Predictive and Prescriptive Analytics Case Study
VerifiedAdded on 2021/05/06
|39
|11702
|43
AI Summary
This assignment involves a detailed analysis of a real-world business problem faced by Southern States Cooperative, a large farmer-owned cooperative. The company aims to maintain and extend its success through better targeting of customers in direct-marketing campaigns. Predictive models are built using historical customer transaction data, including RFM analysis, logistic regression, decision tree, and random forest models. An optimization model is then developed to maximize total income from mailing catalogs to customers, resulting in increased revenue generation and cost reduction.
Contribute Materials
Your contribution can guide someone’s learning journey. Share your
documents today.
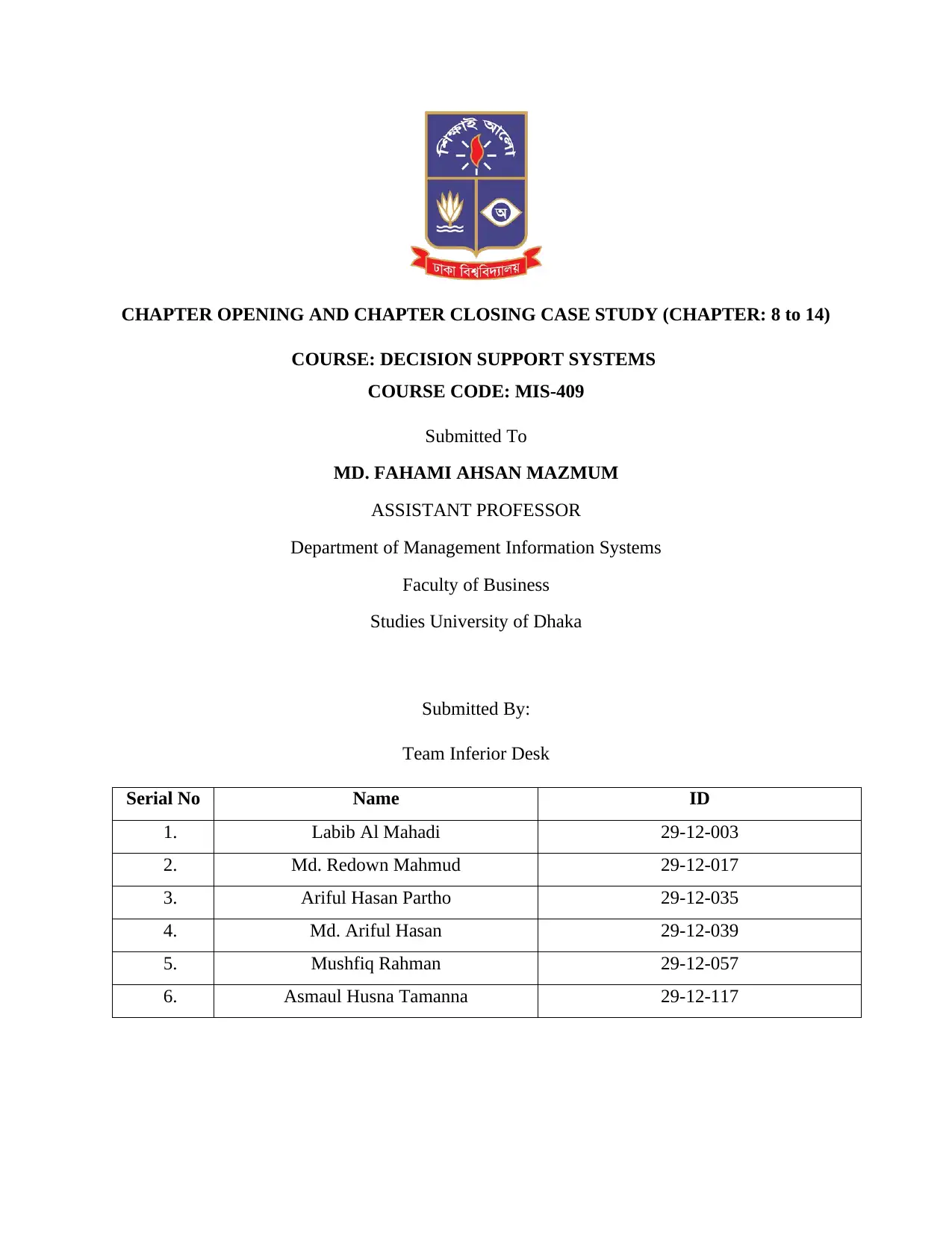
CHAPTER OPENING AND CHAPTER CLOSING CASE STUDY (CHAPTER: 8 to 14)
COURSE: DECISION SUPPORT SYSTEMS
COURSE CODE: MIS-409
Submitted To
MD. FAHAMI AHSAN MAZMUM
ASSISTANT PROFESSOR
Department of Management Information Systems
Faculty of Business
Studies University of Dhaka
Submitted By:
Team Inferior Desk
Serial No Name ID
1. Labib Al Mahadi 29-12-003
2. Md. Redown Mahmud 29-12-017
3. Ariful Hasan Partho 29-12-035
4. Md. Ariful Hasan 29-12-039
5. Mushfiq Rahman 29-12-057
6. Asmaul Husna Tamanna 29-12-117
COURSE: DECISION SUPPORT SYSTEMS
COURSE CODE: MIS-409
Submitted To
MD. FAHAMI AHSAN MAZMUM
ASSISTANT PROFESSOR
Department of Management Information Systems
Faculty of Business
Studies University of Dhaka
Submitted By:
Team Inferior Desk
Serial No Name ID
1. Labib Al Mahadi 29-12-003
2. Md. Redown Mahmud 29-12-017
3. Ariful Hasan Partho 29-12-035
4. Md. Ariful Hasan 29-12-039
5. Mushfiq Rahman 29-12-057
6. Asmaul Husna Tamanna 29-12-117
Secure Best Marks with AI Grader
Need help grading? Try our AI Grader for instant feedback on your assignments.
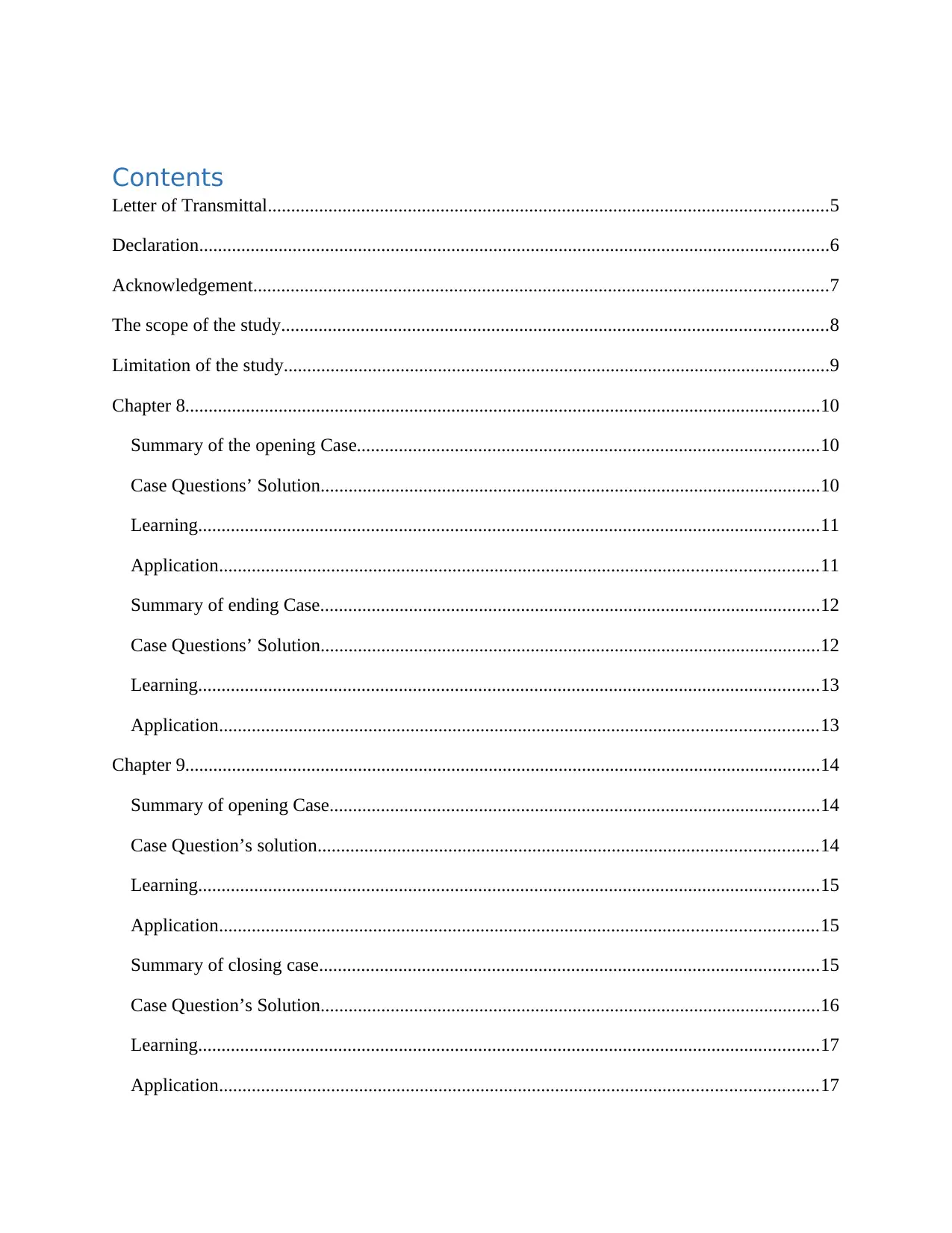
Contents
Letter of Transmittal........................................................................................................................5
Declaration.......................................................................................................................................6
Acknowledgement...........................................................................................................................7
The scope of the study.....................................................................................................................8
Limitation of the study.....................................................................................................................9
Chapter 8........................................................................................................................................10
Summary of the opening Case...................................................................................................10
Case Questions’ Solution...........................................................................................................10
Learning.....................................................................................................................................11
Application................................................................................................................................11
Summary of ending Case...........................................................................................................12
Case Questions’ Solution...........................................................................................................12
Learning.....................................................................................................................................13
Application................................................................................................................................13
Chapter 9........................................................................................................................................14
Summary of opening Case.........................................................................................................14
Case Question’s solution...........................................................................................................14
Learning.....................................................................................................................................15
Application................................................................................................................................15
Summary of closing case...........................................................................................................15
Case Question’s Solution...........................................................................................................16
Learning.....................................................................................................................................17
Application................................................................................................................................17
Letter of Transmittal........................................................................................................................5
Declaration.......................................................................................................................................6
Acknowledgement...........................................................................................................................7
The scope of the study.....................................................................................................................8
Limitation of the study.....................................................................................................................9
Chapter 8........................................................................................................................................10
Summary of the opening Case...................................................................................................10
Case Questions’ Solution...........................................................................................................10
Learning.....................................................................................................................................11
Application................................................................................................................................11
Summary of ending Case...........................................................................................................12
Case Questions’ Solution...........................................................................................................12
Learning.....................................................................................................................................13
Application................................................................................................................................13
Chapter 9........................................................................................................................................14
Summary of opening Case.........................................................................................................14
Case Question’s solution...........................................................................................................14
Learning.....................................................................................................................................15
Application................................................................................................................................15
Summary of closing case...........................................................................................................15
Case Question’s Solution...........................................................................................................16
Learning.....................................................................................................................................17
Application................................................................................................................................17
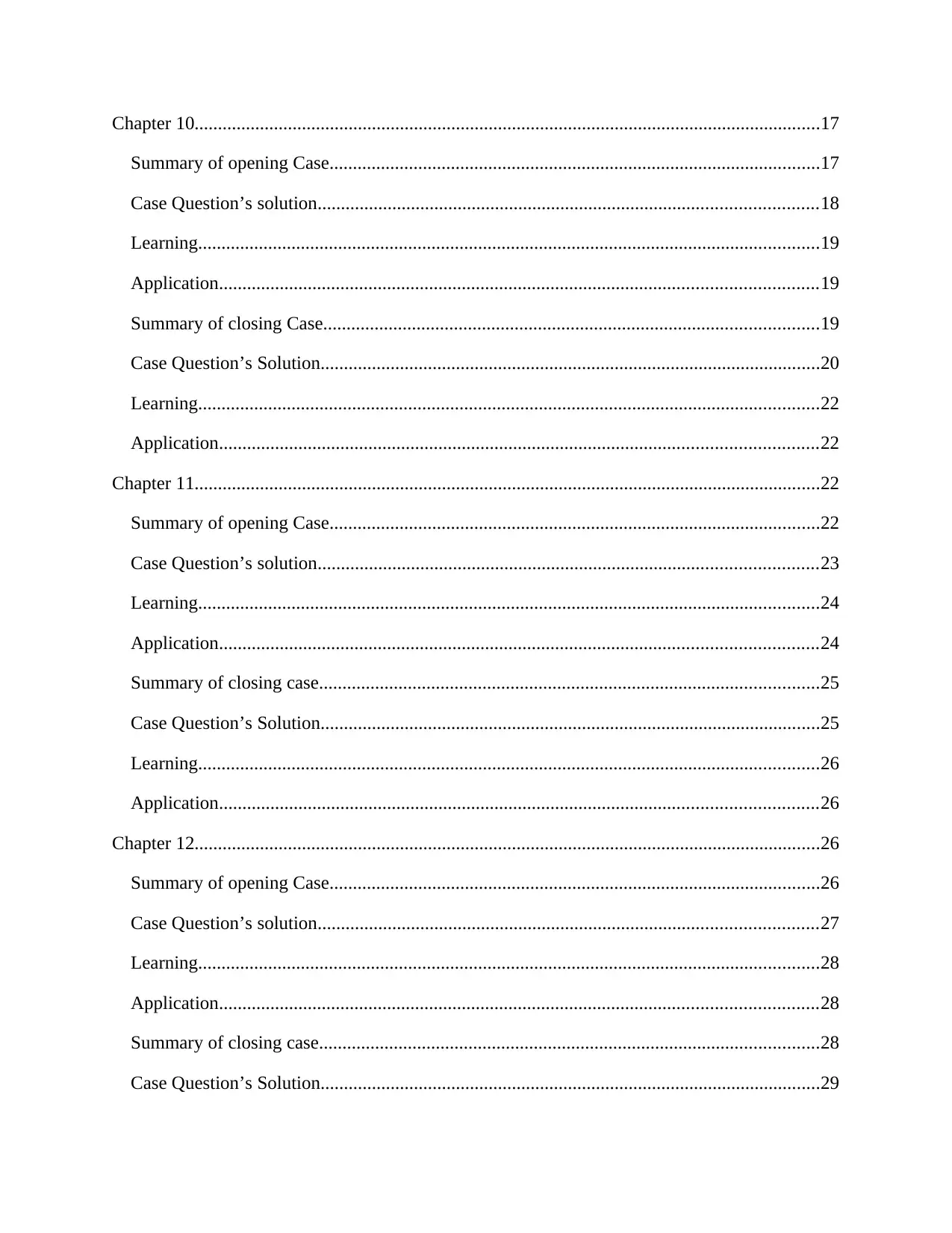
Chapter 10......................................................................................................................................17
Summary of opening Case.........................................................................................................17
Case Question’s solution...........................................................................................................18
Learning.....................................................................................................................................19
Application................................................................................................................................19
Summary of closing Case..........................................................................................................19
Case Question’s Solution...........................................................................................................20
Learning.....................................................................................................................................22
Application................................................................................................................................22
Chapter 11......................................................................................................................................22
Summary of opening Case.........................................................................................................22
Case Question’s solution...........................................................................................................23
Learning.....................................................................................................................................24
Application................................................................................................................................24
Summary of closing case...........................................................................................................25
Case Question’s Solution...........................................................................................................25
Learning.....................................................................................................................................26
Application................................................................................................................................26
Chapter 12......................................................................................................................................26
Summary of opening Case.........................................................................................................26
Case Question’s solution...........................................................................................................27
Learning.....................................................................................................................................28
Application................................................................................................................................28
Summary of closing case...........................................................................................................28
Case Question’s Solution...........................................................................................................29
Summary of opening Case.........................................................................................................17
Case Question’s solution...........................................................................................................18
Learning.....................................................................................................................................19
Application................................................................................................................................19
Summary of closing Case..........................................................................................................19
Case Question’s Solution...........................................................................................................20
Learning.....................................................................................................................................22
Application................................................................................................................................22
Chapter 11......................................................................................................................................22
Summary of opening Case.........................................................................................................22
Case Question’s solution...........................................................................................................23
Learning.....................................................................................................................................24
Application................................................................................................................................24
Summary of closing case...........................................................................................................25
Case Question’s Solution...........................................................................................................25
Learning.....................................................................................................................................26
Application................................................................................................................................26
Chapter 12......................................................................................................................................26
Summary of opening Case.........................................................................................................26
Case Question’s solution...........................................................................................................27
Learning.....................................................................................................................................28
Application................................................................................................................................28
Summary of closing case...........................................................................................................28
Case Question’s Solution...........................................................................................................29
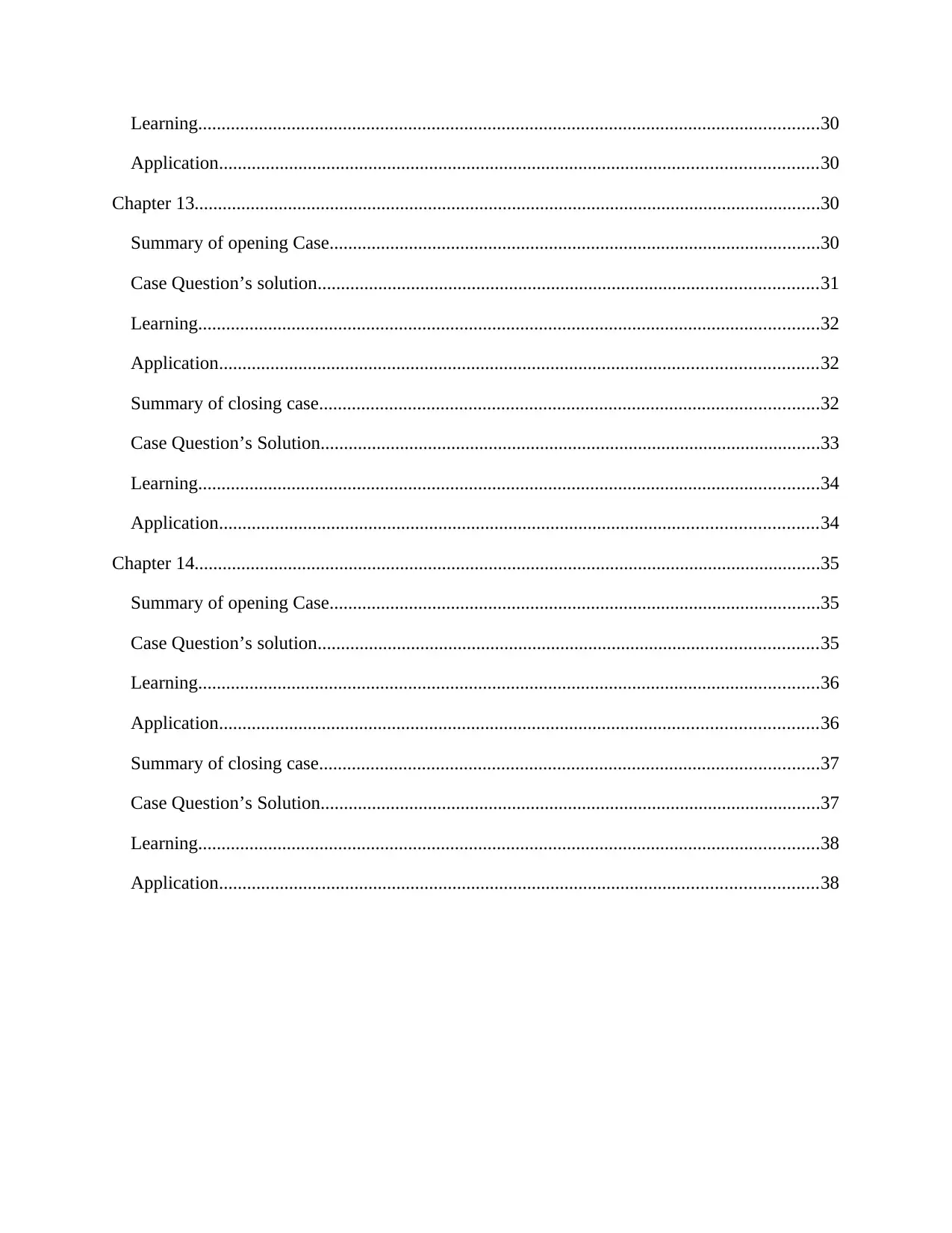
Learning.....................................................................................................................................30
Application................................................................................................................................30
Chapter 13......................................................................................................................................30
Summary of opening Case.........................................................................................................30
Case Question’s solution...........................................................................................................31
Learning.....................................................................................................................................32
Application................................................................................................................................32
Summary of closing case...........................................................................................................32
Case Question’s Solution...........................................................................................................33
Learning.....................................................................................................................................34
Application................................................................................................................................34
Chapter 14......................................................................................................................................35
Summary of opening Case.........................................................................................................35
Case Question’s solution...........................................................................................................35
Learning.....................................................................................................................................36
Application................................................................................................................................36
Summary of closing case...........................................................................................................37
Case Question’s Solution...........................................................................................................37
Learning.....................................................................................................................................38
Application................................................................................................................................38
Application................................................................................................................................30
Chapter 13......................................................................................................................................30
Summary of opening Case.........................................................................................................30
Case Question’s solution...........................................................................................................31
Learning.....................................................................................................................................32
Application................................................................................................................................32
Summary of closing case...........................................................................................................32
Case Question’s Solution...........................................................................................................33
Learning.....................................................................................................................................34
Application................................................................................................................................34
Chapter 14......................................................................................................................................35
Summary of opening Case.........................................................................................................35
Case Question’s solution...........................................................................................................35
Learning.....................................................................................................................................36
Application................................................................................................................................36
Summary of closing case...........................................................................................................37
Case Question’s Solution...........................................................................................................37
Learning.....................................................................................................................................38
Application................................................................................................................................38
Secure Best Marks with AI Grader
Need help grading? Try our AI Grader for instant feedback on your assignments.
