Machine Learning for Customer Retention in Banking
VerifiedAdded on 2023/04/07
|20
|5486
|199
AI Summary
This paper explores the role of consumer behavioral factors in influencing customer retention in the banking industry. It discusses the application of machine learning techniques to minimize customer churn and improve customer satisfaction. The study uses a descriptive research design and a questionnaire to gather data on customer retention in the banking industry. The findings highlight the importance of customer satisfaction and loyalty in long-term customer relationships.
Contribute Materials
Your contribution can guide someone’s learning journey. Share your
documents today.
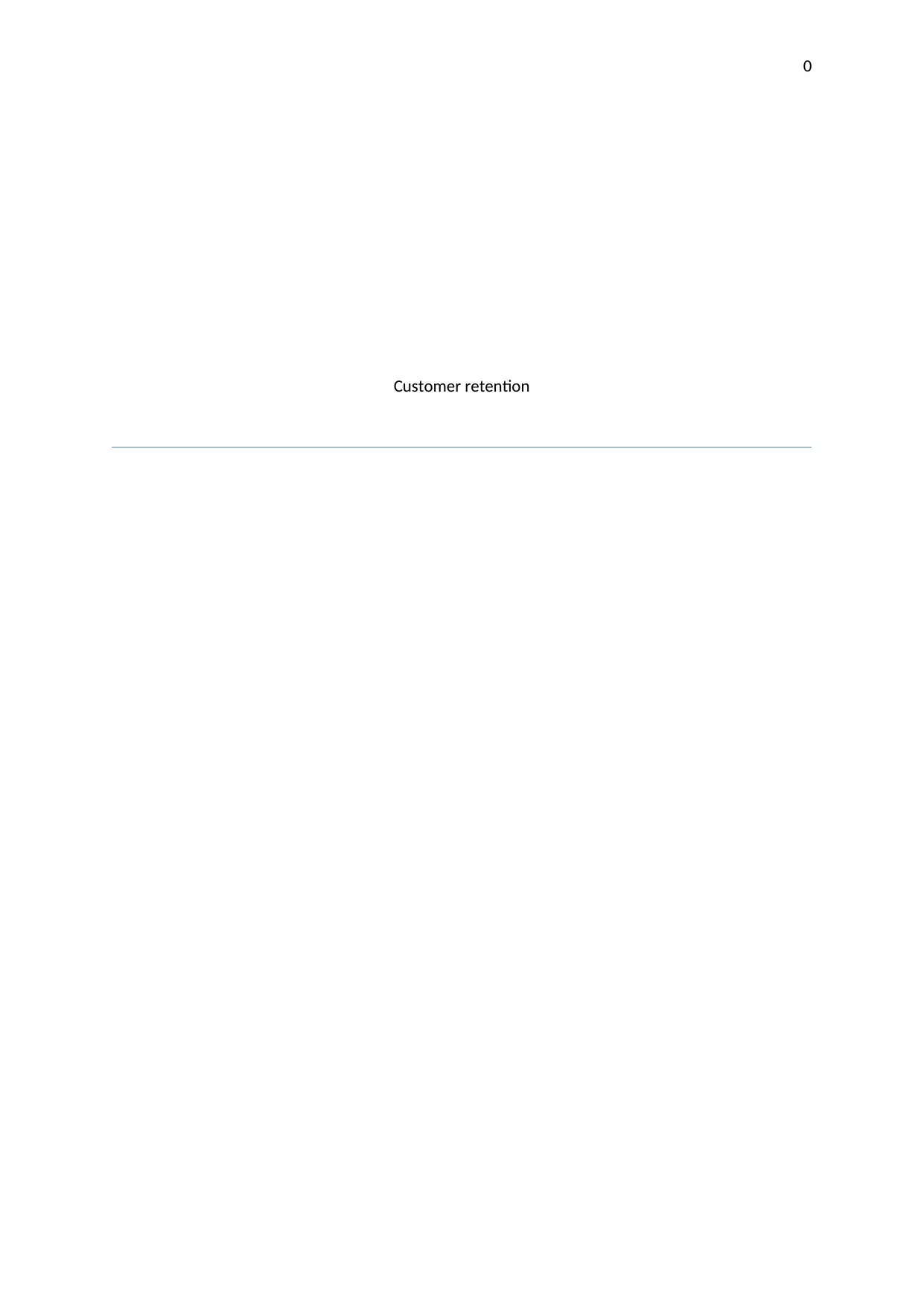
0
Customer retention
Customer retention
Secure Best Marks with AI Grader
Need help grading? Try our AI Grader for instant feedback on your assignments.
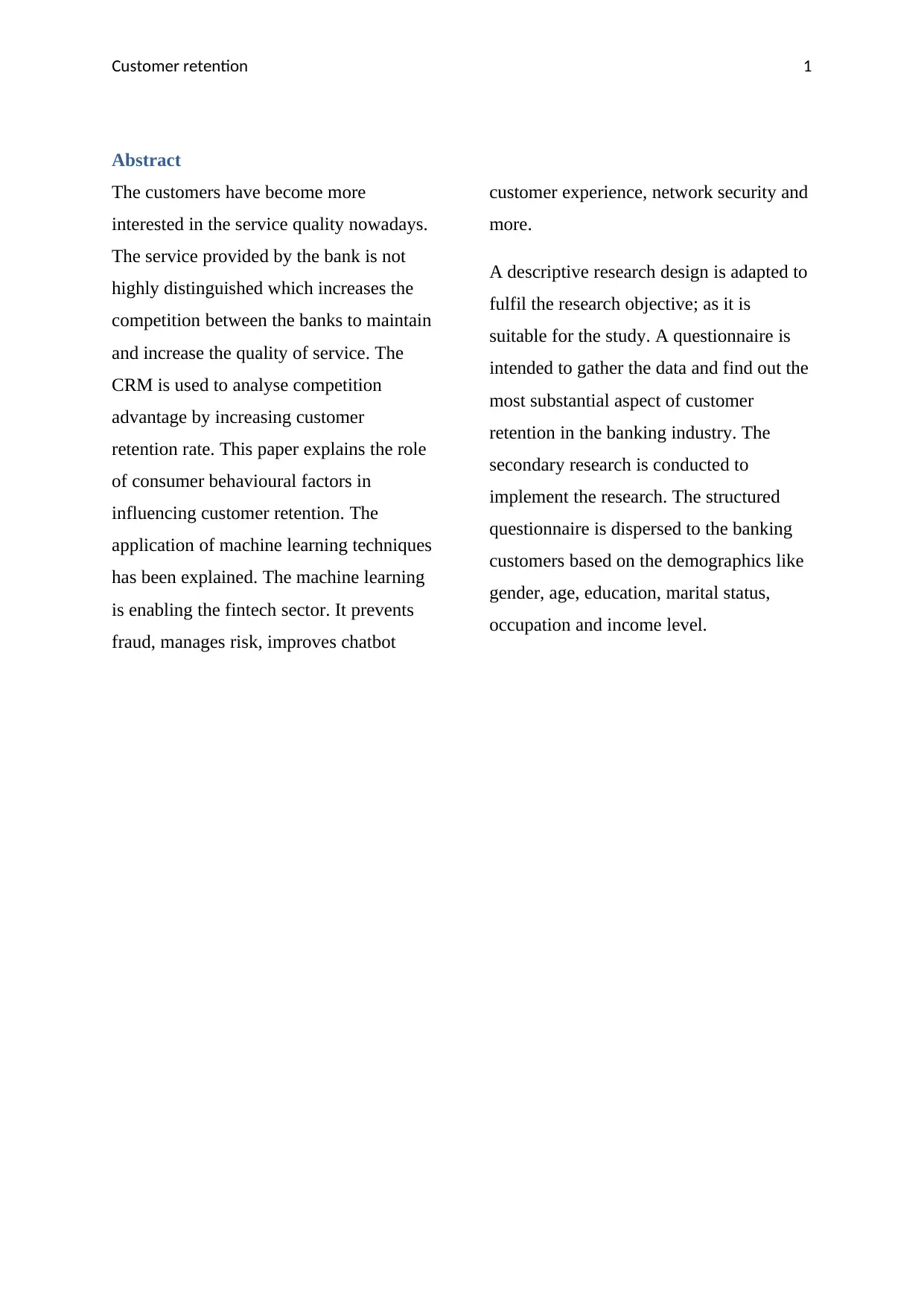
Customer retention 1
Abstract
The customers have become more
interested in the service quality nowadays.
The service provided by the bank is not
highly distinguished which increases the
competition between the banks to maintain
and increase the quality of service. The
CRM is used to analyse competition
advantage by increasing customer
retention rate. This paper explains the role
of consumer behavioural factors in
influencing customer retention. The
application of machine learning techniques
has been explained. The machine learning
is enabling the fintech sector. It prevents
fraud, manages risk, improves chatbot
customer experience, network security and
more.
A descriptive research design is adapted to
fulfil the research objective; as it is
suitable for the study. A questionnaire is
intended to gather the data and find out the
most substantial aspect of customer
retention in the banking industry. The
secondary research is conducted to
implement the research. The structured
questionnaire is dispersed to the banking
customers based on the demographics like
gender, age, education, marital status,
occupation and income level.
Abstract
The customers have become more
interested in the service quality nowadays.
The service provided by the bank is not
highly distinguished which increases the
competition between the banks to maintain
and increase the quality of service. The
CRM is used to analyse competition
advantage by increasing customer
retention rate. This paper explains the role
of consumer behavioural factors in
influencing customer retention. The
application of machine learning techniques
has been explained. The machine learning
is enabling the fintech sector. It prevents
fraud, manages risk, improves chatbot
customer experience, network security and
more.
A descriptive research design is adapted to
fulfil the research objective; as it is
suitable for the study. A questionnaire is
intended to gather the data and find out the
most substantial aspect of customer
retention in the banking industry. The
secondary research is conducted to
implement the research. The structured
questionnaire is dispersed to the banking
customers based on the demographics like
gender, age, education, marital status,
occupation and income level.
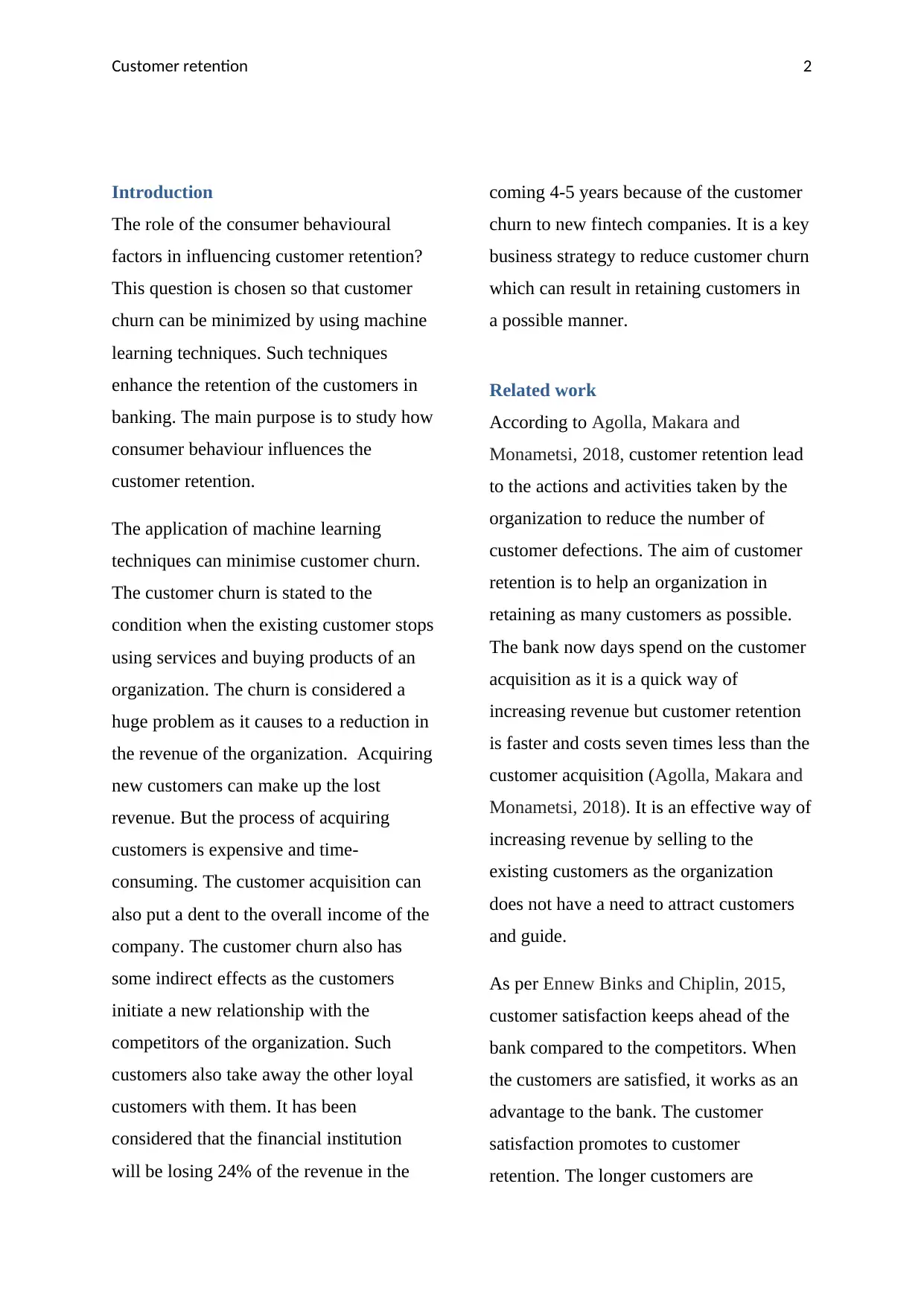
Customer retention 2
Introduction
The role of the consumer behavioural
factors in influencing customer retention?
This question is chosen so that customer
churn can be minimized by using machine
learning techniques. Such techniques
enhance the retention of the customers in
banking. The main purpose is to study how
consumer behaviour influences the
customer retention.
The application of machine learning
techniques can minimise customer churn.
The customer churn is stated to the
condition when the existing customer stops
using services and buying products of an
organization. The churn is considered a
huge problem as it causes to a reduction in
the revenue of the organization. Acquiring
new customers can make up the lost
revenue. But the process of acquiring
customers is expensive and time-
consuming. The customer acquisition can
also put a dent to the overall income of the
company. The customer churn also has
some indirect effects as the customers
initiate a new relationship with the
competitors of the organization. Such
customers also take away the other loyal
customers with them. It has been
considered that the financial institution
will be losing 24% of the revenue in the
coming 4-5 years because of the customer
churn to new fintech companies. It is a key
business strategy to reduce customer churn
which can result in retaining customers in
a possible manner.
Related work
According to Agolla, Makara and
Monametsi, 2018, customer retention lead
to the actions and activities taken by the
organization to reduce the number of
customer defections. The aim of customer
retention is to help an organization in
retaining as many customers as possible.
The bank now days spend on the customer
acquisition as it is a quick way of
increasing revenue but customer retention
is faster and costs seven times less than the
customer acquisition (Agolla, Makara and
Monametsi, 2018). It is an effective way of
increasing revenue by selling to the
existing customers as the organization
does not have a need to attract customers
and guide.
As per Ennew Binks and Chiplin, 2015,
customer satisfaction keeps ahead of the
bank compared to the competitors. When
the customers are satisfied, it works as an
advantage to the bank. The customer
satisfaction promotes to customer
retention. The longer customers are
Introduction
The role of the consumer behavioural
factors in influencing customer retention?
This question is chosen so that customer
churn can be minimized by using machine
learning techniques. Such techniques
enhance the retention of the customers in
banking. The main purpose is to study how
consumer behaviour influences the
customer retention.
The application of machine learning
techniques can minimise customer churn.
The customer churn is stated to the
condition when the existing customer stops
using services and buying products of an
organization. The churn is considered a
huge problem as it causes to a reduction in
the revenue of the organization. Acquiring
new customers can make up the lost
revenue. But the process of acquiring
customers is expensive and time-
consuming. The customer acquisition can
also put a dent to the overall income of the
company. The customer churn also has
some indirect effects as the customers
initiate a new relationship with the
competitors of the organization. Such
customers also take away the other loyal
customers with them. It has been
considered that the financial institution
will be losing 24% of the revenue in the
coming 4-5 years because of the customer
churn to new fintech companies. It is a key
business strategy to reduce customer churn
which can result in retaining customers in
a possible manner.
Related work
According to Agolla, Makara and
Monametsi, 2018, customer retention lead
to the actions and activities taken by the
organization to reduce the number of
customer defections. The aim of customer
retention is to help an organization in
retaining as many customers as possible.
The bank now days spend on the customer
acquisition as it is a quick way of
increasing revenue but customer retention
is faster and costs seven times less than the
customer acquisition (Agolla, Makara and
Monametsi, 2018). It is an effective way of
increasing revenue by selling to the
existing customers as the organization
does not have a need to attract customers
and guide.
