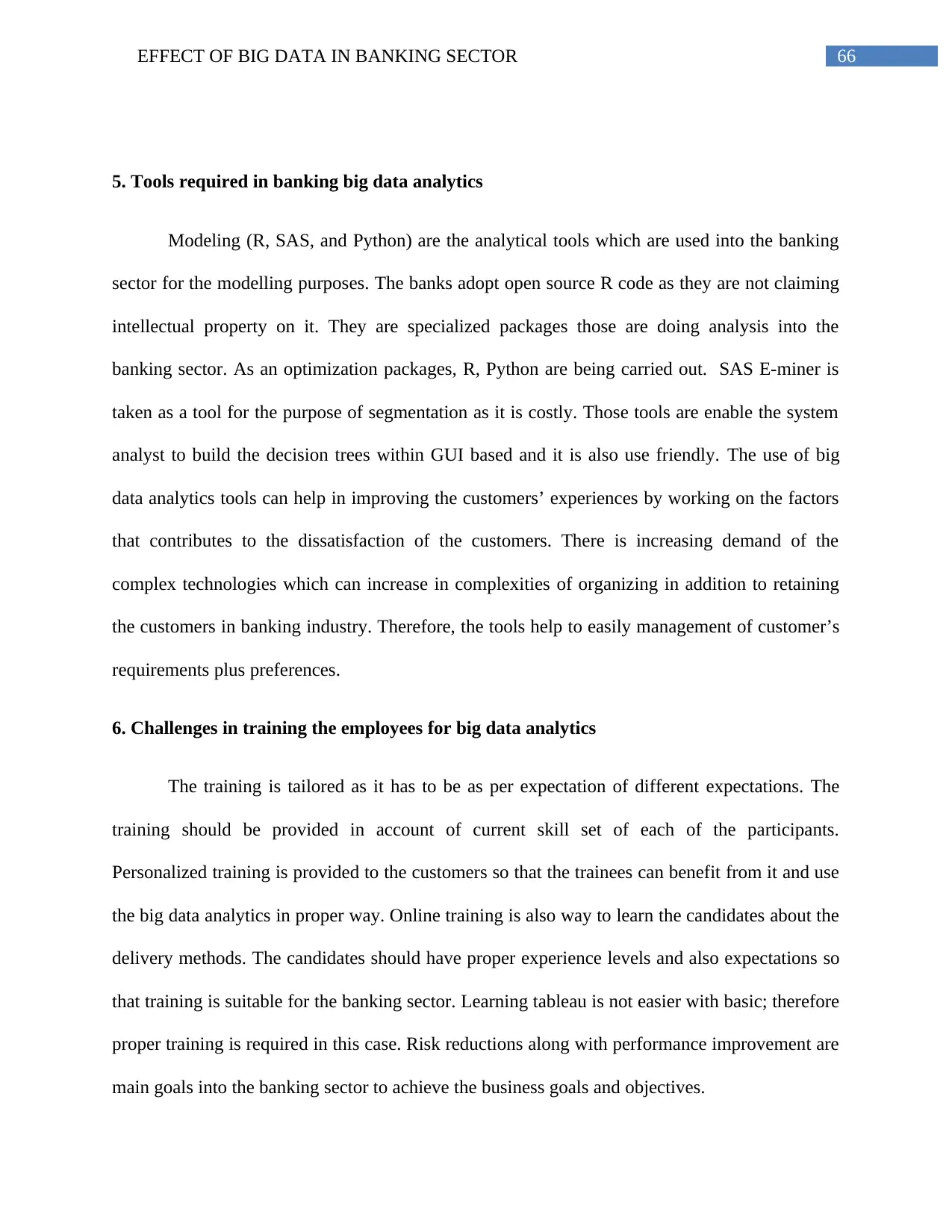
66EFFECT OF BIG DATA IN BANKING SECTOR
5. Tools required in banking big data analytics
Modeling (R, SAS, and Python) are the analytical tools which are used into the banking
sector for the modelling purposes. The banks adopt open source R code as they are not claiming
intellectual property on it. They are specialized packages those are doing analysis into the
banking sector. As an optimization packages, R, Python are being carried out. SAS E-miner is
taken as a tool for the purpose of segmentation as it is costly. Those tools are enable the system
analyst to build the decision trees within GUI based and it is also use friendly. The use of big
data analytics tools can help in improving the customers’ experiences by working on the factors
that contributes to the dissatisfaction of the customers. There is increasing demand of the
complex technologies which can increase in complexities of organizing in addition to retaining
the customers in banking industry. Therefore, the tools help to easily management of customer’s
requirements plus preferences.
6. Challenges in training the employees for big data analytics
The training is tailored as it has to be as per expectation of different expectations. The
training should be provided in account of current skill set of each of the participants.
Personalized training is provided to the customers so that the trainees can benefit from it and use
the big data analytics in proper way. Online training is also way to learn the candidates about the
delivery methods. The candidates should have proper experience levels and also expectations so
that training is suitable for the banking sector. Learning tableau is not easier with basic; therefore
proper training is required in this case. Risk reductions along with performance improvement are
main goals into the banking sector to achieve the business goals and objectives.
5. Tools required in banking big data analytics
Modeling (R, SAS, and Python) are the analytical tools which are used into the banking
sector for the modelling purposes. The banks adopt open source R code as they are not claiming
intellectual property on it. They are specialized packages those are doing analysis into the
banking sector. As an optimization packages, R, Python are being carried out. SAS E-miner is
taken as a tool for the purpose of segmentation as it is costly. Those tools are enable the system
analyst to build the decision trees within GUI based and it is also use friendly. The use of big
data analytics tools can help in improving the customers’ experiences by working on the factors
that contributes to the dissatisfaction of the customers. There is increasing demand of the
complex technologies which can increase in complexities of organizing in addition to retaining
the customers in banking industry. Therefore, the tools help to easily management of customer’s
requirements plus preferences.
6. Challenges in training the employees for big data analytics
The training is tailored as it has to be as per expectation of different expectations. The
training should be provided in account of current skill set of each of the participants.
Personalized training is provided to the customers so that the trainees can benefit from it and use
the big data analytics in proper way. Online training is also way to learn the candidates about the
delivery methods. The candidates should have proper experience levels and also expectations so
that training is suitable for the banking sector. Learning tableau is not easier with basic; therefore
proper training is required in this case. Risk reductions along with performance improvement are
main goals into the banking sector to achieve the business goals and objectives.
Secure Best Marks with AI Grader
Need help grading? Try our AI Grader for instant feedback on your assignments.
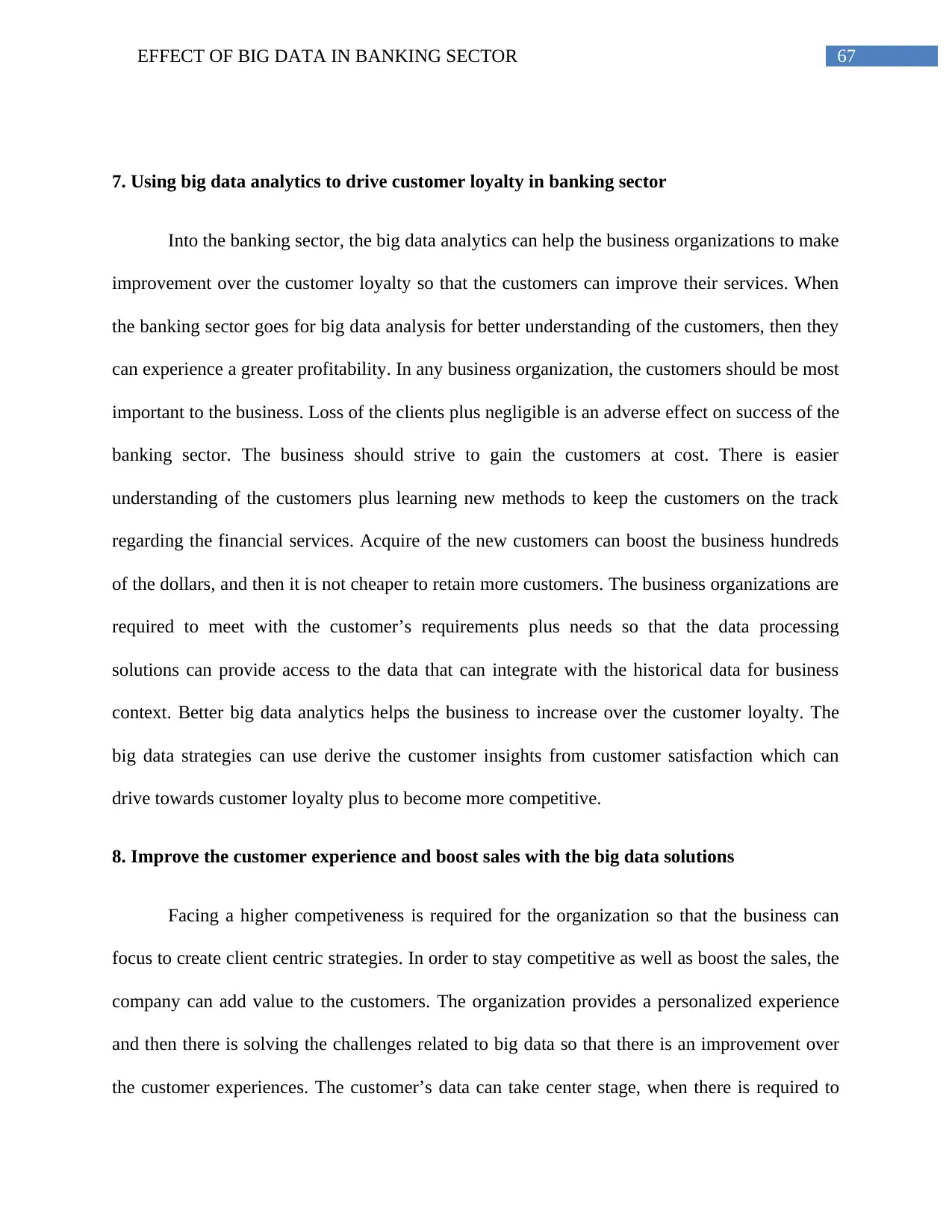
67EFFECT OF BIG DATA IN BANKING SECTOR
7. Using big data analytics to drive customer loyalty in banking sector
Into the banking sector, the big data analytics can help the business organizations to make
improvement over the customer loyalty so that the customers can improve their services. When
the banking sector goes for big data analysis for better understanding of the customers, then they
can experience a greater profitability. In any business organization, the customers should be most
important to the business. Loss of the clients plus negligible is an adverse effect on success of the
banking sector. The business should strive to gain the customers at cost. There is easier
understanding of the customers plus learning new methods to keep the customers on the track
regarding the financial services. Acquire of the new customers can boost the business hundreds
of the dollars, and then it is not cheaper to retain more customers. The business organizations are
required to meet with the customer’s requirements plus needs so that the data processing
solutions can provide access to the data that can integrate with the historical data for business
context. Better big data analytics helps the business to increase over the customer loyalty. The
big data strategies can use derive the customer insights from customer satisfaction which can
drive towards customer loyalty plus to become more competitive.
8. Improve the customer experience and boost sales with the big data solutions
Facing a higher competiveness is required for the organization so that the business can
focus to create client centric strategies. In order to stay competitive as well as boost the sales, the
company can add value to the customers. The organization provides a personalized experience
and then there is solving the challenges related to big data so that there is an improvement over
the customer experiences. The customer’s data can take center stage, when there is required to
7. Using big data analytics to drive customer loyalty in banking sector
Into the banking sector, the big data analytics can help the business organizations to make
improvement over the customer loyalty so that the customers can improve their services. When
the banking sector goes for big data analysis for better understanding of the customers, then they
can experience a greater profitability. In any business organization, the customers should be most
important to the business. Loss of the clients plus negligible is an adverse effect on success of the
banking sector. The business should strive to gain the customers at cost. There is easier
understanding of the customers plus learning new methods to keep the customers on the track
regarding the financial services. Acquire of the new customers can boost the business hundreds
of the dollars, and then it is not cheaper to retain more customers. The business organizations are
required to meet with the customer’s requirements plus needs so that the data processing
solutions can provide access to the data that can integrate with the historical data for business
context. Better big data analytics helps the business to increase over the customer loyalty. The
big data strategies can use derive the customer insights from customer satisfaction which can
drive towards customer loyalty plus to become more competitive.