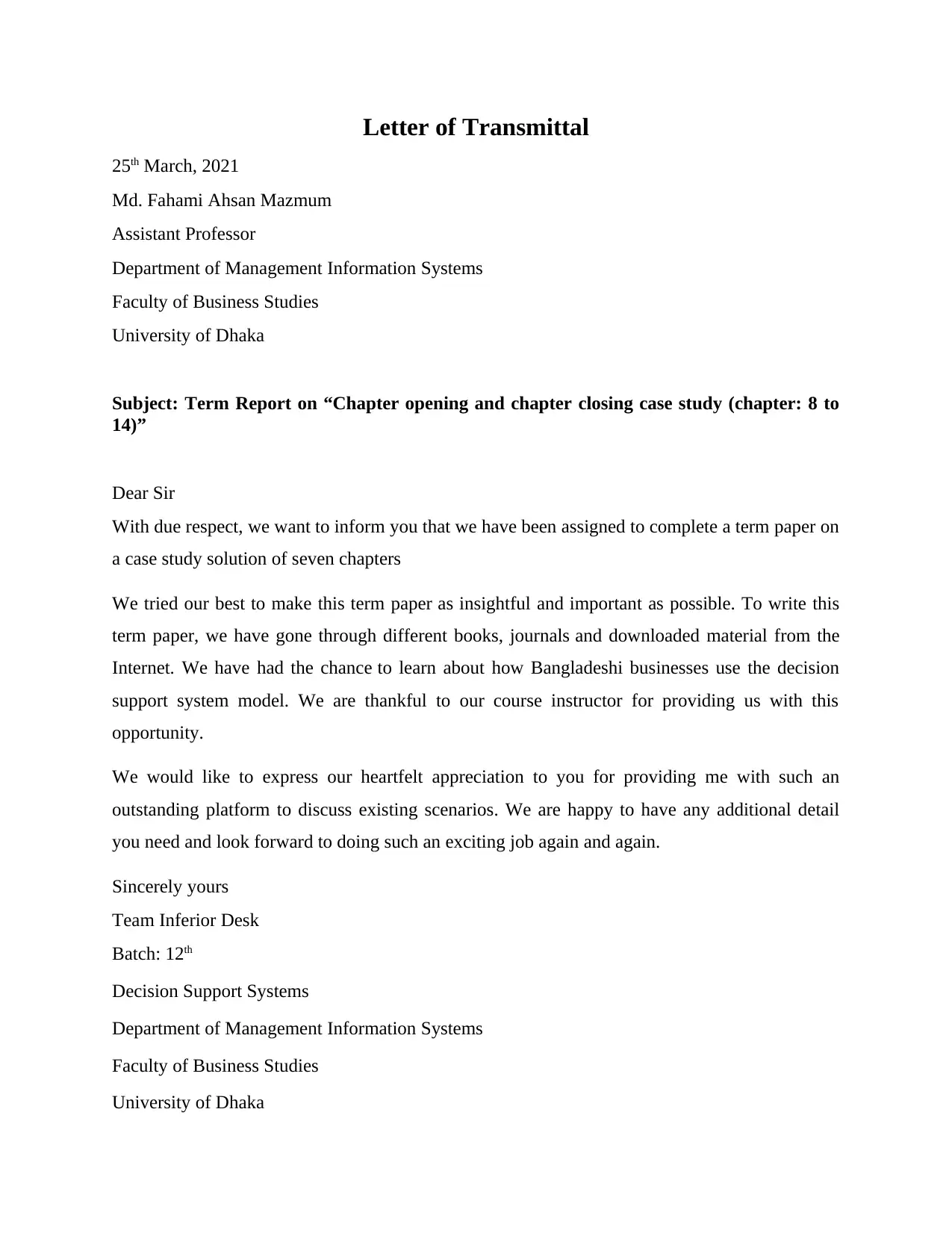
Letter of Transmittal
25th March, 2021
Md. Fahami Ahsan Mazmum
Assistant Professor
Department of Management Information Systems
Faculty of Business Studies
University of Dhaka
Subject: Term Report on “Chapter opening and chapter closing case study (chapter: 8 to
14)”
Dear Sir
With due respect, we want to inform you that we have been assigned to complete a term paper on
a case study solution of seven chapters
We tried our best to make this term paper as insightful and important as possible. To write this
term paper, we have gone through different books, journals and downloaded material from the
Internet. We have had the chance to learn about how Bangladeshi businesses use the decision
support system model. We are thankful to our course instructor for providing us with this
opportunity.
We would like to express our heartfelt appreciation to you for providing me with such an
outstanding platform to discuss existing scenarios. We are happy to have any additional detail
you need and look forward to doing such an exciting job again and again.
Sincerely yours
Team Inferior Desk
Batch: 12th
Decision Support Systems
Department of Management Information Systems
Faculty of Business Studies
University of Dhaka
25th March, 2021
Md. Fahami Ahsan Mazmum
Assistant Professor
Department of Management Information Systems
Faculty of Business Studies
University of Dhaka
Subject: Term Report on “Chapter opening and chapter closing case study (chapter: 8 to
14)”
Dear Sir
With due respect, we want to inform you that we have been assigned to complete a term paper on
a case study solution of seven chapters
We tried our best to make this term paper as insightful and important as possible. To write this
term paper, we have gone through different books, journals and downloaded material from the
Internet. We have had the chance to learn about how Bangladeshi businesses use the decision
support system model. We are thankful to our course instructor for providing us with this
opportunity.
We would like to express our heartfelt appreciation to you for providing me with such an
outstanding platform to discuss existing scenarios. We are happy to have any additional detail
you need and look forward to doing such an exciting job again and again.
Sincerely yours
Team Inferior Desk
Batch: 12th
Decision Support Systems
Department of Management Information Systems
Faculty of Business Studies
University of Dhaka
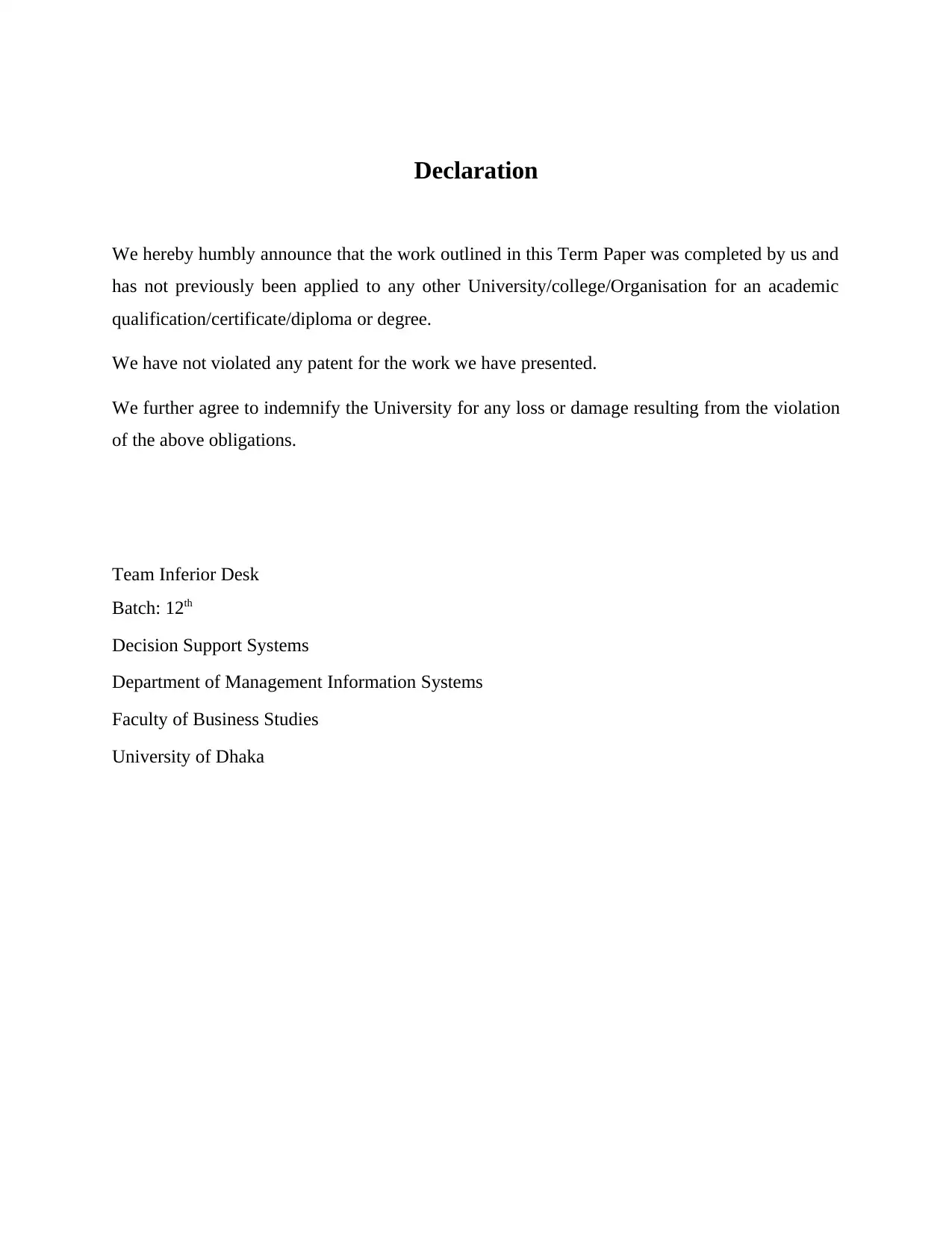
Declaration
We hereby humbly announce that the work outlined in this Term Paper was completed by us and
has not previously been applied to any other University/college/Organisation for an academic
qualification/certificate/diploma or degree.
We have not violated any patent for the work we have presented.
We further agree to indemnify the University for any loss or damage resulting from the violation
of the above obligations.
Team Inferior Desk
Batch: 12th
Decision Support Systems
Department of Management Information Systems
Faculty of Business Studies
University of Dhaka
We hereby humbly announce that the work outlined in this Term Paper was completed by us and
has not previously been applied to any other University/college/Organisation for an academic
qualification/certificate/diploma or degree.
We have not violated any patent for the work we have presented.
We further agree to indemnify the University for any loss or damage resulting from the violation
of the above obligations.
Team Inferior Desk
Batch: 12th
Decision Support Systems
Department of Management Information Systems
Faculty of Business Studies
University of Dhaka
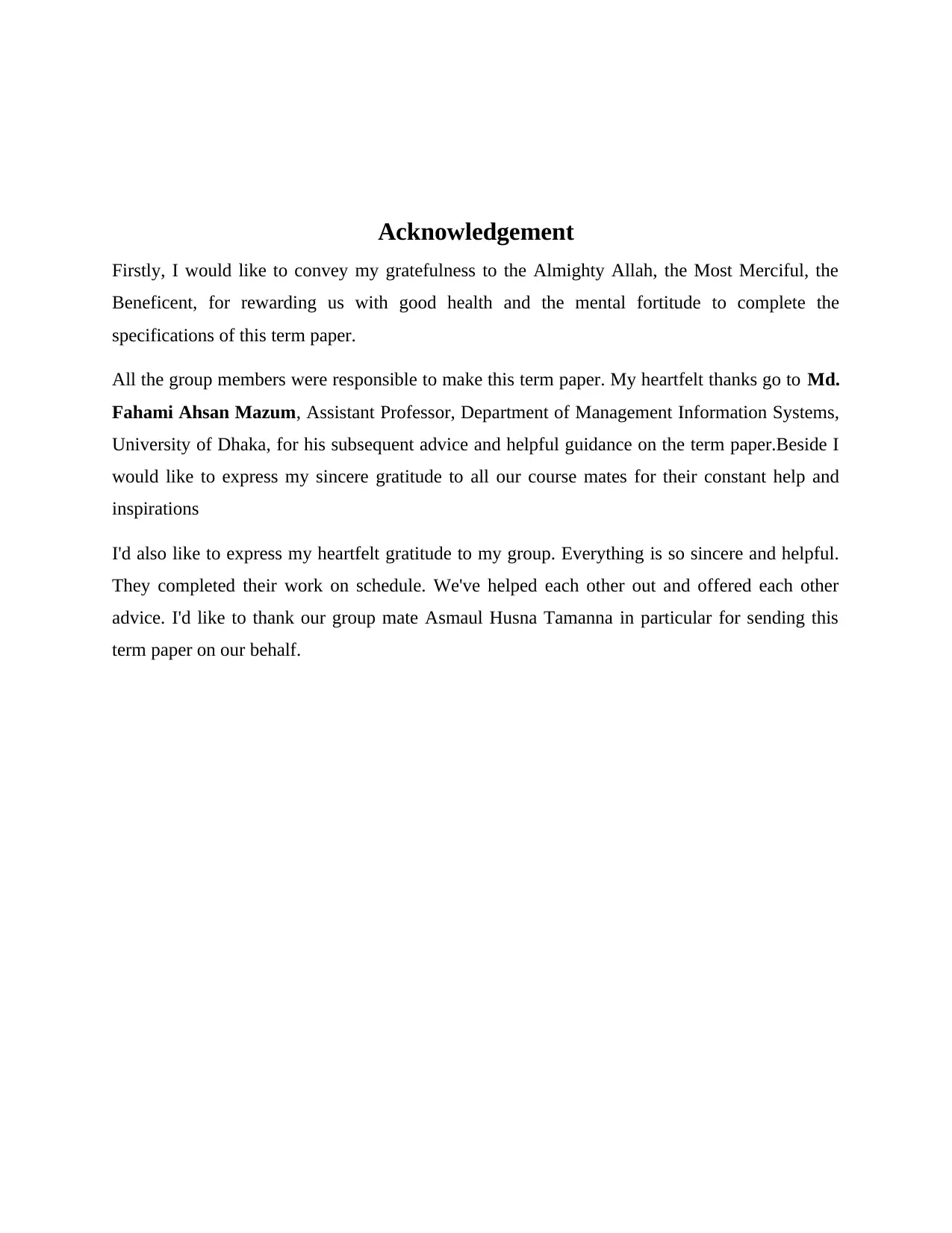
Acknowledgement
Firstly, I would like to convey my gratefulness to the Almighty Allah, the Most Merciful, the
Beneficent, for rewarding us with good health and the mental fortitude to complete the
specifications of this term paper.
All the group members were responsible to make this term paper. My heartfelt thanks go to Md.
Fahami Ahsan Mazum, Assistant Professor, Department of Management Information Systems,
University of Dhaka, for his subsequent advice and helpful guidance on the term paper.Beside I
would like to express my sincere gratitude to all our course mates for their constant help and
inspirations
I'd also like to express my heartfelt gratitude to my group. Everything is so sincere and helpful.
They completed their work on schedule. We've helped each other out and offered each other
advice. I'd like to thank our group mate Asmaul Husna Tamanna in particular for sending this
term paper on our behalf.
Firstly, I would like to convey my gratefulness to the Almighty Allah, the Most Merciful, the
Beneficent, for rewarding us with good health and the mental fortitude to complete the
specifications of this term paper.
All the group members were responsible to make this term paper. My heartfelt thanks go to Md.
Fahami Ahsan Mazum, Assistant Professor, Department of Management Information Systems,
University of Dhaka, for his subsequent advice and helpful guidance on the term paper.Beside I
would like to express my sincere gratitude to all our course mates for their constant help and
inspirations
I'd also like to express my heartfelt gratitude to my group. Everything is so sincere and helpful.
They completed their work on schedule. We've helped each other out and offered each other
advice. I'd like to thank our group mate Asmaul Husna Tamanna in particular for sending this
term paper on our behalf.
Paraphrase This Document
Need a fresh take? Get an instant paraphrase of this document with our AI Paraphraser
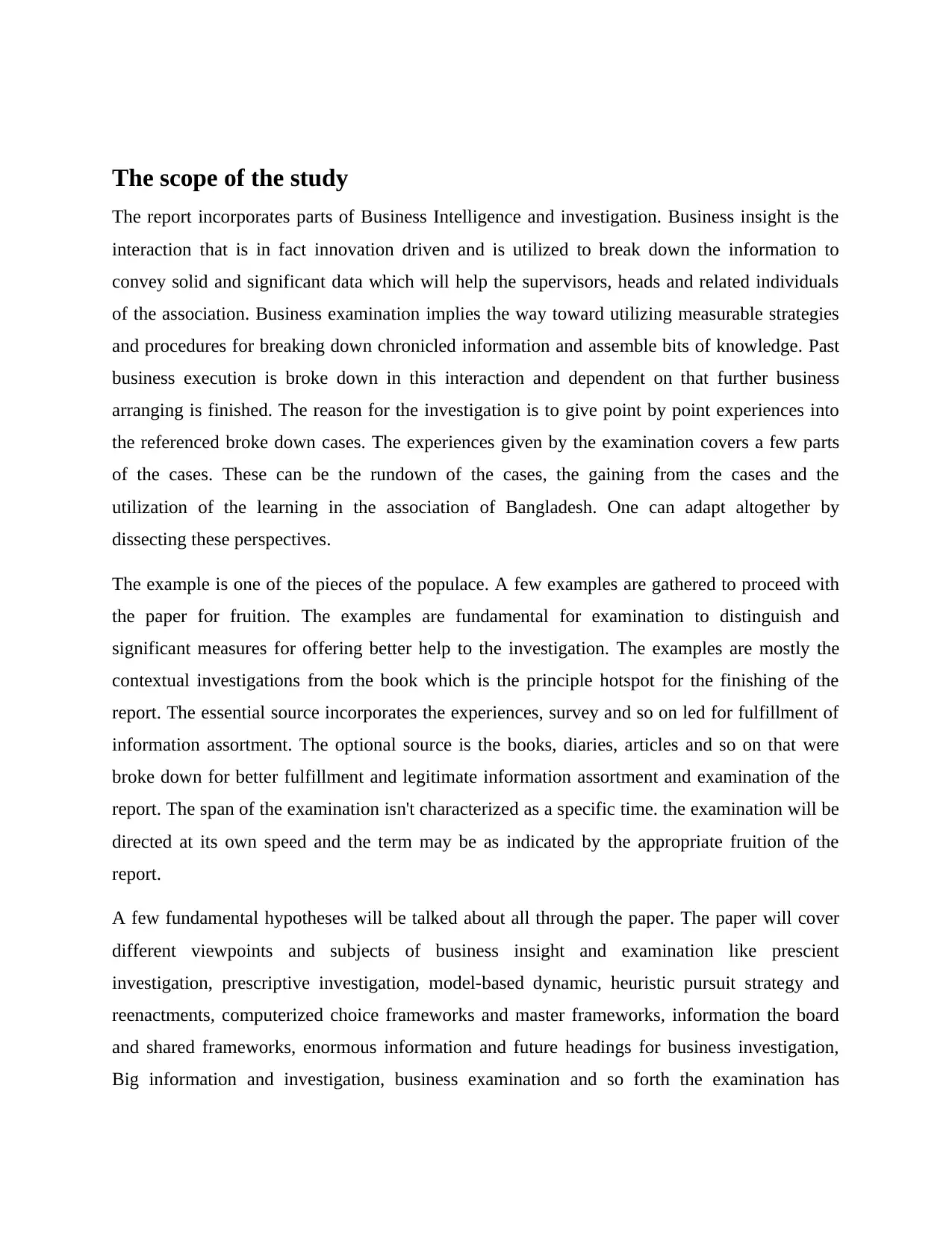
The scope of the study
The report incorporates parts of Business Intelligence and investigation. Business insight is the
interaction that is in fact innovation driven and is utilized to break down the information to
convey solid and significant data which will help the supervisors, heads and related individuals
of the association. Business examination implies the way toward utilizing measurable strategies
and procedures for breaking down chronicled information and assemble bits of knowledge. Past
business execution is broke down in this interaction and dependent on that further business
arranging is finished. The reason for the investigation is to give point by point experiences into
the referenced broke down cases. The experiences given by the examination covers a few parts
of the cases. These can be the rundown of the cases, the gaining from the cases and the
utilization of the learning in the association of Bangladesh. One can adapt altogether by
dissecting these perspectives.
The example is one of the pieces of the populace. A few examples are gathered to proceed with
the paper for fruition. The examples are fundamental for examination to distinguish and
significant measures for offering better help to the investigation. The examples are mostly the
contextual investigations from the book which is the principle hotspot for the finishing of the
report. The essential source incorporates the experiences, survey and so on led for fulfillment of
information assortment. The optional source is the books, diaries, articles and so on that were
broke down for better fulfillment and legitimate information assortment and examination of the
report. The span of the examination isn't characterized as a specific time. the examination will be
directed at its own speed and the term may be as indicated by the appropriate fruition of the
report.
A few fundamental hypotheses will be talked about all through the paper. The paper will cover
different viewpoints and subjects of business insight and examination like prescient
investigation, prescriptive investigation, model-based dynamic, heuristic pursuit strategy and
reenactments, computerized choice frameworks and master frameworks, information the board
and shared frameworks, enormous information and future headings for business investigation,
Big information and investigation, business examination and so forth the examination has
The report incorporates parts of Business Intelligence and investigation. Business insight is the
interaction that is in fact innovation driven and is utilized to break down the information to
convey solid and significant data which will help the supervisors, heads and related individuals
of the association. Business examination implies the way toward utilizing measurable strategies
and procedures for breaking down chronicled information and assemble bits of knowledge. Past
business execution is broke down in this interaction and dependent on that further business
arranging is finished. The reason for the investigation is to give point by point experiences into
the referenced broke down cases. The experiences given by the examination covers a few parts
of the cases. These can be the rundown of the cases, the gaining from the cases and the
utilization of the learning in the association of Bangladesh. One can adapt altogether by
dissecting these perspectives.
The example is one of the pieces of the populace. A few examples are gathered to proceed with
the paper for fruition. The examples are fundamental for examination to distinguish and
significant measures for offering better help to the investigation. The examples are mostly the
contextual investigations from the book which is the principle hotspot for the finishing of the
report. The essential source incorporates the experiences, survey and so on led for fulfillment of
information assortment. The optional source is the books, diaries, articles and so on that were
broke down for better fulfillment and legitimate information assortment and examination of the
report. The span of the examination isn't characterized as a specific time. the examination will be
directed at its own speed and the term may be as indicated by the appropriate fruition of the
report.
A few fundamental hypotheses will be talked about all through the paper. The paper will cover
different viewpoints and subjects of business insight and examination like prescient
investigation, prescriptive investigation, model-based dynamic, heuristic pursuit strategy and
reenactments, computerized choice frameworks and master frameworks, information the board
and shared frameworks, enormous information and future headings for business investigation,
Big information and investigation, business examination and so forth the examination has
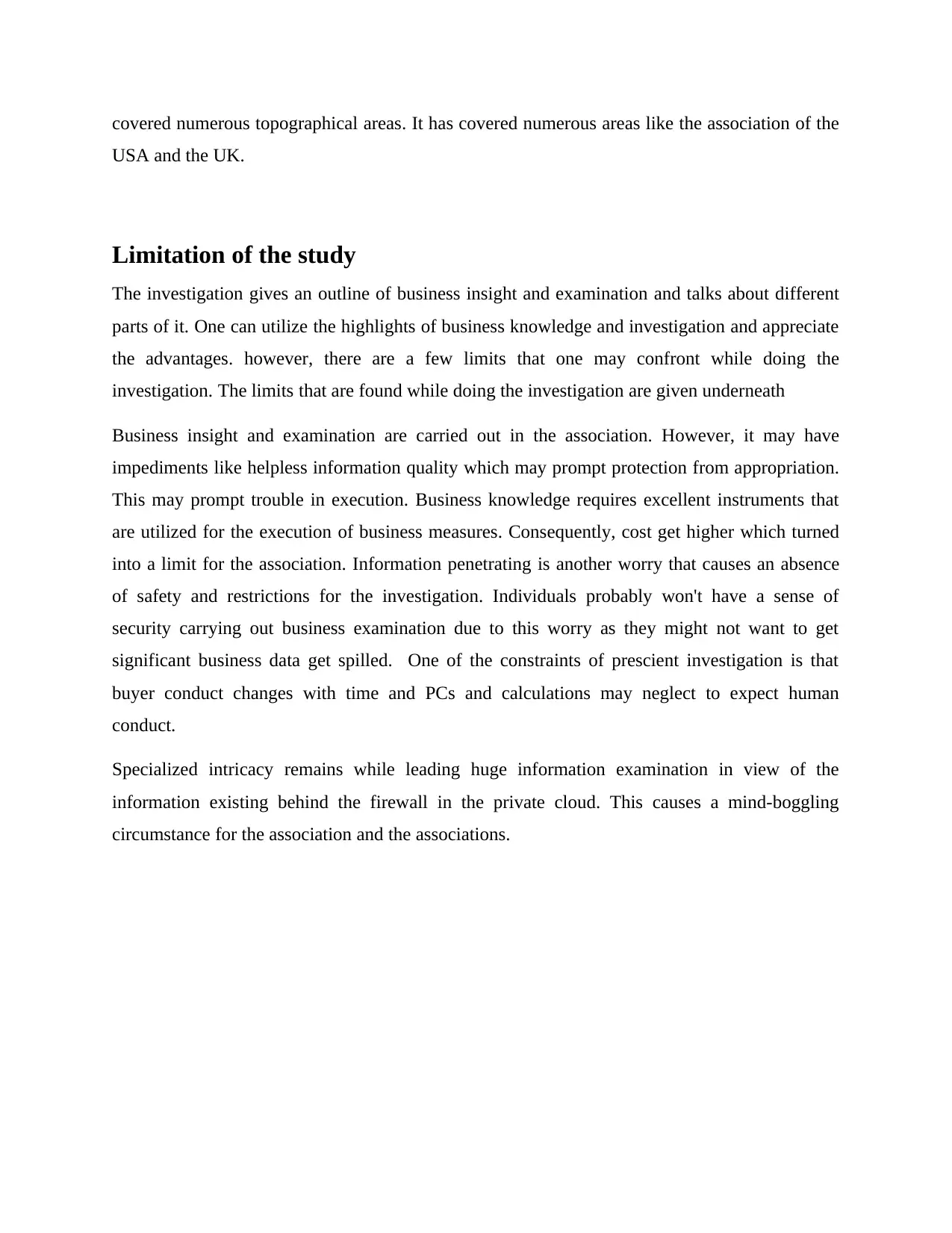
covered numerous topographical areas. It has covered numerous areas like the association of the
USA and the UK.
Limitation of the study
The investigation gives an outline of business insight and examination and talks about different
parts of it. One can utilize the highlights of business knowledge and investigation and appreciate
the advantages. however, there are a few limits that one may confront while doing the
investigation. The limits that are found while doing the investigation are given underneath
Business insight and examination are carried out in the association. However, it may have
impediments like helpless information quality which may prompt protection from appropriation.
