Accounting Decision Support Tools
VerifiedAdded on 2023/04/08
|10
|1397
|275
AI Summary
This document discusses various topics related to accounting decision support tools. It covers concepts such as expected value, sales of loaves, four machines, average sales of apples, control limits, and hypothesis testing. The document provides explanations, calculations, and examples for each topic.
Contribute Materials
Your contribution can guide someone’s learning journey. Share your
documents today.
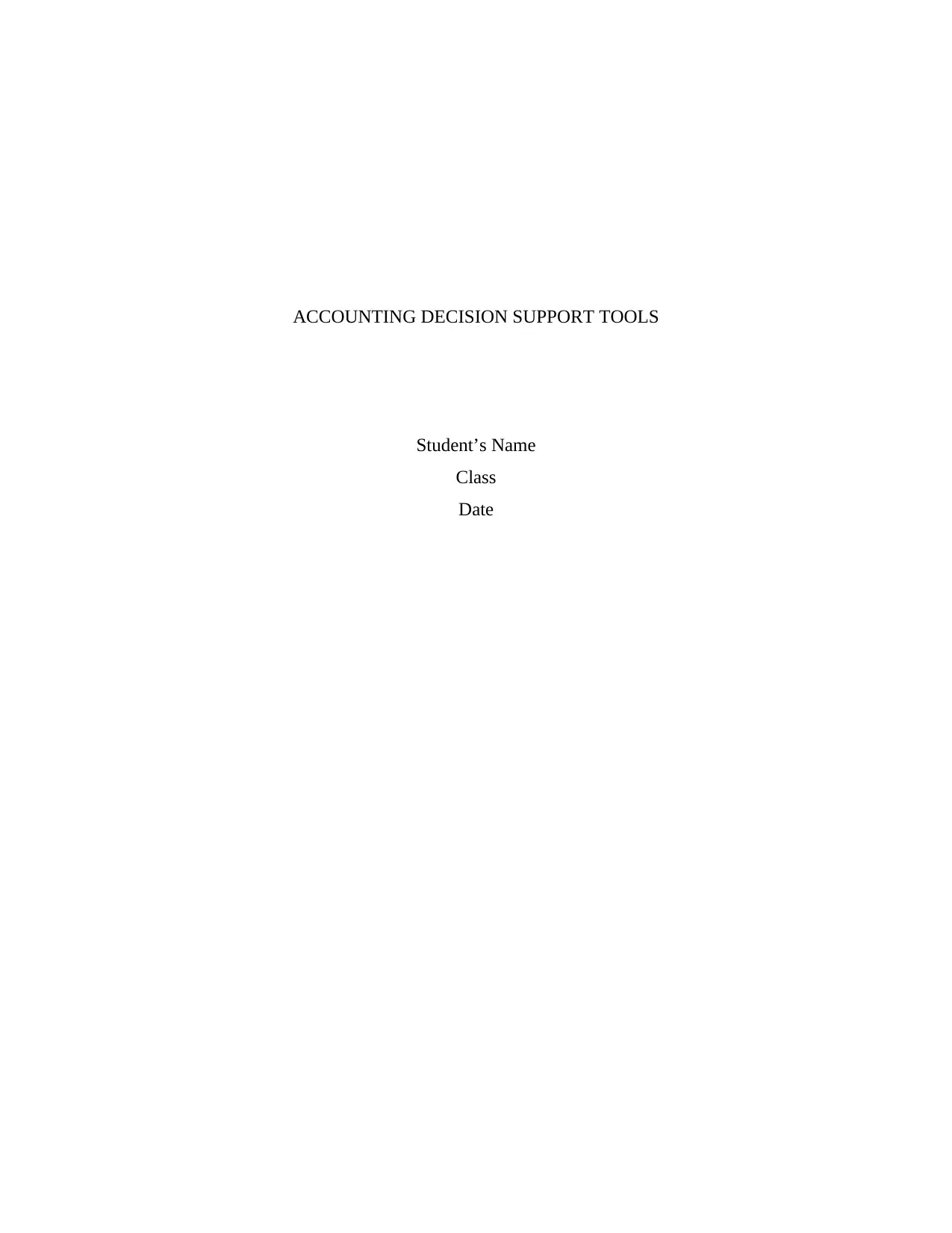
ACCOUNTING DECISION SUPPORT TOOLS
Student’s Name
Class
Date
Student’s Name
Class
Date
Secure Best Marks with AI Grader
Need help grading? Try our AI Grader for instant feedback on your assignments.
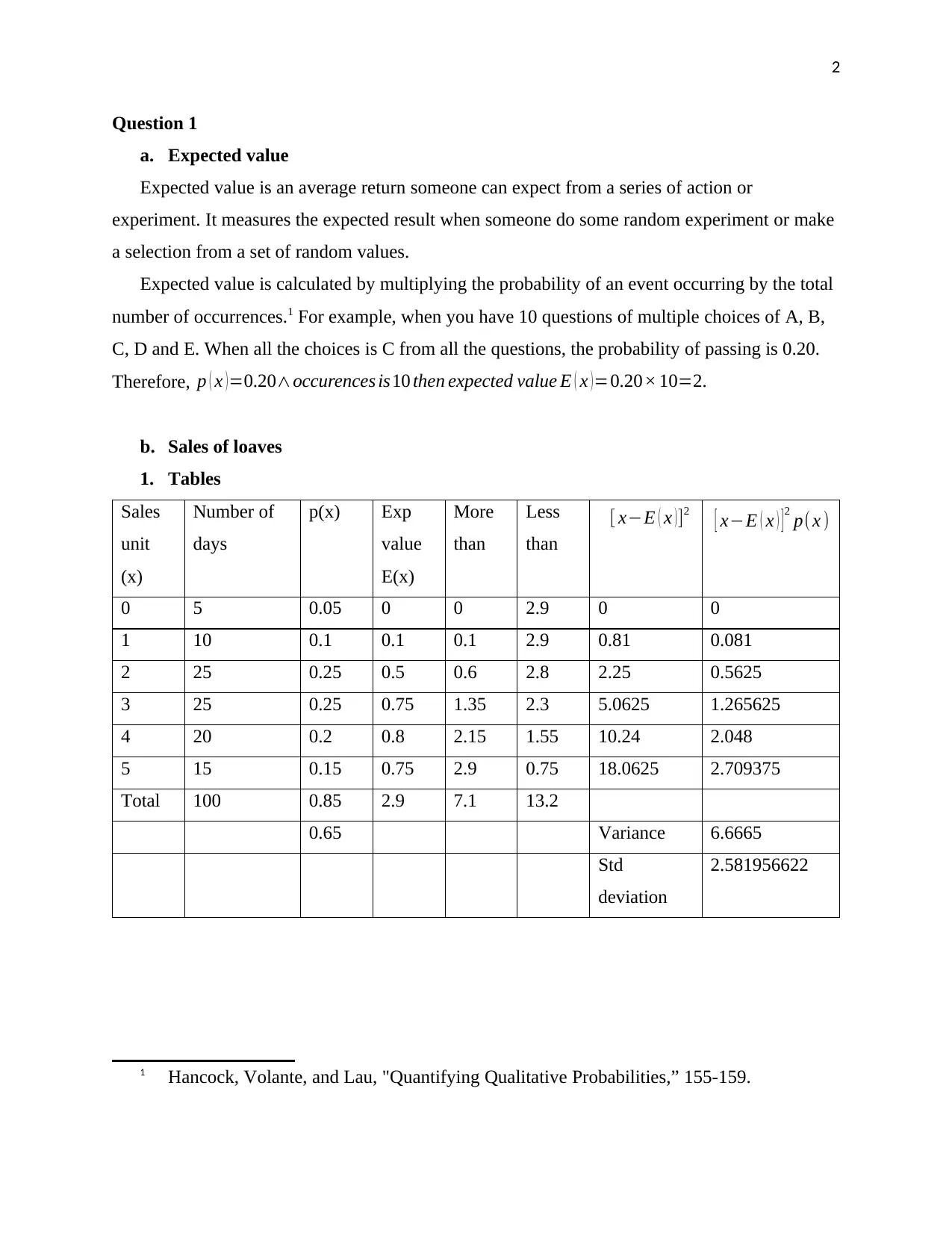
2
Question 1
a. Expected value
Expected value is an average return someone can expect from a series of action or
experiment. It measures the expected result when someone do some random experiment or make
a selection from a set of random values.
