THE STATISTICAL ANALYSIS PROJECT
VerifiedAdded on 2022/08/23
|5
|881
|13
AI Summary
Contribute Materials
Your contribution can guide someone’s learning journey. Share your
documents today.
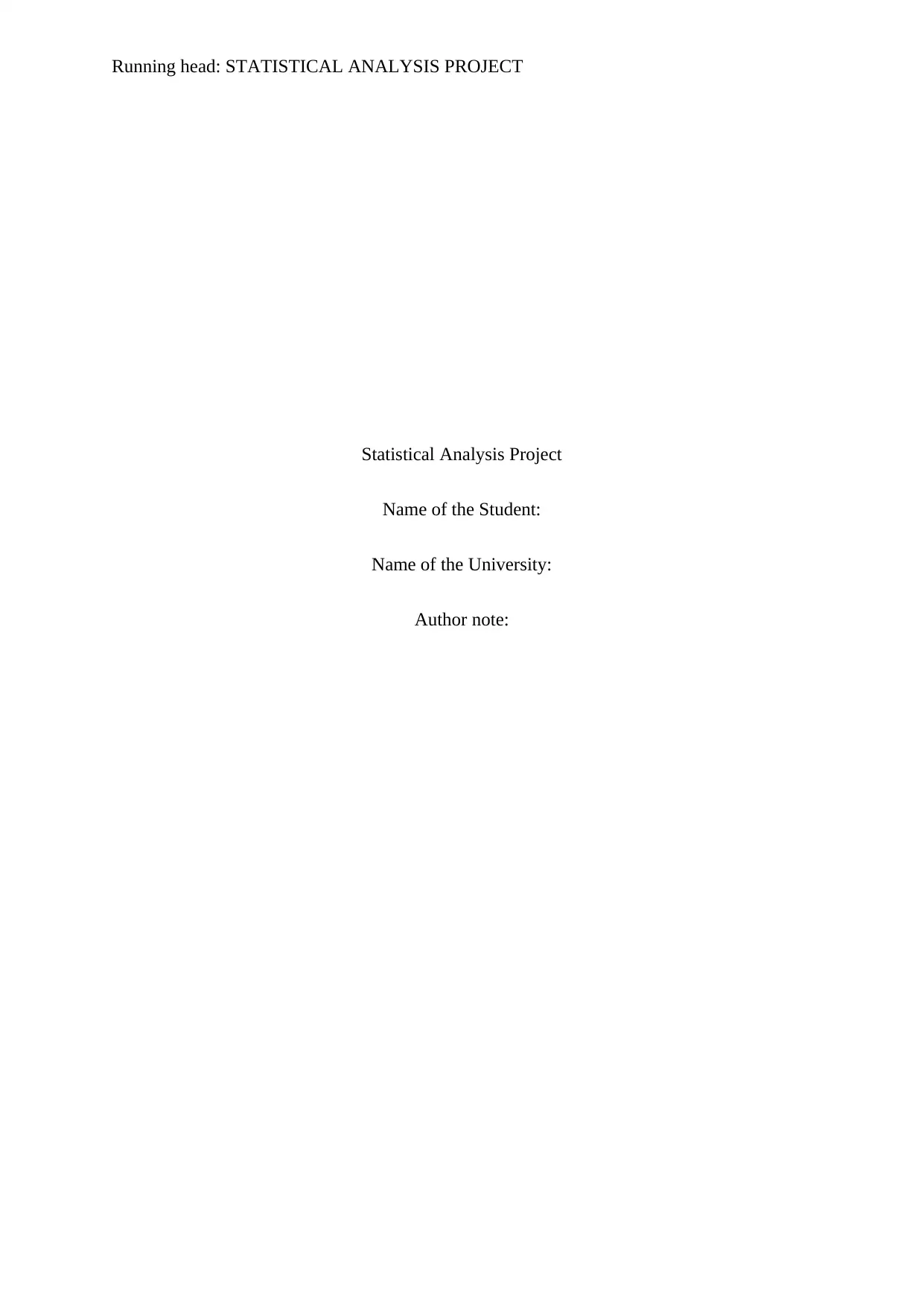
Running head: STATISTICAL ANALYSIS PROJECT
Statistical Analysis Project
Name of the Student:
Name of the University:
Author note:
Statistical Analysis Project
Name of the Student:
Name of the University:
Author note:
Secure Best Marks with AI Grader
Need help grading? Try our AI Grader for instant feedback on your assignments.
