Analysis of Call Centre Operations using Statistical Techniques
VerifiedAdded on 2023/04/21
|11
|3165
|282
AI Summary
This report presents an analysis of the average call duration in a call centre using descriptive and inferential statistics. It includes measures of central tendency, dispersion, skewness, and kurtosis. The distribution of call duration closely resembles a normal distribution. The report also explores the relationship between call duration and the number of calls using regression analysis. Subject: Statistics
Contribute Materials
Your contribution can guide someone’s learning journey. Share your
documents today.
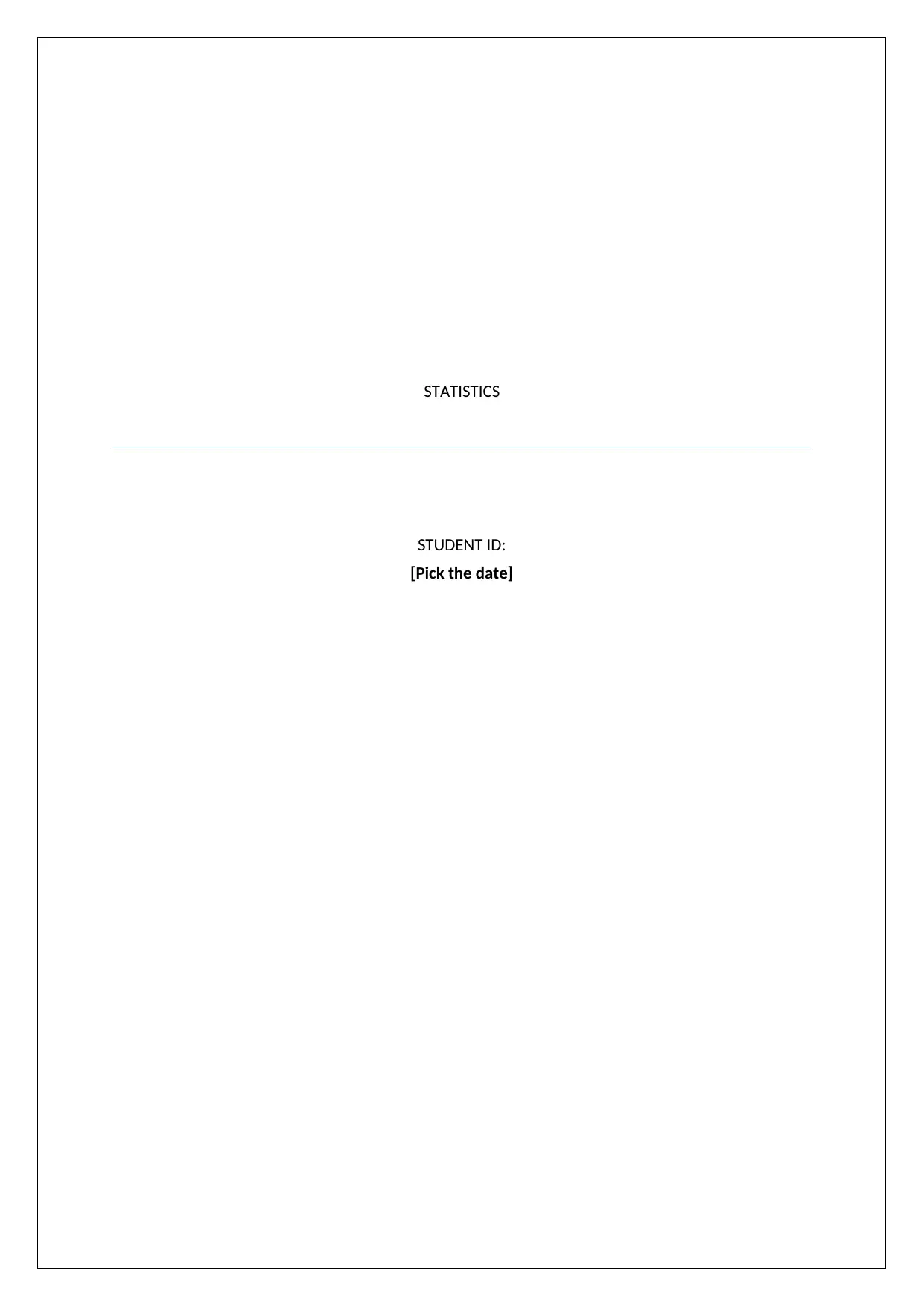
STATISTICS
STUDENT ID:
[Pick the date]
STUDENT ID:
[Pick the date]
Secure Best Marks with AI Grader
Need help grading? Try our AI Grader for instant feedback on your assignments.
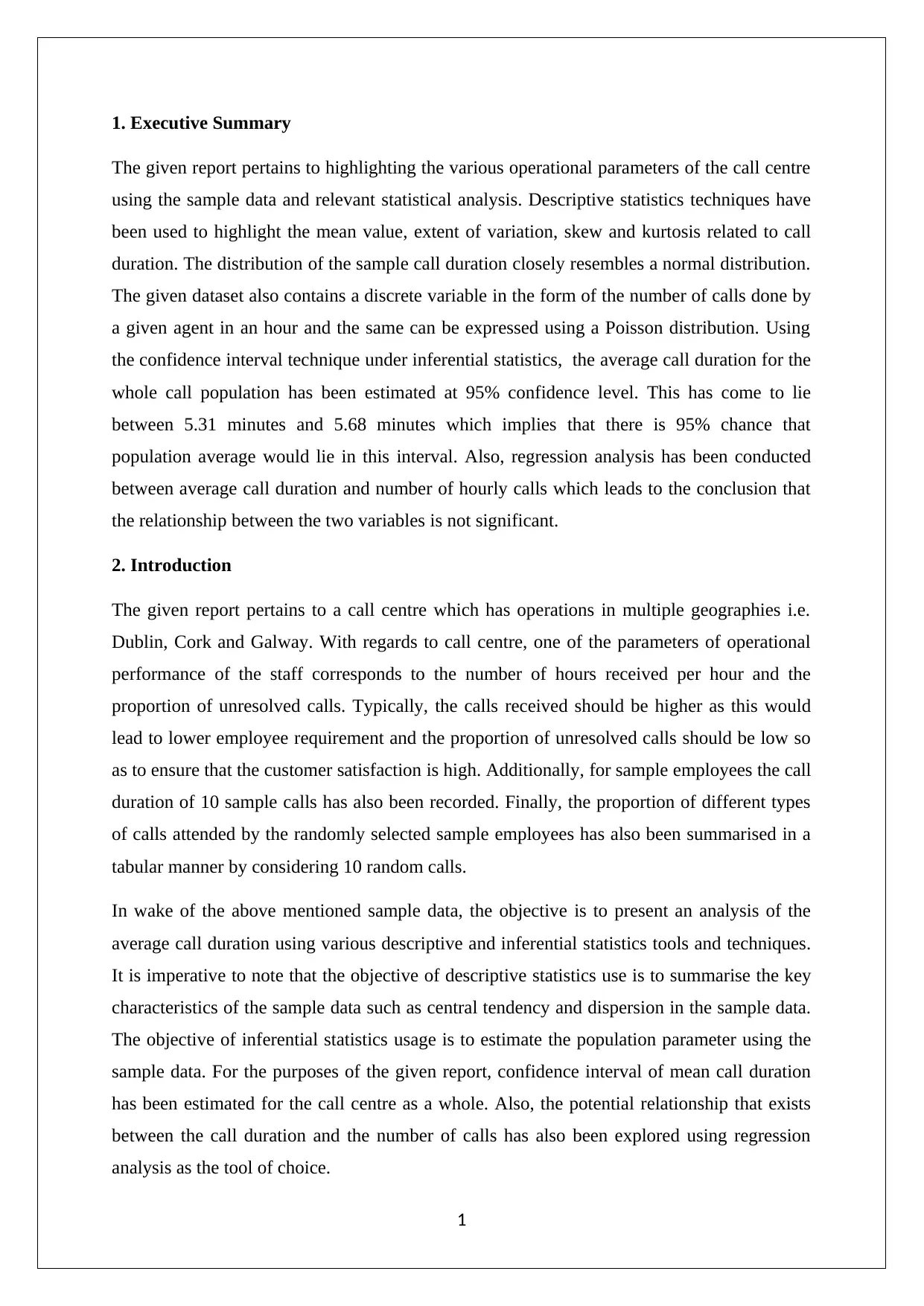
1. Executive Summary
The given report pertains to highlighting the various operational parameters of the call centre
using the sample data and relevant statistical analysis. Descriptive statistics techniques have
been used to highlight the mean value, extent of variation, skew and kurtosis related to call
duration. The distribution of the sample call duration closely resembles a normal distribution.