Expected value is calculated by multiplying the probability of an event occurring by the total
number of occurrences.1 For example, when you have 10 questions of multiple choices of A, B,
C, D and E. When all the choices is C from all the questions, the probability of passing is 0.20.
Therefore, p ( x )=0.20∧occurences is10 then expected value E ( x )=0.20× 10=2.
b. Sales of loaves
1. Tables
Sales
unit
(x)
Number of
days
p(x) Exp
value
E(x)
More
than
Less
than
0 5 0.05 0 0 2.9 0 0
1 10 0.1 0.1 0.1 2.9 0.81 0.081
2 25 0.25 0.5 0.6 2.8 2.25 0.5625
3 25 0.25 0.75 1.35 2.3 5.0625 1.265625
4 20 0.2 0.8 2.15 1.55 10.24 2.048
5 15 0.15 0.75 2.9 0.75 18.0625 2.709375
Total 100 0.85 2.9 7.1 13.2
0.65 Variance 6.6665
Std
deviation
2.581956622
1 Hancock, Volante, and Lau, "Quantifying Qualitative Probabilities,” 155-159.
[ x−E ( x ) ]2
[ x−E ( x ) ]2
p( x )
Question 1
a. Expected value
Expected value is an average return someone can expect from a series of action or
experiment. It measures the expected result when someone do some random experiment or make
a selection from a set of random values.
Expected value is calculated by multiplying the probability of an event occurring by the total
number of occurrences.1 For example, when you have 10 questions of multiple choices of A, B,
C, D and E. When all the choices is C from all the questions, the probability of passing is 0.20.
Therefore, p ( x )=0.20∧occurences is10 then expected value E ( x )=0.20× 10=2.
b. Sales of loaves
1. Tables
Sales
unit
(x)
Number of
days
p(x) Exp
value
E(x)
More
than
Less
than
0 5 0.05 0 0 2.9 0 0
1 10 0.1 0.1 0.1 2.9 0.81 0.081
2 25 0.25 0.5 0.6 2.8 2.25 0.5625
3 25 0.25 0.75 1.35 2.3 5.0625 1.265625
4 20 0.2 0.8 2.15 1.55 10.24 2.048
5 15 0.15 0.75 2.9 0.75 18.0625 2.709375
Total 100 0.85 2.9 7.1 13.2
0.65 Variance 6.6665
Std
deviation
2.581956622
1 Hancock, Volante, and Lau, "Quantifying Qualitative Probabilities,” 155-159.
[ x−E ( x ) ]2
[ x−E ( x ) ]2
p( x )
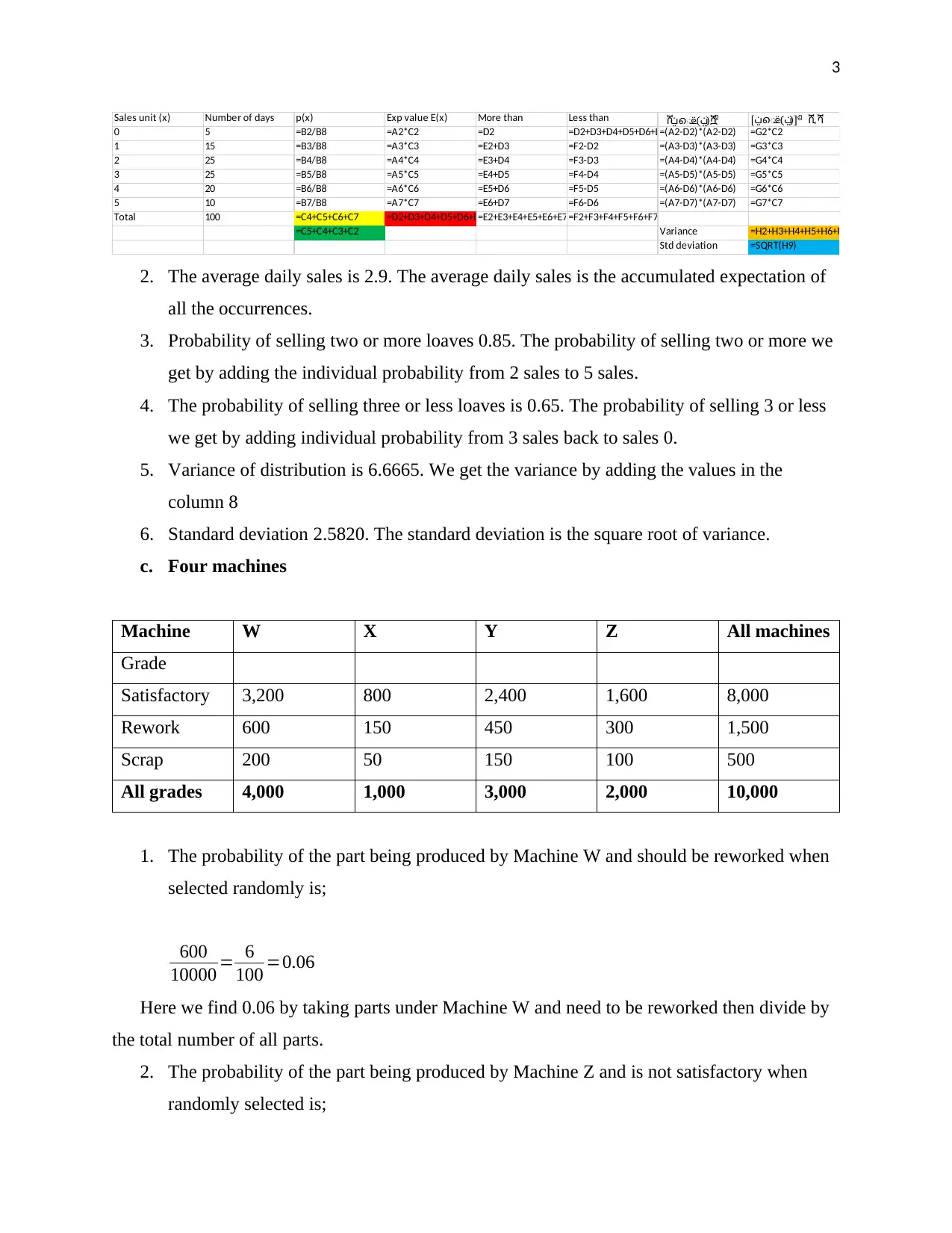
3
Sales unit (x) Number of days p(x) Exp value E(x) More than Less than
0 5 =B2/B8 =A2*C2 =D2 =D2+D3+D4+D5+D6+D7=(A2-D2)*(A2-D2) =G2*C2
1 15 =B3/B8 =A3*C3 =E2+D3 =F2-D2 =(A3-D3)*(A3-D3) =G3*C3
2 25 =B4/B8 =A4*C4 =E3+D4 =F3-D3 =(A4-D4)*(A4-D4) =G4*C4
3 25 =B5/B8 =A5*C5 =E4+D5 =F4-D4 =(A5-D5)*(A5-D5) =G5*C5
4 20 =B6/B8 =A6*C6 =E5+D6 =F5-D5 =(A6-D6)*(A6-D6) =G6*C6
5 10 =B7/B8 =A7*C7 =E6+D7 =F6-D6 =(A7-D7)*(A7-D7) =G7*C7
Total 100 =C4+C5+C6+C7 =D2+D3+D4+D5+D6+D7=E2+E3+E4+E5+E6+E7=F2+F3+F4+F5+F6+F7
=C5+C4+C3+C2 Variance =H2+H3+H4+H5+H6+H7
Std deviation =SQRT(H9)
ሾݔെܧ ݔ ሿଶ ݔെܧ ݔ ଶ ሺ ሻ
2. The average daily sales is 2.9. The average daily sales is the accumulated expectation of
all the occurrences.
