Impact of Macroeconomic Factors on FDI and Environment: A Regression Analysis
VerifiedAdded on 2023/06/15
|29
|7395
|170
AI Summary
This research examines the impact of different macroeconomic factors on the inflow of FDI and the environment. Multiple regression analysis, correlation analysis, and descriptive statistics have been used to analyze the data. The study also examines the pollution haven hypothesis. Data is from 2003 to 2015 for a particular country.
Contribute Materials
Your contribution can guide someone’s learning journey. Share your
documents today.
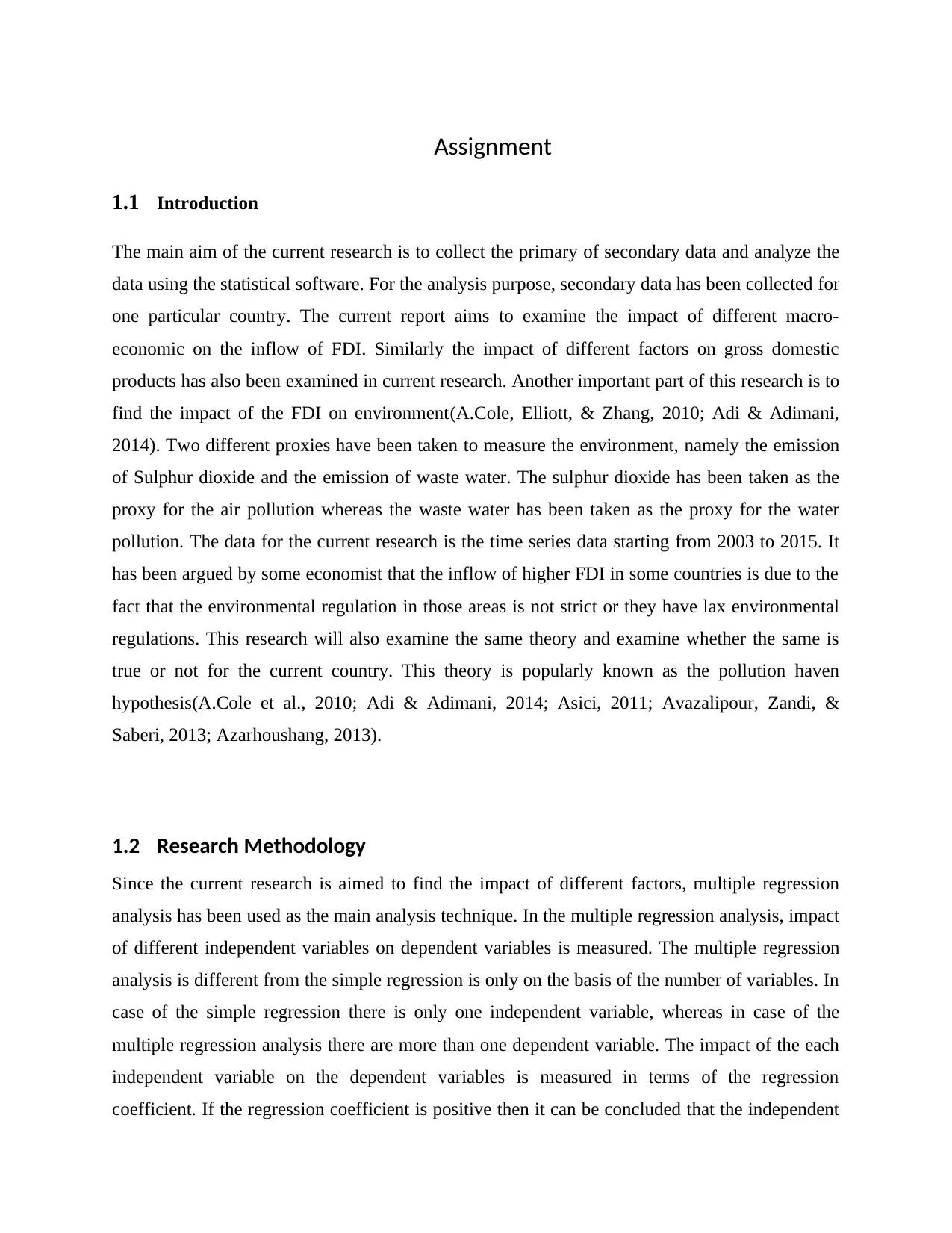
Assignment
1.1 Introduction
The main aim of the current research is to collect the primary of secondary data and analyze the
data using the statistical software. For the analysis purpose, secondary data has been collected for
one particular country. The current report aims to examine the impact of different macro-
economic on the inflow of FDI. Similarly the impact of different factors on gross domestic
products has also been examined in current research. Another important part of this research is to
find the impact of the FDI on environment(A.Cole, Elliott, & Zhang, 2010; Adi & Adimani,
2014). Two different proxies have been taken to measure the environment, namely the emission
of Sulphur dioxide and the emission of waste water. The sulphur dioxide has been taken as the
proxy for the air pollution whereas the waste water has been taken as the proxy for the water
pollution. The data for the current research is the time series data starting from 2003 to 2015. It
has been argued by some economist that the inflow of higher FDI in some countries is due to the
fact that the environmental regulation in those areas is not strict or they have lax environmental
regulations. This research will also examine the same theory and examine whether the same is
true or not for the current country. This theory is popularly known as the pollution haven
hypothesis(A.Cole et al., 2010; Adi & Adimani, 2014; Asici, 2011; Avazalipour, Zandi, &
Saberi, 2013; Azarhoushang, 2013).
1.2 Research Methodology
Since the current research is aimed to find the impact of different factors, multiple regression
analysis has been used as the main analysis technique. In the multiple regression analysis, impact
of different independent variables on dependent variables is measured. The multiple regression
analysis is different from the simple regression is only on the basis of the number of variables. In
case of the simple regression there is only one independent variable, whereas in case of the
multiple regression analysis there are more than one dependent variable. The impact of the each
independent variable on the dependent variables is measured in terms of the regression
coefficient. If the regression coefficient is positive then it can be concluded that the independent
1.1 Introduction
The main aim of the current research is to collect the primary of secondary data and analyze the
data using the statistical software. For the analysis purpose, secondary data has been collected for
one particular country. The current report aims to examine the impact of different macro-
economic on the inflow of FDI. Similarly the impact of different factors on gross domestic
products has also been examined in current research. Another important part of this research is to
find the impact of the FDI on environment(A.Cole, Elliott, & Zhang, 2010; Adi & Adimani,
2014). Two different proxies have been taken to measure the environment, namely the emission
of Sulphur dioxide and the emission of waste water. The sulphur dioxide has been taken as the
proxy for the air pollution whereas the waste water has been taken as the proxy for the water
pollution. The data for the current research is the time series data starting from 2003 to 2015. It
has been argued by some economist that the inflow of higher FDI in some countries is due to the
fact that the environmental regulation in those areas is not strict or they have lax environmental
regulations. This research will also examine the same theory and examine whether the same is
true or not for the current country. This theory is popularly known as the pollution haven
hypothesis(A.Cole et al., 2010; Adi & Adimani, 2014; Asici, 2011; Avazalipour, Zandi, &
Saberi, 2013; Azarhoushang, 2013).
1.2 Research Methodology
Since the current research is aimed to find the impact of different factors, multiple regression
analysis has been used as the main analysis technique. In the multiple regression analysis, impact
of different independent variables on dependent variables is measured. The multiple regression
analysis is different from the simple regression is only on the basis of the number of variables. In
case of the simple regression there is only one independent variable, whereas in case of the
multiple regression analysis there are more than one dependent variable. The impact of the each
independent variable on the dependent variables is measured in terms of the regression
coefficient. If the regression coefficient is positive then it can be concluded that the independent
Secure Best Marks with AI Grader
Need help grading? Try our AI Grader for instant feedback on your assignments.
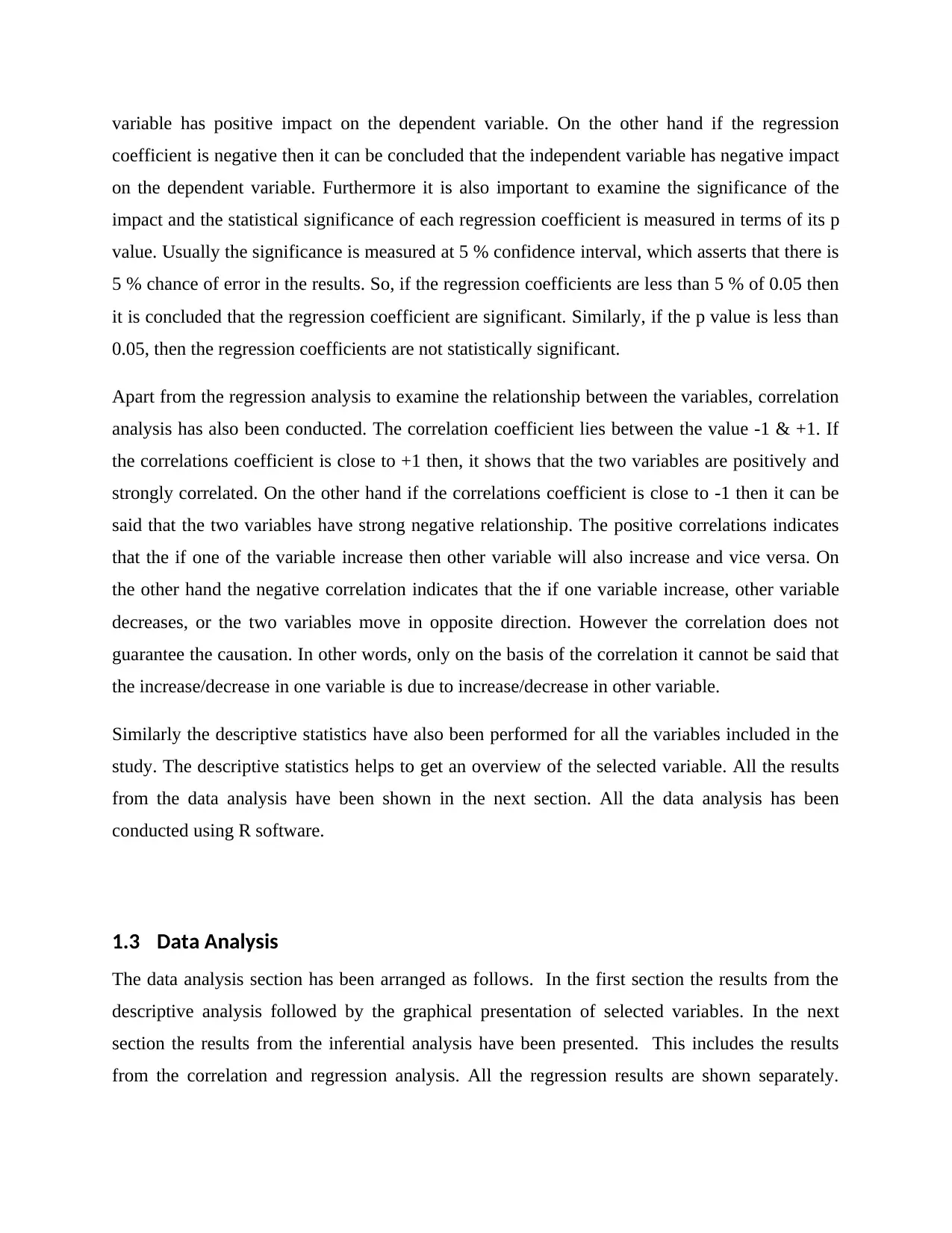
variable has positive impact on the dependent variable. On the other hand if the regression
coefficient is negative then it can be concluded that the independent variable has negative impact
on the dependent variable. Furthermore it is also important to examine the significance of the
impact and the statistical significance of each regression coefficient is measured in terms of its p
value. Usually the significance is measured at 5 % confidence interval, which asserts that there is
5 % chance of error in the results. So, if the regression coefficients are less than 5 % of 0.05 then
it is concluded that the regression coefficient are significant. Similarly, if the p value is less than
0.05, then the regression coefficients are not statistically significant.
Apart from the regression analysis to examine the relationship between the variables, correlation
analysis has also been conducted. The correlation coefficient lies between the value -1 & +1. If
the correlations coefficient is close to +1 then, it shows that the two variables are positively and
strongly correlated. On the other hand if the correlations coefficient is close to -1 then it can be
said that the two variables have strong negative relationship. The positive correlations indicates
that the if one of the variable increase then other variable will also increase and vice versa. On
the other hand the negative correlation indicates that the if one variable increase, other variable
decreases, or the two variables move in opposite direction. However the correlation does not
guarantee the causation. In other words, only on the basis of the correlation it cannot be said that
the increase/decrease in one variable is due to increase/decrease in other variable.
Similarly the descriptive statistics have also been performed for all the variables included in the
study. The descriptive statistics helps to get an overview of the selected variable. All the results
from the data analysis have been shown in the next section. All the data analysis has been
conducted using R software.
1.3 Data Analysis
The data analysis section has been arranged as follows. In the first section the results from the
descriptive analysis followed by the graphical presentation of selected variables. In the next
section the results from the inferential analysis have been presented. This includes the results
from the correlation and regression analysis. All the regression results are shown separately.
coefficient is negative then it can be concluded that the independent variable has negative impact
on the dependent variable. Furthermore it is also important to examine the significance of the
impact and the statistical significance of each regression coefficient is measured in terms of its p
value. Usually the significance is measured at 5 % confidence interval, which asserts that there is
5 % chance of error in the results. So, if the regression coefficients are less than 5 % of 0.05 then
it is concluded that the regression coefficient are significant. Similarly, if the p value is less than
0.05, then the regression coefficients are not statistically significant.
Apart from the regression analysis to examine the relationship between the variables, correlation
analysis has also been conducted. The correlation coefficient lies between the value -1 & +1. If
the correlations coefficient is close to +1 then, it shows that the two variables are positively and
strongly correlated. On the other hand if the correlations coefficient is close to -1 then it can be
said that the two variables have strong negative relationship. The positive correlations indicates
that the if one of the variable increase then other variable will also increase and vice versa. On
the other hand the negative correlation indicates that the if one variable increase, other variable
decreases, or the two variables move in opposite direction. However the correlation does not
guarantee the causation. In other words, only on the basis of the correlation it cannot be said that
the increase/decrease in one variable is due to increase/decrease in other variable.
Similarly the descriptive statistics have also been performed for all the variables included in the
study. The descriptive statistics helps to get an overview of the selected variable. All the results
from the data analysis have been shown in the next section. All the data analysis has been
conducted using R software.
1.3 Data Analysis
The data analysis section has been arranged as follows. In the first section the results from the
descriptive analysis followed by the graphical presentation of selected variables. In the next
section the results from the inferential analysis have been presented. This includes the results
from the correlation and regression analysis. All the regression results are shown separately.
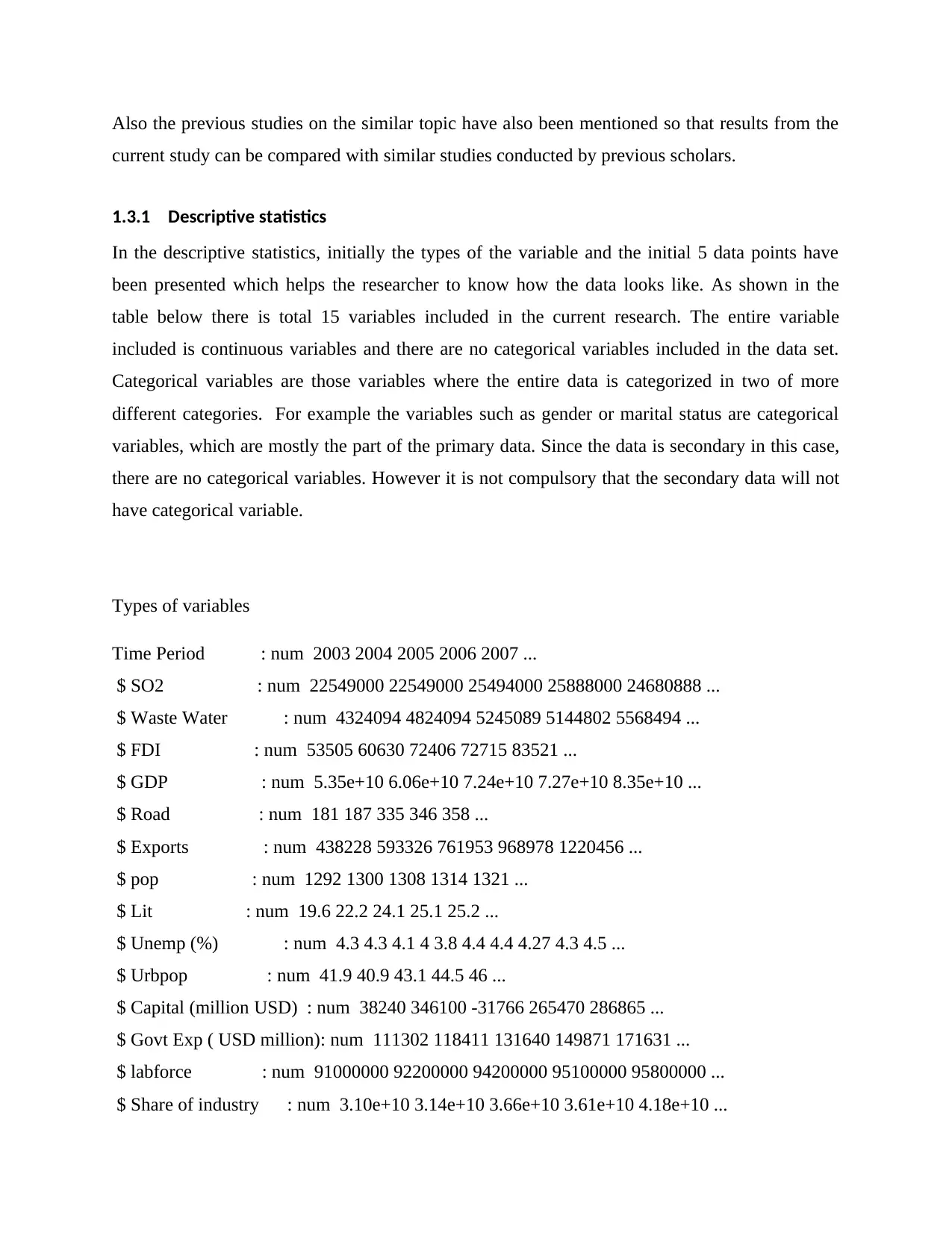
Also the previous studies on the similar topic have also been mentioned so that results from the
current study can be compared with similar studies conducted by previous scholars.
1.3.1 Descriptive statistics
In the descriptive statistics, initially the types of the variable and the initial 5 data points have
been presented which helps the researcher to know how the data looks like. As shown in the
table below there is total 15 variables included in the current research. The entire variable
included is continuous variables and there are no categorical variables included in the data set.
Categorical variables are those variables where the entire data is categorized in two of more
different categories. For example the variables such as gender or marital status are categorical
variables, which are mostly the part of the primary data. Since the data is secondary in this case,
there are no categorical variables. However it is not compulsory that the secondary data will not
have categorical variable.
Types of variables
Time Period : num 2003 2004 2005 2006 2007 ...
$ SO2 : num 22549000 22549000 25494000 25888000 24680888 ...
$ Waste Water : num 4324094 4824094 5245089 5144802 5568494 ...
$ FDI : num 53505 60630 72406 72715 83521 ...
$ GDP : num 5.35e+10 6.06e+10 7.24e+10 7.27e+10 8.35e+10 ...
$ Road : num 181 187 335 346 358 ...
$ Exports : num 438228 593326 761953 968978 1220456 ...
$ pop : num 1292 1300 1308 1314 1321 ...
$ Lit : num 19.6 22.2 24.1 25.1 25.2 ...
$ Unemp (%) : num 4.3 4.3 4.1 4 3.8 4.4 4.4 4.27 4.3 4.5 ...
$ Urbpop : num 41.9 40.9 43.1 44.5 46 ...
$ Capital (million USD) : num 38240 346100 -31766 265470 286865 ...
$ Govt Exp ( USD million): num 111302 118411 131640 149871 171631 ...
$ labforce : num 91000000 92200000 94200000 95100000 95800000 ...
$ Share of industry : num 3.10e+10 3.14e+10 3.66e+10 3.61e+10 4.18e+10 ...
current study can be compared with similar studies conducted by previous scholars.
1.3.1 Descriptive statistics
In the descriptive statistics, initially the types of the variable and the initial 5 data points have
been presented which helps the researcher to know how the data looks like. As shown in the
table below there is total 15 variables included in the current research. The entire variable
included is continuous variables and there are no categorical variables included in the data set.
Categorical variables are those variables where the entire data is categorized in two of more
different categories. For example the variables such as gender or marital status are categorical
variables, which are mostly the part of the primary data. Since the data is secondary in this case,
there are no categorical variables. However it is not compulsory that the secondary data will not
have categorical variable.
Types of variables
Time Period : num 2003 2004 2005 2006 2007 ...
$ SO2 : num 22549000 22549000 25494000 25888000 24680888 ...
$ Waste Water : num 4324094 4824094 5245089 5144802 5568494 ...
$ FDI : num 53505 60630 72406 72715 83521 ...
$ GDP : num 5.35e+10 6.06e+10 7.24e+10 7.27e+10 8.35e+10 ...
$ Road : num 181 187 335 346 358 ...
$ Exports : num 438228 593326 761953 968978 1220456 ...
$ pop : num 1292 1300 1308 1314 1321 ...
$ Lit : num 19.6 22.2 24.1 25.1 25.2 ...
$ Unemp (%) : num 4.3 4.3 4.1 4 3.8 4.4 4.4 4.27 4.3 4.5 ...
$ Urbpop : num 41.9 40.9 43.1 44.5 46 ...
$ Capital (million USD) : num 38240 346100 -31766 265470 286865 ...
$ Govt Exp ( USD million): num 111302 118411 131640 149871 171631 ...
$ labforce : num 91000000 92200000 94200000 95100000 95800000 ...
$ Share of industry : num 3.10e+10 3.14e+10 3.66e+10 3.61e+10 4.18e+10 ...