This may prompt trouble in execution. Business knowledge requires excellent instruments that
are utilized for the execution of business measures. Consequently, cost get higher which turned
into a limit for the association. Information penetrating is another worry that causes an absence
of safety and restrictions for the investigation. Individuals probably won't have a sense of
security carrying out business examination due to this worry as they might not want to get
significant business data get spilled. One of the constraints of prescient investigation is that
buyer conduct changes with time and PCs and calculations may neglect to expect human
conduct.
Specialized intricacy remains while leading huge information examination in view of the
information existing behind the firewall in the private cloud. This causes a mind-boggling
circumstance for the association and the associations.
USA and the UK.
Limitation of the study
The investigation gives an outline of business insight and examination and talks about different
parts of it. One can utilize the highlights of business knowledge and investigation and appreciate
the advantages. however, there are a few limits that one may confront while doing the
investigation. The limits that are found while doing the investigation are given underneath
Business insight and examination are carried out in the association. However, it may have
impediments like helpless information quality which may prompt protection from appropriation.
This may prompt trouble in execution. Business knowledge requires excellent instruments that
are utilized for the execution of business measures. Consequently, cost get higher which turned
into a limit for the association. Information penetrating is another worry that causes an absence
of safety and restrictions for the investigation. Individuals probably won't have a sense of
security carrying out business examination due to this worry as they might not want to get
significant business data get spilled. One of the constraints of prescient investigation is that
buyer conduct changes with time and PCs and calculations may neglect to expect human
conduct.
Specialized intricacy remains while leading huge information examination in view of the
information existing behind the firewall in the private cloud. This causes a mind-boggling
circumstance for the association and the associations.
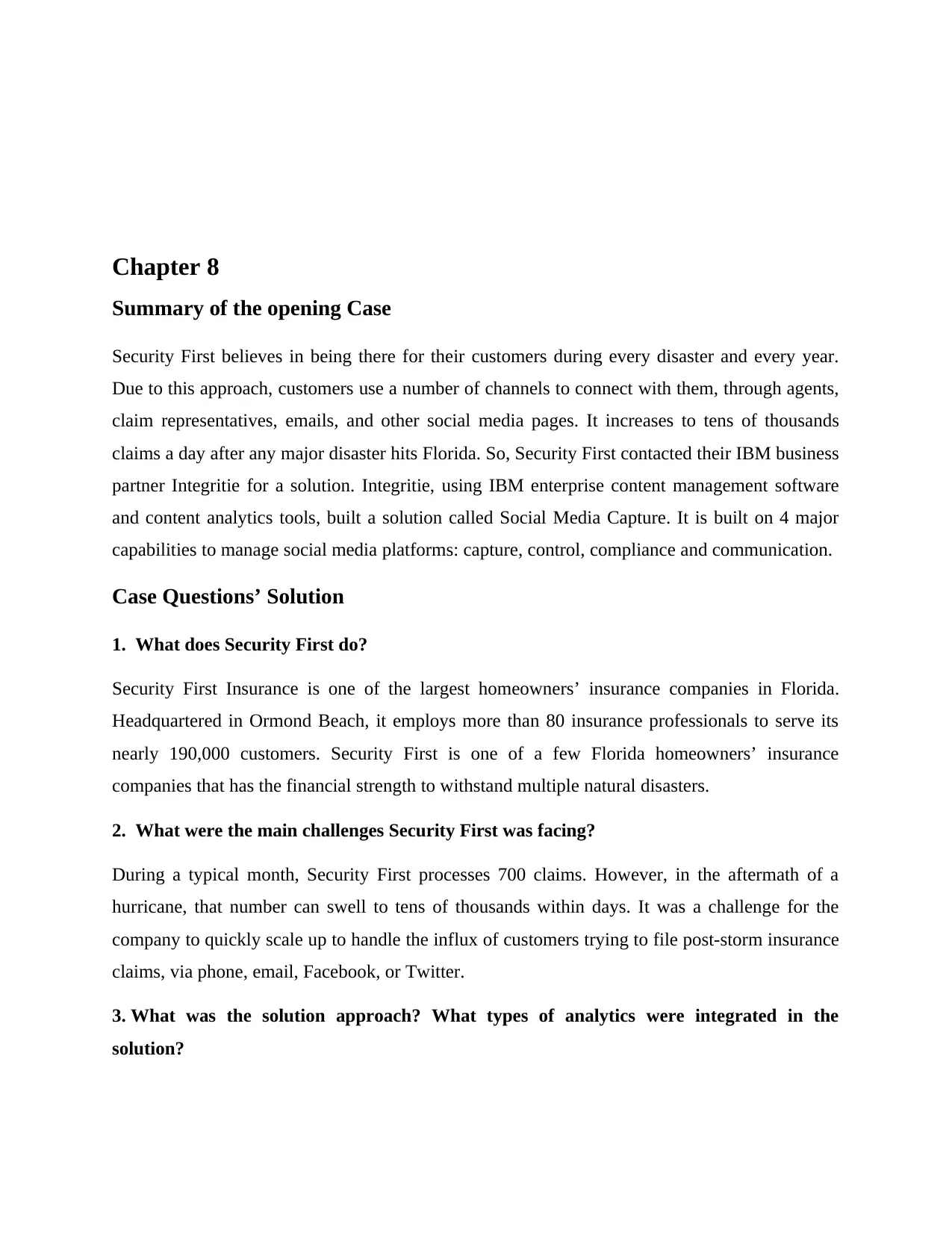
Chapter 8
Summary of the opening Case
Security First believes in being there for their customers during every disaster and every year.
Due to this approach, customers use a number of channels to connect with them, through agents,
claim representatives, emails, and other social media pages. It increases to tens of thousands
claims a day after any major disaster hits Florida. So, Security First contacted their IBM business
partner Integritie for a solution. Integritie, using IBM enterprise content management software
and content analytics tools, built a solution called Social Media Capture. It is built on 4 major
capabilities to manage social media platforms: capture, control, compliance and communication.
Case Questions’ Solution
1. What does Security First do?
Security First Insurance is one of the largest homeowners’ insurance companies in Florida.
Headquartered in Ormond Beach, it employs more than 80 insurance professionals to serve its
nearly 190,000 customers. Security First is one of a few Florida homeowners’ insurance
companies that has the financial strength to withstand multiple natural disasters.
2. What were the main challenges Security First was facing?
During a typical month, Security First processes 700 claims. However, in the aftermath of a
hurricane, that number can swell to tens of thousands within days. It was a challenge for the
company to quickly scale up to handle the influx of customers trying to file post-storm insurance
claims, via phone, email, Facebook, or Twitter.
3. What was the solution approach? What types of analytics were integrated in the
solution?
Summary of the opening Case
Security First believes in being there for their customers during every disaster and every year.
Due to this approach, customers use a number of channels to connect with them, through agents,
claim representatives, emails, and other social media pages. It increases to tens of thousands
claims a day after any major disaster hits Florida. So, Security First contacted their IBM business
partner Integritie for a solution. Integritie, using IBM enterprise content management software
and content analytics tools, built a solution called Social Media Capture. It is built on 4 major
capabilities to manage social media platforms: capture, control, compliance and communication.
Case Questions’ Solution
1. What does Security First do?
Security First Insurance is one of the largest homeowners’ insurance companies in Florida.
Headquartered in Ormond Beach, it employs more than 80 insurance professionals to serve its
nearly 190,000 customers. Security First is one of a few Florida homeowners’ insurance
companies that has the financial strength to withstand multiple natural disasters.
2. What were the main challenges Security First was facing?
During a typical month, Security First processes 700 claims. However, in the aftermath of a
hurricane, that number can swell to tens of thousands within days. It was a challenge for the
company to quickly scale up to handle the influx of customers trying to file post-storm insurance
claims, via phone, email, Facebook, or Twitter.
3. What was the solution approach? What types of analytics were integrated in the
solution?
Secure Best Marks with AI Grader
Need help grading? Try our AI Grader for instant feedback on your assignments.
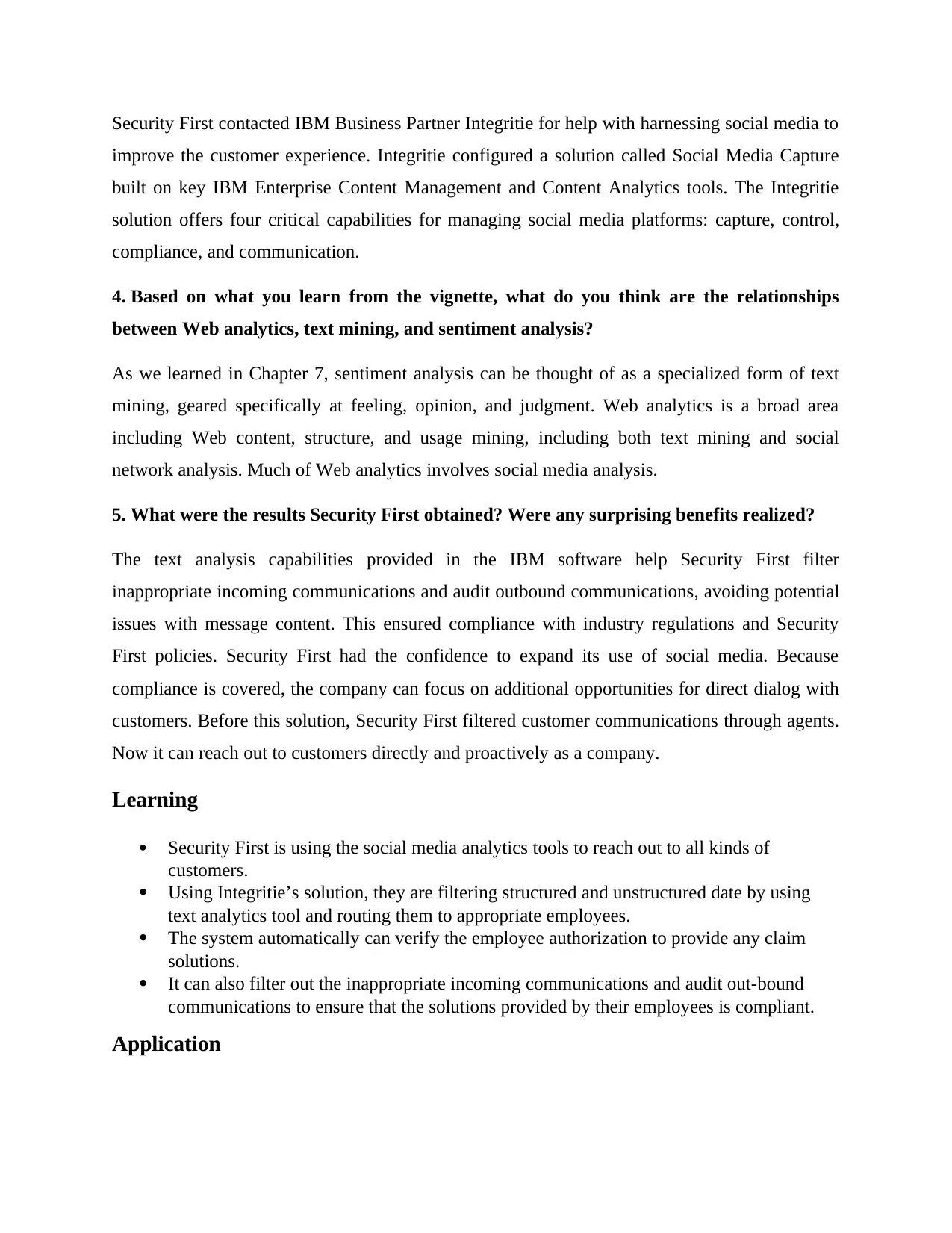
Security First contacted IBM Business Partner Integritie for help with harnessing social media to
improve the customer experience. Integritie configured a solution called Social Media Capture
built on key IBM Enterprise Content Management and Content Analytics tools. The Integritie
solution offers four critical capabilities for managing social media platforms: capture, control,
compliance, and communication.
4. Based on what you learn from the vignette, what do you think are the relationships
between Web analytics, text mining, and sentiment analysis?
As we learned in Chapter 7, sentiment analysis can be thought of as a specialized form of text
mining, geared specifically at feeling, opinion, and judgment. Web analytics is a broad area
including Web content, structure, and usage mining, including both text mining and social
network analysis. Much of Web analytics involves social media analysis.
5. What were the results Security First obtained? Were any surprising benefits realized?
The text analysis capabilities provided in the IBM software help Security First filter
inappropriate incoming communications and audit outbound communications, avoiding potential
issues with message content. This ensured compliance with industry regulations and Security
First policies. Security First had the confidence to expand its use of social media. Because
compliance is covered, the company can focus on additional opportunities for direct dialog with
customers. Before this solution, Security First filtered customer communications through agents.
Now it can reach out to customers directly and proactively as a company.
Learning
Security First is using the social media analytics tools to reach out to all kinds of
customers.
Using Integritie’s solution, they are filtering structured and unstructured date by using
text analytics tool and routing them to appropriate employees.
The system automatically can verify the employee authorization to provide any claim
solutions.
It can also filter out the inappropriate incoming communications and audit out-bound
communications to ensure that the solutions provided by their employees is compliant.
Application
improve the customer experience. Integritie configured a solution called Social Media Capture
built on key IBM Enterprise Content Management and Content Analytics tools. The Integritie
solution offers four critical capabilities for managing social media platforms: capture, control,
compliance, and communication.
4. Based on what you learn from the vignette, what do you think are the relationships
between Web analytics, text mining, and sentiment analysis?
As we learned in Chapter 7, sentiment analysis can be thought of as a specialized form of text
mining, geared specifically at feeling, opinion, and judgment. Web analytics is a broad area
including Web content, structure, and usage mining, including both text mining and social
network analysis. Much of Web analytics involves social media analysis.
5. What were the results Security First obtained? Were any surprising benefits realized?
The text analysis capabilities provided in the IBM software help Security First filter
inappropriate incoming communications and audit outbound communications, avoiding potential
issues with message content. This ensured compliance with industry regulations and Security
First policies. Security First had the confidence to expand its use of social media. Because
compliance is covered, the company can focus on additional opportunities for direct dialog with
customers. Before this solution, Security First filtered customer communications through agents.
Now it can reach out to customers directly and proactively as a company.
Learning
Security First is using the social media analytics tools to reach out to all kinds of
customers.
Using Integritie’s solution, they are filtering structured and unstructured date by using
text analytics tool and routing them to appropriate employees.
The system automatically can verify the employee authorization to provide any claim
solutions.
It can also filter out the inappropriate incoming communications and audit out-bound
communications to ensure that the solutions provided by their employees is compliant.
Application
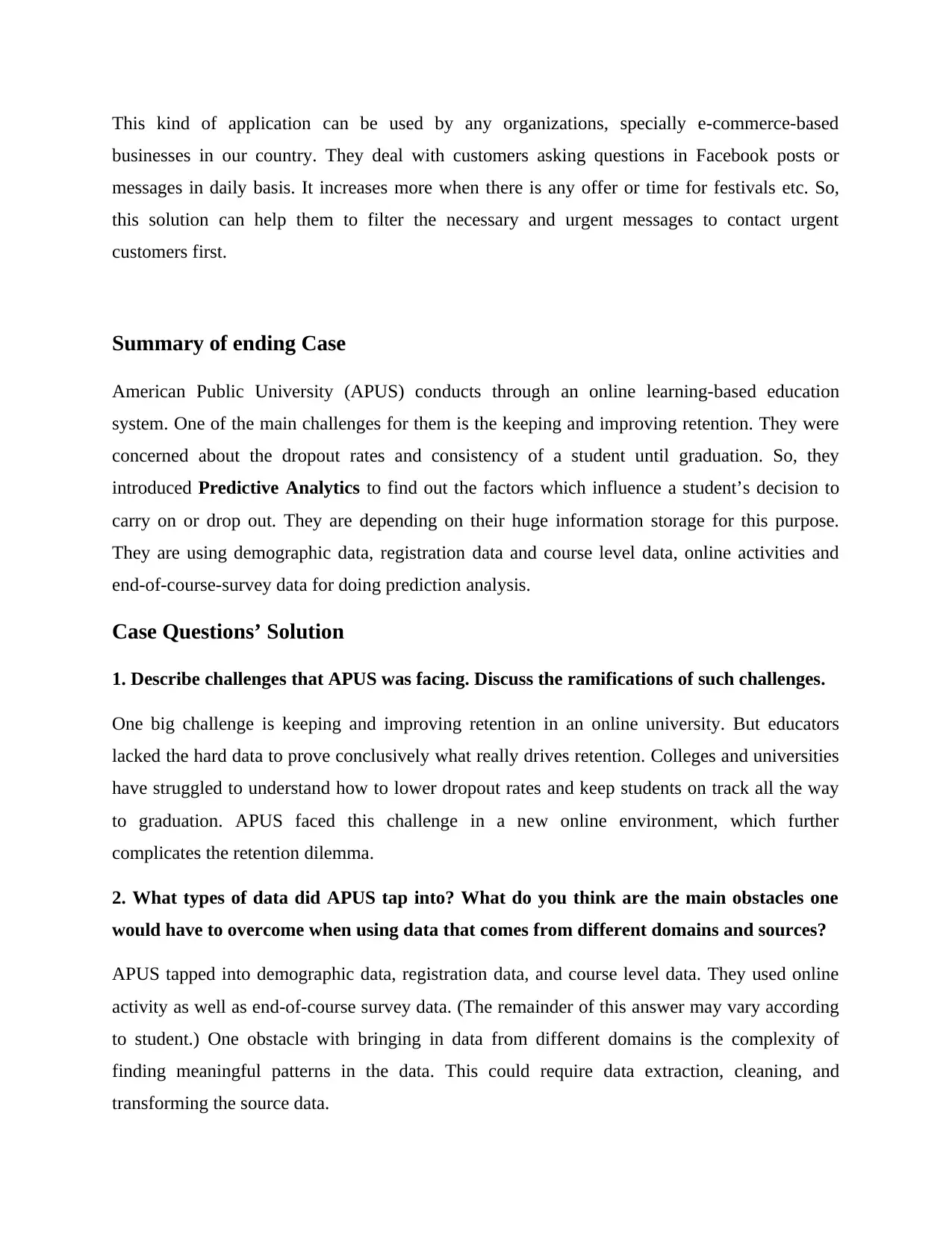
This kind of application can be used by any organizations, specially e-commerce-based
businesses in our country. They deal with customers asking questions in Facebook posts or
messages in daily basis. It increases more when there is any offer or time for festivals etc. So,
this solution can help them to filter the necessary and urgent messages to contact urgent
customers first.
Summary of ending Case
American Public University (APUS) conducts through an online learning-based education
system. One of the main challenges for them is the keeping and improving retention. They were
concerned about the dropout rates and consistency of a student until graduation. So, they
introduced Predictive Analytics to find out the factors which influence a student’s decision to
carry on or drop out. They are depending on their huge information storage for this purpose.
They are using demographic data, registration data and course level data, online activities and
end-of-course-survey data for doing prediction analysis.
Case Questions’ Solution
1. Describe challenges that APUS was facing. Discuss the ramifications of such challenges.
One big challenge is keeping and improving retention in an online university. But educators
lacked the hard data to prove conclusively what really drives retention. Colleges and universities
have struggled to understand how to lower dropout rates and keep students on track all the way
to graduation. APUS faced this challenge in a new online environment, which further
complicates the retention dilemma.
2. What types of data did APUS tap into? What do you think are the main obstacles one
would have to overcome when using data that comes from different domains and sources?
APUS tapped into demographic data, registration data, and course level data. They used online
activity as well as end-of-course survey data. (The remainder of this answer may vary according
to student.) One obstacle with bringing in data from different domains is the complexity of
finding meaningful patterns in the data. This could require data extraction, cleaning, and
transforming the source data.
businesses in our country. They deal with customers asking questions in Facebook posts or
messages in daily basis. It increases more when there is any offer or time for festivals etc. So,
this solution can help them to filter the necessary and urgent messages to contact urgent
customers first.
Summary of ending Case
American Public University (APUS) conducts through an online learning-based education
system. One of the main challenges for them is the keeping and improving retention. They were
concerned about the dropout rates and consistency of a student until graduation. So, they
introduced Predictive Analytics to find out the factors which influence a student’s decision to
carry on or drop out. They are depending on their huge information storage for this purpose.
They are using demographic data, registration data and course level data, online activities and
end-of-course-survey data for doing prediction analysis.
Case Questions’ Solution
1. Describe challenges that APUS was facing. Discuss the ramifications of such challenges.
One big challenge is keeping and improving retention in an online university. But educators
lacked the hard data to prove conclusively what really drives retention. Colleges and universities
have struggled to understand how to lower dropout rates and keep students on track all the way
to graduation. APUS faced this challenge in a new online environment, which further
complicates the retention dilemma.
2. What types of data did APUS tap into? What do you think are the main obstacles one
would have to overcome when using data that comes from different domains and sources?
APUS tapped into demographic data, registration data, and course level data. They used online
activity as well as end-of-course survey data. (The remainder of this answer may vary according
to student.) One obstacle with bringing in data from different domains is the complexity of
finding meaningful patterns in the data. This could require data extraction, cleaning, and
transforming the source data.
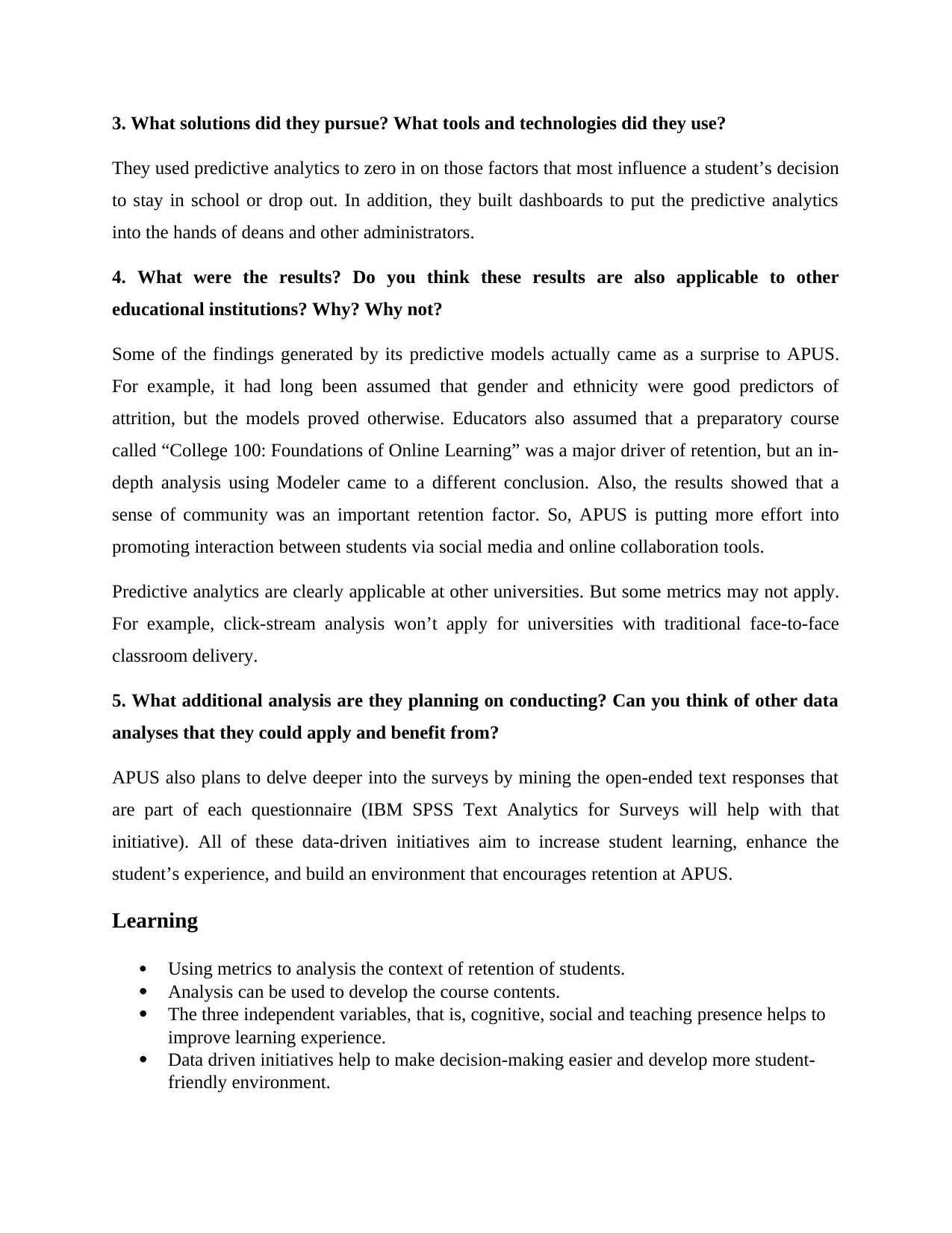
3. What solutions did they pursue? What tools and technologies did they use?
They used predictive analytics to zero in on those factors that most influence a student’s decision
to stay in school or drop out. In addition, they built dashboards to put the predictive analytics
into the hands of deans and other administrators.