As per Ennew Binks and Chiplin, 2015,
customer satisfaction keeps ahead of the
bank compared to the competitors. When
the customers are satisfied, it works as an
advantage to the bank. The customer
satisfaction promotes to customer
retention. The longer customers are
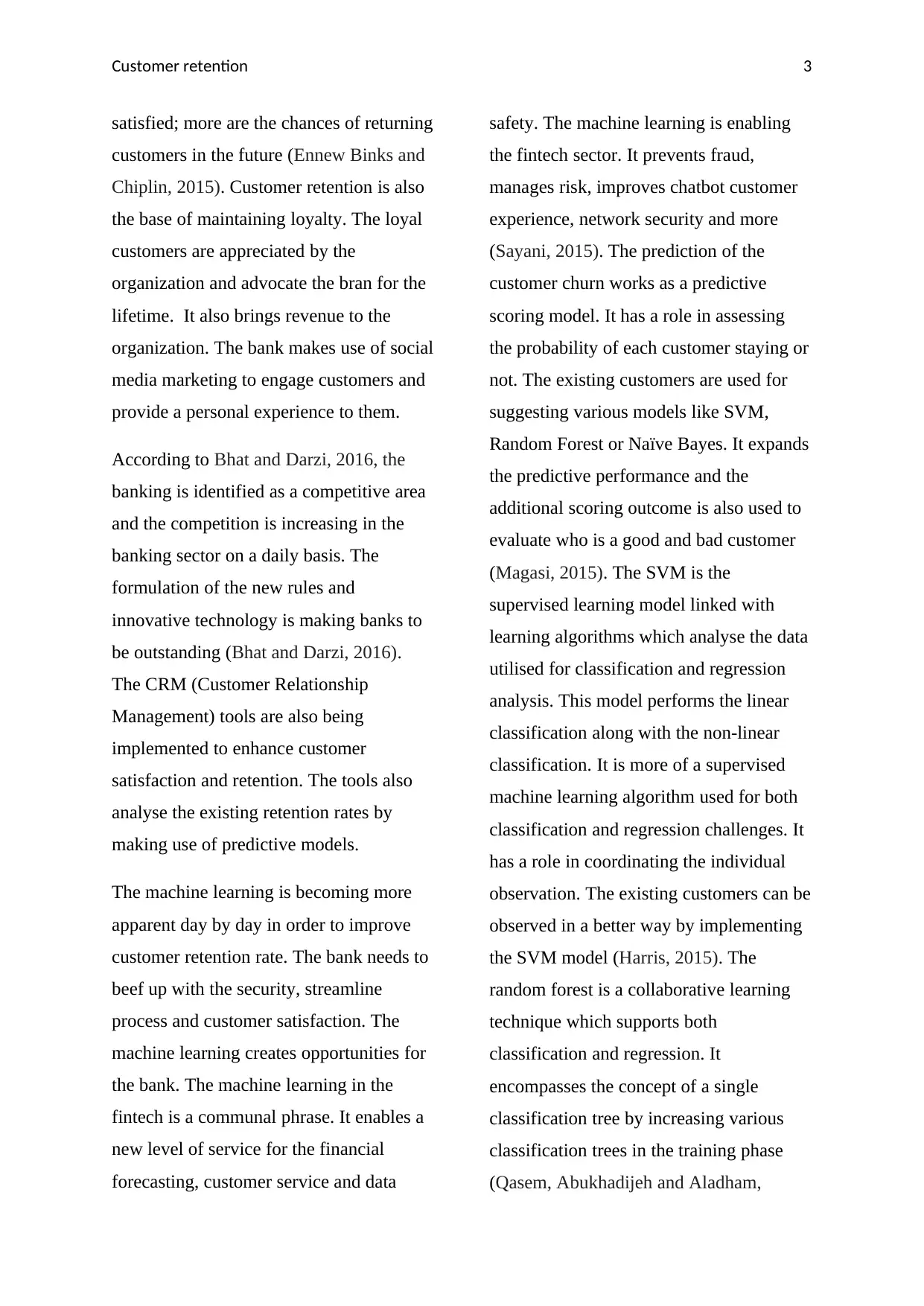
Customer retention 3
satisfied; more are the chances of returning
customers in the future (Ennew Binks and
Chiplin, 2015). Customer retention is also
the base of maintaining loyalty. The loyal
customers are appreciated by the
organization and advocate the bran for the
lifetime. It also brings revenue to the
organization. The bank makes use of social
media marketing to engage customers and
provide a personal experience to them.
According to Bhat and Darzi, 2016, the
banking is identified as a competitive area
and the competition is increasing in the
banking sector on a daily basis. The
formulation of the new rules and
innovative technology is making banks to
be outstanding (Bhat and Darzi, 2016).
The CRM (Customer Relationship
Management) tools are also being
implemented to enhance customer
satisfaction and retention. The tools also
analyse the existing retention rates by
making use of predictive models.
The machine learning is becoming more
apparent day by day in order to improve
customer retention rate. The bank needs to
beef up with the security, streamline
process and customer satisfaction. The
machine learning creates opportunities for
the bank. The machine learning in the
fintech is a communal phrase. It enables a
new level of service for the financial
forecasting, customer service and data
safety. The machine learning is enabling
the fintech sector. It prevents fraud,
manages risk, improves chatbot customer
experience, network security and more
(Sayani, 2015). The prediction of the
customer churn works as a predictive
scoring model. It has a role in assessing
the probability of each customer staying or
not. The existing customers are used for
suggesting various models like SVM,
Random Forest or Naïve Bayes. It expands
the predictive performance and the
additional scoring outcome is also used to
evaluate who is a good and bad customer
(Magasi, 2015). The SVM is the
supervised learning model linked with
learning algorithms which analyse the data
utilised for classification and regression
analysis. This model performs the linear
classification along with the non-linear
classification. It is more of a supervised
machine learning algorithm used for both
classification and regression challenges. It
has a role in coordinating the individual
observation. The existing customers can be
observed in a better way by implementing
the SVM model (Harris, 2015). The
random forest is a collaborative learning
technique which supports both
classification and regression. It
encompasses the concept of a single
classification tree by increasing various
classification trees in the training phase
(Qasem, Abukhadijeh and Aladham,
satisfied; more are the chances of returning
customers in the future (Ennew Binks and
Chiplin, 2015). Customer retention is also
the base of maintaining loyalty. The loyal
customers are appreciated by the
organization and advocate the bran for the
lifetime. It also brings revenue to the
organization. The bank makes use of social
media marketing to engage customers and
provide a personal experience to them.
According to Bhat and Darzi, 2016, the
banking is identified as a competitive area
and the competition is increasing in the
banking sector on a daily basis. The
formulation of the new rules and
innovative technology is making banks to
be outstanding (Bhat and Darzi, 2016).
The CRM (Customer Relationship
Management) tools are also being
implemented to enhance customer
satisfaction and retention. The tools also
analyse the existing retention rates by
making use of predictive models.
The machine learning is becoming more
apparent day by day in order to improve
customer retention rate. The bank needs to
beef up with the security, streamline
process and customer satisfaction. The
machine learning creates opportunities for
the bank. The machine learning in the
fintech is a communal phrase. It enables a
new level of service for the financial
forecasting, customer service and data
safety. The machine learning is enabling
the fintech sector. It prevents fraud,
manages risk, improves chatbot customer
experience, network security and more
(Sayani, 2015). The prediction of the
customer churn works as a predictive
scoring model. It has a role in assessing
the probability of each customer staying or
not. The existing customers are used for
suggesting various models like SVM,
Random Forest or Naïve Bayes. It expands
the predictive performance and the
additional scoring outcome is also used to
evaluate who is a good and bad customer
(Magasi, 2015). The SVM is the
supervised learning model linked with
learning algorithms which analyse the data
utilised for classification and regression
analysis. This model performs the linear
classification along with the non-linear
classification. It is more of a supervised
machine learning algorithm used for both
classification and regression challenges. It
has a role in coordinating the individual
observation. The existing customers can be
observed in a better way by implementing
the SVM model (Harris, 2015). The
random forest is a collaborative learning
technique which supports both
classification and regression. It
encompasses the concept of a single
classification tree by increasing various
classification trees in the training phase
(Qasem, Abukhadijeh and Aladham,
Secure Best Marks with AI Grader
Need help grading? Try our AI Grader for instant feedback on your assignments.
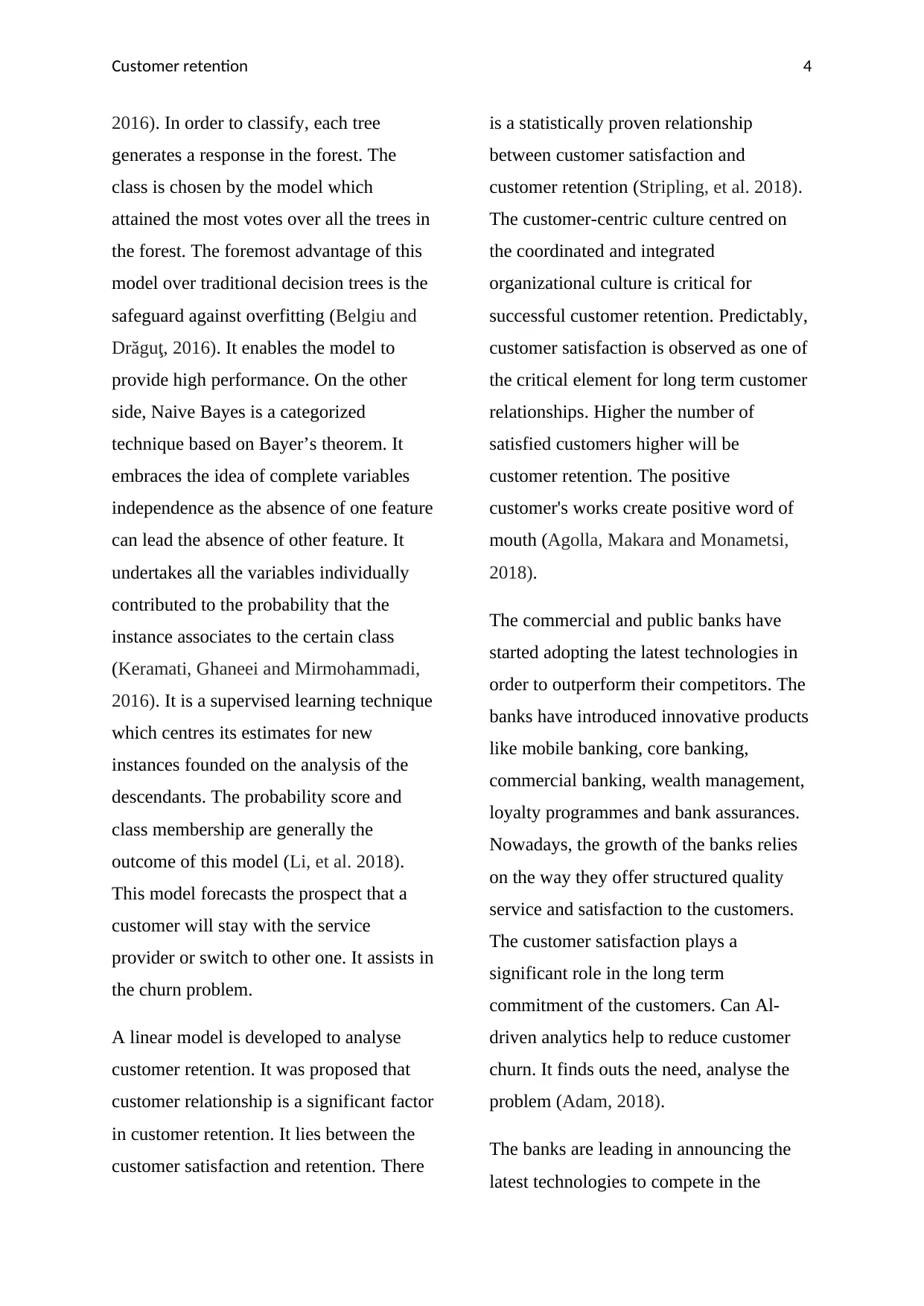
Customer retention 4
2016). In order to classify, each tree
generates a response in the forest. The
class is chosen by the model which
attained the most votes over all the trees in
the forest. The foremost advantage of this
model over traditional decision trees is the
safeguard against overfitting (Belgiu and
Drăguţ, 2016). It enables the model to
provide high performance. On the other
side, Naive Bayes is a categorized
technique based on Bayer’s theorem. It
embraces the idea of complete variables
independence as the absence of one feature
can lead the absence of other feature. It
undertakes all the variables individually
contributed to the probability that the
instance associates to the certain class
(Keramati, Ghaneei and Mirmohammadi,
2016). It is a supervised learning technique
which centres its estimates for new
instances founded on the analysis of the
descendants. The probability score and
class membership are generally the
outcome of this model (Li, et al. 2018).
This model forecasts the prospect that a
customer will stay with the service
provider or switch to other one. It assists in
the churn problem.
A linear model is developed to analyse
customer retention. It was proposed that
customer relationship is a significant factor
in customer retention. It lies between the
customer satisfaction and retention. There
is a statistically proven relationship
between customer satisfaction and
customer retention (Stripling, et al. 2018).
The customer-centric culture centred on
the coordinated and integrated
organizational culture is critical for
successful customer retention. Predictably,
customer satisfaction is observed as one of
the critical element for long term customer
relationships. Higher the number of
satisfied customers higher will be
customer retention. The positive
customer's works create positive word of
mouth (Agolla, Makara and Monametsi,
2018).
The commercial and public banks have
started adopting the latest technologies in
order to outperform their competitors. The
banks have introduced innovative products
like mobile banking, core banking,
commercial banking, wealth management,
loyalty programmes and bank assurances.
Nowadays, the growth of the banks relies
on the way they offer structured quality
service and satisfaction to the customers.
The customer satisfaction plays a
significant role in the long term
commitment of the customers. Can Al-
driven analytics help to reduce customer
churn. It finds outs the need, analyse the
problem (Adam, 2018).
The banks are leading in announcing the
latest technologies to compete in the
2016). In order to classify, each tree
generates a response in the forest. The
class is chosen by the model which
attained the most votes over all the trees in
the forest. The foremost advantage of this
model over traditional decision trees is the
safeguard against overfitting (Belgiu and
Drăguţ, 2016). It enables the model to
provide high performance. On the other
side, Naive Bayes is a categorized
technique based on Bayer’s theorem. It
embraces the idea of complete variables
independence as the absence of one feature
can lead the absence of other feature. It
undertakes all the variables individually
contributed to the probability that the
instance associates to the certain class
(Keramati, Ghaneei and Mirmohammadi,
2016). It is a supervised learning technique
which centres its estimates for new
instances founded on the analysis of the
descendants. The probability score and
class membership are generally the
outcome of this model (Li, et al. 2018).
This model forecasts the prospect that a
customer will stay with the service
provider or switch to other one. It assists in
the churn problem.
A linear model is developed to analyse
customer retention. It was proposed that
customer relationship is a significant factor
in customer retention. It lies between the
customer satisfaction and retention. There
is a statistically proven relationship
between customer satisfaction and
customer retention (Stripling, et al. 2018).
The customer-centric culture centred on
the coordinated and integrated
organizational culture is critical for
successful customer retention. Predictably,
customer satisfaction is observed as one of
the critical element for long term customer
relationships. Higher the number of
satisfied customers higher will be
customer retention. The positive
customer's works create positive word of
mouth (Agolla, Makara and Monametsi,
2018).
The commercial and public banks have
started adopting the latest technologies in
order to outperform their competitors. The
banks have introduced innovative products
like mobile banking, core banking,
commercial banking, wealth management,
loyalty programmes and bank assurances.
Nowadays, the growth of the banks relies
on the way they offer structured quality
service and satisfaction to the customers.
The customer satisfaction plays a
significant role in the long term
commitment of the customers. Can Al-
driven analytics help to reduce customer
churn. It finds outs the need, analyse the
problem (Adam, 2018).