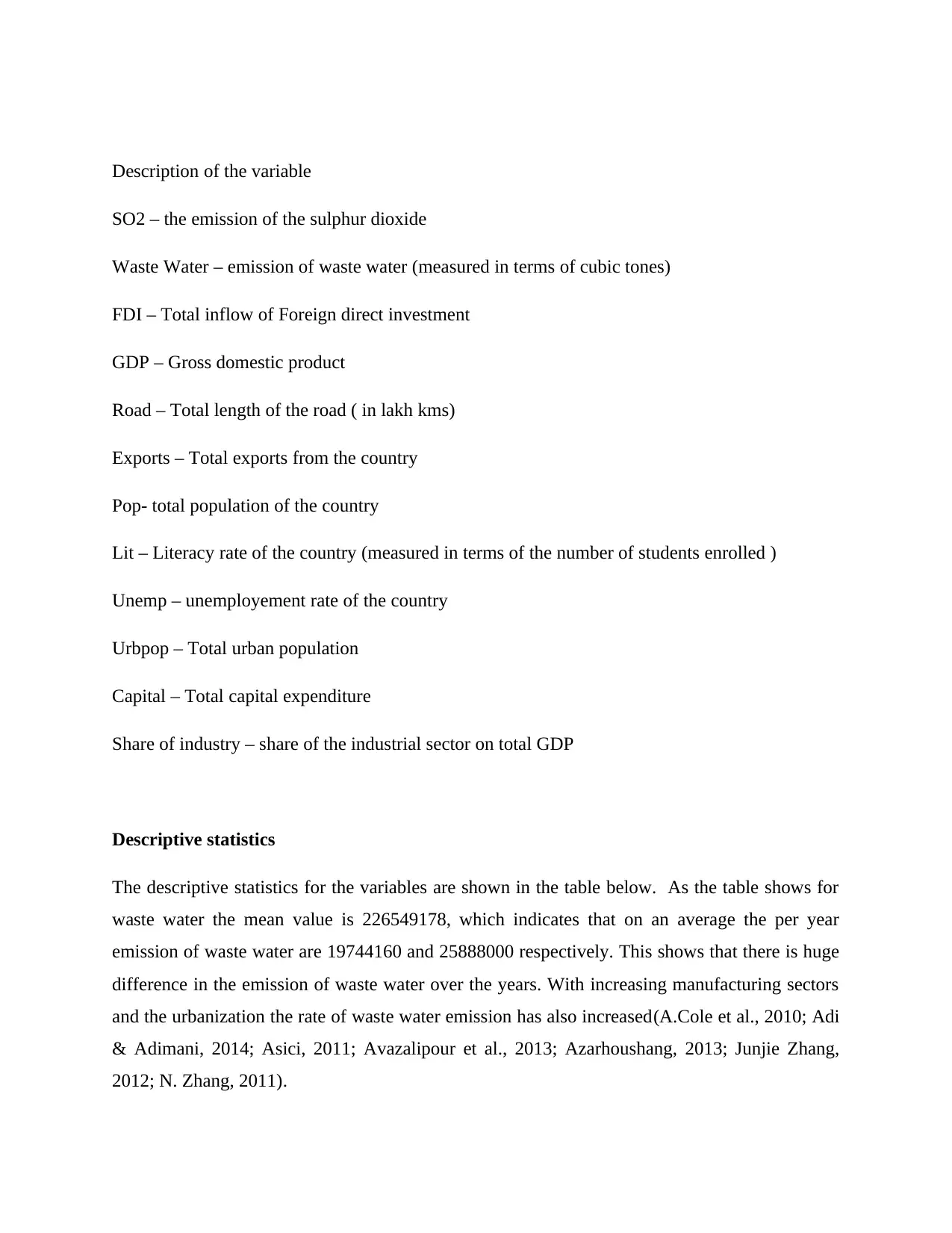
Description of the variable
SO2 – the emission of the sulphur dioxide
Waste Water – emission of waste water (measured in terms of cubic tones)
FDI – Total inflow of Foreign direct investment
GDP – Gross domestic product
Road – Total length of the road ( in lakh kms)
Exports – Total exports from the country
Pop- total population of the country
Lit – Literacy rate of the country (measured in terms of the number of students enrolled )
Unemp – unemployement rate of the country
Urbpop – Total urban population
Capital – Total capital expenditure
Share of industry – share of the industrial sector on total GDP
Descriptive statistics
The descriptive statistics for the variables are shown in the table below. As the table shows for
waste water the mean value is 226549178, which indicates that on an average the per year
emission of waste water are 19744160 and 25888000 respectively. This shows that there is huge
difference in the emission of waste water over the years. With increasing manufacturing sectors
and the urbanization the rate of waste water emission has also increased(A.Cole et al., 2010; Adi
& Adimani, 2014; Asici, 2011; Avazalipour et al., 2013; Azarhoushang, 2013; Junjie Zhang,
2012; N. Zhang, 2011).
SO2 – the emission of the sulphur dioxide
Waste Water – emission of waste water (measured in terms of cubic tones)
FDI – Total inflow of Foreign direct investment
GDP – Gross domestic product
Road – Total length of the road ( in lakh kms)
Exports – Total exports from the country
Pop- total population of the country
Lit – Literacy rate of the country (measured in terms of the number of students enrolled )
Unemp – unemployement rate of the country
Urbpop – Total urban population
Capital – Total capital expenditure
Share of industry – share of the industrial sector on total GDP
Descriptive statistics
The descriptive statistics for the variables are shown in the table below. As the table shows for
waste water the mean value is 226549178, which indicates that on an average the per year
emission of waste water are 19744160 and 25888000 respectively. This shows that there is huge
difference in the emission of waste water over the years. With increasing manufacturing sectors
and the urbanization the rate of waste water emission has also increased(A.Cole et al., 2010; Adi
& Adimani, 2014; Asici, 2011; Avazalipour et al., 2013; Azarhoushang, 2013; Junjie Zhang,
2012; N. Zhang, 2011).
Secure Best Marks with AI Grader
Need help grading? Try our AI Grader for instant feedback on your assignments.
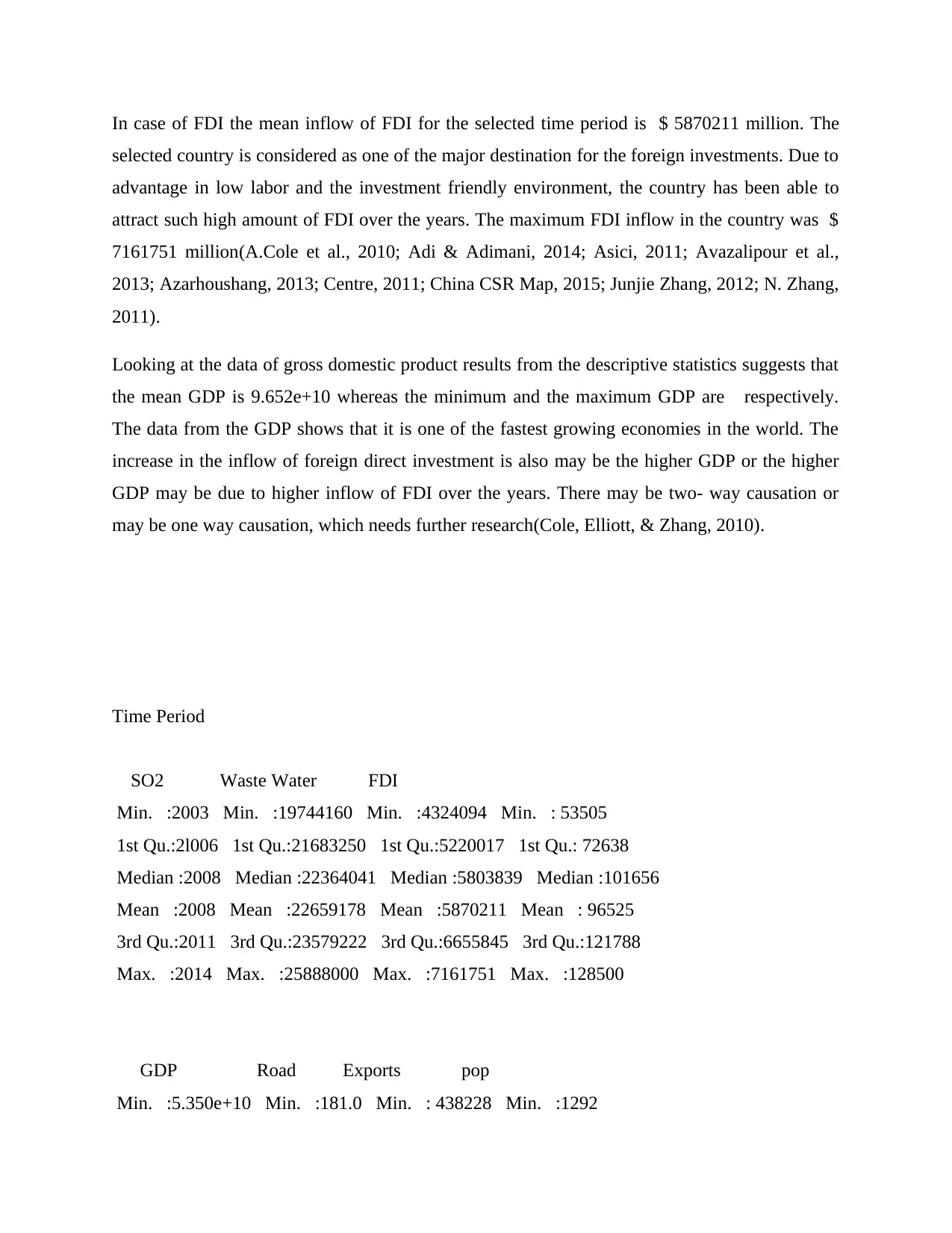
In case of FDI the mean inflow of FDI for the selected time period is $ 5870211 million. The
selected country is considered as one of the major destination for the foreign investments. Due to
advantage in low labor and the investment friendly environment, the country has been able to
attract such high amount of FDI over the years. The maximum FDI inflow in the country was $
7161751 million(A.Cole et al., 2010; Adi & Adimani, 2014; Asici, 2011; Avazalipour et al.,
2013; Azarhoushang, 2013; Centre, 2011; China CSR Map, 2015; Junjie Zhang, 2012; N. Zhang,
2011).
Looking at the data of gross domestic product results from the descriptive statistics suggests that
the mean GDP is 9.652e+10 whereas the minimum and the maximum GDP are respectively.
The data from the GDP shows that it is one of the fastest growing economies in the world. The
increase in the inflow of foreign direct investment is also may be the higher GDP or the higher
GDP may be due to higher inflow of FDI over the years. There may be two- way causation or
may be one way causation, which needs further research(Cole, Elliott, & Zhang, 2010).
Time Period
SO2 Waste Water FDI
Min. :2003 Min. :19744160 Min. :4324094 Min. : 53505
1st Qu.:2l006 1st Qu.:21683250 1st Qu.:5220017 1st Qu.: 72638
Median :2008 Median :22364041 Median :5803839 Median :101656
Mean :2008 Mean :22659178 Mean :5870211 Mean : 96525
3rd Qu.:2011 3rd Qu.:23579222 3rd Qu.:6655845 3rd Qu.:121788
Max. :2014 Max. :25888000 Max. :7161751 Max. :128500
GDP Road Exports pop
Min. :5.350e+10 Min. :181.0 Min. : 438228 Min. :1292
selected country is considered as one of the major destination for the foreign investments. Due to
advantage in low labor and the investment friendly environment, the country has been able to
attract such high amount of FDI over the years. The maximum FDI inflow in the country was $
7161751 million(A.Cole et al., 2010; Adi & Adimani, 2014; Asici, 2011; Avazalipour et al.,
2013; Azarhoushang, 2013; Centre, 2011; China CSR Map, 2015; Junjie Zhang, 2012; N. Zhang,
2011).
Looking at the data of gross domestic product results from the descriptive statistics suggests that
the mean GDP is 9.652e+10 whereas the minimum and the maximum GDP are respectively.
The data from the GDP shows that it is one of the fastest growing economies in the world. The
increase in the inflow of foreign direct investment is also may be the higher GDP or the higher
GDP may be due to higher inflow of FDI over the years. There may be two- way causation or
may be one way causation, which needs further research(Cole, Elliott, & Zhang, 2010).
Time Period
SO2 Waste Water FDI
Min. :2003 Min. :19744160 Min. :4324094 Min. : 53505
1st Qu.:2l006 1st Qu.:21683250 1st Qu.:5220017 1st Qu.: 72638
Median :2008 Median :22364041 Median :5803839 Median :101656
Mean :2008 Mean :22659178 Mean :5870211 Mean : 96525
3rd Qu.:2011 3rd Qu.:23579222 3rd Qu.:6655845 3rd Qu.:121788
Max. :2014 Max. :25888000 Max. :7161751 Max. :128500
GDP Road Exports pop
Min. :5.350e+10 Min. :181.0 Min. : 438228 Min. :1292
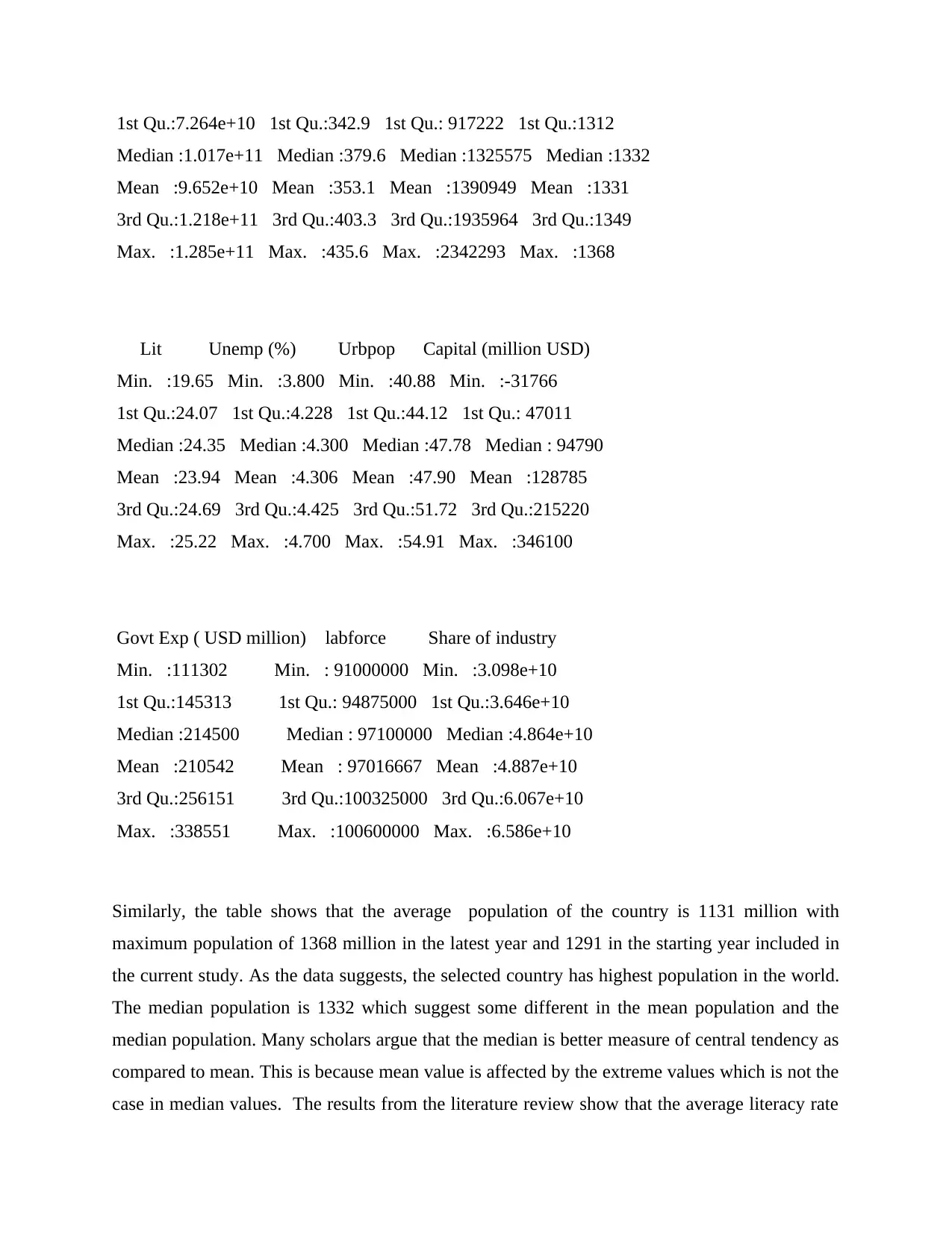
1st Qu.:7.264e+10 1st Qu.:342.9 1st Qu.: 917222 1st Qu.:1312
Median :1.017e+11 Median :379.6 Median :1325575 Median :1332
Mean :9.652e+10 Mean :353.1 Mean :1390949 Mean :1331
3rd Qu.:1.218e+11 3rd Qu.:403.3 3rd Qu.:1935964 3rd Qu.:1349
Max. :1.285e+11 Max. :435.6 Max. :2342293 Max. :1368
Lit Unemp (%) Urbpop Capital (million USD)
Min. :19.65 Min. :3.800 Min. :40.88 Min. :-31766
1st Qu.:24.07 1st Qu.:4.228 1st Qu.:44.12 1st Qu.: 47011
Median :24.35 Median :4.300 Median :47.78 Median : 94790
Mean :23.94 Mean :4.306 Mean :47.90 Mean :128785
3rd Qu.:24.69 3rd Qu.:4.425 3rd Qu.:51.72 3rd Qu.:215220
Max. :25.22 Max. :4.700 Max. :54.91 Max. :346100
Govt Exp ( USD million) labforce Share of industry
Min. :111302 Min. : 91000000 Min. :3.098e+10
1st Qu.:145313 1st Qu.: 94875000 1st Qu.:3.646e+10
Median :214500 Median : 97100000 Median :4.864e+10
Mean :210542 Mean : 97016667 Mean :4.887e+10
3rd Qu.:256151 3rd Qu.:100325000 3rd Qu.:6.067e+10
Max. :338551 Max. :100600000 Max. :6.586e+10
Similarly, the table shows that the average population of the country is 1131 million with
maximum population of 1368 million in the latest year and 1291 in the starting year included in
the current study. As the data suggests, the selected country has highest population in the world.
The median population is 1332 which suggest some different in the mean population and the
median population. Many scholars argue that the median is better measure of central tendency as
compared to mean. This is because mean value is affected by the extreme values which is not the
case in median values. The results from the literature review show that the average literacy rate
Median :1.017e+11 Median :379.6 Median :1325575 Median :1332
Mean :9.652e+10 Mean :353.1 Mean :1390949 Mean :1331
3rd Qu.:1.218e+11 3rd Qu.:403.3 3rd Qu.:1935964 3rd Qu.:1349
Max. :1.285e+11 Max. :435.6 Max. :2342293 Max. :1368
Lit Unemp (%) Urbpop Capital (million USD)
Min. :19.65 Min. :3.800 Min. :40.88 Min. :-31766
1st Qu.:24.07 1st Qu.:4.228 1st Qu.:44.12 1st Qu.: 47011
Median :24.35 Median :4.300 Median :47.78 Median : 94790
Mean :23.94 Mean :4.306 Mean :47.90 Mean :128785
3rd Qu.:24.69 3rd Qu.:4.425 3rd Qu.:51.72 3rd Qu.:215220
Max. :25.22 Max. :4.700 Max. :54.91 Max. :346100
Govt Exp ( USD million) labforce Share of industry
Min. :111302 Min. : 91000000 Min. :3.098e+10
1st Qu.:145313 1st Qu.: 94875000 1st Qu.:3.646e+10
Median :214500 Median : 97100000 Median :4.864e+10
Mean :210542 Mean : 97016667 Mean :4.887e+10
3rd Qu.:256151 3rd Qu.:100325000 3rd Qu.:6.067e+10
Max. :338551 Max. :100600000 Max. :6.586e+10
Similarly, the table shows that the average population of the country is 1131 million with
maximum population of 1368 million in the latest year and 1291 in the starting year included in
the current study. As the data suggests, the selected country has highest population in the world.
The median population is 1332 which suggest some different in the mean population and the
median population. Many scholars argue that the median is better measure of central tendency as
compared to mean. This is because mean value is affected by the extreme values which is not the
case in median values. The results from the literature review show that the average literacy rate
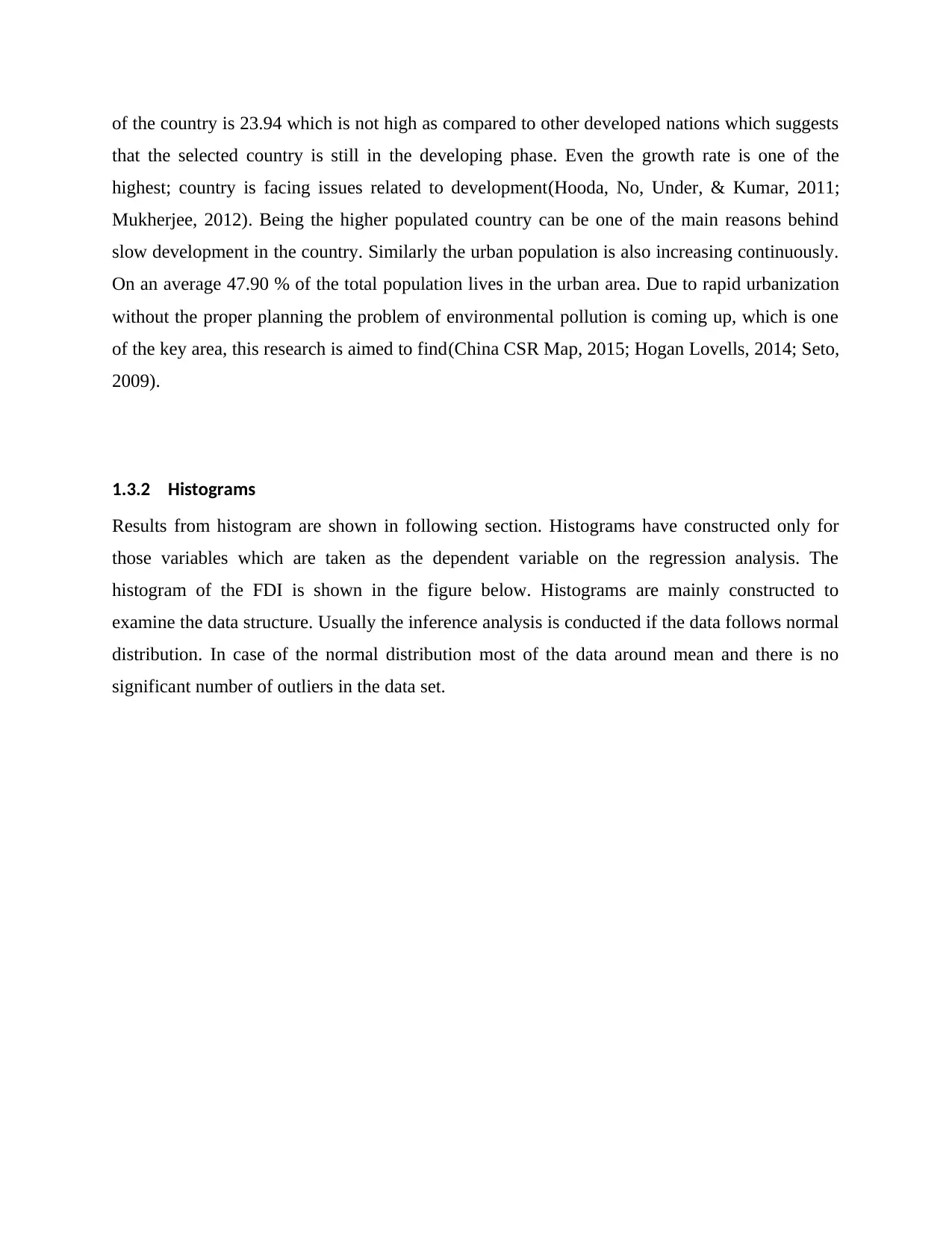
of the country is 23.94 which is not high as compared to other developed nations which suggests
that the selected country is still in the developing phase. Even the growth rate is one of the
highest; country is facing issues related to development(Hooda, No, Under, & Kumar, 2011;
Mukherjee, 2012). Being the higher populated country can be one of the main reasons behind
slow development in the country. Similarly the urban population is also increasing continuously.