3. Probability of selling two or more loaves 0.85. The probability of selling two or more we
get by adding the individual probability from 2 sales to 5 sales.
4. The probability of selling three or less loaves is 0.65. The probability of selling 3 or less
we get by adding individual probability from 3 sales back to sales 0.
5. Variance of distribution is 6.6665. We get the variance by adding the values in the
column 8
6. Standard deviation 2.5820. The standard deviation is the square root of variance.
c. Four machines
Machine W X Y Z All machines
Grade
Satisfactory 3,200 800 2,400 1,600 8,000
Rework 600 150 450 300 1,500
Scrap 200 50 150 100 500
All grades 4,000 1,000 3,000 2,000 10,000
1. The probability of the part being produced by Machine W and should be reworked when
selected randomly is;
600
10000 = 6
100 =0.06
Here we find 0.06 by taking parts under Machine W and need to be reworked then divide by
the total number of all parts.
2. The probability of the part being produced by Machine Z and is not satisfactory when
randomly selected is;
Sales unit (x) Number of days p(x) Exp value E(x) More than Less than
0 5 =B2/B8 =A2*C2 =D2 =D2+D3+D4+D5+D6+D7=(A2-D2)*(A2-D2) =G2*C2
1 15 =B3/B8 =A3*C3 =E2+D3 =F2-D2 =(A3-D3)*(A3-D3) =G3*C3
2 25 =B4/B8 =A4*C4 =E3+D4 =F3-D3 =(A4-D4)*(A4-D4) =G4*C4
3 25 =B5/B8 =A5*C5 =E4+D5 =F4-D4 =(A5-D5)*(A5-D5) =G5*C5
4 20 =B6/B8 =A6*C6 =E5+D6 =F5-D5 =(A6-D6)*(A6-D6) =G6*C6
5 10 =B7/B8 =A7*C7 =E6+D7 =F6-D6 =(A7-D7)*(A7-D7) =G7*C7
Total 100 =C4+C5+C6+C7 =D2+D3+D4+D5+D6+D7=E2+E3+E4+E5+E6+E7=F2+F3+F4+F5+F6+F7
=C5+C4+C3+C2 Variance =H2+H3+H4+H5+H6+H7
Std deviation =SQRT(H9)
ሾݔെܧ ݔ ሿଶ ݔെܧ ݔ ଶ ሺ ሻ
2. The average daily sales is 2.9. The average daily sales is the accumulated expectation of
all the occurrences.
3. Probability of selling two or more loaves 0.85. The probability of selling two or more we
get by adding the individual probability from 2 sales to 5 sales.
4. The probability of selling three or less loaves is 0.65. The probability of selling 3 or less
we get by adding individual probability from 3 sales back to sales 0.
5. Variance of distribution is 6.6665. We get the variance by adding the values in the
column 8
6. Standard deviation 2.5820. The standard deviation is the square root of variance.
c. Four machines
Machine W X Y Z All machines
Grade
Satisfactory 3,200 800 2,400 1,600 8,000
Rework 600 150 450 300 1,500
Scrap 200 50 150 100 500
All grades 4,000 1,000 3,000 2,000 10,000
1. The probability of the part being produced by Machine W and should be reworked when
selected randomly is;
600
10000 = 6
100 =0.06
Here we find 0.06 by taking parts under Machine W and need to be reworked then divide by
the total number of all parts.
2. The probability of the part being produced by Machine Z and is not satisfactory when
randomly selected is;
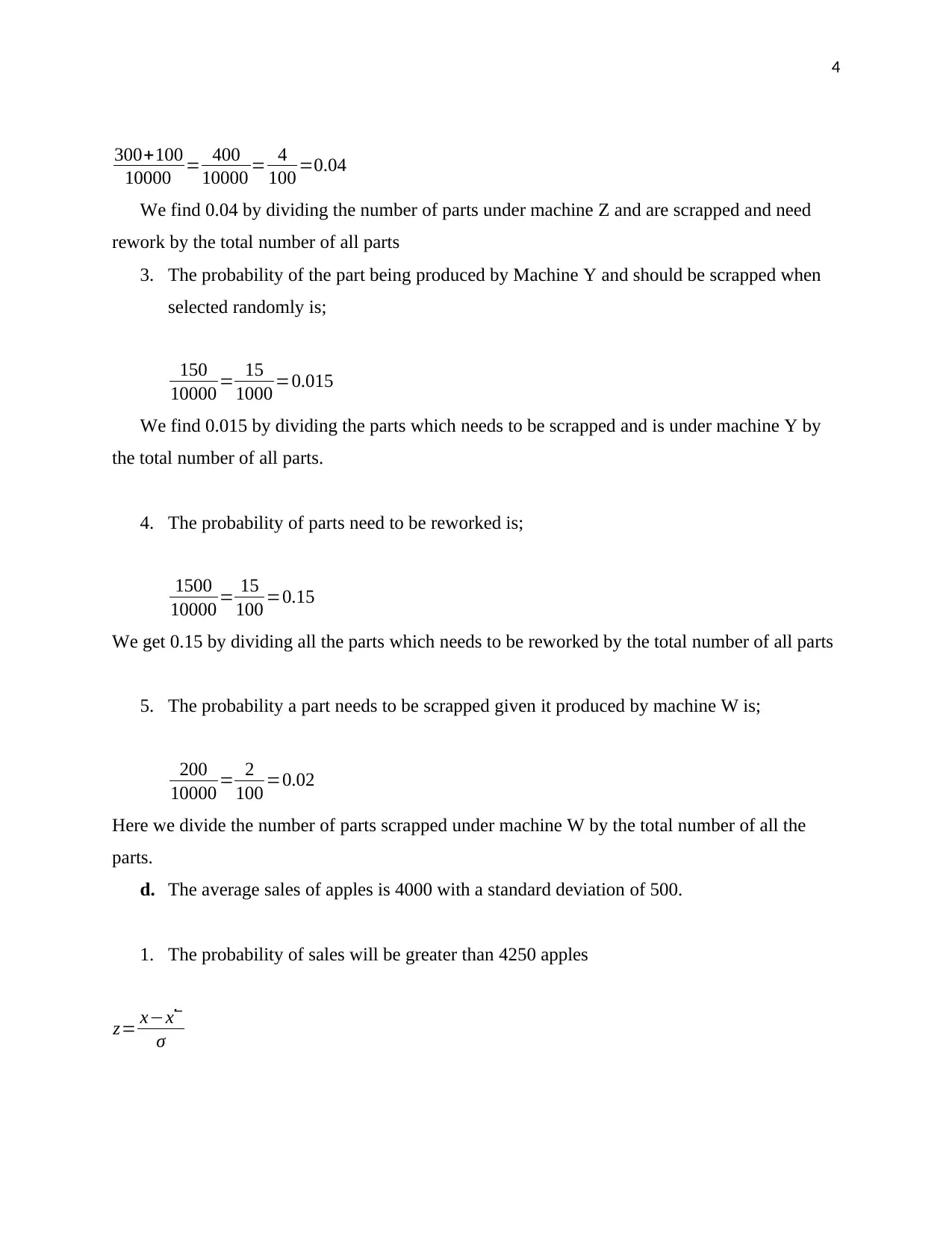
4
300+100
10000 = 400
10000 = 4
100 =0.04
We find 0.04 by dividing the number of parts under machine Z and are scrapped and need
rework by the total number of all parts
3. The probability of the part being produced by Machine Y and should be scrapped when
selected randomly is;
150
10000 = 15
1000 =0.015
We find 0.015 by dividing the parts which needs to be scrapped and is under machine Y by
the total number of all parts.