4. What were the results? Do you think these results are also applicable to other
educational institutions? Why? Why not?
Some of the findings generated by its predictive models actually came as a surprise to APUS.
For example, it had long been assumed that gender and ethnicity were good predictors of
attrition, but the models proved otherwise. Educators also assumed that a preparatory course
called “College 100: Foundations of Online Learning” was a major driver of retention, but an in-
depth analysis using Modeler came to a different conclusion. Also, the results showed that a
sense of community was an important retention factor. So, APUS is putting more effort into
promoting interaction between students via social media and online collaboration tools.
Predictive analytics are clearly applicable at other universities. But some metrics may not apply.
For example, click-stream analysis won’t apply for universities with traditional face-to-face
classroom delivery.
5. What additional analysis are they planning on conducting? Can you think of other data
analyses that they could apply and benefit from?
APUS also plans to delve deeper into the surveys by mining the open-ended text responses that
are part of each questionnaire (IBM SPSS Text Analytics for Surveys will help with that
initiative). All of these data-driven initiatives aim to increase student learning, enhance the
student’s experience, and build an environment that encourages retention at APUS.
Learning
Using metrics to analysis the context of retention of students.
Analysis can be used to develop the course contents.
The three independent variables, that is, cognitive, social and teaching presence helps to
improve learning experience.
Data driven initiatives help to make decision-making easier and develop more student-
friendly environment.
They used predictive analytics to zero in on those factors that most influence a student’s decision
to stay in school or drop out. In addition, they built dashboards to put the predictive analytics
into the hands of deans and other administrators.
4. What were the results? Do you think these results are also applicable to other
educational institutions? Why? Why not?
Some of the findings generated by its predictive models actually came as a surprise to APUS.
For example, it had long been assumed that gender and ethnicity were good predictors of
attrition, but the models proved otherwise. Educators also assumed that a preparatory course
called “College 100: Foundations of Online Learning” was a major driver of retention, but an in-
depth analysis using Modeler came to a different conclusion. Also, the results showed that a
sense of community was an important retention factor. So, APUS is putting more effort into
promoting interaction between students via social media and online collaboration tools.
Predictive analytics are clearly applicable at other universities. But some metrics may not apply.
For example, click-stream analysis won’t apply for universities with traditional face-to-face
classroom delivery.
5. What additional analysis are they planning on conducting? Can you think of other data
analyses that they could apply and benefit from?
APUS also plans to delve deeper into the surveys by mining the open-ended text responses that
are part of each questionnaire (IBM SPSS Text Analytics for Surveys will help with that
initiative). All of these data-driven initiatives aim to increase student learning, enhance the
student’s experience, and build an environment that encourages retention at APUS.
Learning
Using metrics to analysis the context of retention of students.
Analysis can be used to develop the course contents.
The three independent variables, that is, cognitive, social and teaching presence helps to
improve learning experience.
Data driven initiatives help to make decision-making easier and develop more student-
friendly environment.
Paraphrase This Document
Need a fresh take? Get an instant paraphrase of this document with our AI Paraphraser
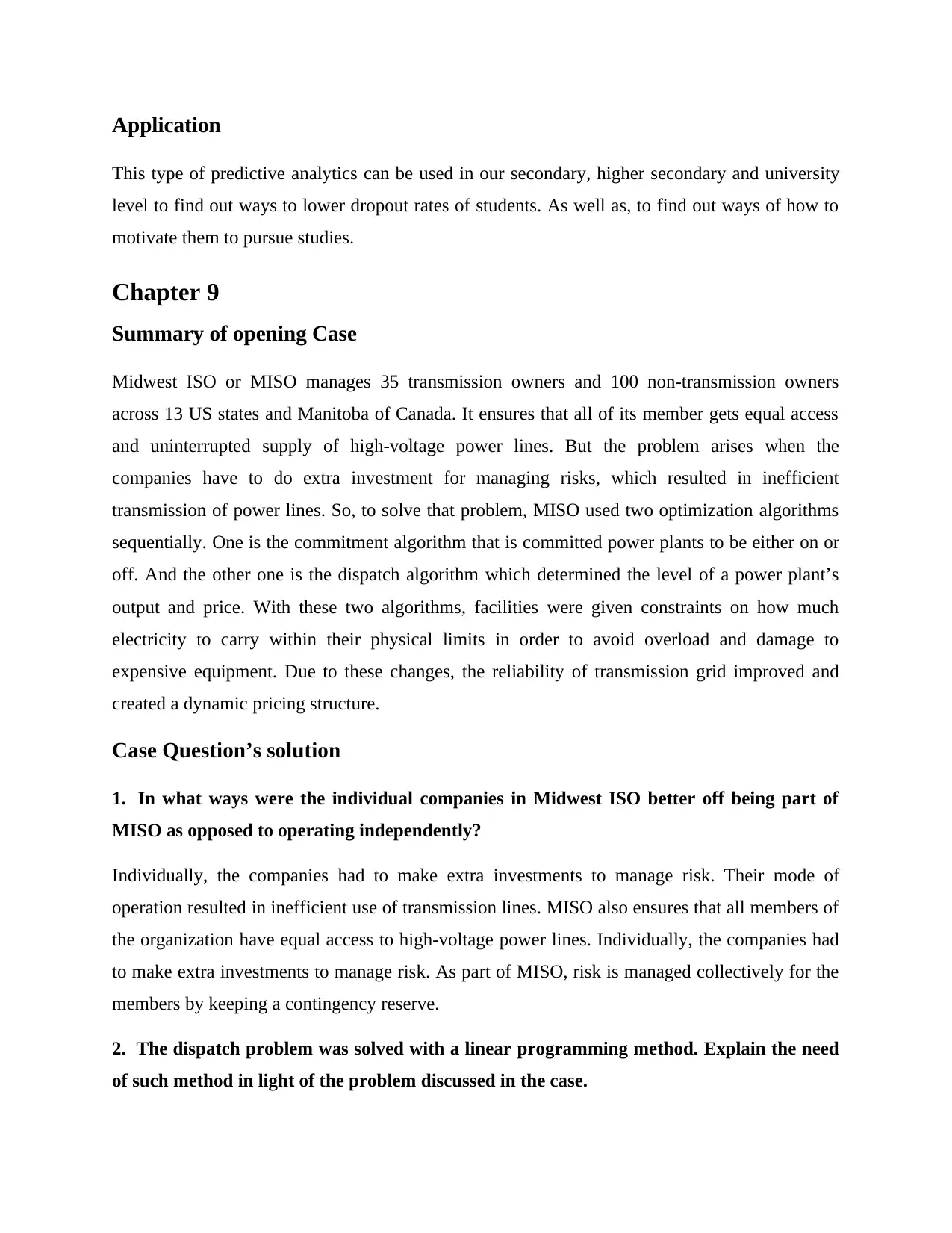
Application
This type of predictive analytics can be used in our secondary, higher secondary and university
level to find out ways to lower dropout rates of students. As well as, to find out ways of how to
motivate them to pursue studies.
Chapter 9
Summary of opening Case
Midwest ISO or MISO manages 35 transmission owners and 100 non-transmission owners
across 13 US states and Manitoba of Canada. It ensures that all of its member gets equal access
and uninterrupted supply of high-voltage power lines. But the problem arises when the
companies have to do extra investment for managing risks, which resulted in inefficient
transmission of power lines. So, to solve that problem, MISO used two optimization algorithms
sequentially. One is the commitment algorithm that is committed power plants to be either on or
off. And the other one is the dispatch algorithm which determined the level of a power plant’s
output and price. With these two algorithms, facilities were given constraints on how much
electricity to carry within their physical limits in order to avoid overload and damage to
expensive equipment. Due to these changes, the reliability of transmission grid improved and
created a dynamic pricing structure.
Case Question’s solution
1. In what ways were the individual companies in Midwest ISO better off being part of
MISO as opposed to operating independently?
Individually, the companies had to make extra investments to manage risk. Their mode of
operation resulted in inefficient use of transmission lines. MISO also ensures that all members of
the organization have equal access to high-voltage power lines. Individually, the companies had
to make extra investments to manage risk. As part of MISO, risk is managed collectively for the
members by keeping a contingency reserve.
2. The dispatch problem was solved with a linear programming method. Explain the need
of such method in light of the problem discussed in the case.
This type of predictive analytics can be used in our secondary, higher secondary and university
level to find out ways to lower dropout rates of students. As well as, to find out ways of how to
motivate them to pursue studies.
Chapter 9
Summary of opening Case
Midwest ISO or MISO manages 35 transmission owners and 100 non-transmission owners
across 13 US states and Manitoba of Canada. It ensures that all of its member gets equal access
and uninterrupted supply of high-voltage power lines. But the problem arises when the
companies have to do extra investment for managing risks, which resulted in inefficient
transmission of power lines. So, to solve that problem, MISO used two optimization algorithms
sequentially. One is the commitment algorithm that is committed power plants to be either on or
off. And the other one is the dispatch algorithm which determined the level of a power plant’s
output and price. With these two algorithms, facilities were given constraints on how much
electricity to carry within their physical limits in order to avoid overload and damage to
expensive equipment. Due to these changes, the reliability of transmission grid improved and
created a dynamic pricing structure.
Case Question’s solution
1. In what ways were the individual companies in Midwest ISO better off being part of
MISO as opposed to operating independently?
Individually, the companies had to make extra investments to manage risk. Their mode of
operation resulted in inefficient use of transmission lines. MISO also ensures that all members of
the organization have equal access to high-voltage power lines. Individually, the companies had
to make extra investments to manage risk. As part of MISO, risk is managed collectively for the
members by keeping a contingency reserve.
2. The dispatch problem was solved with a linear programming method. Explain the need
of such method in light of the problem discussed in the case.
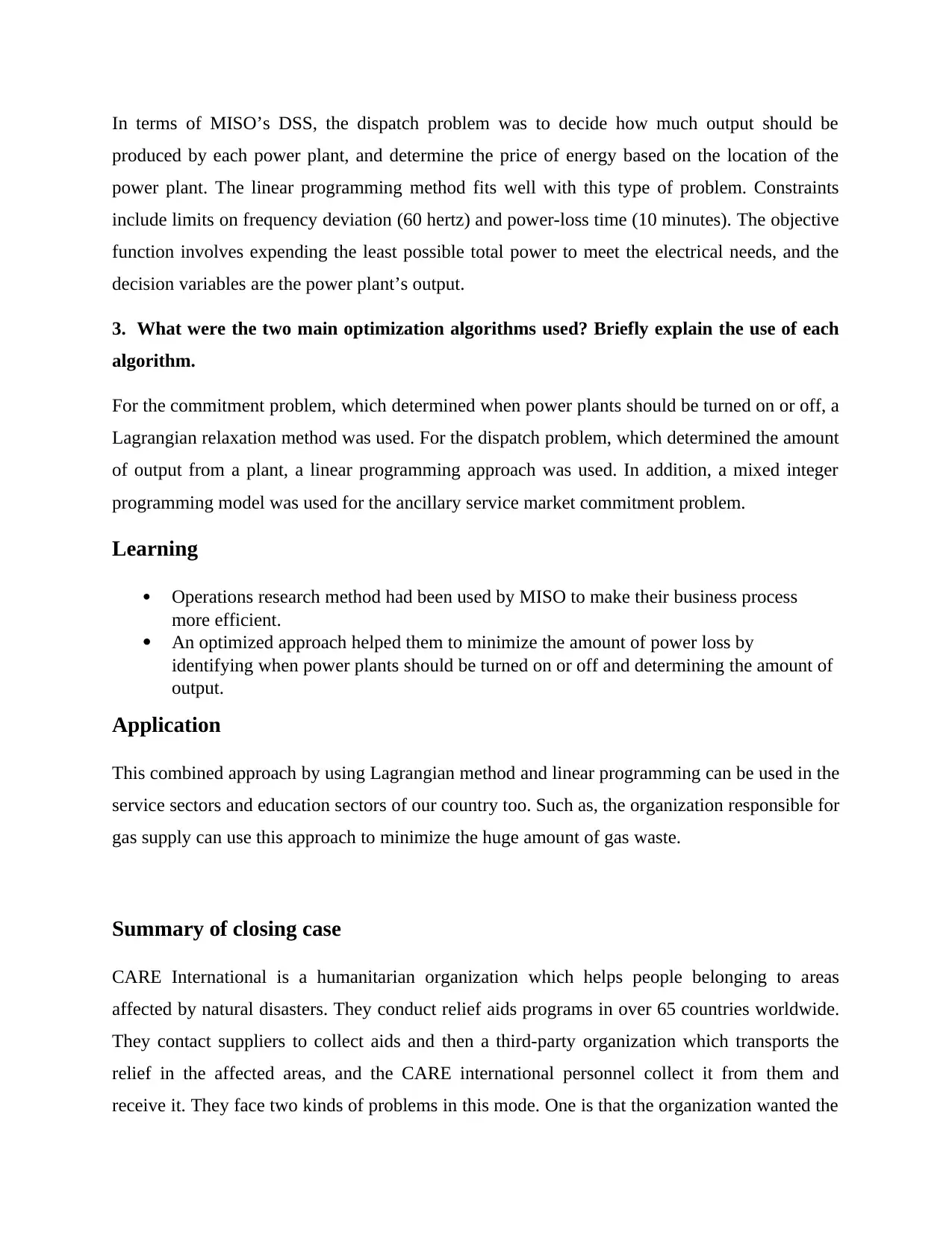
In terms of MISO’s DSS, the dispatch problem was to decide how much output should be
produced by each power plant, and determine the price of energy based on the location of the
power plant. The linear programming method fits well with this type of problem. Constraints
include limits on frequency deviation (60 hertz) and power-loss time (10 minutes). The objective
function involves expending the least possible total power to meet the electrical needs, and the
decision variables are the power plant’s output.
3. What were the two main optimization algorithms used? Briefly explain the use of each
algorithm.
For the commitment problem, which determined when power plants should be turned on or off, a
Lagrangian relaxation method was used. For the dispatch problem, which determined the amount
of output from a plant, a linear programming approach was used. In addition, a mixed integer
programming model was used for the ancillary service market commitment problem.
Learning
Operations research method had been used by MISO to make their business process
more efficient.
An optimized approach helped them to minimize the amount of power loss by
identifying when power plants should be turned on or off and determining the amount of
output.
Application
This combined approach by using Lagrangian method and linear programming can be used in the
service sectors and education sectors of our country too. Such as, the organization responsible for
gas supply can use this approach to minimize the huge amount of gas waste.
Summary of closing case
CARE International is a humanitarian organization which helps people belonging to areas
affected by natural disasters. They conduct relief aids programs in over 65 countries worldwide.
They contact suppliers to collect aids and then a third-party organization which transports the
relief in the affected areas, and the CARE international personnel collect it from them and
receive it. They face two kinds of problems in this mode. One is that the organization wanted the
produced by each power plant, and determine the price of energy based on the location of the
power plant. The linear programming method fits well with this type of problem. Constraints
include limits on frequency deviation (60 hertz) and power-loss time (10 minutes). The objective
function involves expending the least possible total power to meet the electrical needs, and the
decision variables are the power plant’s output.
3. What were the two main optimization algorithms used? Briefly explain the use of each
algorithm.
For the commitment problem, which determined when power plants should be turned on or off, a
Lagrangian relaxation method was used. For the dispatch problem, which determined the amount
of output from a plant, a linear programming approach was used. In addition, a mixed integer
programming model was used for the ancillary service market commitment problem.
Learning
Operations research method had been used by MISO to make their business process
more efficient.
An optimized approach helped them to minimize the amount of power loss by
identifying when power plants should be turned on or off and determining the amount of
output.
Application
This combined approach by using Lagrangian method and linear programming can be used in the
service sectors and education sectors of our country too. Such as, the organization responsible for
gas supply can use this approach to minimize the huge amount of gas waste.
Summary of closing case
CARE International is a humanitarian organization which helps people belonging to areas
affected by natural disasters. They conduct relief aids programs in over 65 countries worldwide.
They contact suppliers to collect aids and then a third-party organization which transports the
relief in the affected areas, and the CARE international personnel collect it from them and
receive it. They face two kinds of problems in this mode. One is that the organization wanted the
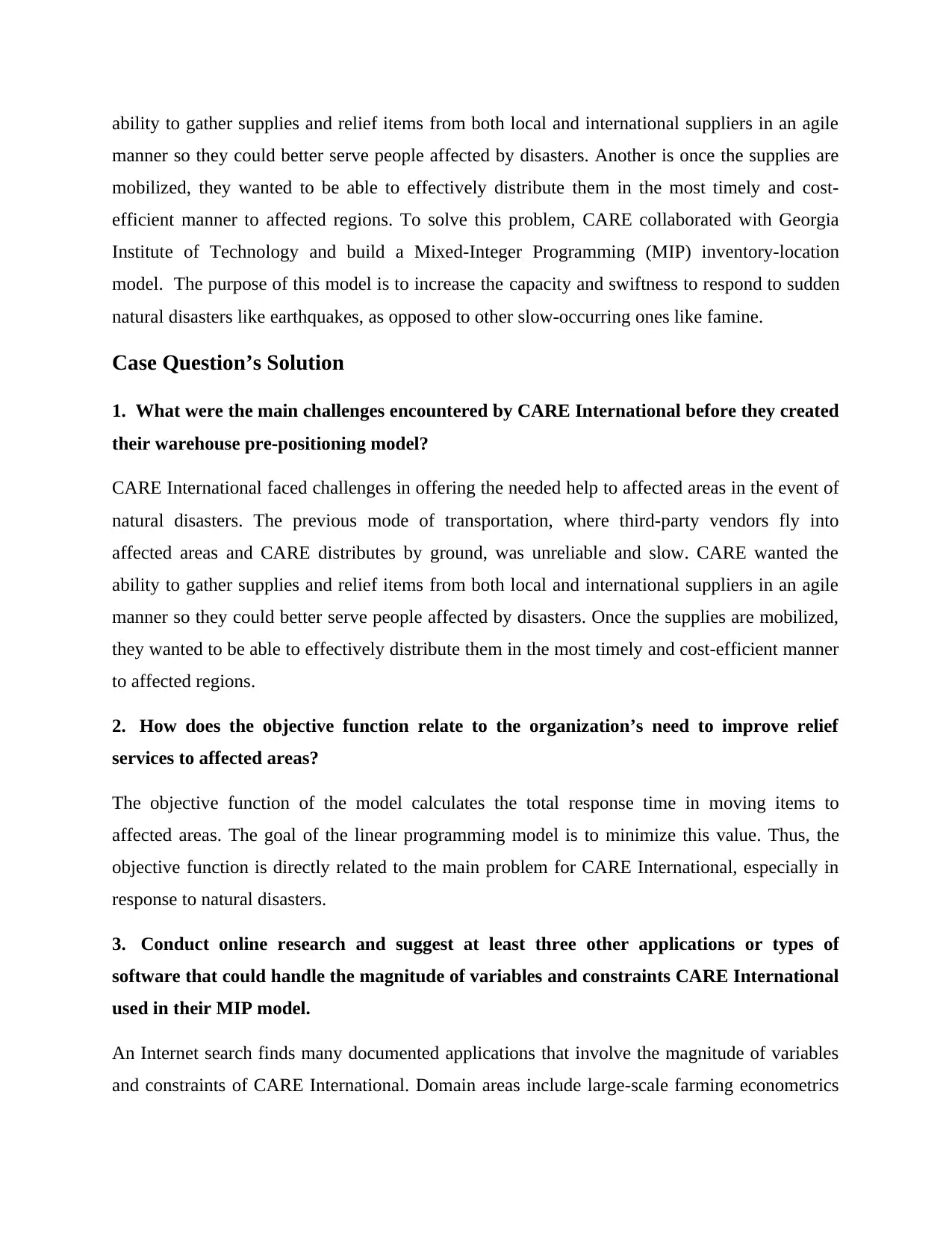
ability to gather supplies and relief items from both local and international suppliers in an agile
manner so they could better serve people affected by disasters. Another is once the supplies are
mobilized, they wanted to be able to effectively distribute them in the most timely and cost-
efficient manner to affected regions. To solve this problem, CARE collaborated with Georgia
Institute of Technology and build a Mixed-Integer Programming (MIP) inventory-location
model. The purpose of this model is to increase the capacity and swiftness to respond to sudden
natural disasters like earthquakes, as opposed to other slow-occurring ones like famine.
Case Question’s Solution
1. What were the main challenges encountered by CARE International before they created
their warehouse pre-positioning model?
CARE International faced challenges in offering the needed help to affected areas in the event of
natural disasters. The previous mode of transportation, where third-party vendors fly into
affected areas and CARE distributes by ground, was unreliable and slow. CARE wanted the
ability to gather supplies and relief items from both local and international suppliers in an agile
manner so they could better serve people affected by disasters. Once the supplies are mobilized,
they wanted to be able to effectively distribute them in the most timely and cost-efficient manner
to affected regions.
2. How does the objective function relate to the organization’s need to improve relief
services to affected areas?
The objective function of the model calculates the total response time in moving items to
affected areas. The goal of the linear programming model is to minimize this value. Thus, the
objective function is directly related to the main problem for CARE International, especially in
response to natural disasters.
3. Conduct online research and suggest at least three other applications or types of
software that could handle the magnitude of variables and constraints CARE International
used in their MIP model.
An Internet search finds many documented applications that involve the magnitude of variables
and constraints of CARE International. Domain areas include large-scale farming econometrics
manner so they could better serve people affected by disasters. Another is once the supplies are
mobilized, they wanted to be able to effectively distribute them in the most timely and cost-
efficient manner to affected regions. To solve this problem, CARE collaborated with Georgia
Institute of Technology and build a Mixed-Integer Programming (MIP) inventory-location
model. The purpose of this model is to increase the capacity and swiftness to respond to sudden
natural disasters like earthquakes, as opposed to other slow-occurring ones like famine.
Case Question’s Solution
1. What were the main challenges encountered by CARE International before they created
their warehouse pre-positioning model?
CARE International faced challenges in offering the needed help to affected areas in the event of
natural disasters. The previous mode of transportation, where third-party vendors fly into
affected areas and CARE distributes by ground, was unreliable and slow. CARE wanted the
ability to gather supplies and relief items from both local and international suppliers in an agile
manner so they could better serve people affected by disasters. Once the supplies are mobilized,
they wanted to be able to effectively distribute them in the most timely and cost-efficient manner
to affected regions.
2. How does the objective function relate to the organization’s need to improve relief
services to affected areas?
The objective function of the model calculates the total response time in moving items to
affected areas. The goal of the linear programming model is to minimize this value. Thus, the
objective function is directly related to the main problem for CARE International, especially in
response to natural disasters.
3. Conduct online research and suggest at least three other applications or types of
software that could handle the magnitude of variables and constraints CARE International
used in their MIP model.
An Internet search finds many documented applications that involve the magnitude of variables
and constraints of CARE International. Domain areas include large-scale farming econometrics
Secure Best Marks with AI Grader
Need help grading? Try our AI Grader for instant feedback on your assignments.