On an average 47.90 % of the total population lives in the urban area. Due to rapid urbanization
without the proper planning the problem of environmental pollution is coming up, which is one
of the key area, this research is aimed to find(China CSR Map, 2015; Hogan Lovells, 2014; Seto,
2009).
1.3.2 Histograms
Results from histogram are shown in following section. Histograms have constructed only for
those variables which are taken as the dependent variable on the regression analysis. The
histogram of the FDI is shown in the figure below. Histograms are mainly constructed to
examine the data structure. Usually the inference analysis is conducted if the data follows normal
distribution. In case of the normal distribution most of the data around mean and there is no
significant number of outliers in the data set.
that the selected country is still in the developing phase. Even the growth rate is one of the
highest; country is facing issues related to development(Hooda, No, Under, & Kumar, 2011;
Mukherjee, 2012). Being the higher populated country can be one of the main reasons behind
slow development in the country. Similarly the urban population is also increasing continuously.
On an average 47.90 % of the total population lives in the urban area. Due to rapid urbanization
without the proper planning the problem of environmental pollution is coming up, which is one
of the key area, this research is aimed to find(China CSR Map, 2015; Hogan Lovells, 2014; Seto,
2009).
1.3.2 Histograms
Results from histogram are shown in following section. Histograms have constructed only for
those variables which are taken as the dependent variable on the regression analysis. The
histogram of the FDI is shown in the figure below. Histograms are mainly constructed to
examine the data structure. Usually the inference analysis is conducted if the data follows normal
distribution. In case of the normal distribution most of the data around mean and there is no
significant number of outliers in the data set.
Paraphrase This Document
Need a fresh take? Get an instant paraphrase of this document with our AI Paraphraser
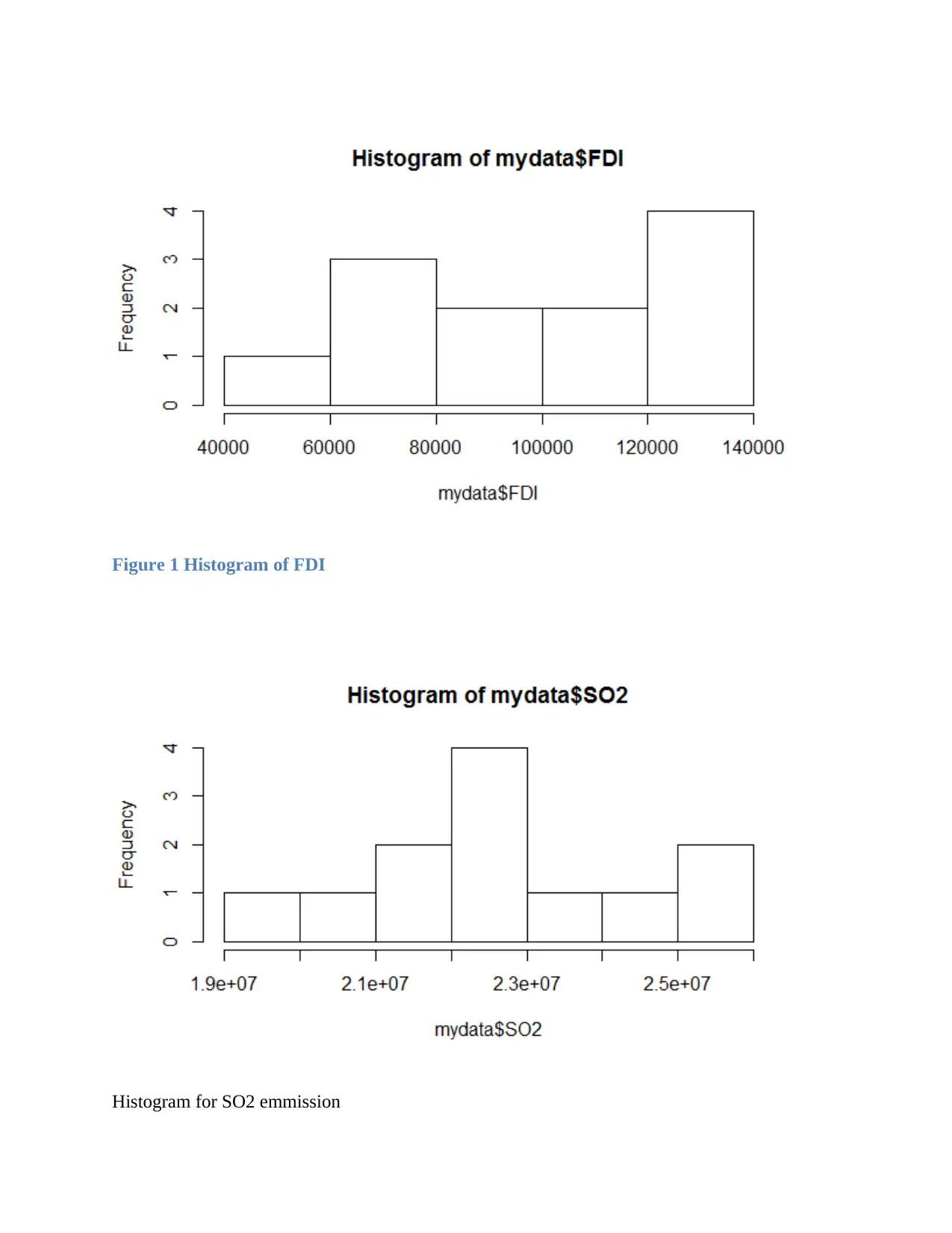
Figure 1 Histogram of FDI
Histogram for SO2 emmission
Histogram for SO2 emmission
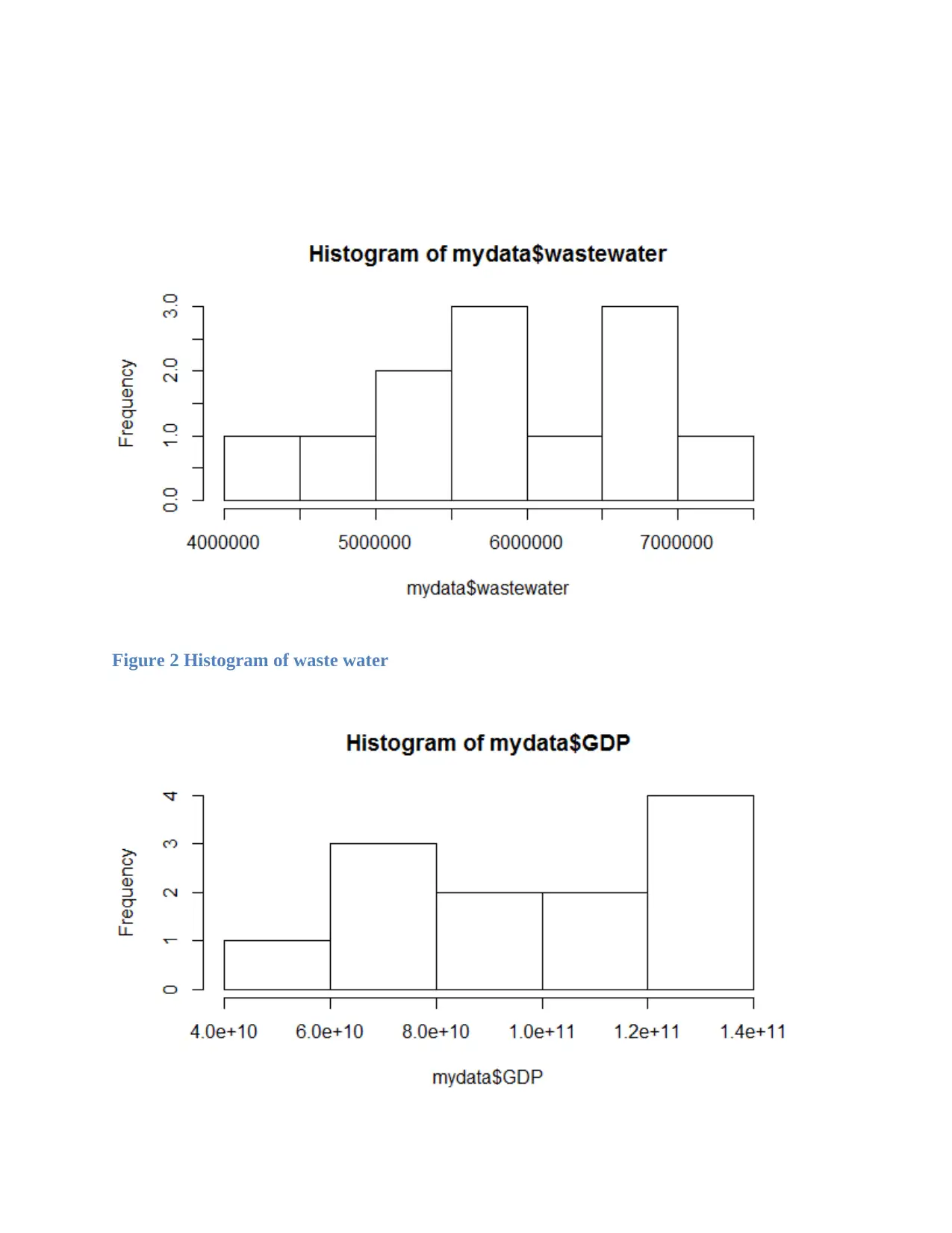
Figure 2 Histogram of waste water
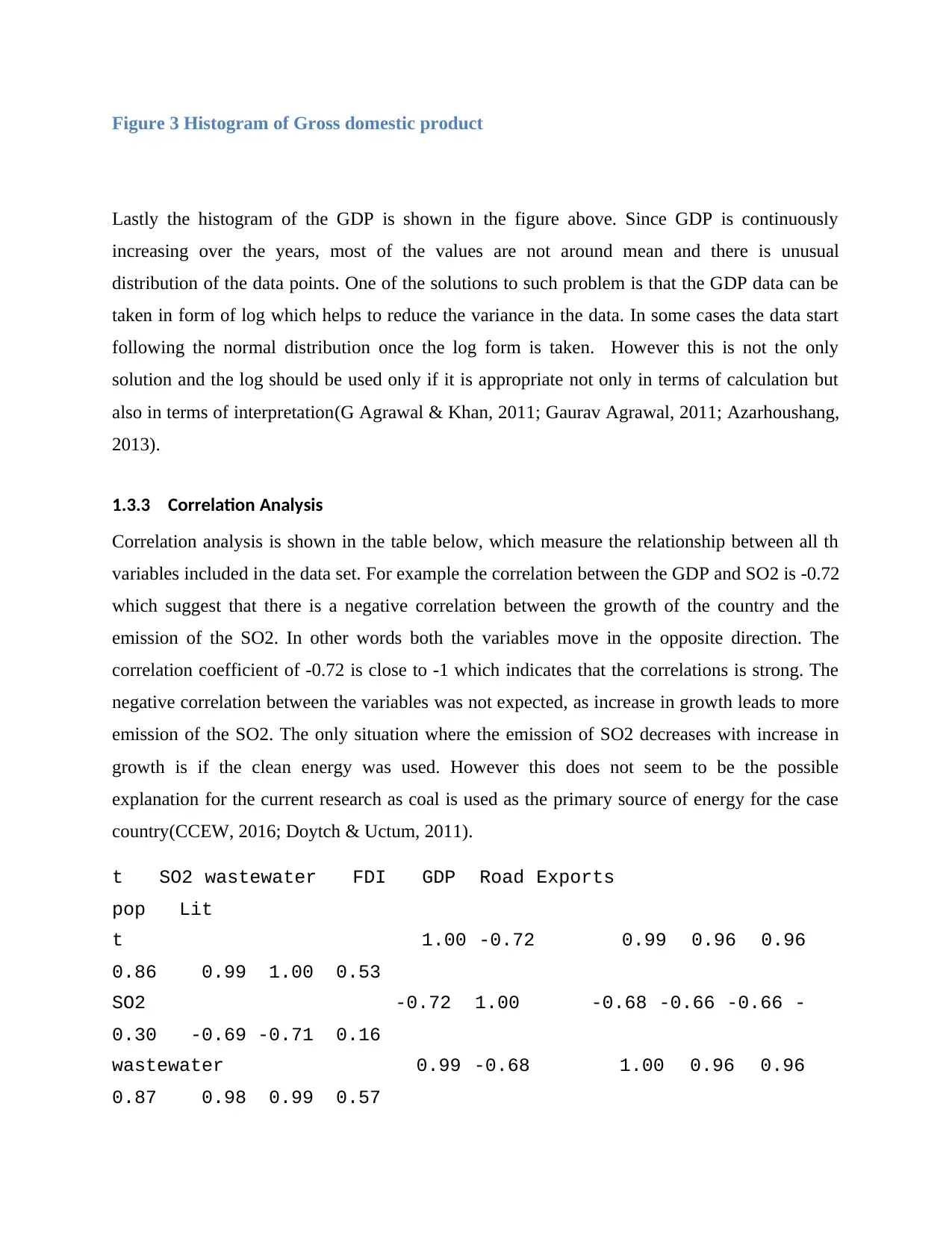
Figure 3 Histogram of Gross domestic product
Lastly the histogram of the GDP is shown in the figure above. Since GDP is continuously
increasing over the years, most of the values are not around mean and there is unusual
distribution of the data points. One of the solutions to such problem is that the GDP data can be
taken in form of log which helps to reduce the variance in the data. In some cases the data start
following the normal distribution once the log form is taken. However this is not the only
solution and the log should be used only if it is appropriate not only in terms of calculation but
also in terms of interpretation(G Agrawal & Khan, 2011; Gaurav Agrawal, 2011; Azarhoushang,
2013).
1.3.3 Correlation Analysis
Correlation analysis is shown in the table below, which measure the relationship between all th
variables included in the data set. For example the correlation between the GDP and SO2 is -0.72
which suggest that there is a negative correlation between the growth of the country and the
emission of the SO2. In other words both the variables move in the opposite direction. The
correlation coefficient of -0.72 is close to -1 which indicates that the correlations is strong. The
negative correlation between the variables was not expected, as increase in growth leads to more
emission of the SO2. The only situation where the emission of SO2 decreases with increase in
growth is if the clean energy was used. However this does not seem to be the possible
explanation for the current research as coal is used as the primary source of energy for the case
country(CCEW, 2016; Doytch & Uctum, 2011).
t SO2 wastewater FDI GDP Road Exports
pop Lit
t 1.00 -0.72 0.99 0.96 0.96
0.86 0.99 1.00 0.53
SO2 -0.72 1.00 -0.68 -0.66 -0.66 -
0.30 -0.69 -0.71 0.16
wastewater 0.99 -0.68 1.00 0.96 0.96
0.87 0.98 0.99 0.57
Lastly the histogram of the GDP is shown in the figure above. Since GDP is continuously
increasing over the years, most of the values are not around mean and there is unusual
distribution of the data points. One of the solutions to such problem is that the GDP data can be
taken in form of log which helps to reduce the variance in the data. In some cases the data start
following the normal distribution once the log form is taken. However this is not the only
solution and the log should be used only if it is appropriate not only in terms of calculation but
also in terms of interpretation(G Agrawal & Khan, 2011; Gaurav Agrawal, 2011; Azarhoushang,
2013).
1.3.3 Correlation Analysis
Correlation analysis is shown in the table below, which measure the relationship between all th
variables included in the data set. For example the correlation between the GDP and SO2 is -0.72
which suggest that there is a negative correlation between the growth of the country and the
emission of the SO2. In other words both the variables move in the opposite direction. The
correlation coefficient of -0.72 is close to -1 which indicates that the correlations is strong. The
negative correlation between the variables was not expected, as increase in growth leads to more
emission of the SO2. The only situation where the emission of SO2 decreases with increase in
growth is if the clean energy was used. However this does not seem to be the possible
explanation for the current research as coal is used as the primary source of energy for the case
country(CCEW, 2016; Doytch & Uctum, 2011).
t SO2 wastewater FDI GDP Road Exports
pop Lit
t 1.00 -0.72 0.99 0.96 0.96
0.86 0.99 1.00 0.53
SO2 -0.72 1.00 -0.68 -0.66 -0.66 -
0.30 -0.69 -0.71 0.16
wastewater 0.99 -0.68 1.00 0.96 0.96
0.87 0.98 0.99 0.57
Secure Best Marks with AI Grader
Need help grading? Try our AI Grader for instant feedback on your assignments.