4. The probability of parts need to be reworked is;
1500
10000 = 15
100 =0.15
We get 0.15 by dividing all the parts which needs to be reworked by the total number of all parts
5. The probability a part needs to be scrapped given it produced by machine W is;
200
10000 = 2
100 =0.02
Here we divide the number of parts scrapped under machine W by the total number of all the
parts.
d. The average sales of apples is 4000 with a standard deviation of 500.
1. The probability of sales will be greater than 4250 apples
z= x−x՟
σ
300+100
10000 = 400
10000 = 4
100 =0.04
We find 0.04 by dividing the number of parts under machine Z and are scrapped and need
rework by the total number of all parts
3. The probability of the part being produced by Machine Y and should be scrapped when
selected randomly is;
150
10000 = 15
1000 =0.015
We find 0.015 by dividing the parts which needs to be scrapped and is under machine Y by
the total number of all parts.
4. The probability of parts need to be reworked is;
1500
10000 = 15
100 =0.15
We get 0.15 by dividing all the parts which needs to be reworked by the total number of all parts
5. The probability a part needs to be scrapped given it produced by machine W is;
200
10000 = 2
100 =0.02
Here we divide the number of parts scrapped under machine W by the total number of all the
parts.
d. The average sales of apples is 4000 with a standard deviation of 500.
1. The probability of sales will be greater than 4250 apples
z= x−x՟
σ
Secure Best Marks with AI Grader
Need help grading? Try our AI Grader for instant feedback on your assignments.
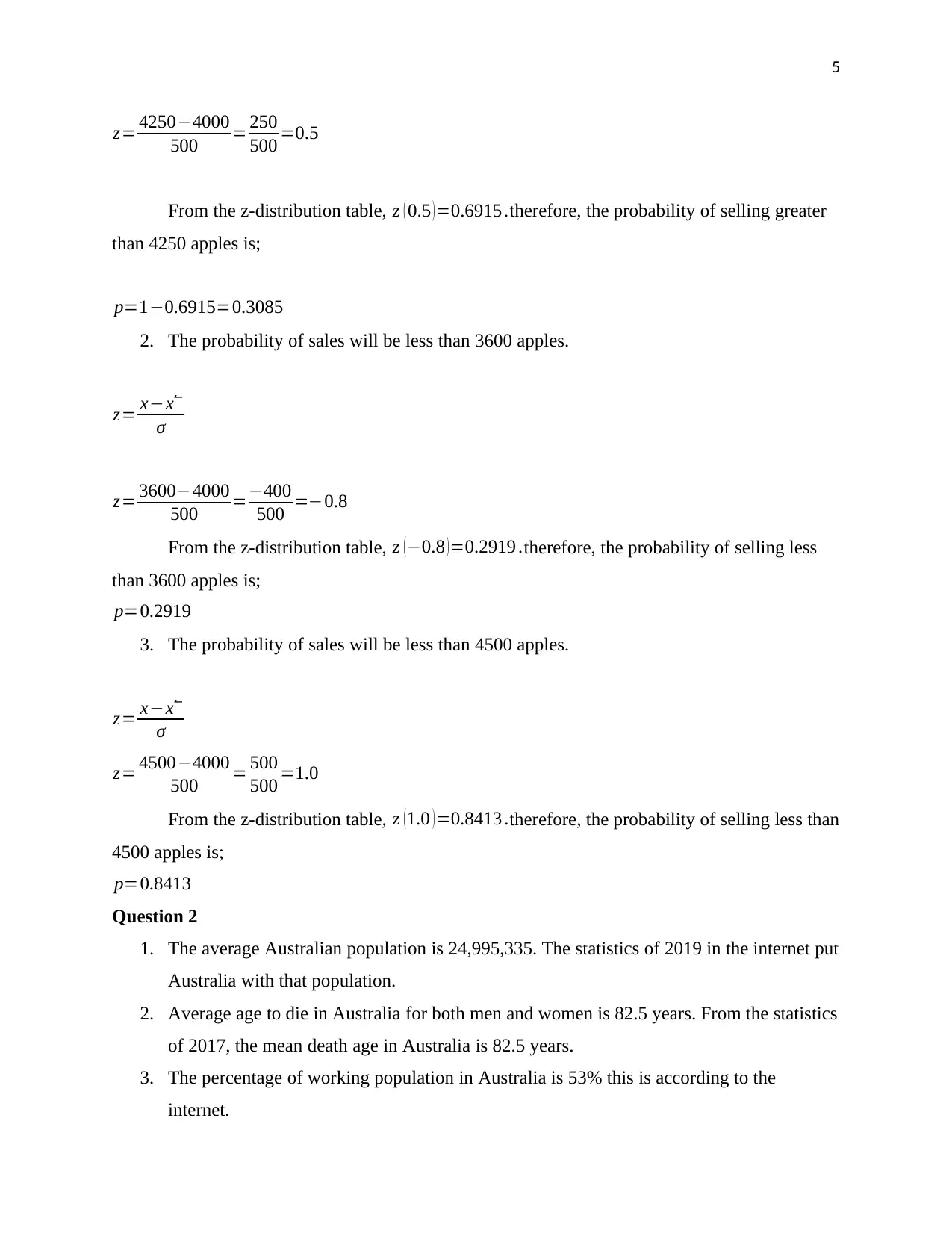
5
z= 4250−4000
500 = 250
500 =0.5
From the z-distribution table, z ( 0.5 ) =0.6915 .therefore, the probability of selling greater
than 4250 apples is;
p=1−0.6915=0.3085
2. The probability of sales will be less than 3600 apples.
z= x−x՟
σ
z= 3600−4000
500 =−400
500 =−0.8
From the z-distribution table, z (−0.8 )=0.2919 .therefore, the probability of selling less
than 3600 apples is;
p=0.2919
3. The probability of sales will be less than 4500 apples.
z= x−x՟
σ
z= 4500−4000
500 = 500
500 =1.0
From the z-distribution table, z (1.0 )=0.8413 .therefore, the probability of selling less than
4500 apples is;
p=0.8413
Question 2
1. The average Australian population is 24,995,335. The statistics of 2019 in the internet put
Australia with that population.