The given dataset also contains a discrete variable in the form of the number of calls done by
a given agent in an hour and the same can be expressed using a Poisson distribution. Using
the confidence interval technique under inferential statistics, the average call duration for the
whole call population has been estimated at 95% confidence level. This has come to lie
between 5.31 minutes and 5.68 minutes which implies that there is 95% chance that
population average would lie in this interval. Also, regression analysis has been conducted
between average call duration and number of hourly calls which leads to the conclusion that
the relationship between the two variables is not significant.
2. Introduction
The given report pertains to a call centre which has operations in multiple geographies i.e.
Dublin, Cork and Galway. With regards to call centre, one of the parameters of operational
performance of the staff corresponds to the number of hours received per hour and the
proportion of unresolved calls. Typically, the calls received should be higher as this would
lead to lower employee requirement and the proportion of unresolved calls should be low so
as to ensure that the customer satisfaction is high. Additionally, for sample employees the call
duration of 10 sample calls has also been recorded. Finally, the proportion of different types
of calls attended by the randomly selected sample employees has also been summarised in a
tabular manner by considering 10 random calls.
In wake of the above mentioned sample data, the objective is to present an analysis of the
average call duration using various descriptive and inferential statistics tools and techniques.
It is imperative to note that the objective of descriptive statistics use is to summarise the key
characteristics of the sample data such as central tendency and dispersion in the sample data.
The objective of inferential statistics usage is to estimate the population parameter using the
sample data. For the purposes of the given report, confidence interval of mean call duration
has been estimated for the call centre as a whole. Also, the potential relationship that exists
between the call duration and the number of calls has also been explored using regression
analysis as the tool of choice.
1
The given report pertains to highlighting the various operational parameters of the call centre
using the sample data and relevant statistical analysis. Descriptive statistics techniques have
been used to highlight the mean value, extent of variation, skew and kurtosis related to call
duration. The distribution of the sample call duration closely resembles a normal distribution.
The given dataset also contains a discrete variable in the form of the number of calls done by
a given agent in an hour and the same can be expressed using a Poisson distribution. Using
the confidence interval technique under inferential statistics, the average call duration for the
whole call population has been estimated at 95% confidence level. This has come to lie
between 5.31 minutes and 5.68 minutes which implies that there is 95% chance that
population average would lie in this interval. Also, regression analysis has been conducted
between average call duration and number of hourly calls which leads to the conclusion that
the relationship between the two variables is not significant.
2. Introduction
The given report pertains to a call centre which has operations in multiple geographies i.e.
Dublin, Cork and Galway. With regards to call centre, one of the parameters of operational
performance of the staff corresponds to the number of hours received per hour and the
proportion of unresolved calls. Typically, the calls received should be higher as this would
lead to lower employee requirement and the proportion of unresolved calls should be low so
as to ensure that the customer satisfaction is high. Additionally, for sample employees the call
duration of 10 sample calls has also been recorded. Finally, the proportion of different types
of calls attended by the randomly selected sample employees has also been summarised in a
tabular manner by considering 10 random calls.
In wake of the above mentioned sample data, the objective is to present an analysis of the
average call duration using various descriptive and inferential statistics tools and techniques.
It is imperative to note that the objective of descriptive statistics use is to summarise the key
characteristics of the sample data such as central tendency and dispersion in the sample data.
The objective of inferential statistics usage is to estimate the population parameter using the
sample data. For the purposes of the given report, confidence interval of mean call duration
has been estimated for the call centre as a whole. Also, the potential relationship that exists
between the call duration and the number of calls has also been explored using regression
analysis as the tool of choice.
1
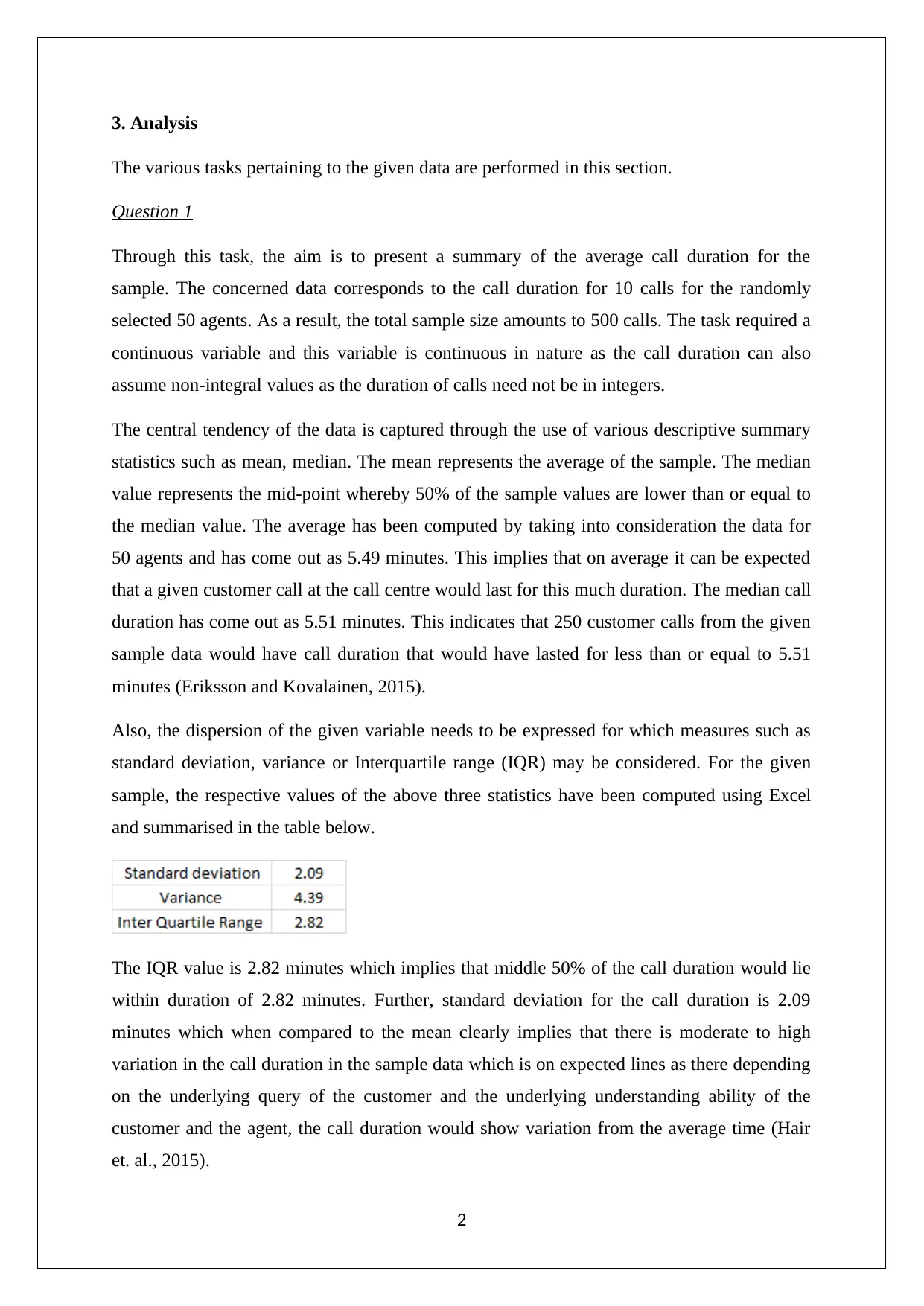
3. Analysis
The various tasks pertaining to the given data are performed in this section.
Question 1
Through this task, the aim is to present a summary of the average call duration for the
sample. The concerned data corresponds to the call duration for 10 calls for the randomly
selected 50 agents. As a result, the total sample size amounts to 500 calls. The task required a
continuous variable and this variable is continuous in nature as the call duration can also
assume non-integral values as the duration of calls need not be in integers.
The central tendency of the data is captured through the use of various descriptive summary
statistics such as mean, median. The mean represents the average of the sample. The median
value represents the mid-point whereby 50% of the sample values are lower than or equal to
the median value. The average has been computed by taking into consideration the data for
50 agents and has come out as 5.49 minutes. This implies that on average it can be expected
that a given customer call at the call centre would last for this much duration. The median call
duration has come out as 5.51 minutes. This indicates that 250 customer calls from the given
sample data would have call duration that would have lasted for less than or equal to 5.51
minutes (Eriksson and Kovalainen, 2015).
Also, the dispersion of the given variable needs to be expressed for which measures such as
standard deviation, variance or Interquartile range (IQR) may be considered. For the given
sample, the respective values of the above three statistics have been computed using Excel
and summarised in the table below.
The IQR value is 2.82 minutes which implies that middle 50% of the call duration would lie
within duration of 2.82 minutes. Further, standard deviation for the call duration is 2.09
minutes which when compared to the mean clearly implies that there is moderate to high
variation in the call duration in the sample data which is on expected lines as there depending
on the underlying query of the customer and the underlying understanding ability of the
customer and the agent, the call duration would show variation from the average time (Hair
et. al., 2015).