The banks are leading in announcing the
latest technologies to compete in the
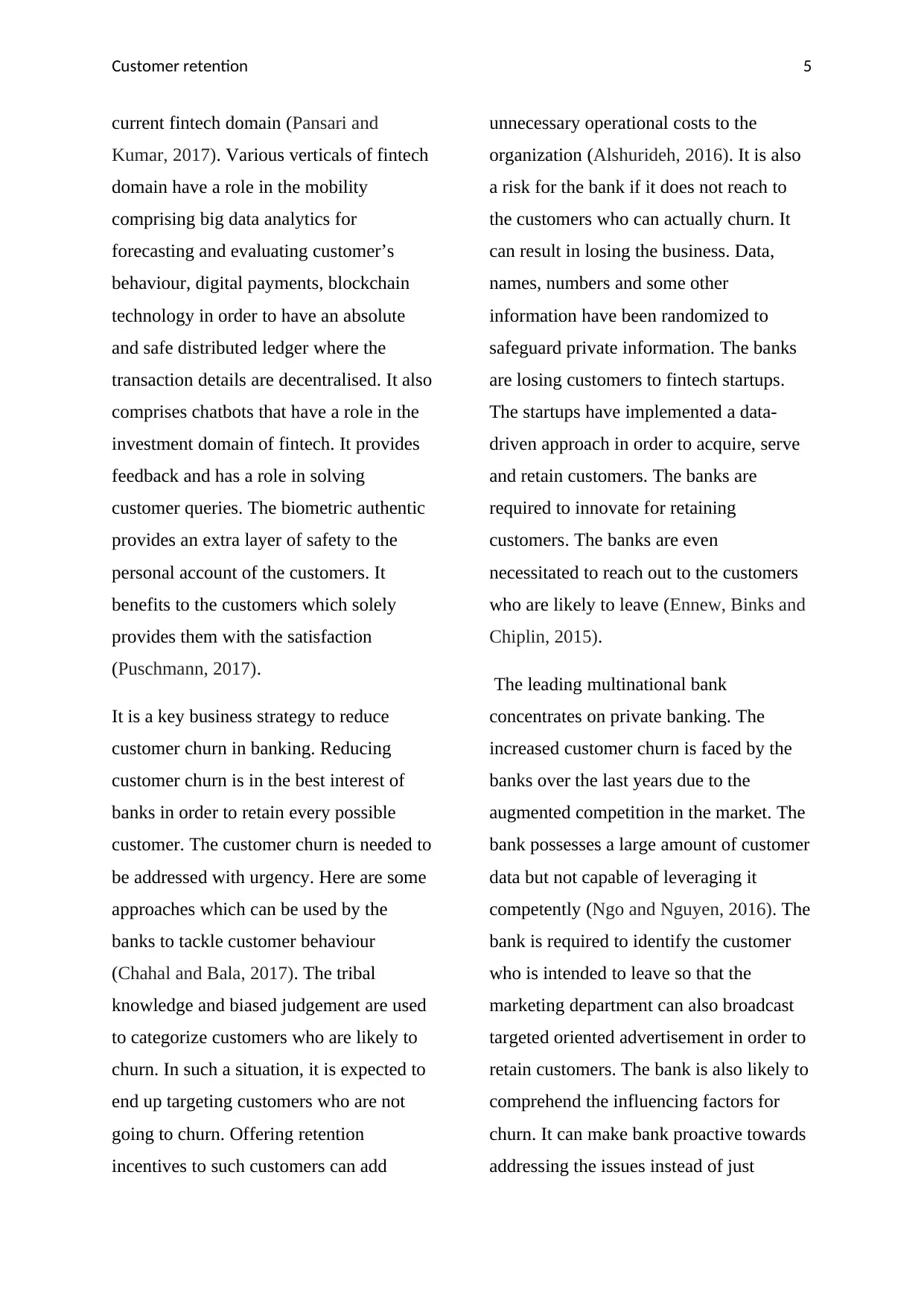
Customer retention 5
current fintech domain (Pansari and
Kumar, 2017). Various verticals of fintech
domain have a role in the mobility
comprising big data analytics for
forecasting and evaluating customer’s
behaviour, digital payments, blockchain
technology in order to have an absolute
and safe distributed ledger where the
transaction details are decentralised. It also
comprises chatbots that have a role in the
investment domain of fintech. It provides
feedback and has a role in solving
customer queries. The biometric authentic
provides an extra layer of safety to the
personal account of the customers. It
benefits to the customers which solely
provides them with the satisfaction
(Puschmann, 2017).
It is a key business strategy to reduce
customer churn in banking. Reducing
customer churn is in the best interest of
banks in order to retain every possible
customer. The customer churn is needed to
be addressed with urgency. Here are some
approaches which can be used by the
banks to tackle customer behaviour
(Chahal and Bala, 2017). The tribal
knowledge and biased judgement are used
to categorize customers who are likely to
churn. In such a situation, it is expected to
end up targeting customers who are not
going to churn. Offering retention
incentives to such customers can add
unnecessary operational costs to the
organization (Alshurideh, 2016). It is also
a risk for the bank if it does not reach to
the customers who can actually churn. It
can result in losing the business. Data,
names, numbers and some other
information have been randomized to
safeguard private information. The banks
are losing customers to fintech startups.
The startups have implemented a data-
driven approach in order to acquire, serve
and retain customers. The banks are
required to innovate for retaining
customers. The banks are even
necessitated to reach out to the customers
who are likely to leave (Ennew, Binks and
Chiplin, 2015).
The leading multinational bank
concentrates on private banking. The
increased customer churn is faced by the
banks over the last years due to the
augmented competition in the market. The
bank possesses a large amount of customer
data but not capable of leveraging it
competently (Ngo and Nguyen, 2016). The
bank is required to identify the customer
who is intended to leave so that the
marketing department can also broadcast
targeted oriented advertisement in order to
retain customers. The bank is also likely to
comprehend the influencing factors for
churn. It can make bank proactive towards
addressing the issues instead of just
current fintech domain (Pansari and
Kumar, 2017). Various verticals of fintech
domain have a role in the mobility
comprising big data analytics for
forecasting and evaluating customer’s
behaviour, digital payments, blockchain
technology in order to have an absolute
and safe distributed ledger where the
transaction details are decentralised. It also
comprises chatbots that have a role in the
investment domain of fintech. It provides
feedback and has a role in solving
customer queries. The biometric authentic
provides an extra layer of safety to the
personal account of the customers. It
benefits to the customers which solely
provides them with the satisfaction
(Puschmann, 2017).
It is a key business strategy to reduce
customer churn in banking. Reducing
customer churn is in the best interest of
banks in order to retain every possible
customer. The customer churn is needed to
be addressed with urgency. Here are some
approaches which can be used by the
banks to tackle customer behaviour
(Chahal and Bala, 2017). The tribal
knowledge and biased judgement are used
to categorize customers who are likely to
churn. In such a situation, it is expected to
end up targeting customers who are not
going to churn. Offering retention
incentives to such customers can add
unnecessary operational costs to the
organization (Alshurideh, 2016). It is also
a risk for the bank if it does not reach to
the customers who can actually churn. It
can result in losing the business. Data,
names, numbers and some other
information have been randomized to
safeguard private information. The banks
are losing customers to fintech startups.
The startups have implemented a data-
driven approach in order to acquire, serve
and retain customers. The banks are
required to innovate for retaining
customers. The banks are even
necessitated to reach out to the customers
who are likely to leave (Ennew, Binks and
Chiplin, 2015).
The leading multinational bank
concentrates on private banking. The
increased customer churn is faced by the
banks over the last years due to the
augmented competition in the market. The
bank possesses a large amount of customer
data but not capable of leveraging it
competently (Ngo and Nguyen, 2016). The
bank is required to identify the customer
who is intended to leave so that the
marketing department can also broadcast
targeted oriented advertisement in order to
retain customers. The bank is also likely to
comprehend the influencing factors for
churn. It can make bank proactive towards
addressing the issues instead of just
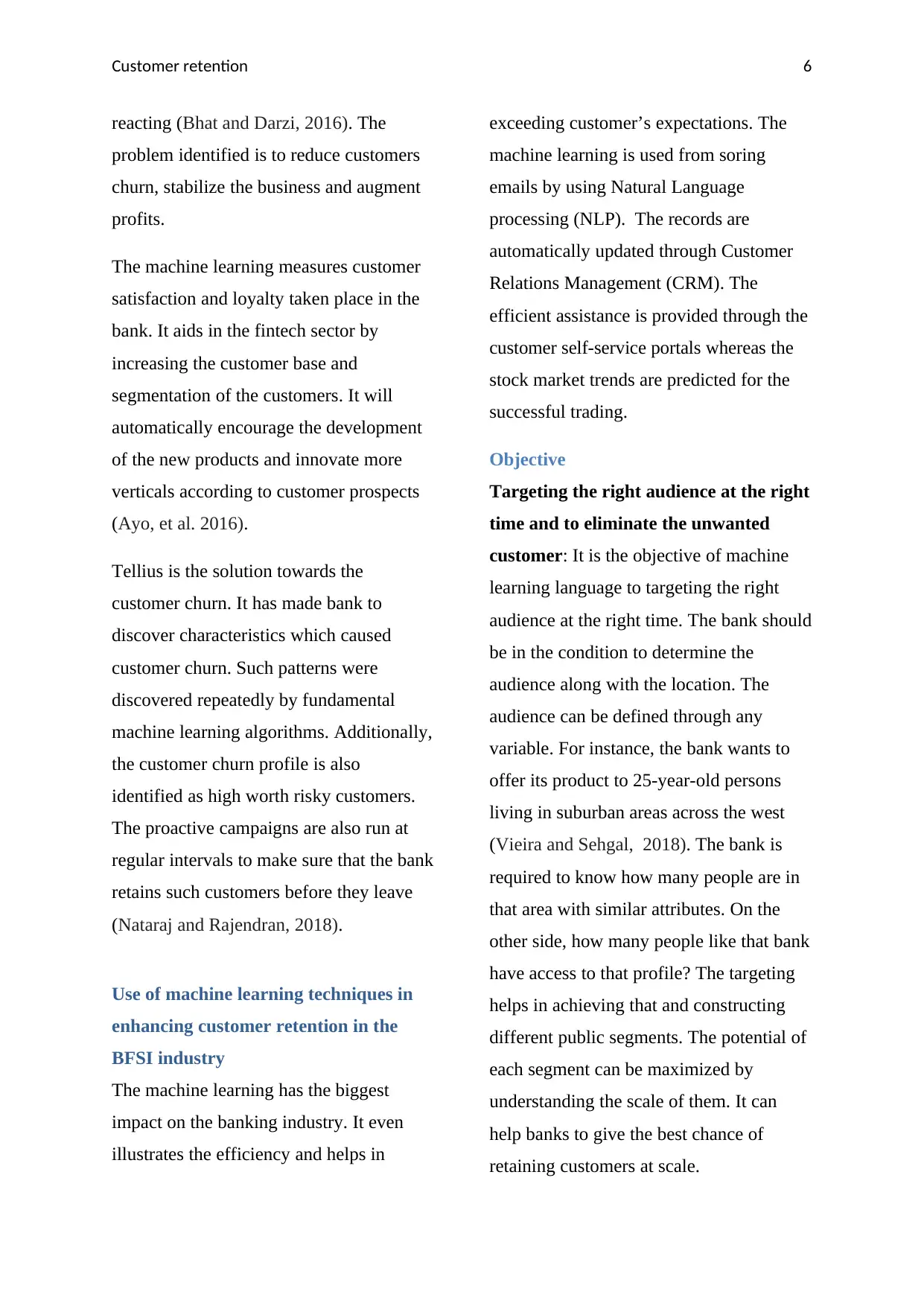
Customer retention 6
reacting (Bhat and Darzi, 2016). The
problem identified is to reduce customers
churn, stabilize the business and augment
profits.
The machine learning measures customer
satisfaction and loyalty taken place in the
bank. It aids in the fintech sector by
increasing the customer base and
segmentation of the customers. It will
automatically encourage the development
of the new products and innovate more
verticals according to customer prospects
(Ayo, et al. 2016).
Tellius is the solution towards the
customer churn. It has made bank to
discover characteristics which caused
customer churn. Such patterns were
discovered repeatedly by fundamental
machine learning algorithms. Additionally,
the customer churn profile is also
identified as high worth risky customers.
The proactive campaigns are also run at
regular intervals to make sure that the bank
retains such customers before they leave
(Nataraj and Rajendran, 2018).
Use of machine learning techniques in
enhancing customer retention in the
BFSI industry
The machine learning has the biggest
impact on the banking industry. It even
illustrates the efficiency and helps in
exceeding customer’s expectations. The
machine learning is used from soring
emails by using Natural Language
processing (NLP). The records are
automatically updated through Customer
Relations Management (CRM). The
efficient assistance is provided through the
customer self-service portals whereas the
stock market trends are predicted for the
successful trading.
Objective
Targeting the right audience at the right
time and to eliminate the unwanted
customer: It is the objective of machine
learning language to targeting the right
audience at the right time. The bank should
be in the condition to determine the
audience along with the location. The
audience can be defined through any
variable. For instance, the bank wants to
offer its product to 25-year-old persons
living in suburban areas across the west
(Vieira and Sehgal, 2018). The bank is
required to know how many people are in
that area with similar attributes. On the
other side, how many people like that bank
have access to that profile? The targeting
helps in achieving that and constructing
different public segments. The potential of
each segment can be maximized by
understanding the scale of them. It can
help banks to give the best chance of
retaining customers at scale.
reacting (Bhat and Darzi, 2016). The
problem identified is to reduce customers
churn, stabilize the business and augment
profits.
The machine learning measures customer
satisfaction and loyalty taken place in the
bank. It aids in the fintech sector by
increasing the customer base and
segmentation of the customers. It will
automatically encourage the development
of the new products and innovate more
verticals according to customer prospects
(Ayo, et al. 2016).
Tellius is the solution towards the
customer churn. It has made bank to
discover characteristics which caused
customer churn. Such patterns were
discovered repeatedly by fundamental
machine learning algorithms. Additionally,
the customer churn profile is also
identified as high worth risky customers.
The proactive campaigns are also run at
regular intervals to make sure that the bank
retains such customers before they leave
(Nataraj and Rajendran, 2018).
Use of machine learning techniques in
enhancing customer retention in the
BFSI industry
The machine learning has the biggest
impact on the banking industry. It even
illustrates the efficiency and helps in
exceeding customer’s expectations. The
machine learning is used from soring
emails by using Natural Language
processing (NLP). The records are
automatically updated through Customer
Relations Management (CRM). The
efficient assistance is provided through the
customer self-service portals whereas the
stock market trends are predicted for the
successful trading.
Objective
Targeting the right audience at the right
time and to eliminate the unwanted
customer: It is the objective of machine
learning language to targeting the right
audience at the right time. The bank should
be in the condition to determine the
audience along with the location. The
audience can be defined through any
variable. For instance, the bank wants to
offer its product to 25-year-old persons
living in suburban areas across the west
(Vieira and Sehgal, 2018). The bank is
required to know how many people are in
that area with similar attributes. On the
other side, how many people like that bank
have access to that profile? The targeting
helps in achieving that and constructing
different public segments. The potential of
each segment can be maximized by
understanding the scale of them. It can
help banks to give the best chance of
retaining customers at scale.
Paraphrase This Document
Need a fresh take? Get an instant paraphrase of this document with our AI Paraphraser
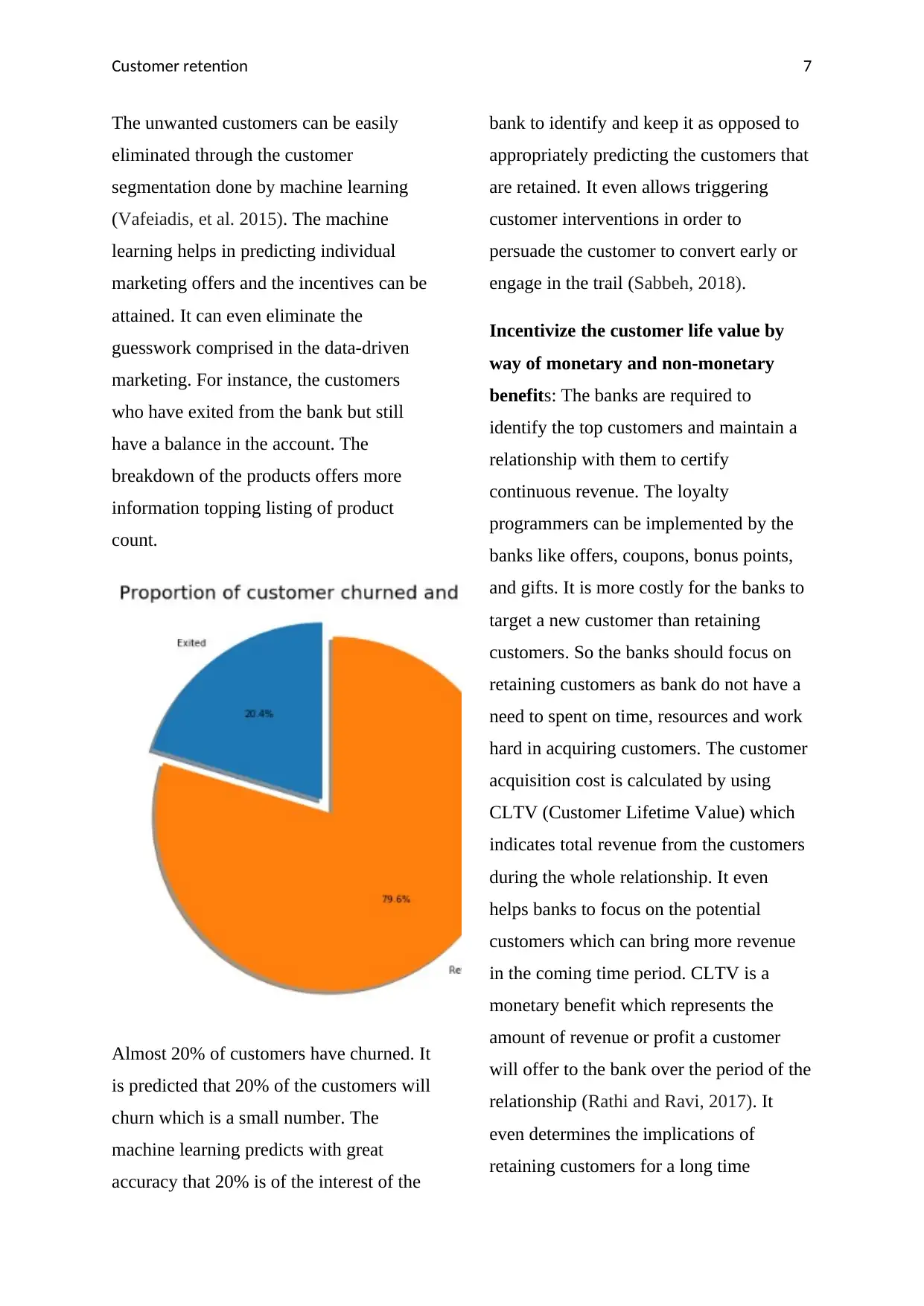
Customer retention 7
The unwanted customers can be easily
eliminated through the customer
segmentation done by machine learning
(Vafeiadis, et al. 2015). The machine
learning helps in predicting individual
marketing offers and the incentives can be
attained. It can even eliminate the
guesswork comprised in the data-driven
marketing. For instance, the customers
who have exited from the bank but still
have a balance in the account. The
breakdown of the products offers more
information topping listing of product
count.