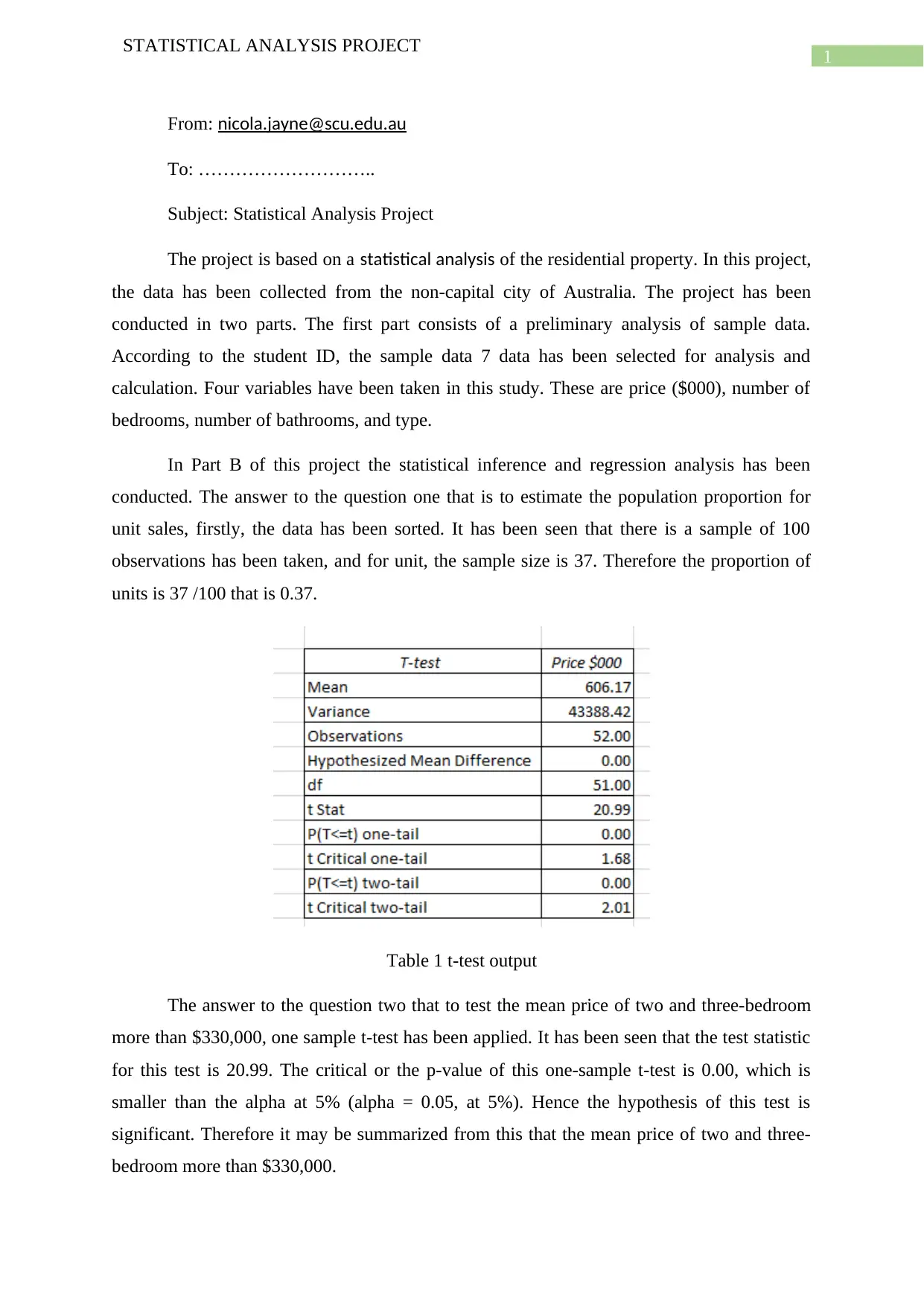
1
STATISTICAL ANALYSIS PROJECT
From: nicola.jayne@scu.edu.au
To: ………………………..