2. Average age to die in Australia for both men and women is 82.5 years. From the statistics
of 2017, the mean death age in Australia is 82.5 years.
3. The percentage of working population in Australia is 53% this is according to the
internet.
z= 4250−4000
500 = 250
500 =0.5
From the z-distribution table, z ( 0.5 ) =0.6915 .therefore, the probability of selling greater
than 4250 apples is;
p=1−0.6915=0.3085
2. The probability of sales will be less than 3600 apples.
z= x−x՟
σ
z= 3600−4000
500 =−400
500 =−0.8
From the z-distribution table, z (−0.8 )=0.2919 .therefore, the probability of selling less
than 3600 apples is;
p=0.2919
3. The probability of sales will be less than 4500 apples.
z= x−x՟
σ
z= 4500−4000
500 = 500
500 =1.0
From the z-distribution table, z (1.0 )=0.8413 .therefore, the probability of selling less than
4500 apples is;
p=0.8413
Question 2
1. The average Australian population is 24,995,335. The statistics of 2019 in the internet put
Australia with that population.
2. Average age to die in Australia for both men and women is 82.5 years. From the statistics
of 2017, the mean death age in Australia is 82.5 years.
3. The percentage of working population in Australia is 53% this is according to the
internet.
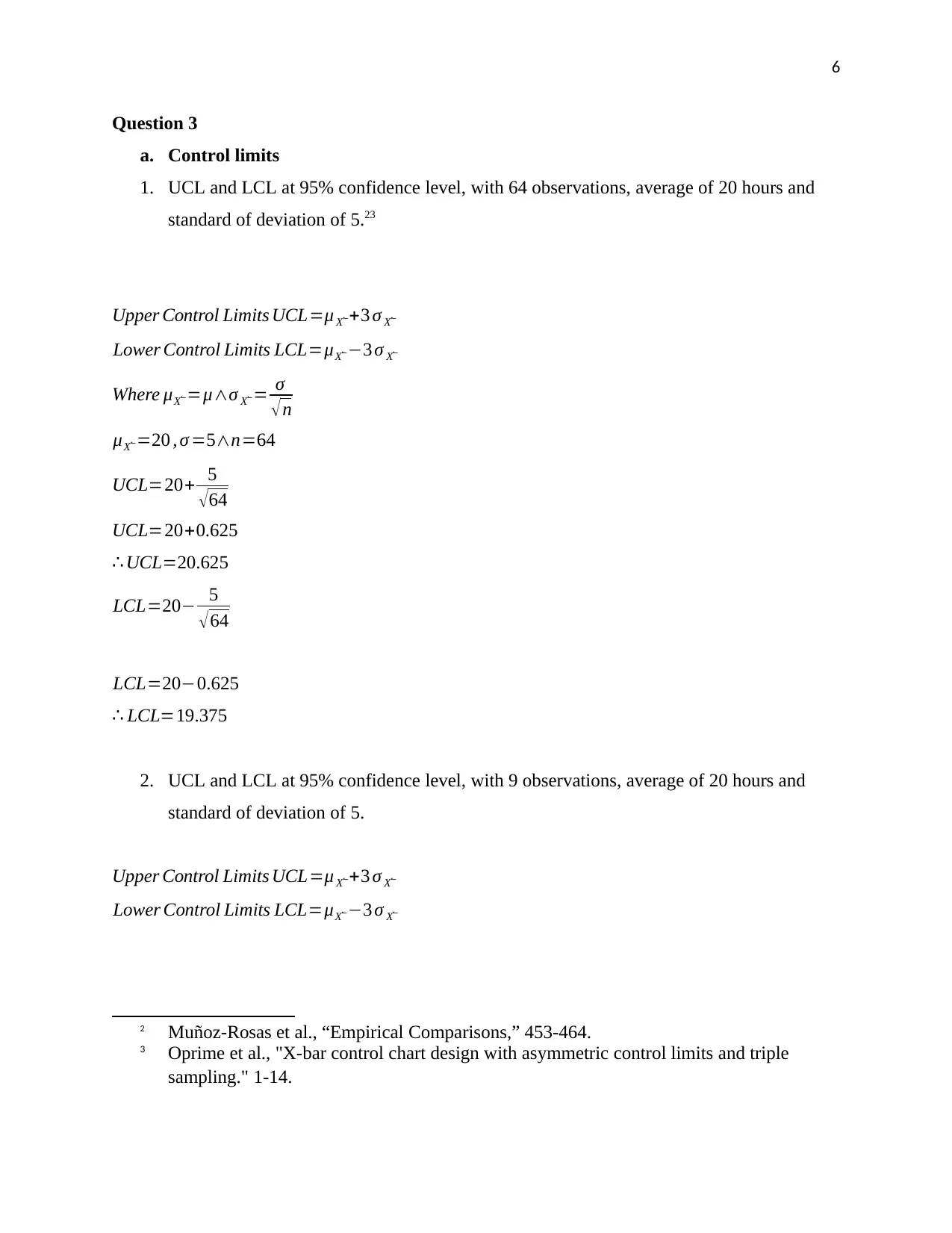
6
Question 3
a. Control limits
1. UCL and LCL at 95% confidence level, with 64 observations, average of 20 hours and
standard of deviation of 5.23
Upper Control Limits UCL=μ X՟+3 σ X՟
Lower Control Limits LCL=μX՟−3 σ X՟
Where μX՟=μ∧σ X՟= σ
√ n
μX՟=20 , σ =5∧n=64
UCL=20+ 5
√64
UCL=20+0.625
∴ UCL=20.625
LCL=20− 5
√ 64
LCL=20−0.625
∴ LCL=19.375
2. UCL and LCL at 95% confidence level, with 9 observations, average of 20 hours and
standard of deviation of 5.
Upper Control Limits UCL=μ X՟+3 σ X՟
Lower Control Limits LCL=μX՟−3 σ X՟
2 Muñoz-Rosas et al., “Empirical Comparisons,” 453-464.
3 Oprime et al., "X-bar control chart design with asymmetric control limits and triple
sampling." 1-14.
Question 3
a. Control limits
1. UCL and LCL at 95% confidence level, with 64 observations, average of 20 hours and
standard of deviation of 5.23
Upper Control Limits UCL=μ X՟+3 σ X՟
Lower Control Limits LCL=μX՟−3 σ X՟
Where μX՟=μ∧σ X՟= σ
√ n
μX՟=20 , σ =5∧n=64
UCL=20+ 5
√64
UCL=20+0.625
∴ UCL=20.625
LCL=20− 5
√ 64
LCL=20−0.625
∴ LCL=19.375
2. UCL and LCL at 95% confidence level, with 9 observations, average of 20 hours and
standard of deviation of 5.
Upper Control Limits UCL=μ X՟+3 σ X՟
Lower Control Limits LCL=μX՟−3 σ X՟
2 Muñoz-Rosas et al., “Empirical Comparisons,” 453-464.
3 Oprime et al., "X-bar control chart design with asymmetric control limits and triple
sampling." 1-14.