Almost 20% of customers have churned. It
is predicted that 20% of the customers will
churn which is a small number. The
machine learning predicts with great
accuracy that 20% is of the interest of the
bank to identify and keep it as opposed to
appropriately predicting the customers that
are retained. It even allows triggering
customer interventions in order to
persuade the customer to convert early or
engage in the trail (Sabbeh, 2018).
Incentivize the customer life value by
way of monetary and non-monetary
benefits: The banks are required to
identify the top customers and maintain a
relationship with them to certify
continuous revenue. The loyalty
programmers can be implemented by the
banks like offers, coupons, bonus points,
and gifts. It is more costly for the banks to
target a new customer than retaining
customers. So the banks should focus on
retaining customers as bank do not have a
need to spent on time, resources and work
hard in acquiring customers. The customer
acquisition cost is calculated by using
CLTV (Customer Lifetime Value) which
indicates total revenue from the customers
during the whole relationship. It even
helps banks to focus on the potential
customers which can bring more revenue
in the coming time period. CLTV is a
monetary benefit which represents the
amount of revenue or profit a customer
will offer to the bank over the period of the
relationship (Rathi and Ravi, 2017). It
even determines the implications of
retaining customers for a long time
The unwanted customers can be easily
eliminated through the customer
segmentation done by machine learning
(Vafeiadis, et al. 2015). The machine
learning helps in predicting individual
marketing offers and the incentives can be
attained. It can even eliminate the
guesswork comprised in the data-driven
marketing. For instance, the customers
who have exited from the bank but still
have a balance in the account. The
breakdown of the products offers more
information topping listing of product
count.
Almost 20% of customers have churned. It
is predicted that 20% of the customers will
churn which is a small number. The
machine learning predicts with great
accuracy that 20% is of the interest of the
bank to identify and keep it as opposed to
appropriately predicting the customers that
are retained. It even allows triggering
customer interventions in order to
persuade the customer to convert early or
engage in the trail (Sabbeh, 2018).
Incentivize the customer life value by
way of monetary and non-monetary
benefits: The banks are required to
identify the top customers and maintain a
relationship with them to certify
continuous revenue. The loyalty
programmers can be implemented by the
banks like offers, coupons, bonus points,
and gifts. It is more costly for the banks to
target a new customer than retaining
customers. So the banks should focus on
retaining customers as bank do not have a
need to spent on time, resources and work
hard in acquiring customers. The customer
acquisition cost is calculated by using
CLTV (Customer Lifetime Value) which
indicates total revenue from the customers
during the whole relationship. It even
helps banks to focus on the potential
customers which can bring more revenue
in the coming time period. CLTV is a
monetary benefit which represents the
amount of revenue or profit a customer
will offer to the bank over the period of the
relationship (Rathi and Ravi, 2017). It
even determines the implications of
retaining customers for a long time
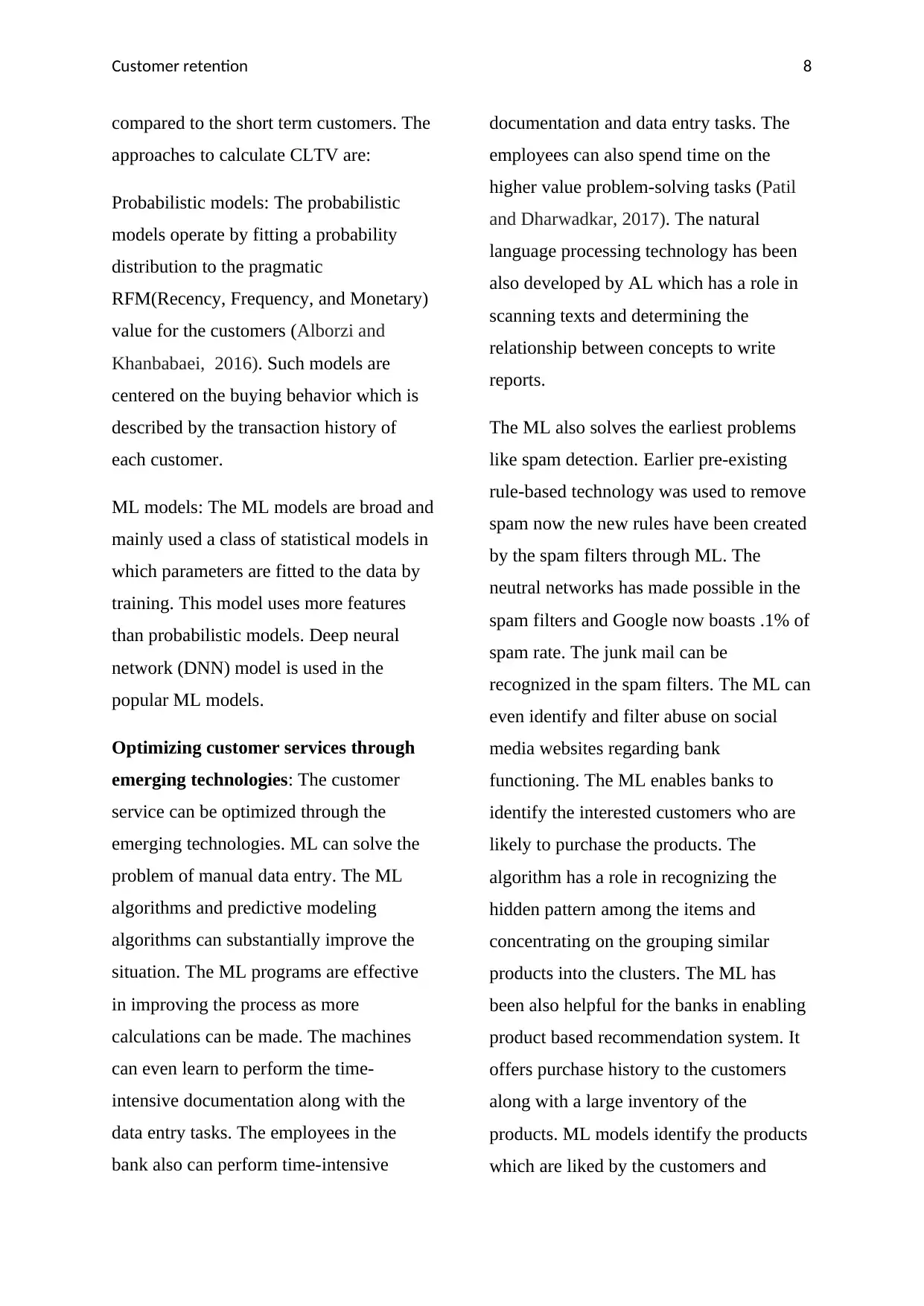
Customer retention 8
compared to the short term customers. The
approaches to calculate CLTV are:
Probabilistic models: The probabilistic
models operate by fitting a probability
distribution to the pragmatic
RFM(Recency, Frequency, and Monetary)
value for the customers (Alborzi and
Khanbabaei, 2016). Such models are
centered on the buying behavior which is
described by the transaction history of
each customer.
ML models: The ML models are broad and
mainly used a class of statistical models in
which parameters are fitted to the data by
training. This model uses more features
than probabilistic models. Deep neural
network (DNN) model is used in the
popular ML models.
Optimizing customer services through
emerging technologies: The customer
service can be optimized through the
emerging technologies. ML can solve the
problem of manual data entry. The ML
algorithms and predictive modeling
algorithms can substantially improve the
situation. The ML programs are effective
in improving the process as more
calculations can be made. The machines
can even learn to perform the time-
intensive documentation along with the
data entry tasks. The employees in the
bank also can perform time-intensive
documentation and data entry tasks. The
employees can also spend time on the
higher value problem-solving tasks (Patil
and Dharwadkar, 2017). The natural
language processing technology has been
also developed by AL which has a role in
scanning texts and determining the
relationship between concepts to write
reports.
The ML also solves the earliest problems
like spam detection. Earlier pre-existing
rule-based technology was used to remove
spam now the new rules have been created
by the spam filters through ML. The
neutral networks has made possible in the
spam filters and Google now boasts .1% of
spam rate. The junk mail can be
recognized in the spam filters. The ML can
even identify and filter abuse on social
media websites regarding bank
functioning. The ML enables banks to
identify the interested customers who are
likely to purchase the products. The
algorithm has a role in recognizing the
hidden pattern among the items and
concentrating on the grouping similar
products into the clusters. The ML has
been also helpful for the banks in enabling
product based recommendation system. It
offers purchase history to the customers
along with a large inventory of the
products. ML models identify the products
which are liked by the customers and
compared to the short term customers. The
approaches to calculate CLTV are:
Probabilistic models: The probabilistic
models operate by fitting a probability
distribution to the pragmatic
RFM(Recency, Frequency, and Monetary)
value for the customers (Alborzi and
Khanbabaei, 2016). Such models are
centered on the buying behavior which is
described by the transaction history of
each customer.
ML models: The ML models are broad and
mainly used a class of statistical models in
which parameters are fitted to the data by
training. This model uses more features
than probabilistic models. Deep neural
network (DNN) model is used in the
popular ML models.
Optimizing customer services through
emerging technologies: The customer
service can be optimized through the
emerging technologies. ML can solve the
problem of manual data entry. The ML
algorithms and predictive modeling
algorithms can substantially improve the
situation. The ML programs are effective
in improving the process as more
calculations can be made. The machines
can even learn to perform the time-
intensive documentation along with the
data entry tasks. The employees in the
bank also can perform time-intensive
documentation and data entry tasks. The
employees can also spend time on the
higher value problem-solving tasks (Patil
and Dharwadkar, 2017). The natural
language processing technology has been
also developed by AL which has a role in
scanning texts and determining the
relationship between concepts to write
reports.
The ML also solves the earliest problems
like spam detection. Earlier pre-existing
rule-based technology was used to remove
spam now the new rules have been created
by the spam filters through ML. The
neutral networks has made possible in the
spam filters and Google now boasts .1% of
spam rate. The junk mail can be
recognized in the spam filters. The ML can
even identify and filter abuse on social
media websites regarding bank
functioning. The ML enables banks to
identify the interested customers who are
likely to purchase the products. The
algorithm has a role in recognizing the
hidden pattern among the items and
concentrating on the grouping similar
products into the clusters. The ML has
been also helpful for the banks in enabling
product based recommendation system. It
offers purchase history to the customers
along with a large inventory of the
products. ML models identify the products
which are liked by the customers and
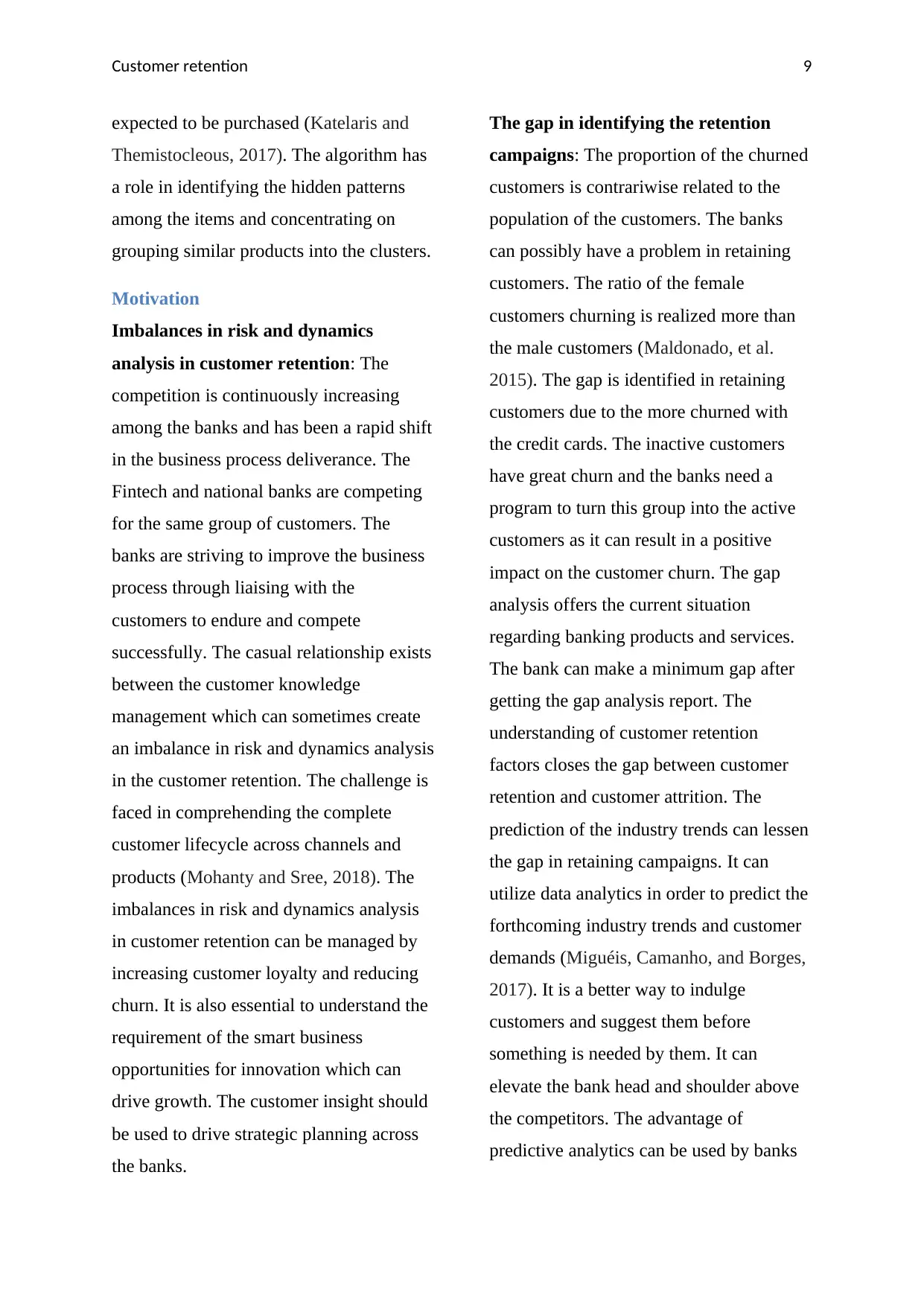
Customer retention 9
expected to be purchased (Katelaris and
Themistocleous, 2017). The algorithm has
a role in identifying the hidden patterns
among the items and concentrating on
grouping similar products into the clusters.
Motivation
Imbalances in risk and dynamics
analysis in customer retention: The
competition is continuously increasing
among the banks and has been a rapid shift
in the business process deliverance. The
Fintech and national banks are competing
for the same group of customers. The
banks are striving to improve the business
process through liaising with the
customers to endure and compete
successfully. The casual relationship exists
between the customer knowledge
management which can sometimes create
an imbalance in risk and dynamics analysis
in the customer retention. The challenge is
faced in comprehending the complete
customer lifecycle across channels and
products (Mohanty and Sree, 2018). The
imbalances in risk and dynamics analysis
in customer retention can be managed by
increasing customer loyalty and reducing
churn. It is also essential to understand the
requirement of the smart business
opportunities for innovation which can
drive growth. The customer insight should
be used to drive strategic planning across
the banks.
The gap in identifying the retention
campaigns: The proportion of the churned
customers is contrariwise related to the
population of the customers. The banks
can possibly have a problem in retaining
customers. The ratio of the female
customers churning is realized more than
the male customers (Maldonado, et al.
2015). The gap is identified in retaining
customers due to the more churned with
the credit cards. The inactive customers
have great churn and the banks need a
program to turn this group into the active
customers as it can result in a positive
impact on the customer churn. The gap
analysis offers the current situation
regarding banking products and services.
The bank can make a minimum gap after
getting the gap analysis report. The
understanding of customer retention
factors closes the gap between customer
retention and customer attrition. The
prediction of the industry trends can lessen
the gap in retaining campaigns. It can
utilize data analytics in order to predict the
forthcoming industry trends and customer
demands (Miguéis, Camanho, and Borges,
2017). It is a better way to indulge
customers and suggest them before
something is needed by them. It can
elevate the bank head and shoulder above
the competitors. The advantage of
predictive analytics can be used by banks
expected to be purchased (Katelaris and
Themistocleous, 2017). The algorithm has
a role in identifying the hidden patterns
among the items and concentrating on
grouping similar products into the clusters.