8. Improve the customer experience and boost sales with the big data solutions
Facing a higher competiveness is required for the organization so that the business can
focus to create client centric strategies. In order to stay competitive as well as boost the sales, the
company can add value to the customers. The organization provides a personalized experience
and then there is solving the challenges related to big data so that there is an improvement over
the customer experiences. The customer’s data can take center stage, when there is required to
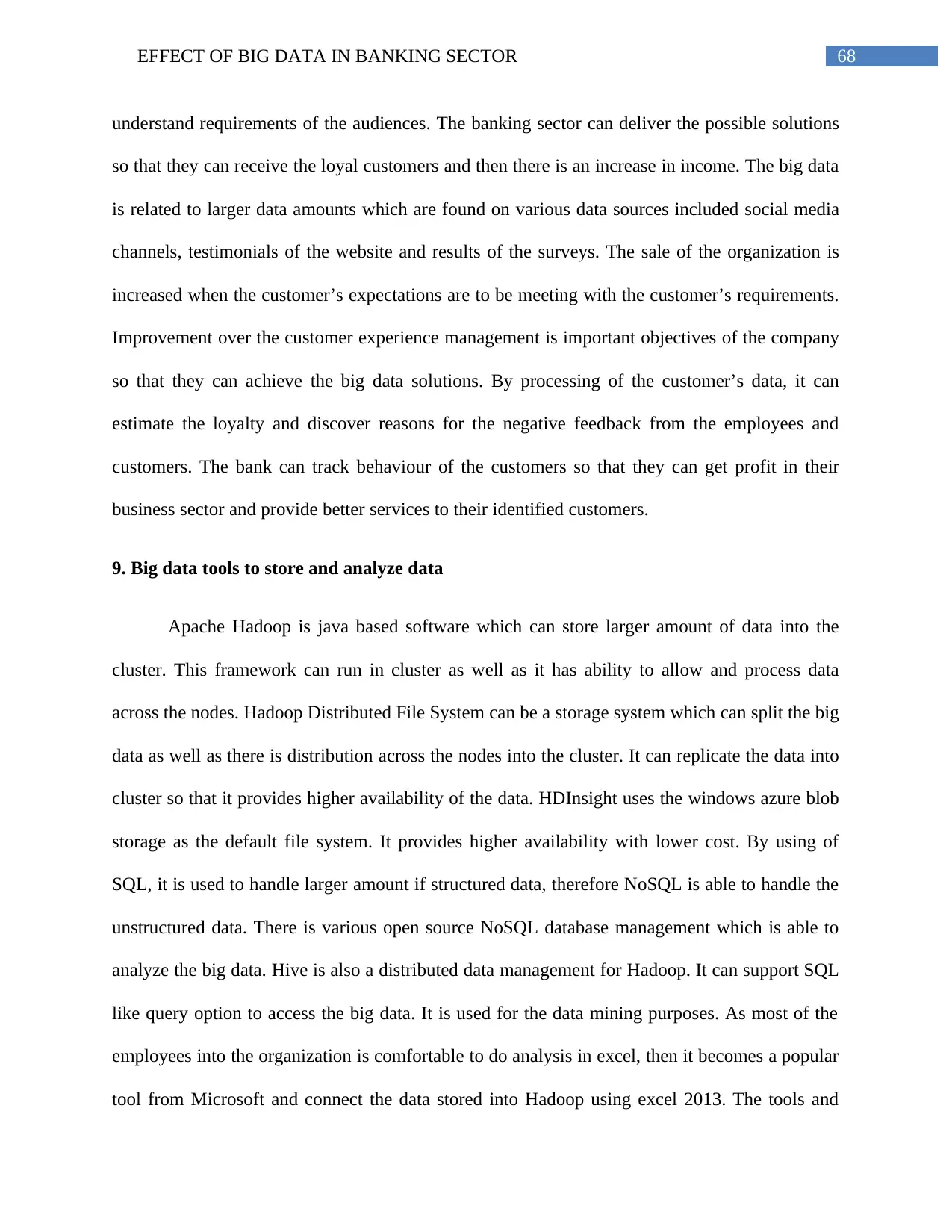
68EFFECT OF BIG DATA IN BANKING SECTOR
understand requirements of the audiences. The banking sector can deliver the possible solutions
so that they can receive the loyal customers and then there is an increase in income. The big data
is related to larger data amounts which are found on various data sources included social media
channels, testimonials of the website and results of the surveys. The sale of the organization is
increased when the customer’s expectations are to be meeting with the customer’s requirements.
Improvement over the customer experience management is important objectives of the company
so that they can achieve the big data solutions. By processing of the customer’s data, it can
estimate the loyalty and discover reasons for the negative feedback from the employees and
customers. The bank can track behaviour of the customers so that they can get profit in their
business sector and provide better services to their identified customers.
9. Big data tools to store and analyze data
Apache Hadoop is java based software which can store larger amount of data into the
cluster. This framework can run in cluster as well as it has ability to allow and process data
across the nodes. Hadoop Distributed File System can be a storage system which can split the big
data as well as there is distribution across the nodes into the cluster. It can replicate the data into
cluster so that it provides higher availability of the data. HDInsight uses the windows azure blob
storage as the default file system. It provides higher availability with lower cost. By using of
SQL, it is used to handle larger amount if structured data, therefore NoSQL is able to handle the
unstructured data. There is various open source NoSQL database management which is able to
analyze the big data. Hive is also a distributed data management for Hadoop. It can support SQL
like query option to access the big data. It is used for the data mining purposes. As most of the
employees into the organization is comfortable to do analysis in excel, then it becomes a popular
tool from Microsoft and connect the data stored into Hadoop using excel 2013. The tools and
understand requirements of the audiences. The banking sector can deliver the possible solutions
so that they can receive the loyal customers and then there is an increase in income. The big data
is related to larger data amounts which are found on various data sources included social media
channels, testimonials of the website and results of the surveys. The sale of the organization is
increased when the customer’s expectations are to be meeting with the customer’s requirements.
Improvement over the customer experience management is important objectives of the company
so that they can achieve the big data solutions. By processing of the customer’s data, it can
estimate the loyalty and discover reasons for the negative feedback from the employees and
customers. The bank can track behaviour of the customers so that they can get profit in their
business sector and provide better services to their identified customers.
9. Big data tools to store and analyze data
Apache Hadoop is java based software which can store larger amount of data into the
cluster. This framework can run in cluster as well as it has ability to allow and process data
across the nodes. Hadoop Distributed File System can be a storage system which can split the big
data as well as there is distribution across the nodes into the cluster. It can replicate the data into
cluster so that it provides higher availability of the data. HDInsight uses the windows azure blob
storage as the default file system. It provides higher availability with lower cost. By using of
SQL, it is used to handle larger amount if structured data, therefore NoSQL is able to handle the
unstructured data. There is various open source NoSQL database management which is able to
analyze the big data. Hive is also a distributed data management for Hadoop. It can support SQL
like query option to access the big data. It is used for the data mining purposes. As most of the
employees into the organization is comfortable to do analysis in excel, then it becomes a popular
tool from Microsoft and connect the data stored into Hadoop using excel 2013. The tools and
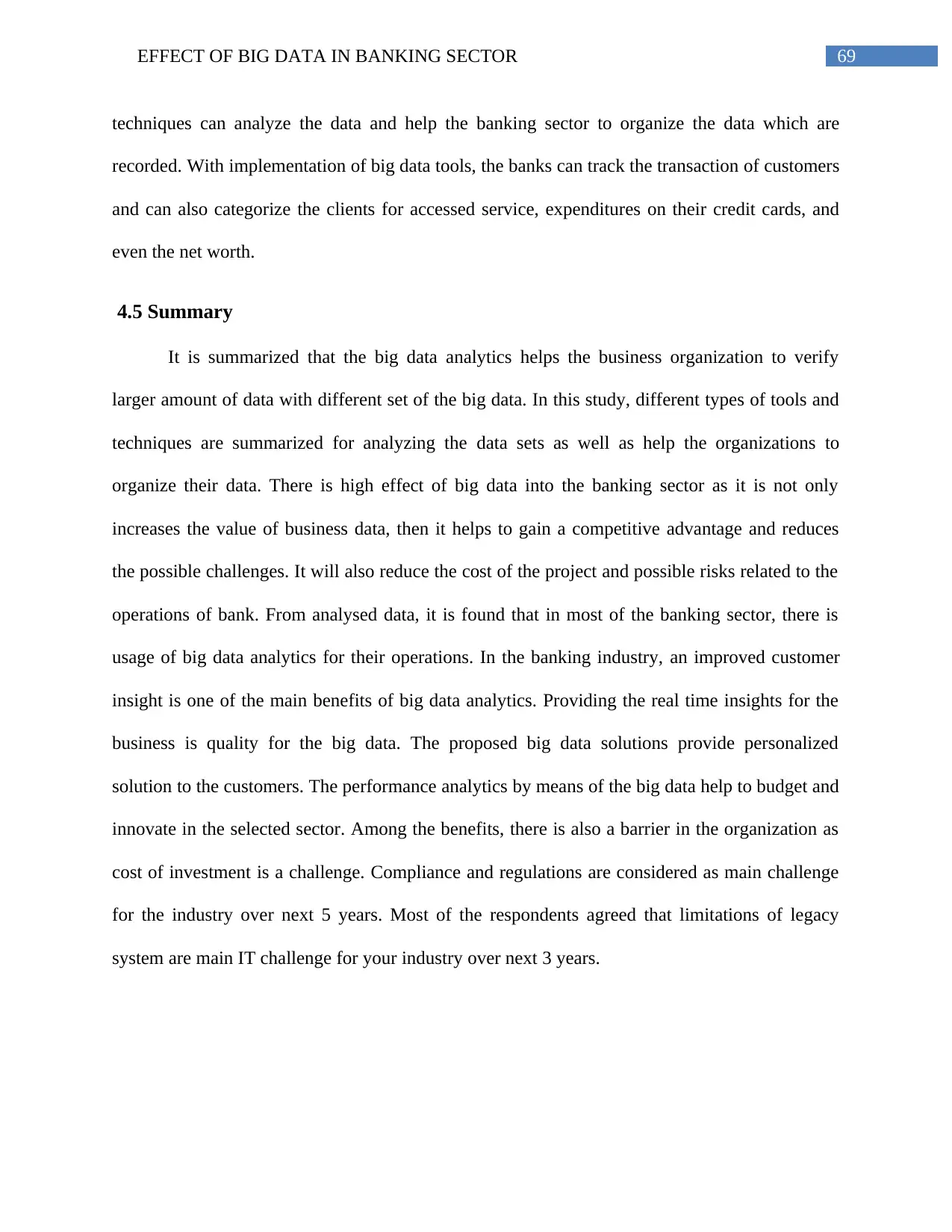
69EFFECT OF BIG DATA IN BANKING SECTOR
techniques can analyze the data and help the banking sector to organize the data which are
recorded. With implementation of big data tools, the banks can track the transaction of customers
and can also categorize the clients for accessed service, expenditures on their credit cards, and
even the net worth.
4.5 Summary
It is summarized that the big data analytics helps the business organization to verify
larger amount of data with different set of the big data. In this study, different types of tools and
techniques are summarized for analyzing the data sets as well as help the organizations to
organize their data. There is high effect of big data into the banking sector as it is not only
increases the value of business data, then it helps to gain a competitive advantage and reduces
the possible challenges. It will also reduce the cost of the project and possible risks related to the
operations of bank. From analysed data, it is found that in most of the banking sector, there is
usage of big data analytics for their operations. In the banking industry, an improved customer
insight is one of the main benefits of big data analytics. Providing the real time insights for the
business is quality for the big data. The proposed big data solutions provide personalized
solution to the customers. The performance analytics by means of the big data help to budget and
innovate in the selected sector. Among the benefits, there is also a barrier in the organization as
cost of investment is a challenge. Compliance and regulations are considered as main challenge
for the industry over next 5 years. Most of the respondents agreed that limitations of legacy
system are main IT challenge for your industry over next 3 years.
techniques can analyze the data and help the banking sector to organize the data which are
recorded. With implementation of big data tools, the banks can track the transaction of customers
and can also categorize the clients for accessed service, expenditures on their credit cards, and
even the net worth.
4.5 Summary
It is summarized that the big data analytics helps the business organization to verify
larger amount of data with different set of the big data. In this study, different types of tools and
techniques are summarized for analyzing the data sets as well as help the organizations to
organize their data. There is high effect of big data into the banking sector as it is not only
increases the value of business data, then it helps to gain a competitive advantage and reduces
the possible challenges. It will also reduce the cost of the project and possible risks related to the
operations of bank. From analysed data, it is found that in most of the banking sector, there is
usage of big data analytics for their operations. In the banking industry, an improved customer
insight is one of the main benefits of big data analytics. Providing the real time insights for the
business is quality for the big data. The proposed big data solutions provide personalized
solution to the customers. The performance analytics by means of the big data help to budget and
innovate in the selected sector. Among the benefits, there is also a barrier in the organization as
cost of investment is a challenge. Compliance and regulations are considered as main challenge
for the industry over next 5 years. Most of the respondents agreed that limitations of legacy
system are main IT challenge for your industry over next 3 years.