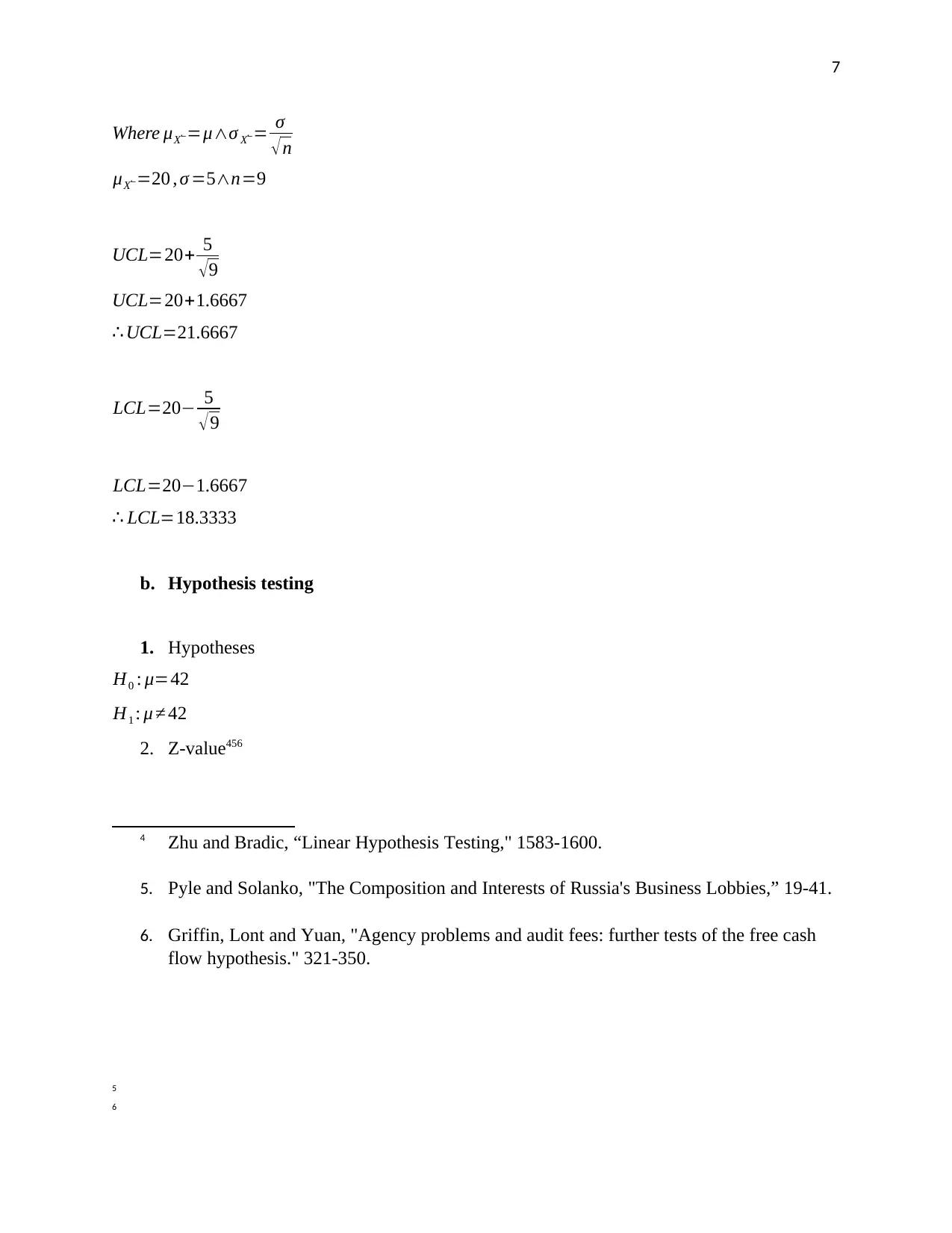
7
Where μX՟=μ∧σ X՟= σ
√ n
μX՟=20 , σ =5∧n=9
UCL=20+ 5
√9
UCL=20+1.6667
∴ UCL=21.6667
LCL=20− 5
√9
LCL=20−1.6667
∴ LCL=18.3333
b. Hypothesis testing
1. Hypotheses
H0 : μ=42
H1 : μ ≠ 42
2. Z-value456
4 Zhu and Bradic, “Linear Hypothesis Testing," 1583-1600.
5. Pyle and Solanko, "The Composition and Interests of Russia's Business Lobbies,” 19-41.
6. Griffin, Lont and Yuan, "Agency problems and audit fees: further tests of the free cash
flow hypothesis." 321-350.
5
6
Where μX՟=μ∧σ X՟= σ
√ n
μX՟=20 , σ =5∧n=9
UCL=20+ 5
√9
UCL=20+1.6667
∴ UCL=21.6667
LCL=20− 5
√9
LCL=20−1.6667
∴ LCL=18.3333
b. Hypothesis testing
1. Hypotheses
H0 : μ=42
H1 : μ ≠ 42
2. Z-value456
4 Zhu and Bradic, “Linear Hypothesis Testing," 1583-1600.
5. Pyle and Solanko, "The Composition and Interests of Russia's Business Lobbies,” 19-41.
6. Griffin, Lont and Yuan, "Agency problems and audit fees: further tests of the free cash
flow hypothesis." 321-350.
5
6
Paraphrase This Document
Need a fresh take? Get an instant paraphrase of this document with our AI Paraphraser