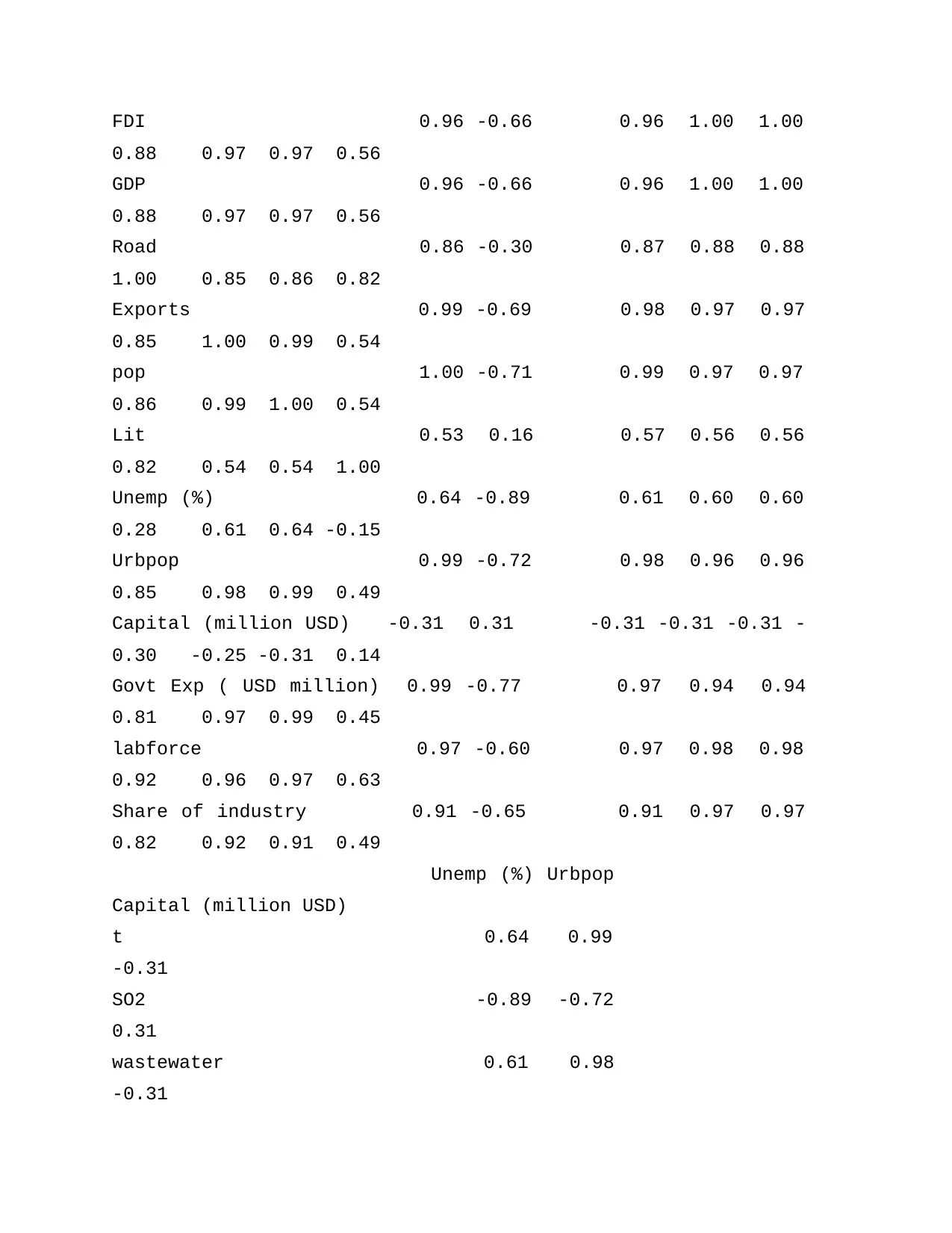
FDI 0.96 -0.66 0.96 1.00 1.00
0.88 0.97 0.97 0.56
GDP 0.96 -0.66 0.96 1.00 1.00
0.88 0.97 0.97 0.56
Road 0.86 -0.30 0.87 0.88 0.88
1.00 0.85 0.86 0.82
Exports 0.99 -0.69 0.98 0.97 0.97
0.85 1.00 0.99 0.54
pop 1.00 -0.71 0.99 0.97 0.97
0.86 0.99 1.00 0.54
Lit 0.53 0.16 0.57 0.56 0.56
0.82 0.54 0.54 1.00
Unemp (%) 0.64 -0.89 0.61 0.60 0.60
0.28 0.61 0.64 -0.15
Urbpop 0.99 -0.72 0.98 0.96 0.96
0.85 0.98 0.99 0.49
Capital (million USD) -0.31 0.31 -0.31 -0.31 -0.31 -
0.30 -0.25 -0.31 0.14
Govt Exp ( USD million) 0.99 -0.77 0.97 0.94 0.94
0.81 0.97 0.99 0.45
labforce 0.97 -0.60 0.97 0.98 0.98
0.92 0.96 0.97 0.63
Share of industry 0.91 -0.65 0.91 0.97 0.97
0.82 0.92 0.91 0.49
Unemp (%) Urbpop
Capital (million USD)
t 0.64 0.99
-0.31
SO2 -0.89 -0.72
0.31
wastewater 0.61 0.98
-0.31
0.88 0.97 0.97 0.56
GDP 0.96 -0.66 0.96 1.00 1.00
0.88 0.97 0.97 0.56
Road 0.86 -0.30 0.87 0.88 0.88
1.00 0.85 0.86 0.82
Exports 0.99 -0.69 0.98 0.97 0.97
0.85 1.00 0.99 0.54
pop 1.00 -0.71 0.99 0.97 0.97
0.86 0.99 1.00 0.54
Lit 0.53 0.16 0.57 0.56 0.56
0.82 0.54 0.54 1.00
Unemp (%) 0.64 -0.89 0.61 0.60 0.60
0.28 0.61 0.64 -0.15
Urbpop 0.99 -0.72 0.98 0.96 0.96
0.85 0.98 0.99 0.49
Capital (million USD) -0.31 0.31 -0.31 -0.31 -0.31 -
0.30 -0.25 -0.31 0.14
Govt Exp ( USD million) 0.99 -0.77 0.97 0.94 0.94
0.81 0.97 0.99 0.45
labforce 0.97 -0.60 0.97 0.98 0.98
0.92 0.96 0.97 0.63
Share of industry 0.91 -0.65 0.91 0.97 0.97
0.82 0.92 0.91 0.49
Unemp (%) Urbpop
Capital (million USD)
t 0.64 0.99
-0.31
SO2 -0.89 -0.72
0.31
wastewater 0.61 0.98
-0.31
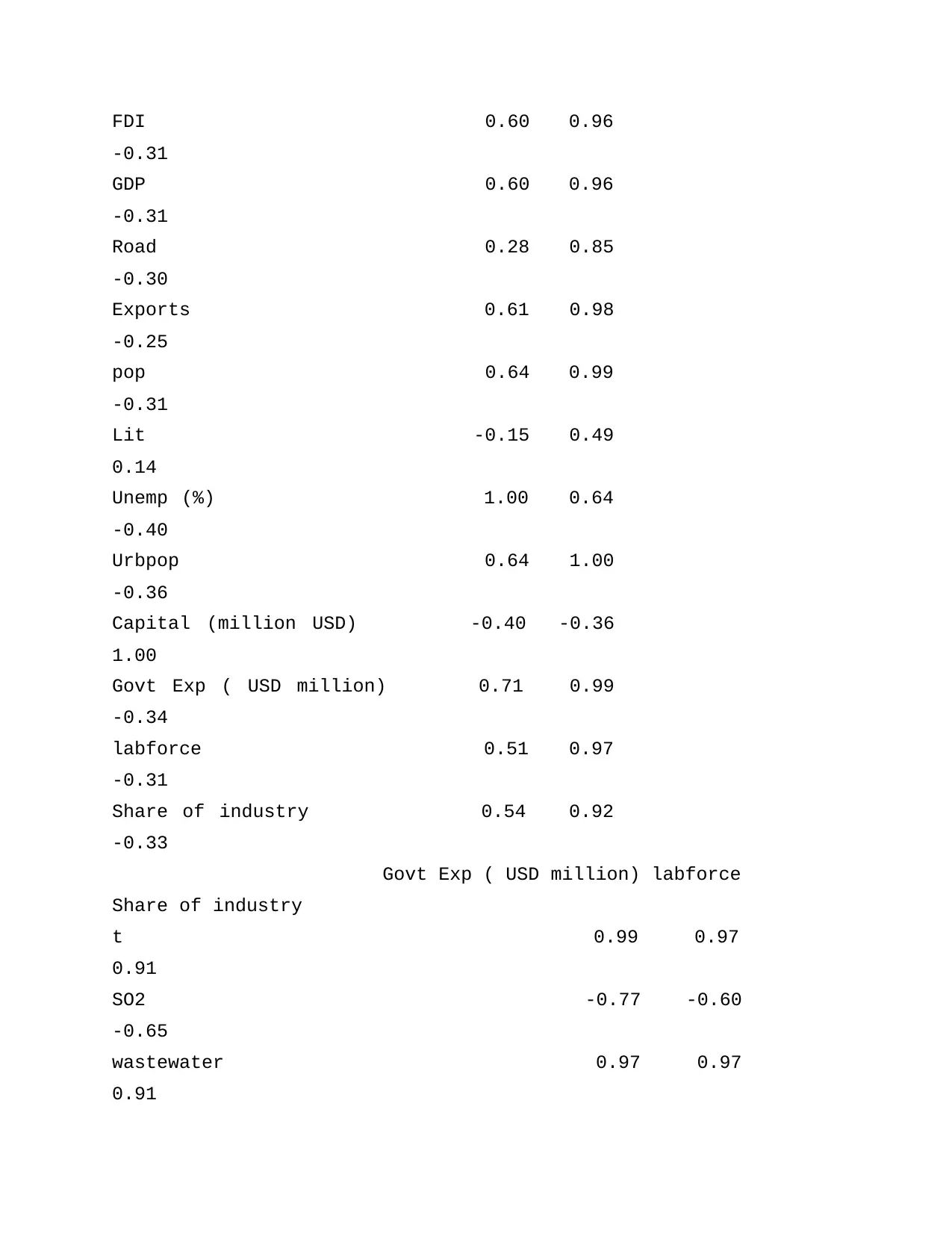
FDI 0.60 0.96
-0.31
GDP 0.60 0.96
-0.31
Road 0.28 0.85
-0.30
Exports 0.61 0.98
-0.25
pop 0.64 0.99
-0.31
Lit -0.15 0.49
0.14
Unemp (%) 1.00 0.64
-0.40
Urbpop 0.64 1.00
-0.36
Capital (million USD) -0.40 -0.36
1.00
Govt Exp ( USD million) 0.71 0.99
-0.34
labforce 0.51 0.97
-0.31
Share of industry 0.54 0.92
-0.33
Govt Exp ( USD million) labforce
Share of industry
t 0.99 0.97
0.91
SO2 -0.77 -0.60
-0.65
wastewater 0.97 0.97
0.91
-0.31
GDP 0.60 0.96
-0.31
Road 0.28 0.85
-0.30
Exports 0.61 0.98
-0.25
pop 0.64 0.99
-0.31
Lit -0.15 0.49
0.14
Unemp (%) 1.00 0.64
-0.40
Urbpop 0.64 1.00
-0.36
Capital (million USD) -0.40 -0.36
1.00
Govt Exp ( USD million) 0.71 0.99
-0.34
labforce 0.51 0.97
-0.31
Share of industry 0.54 0.92
-0.33
Govt Exp ( USD million) labforce
Share of industry
t 0.99 0.97
0.91
SO2 -0.77 -0.60
-0.65
wastewater 0.97 0.97
0.91
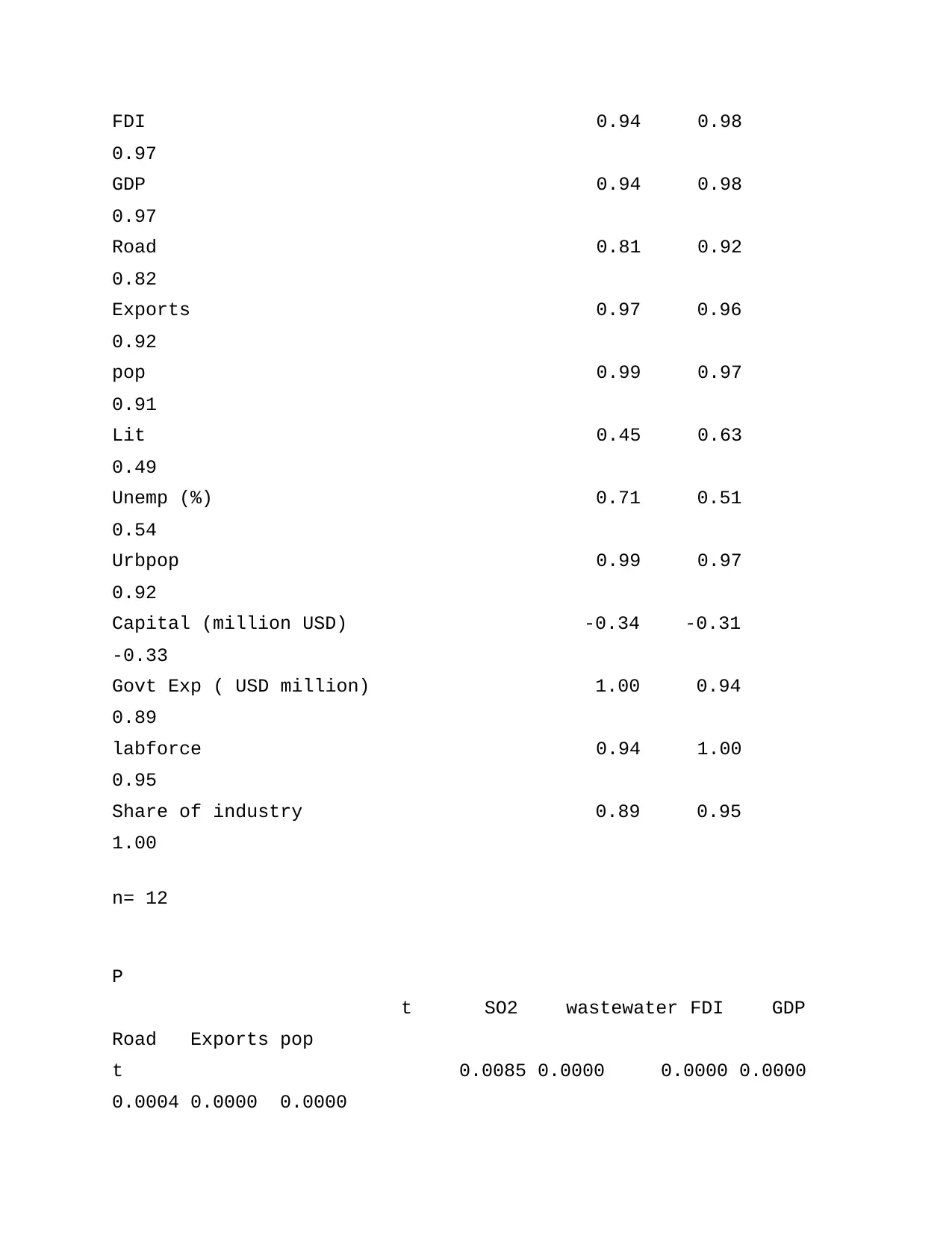
FDI 0.94 0.98
0.97
GDP 0.94 0.98
0.97
Road 0.81 0.92
0.82
Exports 0.97 0.96
0.92
pop 0.99 0.97
0.91
Lit 0.45 0.63
0.49
Unemp (%) 0.71 0.51
0.54
Urbpop 0.99 0.97
0.92
Capital (million USD) -0.34 -0.31
-0.33
Govt Exp ( USD million) 1.00 0.94
0.89
labforce 0.94 1.00
0.95
Share of industry 0.89 0.95
1.00
n= 12
P
t SO2 wastewater FDI GDP
Road Exports pop
t 0.0085 0.0000 0.0000 0.0000
0.0004 0.0000 0.0000
0.97
GDP 0.94 0.98
0.97
Road 0.81 0.92
0.82
Exports 0.97 0.96
0.92
pop 0.99 0.97
0.91
Lit 0.45 0.63
0.49
Unemp (%) 0.71 0.51
0.54
Urbpop 0.99 0.97
0.92
Capital (million USD) -0.34 -0.31
-0.33
Govt Exp ( USD million) 1.00 0.94
0.89
labforce 0.94 1.00
0.95
Share of industry 0.89 0.95
1.00
n= 12
P
t SO2 wastewater FDI GDP
Road Exports pop
t 0.0085 0.0000 0.0000 0.0000
0.0004 0.0000 0.0000
Paraphrase This Document
Need a fresh take? Get an instant paraphrase of this document with our AI Paraphraser
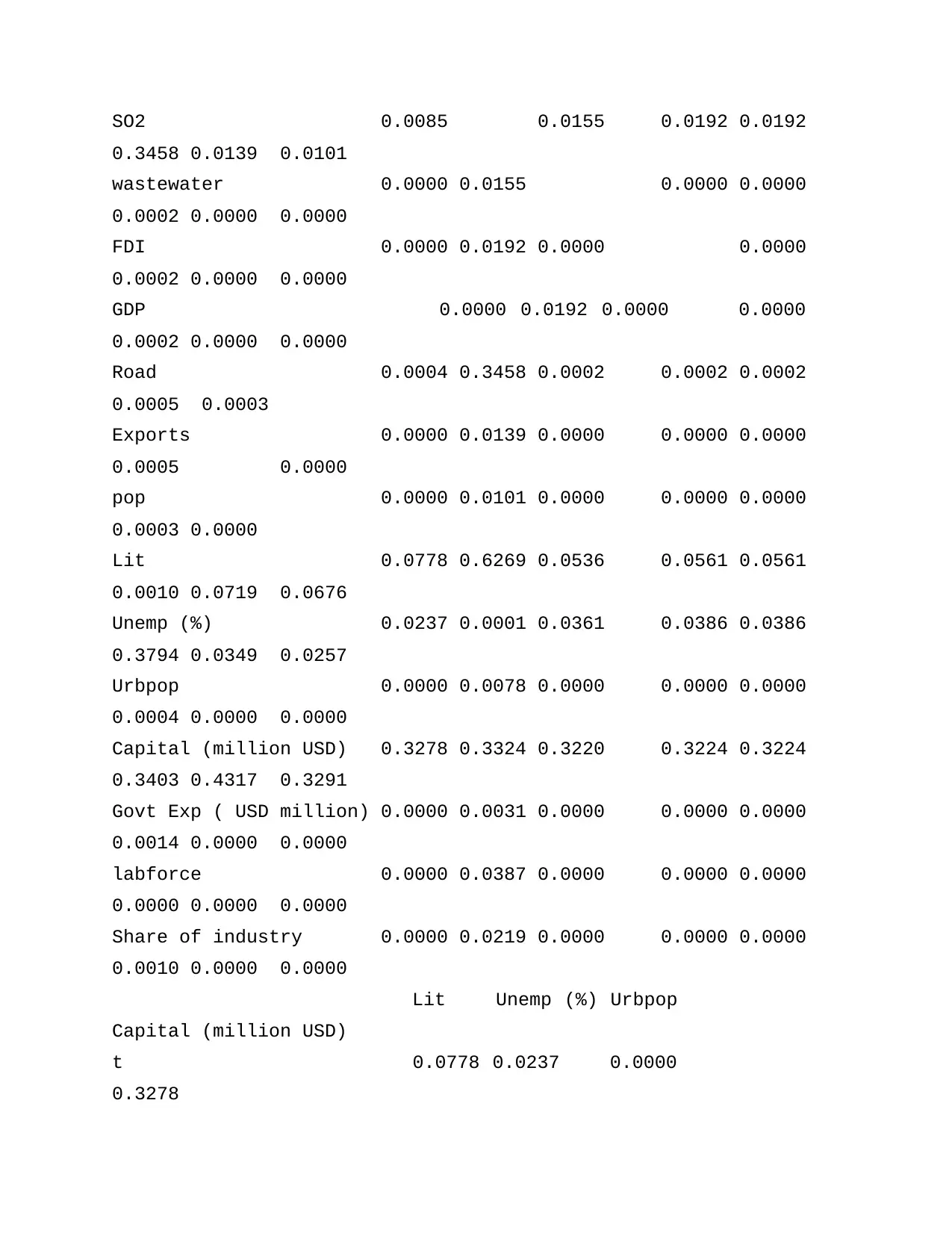
SO2 0.0085 0.0155 0.0192 0.0192
0.3458 0.0139 0.0101
wastewater 0.0000 0.0155 0.0000 0.0000
0.0002 0.0000 0.0000
FDI 0.0000 0.0192 0.0000 0.0000
0.0002 0.0000 0.0000
GDP 0.0000 0.0192 0.0000 0.0000
0.0002 0.0000 0.0000
Road 0.0004 0.3458 0.0002 0.0002 0.0002
0.0005 0.0003
Exports 0.0000 0.0139 0.0000 0.0000 0.0000
0.0005 0.0000
pop 0.0000 0.0101 0.0000 0.0000 0.0000
0.0003 0.0000
Lit 0.0778 0.6269 0.0536 0.0561 0.0561
0.0010 0.0719 0.0676
Unemp (%) 0.0237 0.0001 0.0361 0.0386 0.0386
0.3794 0.0349 0.0257
Urbpop 0.0000 0.0078 0.0000 0.0000 0.0000
0.0004 0.0000 0.0000
Capital (million USD) 0.3278 0.3324 0.3220 0.3224 0.3224
0.3403 0.4317 0.3291
Govt Exp ( USD million) 0.0000 0.0031 0.0000 0.0000 0.0000
0.0014 0.0000 0.0000
labforce 0.0000 0.0387 0.0000 0.0000 0.0000
0.0000 0.0000 0.0000
Share of industry 0.0000 0.0219 0.0000 0.0000 0.0000
0.0010 0.0000 0.0000
Lit Unemp (%) Urbpop
Capital (million USD)
t 0.0778 0.0237 0.0000
0.3278
0.3458 0.0139 0.0101
wastewater 0.0000 0.0155 0.0000 0.0000
0.0002 0.0000 0.0000
FDI 0.0000 0.0192 0.0000 0.0000
0.0002 0.0000 0.0000
GDP 0.0000 0.0192 0.0000 0.0000
0.0002 0.0000 0.0000
Road 0.0004 0.3458 0.0002 0.0002 0.0002
0.0005 0.0003
Exports 0.0000 0.0139 0.0000 0.0000 0.0000
0.0005 0.0000
pop 0.0000 0.0101 0.0000 0.0000 0.0000
0.0003 0.0000
Lit 0.0778 0.6269 0.0536 0.0561 0.0561
0.0010 0.0719 0.0676
Unemp (%) 0.0237 0.0001 0.0361 0.0386 0.0386
0.3794 0.0349 0.0257
Urbpop 0.0000 0.0078 0.0000 0.0000 0.0000
0.0004 0.0000 0.0000
Capital (million USD) 0.3278 0.3324 0.3220 0.3224 0.3224
0.3403 0.4317 0.3291
Govt Exp ( USD million) 0.0000 0.0031 0.0000 0.0000 0.0000
0.0014 0.0000 0.0000
labforce 0.0000 0.0387 0.0000 0.0000 0.0000
0.0000 0.0000 0.0000
Share of industry 0.0000 0.0219 0.0000 0.0000 0.0000
0.0010 0.0000 0.0000
Lit Unemp (%) Urbpop
Capital (million USD)
t 0.0778 0.0237 0.0000
0.3278
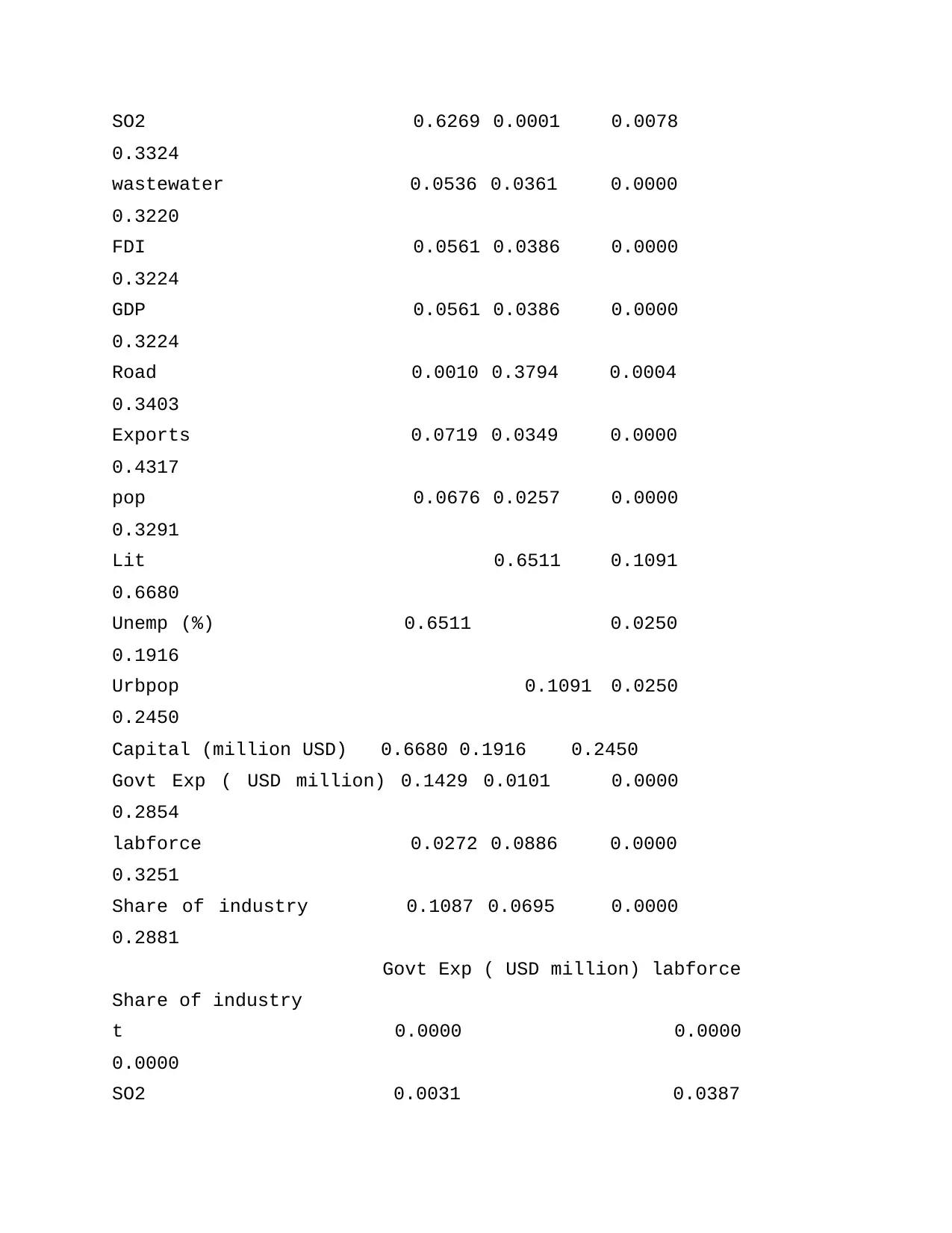
SO2 0.6269 0.0001 0.0078
0.3324
wastewater 0.0536 0.0361 0.0000
0.3220
FDI 0.0561 0.0386 0.0000
0.3224
GDP 0.0561 0.0386 0.0000
0.3224
Road 0.0010 0.3794 0.0004
0.3403
Exports 0.0719 0.0349 0.0000
0.4317
pop 0.0676 0.0257 0.0000
0.3291
Lit 0.6511 0.1091
0.6680
Unemp (%) 0.6511 0.0250
0.1916
Urbpop 0.1091 0.0250
0.2450
Capital (million USD) 0.6680 0.1916 0.2450
Govt Exp ( USD million) 0.1429 0.0101 0.0000
0.2854
labforce 0.0272 0.0886 0.0000
0.3251
Share of industry 0.1087 0.0695 0.0000
0.2881
Govt Exp ( USD million) labforce
Share of industry
t 0.0000 0.0000
0.0000
SO2 0.0031 0.0387
0.3324
wastewater 0.0536 0.0361 0.0000
0.3220
FDI 0.0561 0.0386 0.0000
0.3224
GDP 0.0561 0.0386 0.0000
0.3224
Road 0.0010 0.3794 0.0004
0.3403
Exports 0.0719 0.0349 0.0000
0.4317
pop 0.0676 0.0257 0.0000
0.3291
Lit 0.6511 0.1091
0.6680
Unemp (%) 0.6511 0.0250
0.1916
Urbpop 0.1091 0.0250
0.2450
Capital (million USD) 0.6680 0.1916 0.2450
Govt Exp ( USD million) 0.1429 0.0101 0.0000
0.2854
labforce 0.0272 0.0886 0.0000
0.3251
Share of industry 0.1087 0.0695 0.0000
0.2881
Govt Exp ( USD million) labforce
Share of industry
t 0.0000 0.0000
0.0000
SO2 0.0031 0.0387
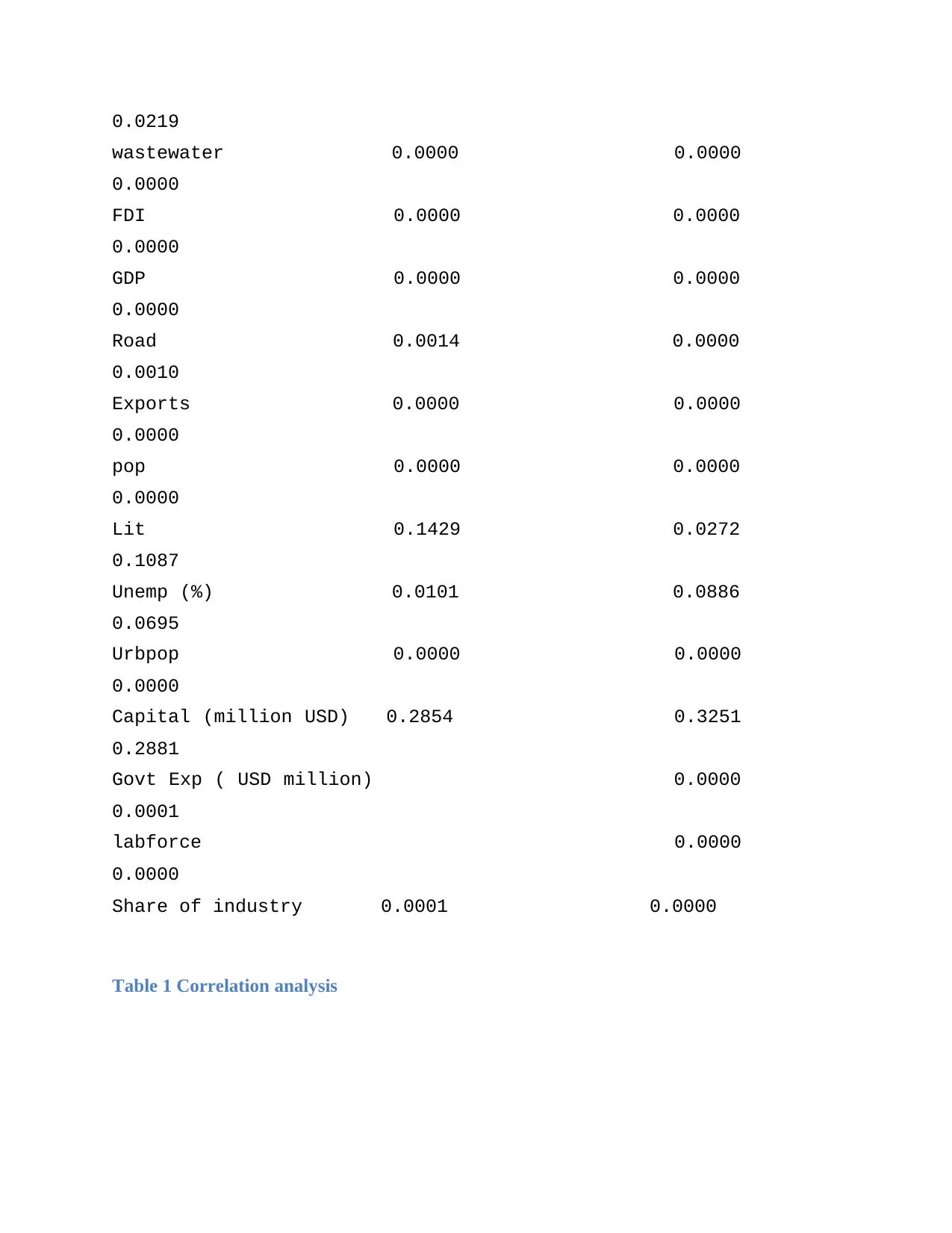
0.0219
wastewater 0.0000 0.0000
0.0000
FDI 0.0000 0.0000
0.0000
GDP 0.0000 0.0000
0.0000
Road 0.0014 0.0000
0.0010
Exports 0.0000 0.0000
0.0000
pop 0.0000 0.0000
0.0000
Lit 0.1429 0.0272
0.1087
Unemp (%) 0.0101 0.0886
0.0695
Urbpop 0.0000 0.0000
0.0000
Capital (million USD) 0.2854 0.3251
0.2881
Govt Exp ( USD million) 0.0000
0.0001
labforce 0.0000
0.0000
Share of industry 0.0001 0.0000
Table 1 Correlation analysis
wastewater 0.0000 0.0000
0.0000
FDI 0.0000 0.0000
0.0000
GDP 0.0000 0.0000
0.0000
Road 0.0014 0.0000
0.0010
Exports 0.0000 0.0000
0.0000
pop 0.0000 0.0000
0.0000
Lit 0.1429 0.0272
0.1087
Unemp (%) 0.0101 0.0886
0.0695
Urbpop 0.0000 0.0000
0.0000
Capital (million USD) 0.2854 0.3251
0.2881
Govt Exp ( USD million) 0.0000
0.0001
labforce 0.0000
0.0000
Share of industry 0.0001 0.0000
Table 1 Correlation analysis
Secure Best Marks with AI Grader
Need help grading? Try our AI Grader for instant feedback on your assignments.