Motivation
Imbalances in risk and dynamics
analysis in customer retention: The
competition is continuously increasing
among the banks and has been a rapid shift
in the business process deliverance. The
Fintech and national banks are competing
for the same group of customers. The
banks are striving to improve the business
process through liaising with the
customers to endure and compete
successfully. The casual relationship exists
between the customer knowledge
management which can sometimes create
an imbalance in risk and dynamics analysis
in the customer retention. The challenge is
faced in comprehending the complete
customer lifecycle across channels and
products (Mohanty and Sree, 2018). The
imbalances in risk and dynamics analysis
in customer retention can be managed by
increasing customer loyalty and reducing
churn. It is also essential to understand the
requirement of the smart business
opportunities for innovation which can
drive growth. The customer insight should
be used to drive strategic planning across
the banks.
The gap in identifying the retention
campaigns: The proportion of the churned
customers is contrariwise related to the
population of the customers. The banks
can possibly have a problem in retaining
customers. The ratio of the female
customers churning is realized more than
the male customers (Maldonado, et al.
2015). The gap is identified in retaining
customers due to the more churned with
the credit cards. The inactive customers
have great churn and the banks need a
program to turn this group into the active
customers as it can result in a positive
impact on the customer churn. The gap
analysis offers the current situation
regarding banking products and services.
The bank can make a minimum gap after
getting the gap analysis report. The
understanding of customer retention
factors closes the gap between customer
retention and customer attrition. The
prediction of the industry trends can lessen
the gap in retaining campaigns. It can
utilize data analytics in order to predict the
forthcoming industry trends and customer
demands (Miguéis, Camanho, and Borges,
2017). It is a better way to indulge
customers and suggest them before
something is needed by them. It can
elevate the bank head and shoulder above
the competitors. The advantage of
predictive analytics can be used by banks
Secure Best Marks with AI Grader
Need help grading? Try our AI Grader for instant feedback on your assignments.
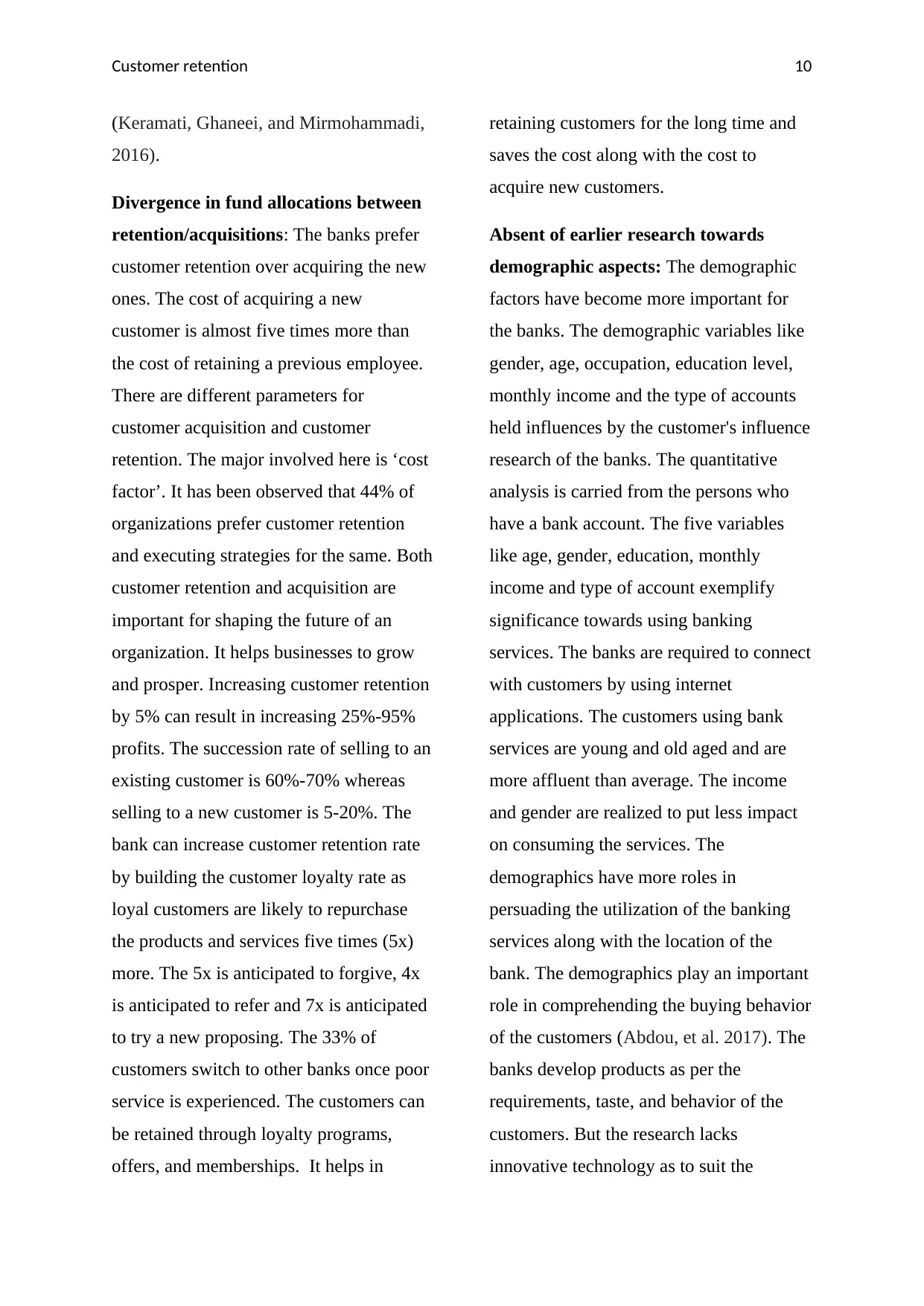
Customer retention 10
(Keramati, Ghaneei, and Mirmohammadi,
2016).
Divergence in fund allocations between
retention/acquisitions: The banks prefer
customer retention over acquiring the new
ones. The cost of acquiring a new
customer is almost five times more than
the cost of retaining a previous employee.
There are different parameters for
customer acquisition and customer
retention. The major involved here is ‘cost
factor’. It has been observed that 44% of
organizations prefer customer retention
and executing strategies for the same. Both
customer retention and acquisition are
important for shaping the future of an
organization. It helps businesses to grow
and prosper. Increasing customer retention
by 5% can result in increasing 25%-95%
profits. The succession rate of selling to an
existing customer is 60%-70% whereas
selling to a new customer is 5-20%. The
bank can increase customer retention rate
by building the customer loyalty rate as
loyal customers are likely to repurchase
the products and services five times (5x)
more. The 5x is anticipated to forgive, 4x
is anticipated to refer and 7x is anticipated
to try a new proposing. The 33% of
customers switch to other banks once poor
service is experienced. The customers can
be retained through loyalty programs,
offers, and memberships. It helps in
retaining customers for the long time and
saves the cost along with the cost to
acquire new customers.
Absent of earlier research towards
demographic aspects: The demographic
factors have become more important for
the banks. The demographic variables like
gender, age, occupation, education level,
monthly income and the type of accounts
held influences by the customer's influence
research of the banks. The quantitative
analysis is carried from the persons who
have a bank account. The five variables
like age, gender, education, monthly
income and type of account exemplify
significance towards using banking
services. The banks are required to connect
with customers by using internet
applications. The customers using bank
services are young and old aged and are
more affluent than average. The income
and gender are realized to put less impact
on consuming the services. The
demographics have more roles in
persuading the utilization of the banking
services along with the location of the
bank. The demographics play an important
role in comprehending the buying behavior
of the customers (Abdou, et al. 2017). The
banks develop products as per the
requirements, taste, and behavior of the
customers. But the research lacks
innovative technology as to suit the
(Keramati, Ghaneei, and Mirmohammadi,
2016).
Divergence in fund allocations between
retention/acquisitions: The banks prefer
customer retention over acquiring the new
ones. The cost of acquiring a new
customer is almost five times more than
the cost of retaining a previous employee.
There are different parameters for
customer acquisition and customer
retention. The major involved here is ‘cost
factor’. It has been observed that 44% of
organizations prefer customer retention
and executing strategies for the same. Both
customer retention and acquisition are
important for shaping the future of an
organization. It helps businesses to grow
and prosper. Increasing customer retention
by 5% can result in increasing 25%-95%
profits. The succession rate of selling to an
existing customer is 60%-70% whereas
selling to a new customer is 5-20%. The
bank can increase customer retention rate
by building the customer loyalty rate as
loyal customers are likely to repurchase
the products and services five times (5x)
more. The 5x is anticipated to forgive, 4x
is anticipated to refer and 7x is anticipated
to try a new proposing. The 33% of
customers switch to other banks once poor
service is experienced. The customers can
be retained through loyalty programs,
offers, and memberships. It helps in
retaining customers for the long time and
saves the cost along with the cost to
acquire new customers.
Absent of earlier research towards
demographic aspects: The demographic
factors have become more important for
the banks. The demographic variables like
gender, age, occupation, education level,
monthly income and the type of accounts
held influences by the customer's influence
research of the banks. The quantitative
analysis is carried from the persons who
have a bank account. The five variables
like age, gender, education, monthly
income and type of account exemplify
significance towards using banking
services. The banks are required to connect
with customers by using internet
applications. The customers using bank
services are young and old aged and are
more affluent than average. The income
and gender are realized to put less impact
on consuming the services. The
demographics have more roles in
persuading the utilization of the banking
services along with the location of the
bank. The demographics play an important
role in comprehending the buying behavior
of the customers (Abdou, et al. 2017). The
banks develop products as per the
requirements, taste, and behavior of the
customers. But the research lacks
innovative technology as to suit the
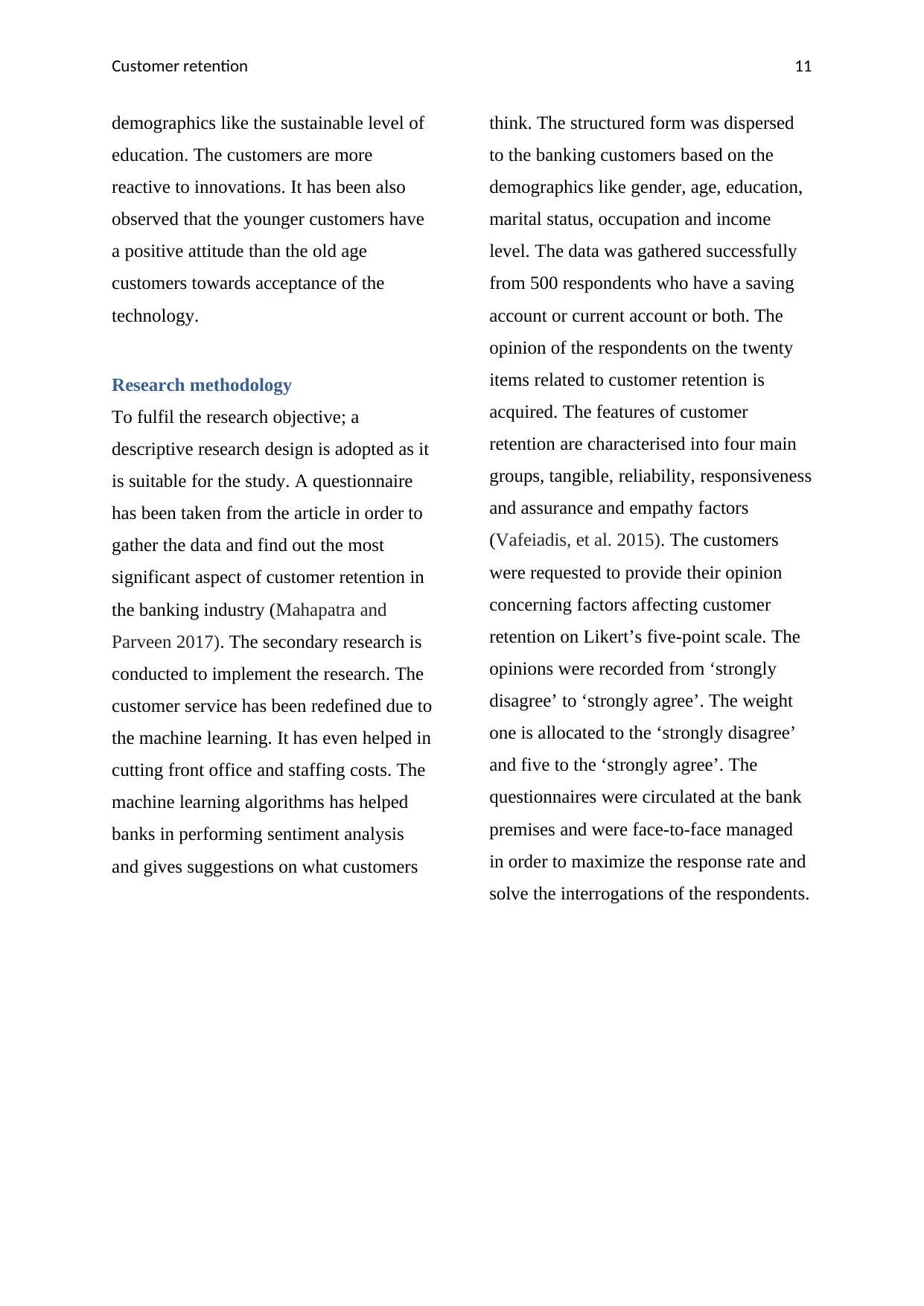
Customer retention 11
demographics like the sustainable level of
education. The customers are more
reactive to innovations. It has been also
observed that the younger customers have
a positive attitude than the old age
customers towards acceptance of the
technology.
Research methodology
To fulfil the research objective; a
descriptive research design is adopted as it
is suitable for the study. A questionnaire
has been taken from the article in order to
gather the data and find out the most
significant aspect of customer retention in
the banking industry (Mahapatra and
Parveen 2017). The secondary research is
conducted to implement the research. The
customer service has been redefined due to
the machine learning. It has even helped in
cutting front office and staffing costs. The
machine learning algorithms has helped
banks in performing sentiment analysis
and gives suggestions on what customers
think. The structured form was dispersed
to the banking customers based on the
demographics like gender, age, education,
marital status, occupation and income
level. The data was gathered successfully
from 500 respondents who have a saving
account or current account or both. The
opinion of the respondents on the twenty
items related to customer retention is
acquired. The features of customer
retention are characterised into four main
groups, tangible, reliability, responsiveness
and assurance and empathy factors
(Vafeiadis, et al. 2015). The customers
were requested to provide their opinion
concerning factors affecting customer
retention on Likert’s five-point scale. The
opinions were recorded from ‘strongly
disagree’ to ‘strongly agree’. The weight
one is allocated to the ‘strongly disagree’
and five to the ‘strongly agree’. The
questionnaires were circulated at the bank
premises and were face-to-face managed
in order to maximize the response rate and
solve the interrogations of the respondents.
demographics like the sustainable level of
education. The customers are more
reactive to innovations. It has been also
observed that the younger customers have
a positive attitude than the old age
customers towards acceptance of the
technology.
Research methodology
To fulfil the research objective; a
descriptive research design is adopted as it
is suitable for the study. A questionnaire
has been taken from the article in order to
gather the data and find out the most
significant aspect of customer retention in
the banking industry (Mahapatra and
Parveen 2017). The secondary research is
conducted to implement the research. The
customer service has been redefined due to
the machine learning. It has even helped in
cutting front office and staffing costs. The
machine learning algorithms has helped
banks in performing sentiment analysis
and gives suggestions on what customers
think. The structured form was dispersed
to the banking customers based on the
demographics like gender, age, education,
marital status, occupation and income
level. The data was gathered successfully
from 500 respondents who have a saving
account or current account or both. The
opinion of the respondents on the twenty
items related to customer retention is
acquired. The features of customer
retention are characterised into four main
groups, tangible, reliability, responsiveness
and assurance and empathy factors
(Vafeiadis, et al. 2015). The customers
were requested to provide their opinion
concerning factors affecting customer
retention on Likert’s five-point scale. The
opinions were recorded from ‘strongly
disagree’ to ‘strongly agree’. The weight
one is allocated to the ‘strongly disagree’
and five to the ‘strongly agree’. The
questionnaires were circulated at the bank
premises and were face-to-face managed
in order to maximize the response rate and
solve the interrogations of the respondents.