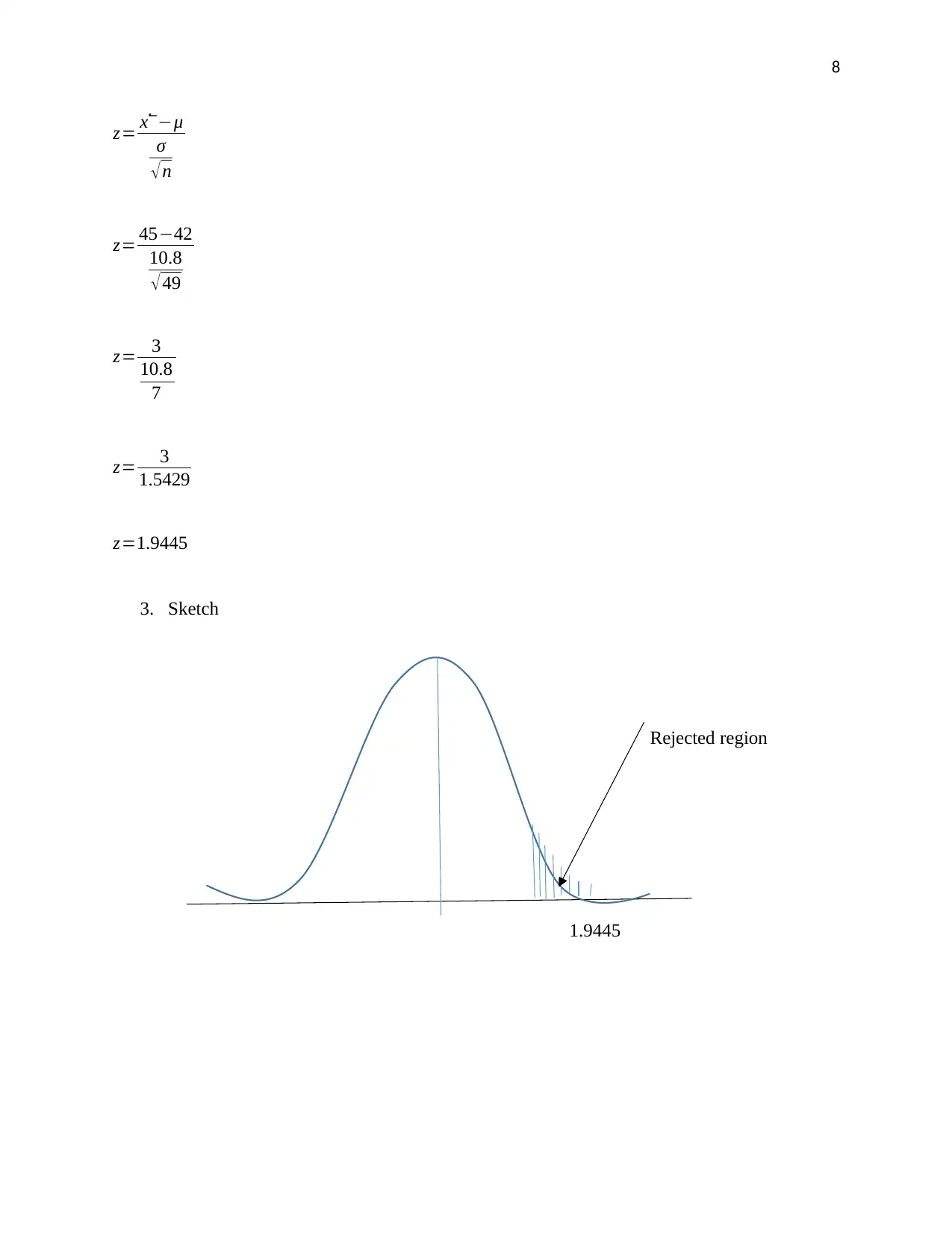
8
z= x՟−μ
σ
√ n
z= 45−42
10.8
√ 49
z= 3
10.8
7
z= 3
1.5429
z=1.9445
3. Sketch
Rejected region
1.9445
z= x՟−μ
σ
√ n
z= 45−42
10.8
√ 49
z= 3
10.8
7
z= 3
1.5429
z=1.9445
3. Sketch
Rejected region
1.9445
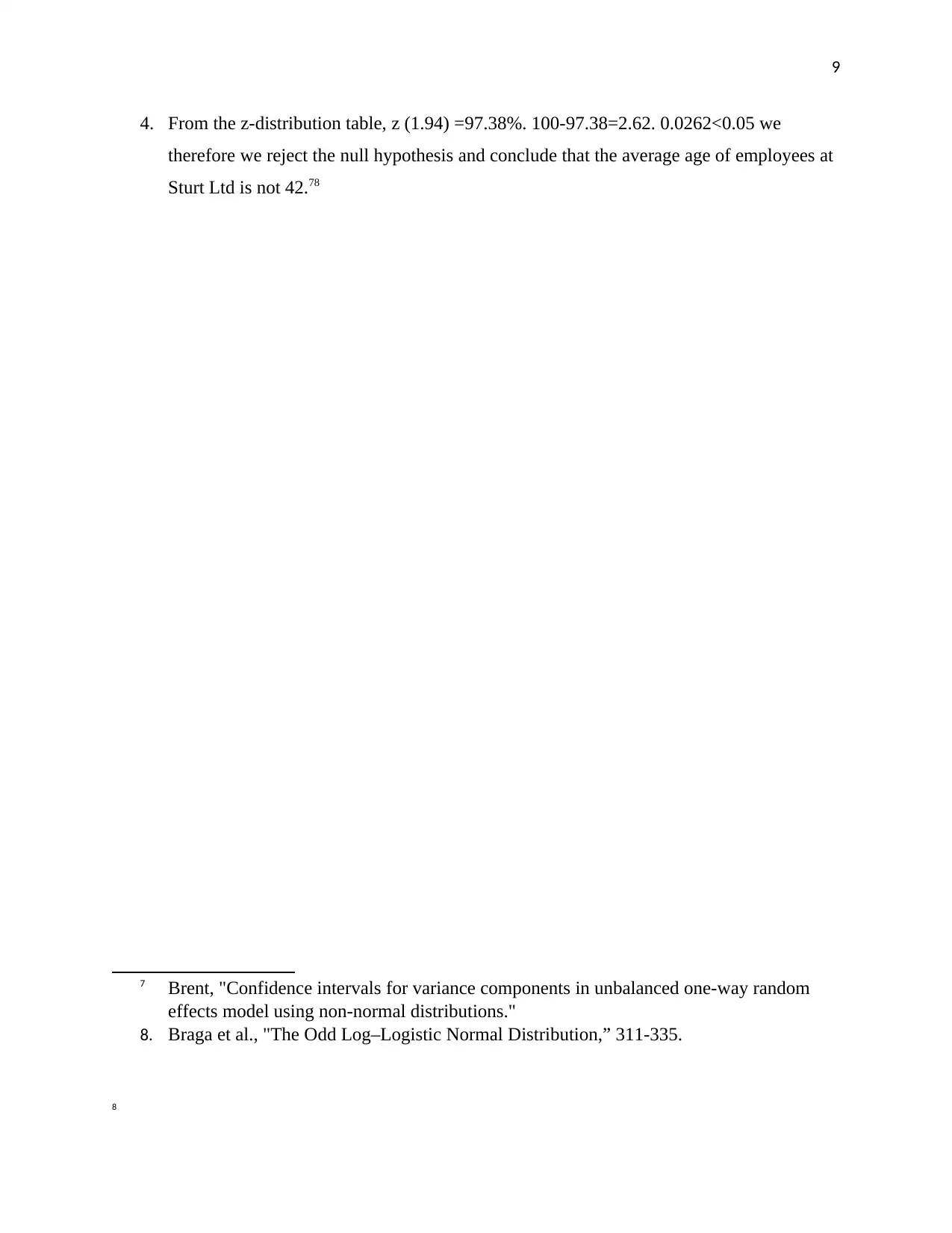
9
4. From the z-distribution table, z (1.94) =97.38%. 100-97.38=2.62. 0.0262<0.05 we
therefore we reject the null hypothesis and conclude that the average age of employees at
Sturt Ltd is not 42.78
7 Brent, "Confidence intervals for variance components in unbalanced one-way random
effects model using non-normal distributions."
8. Braga et al., "The Odd Log–Logistic Normal Distribution,” 311-335.
8
4. From the z-distribution table, z (1.94) =97.38%. 100-97.38=2.62. 0.0262<0.05 we
therefore we reject the null hypothesis and conclude that the average age of employees at
Sturt Ltd is not 42.78
7 Brent, "Confidence intervals for variance components in unbalanced one-way random
effects model using non-normal distributions."