Subject: Statistical Analysis Project
The project is based on a statistical analysis of the residential property. In this project,
the data has been collected from the non-capital city of Australia. The project has been
conducted in two parts. The first part consists of a preliminary analysis of sample data.
According to the student ID, the sample data 7 data has been selected for analysis and
calculation. Four variables have been taken in this study. These are price ($000), number of
bedrooms, number of bathrooms, and type.
In Part B of this project the statistical inference and regression analysis has been
conducted. The answer to the question one that is to estimate the population proportion for
unit sales, firstly, the data has been sorted. It has been seen that there is a sample of 100
observations has been taken, and for unit, the sample size is 37. Therefore the proportion of
units is 37 /100 that is 0.37.
Table 1 t-test output
The answer to the question two that to test the mean price of two and three-bedroom
more than $330,000, one sample t-test has been applied. It has been seen that the test statistic
for this test is 20.99. The critical or the p-value of this one-sample t-test is 0.00, which is
smaller than the alpha at 5% (alpha = 0.05, at 5%). Hence the hypothesis of this test is
significant. Therefore it may be summarized from this that the mean price of two and three-
bedroom more than $330,000.
STATISTICAL ANALYSIS PROJECT
From: nicola.jayne@scu.edu.au
To: ………………………..
Subject: Statistical Analysis Project
The project is based on a statistical analysis of the residential property. In this project,
the data has been collected from the non-capital city of Australia. The project has been
conducted in two parts. The first part consists of a preliminary analysis of sample data.
According to the student ID, the sample data 7 data has been selected for analysis and
calculation. Four variables have been taken in this study. These are price ($000), number of
bedrooms, number of bathrooms, and type.
In Part B of this project the statistical inference and regression analysis has been
conducted. The answer to the question one that is to estimate the population proportion for
unit sales, firstly, the data has been sorted. It has been seen that there is a sample of 100
observations has been taken, and for unit, the sample size is 37. Therefore the proportion of
units is 37 /100 that is 0.37.
Table 1 t-test output
The answer to the question two that to test the mean price of two and three-bedroom
more than $330,000, one sample t-test has been applied. It has been seen that the test statistic
for this test is 20.99. The critical or the p-value of this one-sample t-test is 0.00, which is
smaller than the alpha at 5% (alpha = 0.05, at 5%). Hence the hypothesis of this test is
significant. Therefore it may be summarized from this that the mean price of two and three-
bedroom more than $330,000.