2
The various tasks pertaining to the given data are performed in this section.
Question 1
Through this task, the aim is to present a summary of the average call duration for the
sample. The concerned data corresponds to the call duration for 10 calls for the randomly
selected 50 agents. As a result, the total sample size amounts to 500 calls. The task required a
continuous variable and this variable is continuous in nature as the call duration can also
assume non-integral values as the duration of calls need not be in integers.
The central tendency of the data is captured through the use of various descriptive summary
statistics such as mean, median. The mean represents the average of the sample. The median
value represents the mid-point whereby 50% of the sample values are lower than or equal to
the median value. The average has been computed by taking into consideration the data for
50 agents and has come out as 5.49 minutes. This implies that on average it can be expected
that a given customer call at the call centre would last for this much duration. The median call
duration has come out as 5.51 minutes. This indicates that 250 customer calls from the given
sample data would have call duration that would have lasted for less than or equal to 5.51
minutes (Eriksson and Kovalainen, 2015).
Also, the dispersion of the given variable needs to be expressed for which measures such as
standard deviation, variance or Interquartile range (IQR) may be considered. For the given
sample, the respective values of the above three statistics have been computed using Excel
and summarised in the table below.
The IQR value is 2.82 minutes which implies that middle 50% of the call duration would lie
within duration of 2.82 minutes. Further, standard deviation for the call duration is 2.09
minutes which when compared to the mean clearly implies that there is moderate to high
variation in the call duration in the sample data which is on expected lines as there depending
on the underlying query of the customer and the underlying understanding ability of the
customer and the agent, the call duration would show variation from the average time (Hair
et. al., 2015).
2
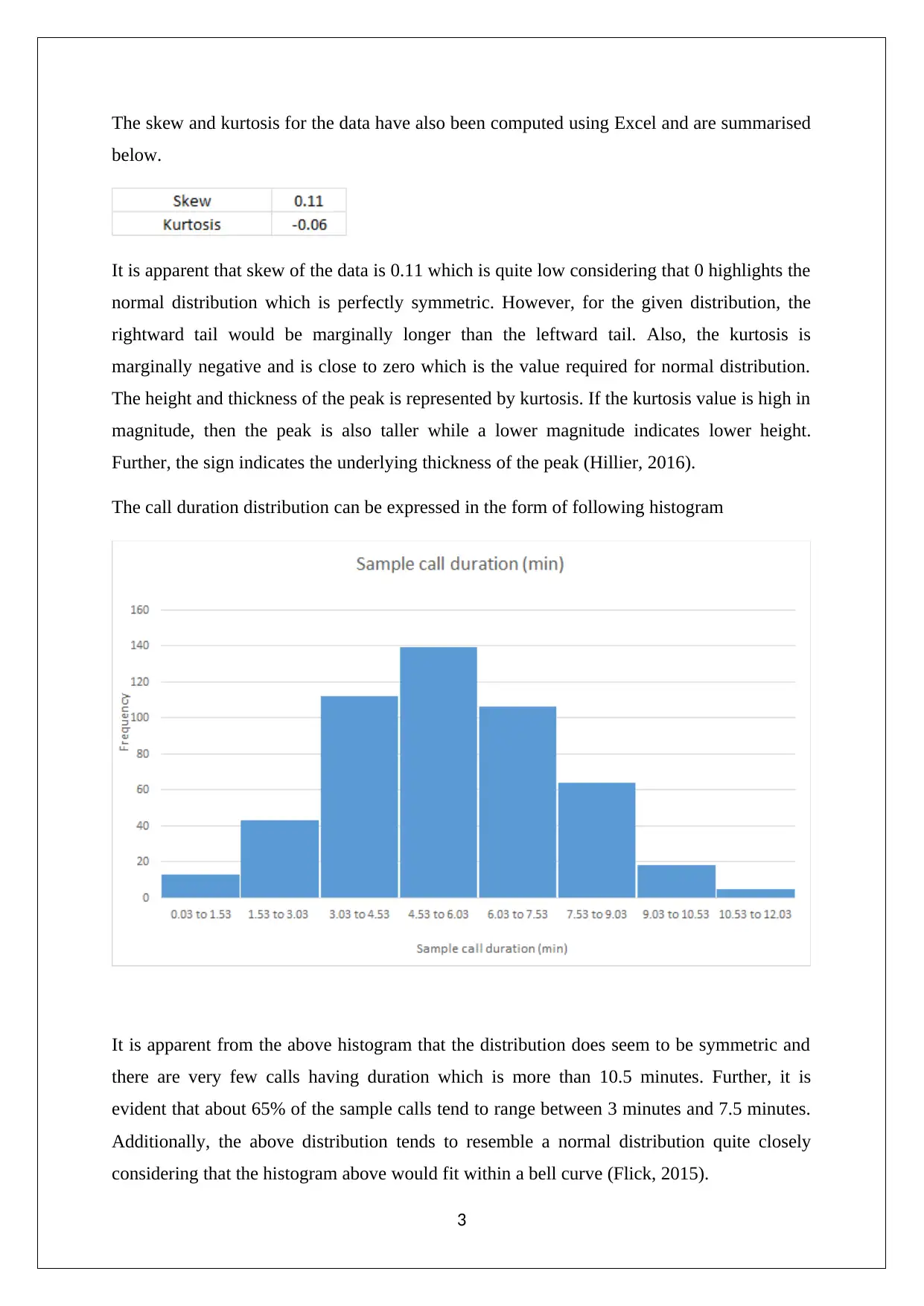
The skew and kurtosis for the data have also been computed using Excel and are summarised
below.
It is apparent that skew of the data is 0.11 which is quite low considering that 0 highlights the
normal distribution which is perfectly symmetric. However, for the given distribution, the
rightward tail would be marginally longer than the leftward tail. Also, the kurtosis is
marginally negative and is close to zero which is the value required for normal distribution.
The height and thickness of the peak is represented by kurtosis. If the kurtosis value is high in
magnitude, then the peak is also taller while a lower magnitude indicates lower height.
Further, the sign indicates the underlying thickness of the peak (Hillier, 2016).
The call duration distribution can be expressed in the form of following histogram
It is apparent from the above histogram that the distribution does seem to be symmetric and
there are very few calls having duration which is more than 10.5 minutes. Further, it is
evident that about 65% of the sample calls tend to range between 3 minutes and 7.5 minutes.
Additionally, the above distribution tends to resemble a normal distribution quite closely
considering that the histogram above would fit within a bell curve (Flick, 2015).
3
below.
It is apparent that skew of the data is 0.11 which is quite low considering that 0 highlights the
normal distribution which is perfectly symmetric. However, for the given distribution, the
rightward tail would be marginally longer than the leftward tail. Also, the kurtosis is
marginally negative and is close to zero which is the value required for normal distribution.
The height and thickness of the peak is represented by kurtosis. If the kurtosis value is high in
magnitude, then the peak is also taller while a lower magnitude indicates lower height.
Further, the sign indicates the underlying thickness of the peak (Hillier, 2016).
The call duration distribution can be expressed in the form of following histogram
It is apparent from the above histogram that the distribution does seem to be symmetric and
there are very few calls having duration which is more than 10.5 minutes. Further, it is
evident that about 65% of the sample calls tend to range between 3 minutes and 7.5 minutes.
Additionally, the above distribution tends to resemble a normal distribution quite closely
considering that the histogram above would fit within a bell curve (Flick, 2015).
3
Secure Best Marks with AI Grader
Need help grading? Try our AI Grader for instant feedback on your assignments.