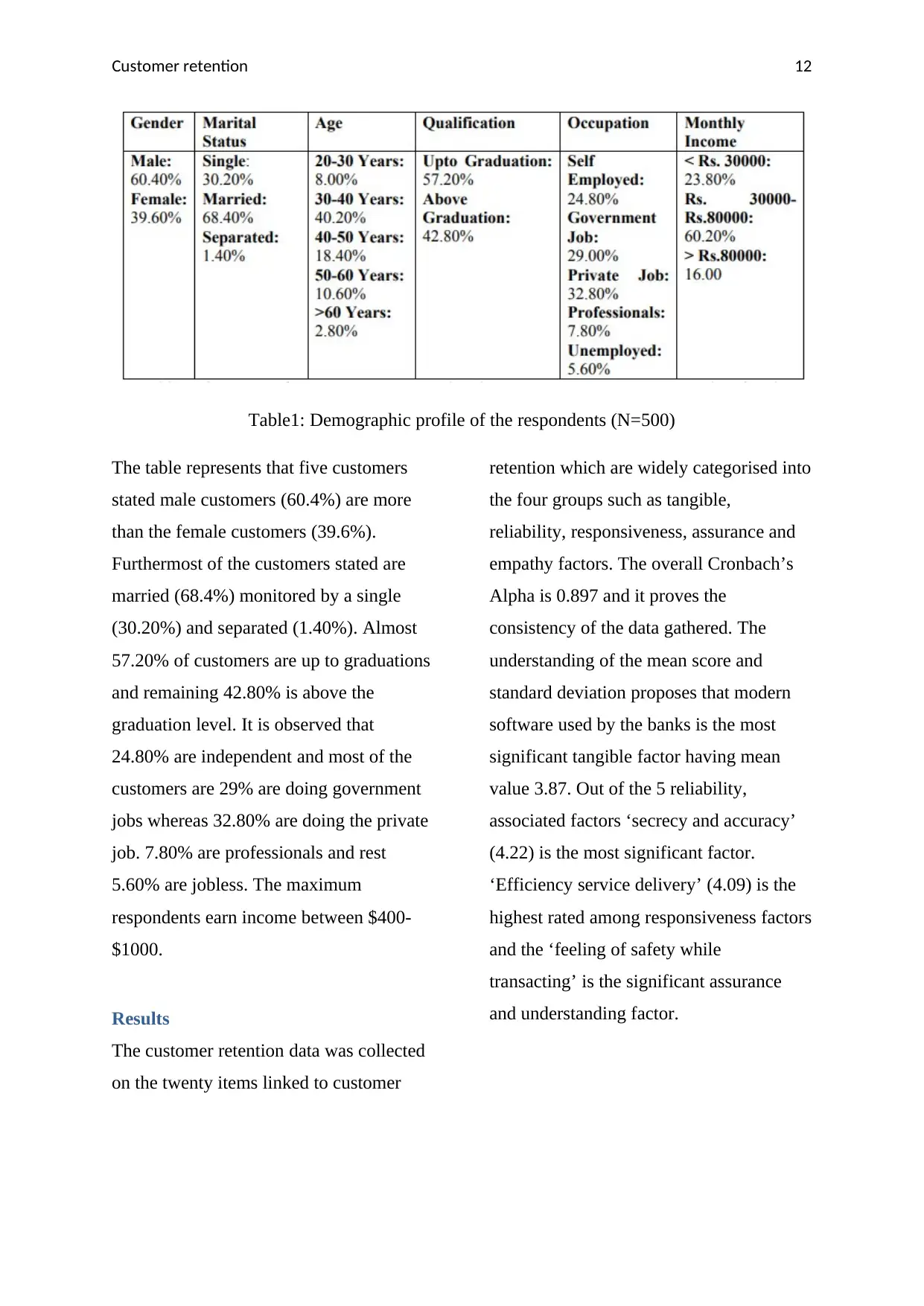
Customer retention 12
Table1: Demographic profile of the respondents (N=500)
The table represents that five customers
stated male customers (60.4%) are more
than the female customers (39.6%).
Furthermost of the customers stated are
married (68.4%) monitored by a single
(30.20%) and separated (1.40%). Almost
57.20% of customers are up to graduations
and remaining 42.80% is above the
graduation level. It is observed that
24.80% are independent and most of the
customers are 29% are doing government
jobs whereas 32.80% are doing the private
job. 7.80% are professionals and rest
5.60% are jobless. The maximum
respondents earn income between $400-
$1000.
Results
The customer retention data was collected
on the twenty items linked to customer
retention which are widely categorised into
the four groups such as tangible,
reliability, responsiveness, assurance and
empathy factors. The overall Cronbach’s
Alpha is 0.897 and it proves the
consistency of the data gathered. The
understanding of the mean score and
standard deviation proposes that modern
software used by the banks is the most
significant tangible factor having mean
value 3.87. Out of the 5 reliability,
associated factors ‘secrecy and accuracy’
(4.22) is the most significant factor.
‘Efficiency service delivery’ (4.09) is the
highest rated among responsiveness factors
and the ‘feeling of safety while
transacting’ is the significant assurance
and understanding factor.
Table1: Demographic profile of the respondents (N=500)
The table represents that five customers
stated male customers (60.4%) are more
than the female customers (39.6%).
Furthermost of the customers stated are
married (68.4%) monitored by a single
(30.20%) and separated (1.40%). Almost
57.20% of customers are up to graduations
and remaining 42.80% is above the
graduation level. It is observed that
24.80% are independent and most of the
customers are 29% are doing government
jobs whereas 32.80% are doing the private
job. 7.80% are professionals and rest
5.60% are jobless. The maximum
respondents earn income between $400-
$1000.
Results
The customer retention data was collected
on the twenty items linked to customer
retention which are widely categorised into
the four groups such as tangible,
reliability, responsiveness, assurance and
empathy factors. The overall Cronbach’s
Alpha is 0.897 and it proves the
consistency of the data gathered. The
understanding of the mean score and
standard deviation proposes that modern
software used by the banks is the most
significant tangible factor having mean
value 3.87. Out of the 5 reliability,
associated factors ‘secrecy and accuracy’
(4.22) is the most significant factor.
‘Efficiency service delivery’ (4.09) is the
highest rated among responsiveness factors
and the ‘feeling of safety while
transacting’ is the significant assurance
and understanding factor.
Paraphrase This Document
Need a fresh take? Get an instant paraphrase of this document with our AI Paraphraser
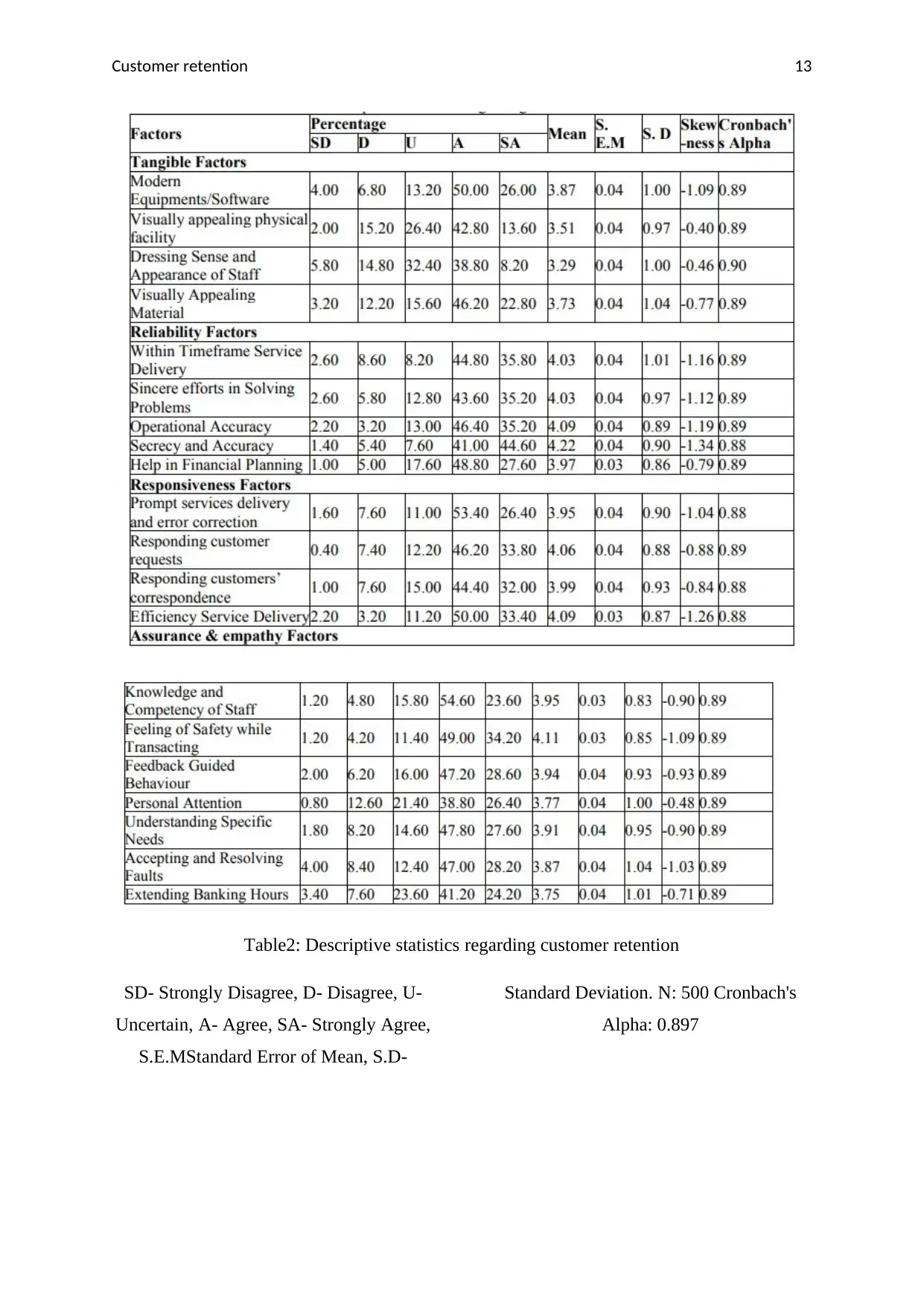
Customer retention 13
Table2: Descriptive statistics regarding customer retention
SD- Strongly Disagree, D- Disagree, U-
Uncertain, A- Agree, SA- Strongly Agree,
S.E.MStandard Error of Mean, S.D-
Standard Deviation. N: 500 Cronbach's
Alpha: 0.897
Table2: Descriptive statistics regarding customer retention
SD- Strongly Disagree, D- Disagree, U-
Uncertain, A- Agree, SA- Strongly Agree,
S.E.MStandard Error of Mean, S.D-
Standard Deviation. N: 500 Cronbach's
Alpha: 0.897
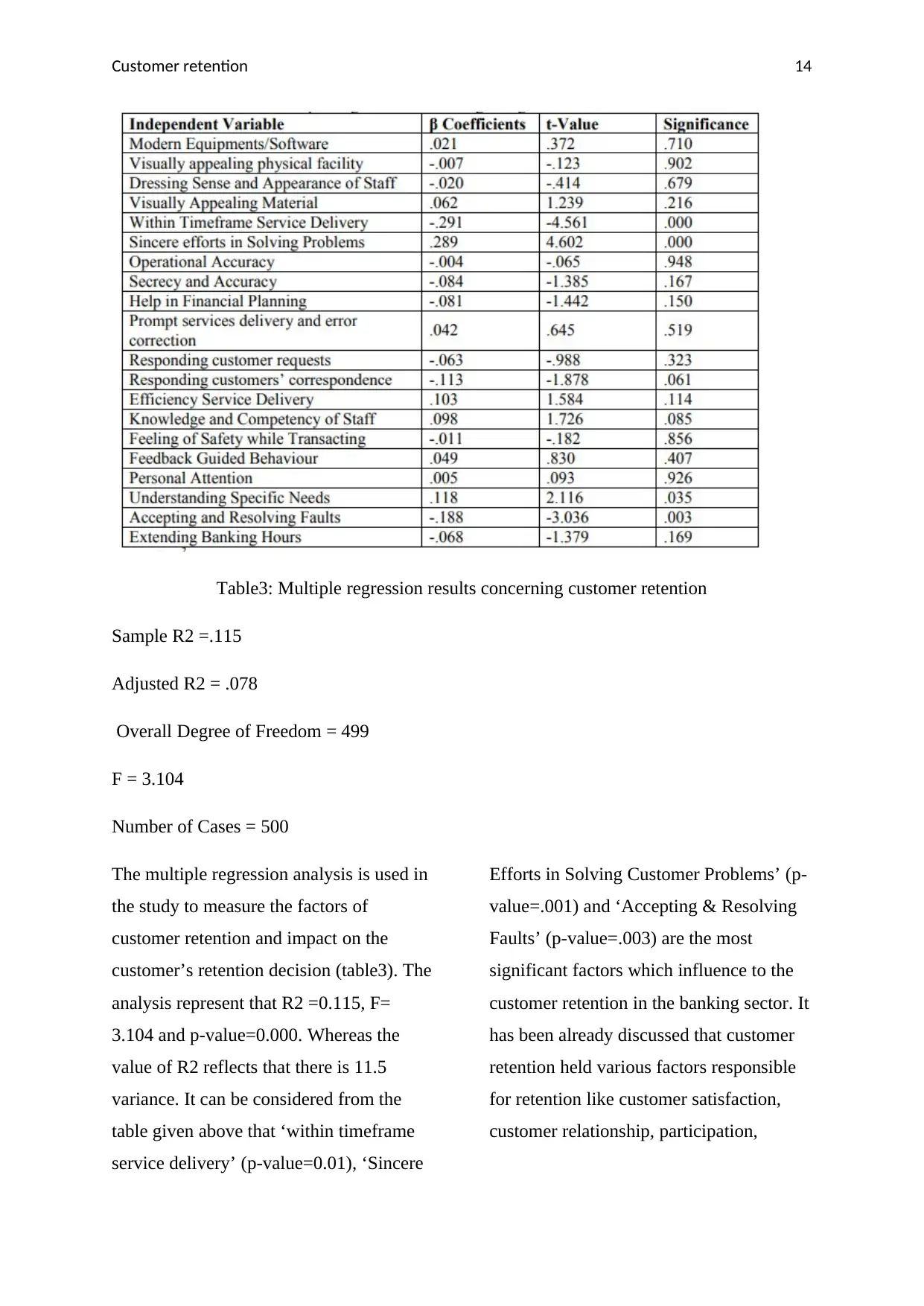
Customer retention 14
Table3: Multiple regression results concerning customer retention
Sample R2 =.115
Adjusted R2 = .078
Overall Degree of Freedom = 499
F = 3.104
Number of Cases = 500
The multiple regression analysis is used in
the study to measure the factors of
customer retention and impact on the
customer’s retention decision (table3). The
analysis represent that R2 =0.115, F=
3.104 and p-value=0.000. Whereas the
value of R2 reflects that there is 11.5
variance. It can be considered from the
table given above that ‘within timeframe
service delivery’ (p-value=0.01), ‘Sincere
Efforts in Solving Customer Problems’ (p-
value=.001) and ‘Accepting & Resolving
Faults’ (p-value=.003) are the most
significant factors which influence to the
customer retention in the banking sector. It
has been already discussed that customer
retention held various factors responsible
for retention like customer satisfaction,
customer relationship, participation,
Table3: Multiple regression results concerning customer retention
Sample R2 =.115
Adjusted R2 = .078
Overall Degree of Freedom = 499
F = 3.104
Number of Cases = 500
The multiple regression analysis is used in
the study to measure the factors of
customer retention and impact on the
customer’s retention decision (table3). The
analysis represent that R2 =0.115, F=
3.104 and p-value=0.000. Whereas the
value of R2 reflects that there is 11.5
variance. It can be considered from the
table given above that ‘within timeframe
service delivery’ (p-value=0.01), ‘Sincere
Efforts in Solving Customer Problems’ (p-
value=.001) and ‘Accepting & Resolving
Faults’ (p-value=.003) are the most
significant factors which influence to the
customer retention in the banking sector. It
has been already discussed that customer
retention held various factors responsible
for retention like customer satisfaction,
customer relationship, participation,
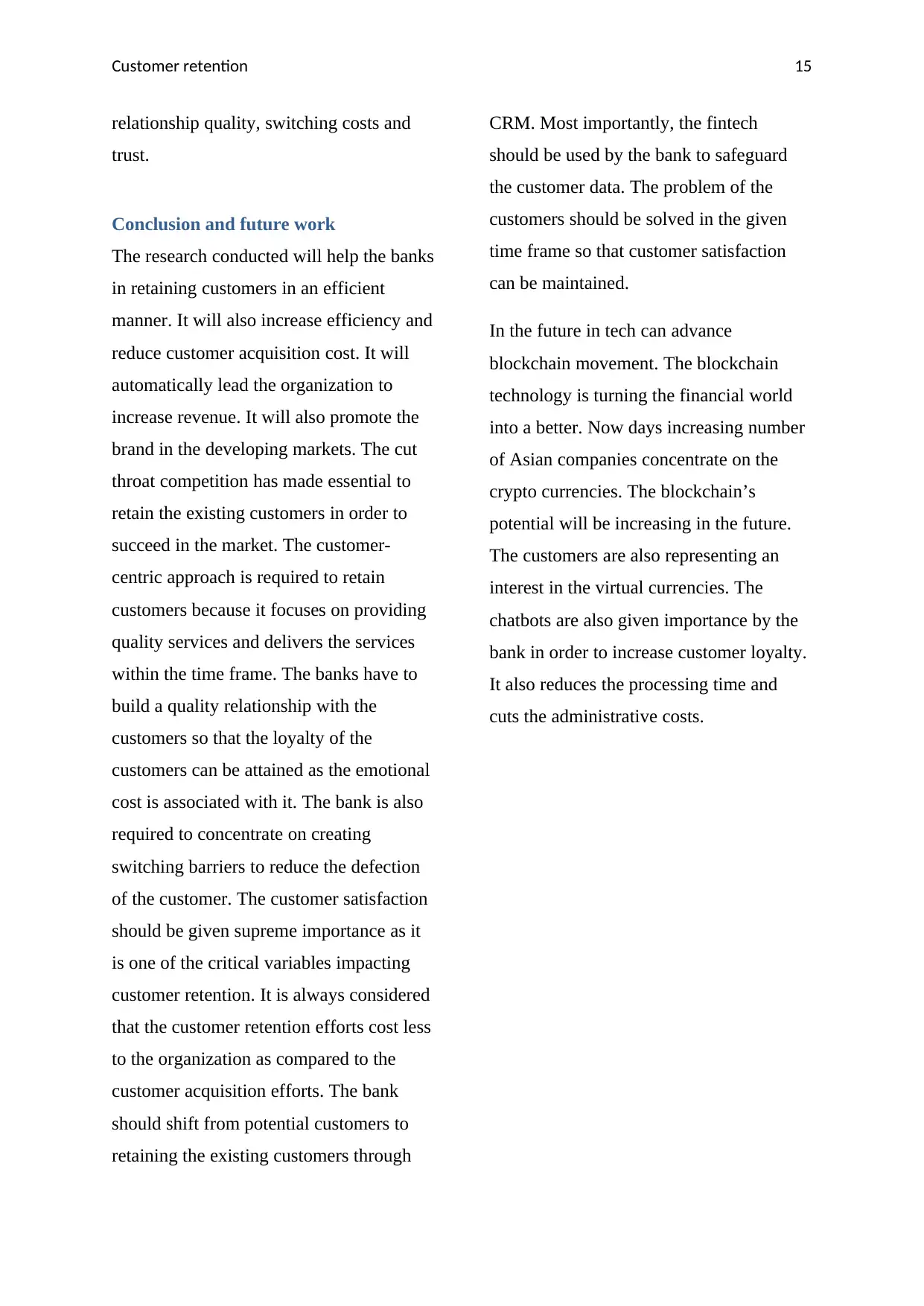
Customer retention 15
relationship quality, switching costs and
trust.