Paraphrase This Document
Need a fresh take? Get an instant paraphrase of this document with our AI Paraphraser
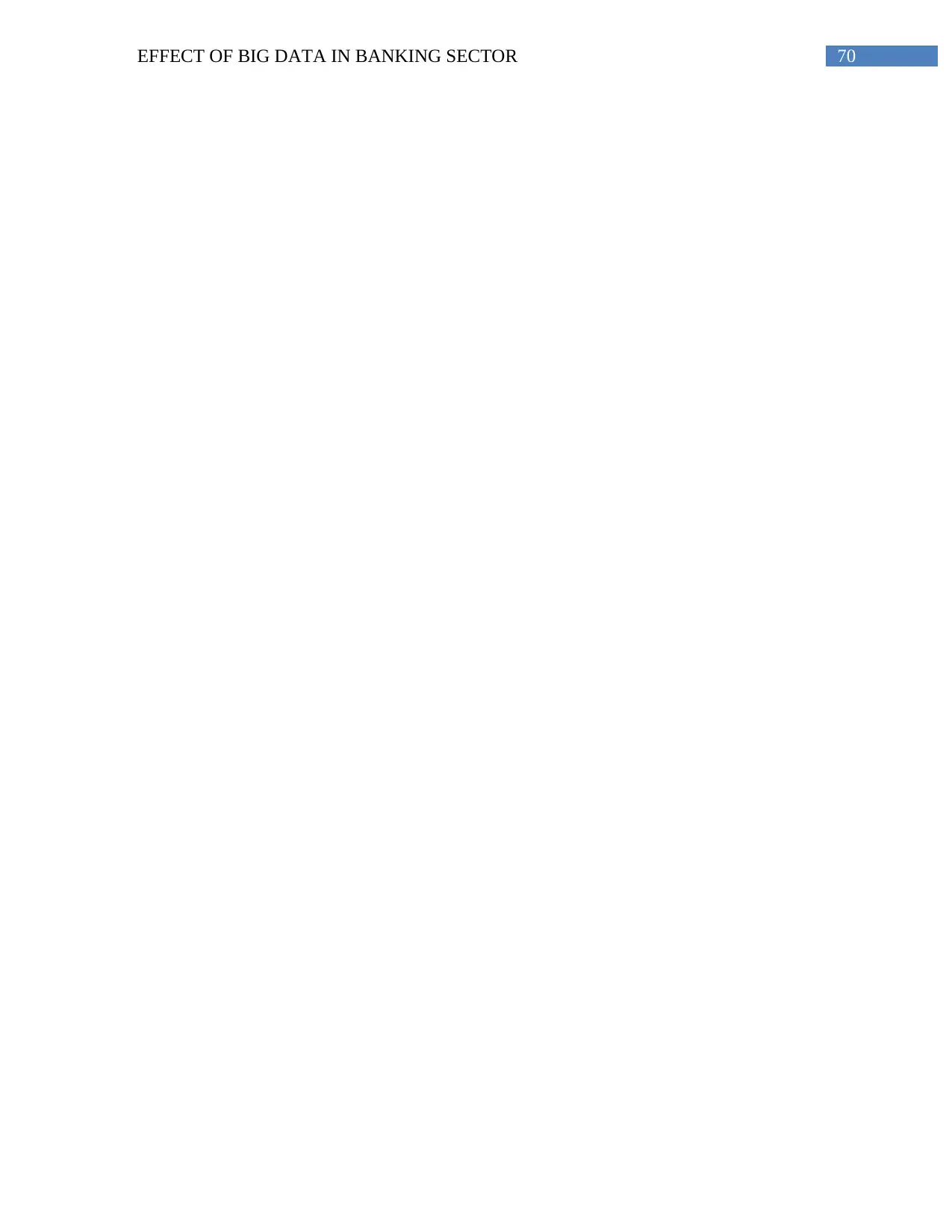
70EFFECT OF BIG DATA IN BANKING SECTOR
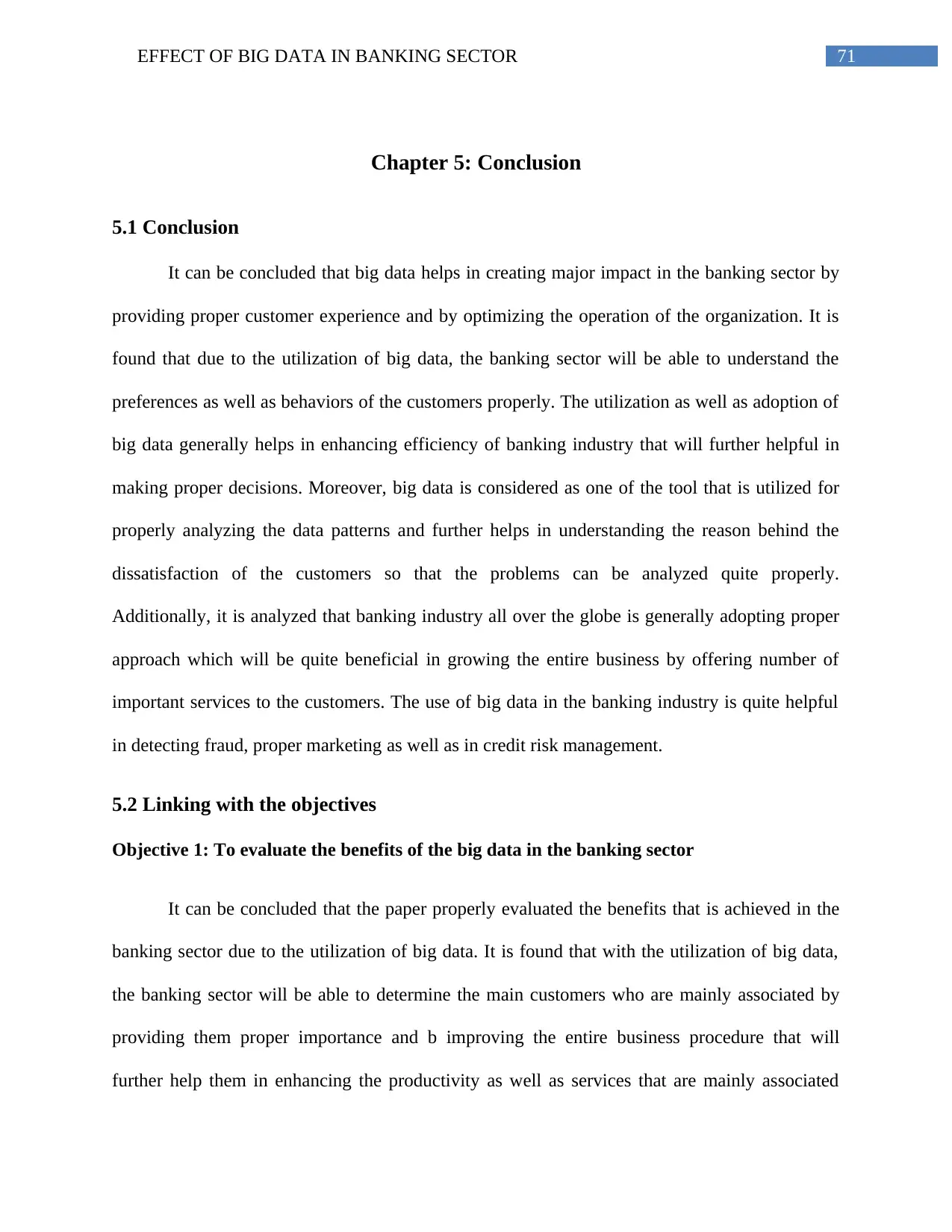
71EFFECT OF BIG DATA IN BANKING SECTOR
Chapter 5: Conclusion
5.1 Conclusion
It can be concluded that big data helps in creating major impact in the banking sector by
providing proper customer experience and by optimizing the operation of the organization. It is
found that due to the utilization of big data, the banking sector will be able to understand the
preferences as well as behaviors of the customers properly. The utilization as well as adoption of
big data generally helps in enhancing efficiency of banking industry that will further helpful in
making proper decisions. Moreover, big data is considered as one of the tool that is utilized for
properly analyzing the data patterns and further helps in understanding the reason behind the
dissatisfaction of the customers so that the problems can be analyzed quite properly.
Additionally, it is analyzed that banking industry all over the globe is generally adopting proper
approach which will be quite beneficial in growing the entire business by offering number of
important services to the customers. The use of big data in the banking industry is quite helpful
in detecting fraud, proper marketing as well as in credit risk management.
5.2 Linking with the objectives
Objective 1: To evaluate the benefits of the big data in the banking sector
It can be concluded that the paper properly evaluated the benefits that is achieved in the
banking sector due to the utilization of big data. It is found that with the utilization of big data,
the banking sector will be able to determine the main customers who are mainly associated by
providing them proper importance and b improving the entire business procedure that will
further help them in enhancing the productivity as well as services that are mainly associated
Chapter 5: Conclusion
5.1 Conclusion
It can be concluded that big data helps in creating major impact in the banking sector by
providing proper customer experience and by optimizing the operation of the organization. It is
found that due to the utilization of big data, the banking sector will be able to understand the
preferences as well as behaviors of the customers properly. The utilization as well as adoption of
big data generally helps in enhancing efficiency of banking industry that will further helpful in
making proper decisions. Moreover, big data is considered as one of the tool that is utilized for
properly analyzing the data patterns and further helps in understanding the reason behind the
dissatisfaction of the customers so that the problems can be analyzed quite properly.
Additionally, it is analyzed that banking industry all over the globe is generally adopting proper
approach which will be quite beneficial in growing the entire business by offering number of
important services to the customers. The use of big data in the banking industry is quite helpful
in detecting fraud, proper marketing as well as in credit risk management.
5.2 Linking with the objectives
Objective 1: To evaluate the benefits of the big data in the banking sector
It can be concluded that the paper properly evaluated the benefits that is achieved in the
banking sector due to the utilization of big data. It is found that with the utilization of big data,
the banking sector will be able to determine the main customers who are mainly associated by
providing them proper importance and b improving the entire business procedure that will
further help them in enhancing the productivity as well as services that are mainly associated
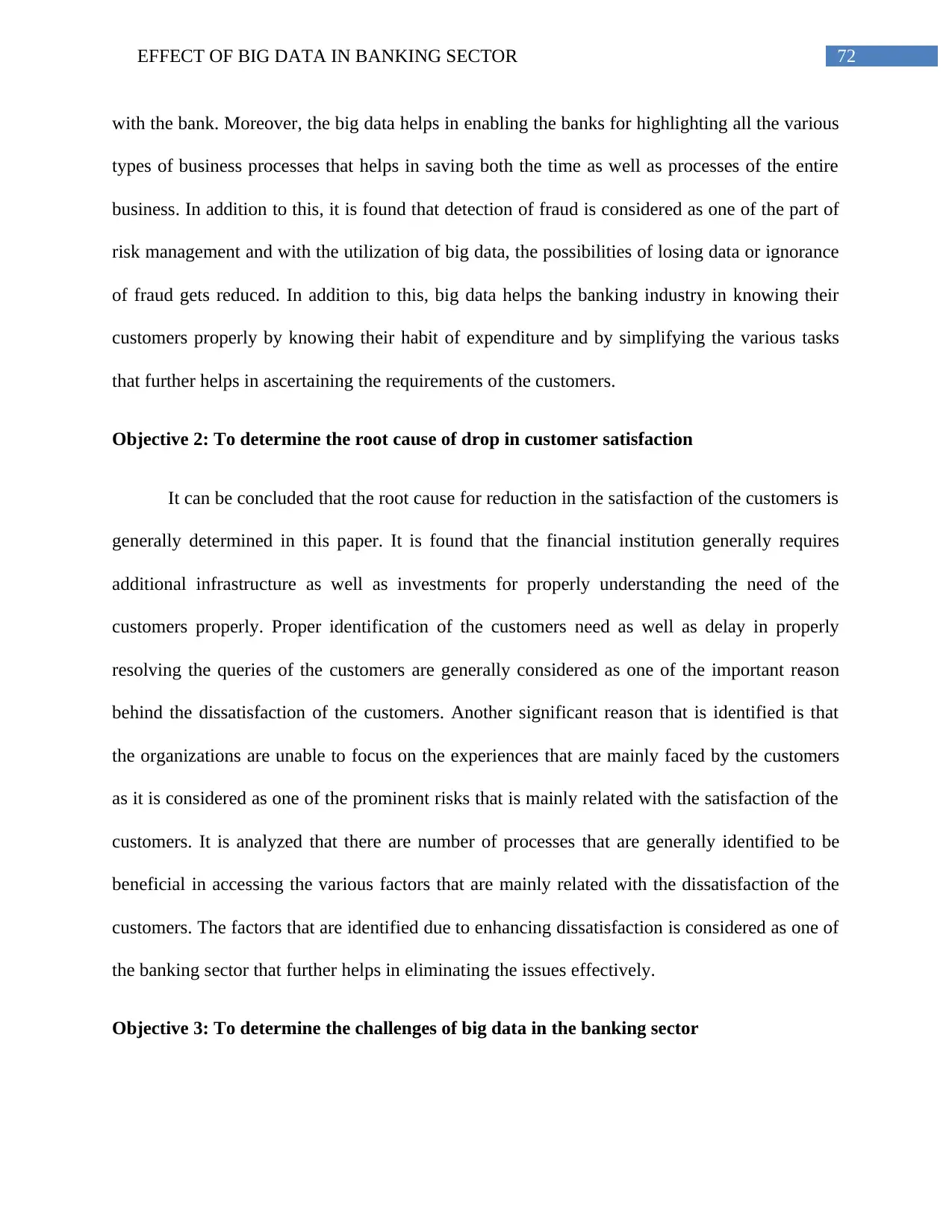
72EFFECT OF BIG DATA IN BANKING SECTOR
with the bank. Moreover, the big data helps in enabling the banks for highlighting all the various
types of business processes that helps in saving both the time as well as processes of the entire
business. In addition to this, it is found that detection of fraud is considered as one of the part of
risk management and with the utilization of big data, the possibilities of losing data or ignorance
of fraud gets reduced. In addition to this, big data helps the banking industry in knowing their
customers properly by knowing their habit of expenditure and by simplifying the various tasks
that further helps in ascertaining the requirements of the customers.