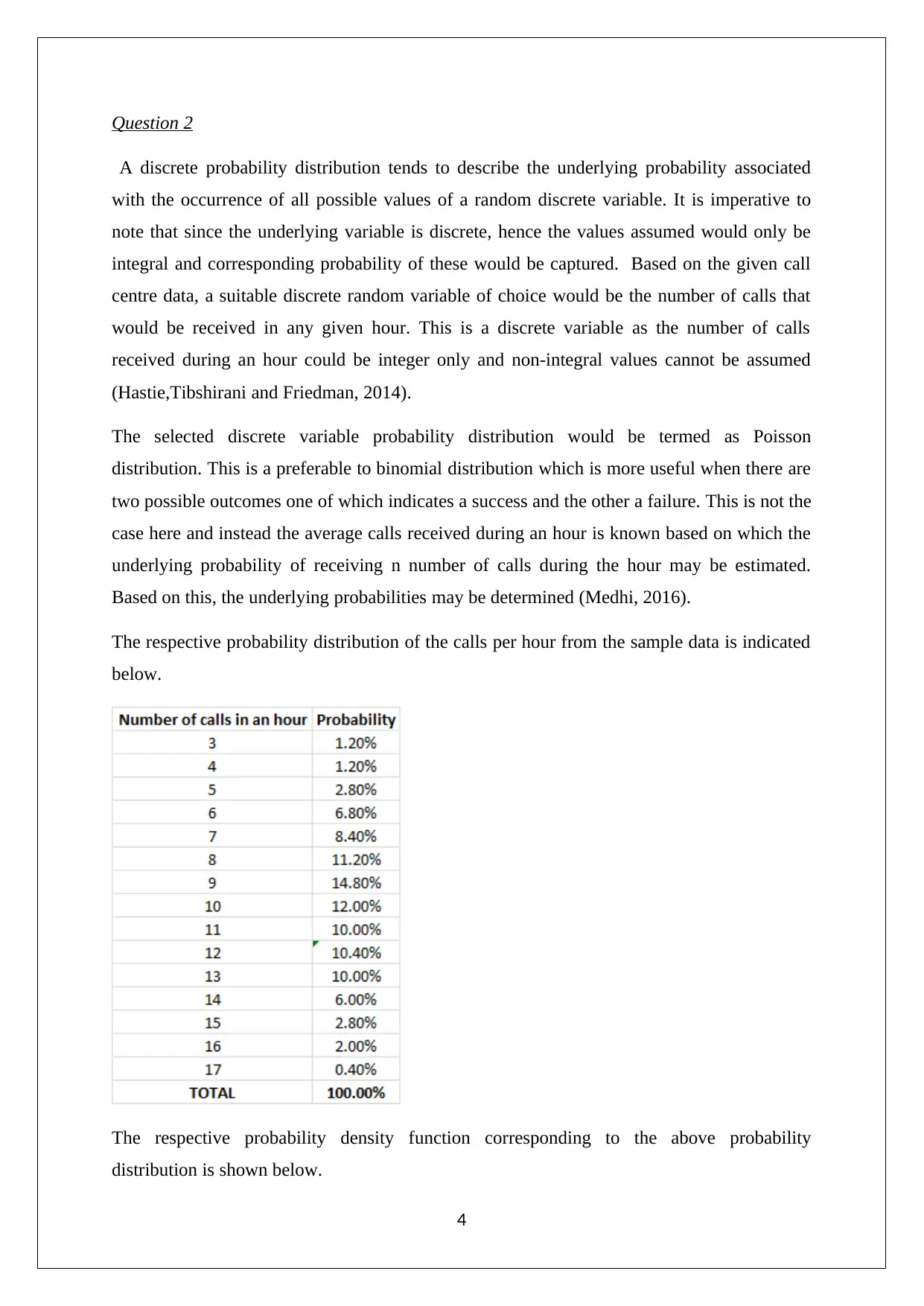
Question 2
A discrete probability distribution tends to describe the underlying probability associated
with the occurrence of all possible values of a random discrete variable. It is imperative to
note that since the underlying variable is discrete, hence the values assumed would only be
integral and corresponding probability of these would be captured. Based on the given call
centre data, a suitable discrete random variable of choice would be the number of calls that
would be received in any given hour. This is a discrete variable as the number of calls
received during an hour could be integer only and non-integral values cannot be assumed
(Hastie,Tibshirani and Friedman, 2014).
The selected discrete variable probability distribution would be termed as Poisson
distribution. This is a preferable to binomial distribution which is more useful when there are
two possible outcomes one of which indicates a success and the other a failure. This is not the
case here and instead the average calls received during an hour is known based on which the
underlying probability of receiving n number of calls during the hour may be estimated.
Based on this, the underlying probabilities may be determined (Medhi, 2016).
The respective probability distribution of the calls per hour from the sample data is indicated
below.
The respective probability density function corresponding to the above probability
distribution is shown below.
4
A discrete probability distribution tends to describe the underlying probability associated
with the occurrence of all possible values of a random discrete variable. It is imperative to
note that since the underlying variable is discrete, hence the values assumed would only be
integral and corresponding probability of these would be captured. Based on the given call
centre data, a suitable discrete random variable of choice would be the number of calls that
would be received in any given hour. This is a discrete variable as the number of calls
received during an hour could be integer only and non-integral values cannot be assumed
(Hastie,Tibshirani and Friedman, 2014).
The selected discrete variable probability distribution would be termed as Poisson
distribution. This is a preferable to binomial distribution which is more useful when there are
two possible outcomes one of which indicates a success and the other a failure. This is not the
case here and instead the average calls received during an hour is known based on which the
underlying probability of receiving n number of calls during the hour may be estimated.
Based on this, the underlying probabilities may be determined (Medhi, 2016).
The respective probability distribution of the calls per hour from the sample data is indicated
below.
The respective probability density function corresponding to the above probability
distribution is shown below.
4
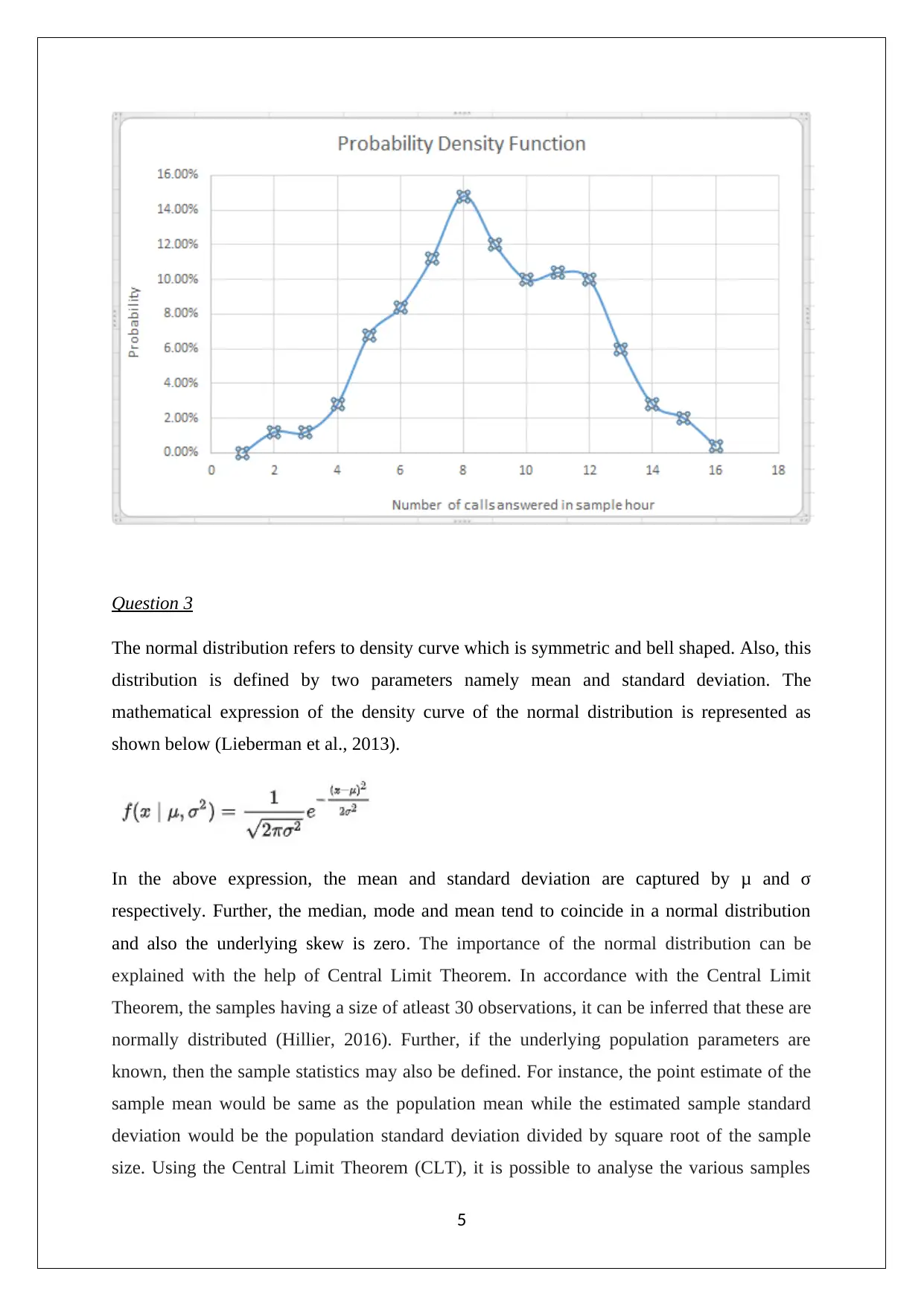
Question 3
The normal distribution refers to density curve which is symmetric and bell shaped. Also, this
distribution is defined by two parameters namely mean and standard deviation. The
mathematical expression of the density curve of the normal distribution is represented as
shown below (Lieberman et al., 2013).