Conclusion and future work
The research conducted will help the banks
in retaining customers in an efficient
manner. It will also increase efficiency and
reduce customer acquisition cost. It will
automatically lead the organization to
increase revenue. It will also promote the
brand in the developing markets. The cut
throat competition has made essential to
retain the existing customers in order to
succeed in the market. The customer-
centric approach is required to retain
customers because it focuses on providing
quality services and delivers the services
within the time frame. The banks have to
build a quality relationship with the
customers so that the loyalty of the
customers can be attained as the emotional
cost is associated with it. The bank is also
required to concentrate on creating
switching barriers to reduce the defection
of the customer. The customer satisfaction
should be given supreme importance as it
is one of the critical variables impacting
customer retention. It is always considered
that the customer retention efforts cost less
to the organization as compared to the
customer acquisition efforts. The bank
should shift from potential customers to
retaining the existing customers through
CRM. Most importantly, the fintech
should be used by the bank to safeguard
the customer data. The problem of the
customers should be solved in the given
time frame so that customer satisfaction
can be maintained.
In the future in tech can advance
blockchain movement. The blockchain
technology is turning the financial world
into a better. Now days increasing number
of Asian companies concentrate on the
crypto currencies. The blockchain’s
potential will be increasing in the future.
The customers are also representing an
interest in the virtual currencies. The
chatbots are also given importance by the
bank in order to increase customer loyalty.
It also reduces the processing time and
cuts the administrative costs.
relationship quality, switching costs and
trust.
Conclusion and future work
The research conducted will help the banks
in retaining customers in an efficient
manner. It will also increase efficiency and
reduce customer acquisition cost. It will
automatically lead the organization to
increase revenue. It will also promote the
brand in the developing markets. The cut
throat competition has made essential to
retain the existing customers in order to
succeed in the market. The customer-
centric approach is required to retain
customers because it focuses on providing
quality services and delivers the services
within the time frame. The banks have to
build a quality relationship with the
customers so that the loyalty of the
customers can be attained as the emotional
cost is associated with it. The bank is also
required to concentrate on creating
switching barriers to reduce the defection
of the customer. The customer satisfaction
should be given supreme importance as it
is one of the critical variables impacting
customer retention. It is always considered
that the customer retention efforts cost less
to the organization as compared to the
customer acquisition efforts. The bank
should shift from potential customers to
retaining the existing customers through
CRM. Most importantly, the fintech
should be used by the bank to safeguard
the customer data. The problem of the
customers should be solved in the given
time frame so that customer satisfaction
can be maintained.
In the future in tech can advance
blockchain movement. The blockchain
technology is turning the financial world
into a better. Now days increasing number
of Asian companies concentrate on the
crypto currencies. The blockchain’s
potential will be increasing in the future.
The customers are also representing an
interest in the virtual currencies. The
chatbots are also given importance by the
bank in order to increase customer loyalty.
It also reduces the processing time and
cuts the administrative costs.
Secure Best Marks with AI Grader
Need help grading? Try our AI Grader for instant feedback on your assignments.
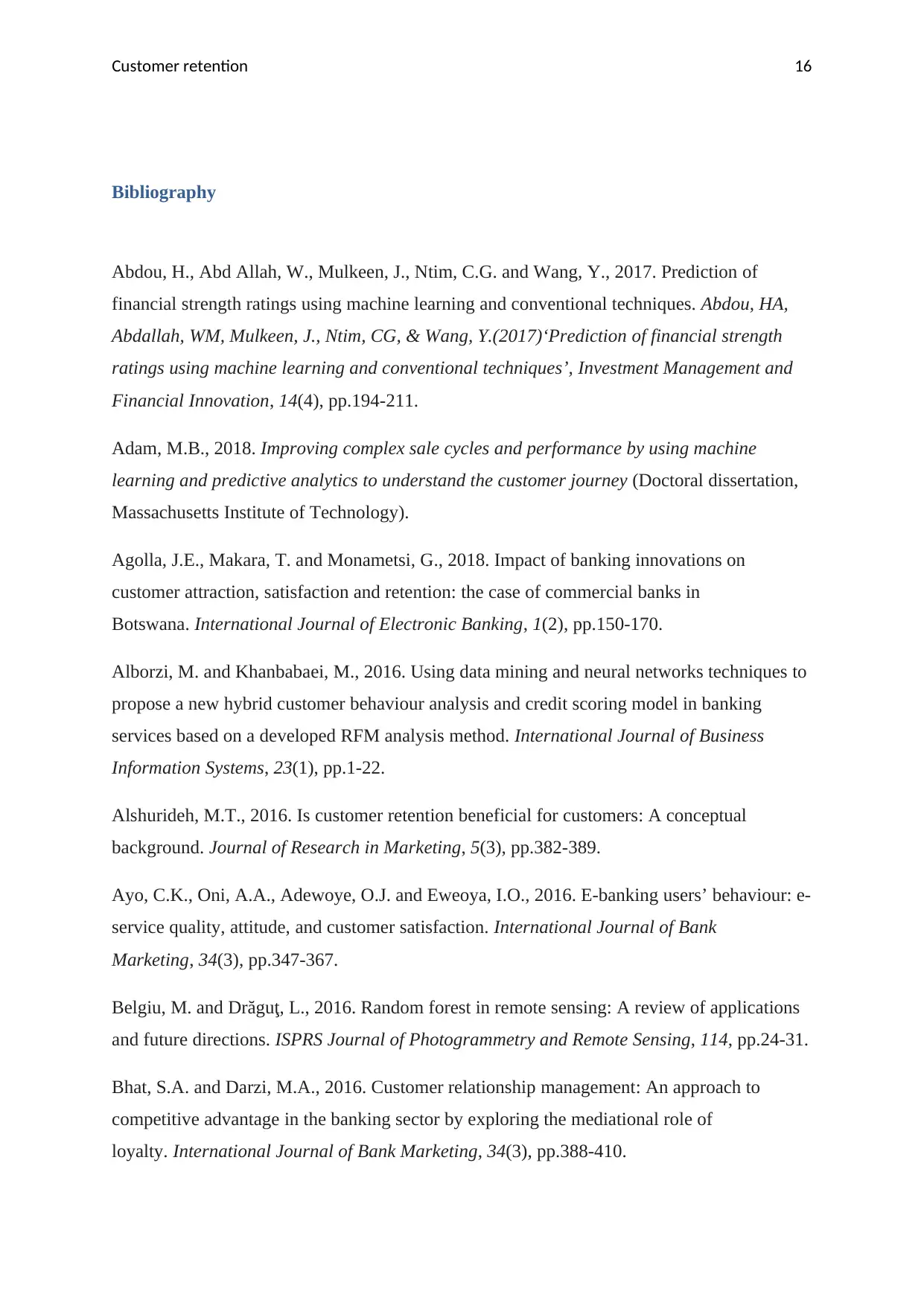
Customer retention 16
Bibliography
Abdou, H., Abd Allah, W., Mulkeen, J., Ntim, C.G. and Wang, Y., 2017. Prediction of
financial strength ratings using machine learning and conventional techniques. Abdou, HA,
Abdallah, WM, Mulkeen, J., Ntim, CG, & Wang, Y.(2017)‘Prediction of financial strength
ratings using machine learning and conventional techniques’, Investment Management and
Financial Innovation, 14(4), pp.194-211.
Adam, M.B., 2018. Improving complex sale cycles and performance by using machine
learning and predictive analytics to understand the customer journey (Doctoral dissertation,
Massachusetts Institute of Technology).
Agolla, J.E., Makara, T. and Monametsi, G., 2018. Impact of banking innovations on
customer attraction, satisfaction and retention: the case of commercial banks in
Botswana. International Journal of Electronic Banking, 1(2), pp.150-170.
Alborzi, M. and Khanbabaei, M., 2016. Using data mining and neural networks techniques to
propose a new hybrid customer behaviour analysis and credit scoring model in banking
services based on a developed RFM analysis method. International Journal of Business
Information Systems, 23(1), pp.1-22.
Alshurideh, M.T., 2016. Is customer retention beneficial for customers: A conceptual
background. Journal of Research in Marketing, 5(3), pp.382-389.
Ayo, C.K., Oni, A.A., Adewoye, O.J. and Eweoya, I.O., 2016. E-banking users’ behaviour: e-
service quality, attitude, and customer satisfaction. International Journal of Bank
Marketing, 34(3), pp.347-367.
Belgiu, M. and Drăguţ, L., 2016. Random forest in remote sensing: A review of applications
and future directions. ISPRS Journal of Photogrammetry and Remote Sensing, 114, pp.24-31.
Bhat, S.A. and Darzi, M.A., 2016. Customer relationship management: An approach to
competitive advantage in the banking sector by exploring the mediational role of
loyalty. International Journal of Bank Marketing, 34(3), pp.388-410.
Bibliography
Abdou, H., Abd Allah, W., Mulkeen, J., Ntim, C.G. and Wang, Y., 2017. Prediction of
financial strength ratings using machine learning and conventional techniques. Abdou, HA,
Abdallah, WM, Mulkeen, J., Ntim, CG, & Wang, Y.(2017)‘Prediction of financial strength
ratings using machine learning and conventional techniques’, Investment Management and
Financial Innovation, 14(4), pp.194-211.
Adam, M.B., 2018. Improving complex sale cycles and performance by using machine
learning and predictive analytics to understand the customer journey (Doctoral dissertation,
Massachusetts Institute of Technology).
Agolla, J.E., Makara, T. and Monametsi, G., 2018. Impact of banking innovations on
customer attraction, satisfaction and retention: the case of commercial banks in
Botswana. International Journal of Electronic Banking, 1(2), pp.150-170.
Alborzi, M. and Khanbabaei, M., 2016. Using data mining and neural networks techniques to
propose a new hybrid customer behaviour analysis and credit scoring model in banking
services based on a developed RFM analysis method. International Journal of Business
Information Systems, 23(1), pp.1-22.
Alshurideh, M.T., 2016. Is customer retention beneficial for customers: A conceptual
background. Journal of Research in Marketing, 5(3), pp.382-389.
Ayo, C.K., Oni, A.A., Adewoye, O.J. and Eweoya, I.O., 2016. E-banking users’ behaviour: e-
service quality, attitude, and customer satisfaction. International Journal of Bank
Marketing, 34(3), pp.347-367.
Belgiu, M. and Drăguţ, L., 2016. Random forest in remote sensing: A review of applications
and future directions. ISPRS Journal of Photogrammetry and Remote Sensing, 114, pp.24-31.
Bhat, S.A. and Darzi, M.A., 2016. Customer relationship management: An approach to
competitive advantage in the banking sector by exploring the mediational role of
loyalty. International Journal of Bank Marketing, 34(3), pp.388-410.
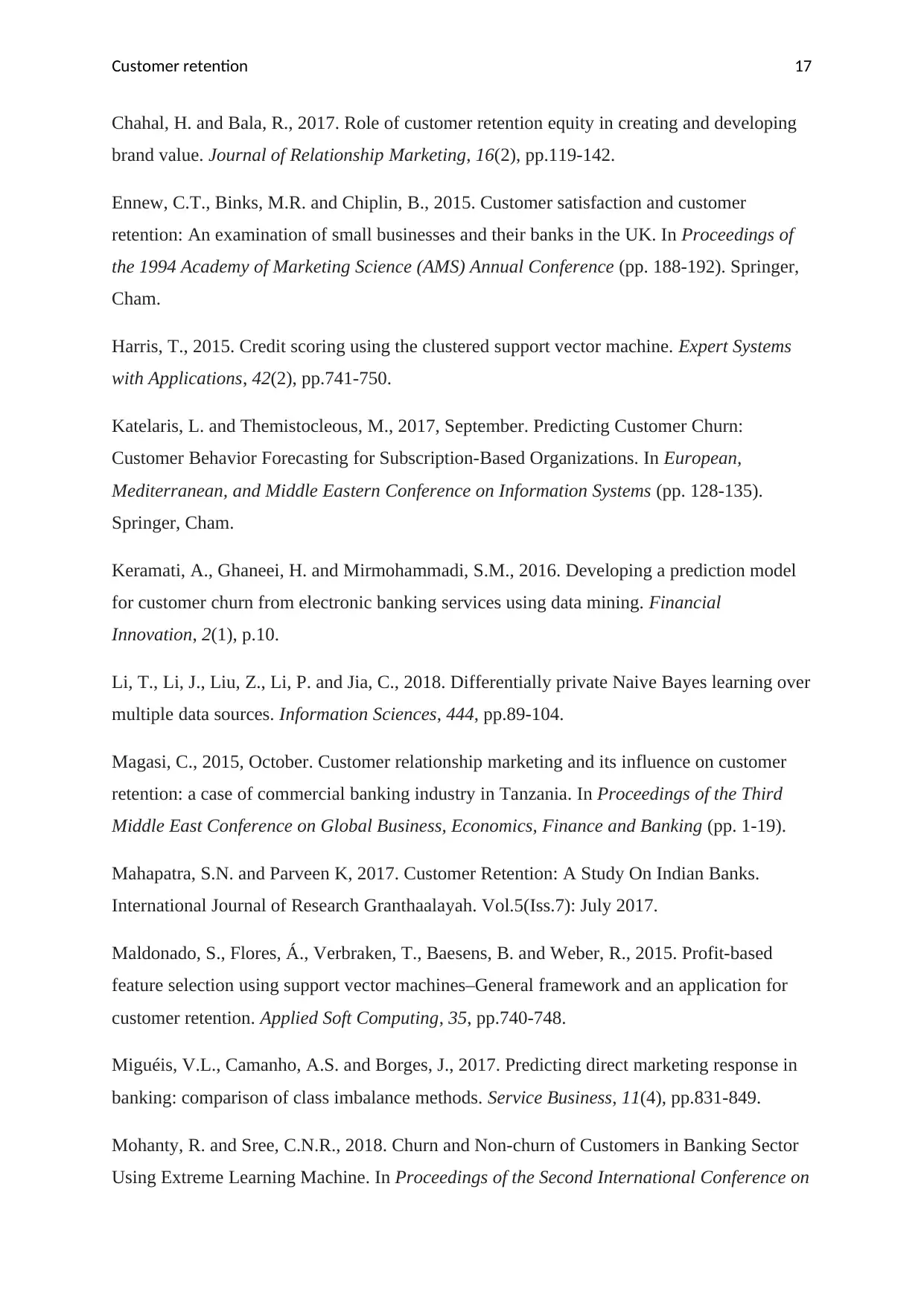
Customer retention 17
Chahal, H. and Bala, R., 2017. Role of customer retention equity in creating and developing
brand value. Journal of Relationship Marketing, 16(2), pp.119-142.
Ennew, C.T., Binks, M.R. and Chiplin, B., 2015. Customer satisfaction and customer
retention: An examination of small businesses and their banks in the UK. In Proceedings of
the 1994 Academy of Marketing Science (AMS) Annual Conference (pp. 188-192). Springer,
Cham.