Objective 2: To determine the root cause of drop in customer satisfaction
It can be concluded that the root cause for reduction in the satisfaction of the customers is
generally determined in this paper. It is found that the financial institution generally requires
additional infrastructure as well as investments for properly understanding the need of the
customers properly. Proper identification of the customers need as well as delay in properly
resolving the queries of the customers are generally considered as one of the important reason
behind the dissatisfaction of the customers. Another significant reason that is identified is that
the organizations are unable to focus on the experiences that are mainly faced by the customers
as it is considered as one of the prominent risks that is mainly related with the satisfaction of the
customers. It is analyzed that there are number of processes that are generally identified to be
beneficial in accessing the various factors that are mainly related with the dissatisfaction of the
customers. The factors that are identified due to enhancing dissatisfaction is considered as one of
the banking sector that further helps in eliminating the issues effectively.
Objective 3: To determine the challenges of big data in the banking sector
with the bank. Moreover, the big data helps in enabling the banks for highlighting all the various
types of business processes that helps in saving both the time as well as processes of the entire
business. In addition to this, it is found that detection of fraud is considered as one of the part of
risk management and with the utilization of big data, the possibilities of losing data or ignorance
of fraud gets reduced. In addition to this, big data helps the banking industry in knowing their
customers properly by knowing their habit of expenditure and by simplifying the various tasks
that further helps in ascertaining the requirements of the customers.
Objective 2: To determine the root cause of drop in customer satisfaction
It can be concluded that the root cause for reduction in the satisfaction of the customers is
generally determined in this paper. It is found that the financial institution generally requires
additional infrastructure as well as investments for properly understanding the need of the
customers properly. Proper identification of the customers need as well as delay in properly
resolving the queries of the customers are generally considered as one of the important reason
behind the dissatisfaction of the customers. Another significant reason that is identified is that
the organizations are unable to focus on the experiences that are mainly faced by the customers
as it is considered as one of the prominent risks that is mainly related with the satisfaction of the
customers. It is analyzed that there are number of processes that are generally identified to be
beneficial in accessing the various factors that are mainly related with the dissatisfaction of the
customers. The factors that are identified due to enhancing dissatisfaction is considered as one of
the banking sector that further helps in eliminating the issues effectively.
Objective 3: To determine the challenges of big data in the banking sector
Secure Best Marks with AI Grader
Need help grading? Try our AI Grader for instant feedback on your assignments.
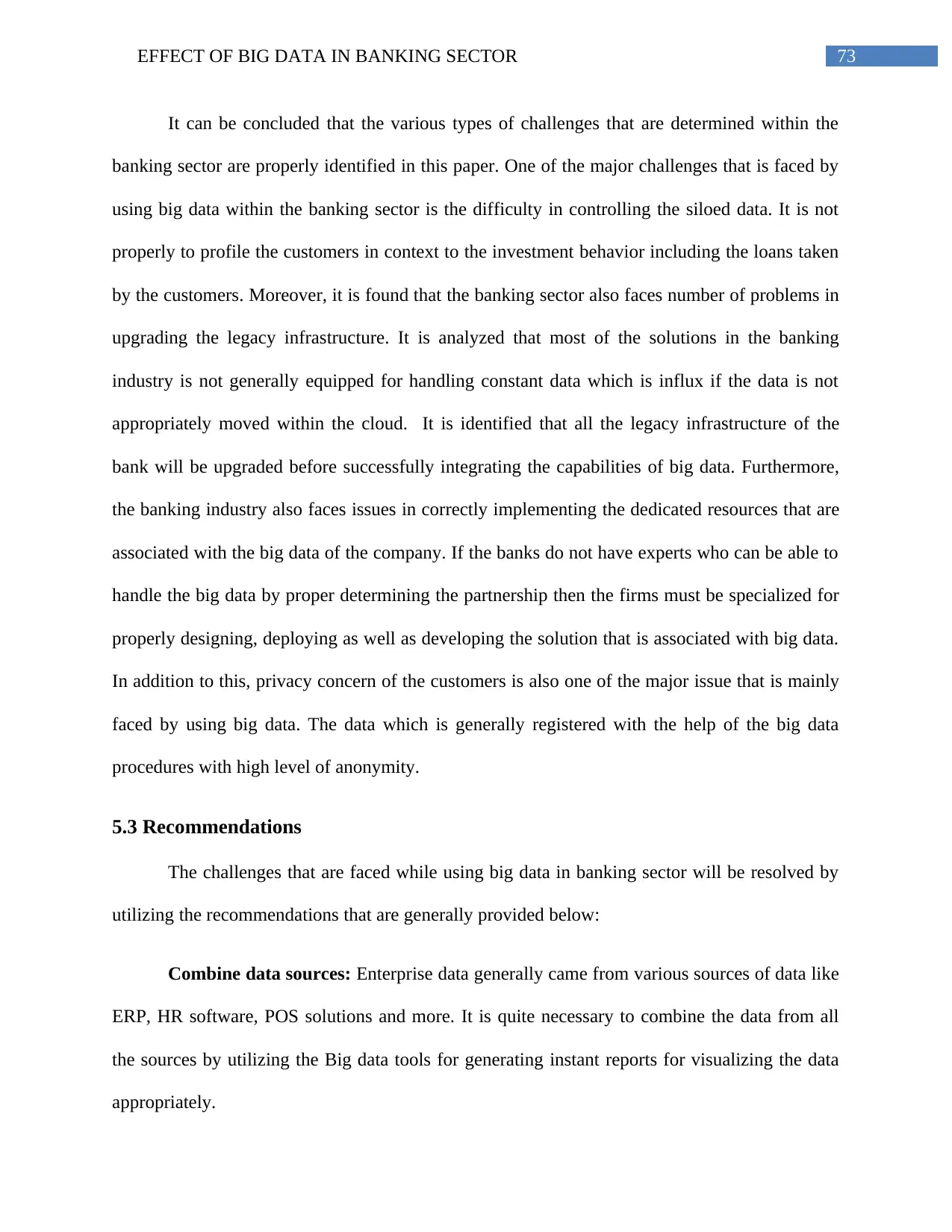
73EFFECT OF BIG DATA IN BANKING SECTOR
It can be concluded that the various types of challenges that are determined within the
banking sector are properly identified in this paper. One of the major challenges that is faced by
using big data within the banking sector is the difficulty in controlling the siloed data. It is not
properly to profile the customers in context to the investment behavior including the loans taken
by the customers. Moreover, it is found that the banking sector also faces number of problems in
upgrading the legacy infrastructure. It is analyzed that most of the solutions in the banking
industry is not generally equipped for handling constant data which is influx if the data is not
appropriately moved within the cloud. It is identified that all the legacy infrastructure of the
bank will be upgraded before successfully integrating the capabilities of big data. Furthermore,
the banking industry also faces issues in correctly implementing the dedicated resources that are
associated with the big data of the company. If the banks do not have experts who can be able to
handle the big data by proper determining the partnership then the firms must be specialized for
properly designing, deploying as well as developing the solution that is associated with big data.
In addition to this, privacy concern of the customers is also one of the major issue that is mainly
faced by using big data. The data which is generally registered with the help of the big data
procedures with high level of anonymity.
5.3 Recommendations
The challenges that are faced while using big data in banking sector will be resolved by
utilizing the recommendations that are generally provided below:
Combine data sources: Enterprise data generally came from various sources of data like
ERP, HR software, POS solutions and more. It is quite necessary to combine the data from all
the sources by utilizing the Big data tools for generating instant reports for visualizing the data
appropriately.
It can be concluded that the various types of challenges that are determined within the
banking sector are properly identified in this paper. One of the major challenges that is faced by
using big data within the banking sector is the difficulty in controlling the siloed data. It is not
properly to profile the customers in context to the investment behavior including the loans taken
by the customers. Moreover, it is found that the banking sector also faces number of problems in
upgrading the legacy infrastructure. It is analyzed that most of the solutions in the banking
industry is not generally equipped for handling constant data which is influx if the data is not
appropriately moved within the cloud. It is identified that all the legacy infrastructure of the
bank will be upgraded before successfully integrating the capabilities of big data. Furthermore,
the banking industry also faces issues in correctly implementing the dedicated resources that are
associated with the big data of the company. If the banks do not have experts who can be able to
handle the big data by proper determining the partnership then the firms must be specialized for
properly designing, deploying as well as developing the solution that is associated with big data.
In addition to this, privacy concern of the customers is also one of the major issue that is mainly
faced by using big data. The data which is generally registered with the help of the big data
procedures with high level of anonymity.
5.3 Recommendations
The challenges that are faced while using big data in banking sector will be resolved by
utilizing the recommendations that are generally provided below:
Combine data sources: Enterprise data generally came from various sources of data like
ERP, HR software, POS solutions and more. It is quite necessary to combine the data from all
the sources by utilizing the Big data tools for generating instant reports for visualizing the data
appropriately.
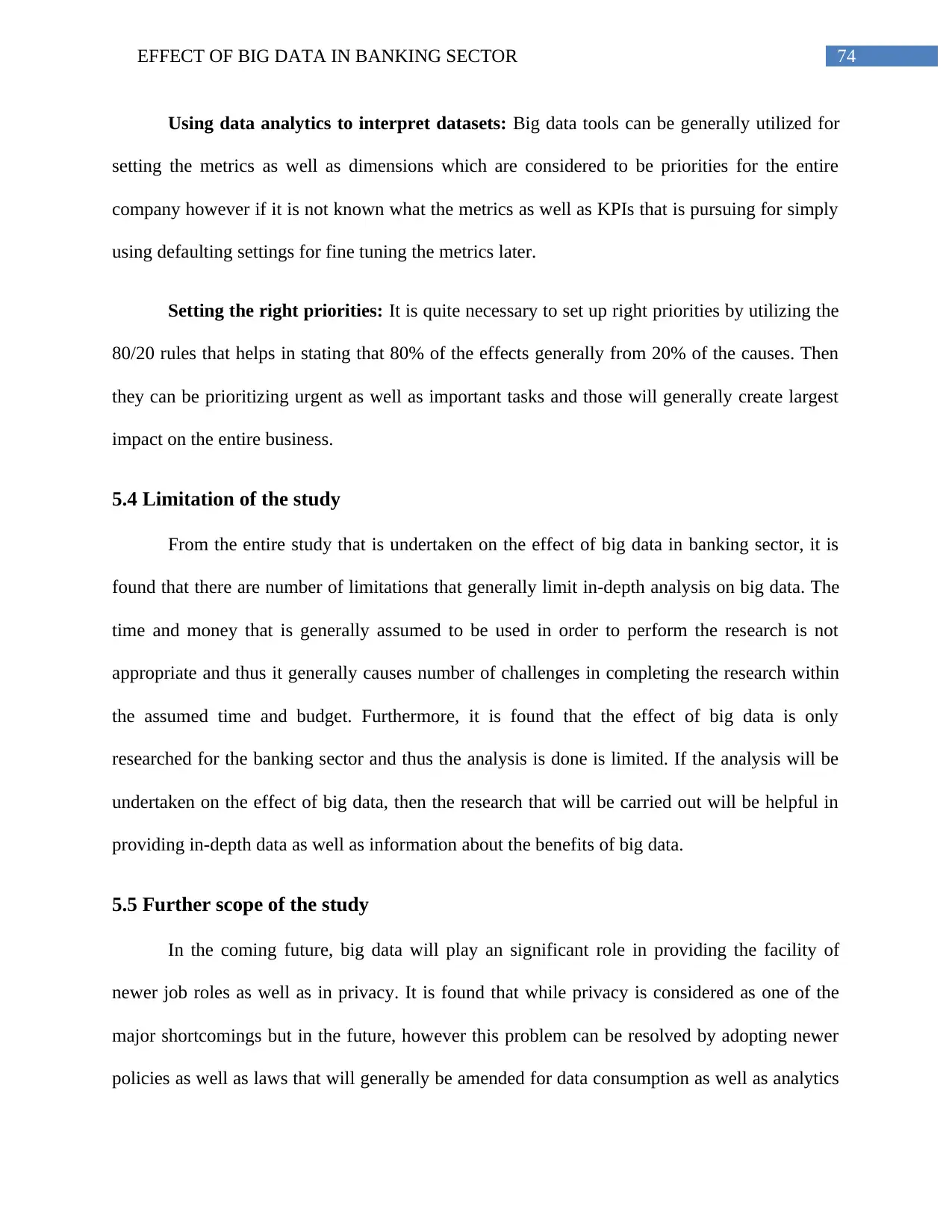
74EFFECT OF BIG DATA IN BANKING SECTOR
Using data analytics to interpret datasets: Big data tools can be generally utilized for
setting the metrics as well as dimensions which are considered to be priorities for the entire
company however if it is not known what the metrics as well as KPIs that is pursuing for simply
using defaulting settings for fine tuning the metrics later.