In the above expression, the mean and standard deviation are captured by μ and σ
respectively. Further, the median, mode and mean tend to coincide in a normal distribution
and also the underlying skew is zero. The importance of the normal distribution can be
explained with the help of Central Limit Theorem. In accordance with the Central Limit
Theorem, the samples having a size of atleast 30 observations, it can be inferred that these are
normally distributed (Hillier, 2016). Further, if the underlying population parameters are
known, then the sample statistics may also be defined. For instance, the point estimate of the
sample mean would be same as the population mean while the estimated sample standard
deviation would be the population standard deviation divided by square root of the sample
size. Using the Central Limit Theorem (CLT), it is possible to analyse the various samples
5
The normal distribution refers to density curve which is symmetric and bell shaped. Also, this
distribution is defined by two parameters namely mean and standard deviation. The
mathematical expression of the density curve of the normal distribution is represented as
shown below (Lieberman et al., 2013).
In the above expression, the mean and standard deviation are captured by μ and σ
respectively. Further, the median, mode and mean tend to coincide in a normal distribution
and also the underlying skew is zero. The importance of the normal distribution can be
explained with the help of Central Limit Theorem. In accordance with the Central Limit
Theorem, the samples having a size of atleast 30 observations, it can be inferred that these are
normally distributed (Hillier, 2016). Further, if the underlying population parameters are
known, then the sample statistics may also be defined. For instance, the point estimate of the
sample mean would be same as the population mean while the estimated sample standard
deviation would be the population standard deviation divided by square root of the sample
size. Using the Central Limit Theorem (CLT), it is possible to analyse the various samples
5
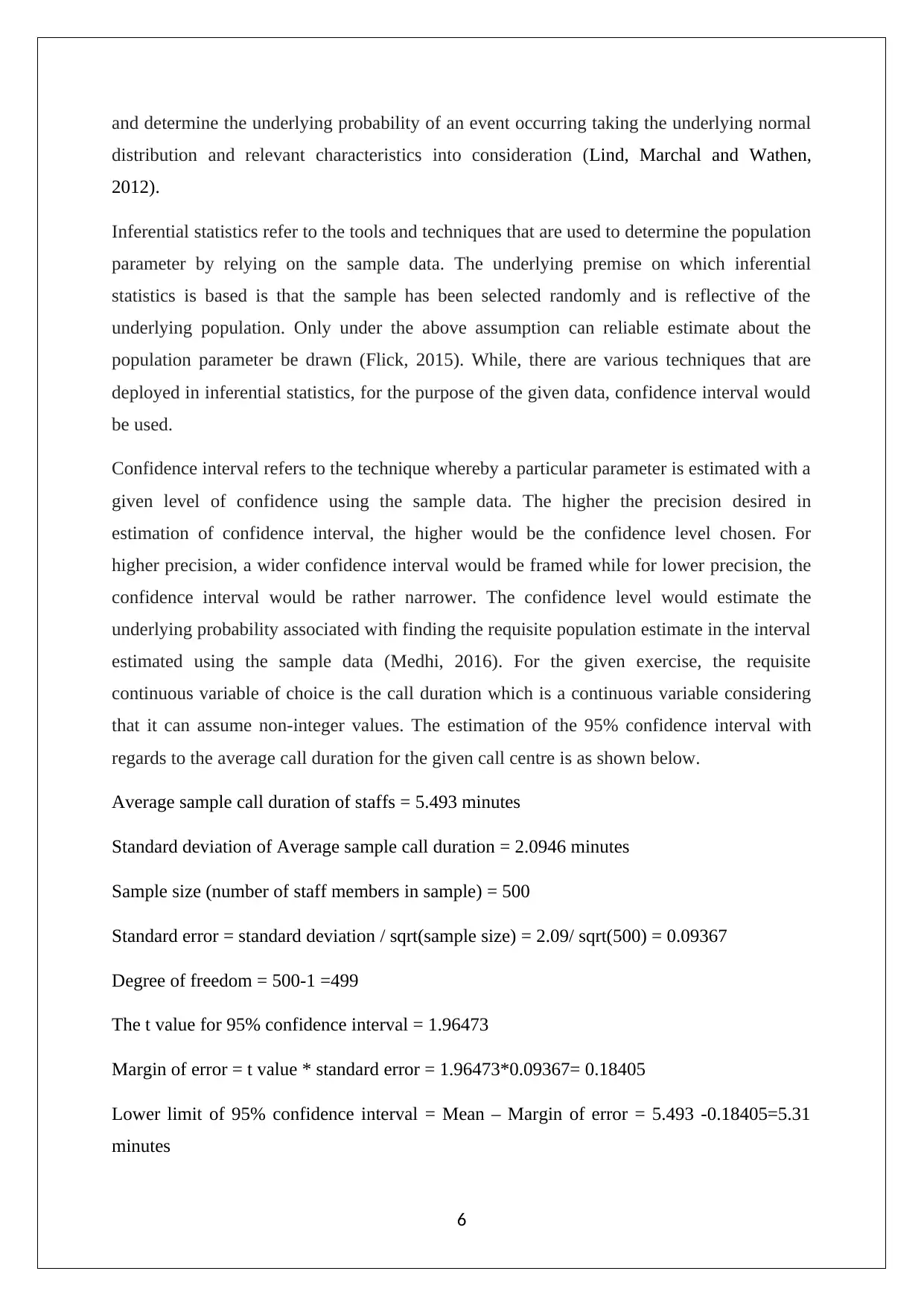
and determine the underlying probability of an event occurring taking the underlying normal
distribution and relevant characteristics into consideration (Lind, Marchal and Wathen,
2012).
Inferential statistics refer to the tools and techniques that are used to determine the population
parameter by relying on the sample data. The underlying premise on which inferential
statistics is based is that the sample has been selected randomly and is reflective of the
underlying population. Only under the above assumption can reliable estimate about the
population parameter be drawn (Flick, 2015). While, there are various techniques that are
deployed in inferential statistics, for the purpose of the given data, confidence interval would
be used.
Confidence interval refers to the technique whereby a particular parameter is estimated with a
given level of confidence using the sample data. The higher the precision desired in
estimation of confidence interval, the higher would be the confidence level chosen. For
higher precision, a wider confidence interval would be framed while for lower precision, the
confidence interval would be rather narrower. The confidence level would estimate the
underlying probability associated with finding the requisite population estimate in the interval
estimated using the sample data (Medhi, 2016). For the given exercise, the requisite
continuous variable of choice is the call duration which is a continuous variable considering
that it can assume non-integer values. The estimation of the 95% confidence interval with
regards to the average call duration for the given call centre is as shown below.
Average sample call duration of staffs = 5.493 minutes
Standard deviation of Average sample call duration = 2.0946 minutes
Sample size (number of staff members in sample) = 500
Standard error = standard deviation / sqrt(sample size) = 2.09/ sqrt(500) = 0.09367
Degree of freedom = 500-1 =499
The t value for 95% confidence interval = 1.96473
Margin of error = t value * standard error = 1.96473*0.09367= 0.18405
Lower limit of 95% confidence interval = Mean – Margin of error = 5.493 -0.18405=5.31
minutes
6
distribution and relevant characteristics into consideration (Lind, Marchal and Wathen,
2012).
Inferential statistics refer to the tools and techniques that are used to determine the population
parameter by relying on the sample data. The underlying premise on which inferential
statistics is based is that the sample has been selected randomly and is reflective of the
underlying population. Only under the above assumption can reliable estimate about the
population parameter be drawn (Flick, 2015). While, there are various techniques that are
deployed in inferential statistics, for the purpose of the given data, confidence interval would
be used.
Confidence interval refers to the technique whereby a particular parameter is estimated with a
given level of confidence using the sample data. The higher the precision desired in
estimation of confidence interval, the higher would be the confidence level chosen. For
higher precision, a wider confidence interval would be framed while for lower precision, the
confidence interval would be rather narrower. The confidence level would estimate the
underlying probability associated with finding the requisite population estimate in the interval
estimated using the sample data (Medhi, 2016). For the given exercise, the requisite
continuous variable of choice is the call duration which is a continuous variable considering
that it can assume non-integer values. The estimation of the 95% confidence interval with
regards to the average call duration for the given call centre is as shown below.