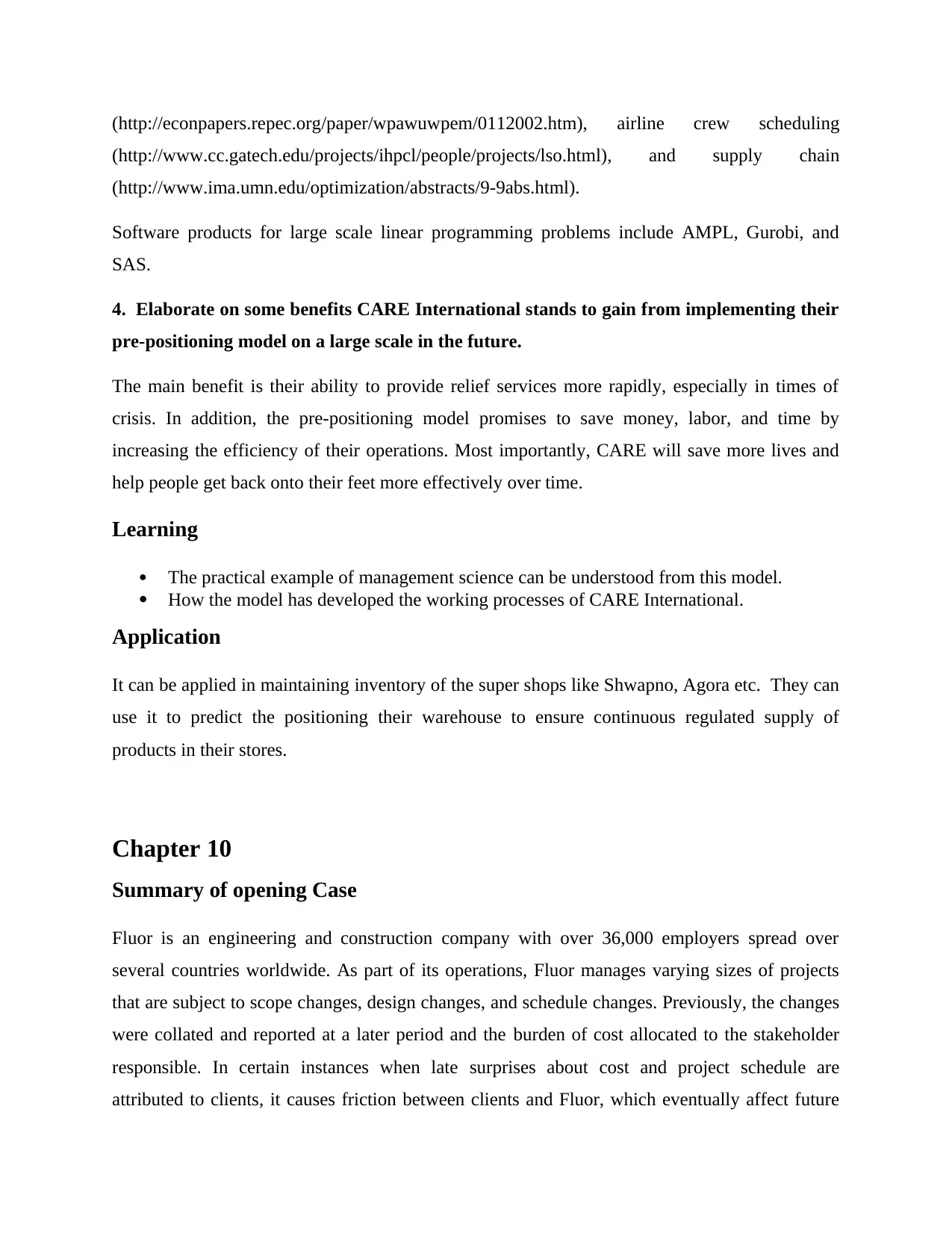
(http://econpapers.repec.org/paper/wpawuwpem/0112002.htm), airline crew scheduling
(http://www.cc.gatech.edu/projects/ihpcl/people/projects/lso.html), and supply chain
(http://www.ima.umn.edu/optimization/abstracts/9-9abs.html).
Software products for large scale linear programming problems include AMPL, Gurobi, and
SAS.
4. Elaborate on some benefits CARE International stands to gain from implementing their
pre-positioning model on a large scale in the future.
The main benefit is their ability to provide relief services more rapidly, especially in times of
crisis. In addition, the pre-positioning model promises to save money, labor, and time by
increasing the efficiency of their operations. Most importantly, CARE will save more lives and
help people get back onto their feet more effectively over time.
Learning
The practical example of management science can be understood from this model.
How the model has developed the working processes of CARE International.
Application
It can be applied in maintaining inventory of the super shops like Shwapno, Agora etc. They can
use it to predict the positioning their warehouse to ensure continuous regulated supply of
products in their stores.
Chapter 10
Summary of opening Case
Fluor is an engineering and construction company with over 36,000 employers spread over
several countries worldwide. As part of its operations, Fluor manages varying sizes of projects
that are subject to scope changes, design changes, and schedule changes. Previously, the changes
were collated and reported at a later period and the burden of cost allocated to the stakeholder
responsible. In certain instances when late surprises about cost and project schedule are
attributed to clients, it causes friction between clients and Fluor, which eventually affect future
(http://www.cc.gatech.edu/projects/ihpcl/people/projects/lso.html), and supply chain
(http://www.ima.umn.edu/optimization/abstracts/9-9abs.html).
Software products for large scale linear programming problems include AMPL, Gurobi, and
SAS.
4. Elaborate on some benefits CARE International stands to gain from implementing their
pre-positioning model on a large scale in the future.
The main benefit is their ability to provide relief services more rapidly, especially in times of
crisis. In addition, the pre-positioning model promises to save money, labor, and time by
increasing the efficiency of their operations. Most importantly, CARE will save more lives and
help people get back onto their feet more effectively over time.
Learning
The practical example of management science can be understood from this model.
How the model has developed the working processes of CARE International.
Application
It can be applied in maintaining inventory of the super shops like Shwapno, Agora etc. They can
use it to predict the positioning their warehouse to ensure continuous regulated supply of
products in their stores.
Chapter 10
Summary of opening Case
Fluor is an engineering and construction company with over 36,000 employers spread over
several countries worldwide. As part of its operations, Fluor manages varying sizes of projects
that are subject to scope changes, design changes, and schedule changes. Previously, the changes
were collated and reported at a later period and the burden of cost allocated to the stakeholder
responsible. In certain instances when late surprises about cost and project schedule are
attributed to clients, it causes friction between clients and Fluor, which eventually affect future
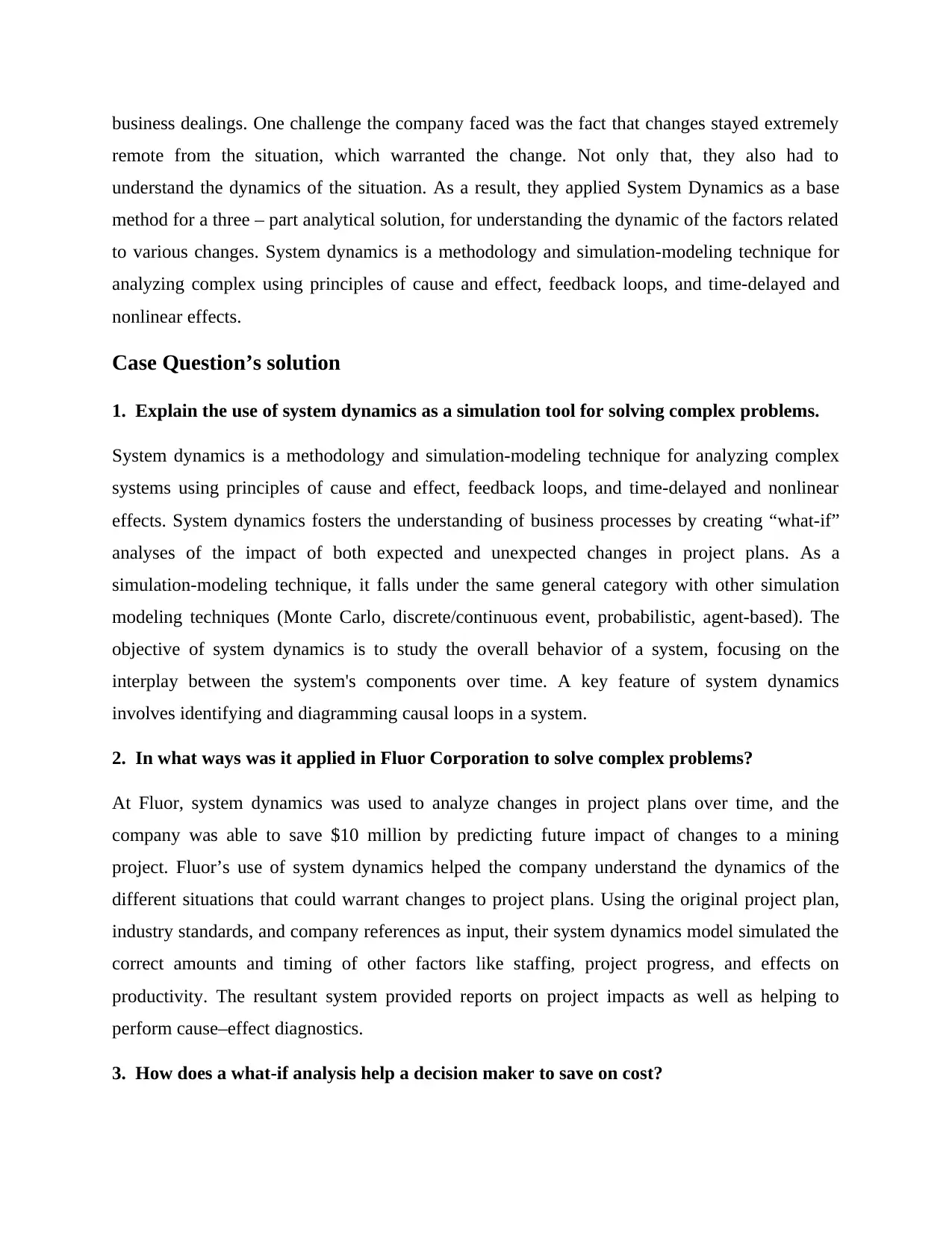
business dealings. One challenge the company faced was the fact that changes stayed extremely
remote from the situation, which warranted the change. Not only that, they also had to
understand the dynamics of the situation. As a result, they applied System Dynamics as a base
method for a three – part analytical solution, for understanding the dynamic of the factors related
to various changes. System dynamics is a methodology and simulation-modeling technique for
analyzing complex using principles of cause and effect, feedback loops, and time-delayed and
nonlinear effects.
Case Question’s solution
1. Explain the use of system dynamics as a simulation tool for solving complex problems.
System dynamics is a methodology and simulation-modeling technique for analyzing complex
systems using principles of cause and effect, feedback loops, and time-delayed and nonlinear
effects. System dynamics fosters the understanding of business processes by creating “what-if”
analyses of the impact of both expected and unexpected changes in project plans. As a
simulation-modeling technique, it falls under the same general category with other simulation
modeling techniques (Monte Carlo, discrete/continuous event, probabilistic, agent-based). The
objective of system dynamics is to study the overall behavior of a system, focusing on the
interplay between the system's components over time. A key feature of system dynamics
involves identifying and diagramming causal loops in a system.
2. In what ways was it applied in Fluor Corporation to solve complex problems?
At Fluor, system dynamics was used to analyze changes in project plans over time, and the
company was able to save $10 million by predicting future impact of changes to a mining
project. Fluor’s use of system dynamics helped the company understand the dynamics of the
different situations that could warrant changes to project plans. Using the original project plan,
industry standards, and company references as input, their system dynamics model simulated the
correct amounts and timing of other factors like staffing, project progress, and effects on
productivity. The resultant system provided reports on project impacts as well as helping to
perform cause–effect diagnostics.
3. How does a what-if analysis help a decision maker to save on cost?
remote from the situation, which warranted the change. Not only that, they also had to
understand the dynamics of the situation. As a result, they applied System Dynamics as a base
method for a three – part analytical solution, for understanding the dynamic of the factors related
to various changes. System dynamics is a methodology and simulation-modeling technique for
analyzing complex using principles of cause and effect, feedback loops, and time-delayed and
nonlinear effects.
Case Question’s solution
1. Explain the use of system dynamics as a simulation tool for solving complex problems.
System dynamics is a methodology and simulation-modeling technique for analyzing complex
systems using principles of cause and effect, feedback loops, and time-delayed and nonlinear
effects. System dynamics fosters the understanding of business processes by creating “what-if”
analyses of the impact of both expected and unexpected changes in project plans. As a
simulation-modeling technique, it falls under the same general category with other simulation
modeling techniques (Monte Carlo, discrete/continuous event, probabilistic, agent-based). The
objective of system dynamics is to study the overall behavior of a system, focusing on the
interplay between the system's components over time. A key feature of system dynamics
involves identifying and diagramming causal loops in a system.
2. In what ways was it applied in Fluor Corporation to solve complex problems?
At Fluor, system dynamics was used to analyze changes in project plans over time, and the
company was able to save $10 million by predicting future impact of changes to a mining
project. Fluor’s use of system dynamics helped the company understand the dynamics of the
different situations that could warrant changes to project plans. Using the original project plan,
industry standards, and company references as input, their system dynamics model simulated the
correct amounts and timing of other factors like staffing, project progress, and effects on
productivity. The resultant system provided reports on project impacts as well as helping to
perform cause–effect diagnostics.
3. How does a what-if analysis help a decision maker to save on cost?
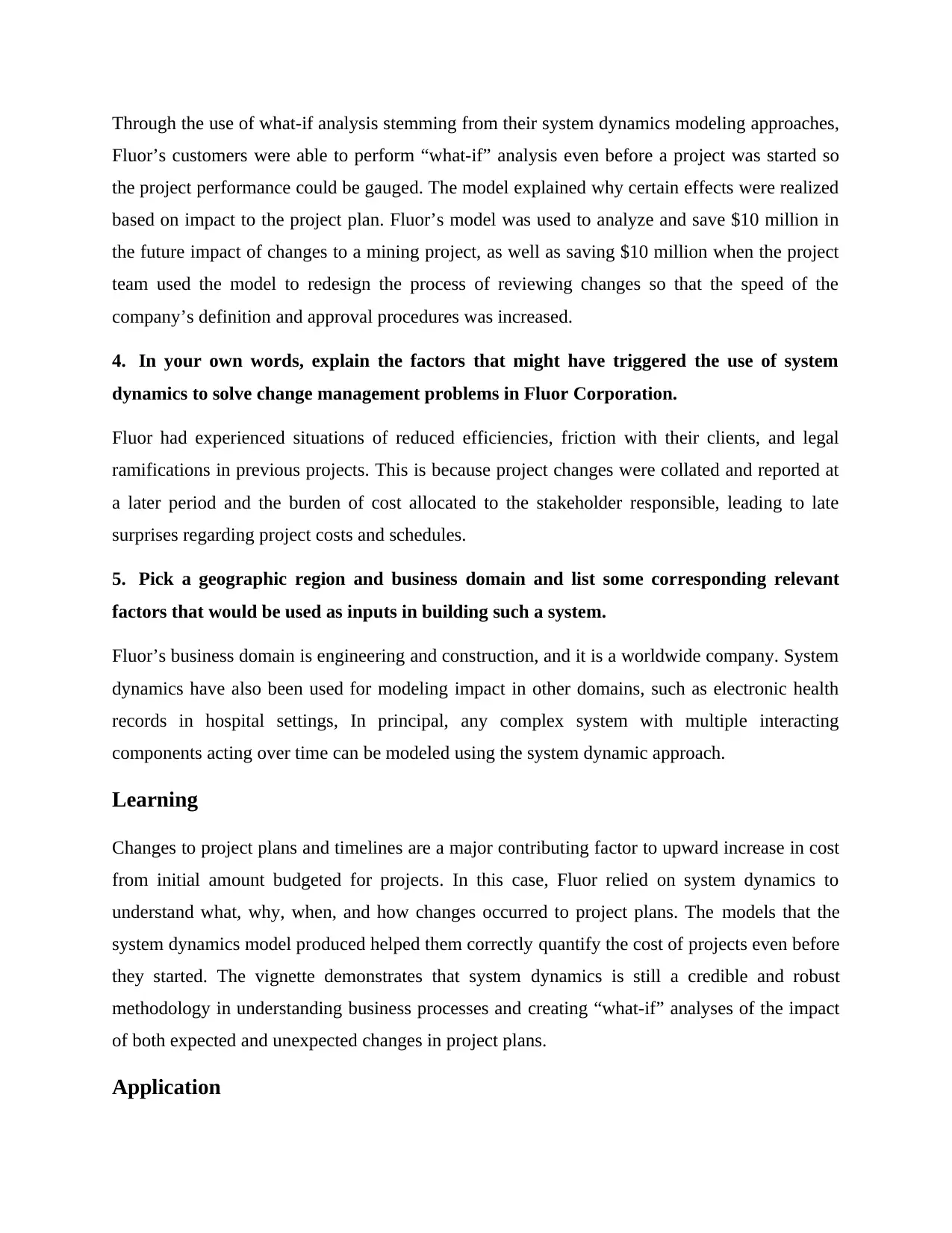
Through the use of what-if analysis stemming from their system dynamics modeling approaches,
Fluor’s customers were able to perform “what-if” analysis even before a project was started so
the project performance could be gauged. The model explained why certain effects were realized
based on impact to the project plan. Fluor’s model was used to analyze and save $10 million in
the future impact of changes to a mining project, as well as saving $10 million when the project
team used the model to redesign the process of reviewing changes so that the speed of the
company’s definition and approval procedures was increased.
4. In your own words, explain the factors that might have triggered the use of system
dynamics to solve change management problems in Fluor Corporation.
Fluor had experienced situations of reduced efficiencies, friction with their clients, and legal
ramifications in previous projects. This is because project changes were collated and reported at
a later period and the burden of cost allocated to the stakeholder responsible, leading to late
surprises regarding project costs and schedules.
5. Pick a geographic region and business domain and list some corresponding relevant
factors that would be used as inputs in building such a system.
Fluor’s business domain is engineering and construction, and it is a worldwide company. System
dynamics have also been used for modeling impact in other domains, such as electronic health
records in hospital settings, In principal, any complex system with multiple interacting
components acting over time can be modeled using the system dynamic approach.
Learning
Changes to project plans and timelines are a major contributing factor to upward increase in cost
from initial amount budgeted for projects. In this case, Fluor relied on system dynamics to
understand what, why, when, and how changes occurred to project plans. The models that the
system dynamics model produced helped them correctly quantify the cost of projects even before
they started. The vignette demonstrates that system dynamics is still a credible and robust
methodology in understanding business processes and creating “what-if” analyses of the impact
of both expected and unexpected changes in project plans.
Application
Fluor’s customers were able to perform “what-if” analysis even before a project was started so
the project performance could be gauged. The model explained why certain effects were realized
based on impact to the project plan. Fluor’s model was used to analyze and save $10 million in
the future impact of changes to a mining project, as well as saving $10 million when the project
team used the model to redesign the process of reviewing changes so that the speed of the
company’s definition and approval procedures was increased.
4. In your own words, explain the factors that might have triggered the use of system
dynamics to solve change management problems in Fluor Corporation.
Fluor had experienced situations of reduced efficiencies, friction with their clients, and legal
ramifications in previous projects. This is because project changes were collated and reported at
a later period and the burden of cost allocated to the stakeholder responsible, leading to late
surprises regarding project costs and schedules.
5. Pick a geographic region and business domain and list some corresponding relevant
factors that would be used as inputs in building such a system.
Fluor’s business domain is engineering and construction, and it is a worldwide company. System
dynamics have also been used for modeling impact in other domains, such as electronic health
records in hospital settings, In principal, any complex system with multiple interacting
components acting over time can be modeled using the system dynamic approach.
Learning
Changes to project plans and timelines are a major contributing factor to upward increase in cost
from initial amount budgeted for projects. In this case, Fluor relied on system dynamics to
understand what, why, when, and how changes occurred to project plans. The models that the
system dynamics model produced helped them correctly quantify the cost of projects even before
they started. The vignette demonstrates that system dynamics is still a credible and robust
methodology in understanding business processes and creating “what-if” analyses of the impact
of both expected and unexpected changes in project plans.
Application
Paraphrase This Document
Need a fresh take? Get an instant paraphrase of this document with our AI Paraphraser
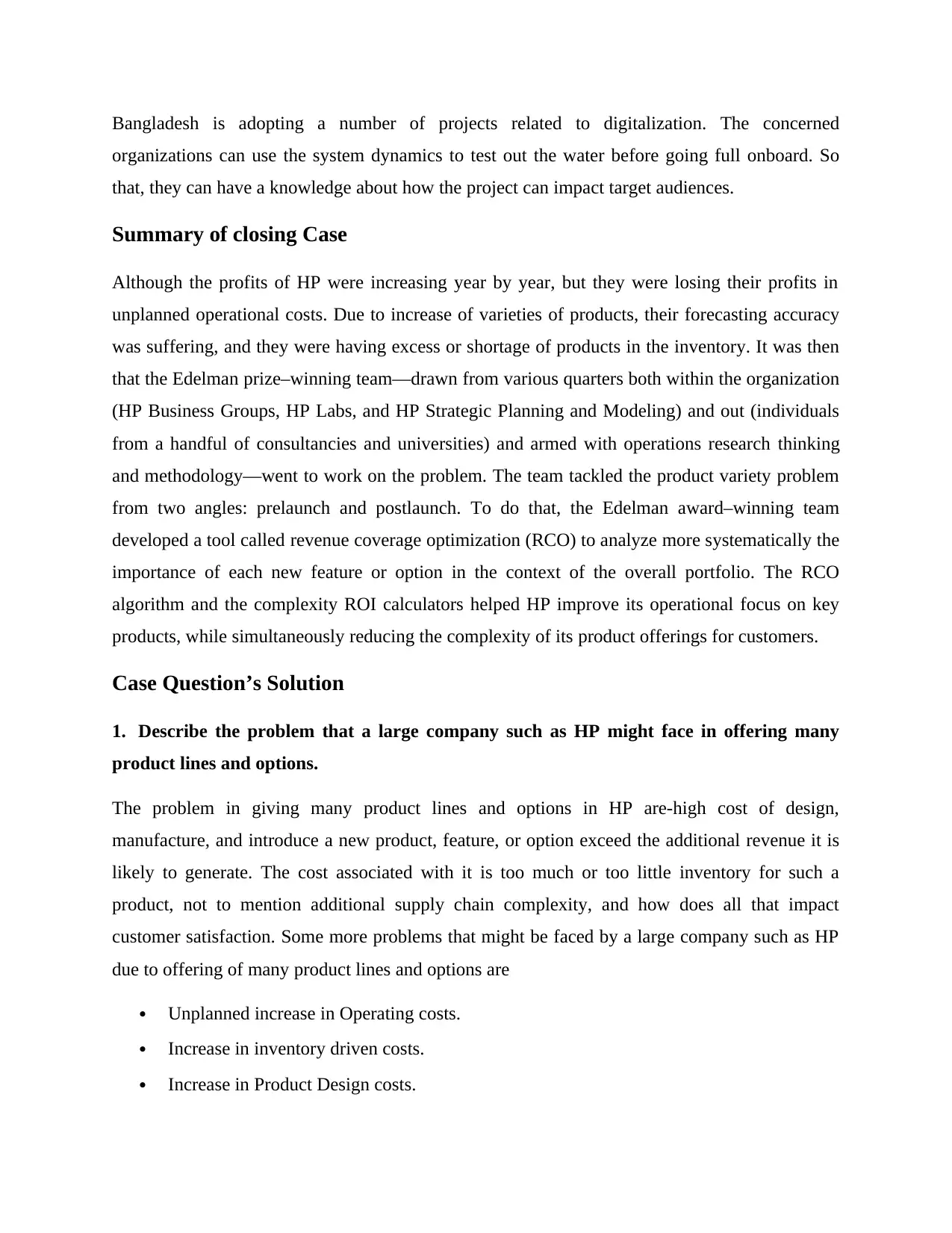
Bangladesh is adopting a number of projects related to digitalization. The concerned
organizations can use the system dynamics to test out the water before going full onboard. So
that, they can have a knowledge about how the project can impact target audiences.
Summary of closing Case
Although the profits of HP were increasing year by year, but they were losing their profits in
unplanned operational costs. Due to increase of varieties of products, their forecasting accuracy
was suffering, and they were having excess or shortage of products in the inventory. It was then
that the Edelman prize–winning team—drawn from various quarters both within the organization
(HP Business Groups, HP Labs, and HP Strategic Planning and Modeling) and out (individuals
from a handful of consultancies and universities) and armed with operations research thinking
and methodology—went to work on the problem. The team tackled the product variety problem
from two angles: prelaunch and postlaunch. To do that, the Edelman award–winning team
developed a tool called revenue coverage optimization (RCO) to analyze more systematically the
importance of each new feature or option in the context of the overall portfolio. The RCO
algorithm and the complexity ROI calculators helped HP improve its operational focus on key
products, while simultaneously reducing the complexity of its product offerings for customers.
Case Question’s Solution
1. Describe the problem that a large company such as HP might face in offering many
product lines and options.