8. Braga et al., "The Odd Log–Logistic Normal Distribution,” 311-335.
8
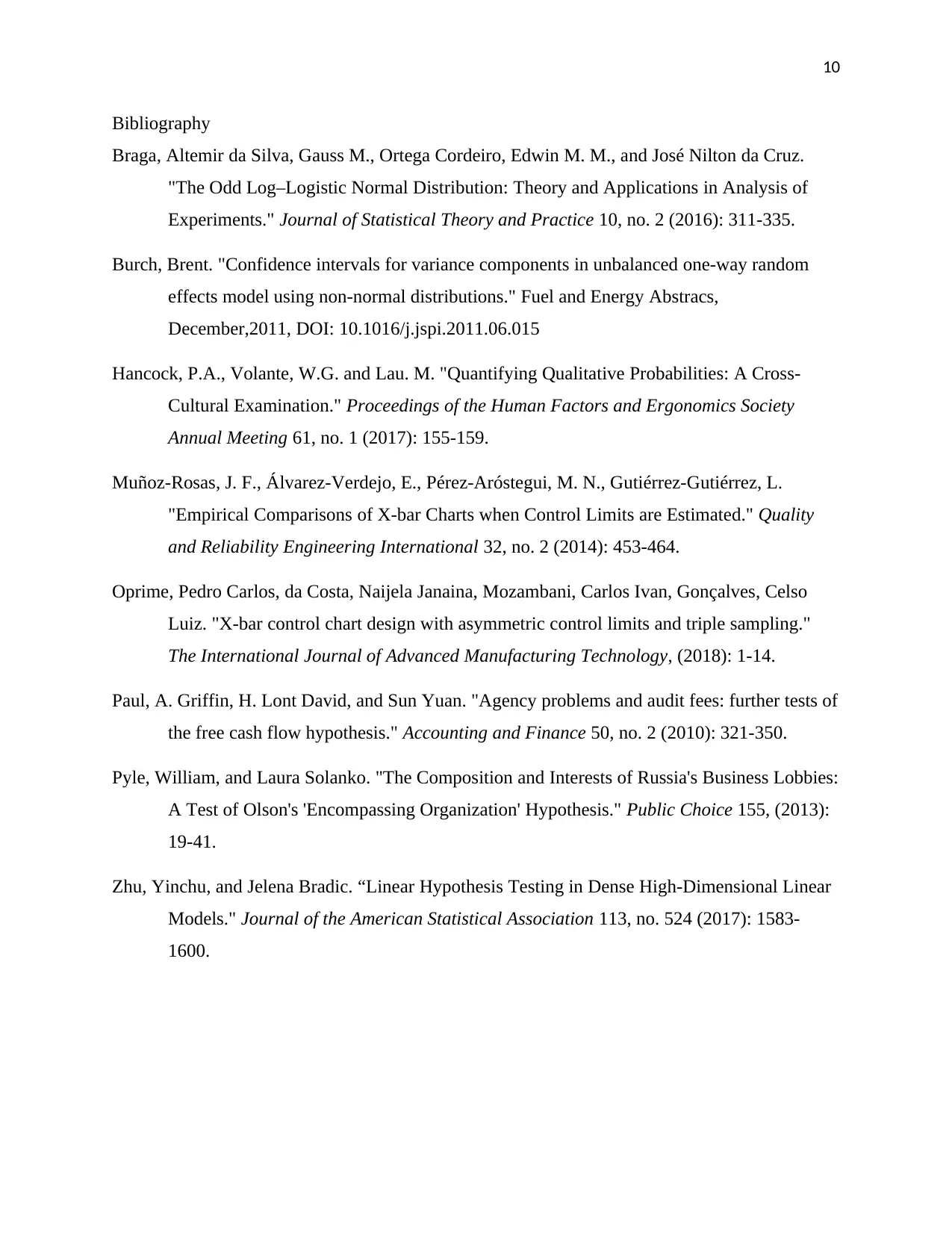
10
Bibliography
Braga, Altemir da Silva, Gauss M., Ortega Cordeiro, Edwin M. M., and José Nilton da Cruz.
"The Odd Log–Logistic Normal Distribution: Theory and Applications in Analysis of
Experiments." Journal of Statistical Theory and Practice 10, no. 2 (2016): 311-335.
Burch, Brent. "Confidence intervals for variance components in unbalanced one-way random
effects model using non-normal distributions." Fuel and Energy Abstracs,
December,2011, DOI: 10.1016/j.jspi.2011.06.015
Hancock, P.A., Volante, W.G. and Lau. M. "Quantifying Qualitative Probabilities: A Cross-
Cultural Examination." Proceedings of the Human Factors and Ergonomics Society
Annual Meeting 61, no. 1 (2017): 155-159.
Muñoz-Rosas, J. F., Álvarez-Verdejo, E., Pérez-Aróstegui, M. N., Gutiérrez-Gutiérrez, L.
"Empirical Comparisons of X-bar Charts when Control Limits are Estimated." Quality
and Reliability Engineering International 32, no. 2 (2014): 453-464.
Oprime, Pedro Carlos, da Costa, Naijela Janaina, Mozambani, Carlos Ivan, Gonçalves, Celso
Luiz. "X-bar control chart design with asymmetric control limits and triple sampling."
The International Journal of Advanced Manufacturing Technology, (2018): 1-14.
Paul, A. Griffin, H. Lont David, and Sun Yuan. "Agency problems and audit fees: further tests of
the free cash flow hypothesis." Accounting and Finance 50, no. 2 (2010): 321-350.
Pyle, William, and Laura Solanko. "The Composition and Interests of Russia's Business Lobbies:
A Test of Olson's 'Encompassing Organization' Hypothesis." Public Choice 155, (2013):
19-41.
Zhu, Yinchu, and Jelena Bradic. “Linear Hypothesis Testing in Dense High-Dimensional Linear
Models." Journal of the American Statistical Association 113, no. 524 (2017): 1583-
1600.
Bibliography
Braga, Altemir da Silva, Gauss M., Ortega Cordeiro, Edwin M. M., and José Nilton da Cruz.
"The Odd Log–Logistic Normal Distribution: Theory and Applications in Analysis of
Experiments." Journal of Statistical Theory and Practice 10, no. 2 (2016): 311-335.
Burch, Brent. "Confidence intervals for variance components in unbalanced one-way random
effects model using non-normal distributions." Fuel and Energy Abstracs,
December,2011, DOI: 10.1016/j.jspi.2011.06.015
Hancock, P.A., Volante, W.G. and Lau. M. "Quantifying Qualitative Probabilities: A Cross-
Cultural Examination." Proceedings of the Human Factors and Ergonomics Society
Annual Meeting 61, no. 1 (2017): 155-159.
Muñoz-Rosas, J. F., Álvarez-Verdejo, E., Pérez-Aróstegui, M. N., Gutiérrez-Gutiérrez, L.
"Empirical Comparisons of X-bar Charts when Control Limits are Estimated." Quality
and Reliability Engineering International 32, no. 2 (2014): 453-464.
Oprime, Pedro Carlos, da Costa, Naijela Janaina, Mozambani, Carlos Ivan, Gonçalves, Celso
Luiz. "X-bar control chart design with asymmetric control limits and triple sampling."
The International Journal of Advanced Manufacturing Technology, (2018): 1-14.
Paul, A. Griffin, H. Lont David, and Sun Yuan. "Agency problems and audit fees: further tests of
the free cash flow hypothesis." Accounting and Finance 50, no. 2 (2010): 321-350.
Pyle, William, and Laura Solanko. "The Composition and Interests of Russia's Business Lobbies:
A Test of Olson's 'Encompassing Organization' Hypothesis." Public Choice 155, (2013):
19-41.
Zhu, Yinchu, and Jelena Bradic. “Linear Hypothesis Testing in Dense High-Dimensional Linear
Models." Journal of the American Statistical Association 113, no. 524 (2017): 1583-
1600.
1 out of 10
Related Documents
![[object Object]](/_next/image/?url=%2F_next%2Fstatic%2Fmedia%2Flogo.6d15ce61.png&w=640&q=75)
Your All-in-One AI-Powered Toolkit for Academic Success.
+13062052269
info@desklib.com
Available 24*7 on WhatsApp / Email
Unlock your academic potential
© 2024 | Zucol Services PVT LTD | All rights reserved.