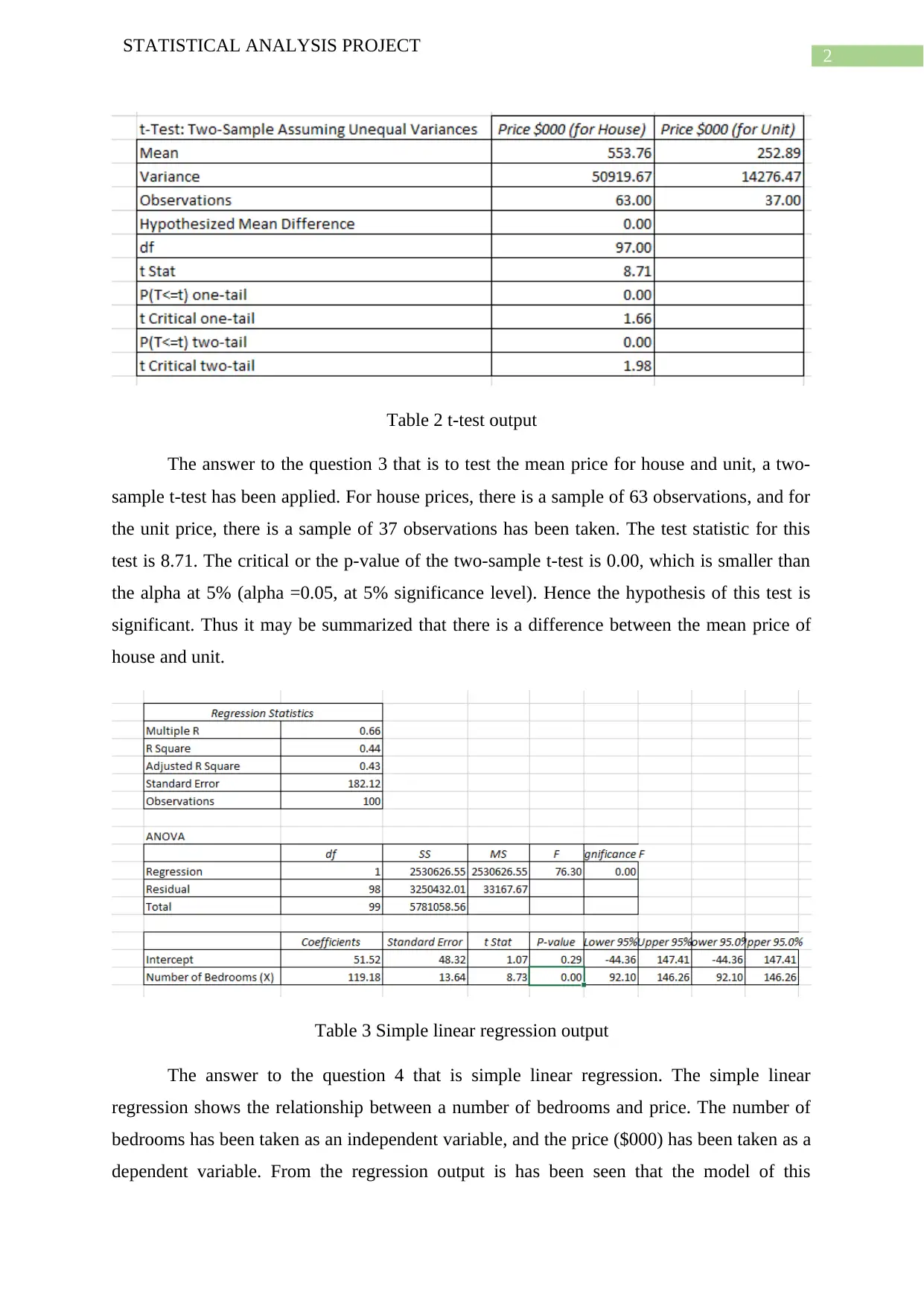
2
STATISTICAL ANALYSIS PROJECT
Table 2 t-test output
The answer to the question 3 that is to test the mean price for house and unit, a two-
sample t-test has been applied. For house prices, there is a sample of 63 observations, and for
the unit price, there is a sample of 37 observations has been taken. The test statistic for this
test is 8.71. The critical or the p-value of the two-sample t-test is 0.00, which is smaller than
the alpha at 5% (alpha =0.05, at 5% significance level). Hence the hypothesis of this test is
significant. Thus it may be summarized that there is a difference between the mean price of
house and unit.
Table 3 Simple linear regression output
The answer to the question 4 that is simple linear regression. The simple linear
regression shows the relationship between a number of bedrooms and price. The number of
bedrooms has been taken as an independent variable, and the price ($000) has been taken as a
dependent variable. From the regression output is has been seen that the model of this
STATISTICAL ANALYSIS PROJECT
Table 2 t-test output
The answer to the question 3 that is to test the mean price for house and unit, a two-
sample t-test has been applied. For house prices, there is a sample of 63 observations, and for
the unit price, there is a sample of 37 observations has been taken. The test statistic for this
test is 8.71. The critical or the p-value of the two-sample t-test is 0.00, which is smaller than
the alpha at 5% (alpha =0.05, at 5% significance level). Hence the hypothesis of this test is
significant. Thus it may be summarized that there is a difference between the mean price of
house and unit.