The problem in giving many product lines and options in HP are-high cost of design,
manufacture, and introduce a new product, feature, or option exceed the additional revenue it is
likely to generate. The cost associated with it is too much or too little inventory for such a
product, not to mention additional supply chain complexity, and how does all that impact
customer satisfaction. Some more problems that might be faced by a large company such as HP
due to offering of many product lines and options are
Unplanned increase in Operating costs.
Increase in inventory driven costs.
Increase in Product Design costs.
organizations can use the system dynamics to test out the water before going full onboard. So
that, they can have a knowledge about how the project can impact target audiences.
Summary of closing Case
Although the profits of HP were increasing year by year, but they were losing their profits in
unplanned operational costs. Due to increase of varieties of products, their forecasting accuracy
was suffering, and they were having excess or shortage of products in the inventory. It was then
that the Edelman prize–winning team—drawn from various quarters both within the organization
(HP Business Groups, HP Labs, and HP Strategic Planning and Modeling) and out (individuals
from a handful of consultancies and universities) and armed with operations research thinking
and methodology—went to work on the problem. The team tackled the product variety problem
from two angles: prelaunch and postlaunch. To do that, the Edelman award–winning team
developed a tool called revenue coverage optimization (RCO) to analyze more systematically the
importance of each new feature or option in the context of the overall portfolio. The RCO
algorithm and the complexity ROI calculators helped HP improve its operational focus on key
products, while simultaneously reducing the complexity of its product offerings for customers.
Case Question’s Solution
1. Describe the problem that a large company such as HP might face in offering many
product lines and options.
The problem in giving many product lines and options in HP are-high cost of design,
manufacture, and introduce a new product, feature, or option exceed the additional revenue it is
likely to generate. The cost associated with it is too much or too little inventory for such a
product, not to mention additional supply chain complexity, and how does all that impact
customer satisfaction. Some more problems that might be faced by a large company such as HP
due to offering of many product lines and options are
Unplanned increase in Operating costs.
Increase in inventory driven costs.
Increase in Product Design costs.
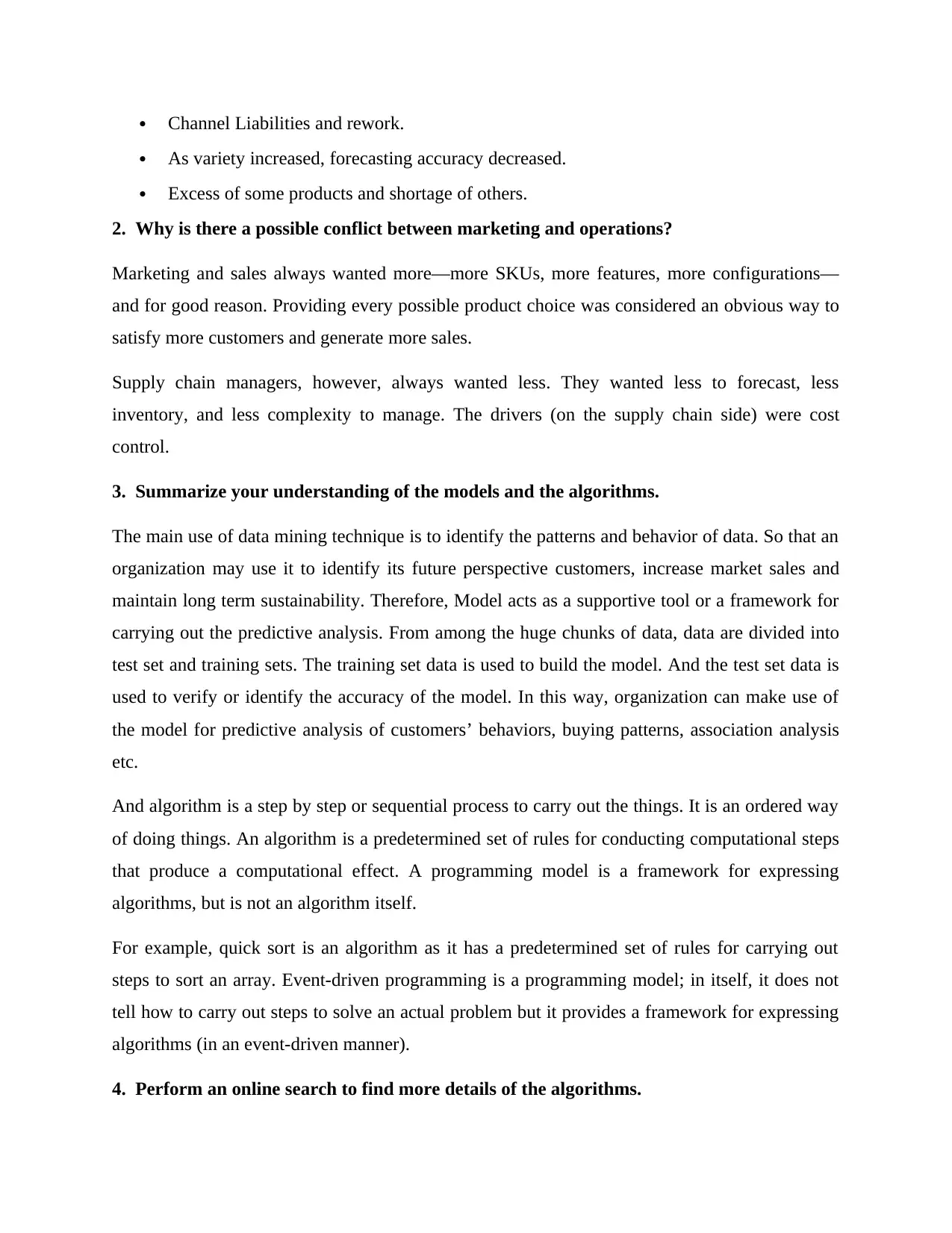
Channel Liabilities and rework.
As variety increased, forecasting accuracy decreased.
Excess of some products and shortage of others.
2. Why is there a possible conflict between marketing and operations?
Marketing and sales always wanted more—more SKUs, more features, more configurations—
and for good reason. Providing every possible product choice was considered an obvious way to
satisfy more customers and generate more sales.
Supply chain managers, however, always wanted less. They wanted less to forecast, less
inventory, and less complexity to manage. The drivers (on the supply chain side) were cost
control.
3. Summarize your understanding of the models and the algorithms.
The main use of data mining technique is to identify the patterns and behavior of data. So that an
organization may use it to identify its future perspective customers, increase market sales and
maintain long term sustainability. Therefore, Model acts as a supportive tool or a framework for
carrying out the predictive analysis. From among the huge chunks of data, data are divided into
test set and training sets. The training set data is used to build the model. And the test set data is
used to verify or identify the accuracy of the model. In this way, organization can make use of
the model for predictive analysis of customers’ behaviors, buying patterns, association analysis
etc.
And algorithm is a step by step or sequential process to carry out the things. It is an ordered way
of doing things. An algorithm is a predetermined set of rules for conducting computational steps
that produce a computational effect. A programming model is a framework for expressing
algorithms, but is not an algorithm itself.
For example, quick sort is an algorithm as it has a predetermined set of rules for carrying out
steps to sort an array. Event-driven programming is a programming model; in itself, it does not
tell how to carry out steps to solve an actual problem but it provides a framework for expressing
algorithms (in an event-driven manner).
4. Perform an online search to find more details of the algorithms.
As variety increased, forecasting accuracy decreased.
Excess of some products and shortage of others.
2. Why is there a possible conflict between marketing and operations?
Marketing and sales always wanted more—more SKUs, more features, more configurations—
and for good reason. Providing every possible product choice was considered an obvious way to
satisfy more customers and generate more sales.
Supply chain managers, however, always wanted less. They wanted less to forecast, less
inventory, and less complexity to manage. The drivers (on the supply chain side) were cost
control.
3. Summarize your understanding of the models and the algorithms.
The main use of data mining technique is to identify the patterns and behavior of data. So that an
organization may use it to identify its future perspective customers, increase market sales and
maintain long term sustainability. Therefore, Model acts as a supportive tool or a framework for
carrying out the predictive analysis. From among the huge chunks of data, data are divided into
test set and training sets. The training set data is used to build the model. And the test set data is
used to verify or identify the accuracy of the model. In this way, organization can make use of
the model for predictive analysis of customers’ behaviors, buying patterns, association analysis
etc.
And algorithm is a step by step or sequential process to carry out the things. It is an ordered way
of doing things. An algorithm is a predetermined set of rules for conducting computational steps
that produce a computational effect. A programming model is a framework for expressing
algorithms, but is not an algorithm itself.
For example, quick sort is an algorithm as it has a predetermined set of rules for carrying out
steps to sort an array. Event-driven programming is a programming model; in itself, it does not
tell how to carry out steps to solve an actual problem but it provides a framework for expressing
algorithms (in an event-driven manner).
4. Perform an online search to find more details of the algorithms.
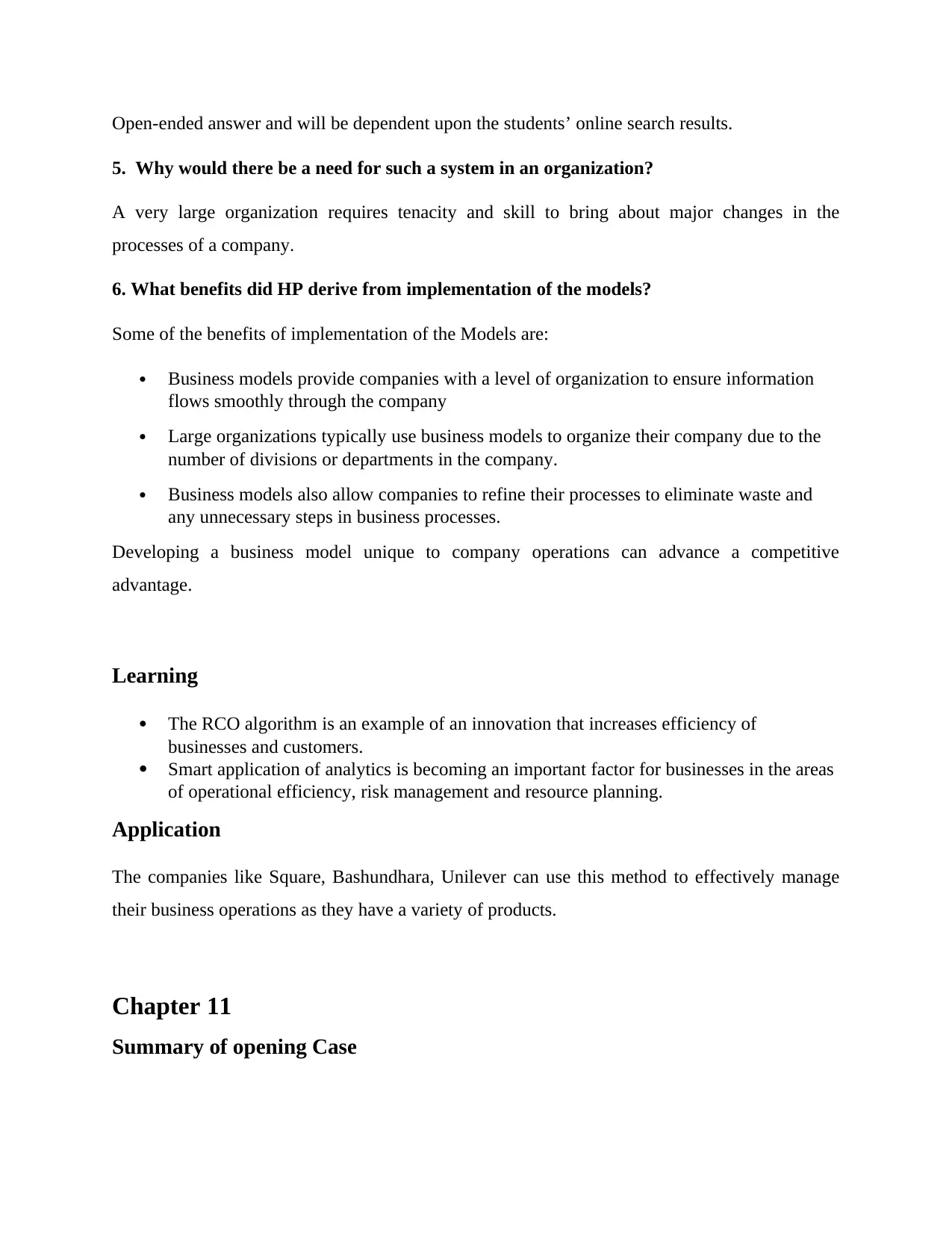
Open-ended answer and will be dependent upon the students’ online search results.
5. Why would there be a need for such a system in an organization?
A very large organization requires tenacity and skill to bring about major changes in the
processes of a company.
6. What benefits did HP derive from implementation of the models?
Some of the benefits of implementation of the Models are:
Business models provide companies with a level of organization to ensure information
flows smoothly through the company
Large organizations typically use business models to organize their company due to the
number of divisions or departments in the company.
Business models also allow companies to refine their processes to eliminate waste and
any unnecessary steps in business processes.
Developing a business model unique to company operations can advance a competitive
advantage.
Learning
The RCO algorithm is an example of an innovation that increases efficiency of
businesses and customers.
Smart application of analytics is becoming an important factor for businesses in the areas
of operational efficiency, risk management and resource planning.
Application
The companies like Square, Bashundhara, Unilever can use this method to effectively manage
their business operations as they have a variety of products.
Chapter 11
Summary of opening Case
5. Why would there be a need for such a system in an organization?
A very large organization requires tenacity and skill to bring about major changes in the
processes of a company.
6. What benefits did HP derive from implementation of the models?
Some of the benefits of implementation of the Models are:
Business models provide companies with a level of organization to ensure information
flows smoothly through the company
Large organizations typically use business models to organize their company due to the
number of divisions or departments in the company.
Business models also allow companies to refine their processes to eliminate waste and
any unnecessary steps in business processes.
Developing a business model unique to company operations can advance a competitive
advantage.
Learning
The RCO algorithm is an example of an innovation that increases efficiency of
businesses and customers.
Smart application of analytics is becoming an important factor for businesses in the areas
of operational efficiency, risk management and resource planning.
Application
The companies like Square, Bashundhara, Unilever can use this method to effectively manage
their business operations as they have a variety of products.
Chapter 11
Summary of opening Case
Secure Best Marks with AI Grader
Need help grading? Try our AI Grader for instant feedback on your assignments.
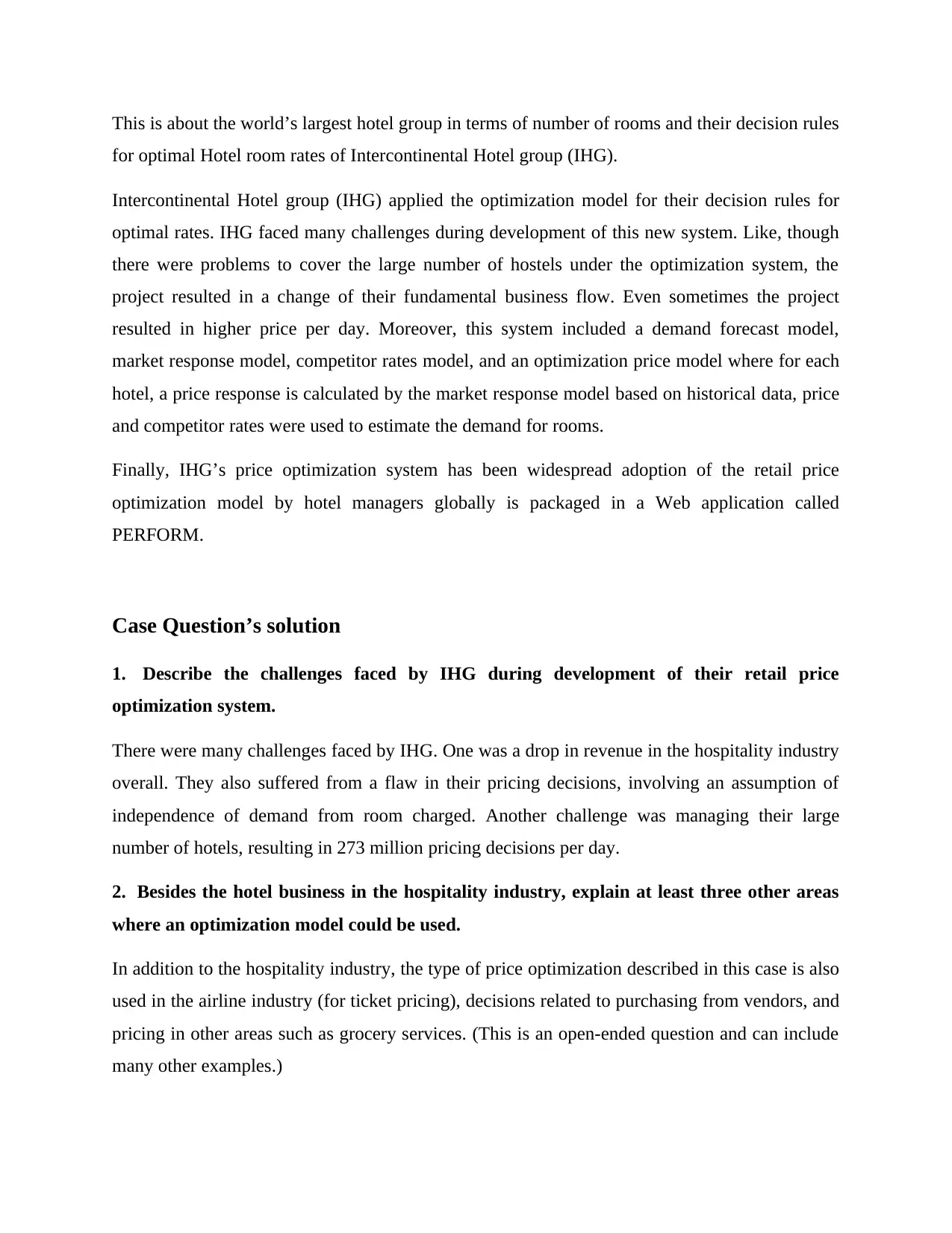
This is about the world’s largest hotel group in terms of number of rooms and their decision rules
for optimal Hotel room rates of Intercontinental Hotel group (IHG).
Intercontinental Hotel group (IHG) applied the optimization model for their decision rules for
optimal rates. IHG faced many challenges during development of this new system. Like, though
there were problems to cover the large number of hostels under the optimization system, the
project resulted in a change of their fundamental business flow. Even sometimes the project
resulted in higher price per day. Moreover, this system included a demand forecast model,
market response model, competitor rates model, and an optimization price model where for each
hotel, a price response is calculated by the market response model based on historical data, price
and competitor rates were used to estimate the demand for rooms.
Finally, IHG’s price optimization system has been widespread adoption of the retail price
optimization model by hotel managers globally is packaged in a Web application called
PERFORM.
Case Question’s solution
1. Describe the challenges faced by IHG during development of their retail price
optimization system.
There were many challenges faced by IHG. One was a drop in revenue in the hospitality industry
overall. They also suffered from a flaw in their pricing decisions, involving an assumption of
independence of demand from room charged. Another challenge was managing their large
number of hotels, resulting in 273 million pricing decisions per day.
2. Besides the hotel business in the hospitality industry, explain at least three other areas
where an optimization model could be used.
In addition to the hospitality industry, the type of price optimization described in this case is also
used in the airline industry (for ticket pricing), decisions related to purchasing from vendors, and
pricing in other areas such as grocery services. (This is an open-ended question and can include
many other examples.)
for optimal Hotel room rates of Intercontinental Hotel group (IHG).
Intercontinental Hotel group (IHG) applied the optimization model for their decision rules for
optimal rates. IHG faced many challenges during development of this new system. Like, though
there were problems to cover the large number of hostels under the optimization system, the
project resulted in a change of their fundamental business flow. Even sometimes the project
resulted in higher price per day. Moreover, this system included a demand forecast model,
market response model, competitor rates model, and an optimization price model where for each
hotel, a price response is calculated by the market response model based on historical data, price
and competitor rates were used to estimate the demand for rooms.
Finally, IHG’s price optimization system has been widespread adoption of the retail price
optimization model by hotel managers globally is packaged in a Web application called
PERFORM.
Case Question’s solution
1. Describe the challenges faced by IHG during development of their retail price
optimization system.
There were many challenges faced by IHG. One was a drop in revenue in the hospitality industry
overall. They also suffered from a flaw in their pricing decisions, involving an assumption of
independence of demand from room charged. Another challenge was managing their large
number of hotels, resulting in 273 million pricing decisions per day.
2. Besides the hotel business in the hospitality industry, explain at least three other areas
where an optimization model could be used.
In addition to the hospitality industry, the type of price optimization described in this case is also
used in the airline industry (for ticket pricing), decisions related to purchasing from vendors, and
pricing in other areas such as grocery services. (This is an open-ended question and can include
many other examples.)
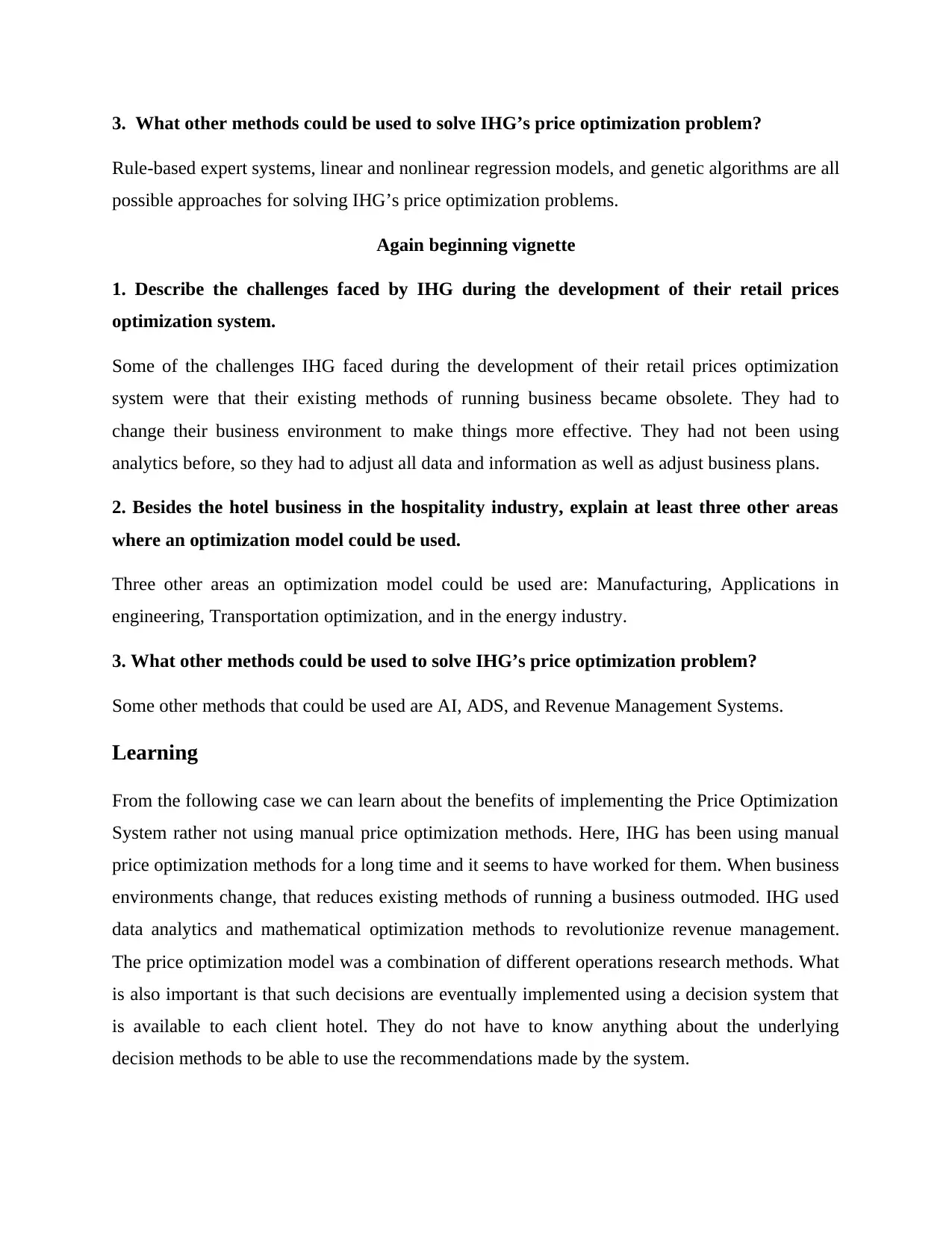
3. What other methods could be used to solve IHG’s price optimization problem?
Rule-based expert systems, linear and nonlinear regression models, and genetic algorithms are all
possible approaches for solving IHG’s price optimization problems.