Setting the right priorities: It is quite necessary to set up right priorities by utilizing the
80/20 rules that helps in stating that 80% of the effects generally from 20% of the causes. Then
they can be prioritizing urgent as well as important tasks and those will generally create largest
impact on the entire business.
5.4 Limitation of the study
From the entire study that is undertaken on the effect of big data in banking sector, it is
found that there are number of limitations that generally limit in-depth analysis on big data. The
time and money that is generally assumed to be used in order to perform the research is not
appropriate and thus it generally causes number of challenges in completing the research within
the assumed time and budget. Furthermore, it is found that the effect of big data is only
researched for the banking sector and thus the analysis is done is limited. If the analysis will be
undertaken on the effect of big data, then the research that will be carried out will be helpful in
providing in-depth data as well as information about the benefits of big data.
5.5 Further scope of the study
In the coming future, big data will play an significant role in providing the facility of
newer job roles as well as in privacy. It is found that while privacy is considered as one of the
major shortcomings but in the future, however this problem can be resolved by adopting newer
policies as well as laws that will generally be amended for data consumption as well as analytics
Using data analytics to interpret datasets: Big data tools can be generally utilized for
setting the metrics as well as dimensions which are considered to be priorities for the entire
company however if it is not known what the metrics as well as KPIs that is pursuing for simply
using defaulting settings for fine tuning the metrics later.
Setting the right priorities: It is quite necessary to set up right priorities by utilizing the
80/20 rules that helps in stating that 80% of the effects generally from 20% of the causes. Then
they can be prioritizing urgent as well as important tasks and those will generally create largest
impact on the entire business.
5.4 Limitation of the study
From the entire study that is undertaken on the effect of big data in banking sector, it is
found that there are number of limitations that generally limit in-depth analysis on big data. The
time and money that is generally assumed to be used in order to perform the research is not
appropriate and thus it generally causes number of challenges in completing the research within
the assumed time and budget. Furthermore, it is found that the effect of big data is only
researched for the banking sector and thus the analysis is done is limited. If the analysis will be
undertaken on the effect of big data, then the research that will be carried out will be helpful in
providing in-depth data as well as information about the benefits of big data.
5.5 Further scope of the study
In the coming future, big data will play an significant role in providing the facility of
newer job roles as well as in privacy. It is found that while privacy is considered as one of the
major shortcomings but in the future, however this problem can be resolved by adopting newer
policies as well as laws that will generally be amended for data consumption as well as analytics
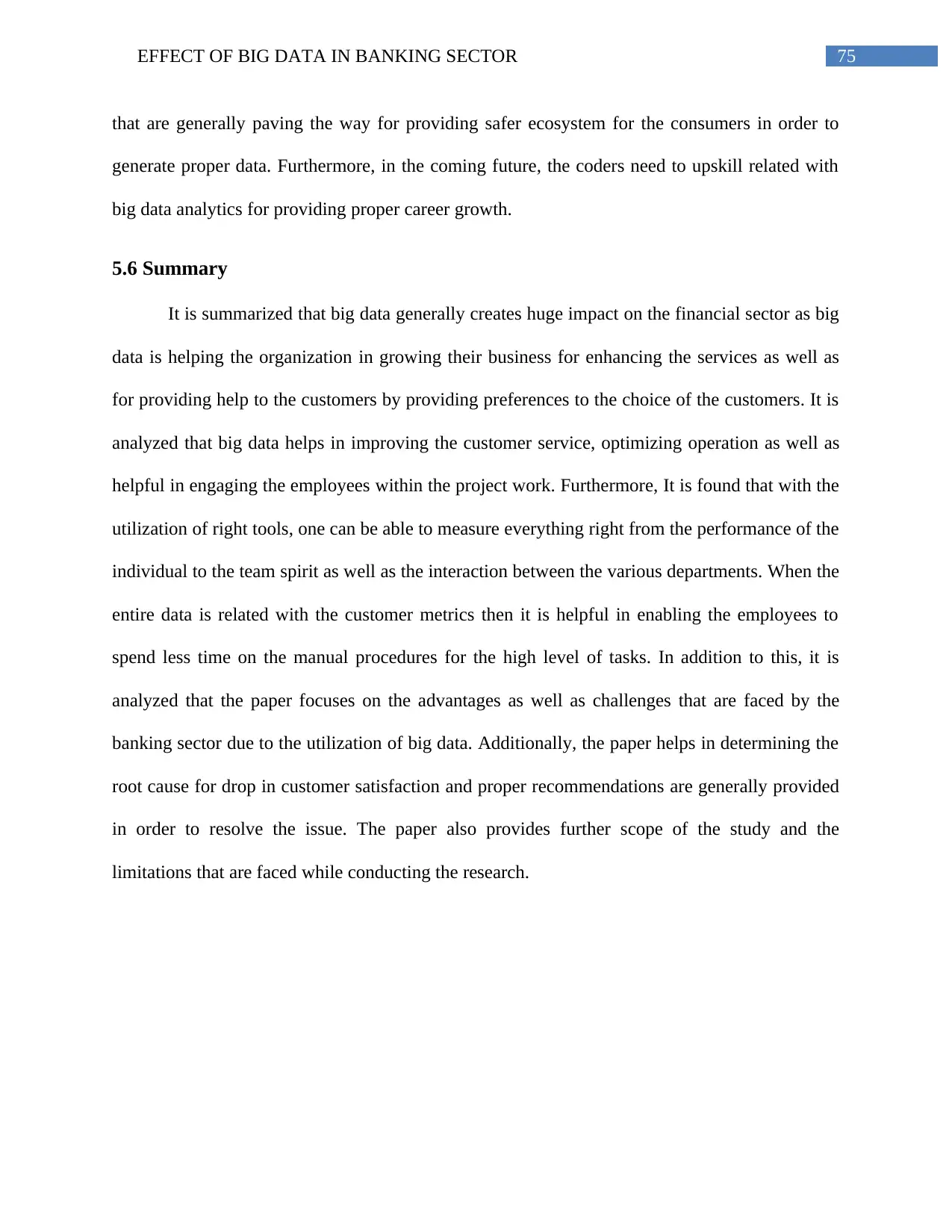
75EFFECT OF BIG DATA IN BANKING SECTOR
that are generally paving the way for providing safer ecosystem for the consumers in order to
generate proper data. Furthermore, in the coming future, the coders need to upskill related with
big data analytics for providing proper career growth.
5.6 Summary
It is summarized that big data generally creates huge impact on the financial sector as big
data is helping the organization in growing their business for enhancing the services as well as
for providing help to the customers by providing preferences to the choice of the customers. It is
analyzed that big data helps in improving the customer service, optimizing operation as well as
helpful in engaging the employees within the project work. Furthermore, It is found that with the
utilization of right tools, one can be able to measure everything right from the performance of the
individual to the team spirit as well as the interaction between the various departments. When the
entire data is related with the customer metrics then it is helpful in enabling the employees to
spend less time on the manual procedures for the high level of tasks. In addition to this, it is
analyzed that the paper focuses on the advantages as well as challenges that are faced by the
banking sector due to the utilization of big data. Additionally, the paper helps in determining the
root cause for drop in customer satisfaction and proper recommendations are generally provided
in order to resolve the issue. The paper also provides further scope of the study and the
limitations that are faced while conducting the research.
that are generally paving the way for providing safer ecosystem for the consumers in order to
generate proper data. Furthermore, in the coming future, the coders need to upskill related with
big data analytics for providing proper career growth.
5.6 Summary
It is summarized that big data generally creates huge impact on the financial sector as big
data is helping the organization in growing their business for enhancing the services as well as
for providing help to the customers by providing preferences to the choice of the customers. It is
analyzed that big data helps in improving the customer service, optimizing operation as well as
helpful in engaging the employees within the project work. Furthermore, It is found that with the
utilization of right tools, one can be able to measure everything right from the performance of the
individual to the team spirit as well as the interaction between the various departments. When the
entire data is related with the customer metrics then it is helpful in enabling the employees to
spend less time on the manual procedures for the high level of tasks. In addition to this, it is
analyzed that the paper focuses on the advantages as well as challenges that are faced by the
banking sector due to the utilization of big data. Additionally, the paper helps in determining the
root cause for drop in customer satisfaction and proper recommendations are generally provided
in order to resolve the issue. The paper also provides further scope of the study and the
limitations that are faced while conducting the research.
Paraphrase This Document
Need a fresh take? Get an instant paraphrase of this document with our AI Paraphraser
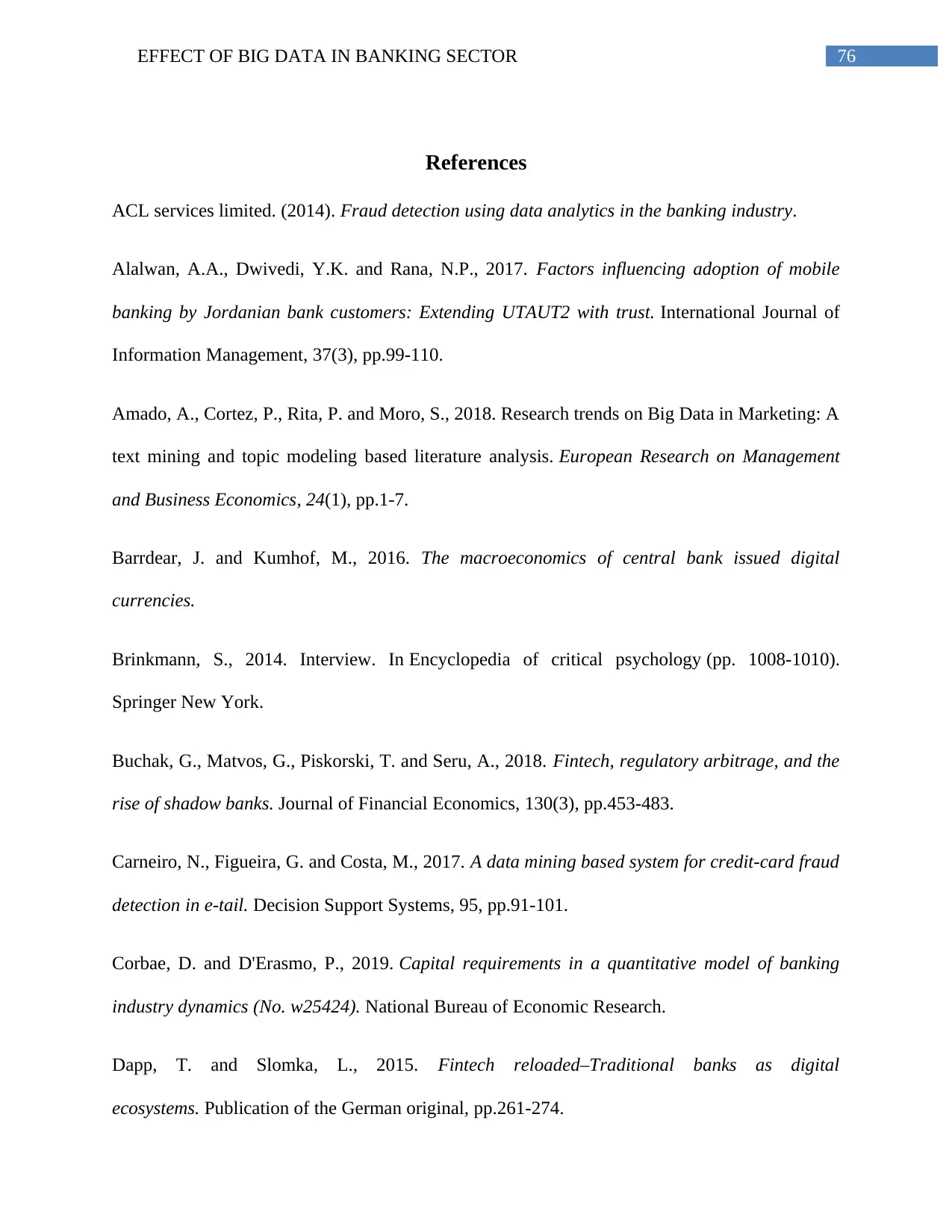
76EFFECT OF BIG DATA IN BANKING SECTOR
References
ACL services limited. (2014). Fraud detection using data analytics in the banking industry.