Table 3 Simple linear regression output
The answer to the question 4 that is simple linear regression. The simple linear
regression shows the relationship between a number of bedrooms and price. The number of
bedrooms has been taken as an independent variable, and the price ($000) has been taken as a
dependent variable. From the regression output is has been seen that the model of this
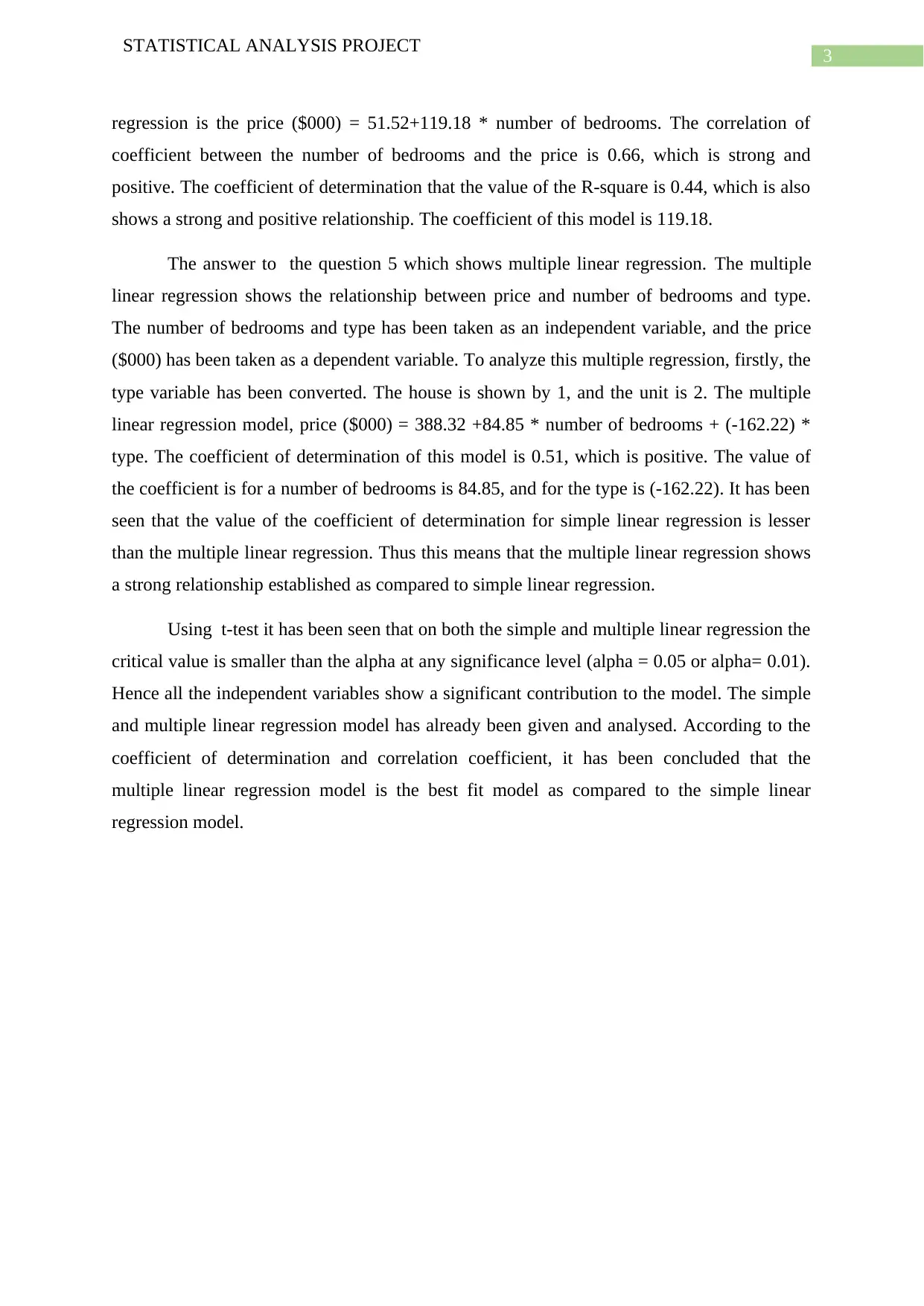
3
STATISTICAL ANALYSIS PROJECT
regression is the price ($000) = 51.52+119.18 * number of bedrooms. The correlation of
coefficient between the number of bedrooms and the price is 0.66, which is strong and
positive. The coefficient of determination that the value of the R-square is 0.44, which is also
shows a strong and positive relationship. The coefficient of this model is 119.18.