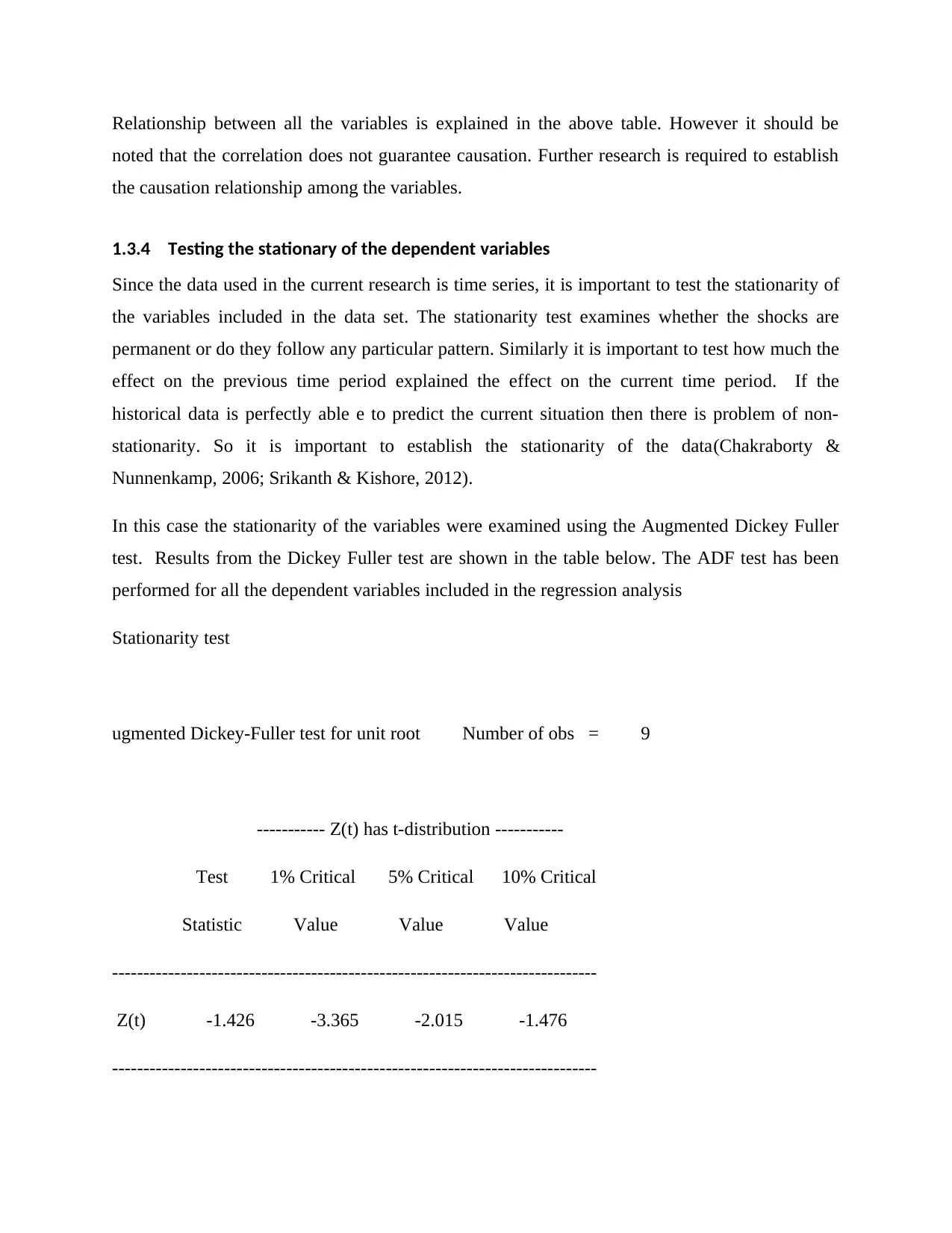
Relationship between all the variables is explained in the above table. However it should be
noted that the correlation does not guarantee causation. Further research is required to establish
the causation relationship among the variables.
1.3.4 Testing the stationary of the dependent variables
Since the data used in the current research is time series, it is important to test the stationarity of
the variables included in the data set. The stationarity test examines whether the shocks are
permanent or do they follow any particular pattern. Similarly it is important to test how much the
effect on the previous time period explained the effect on the current time period. If the
historical data is perfectly able e to predict the current situation then there is problem of non-
stationarity. So it is important to establish the stationarity of the data(Chakraborty &
Nunnenkamp, 2006; Srikanth & Kishore, 2012).
In this case the stationarity of the variables were examined using the Augmented Dickey Fuller
test. Results from the Dickey Fuller test are shown in the table below. The ADF test has been
performed for all the dependent variables included in the regression analysis
Stationarity test
ugmented Dickey-Fuller test for unit root Number of obs = 9
----------- Z(t) has t-distribution -----------
Test 1% Critical 5% Critical 10% Critical
Statistic Value Value Value
------------------------------------------------------------------------------
Z(t) -1.426 -3.365 -2.015 -1.476
------------------------------------------------------------------------------
noted that the correlation does not guarantee causation. Further research is required to establish
the causation relationship among the variables.
1.3.4 Testing the stationary of the dependent variables
Since the data used in the current research is time series, it is important to test the stationarity of
the variables included in the data set. The stationarity test examines whether the shocks are
permanent or do they follow any particular pattern. Similarly it is important to test how much the
effect on the previous time period explained the effect on the current time period. If the
historical data is perfectly able e to predict the current situation then there is problem of non-
stationarity. So it is important to establish the stationarity of the data(Chakraborty &
Nunnenkamp, 2006; Srikanth & Kishore, 2012).
In this case the stationarity of the variables were examined using the Augmented Dickey Fuller
test. Results from the Dickey Fuller test are shown in the table below. The ADF test has been
performed for all the dependent variables included in the regression analysis
Stationarity test
ugmented Dickey-Fuller test for unit root Number of obs = 9
----------- Z(t) has t-distribution -----------
Test 1% Critical 5% Critical 10% Critical
Statistic Value Value Value
------------------------------------------------------------------------------
Z(t) -1.426 -3.365 -2.015 -1.476
------------------------------------------------------------------------------
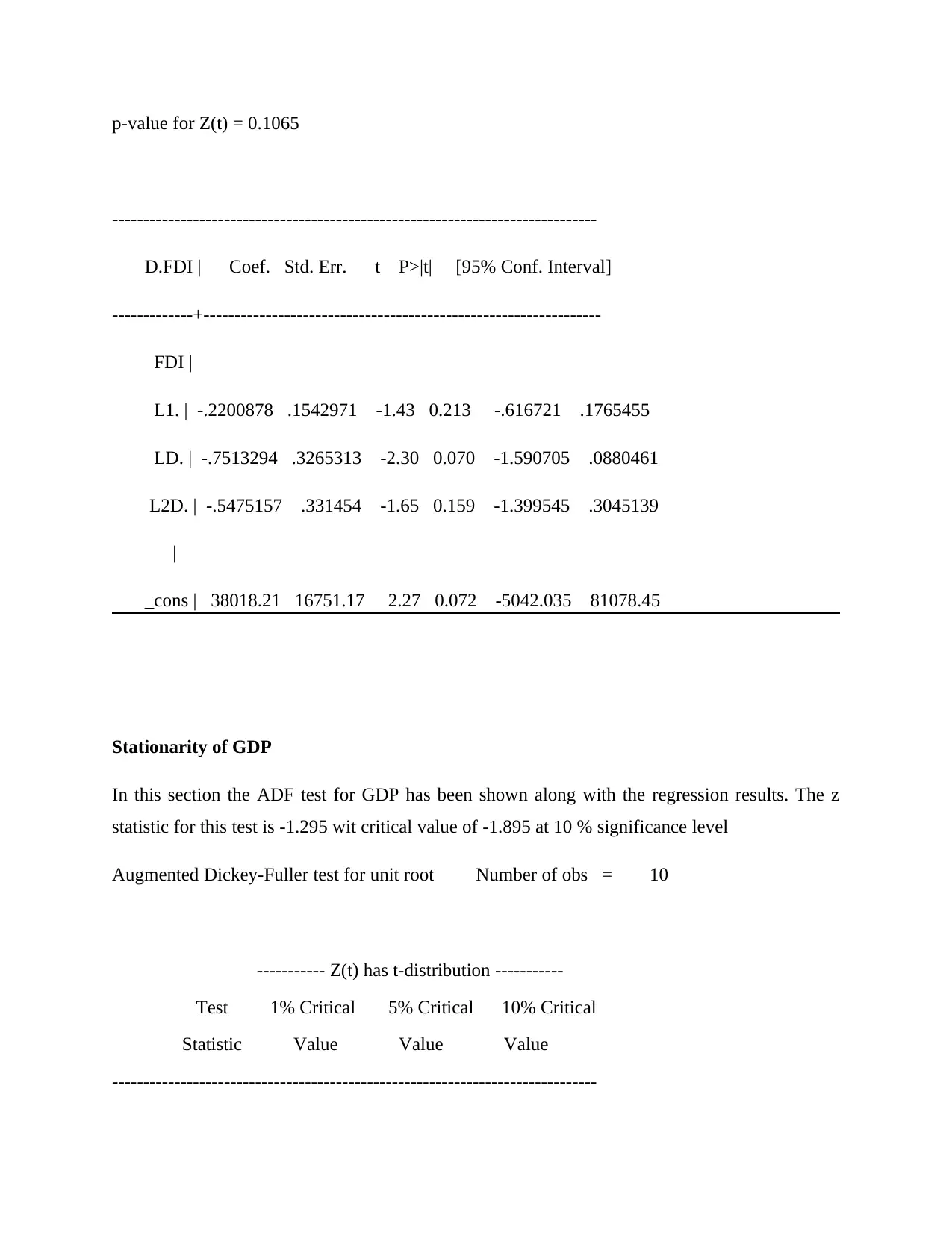
p-value for Z(t) = 0.1065
------------------------------------------------------------------------------
D.FDI | Coef. Std. Err. t P>|t| [95% Conf. Interval]
-------------+----------------------------------------------------------------
FDI |
L1. | -.2200878 .1542971 -1.43 0.213 -.616721 .1765455
LD. | -.7513294 .3265313 -2.30 0.070 -1.590705 .0880461
L2D. | -.5475157 .331454 -1.65 0.159 -1.399545 .3045139
|
_cons | 38018.21 16751.17 2.27 0.072 -5042.035 81078.45
Stationarity of GDP
In this section the ADF test for GDP has been shown along with the regression results. The z
statistic for this test is -1.295 wit critical value of -1.895 at 10 % significance level
Augmented Dickey-Fuller test for unit root Number of obs = 10
----------- Z(t) has t-distribution -----------
Test 1% Critical 5% Critical 10% Critical
Statistic Value Value Value
------------------------------------------------------------------------------
------------------------------------------------------------------------------
D.FDI | Coef. Std. Err. t P>|t| [95% Conf. Interval]
-------------+----------------------------------------------------------------
FDI |
L1. | -.2200878 .1542971 -1.43 0.213 -.616721 .1765455
LD. | -.7513294 .3265313 -2.30 0.070 -1.590705 .0880461
L2D. | -.5475157 .331454 -1.65 0.159 -1.399545 .3045139
|
_cons | 38018.21 16751.17 2.27 0.072 -5042.035 81078.45
Stationarity of GDP
In this section the ADF test for GDP has been shown along with the regression results. The z
statistic for this test is -1.295 wit critical value of -1.895 at 10 % significance level
Augmented Dickey-Fuller test for unit root Number of obs = 10
----------- Z(t) has t-distribution -----------
Test 1% Critical 5% Critical 10% Critical
Statistic Value Value Value
------------------------------------------------------------------------------
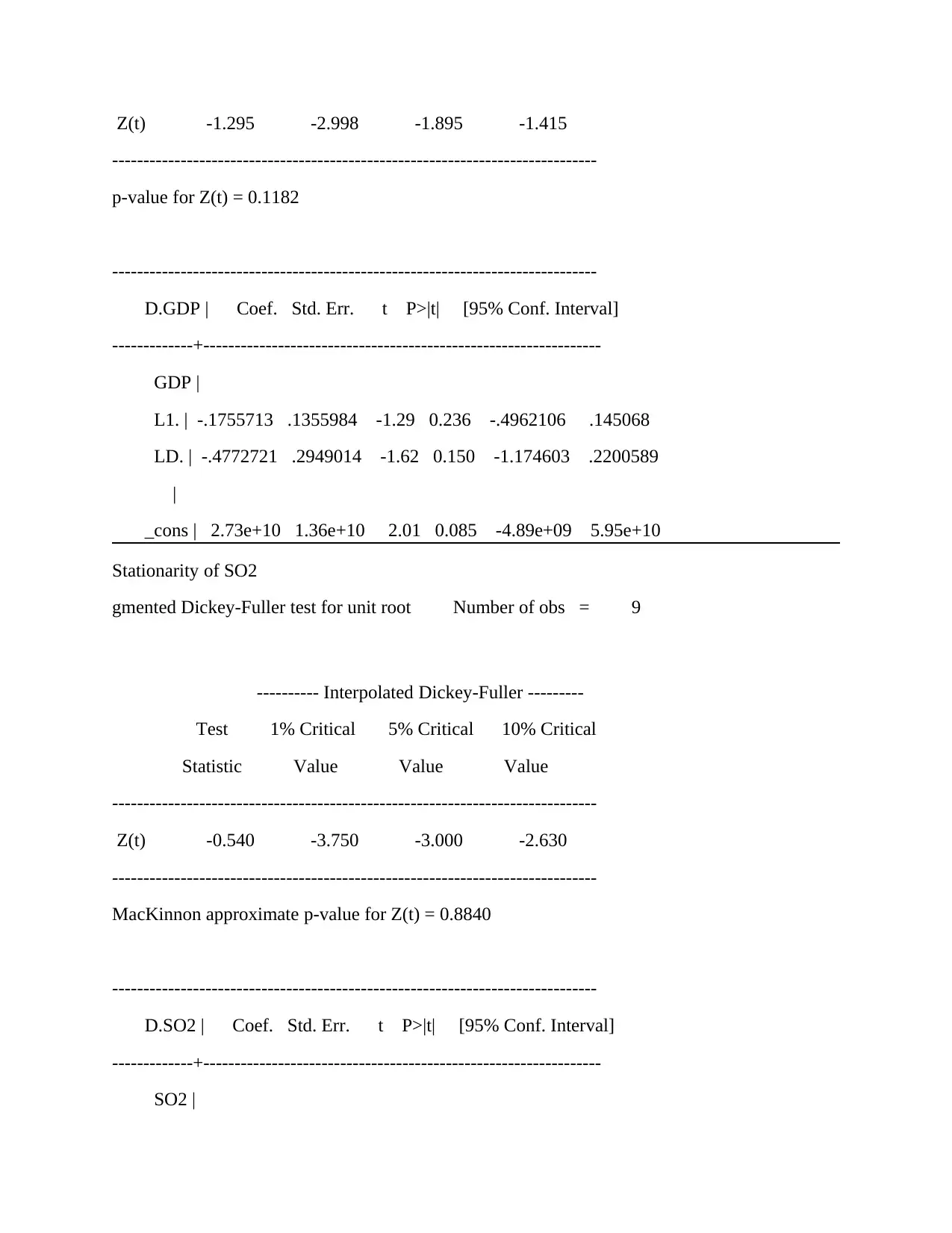
Z(t) -1.295 -2.998 -1.895 -1.415
------------------------------------------------------------------------------
p-value for Z(t) = 0.1182
------------------------------------------------------------------------------
D.GDP | Coef. Std. Err. t P>|t| [95% Conf. Interval]
-------------+----------------------------------------------------------------
GDP |
L1. | -.1755713 .1355984 -1.29 0.236 -.4962106 .145068
LD. | -.4772721 .2949014 -1.62 0.150 -1.174603 .2200589
|
_cons | 2.73e+10 1.36e+10 2.01 0.085 -4.89e+09 5.95e+10
Stationarity of SO2
gmented Dickey-Fuller test for unit root Number of obs = 9
---------- Interpolated Dickey-Fuller ---------
Test 1% Critical 5% Critical 10% Critical
Statistic Value Value Value
------------------------------------------------------------------------------
Z(t) -0.540 -3.750 -3.000 -2.630
------------------------------------------------------------------------------
MacKinnon approximate p-value for Z(t) = 0.8840
------------------------------------------------------------------------------
D.SO2 | Coef. Std. Err. t P>|t| [95% Conf. Interval]
-------------+----------------------------------------------------------------
SO2 |
------------------------------------------------------------------------------
p-value for Z(t) = 0.1182
------------------------------------------------------------------------------
D.GDP | Coef. Std. Err. t P>|t| [95% Conf. Interval]
-------------+----------------------------------------------------------------
GDP |
L1. | -.1755713 .1355984 -1.29 0.236 -.4962106 .145068
LD. | -.4772721 .2949014 -1.62 0.150 -1.174603 .2200589
|
_cons | 2.73e+10 1.36e+10 2.01 0.085 -4.89e+09 5.95e+10
Stationarity of SO2
gmented Dickey-Fuller test for unit root Number of obs = 9
---------- Interpolated Dickey-Fuller ---------
Test 1% Critical 5% Critical 10% Critical
Statistic Value Value Value
------------------------------------------------------------------------------
Z(t) -0.540 -3.750 -3.000 -2.630
------------------------------------------------------------------------------
MacKinnon approximate p-value for Z(t) = 0.8840
------------------------------------------------------------------------------
D.SO2 | Coef. Std. Err. t P>|t| [95% Conf. Interval]
-------------+----------------------------------------------------------------
SO2 |
Paraphrase This Document
Need a fresh take? Get an instant paraphrase of this document with our AI Paraphraser
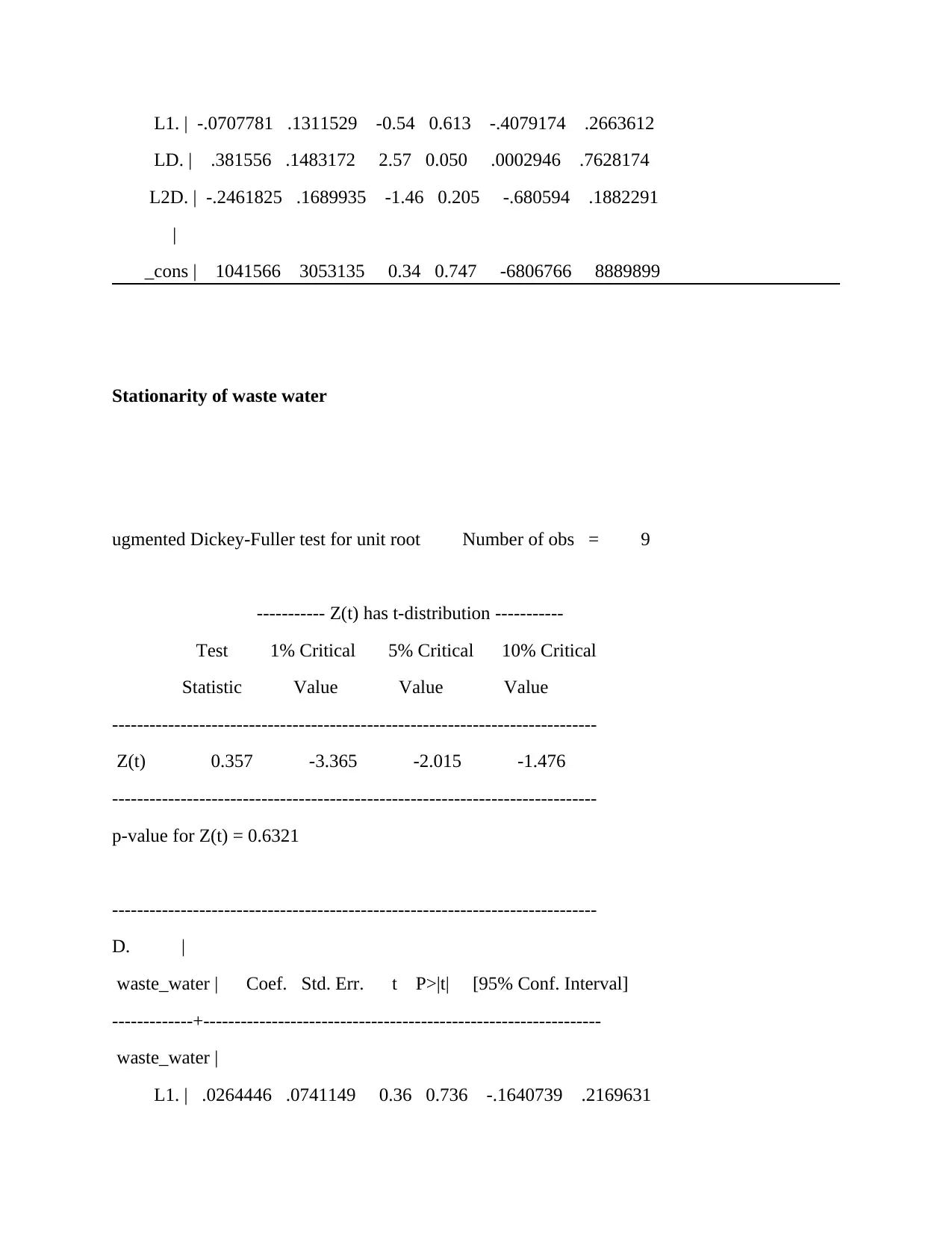
L1. | -.0707781 .1311529 -0.54 0.613 -.4079174 .2663612
LD. | .381556 .1483172 2.57 0.050 .0002946 .7628174
L2D. | -.2461825 .1689935 -1.46 0.205 -.680594 .1882291
|
_cons | 1041566 3053135 0.34 0.747 -6806766 8889899
Stationarity of waste water
ugmented Dickey-Fuller test for unit root Number of obs = 9
----------- Z(t) has t-distribution -----------
Test 1% Critical 5% Critical 10% Critical
Statistic Value Value Value
------------------------------------------------------------------------------
Z(t) 0.357 -3.365 -2.015 -1.476
------------------------------------------------------------------------------
p-value for Z(t) = 0.6321
------------------------------------------------------------------------------
D. |
waste_water | Coef. Std. Err. t P>|t| [95% Conf. Interval]
-------------+----------------------------------------------------------------
waste_water |
L1. | .0264446 .0741149 0.36 0.736 -.1640739 .2169631
LD. | .381556 .1483172 2.57 0.050 .0002946 .7628174
L2D. | -.2461825 .1689935 -1.46 0.205 -.680594 .1882291