Alalwan, A.A., Dwivedi, Y.K. and Rana, N.P., 2017. Factors influencing adoption of mobile
banking by Jordanian bank customers: Extending UTAUT2 with trust. International Journal of
Information Management, 37(3), pp.99-110.
Amado, A., Cortez, P., Rita, P. and Moro, S., 2018. Research trends on Big Data in Marketing: A
text mining and topic modeling based literature analysis. European Research on Management
and Business Economics, 24(1), pp.1-7.
Barrdear, J. and Kumhof, M., 2016. The macroeconomics of central bank issued digital
currencies.
Brinkmann, S., 2014. Interview. In Encyclopedia of critical psychology (pp. 1008-1010).
Springer New York.
Buchak, G., Matvos, G., Piskorski, T. and Seru, A., 2018. Fintech, regulatory arbitrage, and the
rise of shadow banks. Journal of Financial Economics, 130(3), pp.453-483.
Carneiro, N., Figueira, G. and Costa, M., 2017. A data mining based system for credit-card fraud
detection in e-tail. Decision Support Systems, 95, pp.91-101.
Corbae, D. and D'Erasmo, P., 2019. Capital requirements in a quantitative model of banking
industry dynamics (No. w25424). National Bureau of Economic Research.
Dapp, T. and Slomka, L., 2015. Fintech reloaded–Traditional banks as digital
ecosystems. Publication of the German original, pp.261-274.
References
ACL services limited. (2014). Fraud detection using data analytics in the banking industry.
Alalwan, A.A., Dwivedi, Y.K. and Rana, N.P., 2017. Factors influencing adoption of mobile
banking by Jordanian bank customers: Extending UTAUT2 with trust. International Journal of
Information Management, 37(3), pp.99-110.
Amado, A., Cortez, P., Rita, P. and Moro, S., 2018. Research trends on Big Data in Marketing: A
text mining and topic modeling based literature analysis. European Research on Management
and Business Economics, 24(1), pp.1-7.
Barrdear, J. and Kumhof, M., 2016. The macroeconomics of central bank issued digital
currencies.
Brinkmann, S., 2014. Interview. In Encyclopedia of critical psychology (pp. 1008-1010).
Springer New York.
Buchak, G., Matvos, G., Piskorski, T. and Seru, A., 2018. Fintech, regulatory arbitrage, and the
rise of shadow banks. Journal of Financial Economics, 130(3), pp.453-483.
Carneiro, N., Figueira, G. and Costa, M., 2017. A data mining based system for credit-card fraud
detection in e-tail. Decision Support Systems, 95, pp.91-101.
Corbae, D. and D'Erasmo, P., 2019. Capital requirements in a quantitative model of banking
industry dynamics (No. w25424). National Bureau of Economic Research.
Dapp, T. and Slomka, L., 2015. Fintech reloaded–Traditional banks as digital
ecosystems. Publication of the German original, pp.261-274.
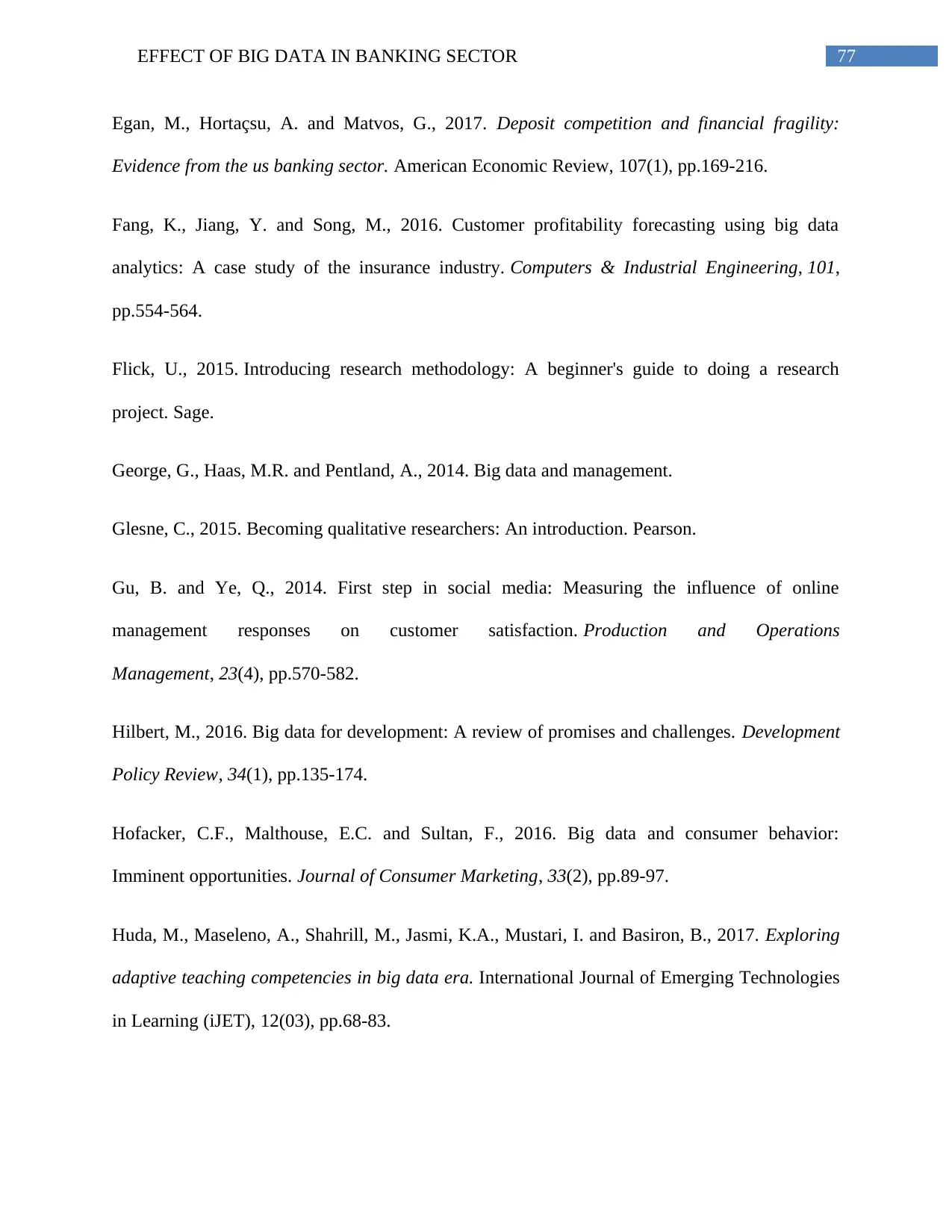
77EFFECT OF BIG DATA IN BANKING SECTOR
Egan, M., Hortaçsu, A. and Matvos, G., 2017. Deposit competition and financial fragility:
Evidence from the us banking sector. American Economic Review, 107(1), pp.169-216.
Fang, K., Jiang, Y. and Song, M., 2016. Customer profitability forecasting using big data
analytics: A case study of the insurance industry. Computers & Industrial Engineering, 101,
pp.554-564.
Flick, U., 2015. Introducing research methodology: A beginner's guide to doing a research
project. Sage.
George, G., Haas, M.R. and Pentland, A., 2014. Big data and management.
Glesne, C., 2015. Becoming qualitative researchers: An introduction. Pearson.
Gu, B. and Ye, Q., 2014. First step in social media: Measuring the influence of online
management responses on customer satisfaction. Production and Operations
Management, 23(4), pp.570-582.
Hilbert, M., 2016. Big data for development: A review of promises and challenges. Development
Policy Review, 34(1), pp.135-174.
Hofacker, C.F., Malthouse, E.C. and Sultan, F., 2016. Big data and consumer behavior:
Imminent opportunities. Journal of Consumer Marketing, 33(2), pp.89-97.
Huda, M., Maseleno, A., Shahrill, M., Jasmi, K.A., Mustari, I. and Basiron, B., 2017. Exploring
adaptive teaching competencies in big data era. International Journal of Emerging Technologies
in Learning (iJET), 12(03), pp.68-83.
Egan, M., Hortaçsu, A. and Matvos, G., 2017. Deposit competition and financial fragility:
Evidence from the us banking sector. American Economic Review, 107(1), pp.169-216.
Fang, K., Jiang, Y. and Song, M., 2016. Customer profitability forecasting using big data
analytics: A case study of the insurance industry. Computers & Industrial Engineering, 101,
pp.554-564.
Flick, U., 2015. Introducing research methodology: A beginner's guide to doing a research
project. Sage.
George, G., Haas, M.R. and Pentland, A., 2014. Big data and management.
Glesne, C., 2015. Becoming qualitative researchers: An introduction. Pearson.
Gu, B. and Ye, Q., 2014. First step in social media: Measuring the influence of online
management responses on customer satisfaction. Production and Operations
Management, 23(4), pp.570-582.
Hilbert, M., 2016. Big data for development: A review of promises and challenges. Development
Policy Review, 34(1), pp.135-174.
Hofacker, C.F., Malthouse, E.C. and Sultan, F., 2016. Big data and consumer behavior:
Imminent opportunities. Journal of Consumer Marketing, 33(2), pp.89-97.
Huda, M., Maseleno, A., Shahrill, M., Jasmi, K.A., Mustari, I. and Basiron, B., 2017. Exploring
adaptive teaching competencies in big data era. International Journal of Emerging Technologies
in Learning (iJET), 12(03), pp.68-83.
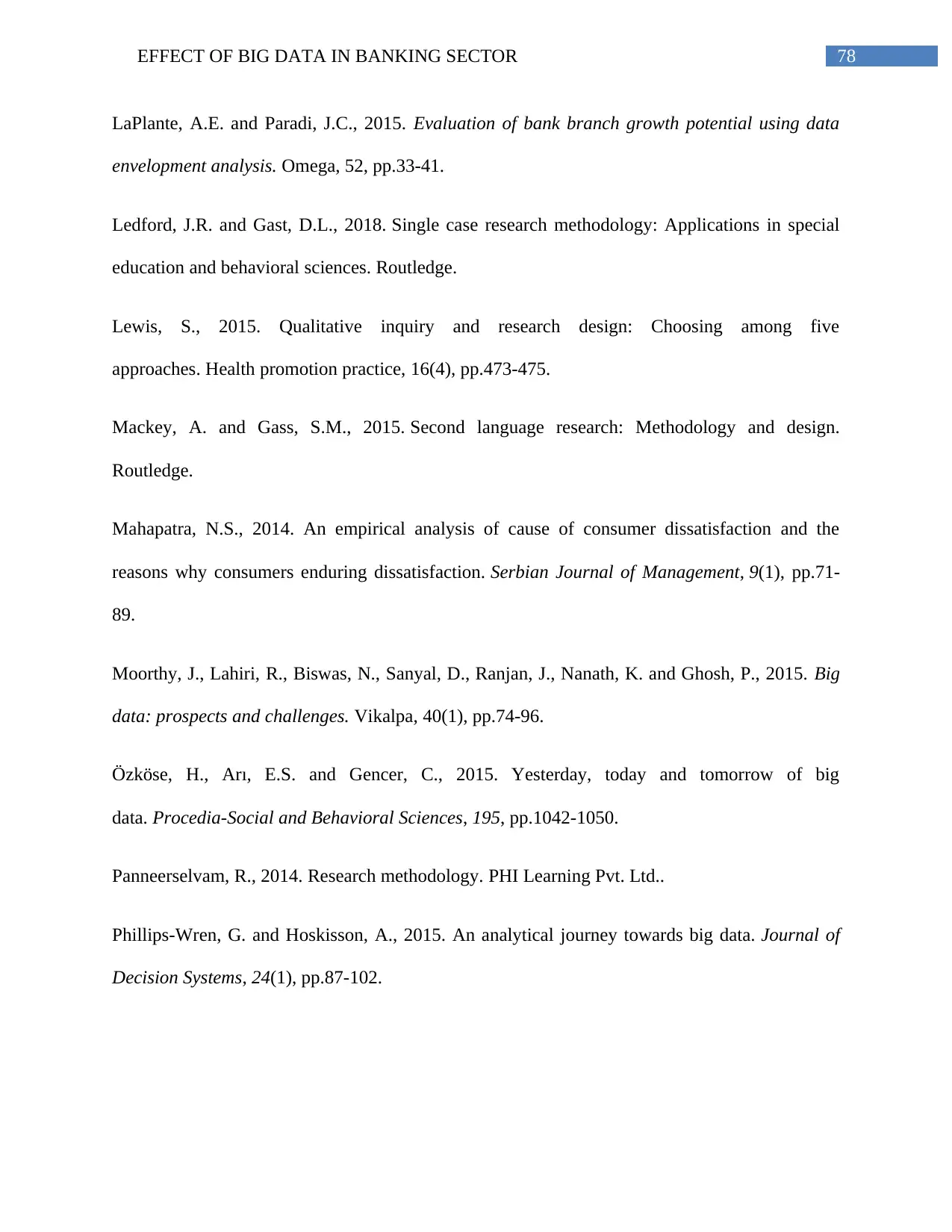
78EFFECT OF BIG DATA IN BANKING SECTOR
LaPlante, A.E. and Paradi, J.C., 2015. Evaluation of bank branch growth potential using data
envelopment analysis. Omega, 52, pp.33-41.