Harris, T., 2015. Credit scoring using the clustered support vector machine. Expert Systems
with Applications, 42(2), pp.741-750.
Katelaris, L. and Themistocleous, M., 2017, September. Predicting Customer Churn:
Customer Behavior Forecasting for Subscription-Based Organizations. In European,
Mediterranean, and Middle Eastern Conference on Information Systems (pp. 128-135).
Springer, Cham.
Keramati, A., Ghaneei, H. and Mirmohammadi, S.M., 2016. Developing a prediction model
for customer churn from electronic banking services using data mining. Financial
Innovation, 2(1), p.10.
Li, T., Li, J., Liu, Z., Li, P. and Jia, C., 2018. Differentially private Naive Bayes learning over
multiple data sources. Information Sciences, 444, pp.89-104.
Magasi, C., 2015, October. Customer relationship marketing and its influence on customer
retention: a case of commercial banking industry in Tanzania. In Proceedings of the Third
Middle East Conference on Global Business, Economics, Finance and Banking (pp. 1-19).
Mahapatra, S.N. and Parveen K, 2017. Customer Retention: A Study On Indian Banks.
International Journal of Research Granthaalayah. Vol.5(Iss.7): July 2017.
Maldonado, S., Flores, Á., Verbraken, T., Baesens, B. and Weber, R., 2015. Profit-based
feature selection using support vector machines–General framework and an application for
customer retention. Applied Soft Computing, 35, pp.740-748.
Miguéis, V.L., Camanho, A.S. and Borges, J., 2017. Predicting direct marketing response in
banking: comparison of class imbalance methods. Service Business, 11(4), pp.831-849.
Mohanty, R. and Sree, C.N.R., 2018. Churn and Non-churn of Customers in Banking Sector
Using Extreme Learning Machine. In Proceedings of the Second International Conference on
Chahal, H. and Bala, R., 2017. Role of customer retention equity in creating and developing
brand value. Journal of Relationship Marketing, 16(2), pp.119-142.
Ennew, C.T., Binks, M.R. and Chiplin, B., 2015. Customer satisfaction and customer
retention: An examination of small businesses and their banks in the UK. In Proceedings of
the 1994 Academy of Marketing Science (AMS) Annual Conference (pp. 188-192). Springer,
Cham.
Harris, T., 2015. Credit scoring using the clustered support vector machine. Expert Systems
with Applications, 42(2), pp.741-750.
Katelaris, L. and Themistocleous, M., 2017, September. Predicting Customer Churn:
Customer Behavior Forecasting for Subscription-Based Organizations. In European,
Mediterranean, and Middle Eastern Conference on Information Systems (pp. 128-135).
Springer, Cham.
Keramati, A., Ghaneei, H. and Mirmohammadi, S.M., 2016. Developing a prediction model
for customer churn from electronic banking services using data mining. Financial
Innovation, 2(1), p.10.
Li, T., Li, J., Liu, Z., Li, P. and Jia, C., 2018. Differentially private Naive Bayes learning over
multiple data sources. Information Sciences, 444, pp.89-104.
Magasi, C., 2015, October. Customer relationship marketing and its influence on customer
retention: a case of commercial banking industry in Tanzania. In Proceedings of the Third
Middle East Conference on Global Business, Economics, Finance and Banking (pp. 1-19).
Mahapatra, S.N. and Parveen K, 2017. Customer Retention: A Study On Indian Banks.
International Journal of Research Granthaalayah. Vol.5(Iss.7): July 2017.
Maldonado, S., Flores, Á., Verbraken, T., Baesens, B. and Weber, R., 2015. Profit-based
feature selection using support vector machines–General framework and an application for
customer retention. Applied Soft Computing, 35, pp.740-748.
Miguéis, V.L., Camanho, A.S. and Borges, J., 2017. Predicting direct marketing response in
banking: comparison of class imbalance methods. Service Business, 11(4), pp.831-849.
Mohanty, R. and Sree, C.N.R., 2018. Churn and Non-churn of Customers in Banking Sector
Using Extreme Learning Machine. In Proceedings of the Second International Conference on
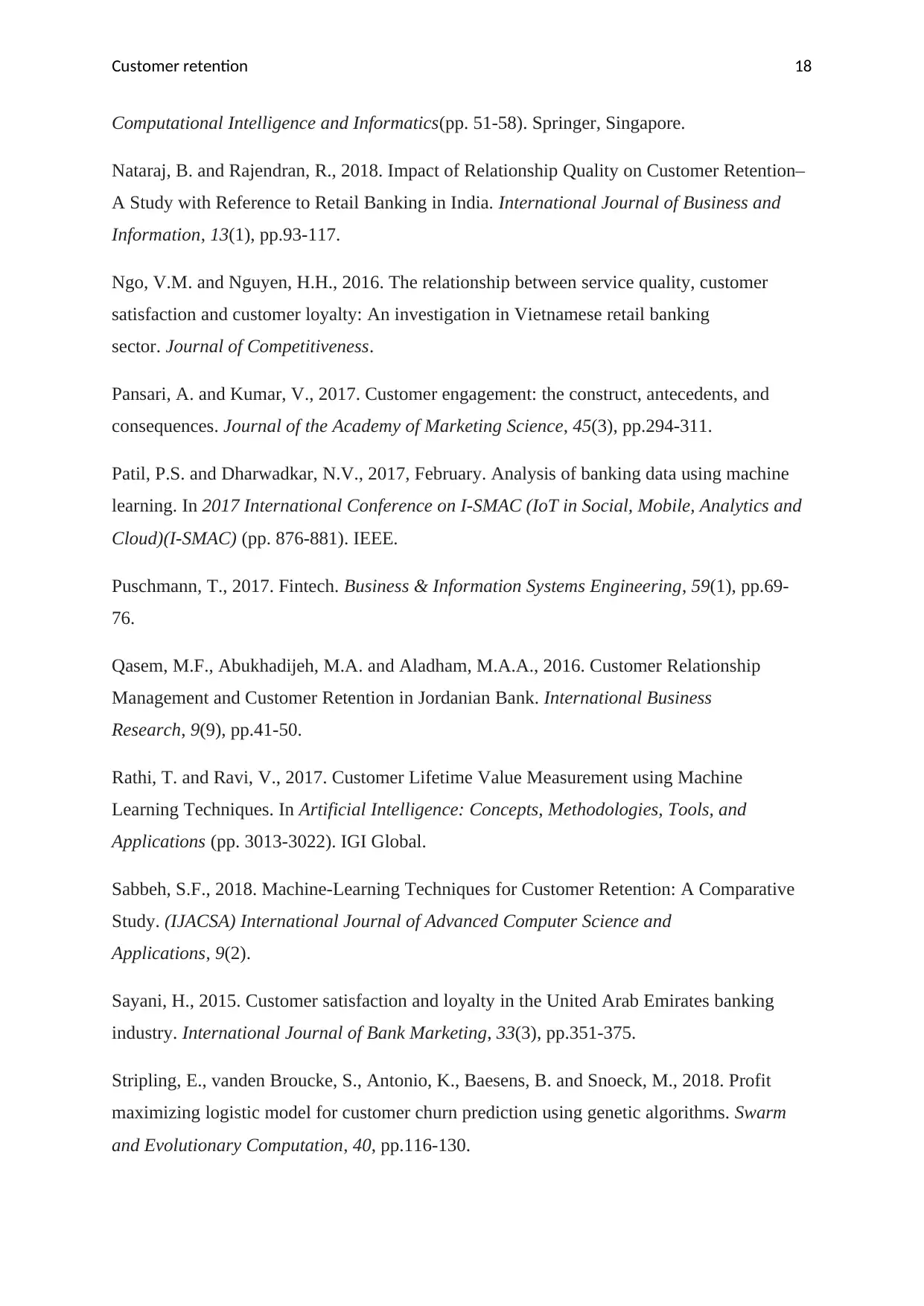
Customer retention 18
Computational Intelligence and Informatics(pp. 51-58). Springer, Singapore.
Nataraj, B. and Rajendran, R., 2018. Impact of Relationship Quality on Customer Retention–
A Study with Reference to Retail Banking in India. International Journal of Business and
Information, 13(1), pp.93-117.
Ngo, V.M. and Nguyen, H.H., 2016. The relationship between service quality, customer
satisfaction and customer loyalty: An investigation in Vietnamese retail banking
sector. Journal of Competitiveness.
Pansari, A. and Kumar, V., 2017. Customer engagement: the construct, antecedents, and
consequences. Journal of the Academy of Marketing Science, 45(3), pp.294-311.
Patil, P.S. and Dharwadkar, N.V., 2017, February. Analysis of banking data using machine
learning. In 2017 International Conference on I-SMAC (IoT in Social, Mobile, Analytics and
Cloud)(I-SMAC) (pp. 876-881). IEEE.
Puschmann, T., 2017. Fintech. Business & Information Systems Engineering, 59(1), pp.69-
76.
Qasem, M.F., Abukhadijeh, M.A. and Aladham, M.A.A., 2016. Customer Relationship
Management and Customer Retention in Jordanian Bank. International Business
Research, 9(9), pp.41-50.
Rathi, T. and Ravi, V., 2017. Customer Lifetime Value Measurement using Machine
Learning Techniques. In Artificial Intelligence: Concepts, Methodologies, Tools, and
Applications (pp. 3013-3022). IGI Global.
Sabbeh, S.F., 2018. Machine-Learning Techniques for Customer Retention: A Comparative
Study. (IJACSA) International Journal of Advanced Computer Science and
Applications, 9(2).
Sayani, H., 2015. Customer satisfaction and loyalty in the United Arab Emirates banking
industry. International Journal of Bank Marketing, 33(3), pp.351-375.
Stripling, E., vanden Broucke, S., Antonio, K., Baesens, B. and Snoeck, M., 2018. Profit
maximizing logistic model for customer churn prediction using genetic algorithms. Swarm
and Evolutionary Computation, 40, pp.116-130.
Computational Intelligence and Informatics(pp. 51-58). Springer, Singapore.
Nataraj, B. and Rajendran, R., 2018. Impact of Relationship Quality on Customer Retention–
A Study with Reference to Retail Banking in India. International Journal of Business and
Information, 13(1), pp.93-117.
Ngo, V.M. and Nguyen, H.H., 2016. The relationship between service quality, customer
satisfaction and customer loyalty: An investigation in Vietnamese retail banking
sector. Journal of Competitiveness.
Pansari, A. and Kumar, V., 2017. Customer engagement: the construct, antecedents, and
consequences. Journal of the Academy of Marketing Science, 45(3), pp.294-311.
Patil, P.S. and Dharwadkar, N.V., 2017, February. Analysis of banking data using machine
learning. In 2017 International Conference on I-SMAC (IoT in Social, Mobile, Analytics and
Cloud)(I-SMAC) (pp. 876-881). IEEE.
Puschmann, T., 2017. Fintech. Business & Information Systems Engineering, 59(1), pp.69-
76.
Qasem, M.F., Abukhadijeh, M.A. and Aladham, M.A.A., 2016. Customer Relationship
Management and Customer Retention in Jordanian Bank. International Business
Research, 9(9), pp.41-50.
Rathi, T. and Ravi, V., 2017. Customer Lifetime Value Measurement using Machine
Learning Techniques. In Artificial Intelligence: Concepts, Methodologies, Tools, and
Applications (pp. 3013-3022). IGI Global.
Sabbeh, S.F., 2018. Machine-Learning Techniques for Customer Retention: A Comparative
Study. (IJACSA) International Journal of Advanced Computer Science and
Applications, 9(2).
Sayani, H., 2015. Customer satisfaction and loyalty in the United Arab Emirates banking
industry. International Journal of Bank Marketing, 33(3), pp.351-375.
Stripling, E., vanden Broucke, S., Antonio, K., Baesens, B. and Snoeck, M., 2018. Profit
maximizing logistic model for customer churn prediction using genetic algorithms. Swarm
and Evolutionary Computation, 40, pp.116-130.
Paraphrase This Document
Need a fresh take? Get an instant paraphrase of this document with our AI Paraphraser
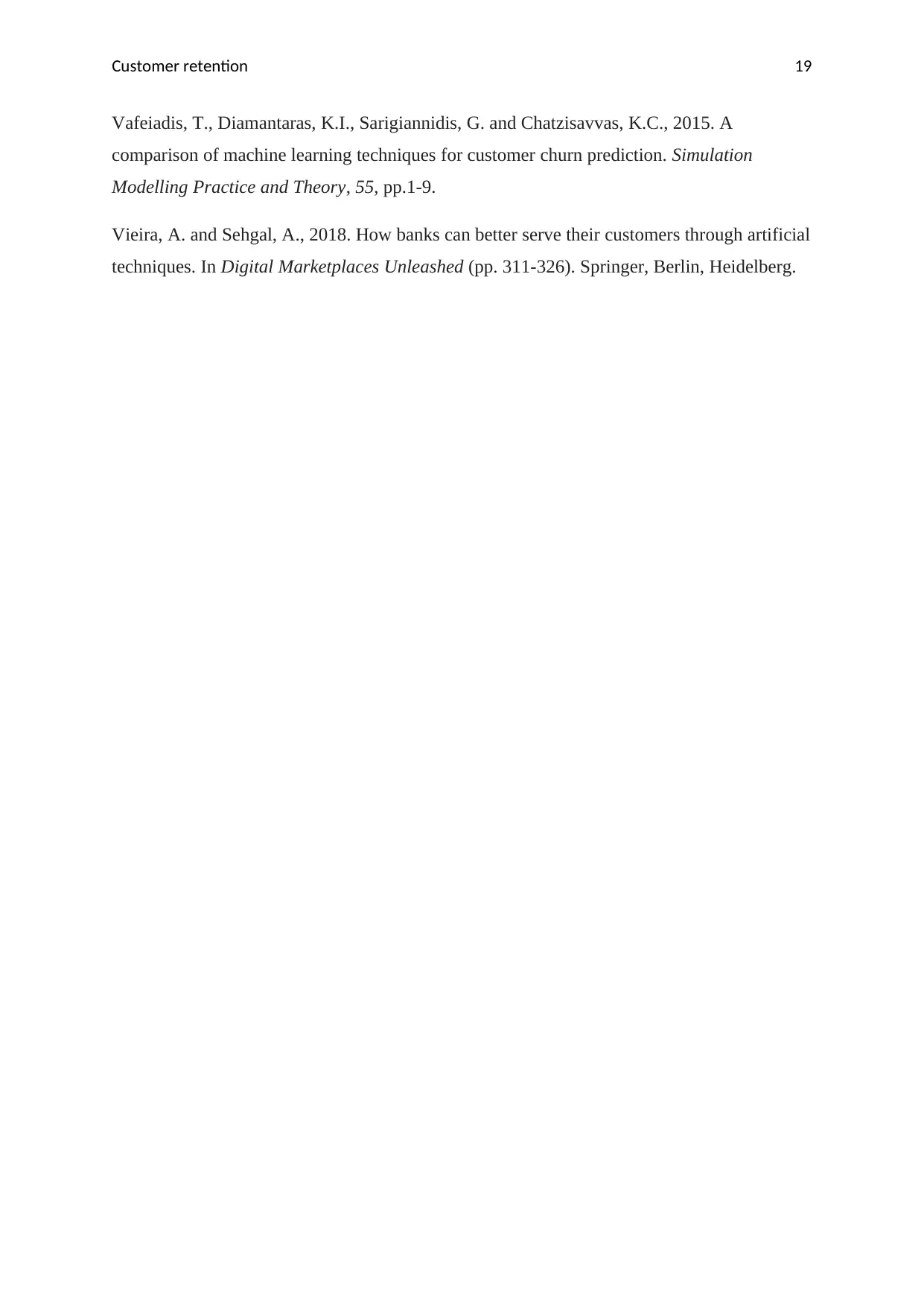
Customer retention 19
Vafeiadis, T., Diamantaras, K.I., Sarigiannidis, G. and Chatzisavvas, K.C., 2015. A
comparison of machine learning techniques for customer churn prediction. Simulation
Modelling Practice and Theory, 55, pp.1-9.
Vieira, A. and Sehgal, A., 2018. How banks can better serve their customers through artificial
techniques. In Digital Marketplaces Unleashed (pp. 311-326). Springer, Berlin, Heidelberg.
Vafeiadis, T., Diamantaras, K.I., Sarigiannidis, G. and Chatzisavvas, K.C., 2015. A
comparison of machine learning techniques for customer churn prediction. Simulation
Modelling Practice and Theory, 55, pp.1-9.
Vieira, A. and Sehgal, A., 2018. How banks can better serve their customers through artificial
techniques. In Digital Marketplaces Unleashed (pp. 311-326). Springer, Berlin, Heidelberg.
1 out of 20
Related Documents
![[object Object]](/_next/image/?url=%2F_next%2Fstatic%2Fmedia%2Flogo.6d15ce61.png&w=640&q=75)
Your All-in-One AI-Powered Toolkit for Academic Success.
+13062052269
info@desklib.com
Available 24*7 on WhatsApp / Email
Unlock your academic potential
© 2024 | Zucol Services PVT LTD | All rights reserved.