The answer to the question 5 which shows multiple linear regression. The multiple
linear regression shows the relationship between price and number of bedrooms and type.
The number of bedrooms and type has been taken as an independent variable, and the price
($000) has been taken as a dependent variable. To analyze this multiple regression, firstly, the
type variable has been converted. The house is shown by 1, and the unit is 2. The multiple
linear regression model, price ($000) = 388.32 +84.85 * number of bedrooms + (-162.22) *
type. The coefficient of determination of this model is 0.51, which is positive. The value of
the coefficient is for a number of bedrooms is 84.85, and for the type is (-162.22). It has been
seen that the value of the coefficient of determination for simple linear regression is lesser
than the multiple linear regression. Thus this means that the multiple linear regression shows
a strong relationship established as compared to simple linear regression.
Using t-test it has been seen that on both the simple and multiple linear regression the
critical value is smaller than the alpha at any significance level (alpha = 0.05 or alpha= 0.01).
Hence all the independent variables show a significant contribution to the model. The simple
and multiple linear regression model has already been given and analysed. According to the
coefficient of determination and correlation coefficient, it has been concluded that the
multiple linear regression model is the best fit model as compared to the simple linear
regression model.
STATISTICAL ANALYSIS PROJECT
regression is the price ($000) = 51.52+119.18 * number of bedrooms. The correlation of
coefficient between the number of bedrooms and the price is 0.66, which is strong and
positive. The coefficient of determination that the value of the R-square is 0.44, which is also
shows a strong and positive relationship. The coefficient of this model is 119.18.
The answer to the question 5 which shows multiple linear regression. The multiple
linear regression shows the relationship between price and number of bedrooms and type.
The number of bedrooms and type has been taken as an independent variable, and the price
($000) has been taken as a dependent variable. To analyze this multiple regression, firstly, the
type variable has been converted. The house is shown by 1, and the unit is 2. The multiple
linear regression model, price ($000) = 388.32 +84.85 * number of bedrooms + (-162.22) *
type. The coefficient of determination of this model is 0.51, which is positive. The value of
the coefficient is for a number of bedrooms is 84.85, and for the type is (-162.22). It has been
seen that the value of the coefficient of determination for simple linear regression is lesser
than the multiple linear regression. Thus this means that the multiple linear regression shows
a strong relationship established as compared to simple linear regression.
Using t-test it has been seen that on both the simple and multiple linear regression the
critical value is smaller than the alpha at any significance level (alpha = 0.05 or alpha= 0.01).
Hence all the independent variables show a significant contribution to the model. The simple
and multiple linear regression model has already been given and analysed. According to the
coefficient of determination and correlation coefficient, it has been concluded that the
multiple linear regression model is the best fit model as compared to the simple linear
regression model.
Secure Best Marks with AI Grader
Need help grading? Try our AI Grader for instant feedback on your assignments.

4
STATISTICAL ANALYSIS PROJECT
STATISTICAL ANALYSIS PROJECT
1 out of 5
Related Documents
![[object Object]](/_next/image/?url=%2F_next%2Fstatic%2Fmedia%2Flogo.6d15ce61.png&w=640&q=75)
Your All-in-One AI-Powered Toolkit for Academic Success.
+13062052269
info@desklib.com
Available 24*7 on WhatsApp / Email
Unlock your academic potential
© 2024 | Zucol Services PVT LTD | All rights reserved.