Ledford, J.R. and Gast, D.L., 2018. Single case research methodology: Applications in special
education and behavioral sciences. Routledge.
Lewis, S., 2015. Qualitative inquiry and research design: Choosing among five
approaches. Health promotion practice, 16(4), pp.473-475.
Mackey, A. and Gass, S.M., 2015. Second language research: Methodology and design.
Routledge.
Mahapatra, N.S., 2014. An empirical analysis of cause of consumer dissatisfaction and the
reasons why consumers enduring dissatisfaction. Serbian Journal of Management, 9(1), pp.71-
89.
Moorthy, J., Lahiri, R., Biswas, N., Sanyal, D., Ranjan, J., Nanath, K. and Ghosh, P., 2015. Big
data: prospects and challenges. Vikalpa, 40(1), pp.74-96.
Özköse, H., Arı, E.S. and Gencer, C., 2015. Yesterday, today and tomorrow of big
data. Procedia-Social and Behavioral Sciences, 195, pp.1042-1050.
Panneerselvam, R., 2014. Research methodology. PHI Learning Pvt. Ltd..
Phillips-Wren, G. and Hoskisson, A., 2015. An analytical journey towards big data. Journal of
Decision Systems, 24(1), pp.87-102.
LaPlante, A.E. and Paradi, J.C., 2015. Evaluation of bank branch growth potential using data
envelopment analysis. Omega, 52, pp.33-41.
Ledford, J.R. and Gast, D.L., 2018. Single case research methodology: Applications in special
education and behavioral sciences. Routledge.
Lewis, S., 2015. Qualitative inquiry and research design: Choosing among five
approaches. Health promotion practice, 16(4), pp.473-475.
Mackey, A. and Gass, S.M., 2015. Second language research: Methodology and design.
Routledge.
Mahapatra, N.S., 2014. An empirical analysis of cause of consumer dissatisfaction and the
reasons why consumers enduring dissatisfaction. Serbian Journal of Management, 9(1), pp.71-
89.
Moorthy, J., Lahiri, R., Biswas, N., Sanyal, D., Ranjan, J., Nanath, K. and Ghosh, P., 2015. Big
data: prospects and challenges. Vikalpa, 40(1), pp.74-96.
Özköse, H., Arı, E.S. and Gencer, C., 2015. Yesterday, today and tomorrow of big
data. Procedia-Social and Behavioral Sciences, 195, pp.1042-1050.
Panneerselvam, R., 2014. Research methodology. PHI Learning Pvt. Ltd..
Phillips-Wren, G. and Hoskisson, A., 2015. An analytical journey towards big data. Journal of
Decision Systems, 24(1), pp.87-102.
Secure Best Marks with AI Grader
Need help grading? Try our AI Grader for instant feedback on your assignments.
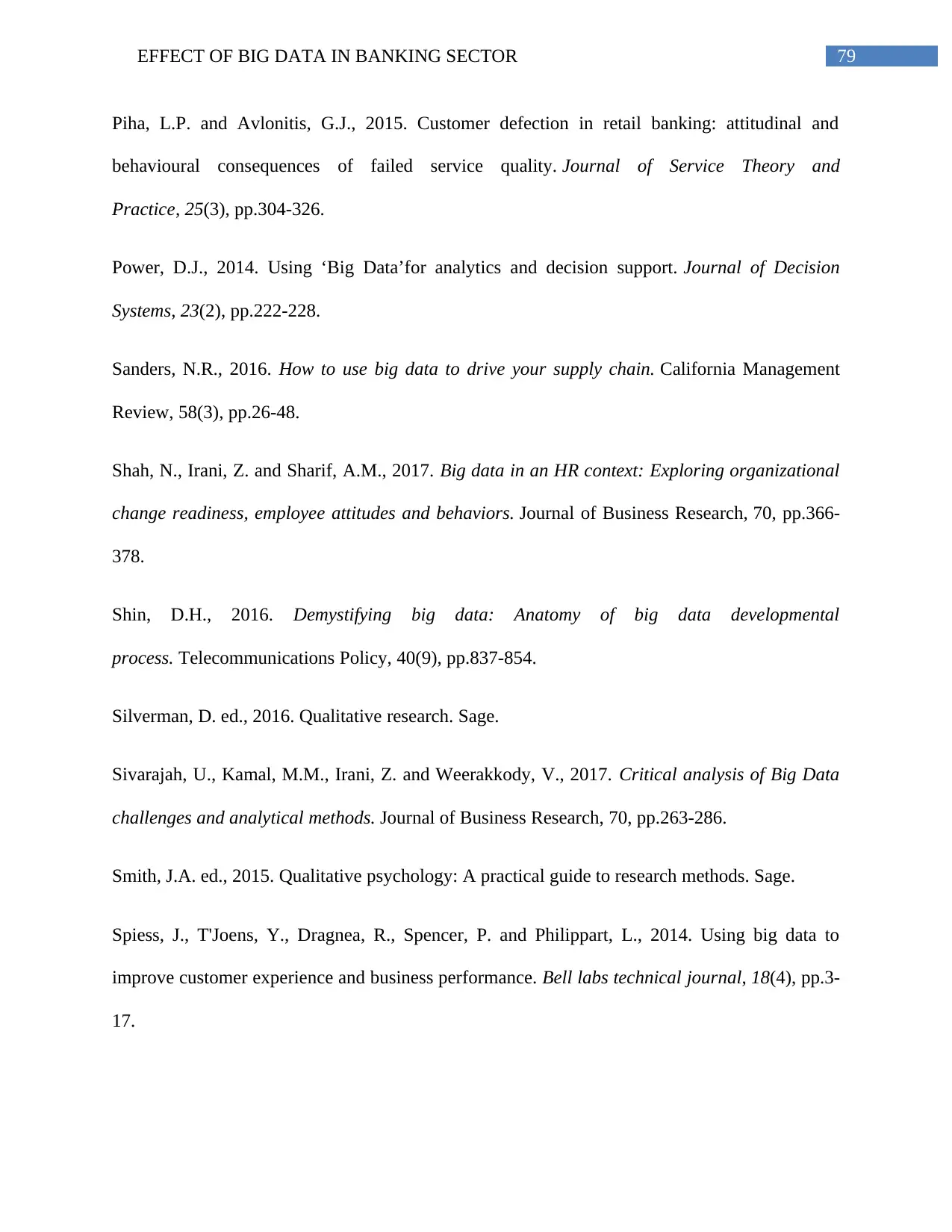
79EFFECT OF BIG DATA IN BANKING SECTOR
Piha, L.P. and Avlonitis, G.J., 2015. Customer defection in retail banking: attitudinal and
behavioural consequences of failed service quality. Journal of Service Theory and
Practice, 25(3), pp.304-326.
Power, D.J., 2014. Using ‘Big Data’for analytics and decision support. Journal of Decision
Systems, 23(2), pp.222-228.
Sanders, N.R., 2016. How to use big data to drive your supply chain. California Management
Review, 58(3), pp.26-48.
Shah, N., Irani, Z. and Sharif, A.M., 2017. Big data in an HR context: Exploring organizational
change readiness, employee attitudes and behaviors. Journal of Business Research, 70, pp.366-
378.
Shin, D.H., 2016. Demystifying big data: Anatomy of big data developmental
process. Telecommunications Policy, 40(9), pp.837-854.
Silverman, D. ed., 2016. Qualitative research. Sage.
Sivarajah, U., Kamal, M.M., Irani, Z. and Weerakkody, V., 2017. Critical analysis of Big Data
challenges and analytical methods. Journal of Business Research, 70, pp.263-286.
Smith, J.A. ed., 2015. Qualitative psychology: A practical guide to research methods. Sage.
Spiess, J., T'Joens, Y., Dragnea, R., Spencer, P. and Philippart, L., 2014. Using big data to
improve customer experience and business performance. Bell labs technical journal, 18(4), pp.3-
17.
Piha, L.P. and Avlonitis, G.J., 2015. Customer defection in retail banking: attitudinal and
behavioural consequences of failed service quality. Journal of Service Theory and
Practice, 25(3), pp.304-326.
Power, D.J., 2014. Using ‘Big Data’for analytics and decision support. Journal of Decision
Systems, 23(2), pp.222-228.
Sanders, N.R., 2016. How to use big data to drive your supply chain. California Management
Review, 58(3), pp.26-48.
Shah, N., Irani, Z. and Sharif, A.M., 2017. Big data in an HR context: Exploring organizational
change readiness, employee attitudes and behaviors. Journal of Business Research, 70, pp.366-
378.
Shin, D.H., 2016. Demystifying big data: Anatomy of big data developmental
process. Telecommunications Policy, 40(9), pp.837-854.
Silverman, D. ed., 2016. Qualitative research. Sage.
Sivarajah, U., Kamal, M.M., Irani, Z. and Weerakkody, V., 2017. Critical analysis of Big Data
challenges and analytical methods. Journal of Business Research, 70, pp.263-286.
Smith, J.A. ed., 2015. Qualitative psychology: A practical guide to research methods. Sage.
Spiess, J., T'Joens, Y., Dragnea, R., Spencer, P. and Philippart, L., 2014. Using big data to
improve customer experience and business performance. Bell labs technical journal, 18(4), pp.3-
17.
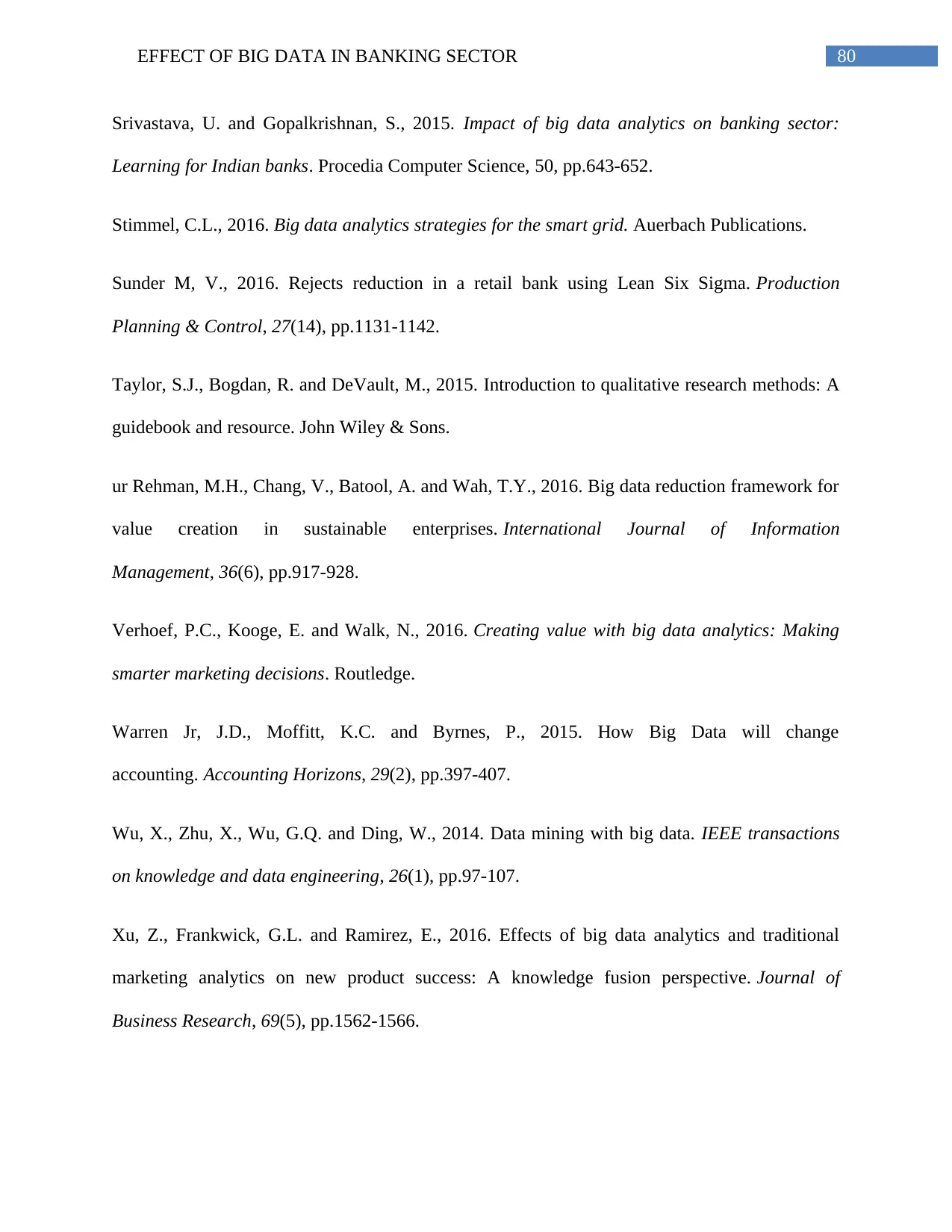
80EFFECT OF BIG DATA IN BANKING SECTOR
Srivastava, U. and Gopalkrishnan, S., 2015. Impact of big data analytics on banking sector:
Learning for Indian banks. Procedia Computer Science, 50, pp.643-652.