Again beginning vignette
1. Describe the challenges faced by IHG during the development of their retail prices
optimization system.
Some of the challenges IHG faced during the development of their retail prices optimization
system were that their existing methods of running business became obsolete. They had to
change their business environment to make things more effective. They had not been using
analytics before, so they had to adjust all data and information as well as adjust business plans.
2. Besides the hotel business in the hospitality industry, explain at least three other areas
where an optimization model could be used.
Three other areas an optimization model could be used are: Manufacturing, Applications in
engineering, Transportation optimization, and in the energy industry.
3. What other methods could be used to solve IHG’s price optimization problem?
Some other methods that could be used are AI, ADS, and Revenue Management Systems.
Learning
From the following case we can learn about the benefits of implementing the Price Optimization
System rather not using manual price optimization methods. Here, IHG has been using manual
price optimization methods for a long time and it seems to have worked for them. When business
environments change, that reduces existing methods of running a business outmoded. IHG used
data analytics and mathematical optimization methods to revolutionize revenue management.
The price optimization model was a combination of different operations research methods. What
is also important is that such decisions are eventually implemented using a decision system that
is available to each client hotel. They do not have to know anything about the underlying
decision methods to be able to use the recommendations made by the system.
Rule-based expert systems, linear and nonlinear regression models, and genetic algorithms are all
possible approaches for solving IHG’s price optimization problems.
Again beginning vignette
1. Describe the challenges faced by IHG during the development of their retail prices
optimization system.
Some of the challenges IHG faced during the development of their retail prices optimization
system were that their existing methods of running business became obsolete. They had to
change their business environment to make things more effective. They had not been using
analytics before, so they had to adjust all data and information as well as adjust business plans.
2. Besides the hotel business in the hospitality industry, explain at least three other areas
where an optimization model could be used.
Three other areas an optimization model could be used are: Manufacturing, Applications in
engineering, Transportation optimization, and in the energy industry.
3. What other methods could be used to solve IHG’s price optimization problem?
Some other methods that could be used are AI, ADS, and Revenue Management Systems.
Learning
From the following case we can learn about the benefits of implementing the Price Optimization
System rather not using manual price optimization methods. Here, IHG has been using manual
price optimization methods for a long time and it seems to have worked for them. When business
environments change, that reduces existing methods of running a business outmoded. IHG used
data analytics and mathematical optimization methods to revolutionize revenue management.
The price optimization model was a combination of different operations research methods. What
is also important is that such decisions are eventually implemented using a decision system that
is available to each client hotel. They do not have to know anything about the underlying
decision methods to be able to use the recommendations made by the system.
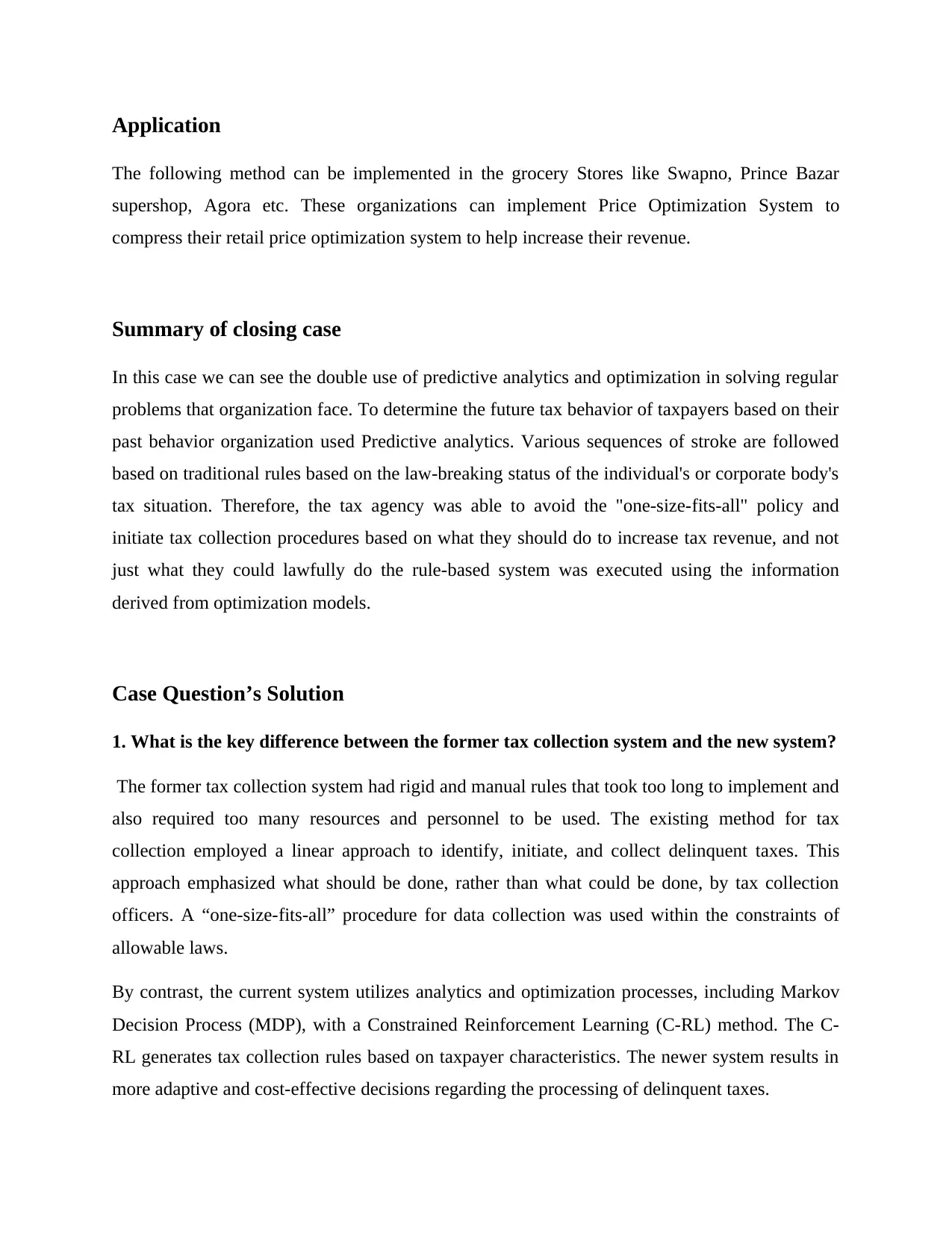
Application
The following method can be implemented in the grocery Stores like Swapno, Prince Bazar
supershop, Agora etc. These organizations can implement Price Optimization System to
compress their retail price optimization system to help increase their revenue.
Summary of closing case
In this case we can see the double use of predictive analytics and optimization in solving regular
problems that organization face. To determine the future tax behavior of taxpayers based on their
past behavior organization used Predictive analytics. Various sequences of stroke are followed
based on traditional rules based on the law-breaking status of the individual's or corporate body's
tax situation. Therefore, the tax agency was able to avoid the "one-size-fits-all" policy and
initiate tax collection procedures based on what they should do to increase tax revenue, and not
just what they could lawfully do the rule-based system was executed using the information
derived from optimization models.
Case Question’s Solution
1. What is the key difference between the former tax collection system and the new system?
The former tax collection system had rigid and manual rules that took too long to implement and
also required too many resources and personnel to be used. The existing method for tax
collection employed a linear approach to identify, initiate, and collect delinquent taxes. This
approach emphasized what should be done, rather than what could be done, by tax collection
officers. A “one-size-fits-all” procedure for data collection was used within the constraints of
allowable laws.
By contrast, the current system utilizes analytics and optimization processes, including Markov
Decision Process (MDP), with a Constrained Reinforcement Learning (C-RL) method. The C-
RL generates tax collection rules based on taxpayer characteristics. The newer system results in
more adaptive and cost-effective decisions regarding the processing of delinquent taxes.
The following method can be implemented in the grocery Stores like Swapno, Prince Bazar
supershop, Agora etc. These organizations can implement Price Optimization System to
compress their retail price optimization system to help increase their revenue.
Summary of closing case
In this case we can see the double use of predictive analytics and optimization in solving regular
problems that organization face. To determine the future tax behavior of taxpayers based on their
past behavior organization used Predictive analytics. Various sequences of stroke are followed
based on traditional rules based on the law-breaking status of the individual's or corporate body's
tax situation. Therefore, the tax agency was able to avoid the "one-size-fits-all" policy and
initiate tax collection procedures based on what they should do to increase tax revenue, and not
just what they could lawfully do the rule-based system was executed using the information
derived from optimization models.
Case Question’s Solution
1. What is the key difference between the former tax collection system and the new system?
The former tax collection system had rigid and manual rules that took too long to implement and
also required too many resources and personnel to be used. The existing method for tax
collection employed a linear approach to identify, initiate, and collect delinquent taxes. This
approach emphasized what should be done, rather than what could be done, by tax collection
officers. A “one-size-fits-all” procedure for data collection was used within the constraints of
allowable laws.
By contrast, the current system utilizes analytics and optimization processes, including Markov
Decision Process (MDP), with a Constrained Reinforcement Learning (C-RL) method. The C-
RL generates tax collection rules based on taxpayer characteristics. The newer system results in
more adaptive and cost-effective decisions regarding the processing of delinquent taxes.
Paraphrase This Document
Need a fresh take? Get an instant paraphrase of this document with our AI Paraphraser
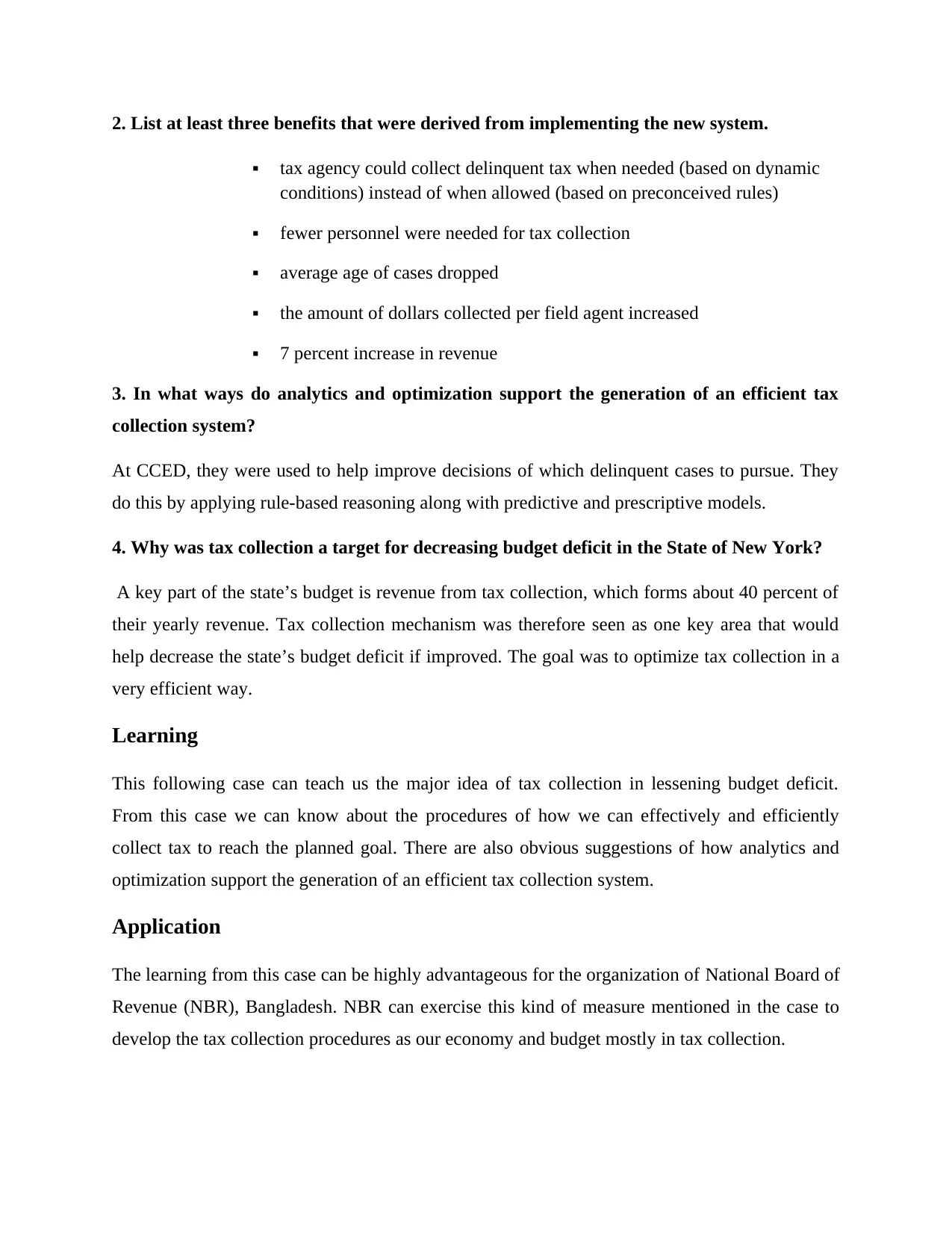
2. List at least three benefits that were derived from implementing the new system.
tax agency could collect delinquent tax when needed (based on dynamic
conditions) instead of when allowed (based on preconceived rules)
fewer personnel were needed for tax collection
average age of cases dropped
the amount of dollars collected per field agent increased
7 percent increase in revenue
3. In what ways do analytics and optimization support the generation of an efficient tax
collection system?
At CCED, they were used to help improve decisions of which delinquent cases to pursue. They
do this by applying rule-based reasoning along with predictive and prescriptive models.
4. Why was tax collection a target for decreasing budget deficit in the State of New York?
A key part of the state’s budget is revenue from tax collection, which forms about 40 percent of
their yearly revenue. Tax collection mechanism was therefore seen as one key area that would
help decrease the state’s budget deficit if improved. The goal was to optimize tax collection in a
very efficient way.
Learning
This following case can teach us the major idea of tax collection in lessening budget deficit.
From this case we can know about the procedures of how we can effectively and efficiently
collect tax to reach the planned goal. There are also obvious suggestions of how analytics and
optimization support the generation of an efficient tax collection system.
Application
The learning from this case can be highly advantageous for the organization of National Board of
Revenue (NBR), Bangladesh. NBR can exercise this kind of measure mentioned in the case to
develop the tax collection procedures as our economy and budget mostly in tax collection.
tax agency could collect delinquent tax when needed (based on dynamic
conditions) instead of when allowed (based on preconceived rules)
fewer personnel were needed for tax collection
average age of cases dropped
the amount of dollars collected per field agent increased
7 percent increase in revenue
3. In what ways do analytics and optimization support the generation of an efficient tax
collection system?
At CCED, they were used to help improve decisions of which delinquent cases to pursue. They
do this by applying rule-based reasoning along with predictive and prescriptive models.
4. Why was tax collection a target for decreasing budget deficit in the State of New York?
A key part of the state’s budget is revenue from tax collection, which forms about 40 percent of
their yearly revenue. Tax collection mechanism was therefore seen as one key area that would
help decrease the state’s budget deficit if improved. The goal was to optimize tax collection in a
very efficient way.
Learning
This following case can teach us the major idea of tax collection in lessening budget deficit.
From this case we can know about the procedures of how we can effectively and efficiently
collect tax to reach the planned goal. There are also obvious suggestions of how analytics and
optimization support the generation of an efficient tax collection system.
Application
The learning from this case can be highly advantageous for the organization of National Board of
Revenue (NBR), Bangladesh. NBR can exercise this kind of measure mentioned in the case to
develop the tax collection procedures as our economy and budget mostly in tax collection.
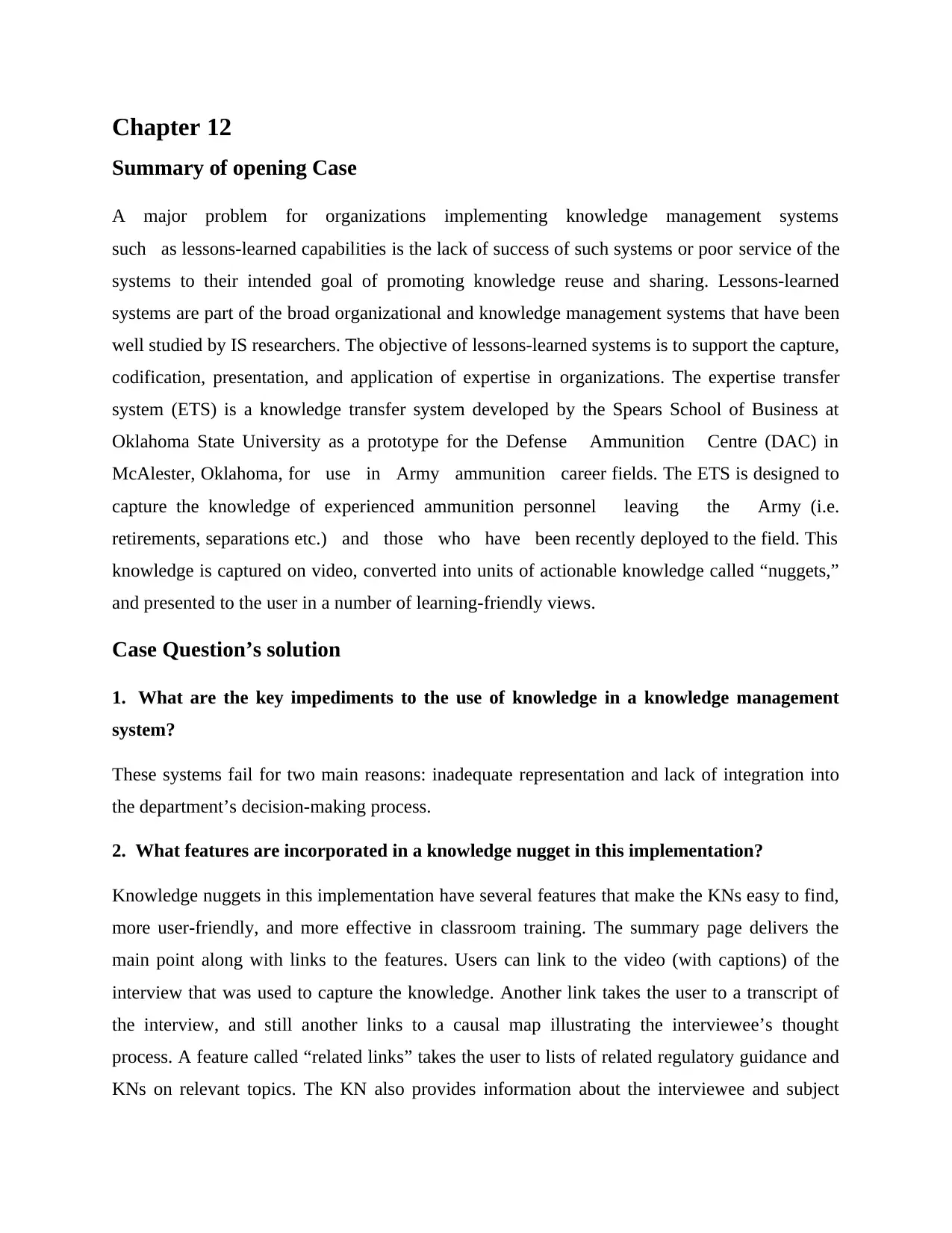
Chapter 12
Summary of opening Case
A major problem for organizations implementing knowledge management systems
such as lessons-learned capabilities is the lack of success of such systems or poor service of the
systems to their intended goal of promoting knowledge reuse and sharing. Lessons-learned
systems are part of the broad organizational and knowledge management systems that have been
well studied by IS researchers. The objective of lessons-learned systems is to support the capture,
codification, presentation, and application of expertise in organizations. The expertise transfer
system (ETS) is a knowledge transfer system developed by the Spears School of Business at
Oklahoma State University as a prototype for the Defense Ammunition Centre (DAC) in
McAlester, Oklahoma, for use in Army ammunition career fields. The ETS is designed to
capture the knowledge of experienced ammunition personnel leaving the Army (i.e.
retirements, separations etc.) and those who have been recently deployed to the field. This
knowledge is captured on video, converted into units of actionable knowledge called “nuggets,”
and presented to the user in a number of learning-friendly views.
Case Question’s solution
1. What are the key impediments to the use of knowledge in a knowledge management
system?
These systems fail for two main reasons: inadequate representation and lack of integration into
the department’s decision-making process.
2. What features are incorporated in a knowledge nugget in this implementation?
Knowledge nuggets in this implementation have several features that make the KNs easy to find,
more user-friendly, and more effective in classroom training. The summary page delivers the
main point along with links to the features. Users can link to the video (with captions) of the
interview that was used to capture the knowledge. Another link takes the user to a transcript of
the interview, and still another links to a causal map illustrating the interviewee’s thought
process. A feature called “related links” takes the user to lists of related regulatory guidance and
KNs on relevant topics. The KN also provides information about the interviewee and subject
Summary of opening Case
A major problem for organizations implementing knowledge management systems
such as lessons-learned capabilities is the lack of success of such systems or poor service of the
systems to their intended goal of promoting knowledge reuse and sharing. Lessons-learned
systems are part of the broad organizational and knowledge management systems that have been
well studied by IS researchers. The objective of lessons-learned systems is to support the capture,
codification, presentation, and application of expertise in organizations. The expertise transfer
system (ETS) is a knowledge transfer system developed by the Spears School of Business at
Oklahoma State University as a prototype for the Defense Ammunition Centre (DAC) in
McAlester, Oklahoma, for use in Army ammunition career fields. The ETS is designed to
capture the knowledge of experienced ammunition personnel leaving the Army (i.e.
retirements, separations etc.) and those who have been recently deployed to the field. This
knowledge is captured on video, converted into units of actionable knowledge called “nuggets,”
and presented to the user in a number of learning-friendly views.
Case Question’s solution
1. What are the key impediments to the use of knowledge in a knowledge management
system?
These systems fail for two main reasons: inadequate representation and lack of integration into
the department’s decision-making process.
2. What features are incorporated in a knowledge nugget in this implementation?
Knowledge nuggets in this implementation have several features that make the KNs easy to find,
more user-friendly, and more effective in classroom training. The summary page delivers the
main point along with links to the features. Users can link to the video (with captions) of the
interview that was used to capture the knowledge. Another link takes the user to a transcript of
the interview, and still another links to a causal map illustrating the interviewee’s thought
process. A feature called “related links” takes the user to lists of related regulatory guidance and
KNs on relevant topics. The KN also provides information about the interviewee and subject
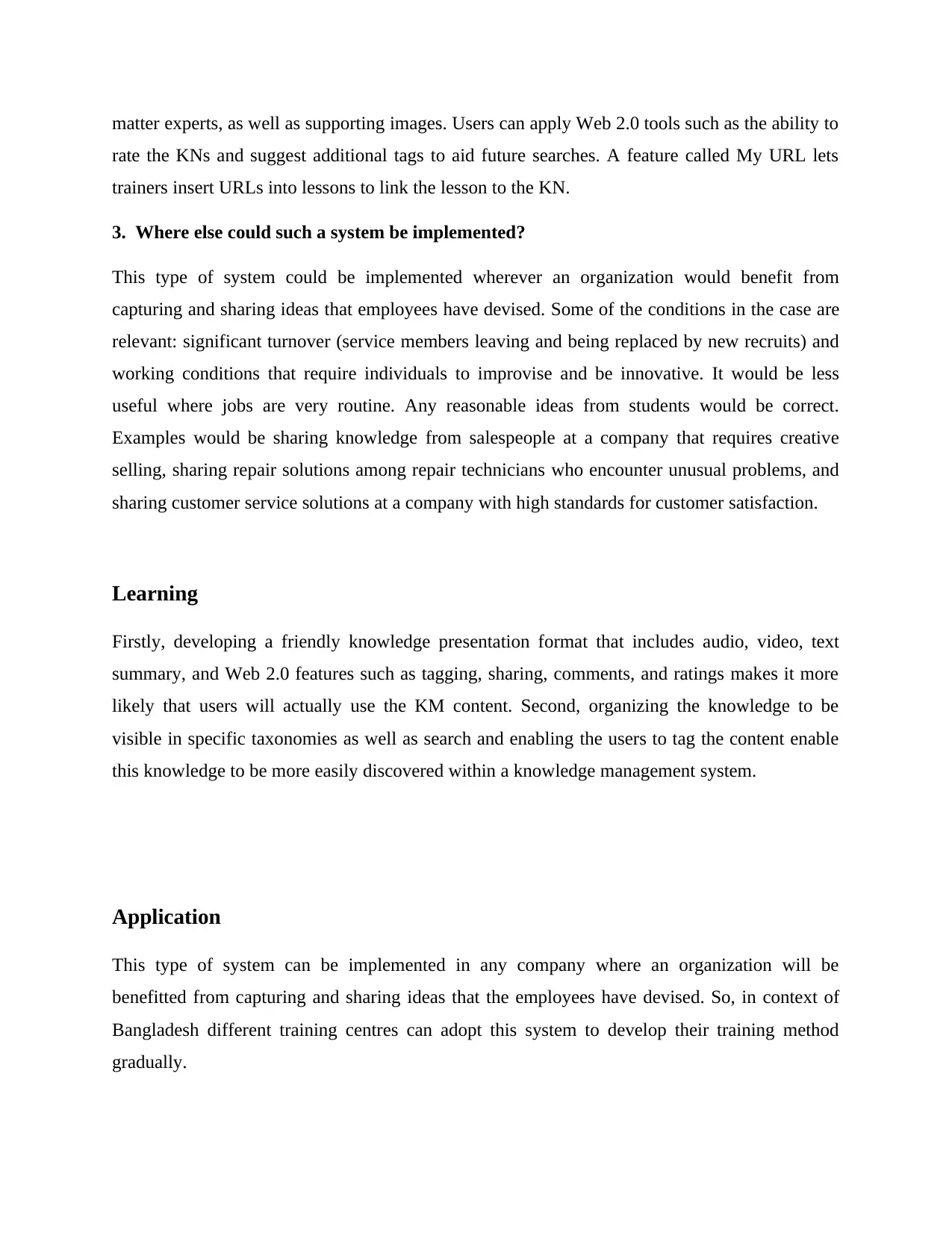
matter experts, as well as supporting images. Users can apply Web 2.0 tools such as the ability to
rate the KNs and suggest additional tags to aid future searches. A feature called My URL lets
trainers insert URLs into lessons to link the lesson to the KN.