Average sample call duration of staffs = 5.493 minutes
Standard deviation of Average sample call duration = 2.0946 minutes
Sample size (number of staff members in sample) = 500
Standard error = standard deviation / sqrt(sample size) = 2.09/ sqrt(500) = 0.09367
Degree of freedom = 500-1 =499
The t value for 95% confidence interval = 1.96473
Margin of error = t value * standard error = 1.96473*0.09367= 0.18405
Lower limit of 95% confidence interval = Mean – Margin of error = 5.493 -0.18405=5.31
minutes
6
Paraphrase This Document
Need a fresh take? Get an instant paraphrase of this document with our AI Paraphraser
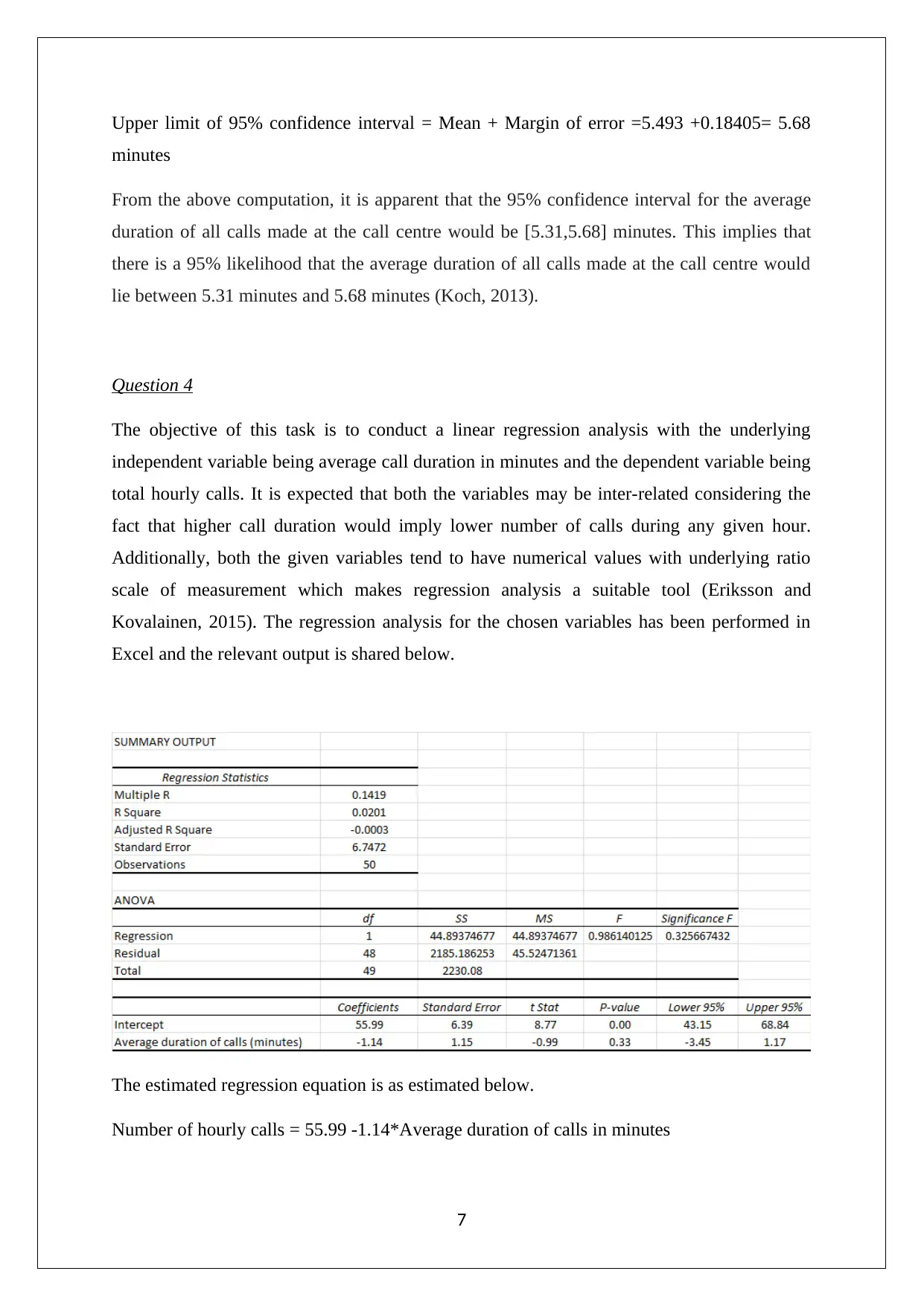
Upper limit of 95% confidence interval = Mean + Margin of error =5.493 +0.18405= 5.68
minutes
From the above computation, it is apparent that the 95% confidence interval for the average
duration of all calls made at the call centre would be [5.31,5.68] minutes. This implies that
there is a 95% likelihood that the average duration of all calls made at the call centre would
lie between 5.31 minutes and 5.68 minutes (Koch, 2013).
Question 4
The objective of this task is to conduct a linear regression analysis with the underlying
independent variable being average call duration in minutes and the dependent variable being
total hourly calls. It is expected that both the variables may be inter-related considering the
fact that higher call duration would imply lower number of calls during any given hour.
Additionally, both the given variables tend to have numerical values with underlying ratio
scale of measurement which makes regression analysis a suitable tool (Eriksson and
Kovalainen, 2015). The regression analysis for the chosen variables has been performed in
Excel and the relevant output is shared below.
The estimated regression equation is as estimated below.
Number of hourly calls = 55.99 -1.14*Average duration of calls in minutes
7
minutes
From the above computation, it is apparent that the 95% confidence interval for the average
duration of all calls made at the call centre would be [5.31,5.68] minutes. This implies that
there is a 95% likelihood that the average duration of all calls made at the call centre would
lie between 5.31 minutes and 5.68 minutes (Koch, 2013).
Question 4
The objective of this task is to conduct a linear regression analysis with the underlying
independent variable being average call duration in minutes and the dependent variable being
total hourly calls. It is expected that both the variables may be inter-related considering the
fact that higher call duration would imply lower number of calls during any given hour.
Additionally, both the given variables tend to have numerical values with underlying ratio
scale of measurement which makes regression analysis a suitable tool (Eriksson and
Kovalainen, 2015). The regression analysis for the chosen variables has been performed in
Excel and the relevant output is shared below.
The estimated regression equation is as estimated below.
Number of hourly calls = 55.99 -1.14*Average duration of calls in minutes
7
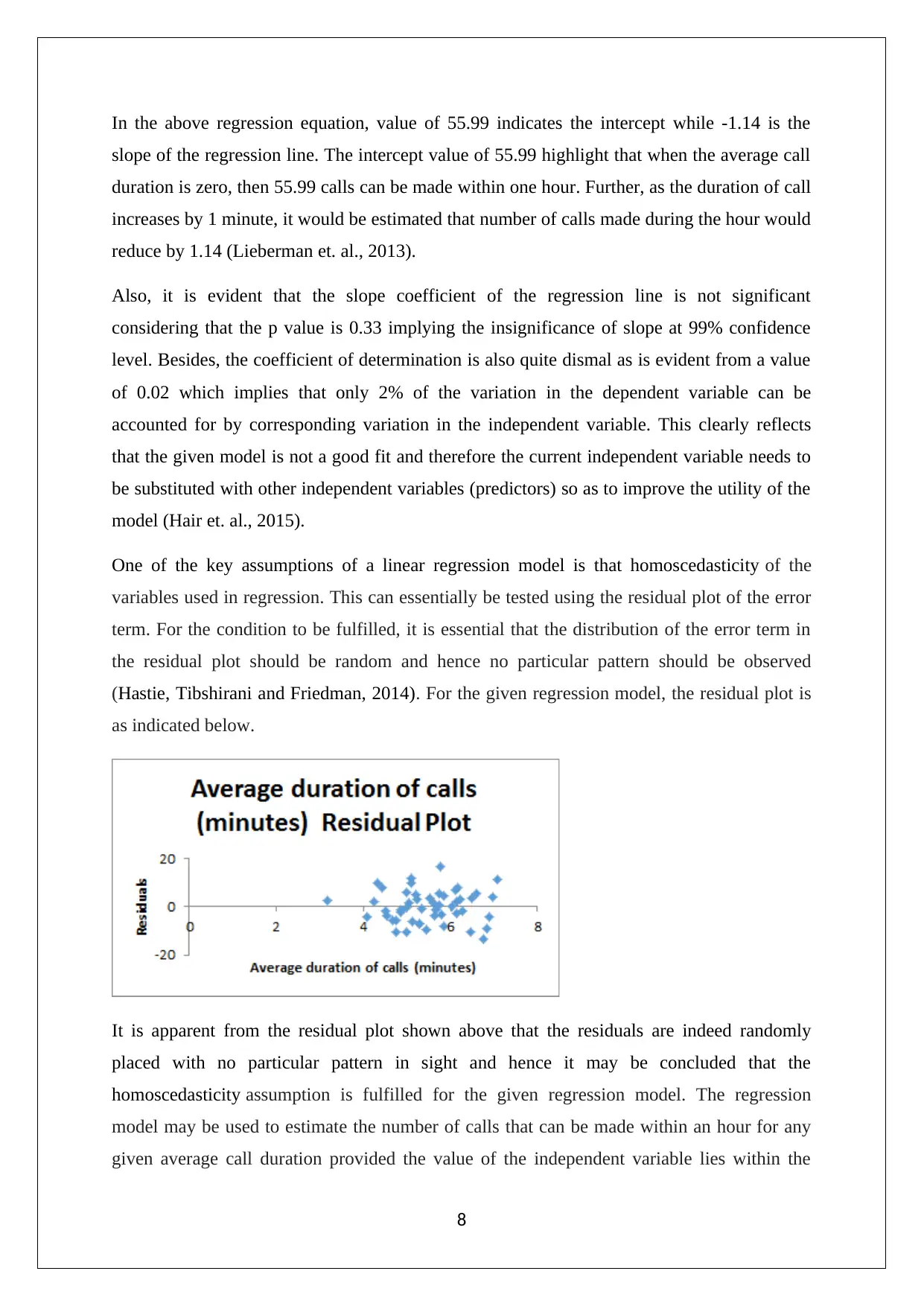
In the above regression equation, value of 55.99 indicates the intercept while -1.14 is the
slope of the regression line. The intercept value of 55.99 highlight that when the average call
duration is zero, then 55.99 calls can be made within one hour. Further, as the duration of call
increases by 1 minute, it would be estimated that number of calls made during the hour would
reduce by 1.14 (Lieberman et. al., 2013).