Stimmel, C.L., 2016. Big data analytics strategies for the smart grid. Auerbach Publications.
Sunder M, V., 2016. Rejects reduction in a retail bank using Lean Six Sigma. Production
Planning & Control, 27(14), pp.1131-1142.
Taylor, S.J., Bogdan, R. and DeVault, M., 2015. Introduction to qualitative research methods: A
guidebook and resource. John Wiley & Sons.
ur Rehman, M.H., Chang, V., Batool, A. and Wah, T.Y., 2016. Big data reduction framework for
value creation in sustainable enterprises. International Journal of Information
Management, 36(6), pp.917-928.
Verhoef, P.C., Kooge, E. and Walk, N., 2016. Creating value with big data analytics: Making
smarter marketing decisions. Routledge.
Warren Jr, J.D., Moffitt, K.C. and Byrnes, P., 2015. How Big Data will change
accounting. Accounting Horizons, 29(2), pp.397-407.
Wu, X., Zhu, X., Wu, G.Q. and Ding, W., 2014. Data mining with big data. IEEE transactions
on knowledge and data engineering, 26(1), pp.97-107.
Xu, Z., Frankwick, G.L. and Ramirez, E., 2016. Effects of big data analytics and traditional
marketing analytics on new product success: A knowledge fusion perspective. Journal of
Business Research, 69(5), pp.1562-1566.
Srivastava, U. and Gopalkrishnan, S., 2015. Impact of big data analytics on banking sector:
Learning for Indian banks. Procedia Computer Science, 50, pp.643-652.
Stimmel, C.L., 2016. Big data analytics strategies for the smart grid. Auerbach Publications.
Sunder M, V., 2016. Rejects reduction in a retail bank using Lean Six Sigma. Production
Planning & Control, 27(14), pp.1131-1142.
Taylor, S.J., Bogdan, R. and DeVault, M., 2015. Introduction to qualitative research methods: A
guidebook and resource. John Wiley & Sons.
ur Rehman, M.H., Chang, V., Batool, A. and Wah, T.Y., 2016. Big data reduction framework for
value creation in sustainable enterprises. International Journal of Information
Management, 36(6), pp.917-928.
Verhoef, P.C., Kooge, E. and Walk, N., 2016. Creating value with big data analytics: Making
smarter marketing decisions. Routledge.
Warren Jr, J.D., Moffitt, K.C. and Byrnes, P., 2015. How Big Data will change
accounting. Accounting Horizons, 29(2), pp.397-407.
Wu, X., Zhu, X., Wu, G.Q. and Ding, W., 2014. Data mining with big data. IEEE transactions
on knowledge and data engineering, 26(1), pp.97-107.
Xu, Z., Frankwick, G.L. and Ramirez, E., 2016. Effects of big data analytics and traditional
marketing analytics on new product success: A knowledge fusion perspective. Journal of
Business Research, 69(5), pp.1562-1566.
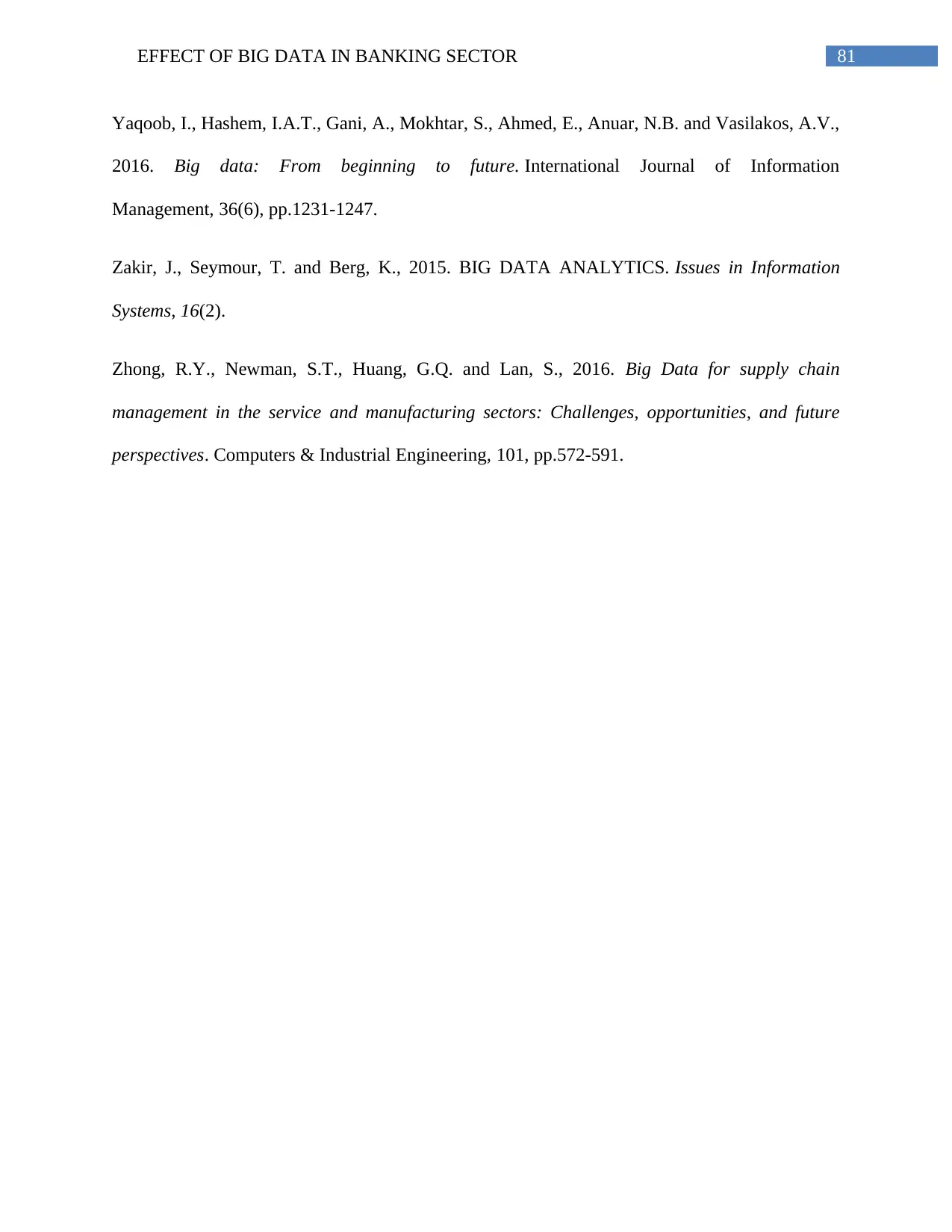
81EFFECT OF BIG DATA IN BANKING SECTOR
Yaqoob, I., Hashem, I.A.T., Gani, A., Mokhtar, S., Ahmed, E., Anuar, N.B. and Vasilakos, A.V.,
2016. Big data: From beginning to future. International Journal of Information
Management, 36(6), pp.1231-1247.
Zakir, J., Seymour, T. and Berg, K., 2015. BIG DATA ANALYTICS. Issues in Information
Systems, 16(2).
Zhong, R.Y., Newman, S.T., Huang, G.Q. and Lan, S., 2016. Big Data for supply chain
management in the service and manufacturing sectors: Challenges, opportunities, and future
perspectives. Computers & Industrial Engineering, 101, pp.572-591.
Yaqoob, I., Hashem, I.A.T., Gani, A., Mokhtar, S., Ahmed, E., Anuar, N.B. and Vasilakos, A.V.,
2016. Big data: From beginning to future. International Journal of Information
Management, 36(6), pp.1231-1247.
Zakir, J., Seymour, T. and Berg, K., 2015. BIG DATA ANALYTICS. Issues in Information
Systems, 16(2).
Zhong, R.Y., Newman, S.T., Huang, G.Q. and Lan, S., 2016. Big Data for supply chain
management in the service and manufacturing sectors: Challenges, opportunities, and future
perspectives. Computers & Industrial Engineering, 101, pp.572-591.
Paraphrase This Document
Need a fresh take? Get an instant paraphrase of this document with our AI Paraphraser
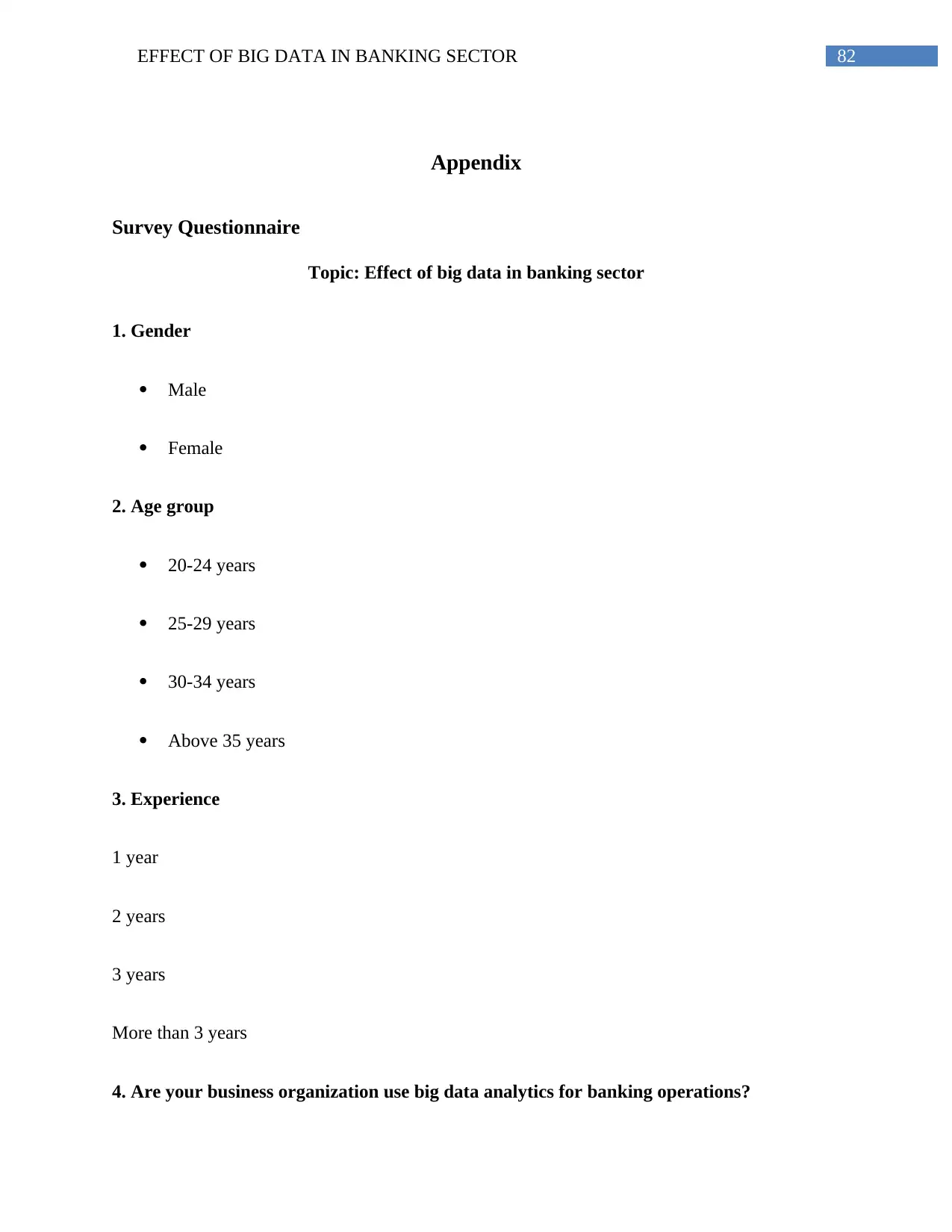
82EFFECT OF BIG DATA IN BANKING SECTOR
Appendix
Survey Questionnaire
Topic: Effect of big data in banking sector
1. Gender
Male
Female
2. Age group
20-24 years
25-29 years
30-34 years
Above 35 years
3. Experience
1 year
2 years
3 years
More than 3 years
4. Are your business organization use big data analytics for banking operations?
Appendix
Survey Questionnaire
Topic: Effect of big data in banking sector
1. Gender
Male
Female
2. Age group
20-24 years
25-29 years
30-34 years
Above 35 years
3. Experience
1 year
2 years
3 years
More than 3 years
4. Are your business organization use big data analytics for banking operations?
1 out of 83
Related Documents
![[object Object]](/_next/image/?url=%2F_next%2Fstatic%2Fmedia%2Flogo.6d15ce61.png&w=640&q=75)
Your All-in-One AI-Powered Toolkit for Academic Success.
+13062052269
info@desklib.com
Available 24*7 on WhatsApp / Email
Unlock your academic potential
© 2024 | Zucol Services PVT LTD | All rights reserved.