|
_cons | 1041566 3053135 0.34 0.747 -6806766 8889899
Stationarity of waste water
ugmented Dickey-Fuller test for unit root Number of obs = 9
----------- Z(t) has t-distribution -----------
Test 1% Critical 5% Critical 10% Critical
Statistic Value Value Value
------------------------------------------------------------------------------
Z(t) 0.357 -3.365 -2.015 -1.476
------------------------------------------------------------------------------
p-value for Z(t) = 0.6321
------------------------------------------------------------------------------
D. |
waste_water | Coef. Std. Err. t P>|t| [95% Conf. Interval]
-------------+----------------------------------------------------------------
waste_water |
L1. | .0264446 .0741149 0.36 0.736 -.1640739 .2169631
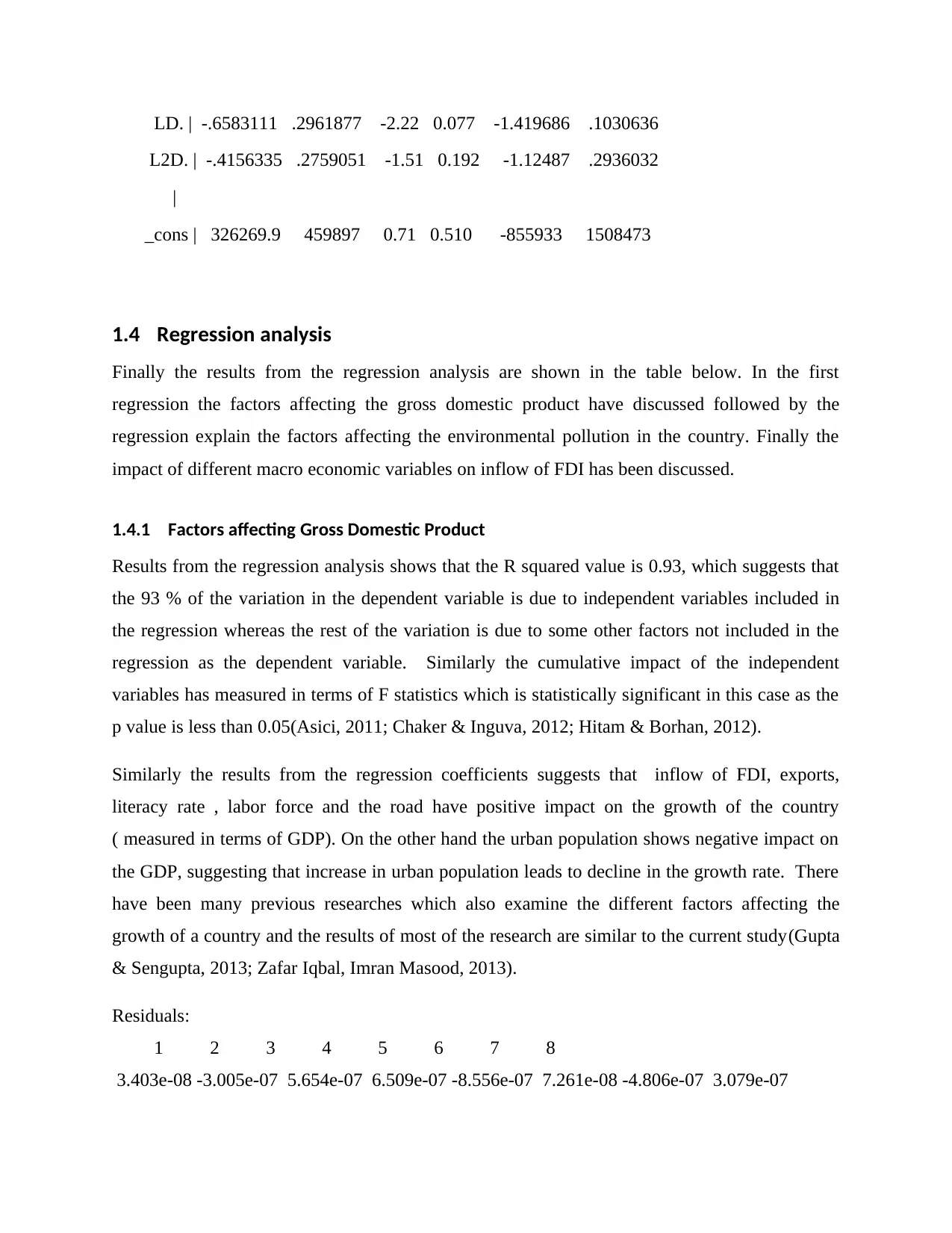
LD. | -.6583111 .2961877 -2.22 0.077 -1.419686 .1030636
L2D. | -.4156335 .2759051 -1.51 0.192 -1.12487 .2936032
|
_cons | 326269.9 459897 0.71 0.510 -855933 1508473
1.4 Regression analysis
Finally the results from the regression analysis are shown in the table below. In the first
regression the factors affecting the gross domestic product have discussed followed by the
regression explain the factors affecting the environmental pollution in the country. Finally the
impact of different macro economic variables on inflow of FDI has been discussed.
1.4.1 Factors affecting Gross Domestic Product
Results from the regression analysis shows that the R squared value is 0.93, which suggests that
the 93 % of the variation in the dependent variable is due to independent variables included in
the regression whereas the rest of the variation is due to some other factors not included in the
regression as the dependent variable. Similarly the cumulative impact of the independent
variables has measured in terms of F statistics which is statistically significant in this case as the
p value is less than 0.05(Asici, 2011; Chaker & Inguva, 2012; Hitam & Borhan, 2012).
Similarly the results from the regression coefficients suggests that inflow of FDI, exports,
literacy rate , labor force and the road have positive impact on the growth of the country
( measured in terms of GDP). On the other hand the urban population shows negative impact on
the GDP, suggesting that increase in urban population leads to decline in the growth rate. There
have been many previous researches which also examine the different factors affecting the
growth of a country and the results of most of the research are similar to the current study(Gupta
& Sengupta, 2013; Zafar Iqbal, Imran Masood, 2013).
Residuals:
1 2 3 4 5 6 7 8
3.403e-08 -3.005e-07 5.654e-07 6.509e-07 -8.556e-07 7.261e-08 -4.806e-07 3.079e-07
L2D. | -.4156335 .2759051 -1.51 0.192 -1.12487 .2936032
|
_cons | 326269.9 459897 0.71 0.510 -855933 1508473
1.4 Regression analysis
Finally the results from the regression analysis are shown in the table below. In the first
regression the factors affecting the gross domestic product have discussed followed by the
regression explain the factors affecting the environmental pollution in the country. Finally the
impact of different macro economic variables on inflow of FDI has been discussed.
1.4.1 Factors affecting Gross Domestic Product
Results from the regression analysis shows that the R squared value is 0.93, which suggests that
the 93 % of the variation in the dependent variable is due to independent variables included in
the regression whereas the rest of the variation is due to some other factors not included in the
regression as the dependent variable. Similarly the cumulative impact of the independent
variables has measured in terms of F statistics which is statistically significant in this case as the
p value is less than 0.05(Asici, 2011; Chaker & Inguva, 2012; Hitam & Borhan, 2012).
Similarly the results from the regression coefficients suggests that inflow of FDI, exports,
literacy rate , labor force and the road have positive impact on the growth of the country
( measured in terms of GDP). On the other hand the urban population shows negative impact on
the GDP, suggesting that increase in urban population leads to decline in the growth rate. There
have been many previous researches which also examine the different factors affecting the
growth of a country and the results of most of the research are similar to the current study(Gupta
& Sengupta, 2013; Zafar Iqbal, Imran Masood, 2013).
Residuals:
1 2 3 4 5 6 7 8
3.403e-08 -3.005e-07 5.654e-07 6.509e-07 -8.556e-07 7.261e-08 -4.806e-07 3.079e-07
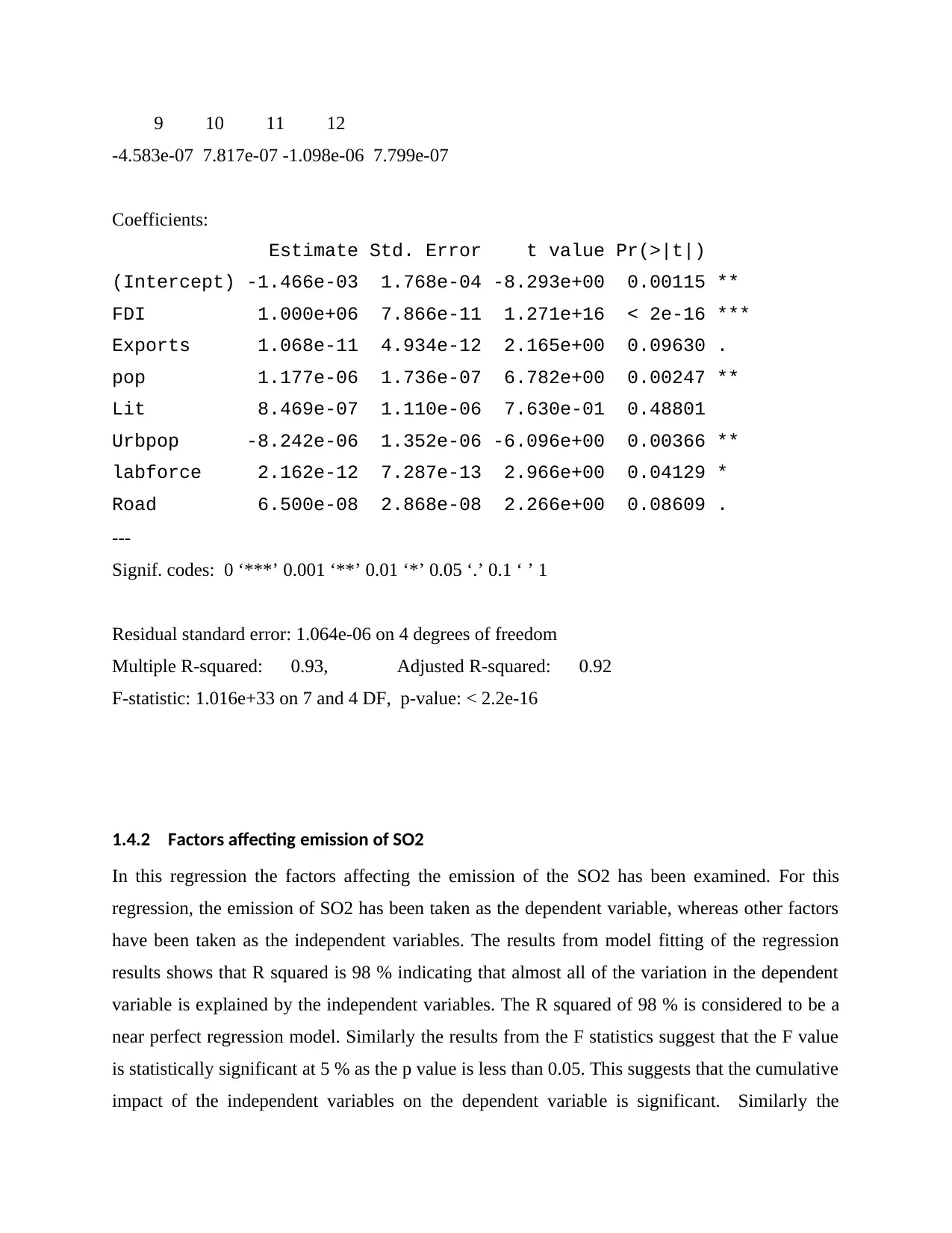
9 10 11 12
-4.583e-07 7.817e-07 -1.098e-06 7.799e-07
Coefficients:
Estimate Std. Error t value Pr(>|t|)
(Intercept) -1.466e-03 1.768e-04 -8.293e+00 0.00115 **
FDI 1.000e+06 7.866e-11 1.271e+16 < 2e-16 ***
Exports 1.068e-11 4.934e-12 2.165e+00 0.09630 .
pop 1.177e-06 1.736e-07 6.782e+00 0.00247 **
Lit 8.469e-07 1.110e-06 7.630e-01 0.48801
Urbpop -8.242e-06 1.352e-06 -6.096e+00 0.00366 **
labforce 2.162e-12 7.287e-13 2.966e+00 0.04129 *
Road 6.500e-08 2.868e-08 2.266e+00 0.08609 .
---
Signif. codes: 0 ‘***’ 0.001 ‘**’ 0.01 ‘*’ 0.05 ‘.’ 0.1 ‘ ’ 1
Residual standard error: 1.064e-06 on 4 degrees of freedom
Multiple R-squared: 0.93, Adjusted R-squared: 0.92
F-statistic: 1.016e+33 on 7 and 4 DF, p-value: < 2.2e-16
1.4.2 Factors affecting emission of SO2
In this regression the factors affecting the emission of the SO2 has been examined. For this
regression, the emission of SO2 has been taken as the dependent variable, whereas other factors
have been taken as the independent variables. The results from model fitting of the regression
results shows that R squared is 98 % indicating that almost all of the variation in the dependent
variable is explained by the independent variables. The R squared of 98 % is considered to be a
near perfect regression model. Similarly the results from the F statistics suggest that the F value
is statistically significant at 5 % as the p value is less than 0.05. This suggests that the cumulative
impact of the independent variables on the dependent variable is significant. Similarly the
-4.583e-07 7.817e-07 -1.098e-06 7.799e-07
Coefficients:
Estimate Std. Error t value Pr(>|t|)
(Intercept) -1.466e-03 1.768e-04 -8.293e+00 0.00115 **
FDI 1.000e+06 7.866e-11 1.271e+16 < 2e-16 ***
Exports 1.068e-11 4.934e-12 2.165e+00 0.09630 .
pop 1.177e-06 1.736e-07 6.782e+00 0.00247 **
Lit 8.469e-07 1.110e-06 7.630e-01 0.48801
Urbpop -8.242e-06 1.352e-06 -6.096e+00 0.00366 **
labforce 2.162e-12 7.287e-13 2.966e+00 0.04129 *
Road 6.500e-08 2.868e-08 2.266e+00 0.08609 .
---
Signif. codes: 0 ‘***’ 0.001 ‘**’ 0.01 ‘*’ 0.05 ‘.’ 0.1 ‘ ’ 1
Residual standard error: 1.064e-06 on 4 degrees of freedom
Multiple R-squared: 0.93, Adjusted R-squared: 0.92
F-statistic: 1.016e+33 on 7 and 4 DF, p-value: < 2.2e-16
1.4.2 Factors affecting emission of SO2
In this regression the factors affecting the emission of the SO2 has been examined. For this
regression, the emission of SO2 has been taken as the dependent variable, whereas other factors
have been taken as the independent variables. The results from model fitting of the regression
results shows that R squared is 98 % indicating that almost all of the variation in the dependent
variable is explained by the independent variables. The R squared of 98 % is considered to be a
near perfect regression model. Similarly the results from the F statistics suggest that the F value
is statistically significant at 5 % as the p value is less than 0.05. This suggests that the cumulative
impact of the independent variables on the dependent variable is significant. Similarly the
Secure Best Marks with AI Grader
Need help grading? Try our AI Grader for instant feedback on your assignments.
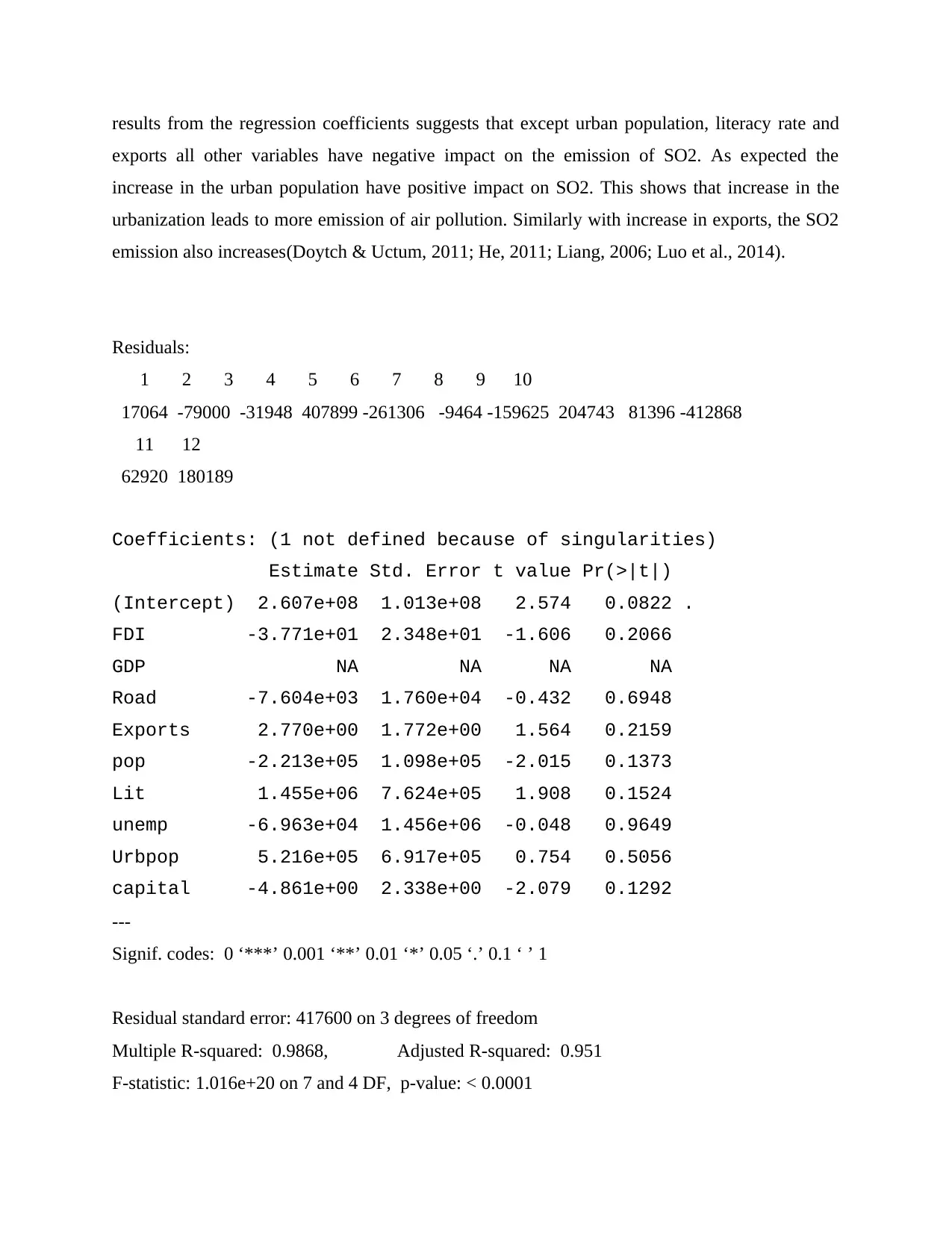
results from the regression coefficients suggests that except urban population, literacy rate and
exports all other variables have negative impact on the emission of SO2. As expected the
increase in the urban population have positive impact on SO2. This shows that increase in the
urbanization leads to more emission of air pollution. Similarly with increase in exports, the SO2
emission also increases(Doytch & Uctum, 2011; He, 2011; Liang, 2006; Luo et al., 2014).
Residuals:
1 2 3 4 5 6 7 8 9 10
17064 -79000 -31948 407899 -261306 -9464 -159625 204743 81396 -412868
11 12
62920 180189
Coefficients: (1 not defined because of singularities)
Estimate Std. Error t value Pr(>|t|)
(Intercept) 2.607e+08 1.013e+08 2.574 0.0822 .