Also, it is evident that the slope coefficient of the regression line is not significant
considering that the p value is 0.33 implying the insignificance of slope at 99% confidence
level. Besides, the coefficient of determination is also quite dismal as is evident from a value
of 0.02 which implies that only 2% of the variation in the dependent variable can be
accounted for by corresponding variation in the independent variable. This clearly reflects
that the given model is not a good fit and therefore the current independent variable needs to
be substituted with other independent variables (predictors) so as to improve the utility of the
model (Hair et. al., 2015).
One of the key assumptions of a linear regression model is that homoscedasticity of the
variables used in regression. This can essentially be tested using the residual plot of the error
term. For the condition to be fulfilled, it is essential that the distribution of the error term in
the residual plot should be random and hence no particular pattern should be observed
(Hastie, Tibshirani and Friedman, 2014). For the given regression model, the residual plot is
as indicated below.
It is apparent from the residual plot shown above that the residuals are indeed randomly
placed with no particular pattern in sight and hence it may be concluded that the
homoscedasticity assumption is fulfilled for the given regression model. The regression
model may be used to estimate the number of calls that can be made within an hour for any
given average call duration provided the value of the independent variable lies within the
8
slope of the regression line. The intercept value of 55.99 highlight that when the average call
duration is zero, then 55.99 calls can be made within one hour. Further, as the duration of call
increases by 1 minute, it would be estimated that number of calls made during the hour would
reduce by 1.14 (Lieberman et. al., 2013).
Also, it is evident that the slope coefficient of the regression line is not significant
considering that the p value is 0.33 implying the insignificance of slope at 99% confidence
level. Besides, the coefficient of determination is also quite dismal as is evident from a value
of 0.02 which implies that only 2% of the variation in the dependent variable can be
accounted for by corresponding variation in the independent variable. This clearly reflects
that the given model is not a good fit and therefore the current independent variable needs to
be substituted with other independent variables (predictors) so as to improve the utility of the
model (Hair et. al., 2015).
One of the key assumptions of a linear regression model is that homoscedasticity of the
variables used in regression. This can essentially be tested using the residual plot of the error
term. For the condition to be fulfilled, it is essential that the distribution of the error term in
the residual plot should be random and hence no particular pattern should be observed
(Hastie, Tibshirani and Friedman, 2014). For the given regression model, the residual plot is
as indicated below.
It is apparent from the residual plot shown above that the residuals are indeed randomly
placed with no particular pattern in sight and hence it may be concluded that the
homoscedasticity assumption is fulfilled for the given regression model. The regression
model may be used to estimate the number of calls that can be made within an hour for any
given average call duration provided the value of the independent variable lies within the
8
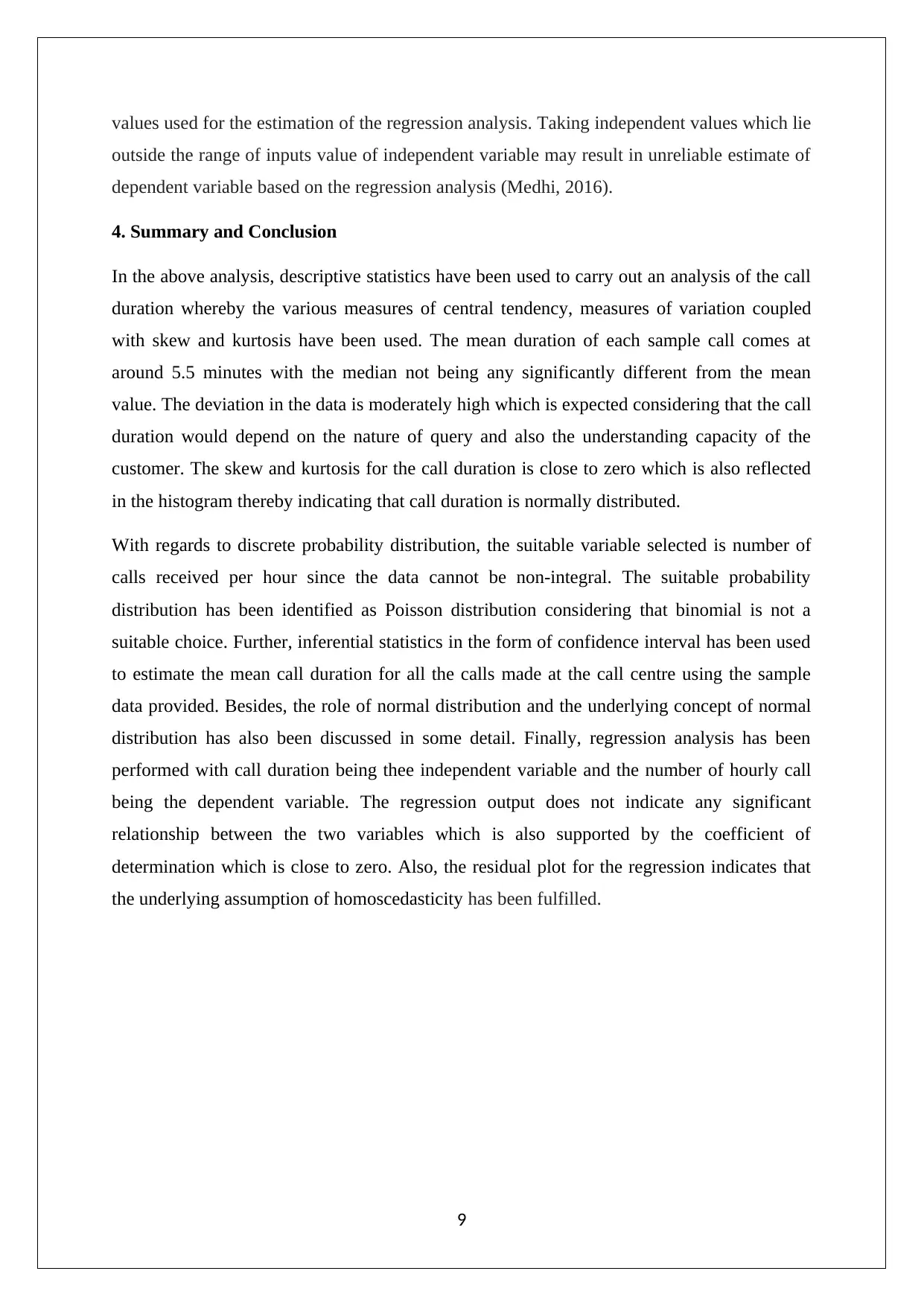
values used for the estimation of the regression analysis. Taking independent values which lie
outside the range of inputs value of independent variable may result in unreliable estimate of
dependent variable based on the regression analysis (Medhi, 2016).
4. Summary and Conclusion
In the above analysis, descriptive statistics have been used to carry out an analysis of the call
duration whereby the various measures of central tendency, measures of variation coupled
with skew and kurtosis have been used. The mean duration of each sample call comes at
around 5.5 minutes with the median not being any significantly different from the mean
value. The deviation in the data is moderately high which is expected considering that the call
duration would depend on the nature of query and also the understanding capacity of the
customer. The skew and kurtosis for the call duration is close to zero which is also reflected
in the histogram thereby indicating that call duration is normally distributed.