FDI -3.771e+01 2.348e+01 -1.606 0.2066
GDP NA NA NA NA
Road -7.604e+03 1.760e+04 -0.432 0.6948
Exports 2.770e+00 1.772e+00 1.564 0.2159
pop -2.213e+05 1.098e+05 -2.015 0.1373
Lit 1.455e+06 7.624e+05 1.908 0.1524
unemp -6.963e+04 1.456e+06 -0.048 0.9649
Urbpop 5.216e+05 6.917e+05 0.754 0.5056
capital -4.861e+00 2.338e+00 -2.079 0.1292
---
Signif. codes: 0 ‘***’ 0.001 ‘**’ 0.01 ‘*’ 0.05 ‘.’ 0.1 ‘ ’ 1
Residual standard error: 417600 on 3 degrees of freedom
Multiple R-squared: 0.9868, Adjusted R-squared: 0.951
F-statistic: 1.016e+20 on 7 and 4 DF, p-value: < 0.0001
exports all other variables have negative impact on the emission of SO2. As expected the
increase in the urban population have positive impact on SO2. This shows that increase in the
urbanization leads to more emission of air pollution. Similarly with increase in exports, the SO2
emission also increases(Doytch & Uctum, 2011; He, 2011; Liang, 2006; Luo et al., 2014).
Residuals:
1 2 3 4 5 6 7 8 9 10
17064 -79000 -31948 407899 -261306 -9464 -159625 204743 81396 -412868
11 12
62920 180189
Coefficients: (1 not defined because of singularities)
Estimate Std. Error t value Pr(>|t|)
(Intercept) 2.607e+08 1.013e+08 2.574 0.0822 .
FDI -3.771e+01 2.348e+01 -1.606 0.2066
GDP NA NA NA NA
Road -7.604e+03 1.760e+04 -0.432 0.6948
Exports 2.770e+00 1.772e+00 1.564 0.2159
pop -2.213e+05 1.098e+05 -2.015 0.1373
Lit 1.455e+06 7.624e+05 1.908 0.1524
unemp -6.963e+04 1.456e+06 -0.048 0.9649
Urbpop 5.216e+05 6.917e+05 0.754 0.5056
capital -4.861e+00 2.338e+00 -2.079 0.1292
---
Signif. codes: 0 ‘***’ 0.001 ‘**’ 0.01 ‘*’ 0.05 ‘.’ 0.1 ‘ ’ 1
Residual standard error: 417600 on 3 degrees of freedom
Multiple R-squared: 0.9868, Adjusted R-squared: 0.951
F-statistic: 1.016e+20 on 7 and 4 DF, p-value: < 0.0001
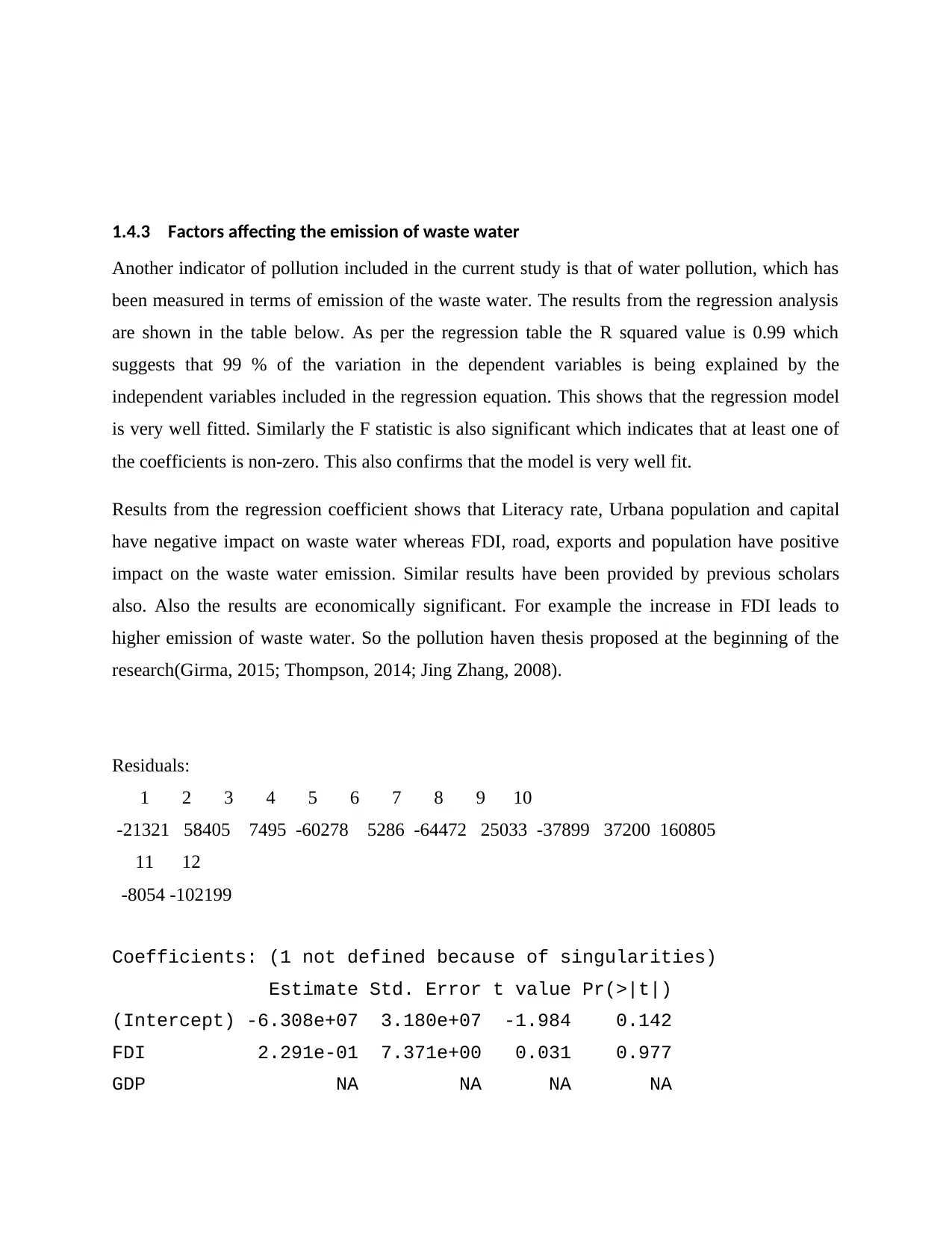
1.4.3 Factors affecting the emission of waste water
Another indicator of pollution included in the current study is that of water pollution, which has
been measured in terms of emission of the waste water. The results from the regression analysis
are shown in the table below. As per the regression table the R squared value is 0.99 which
suggests that 99 % of the variation in the dependent variables is being explained by the
independent variables included in the regression equation. This shows that the regression model
is very well fitted. Similarly the F statistic is also significant which indicates that at least one of
the coefficients is non-zero. This also confirms that the model is very well fit.
Results from the regression coefficient shows that Literacy rate, Urbana population and capital
have negative impact on waste water whereas FDI, road, exports and population have positive
impact on the waste water emission. Similar results have been provided by previous scholars
also. Also the results are economically significant. For example the increase in FDI leads to
higher emission of waste water. So the pollution haven thesis proposed at the beginning of the
research(Girma, 2015; Thompson, 2014; Jing Zhang, 2008).
Residuals:
1 2 3 4 5 6 7 8 9 10
-21321 58405 7495 -60278 5286 -64472 25033 -37899 37200 160805
11 12
-8054 -102199
Coefficients: (1 not defined because of singularities)
Estimate Std. Error t value Pr(>|t|)
(Intercept) -6.308e+07 3.180e+07 -1.984 0.142
FDI 2.291e-01 7.371e+00 0.031 0.977
GDP NA NA NA NA
Another indicator of pollution included in the current study is that of water pollution, which has
been measured in terms of emission of the waste water. The results from the regression analysis
are shown in the table below. As per the regression table the R squared value is 0.99 which
suggests that 99 % of the variation in the dependent variables is being explained by the
independent variables included in the regression equation. This shows that the regression model
is very well fitted. Similarly the F statistic is also significant which indicates that at least one of
the coefficients is non-zero. This also confirms that the model is very well fit.
Results from the regression coefficient shows that Literacy rate, Urbana population and capital
have negative impact on waste water whereas FDI, road, exports and population have positive
impact on the waste water emission. Similar results have been provided by previous scholars
also. Also the results are economically significant. For example the increase in FDI leads to
higher emission of waste water. So the pollution haven thesis proposed at the beginning of the
research(Girma, 2015; Thompson, 2014; Jing Zhang, 2008).
Residuals:
1 2 3 4 5 6 7 8 9 10
-21321 58405 7495 -60278 5286 -64472 25033 -37899 37200 160805
11 12
-8054 -102199
Coefficients: (1 not defined because of singularities)
Estimate Std. Error t value Pr(>|t|)
(Intercept) -6.308e+07 3.180e+07 -1.984 0.142
FDI 2.291e-01 7.371e+00 0.031 0.977
GDP NA NA NA NA
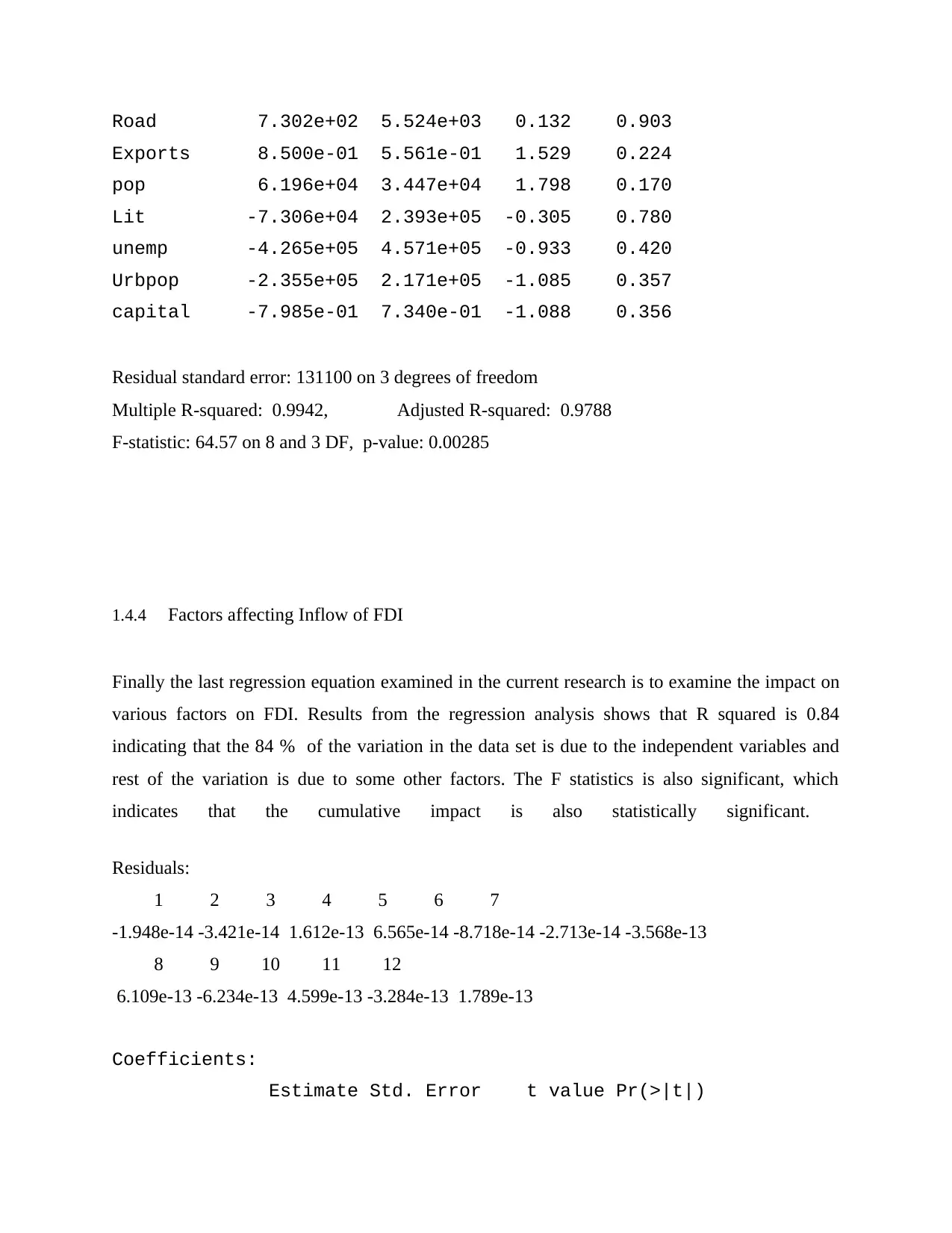
Road 7.302e+02 5.524e+03 0.132 0.903
Exports 8.500e-01 5.561e-01 1.529 0.224
pop 6.196e+04 3.447e+04 1.798 0.170
Lit -7.306e+04 2.393e+05 -0.305 0.780
unemp -4.265e+05 4.571e+05 -0.933 0.420
Urbpop -2.355e+05 2.171e+05 -1.085 0.357
capital -7.985e-01 7.340e-01 -1.088 0.356
Residual standard error: 131100 on 3 degrees of freedom
Multiple R-squared: 0.9942, Adjusted R-squared: 0.9788
F-statistic: 64.57 on 8 and 3 DF, p-value: 0.00285
1.4.4 Factors affecting Inflow of FDI
Finally the last regression equation examined in the current research is to examine the impact on
various factors on FDI. Results from the regression analysis shows that R squared is 0.84
indicating that the 84 % of the variation in the data set is due to the independent variables and
rest of the variation is due to some other factors. The F statistics is also significant, which
indicates that the cumulative impact is also statistically significant.
Residuals:
1 2 3 4 5 6 7
-1.948e-14 -3.421e-14 1.612e-13 6.565e-14 -8.718e-14 -2.713e-14 -3.568e-13
8 9 10 11 12
6.109e-13 -6.234e-13 4.599e-13 -3.284e-13 1.789e-13
Coefficients:
Estimate Std. Error t value Pr(>|t|)
Exports 8.500e-01 5.561e-01 1.529 0.224
pop 6.196e+04 3.447e+04 1.798 0.170
Lit -7.306e+04 2.393e+05 -0.305 0.780
unemp -4.265e+05 4.571e+05 -0.933 0.420
Urbpop -2.355e+05 2.171e+05 -1.085 0.357
capital -7.985e-01 7.340e-01 -1.088 0.356
Residual standard error: 131100 on 3 degrees of freedom
Multiple R-squared: 0.9942, Adjusted R-squared: 0.9788
F-statistic: 64.57 on 8 and 3 DF, p-value: 0.00285
1.4.4 Factors affecting Inflow of FDI
Finally the last regression equation examined in the current research is to examine the impact on
various factors on FDI. Results from the regression analysis shows that R squared is 0.84
indicating that the 84 % of the variation in the data set is due to the independent variables and
rest of the variation is due to some other factors. The F statistics is also significant, which
indicates that the cumulative impact is also statistically significant.
Residuals:
1 2 3 4 5 6 7
-1.948e-14 -3.421e-14 1.612e-13 6.565e-14 -8.718e-14 -2.713e-14 -3.568e-13
8 9 10 11 12
6.109e-13 -6.234e-13 4.599e-13 -3.284e-13 1.789e-13
Coefficients:
Estimate Std. Error t value Pr(>|t|)
Paraphrase This Document
Need a fresh take? Get an instant paraphrase of this document with our AI Paraphraser
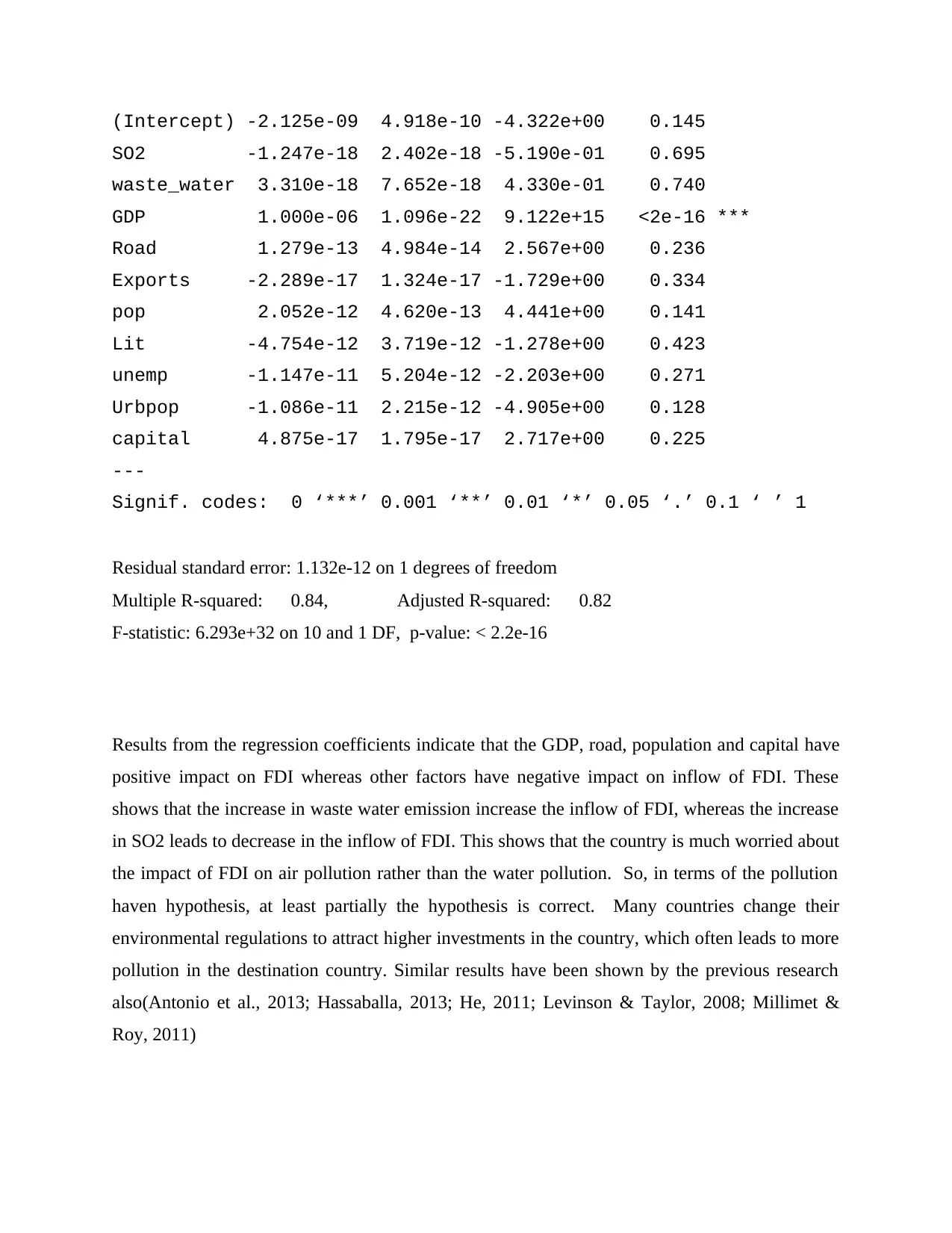
(Intercept) -2.125e-09 4.918e-10 -4.322e+00 0.145
SO2 -1.247e-18 2.402e-18 -5.190e-01 0.695
waste_water 3.310e-18 7.652e-18 4.330e-01 0.740
GDP 1.000e-06 1.096e-22 9.122e+15 <2e-16 ***
Road 1.279e-13 4.984e-14 2.567e+00 0.236
Exports -2.289e-17 1.324e-17 -1.729e+00 0.334
pop 2.052e-12 4.620e-13 4.441e+00 0.141
Lit -4.754e-12 3.719e-12 -1.278e+00 0.423
unemp -1.147e-11 5.204e-12 -2.203e+00 0.271
Urbpop -1.086e-11 2.215e-12 -4.905e+00 0.128
capital 4.875e-17 1.795e-17 2.717e+00 0.225
---
Signif. codes: 0 ‘***’ 0.001 ‘**’ 0.01 ‘*’ 0.05 ‘.’ 0.1 ‘ ’ 1
Residual standard error: 1.132e-12 on 1 degrees of freedom
Multiple R-squared: 0.84, Adjusted R-squared: 0.82
F-statistic: 6.293e+32 on 10 and 1 DF, p-value: < 2.2e-16
Results from the regression coefficients indicate that the GDP, road, population and capital have
positive impact on FDI whereas other factors have negative impact on inflow of FDI. These
shows that the increase in waste water emission increase the inflow of FDI, whereas the increase
in SO2 leads to decrease in the inflow of FDI. This shows that the country is much worried about
the impact of FDI on air pollution rather than the water pollution. So, in terms of the pollution
haven hypothesis, at least partially the hypothesis is correct. Many countries change their
environmental regulations to attract higher investments in the country, which often leads to more
pollution in the destination country. Similar results have been shown by the previous research
also(Antonio et al., 2013; Hassaballa, 2013; He, 2011; Levinson & Taylor, 2008; Millimet &
Roy, 2011)
SO2 -1.247e-18 2.402e-18 -5.190e-01 0.695
waste_water 3.310e-18 7.652e-18 4.330e-01 0.740
GDP 1.000e-06 1.096e-22 9.122e+15 <2e-16 ***
Road 1.279e-13 4.984e-14 2.567e+00 0.236
Exports -2.289e-17 1.324e-17 -1.729e+00 0.334
pop 2.052e-12 4.620e-13 4.441e+00 0.141
Lit -4.754e-12 3.719e-12 -1.278e+00 0.423
unemp -1.147e-11 5.204e-12 -2.203e+00 0.271
Urbpop -1.086e-11 2.215e-12 -4.905e+00 0.128
capital 4.875e-17 1.795e-17 2.717e+00 0.225
---
Signif. codes: 0 ‘***’ 0.001 ‘**’ 0.01 ‘*’ 0.05 ‘.’ 0.1 ‘ ’ 1
Residual standard error: 1.132e-12 on 1 degrees of freedom
Multiple R-squared: 0.84, Adjusted R-squared: 0.82
F-statistic: 6.293e+32 on 10 and 1 DF, p-value: < 2.2e-16
Results from the regression coefficients indicate that the GDP, road, population and capital have
positive impact on FDI whereas other factors have negative impact on inflow of FDI. These
shows that the increase in waste water emission increase the inflow of FDI, whereas the increase
in SO2 leads to decrease in the inflow of FDI. This shows that the country is much worried about
the impact of FDI on air pollution rather than the water pollution. So, in terms of the pollution
haven hypothesis, at least partially the hypothesis is correct. Many countries change their
environmental regulations to attract higher investments in the country, which often leads to more
pollution in the destination country. Similar results have been shown by the previous research
also(Antonio et al., 2013; Hassaballa, 2013; He, 2011; Levinson & Taylor, 2008; Millimet &
Roy, 2011)
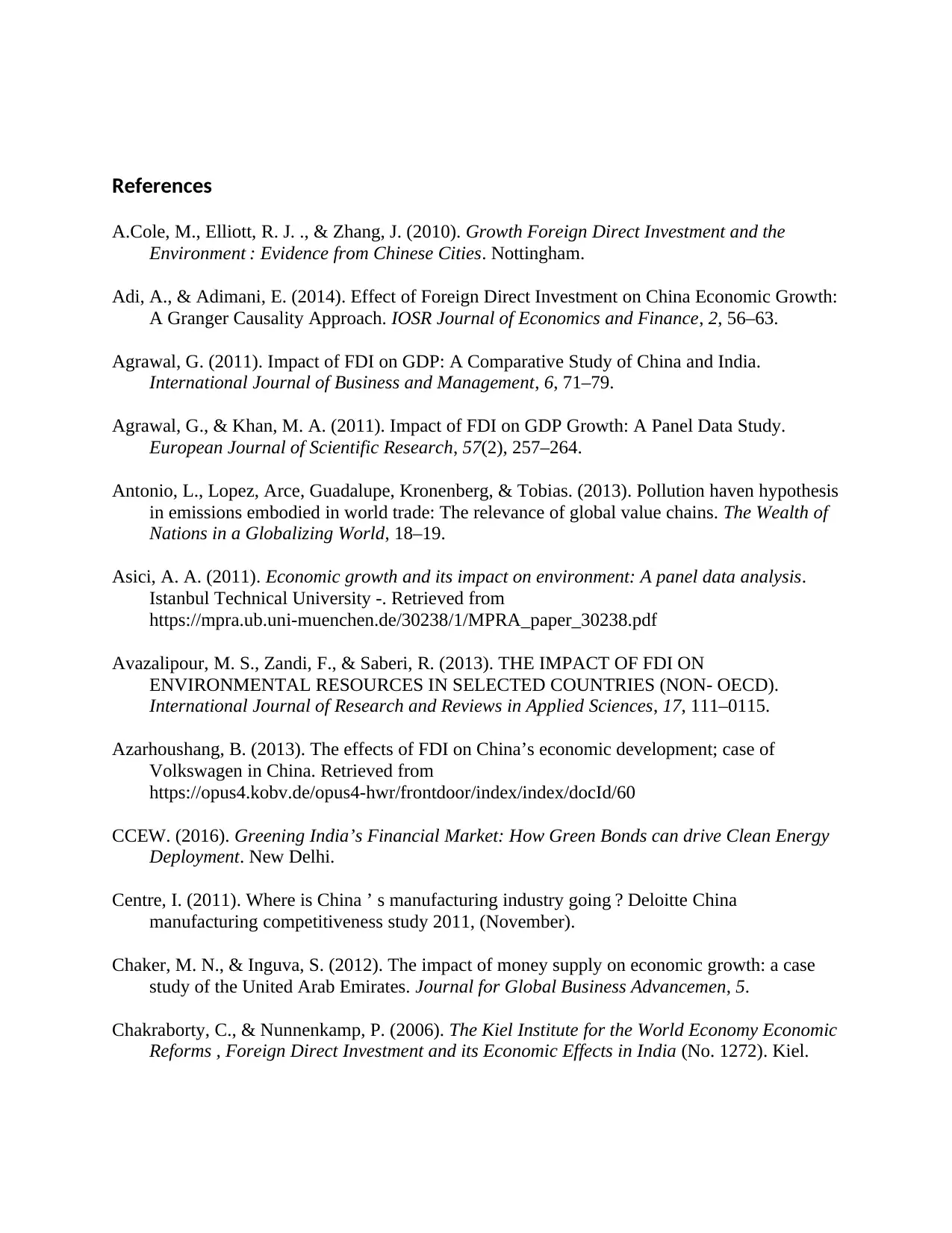
References
A.Cole, M., Elliott, R. J. ., & Zhang, J. (2010). Growth Foreign Direct Investment and the
Environment : Evidence from Chinese Cities. Nottingham.
Adi, A., & Adimani, E. (2014). Effect of Foreign Direct Investment on China Economic Growth:
A Granger Causality Approach. IOSR Journal of Economics and Finance, 2, 56–63.
Agrawal, G. (2011). Impact of FDI on GDP: A Comparative Study of China and India.
International Journal of Business and Management, 6, 71–79.
Agrawal, G., & Khan, M. A. (2011). Impact of FDI on GDP Growth: A Panel Data Study.
European Journal of Scientific Research, 57(2), 257–264.
Antonio, L., Lopez, Arce, Guadalupe, Kronenberg, & Tobias. (2013). Pollution haven hypothesis
in emissions embodied in world trade: The relevance of global value chains. The Wealth of
Nations in a Globalizing World, 18–19.
Asici, A. A. (2011). Economic growth and its impact on environment: A panel data analysis.
Istanbul Technical University -. Retrieved from
https://mpra.ub.uni-muenchen.de/30238/1/MPRA_paper_30238.pdf
Avazalipour, M. S., Zandi, F., & Saberi, R. (2013). THE IMPACT OF FDI ON
ENVIRONMENTAL RESOURCES IN SELECTED COUNTRIES (NON- OECD).
International Journal of Research and Reviews in Applied Sciences, 17, 111–0115.
Azarhoushang, B. (2013). The effects of FDI on China’s economic development; case of
Volkswagen in China. Retrieved from
https://opus4.kobv.de/opus4-hwr/frontdoor/index/index/docId/60
CCEW. (2016). Greening India’s Financial Market: How Green Bonds can drive Clean Energy
Deployment. New Delhi.
Centre, I. (2011). Where is China ’ s manufacturing industry going ? Deloitte China
manufacturing competitiveness study 2011, (November).
Chaker, M. N., & Inguva, S. (2012). The impact of money supply on economic growth: a case
study of the United Arab Emirates. Journal for Global Business Advancemen, 5.
Chakraborty, C., & Nunnenkamp, P. (2006). The Kiel Institute for the World Economy Economic
Reforms , Foreign Direct Investment and its Economic Effects in India (No. 1272). Kiel.
A.Cole, M., Elliott, R. J. ., & Zhang, J. (2010). Growth Foreign Direct Investment and the
Environment : Evidence from Chinese Cities. Nottingham.
Adi, A., & Adimani, E. (2014). Effect of Foreign Direct Investment on China Economic Growth:
A Granger Causality Approach. IOSR Journal of Economics and Finance, 2, 56–63.
Agrawal, G. (2011). Impact of FDI on GDP: A Comparative Study of China and India.
International Journal of Business and Management, 6, 71–79.
Agrawal, G., & Khan, M. A. (2011). Impact of FDI on GDP Growth: A Panel Data Study.
European Journal of Scientific Research, 57(2), 257–264.
Antonio, L., Lopez, Arce, Guadalupe, Kronenberg, & Tobias. (2013). Pollution haven hypothesis
in emissions embodied in world trade: The relevance of global value chains. The Wealth of
Nations in a Globalizing World, 18–19.
Asici, A. A. (2011). Economic growth and its impact on environment: A panel data analysis.
Istanbul Technical University -. Retrieved from
https://mpra.ub.uni-muenchen.de/30238/1/MPRA_paper_30238.pdf
Avazalipour, M. S., Zandi, F., & Saberi, R. (2013). THE IMPACT OF FDI ON
ENVIRONMENTAL RESOURCES IN SELECTED COUNTRIES (NON- OECD).
International Journal of Research and Reviews in Applied Sciences, 17, 111–0115.
Azarhoushang, B. (2013). The effects of FDI on China’s economic development; case of
Volkswagen in China. Retrieved from
https://opus4.kobv.de/opus4-hwr/frontdoor/index/index/docId/60
CCEW. (2016). Greening India’s Financial Market: How Green Bonds can drive Clean Energy
Deployment. New Delhi.
Centre, I. (2011). Where is China ’ s manufacturing industry going ? Deloitte China
manufacturing competitiveness study 2011, (November).
Chaker, M. N., & Inguva, S. (2012). The impact of money supply on economic growth: a case
study of the United Arab Emirates. Journal for Global Business Advancemen, 5.
Chakraborty, C., & Nunnenkamp, P. (2006). The Kiel Institute for the World Economy Economic
Reforms , Foreign Direct Investment and its Economic Effects in India (No. 1272). Kiel.
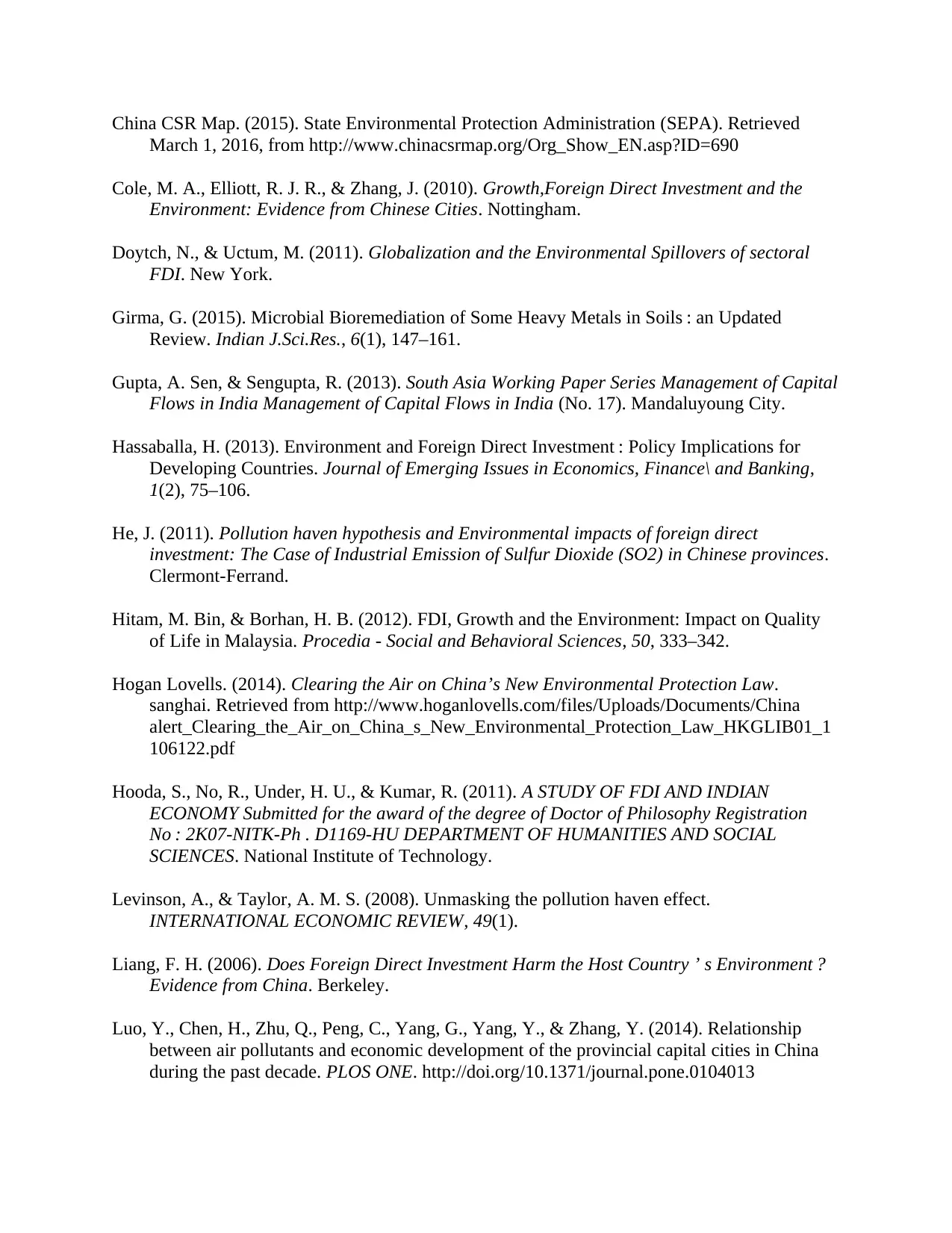
China CSR Map. (2015). State Environmental Protection Administration (SEPA). Retrieved
March 1, 2016, from http://www.chinacsrmap.org/Org_Show_EN.asp?ID=690
Cole, M. A., Elliott, R. J. R., & Zhang, J. (2010). Growth,Foreign Direct Investment and the
Environment: Evidence from Chinese Cities. Nottingham.
Doytch, N., & Uctum, M. (2011). Globalization and the Environmental Spillovers of sectoral
FDI. New York.
Girma, G. (2015). Microbial Bioremediation of Some Heavy Metals in Soils : an Updated
Review. Indian J.Sci.Res., 6(1), 147–161.
Gupta, A. Sen, & Sengupta, R. (2013). South Asia Working Paper Series Management of Capital
Flows in India Management of Capital Flows in India (No. 17). Mandaluyoung City.
Hassaballa, H. (2013). Environment and Foreign Direct Investment : Policy Implications for
Developing Countries. Journal of Emerging Issues in Economics, Finance\ and Banking,
1(2), 75–106.
He, J. (2011). Pollution haven hypothesis and Environmental impacts of foreign direct
investment: The Case of Industrial Emission of Sulfur Dioxide (SO2) in Chinese provinces.
Clermont-Ferrand.
Hitam, M. Bin, & Borhan, H. B. (2012). FDI, Growth and the Environment: Impact on Quality
of Life in Malaysia. Procedia - Social and Behavioral Sciences, 50, 333–342.
Hogan Lovells. (2014). Clearing the Air on China’s New Environmental Protection Law.
sanghai. Retrieved from http://www.hoganlovells.com/files/Uploads/Documents/China
alert_Clearing_the_Air_on_China_s_New_Environmental_Protection_Law_HKGLIB01_1
106122.pdf
Hooda, S., No, R., Under, H. U., & Kumar, R. (2011). A STUDY OF FDI AND INDIAN
ECONOMY Submitted for the award of the degree of Doctor of Philosophy Registration
No : 2K07-NITK-Ph . D1169-HU DEPARTMENT OF HUMANITIES AND SOCIAL
SCIENCES. National Institute of Technology.
Levinson, A., & Taylor, A. M. S. (2008). Unmasking the pollution haven effect.
INTERNATIONAL ECONOMIC REVIEW, 49(1).
Liang, F. H. (2006). Does Foreign Direct Investment Harm the Host Country ’ s Environment ?
Evidence from China. Berkeley.
Luo, Y., Chen, H., Zhu, Q., Peng, C., Yang, G., Yang, Y., & Zhang, Y. (2014). Relationship
between air pollutants and economic development of the provincial capital cities in China
during the past decade. PLOS ONE. http://doi.org/10.1371/journal.pone.0104013
March 1, 2016, from http://www.chinacsrmap.org/Org_Show_EN.asp?ID=690
Cole, M. A., Elliott, R. J. R., & Zhang, J. (2010). Growth,Foreign Direct Investment and the
Environment: Evidence from Chinese Cities. Nottingham.
Doytch, N., & Uctum, M. (2011). Globalization and the Environmental Spillovers of sectoral
FDI. New York.
Girma, G. (2015). Microbial Bioremediation of Some Heavy Metals in Soils : an Updated
Review. Indian J.Sci.Res., 6(1), 147–161.
Gupta, A. Sen, & Sengupta, R. (2013). South Asia Working Paper Series Management of Capital
Flows in India Management of Capital Flows in India (No. 17). Mandaluyoung City.
Hassaballa, H. (2013). Environment and Foreign Direct Investment : Policy Implications for
Developing Countries. Journal of Emerging Issues in Economics, Finance\ and Banking,
1(2), 75–106.
He, J. (2011). Pollution haven hypothesis and Environmental impacts of foreign direct
investment: The Case of Industrial Emission of Sulfur Dioxide (SO2) in Chinese provinces.
Clermont-Ferrand.
Hitam, M. Bin, & Borhan, H. B. (2012). FDI, Growth and the Environment: Impact on Quality
of Life in Malaysia. Procedia - Social and Behavioral Sciences, 50, 333–342.
Hogan Lovells. (2014). Clearing the Air on China’s New Environmental Protection Law.
sanghai. Retrieved from http://www.hoganlovells.com/files/Uploads/Documents/China
alert_Clearing_the_Air_on_China_s_New_Environmental_Protection_Law_HKGLIB01_1
106122.pdf
Hooda, S., No, R., Under, H. U., & Kumar, R. (2011). A STUDY OF FDI AND INDIAN
ECONOMY Submitted for the award of the degree of Doctor of Philosophy Registration
No : 2K07-NITK-Ph . D1169-HU DEPARTMENT OF HUMANITIES AND SOCIAL
SCIENCES. National Institute of Technology.
Levinson, A., & Taylor, A. M. S. (2008). Unmasking the pollution haven effect.
INTERNATIONAL ECONOMIC REVIEW, 49(1).
Liang, F. H. (2006). Does Foreign Direct Investment Harm the Host Country ’ s Environment ?
Evidence from China. Berkeley.
Luo, Y., Chen, H., Zhu, Q., Peng, C., Yang, G., Yang, Y., & Zhang, Y. (2014). Relationship
between air pollutants and economic development of the provincial capital cities in China
during the past decade. PLOS ONE. http://doi.org/10.1371/journal.pone.0104013
Secure Best Marks with AI Grader
Need help grading? Try our AI Grader for instant feedback on your assignments.
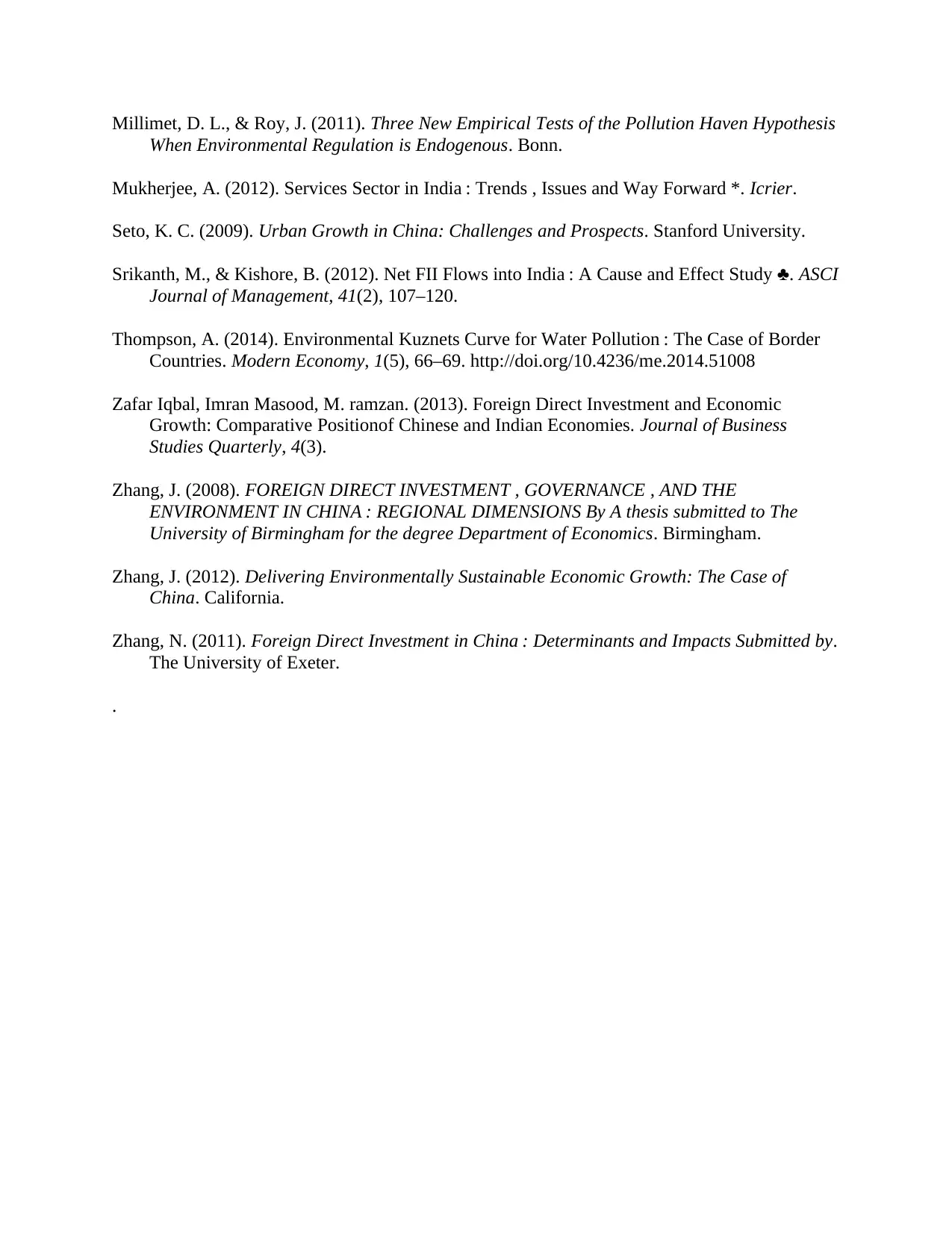
Millimet, D. L., & Roy, J. (2011). Three New Empirical Tests of the Pollution Haven Hypothesis
When Environmental Regulation is Endogenous. Bonn.
Mukherjee, A. (2012). Services Sector in India : Trends , Issues and Way Forward *. Icrier.
Seto, K. C. (2009). Urban Growth in China: Challenges and Prospects. Stanford University.
Srikanth, M., & Kishore, B. (2012). Net FII Flows into India : A Cause and Effect Study ♣. ASCI
Journal of Management, 41(2), 107–120.
Thompson, A. (2014). Environmental Kuznets Curve for Water Pollution : The Case of Border
Countries. Modern Economy, 1(5), 66–69. http://doi.org/10.4236/me.2014.51008
Zafar Iqbal, Imran Masood, M. ramzan. (2013). Foreign Direct Investment and Economic
Growth: Comparative Positionof Chinese and Indian Economies. Journal of Business
Studies Quarterly, 4(3).
Zhang, J. (2008). FOREIGN DIRECT INVESTMENT , GOVERNANCE , AND THE
ENVIRONMENT IN CHINA : REGIONAL DIMENSIONS By A thesis submitted to The
University of Birmingham for the degree Department of Economics. Birmingham.
Zhang, J. (2012). Delivering Environmentally Sustainable Economic Growth: The Case of
China. California.
Zhang, N. (2011). Foreign Direct Investment in China : Determinants and Impacts Submitted by.
The University of Exeter.
.
When Environmental Regulation is Endogenous. Bonn.
Mukherjee, A. (2012). Services Sector in India : Trends , Issues and Way Forward *. Icrier.
Seto, K. C. (2009). Urban Growth in China: Challenges and Prospects. Stanford University.
Srikanth, M., & Kishore, B. (2012). Net FII Flows into India : A Cause and Effect Study ♣. ASCI
Journal of Management, 41(2), 107–120.
Thompson, A. (2014). Environmental Kuznets Curve for Water Pollution : The Case of Border
Countries. Modern Economy, 1(5), 66–69. http://doi.org/10.4236/me.2014.51008
Zafar Iqbal, Imran Masood, M. ramzan. (2013). Foreign Direct Investment and Economic
Growth: Comparative Positionof Chinese and Indian Economies. Journal of Business
Studies Quarterly, 4(3).
Zhang, J. (2008). FOREIGN DIRECT INVESTMENT , GOVERNANCE , AND THE
ENVIRONMENT IN CHINA : REGIONAL DIMENSIONS By A thesis submitted to The
University of Birmingham for the degree Department of Economics. Birmingham.
Zhang, J. (2012). Delivering Environmentally Sustainable Economic Growth: The Case of
China. California.
Zhang, N. (2011). Foreign Direct Investment in China : Determinants and Impacts Submitted by.
The University of Exeter.
.
1 out of 29
Related Documents
![[object Object]](/_next/image/?url=%2F_next%2Fstatic%2Fmedia%2Flogo.6d15ce61.png&w=640&q=75)
Your All-in-One AI-Powered Toolkit for Academic Success.
+13062052269
info@desklib.com
Available 24*7 on WhatsApp / Email
Unlock your academic potential
© 2024 | Zucol Services PVT LTD | All rights reserved.