With regards to discrete probability distribution, the suitable variable selected is number of
calls received per hour since the data cannot be non-integral. The suitable probability
distribution has been identified as Poisson distribution considering that binomial is not a
suitable choice. Further, inferential statistics in the form of confidence interval has been used
to estimate the mean call duration for all the calls made at the call centre using the sample
data provided. Besides, the role of normal distribution and the underlying concept of normal
distribution has also been discussed in some detail. Finally, regression analysis has been
performed with call duration being thee independent variable and the number of hourly call
being the dependent variable. The regression output does not indicate any significant
relationship between the two variables which is also supported by the coefficient of
determination which is close to zero. Also, the residual plot for the regression indicates that
the underlying assumption of homoscedasticity has been fulfilled.
9
outside the range of inputs value of independent variable may result in unreliable estimate of
dependent variable based on the regression analysis (Medhi, 2016).
4. Summary and Conclusion
In the above analysis, descriptive statistics have been used to carry out an analysis of the call
duration whereby the various measures of central tendency, measures of variation coupled
with skew and kurtosis have been used. The mean duration of each sample call comes at
around 5.5 minutes with the median not being any significantly different from the mean
value. The deviation in the data is moderately high which is expected considering that the call
duration would depend on the nature of query and also the understanding capacity of the
customer. The skew and kurtosis for the call duration is close to zero which is also reflected
in the histogram thereby indicating that call duration is normally distributed.
With regards to discrete probability distribution, the suitable variable selected is number of
calls received per hour since the data cannot be non-integral. The suitable probability
distribution has been identified as Poisson distribution considering that binomial is not a
suitable choice. Further, inferential statistics in the form of confidence interval has been used
to estimate the mean call duration for all the calls made at the call centre using the sample
data provided. Besides, the role of normal distribution and the underlying concept of normal
distribution has also been discussed in some detail. Finally, regression analysis has been
performed with call duration being thee independent variable and the number of hourly call
being the dependent variable. The regression output does not indicate any significant
relationship between the two variables which is also supported by the coefficient of
determination which is close to zero. Also, the residual plot for the regression indicates that
the underlying assumption of homoscedasticity has been fulfilled.
9
Secure Best Marks with AI Grader
Need help grading? Try our AI Grader for instant feedback on your assignments.
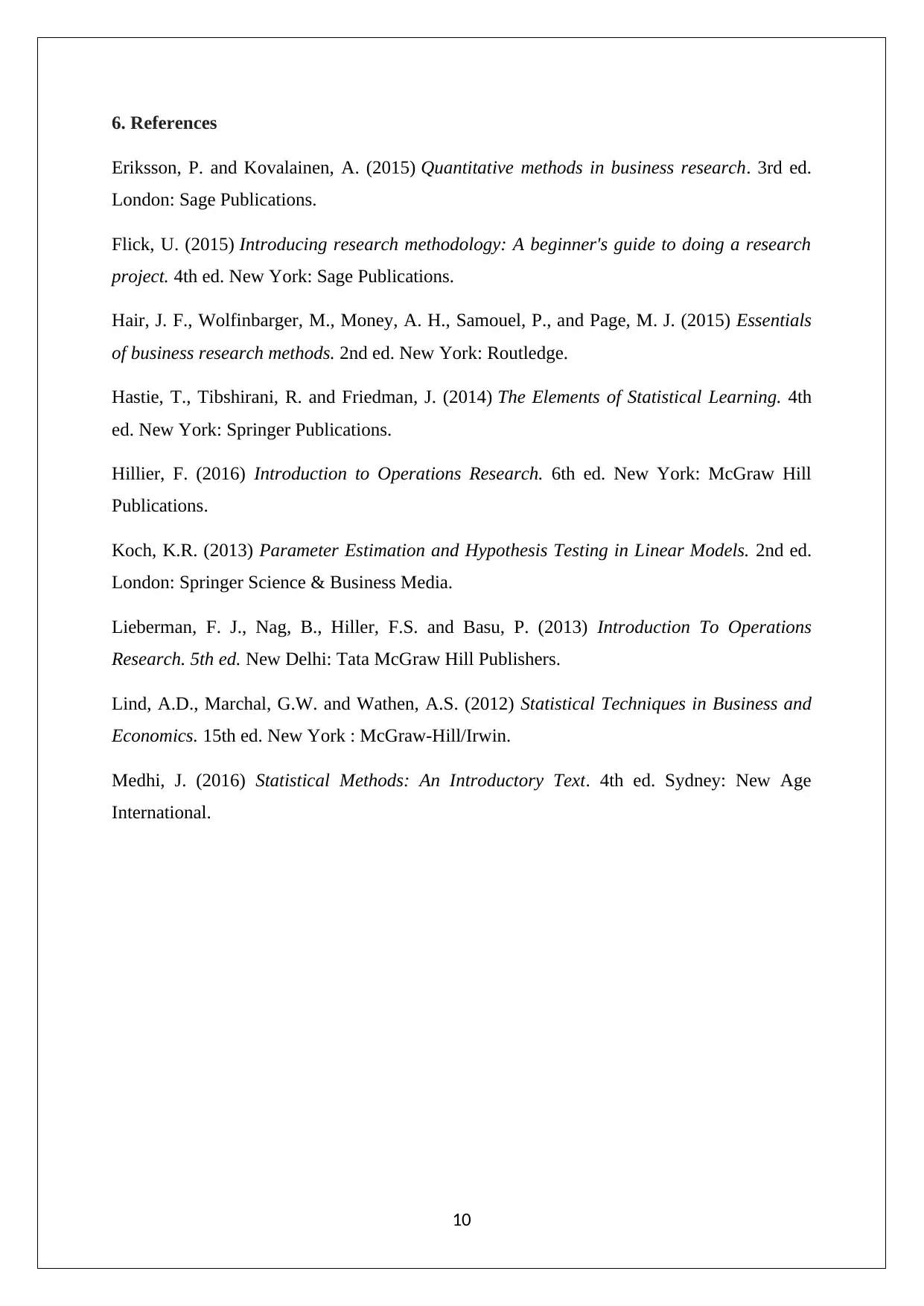
6. References
Eriksson, P. and Kovalainen, A. (2015) Quantitative methods in business research. 3rd ed.
London: Sage Publications.
Flick, U. (2015) Introducing research methodology: A beginner's guide to doing a research
project. 4th ed. New York: Sage Publications.
Hair, J. F., Wolfinbarger, M., Money, A. H., Samouel, P., and Page, M. J. (2015) Essentials
of business research methods. 2nd ed. New York: Routledge.
Hastie, T., Tibshirani, R. and Friedman, J. (2014) The Elements of Statistical Learning. 4th
ed. New York: Springer Publications.
Hillier, F. (2016) Introduction to Operations Research. 6th ed. New York: McGraw Hill
Publications.
Koch, K.R. (2013) Parameter Estimation and Hypothesis Testing in Linear Models. 2nd ed.
London: Springer Science & Business Media.
Lieberman, F. J., Nag, B., Hiller, F.S. and Basu, P. (2013) Introduction To Operations
Research. 5th ed. New Delhi: Tata McGraw Hill Publishers.
Lind, A.D., Marchal, G.W. and Wathen, A.S. (2012) Statistical Techniques in Business and
Economics. 15th ed. New York : McGraw-Hill/Irwin.
Medhi, J. (2016) Statistical Methods: An Introductory Text. 4th ed. Sydney: New Age
International.
10
Eriksson, P. and Kovalainen, A. (2015) Quantitative methods in business research. 3rd ed.
London: Sage Publications.
Flick, U. (2015) Introducing research methodology: A beginner's guide to doing a research
project. 4th ed. New York: Sage Publications.
Hair, J. F., Wolfinbarger, M., Money, A. H., Samouel, P., and Page, M. J. (2015) Essentials
of business research methods. 2nd ed. New York: Routledge.
Hastie, T., Tibshirani, R. and Friedman, J. (2014) The Elements of Statistical Learning. 4th
ed. New York: Springer Publications.
Hillier, F. (2016) Introduction to Operations Research. 6th ed. New York: McGraw Hill
Publications.
Koch, K.R. (2013) Parameter Estimation and Hypothesis Testing in Linear Models. 2nd ed.
London: Springer Science & Business Media.
Lieberman, F. J., Nag, B., Hiller, F.S. and Basu, P. (2013) Introduction To Operations
Research. 5th ed. New Delhi: Tata McGraw Hill Publishers.
Lind, A.D., Marchal, G.W. and Wathen, A.S. (2012) Statistical Techniques in Business and
Economics. 15th ed. New York : McGraw-Hill/Irwin.
Medhi, J. (2016) Statistical Methods: An Introductory Text. 4th ed. Sydney: New Age
International.
10
1 out of 11
Related Documents
![[object Object]](/_next/image/?url=%2F_next%2Fstatic%2Fmedia%2Flogo.6d15ce61.png&w=640&q=75)
Your All-in-One AI-Powered Toolkit for Academic Success.
+13062052269
info@desklib.com
Available 24*7 on WhatsApp / Email
Unlock your academic potential
© 2024 | Zucol Services PVT LTD | All rights reserved.