3. Where else could such a system be implemented?
This type of system could be implemented wherever an organization would benefit from
capturing and sharing ideas that employees have devised. Some of the conditions in the case are
relevant: significant turnover (service members leaving and being replaced by new recruits) and
working conditions that require individuals to improvise and be innovative. It would be less
useful where jobs are very routine. Any reasonable ideas from students would be correct.
Examples would be sharing knowledge from salespeople at a company that requires creative
selling, sharing repair solutions among repair technicians who encounter unusual problems, and
sharing customer service solutions at a company with high standards for customer satisfaction.
Learning
Firstly, developing a friendly knowledge presentation format that includes audio, video, text
summary, and Web 2.0 features such as tagging, sharing, comments, and ratings makes it more
likely that users will actually use the KM content. Second, organizing the knowledge to be
visible in specific taxonomies as well as search and enabling the users to tag the content enable
this knowledge to be more easily discovered within a knowledge management system.
Application
This type of system can be implemented in any company where an organization will be
benefitted from capturing and sharing ideas that the employees have devised. So, in context of
Bangladesh different training centres can adopt this system to develop their training method
gradually.
rate the KNs and suggest additional tags to aid future searches. A feature called My URL lets
trainers insert URLs into lessons to link the lesson to the KN.
3. Where else could such a system be implemented?
This type of system could be implemented wherever an organization would benefit from
capturing and sharing ideas that employees have devised. Some of the conditions in the case are
relevant: significant turnover (service members leaving and being replaced by new recruits) and
working conditions that require individuals to improvise and be innovative. It would be less
useful where jobs are very routine. Any reasonable ideas from students would be correct.
Examples would be sharing knowledge from salespeople at a company that requires creative
selling, sharing repair solutions among repair technicians who encounter unusual problems, and
sharing customer service solutions at a company with high standards for customer satisfaction.
Learning
Firstly, developing a friendly knowledge presentation format that includes audio, video, text
summary, and Web 2.0 features such as tagging, sharing, comments, and ratings makes it more
likely that users will actually use the KM content. Second, organizing the knowledge to be
visible in specific taxonomies as well as search and enabling the users to tag the content enable
this knowledge to be more easily discovered within a knowledge management system.
Application
This type of system can be implemented in any company where an organization will be
benefitted from capturing and sharing ideas that the employees have devised. So, in context of
Bangladesh different training centres can adopt this system to develop their training method
gradually.
Secure Best Marks with AI Grader
Need help grading? Try our AI Grader for instant feedback on your assignments.
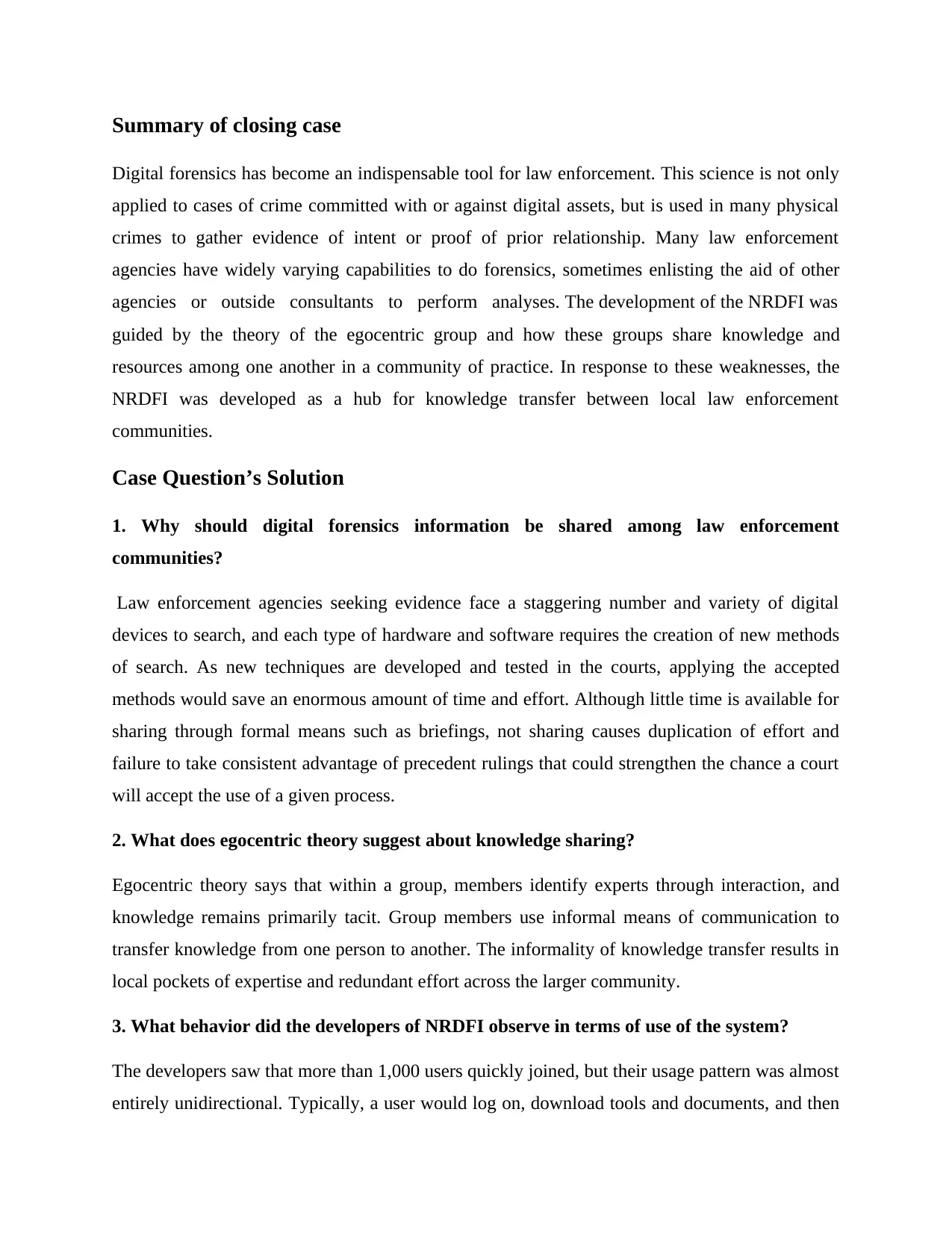
Summary of closing case
Digital forensics has become an indispensable tool for law enforcement. This science is not only
applied to cases of crime committed with or against digital assets, but is used in many physical
crimes to gather evidence of intent or proof of prior relationship. Many law enforcement
agencies have widely varying capabilities to do forensics, sometimes enlisting the aid of other
agencies or outside consultants to perform analyses. The development of the NRDFI was
guided by the theory of the egocentric group and how these groups share knowledge and
resources among one another in a community of practice. In response to these weaknesses, the
NRDFI was developed as a hub for knowledge transfer between local law enforcement
communities.
Case Question’s Solution
1. Why should digital forensics information be shared among law enforcement
communities?
Law enforcement agencies seeking evidence face a staggering number and variety of digital
devices to search, and each type of hardware and software requires the creation of new methods
of search. As new techniques are developed and tested in the courts, applying the accepted
methods would save an enormous amount of time and effort. Although little time is available for
sharing through formal means such as briefings, not sharing causes duplication of effort and
failure to take consistent advantage of precedent rulings that could strengthen the chance a court
will accept the use of a given process.
2. What does egocentric theory suggest about knowledge sharing?
Egocentric theory says that within a group, members identify experts through interaction, and
knowledge remains primarily tacit. Group members use informal means of communication to
transfer knowledge from one person to another. The informality of knowledge transfer results in
local pockets of expertise and redundant effort across the larger community.
3. What behavior did the developers of NRDFI observe in terms of use of the system?
The developers saw that more than 1,000 users quickly joined, but their usage pattern was almost
entirely unidirectional. Typically, a user would log on, download tools and documents, and then
Digital forensics has become an indispensable tool for law enforcement. This science is not only
applied to cases of crime committed with or against digital assets, but is used in many physical
crimes to gather evidence of intent or proof of prior relationship. Many law enforcement
agencies have widely varying capabilities to do forensics, sometimes enlisting the aid of other
agencies or outside consultants to perform analyses. The development of the NRDFI was
guided by the theory of the egocentric group and how these groups share knowledge and
resources among one another in a community of practice. In response to these weaknesses, the
NRDFI was developed as a hub for knowledge transfer between local law enforcement
communities.
Case Question’s Solution
1. Why should digital forensics information be shared among law enforcement
communities?
Law enforcement agencies seeking evidence face a staggering number and variety of digital
devices to search, and each type of hardware and software requires the creation of new methods
of search. As new techniques are developed and tested in the courts, applying the accepted
methods would save an enormous amount of time and effort. Although little time is available for
sharing through formal means such as briefings, not sharing causes duplication of effort and
failure to take consistent advantage of precedent rulings that could strengthen the chance a court
will accept the use of a given process.
2. What does egocentric theory suggest about knowledge sharing?
Egocentric theory says that within a group, members identify experts through interaction, and
knowledge remains primarily tacit. Group members use informal means of communication to
transfer knowledge from one person to another. The informality of knowledge transfer results in
local pockets of expertise and redundant effort across the larger community.
3. What behavior did the developers of NRDFI observe in terms of use of the system?
The developers saw that more than 1,000 users quickly joined, but their usage pattern was almost
entirely unidirectional. Typically, a user would log on, download tools and documents, and then
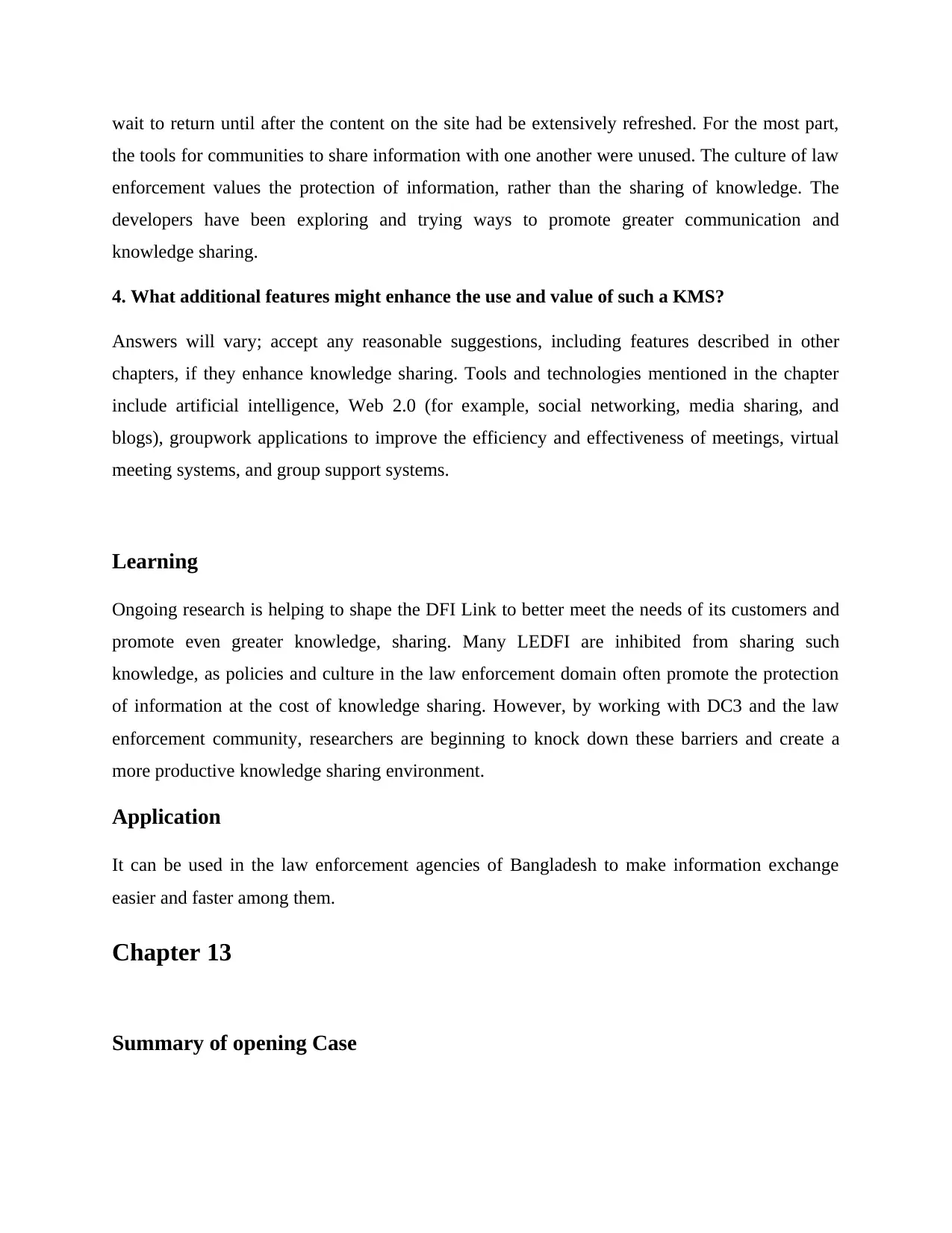
wait to return until after the content on the site had be extensively refreshed. For the most part,
the tools for communities to share information with one another were unused. The culture of law
enforcement values the protection of information, rather than the sharing of knowledge. The
developers have been exploring and trying ways to promote greater communication and
knowledge sharing.
4. What additional features might enhance the use and value of such a KMS?
Answers will vary; accept any reasonable suggestions, including features described in other
chapters, if they enhance knowledge sharing. Tools and technologies mentioned in the chapter
include artificial intelligence, Web 2.0 (for example, social networking, media sharing, and
blogs), groupwork applications to improve the efficiency and effectiveness of meetings, virtual
meeting systems, and group support systems.
Learning
Ongoing research is helping to shape the DFI Link to better meet the needs of its customers and
promote even greater knowledge, sharing. Many LEDFI are inhibited from sharing such
knowledge, as policies and culture in the law enforcement domain often promote the protection
of information at the cost of knowledge sharing. However, by working with DC3 and the law
enforcement community, researchers are beginning to knock down these barriers and create a
more productive knowledge sharing environment.
Application
It can be used in the law enforcement agencies of Bangladesh to make information exchange
easier and faster among them.
Chapter 13
Summary of opening Case
the tools for communities to share information with one another were unused. The culture of law
enforcement values the protection of information, rather than the sharing of knowledge. The
developers have been exploring and trying ways to promote greater communication and
knowledge sharing.
4. What additional features might enhance the use and value of such a KMS?
Answers will vary; accept any reasonable suggestions, including features described in other
chapters, if they enhance knowledge sharing. Tools and technologies mentioned in the chapter
include artificial intelligence, Web 2.0 (for example, social networking, media sharing, and
blogs), groupwork applications to improve the efficiency and effectiveness of meetings, virtual
meeting systems, and group support systems.
Learning
Ongoing research is helping to shape the DFI Link to better meet the needs of its customers and
promote even greater knowledge, sharing. Many LEDFI are inhibited from sharing such
knowledge, as policies and culture in the law enforcement domain often promote the protection
of information at the cost of knowledge sharing. However, by working with DC3 and the law
enforcement community, researchers are beginning to knock down these barriers and create a
more productive knowledge sharing environment.
Application
It can be used in the law enforcement agencies of Bangladesh to make information exchange
easier and faster among them.
Chapter 13
Summary of opening Case
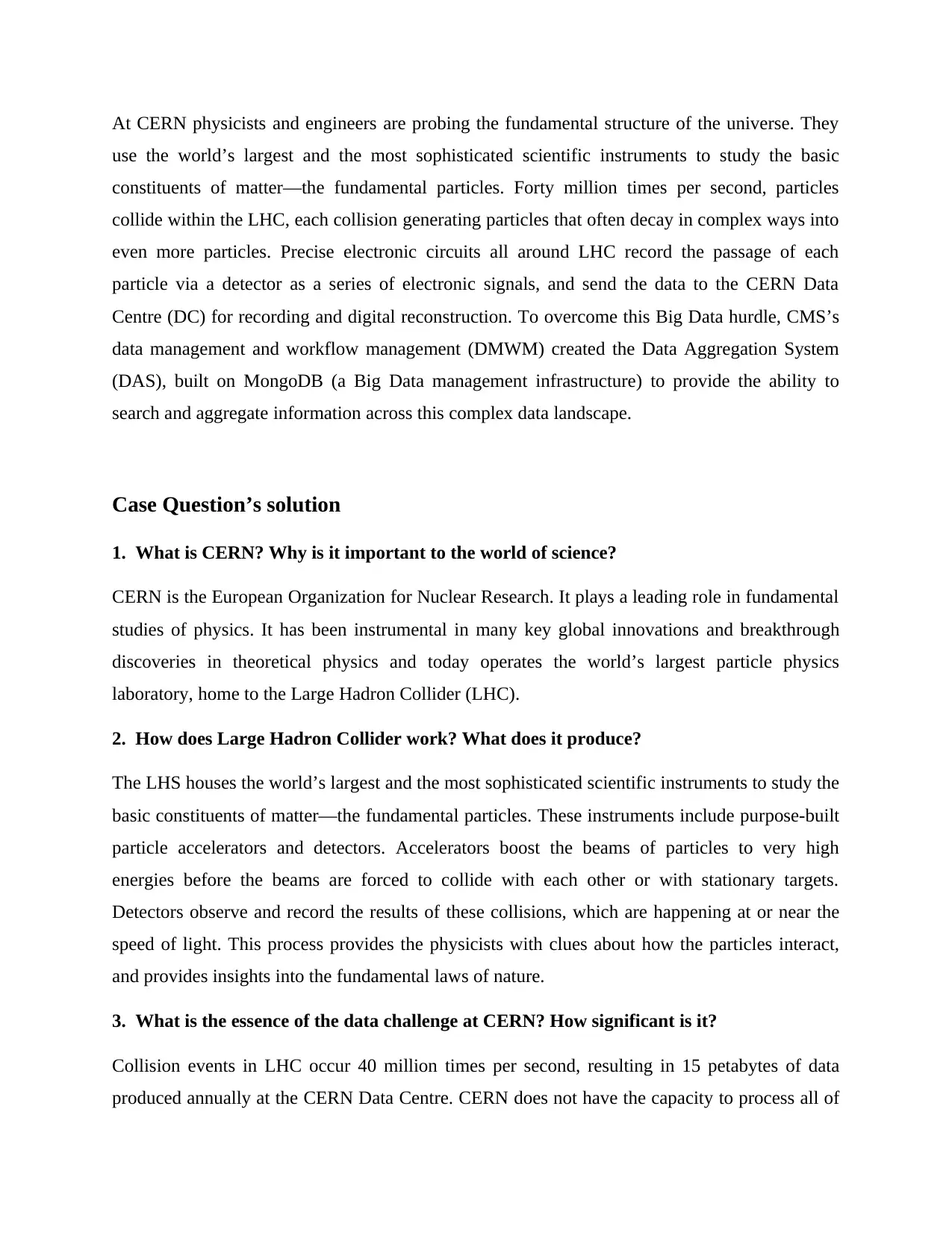
At CERN physicists and engineers are probing the fundamental structure of the universe. They
use the world’s largest and the most sophisticated scientific instruments to study the basic
constituents of matter—the fundamental particles. Forty million times per second, particles
collide within the LHC, each collision generating particles that often decay in complex ways into
even more particles. Precise electronic circuits all around LHC record the passage of each
particle via a detector as a series of electronic signals, and send the data to the CERN Data
Centre (DC) for recording and digital reconstruction. To overcome this Big Data hurdle, CMS’s
data management and workflow management (DMWM) created the Data Aggregation System
(DAS), built on MongoDB (a Big Data management infrastructure) to provide the ability to
search and aggregate information across this complex data landscape.
Case Question’s solution
1. What is CERN? Why is it important to the world of science?
CERN is the European Organization for Nuclear Research. It plays a leading role in fundamental
studies of physics. It has been instrumental in many key global innovations and breakthrough
discoveries in theoretical physics and today operates the world’s largest particle physics
laboratory, home to the Large Hadron Collider (LHC).
2. How does Large Hadron Collider work? What does it produce?
The LHS houses the world’s largest and the most sophisticated scientific instruments to study the
basic constituents of matter—the fundamental particles. These instruments include purpose-built
particle accelerators and detectors. Accelerators boost the beams of particles to very high
energies before the beams are forced to collide with each other or with stationary targets.
Detectors observe and record the results of these collisions, which are happening at or near the
speed of light. This process provides the physicists with clues about how the particles interact,
and provides insights into the fundamental laws of nature.
3. What is the essence of the data challenge at CERN? How significant is it?
Collision events in LHC occur 40 million times per second, resulting in 15 petabytes of data
produced annually at the CERN Data Centre. CERN does not have the capacity to process all of
use the world’s largest and the most sophisticated scientific instruments to study the basic
constituents of matter—the fundamental particles. Forty million times per second, particles
collide within the LHC, each collision generating particles that often decay in complex ways into
even more particles. Precise electronic circuits all around LHC record the passage of each
particle via a detector as a series of electronic signals, and send the data to the CERN Data
Centre (DC) for recording and digital reconstruction. To overcome this Big Data hurdle, CMS’s
data management and workflow management (DMWM) created the Data Aggregation System
(DAS), built on MongoDB (a Big Data management infrastructure) to provide the ability to
search and aggregate information across this complex data landscape.
Case Question’s solution
1. What is CERN? Why is it important to the world of science?
CERN is the European Organization for Nuclear Research. It plays a leading role in fundamental
studies of physics. It has been instrumental in many key global innovations and breakthrough
discoveries in theoretical physics and today operates the world’s largest particle physics
laboratory, home to the Large Hadron Collider (LHC).
2. How does Large Hadron Collider work? What does it produce?
The LHS houses the world’s largest and the most sophisticated scientific instruments to study the
basic constituents of matter—the fundamental particles. These instruments include purpose-built
particle accelerators and detectors. Accelerators boost the beams of particles to very high
energies before the beams are forced to collide with each other or with stationary targets.
Detectors observe and record the results of these collisions, which are happening at or near the
speed of light. This process provides the physicists with clues about how the particles interact,
and provides insights into the fundamental laws of nature.
3. What is the essence of the data challenge at CERN? How significant is it?
Collision events in LHC occur 40 million times per second, resulting in 15 petabytes of data
produced annually at the CERN Data Centre. CERN does not have the capacity to process all of
Paraphrase This Document
Need a fresh take? Get an instant paraphrase of this document with our AI Paraphraser
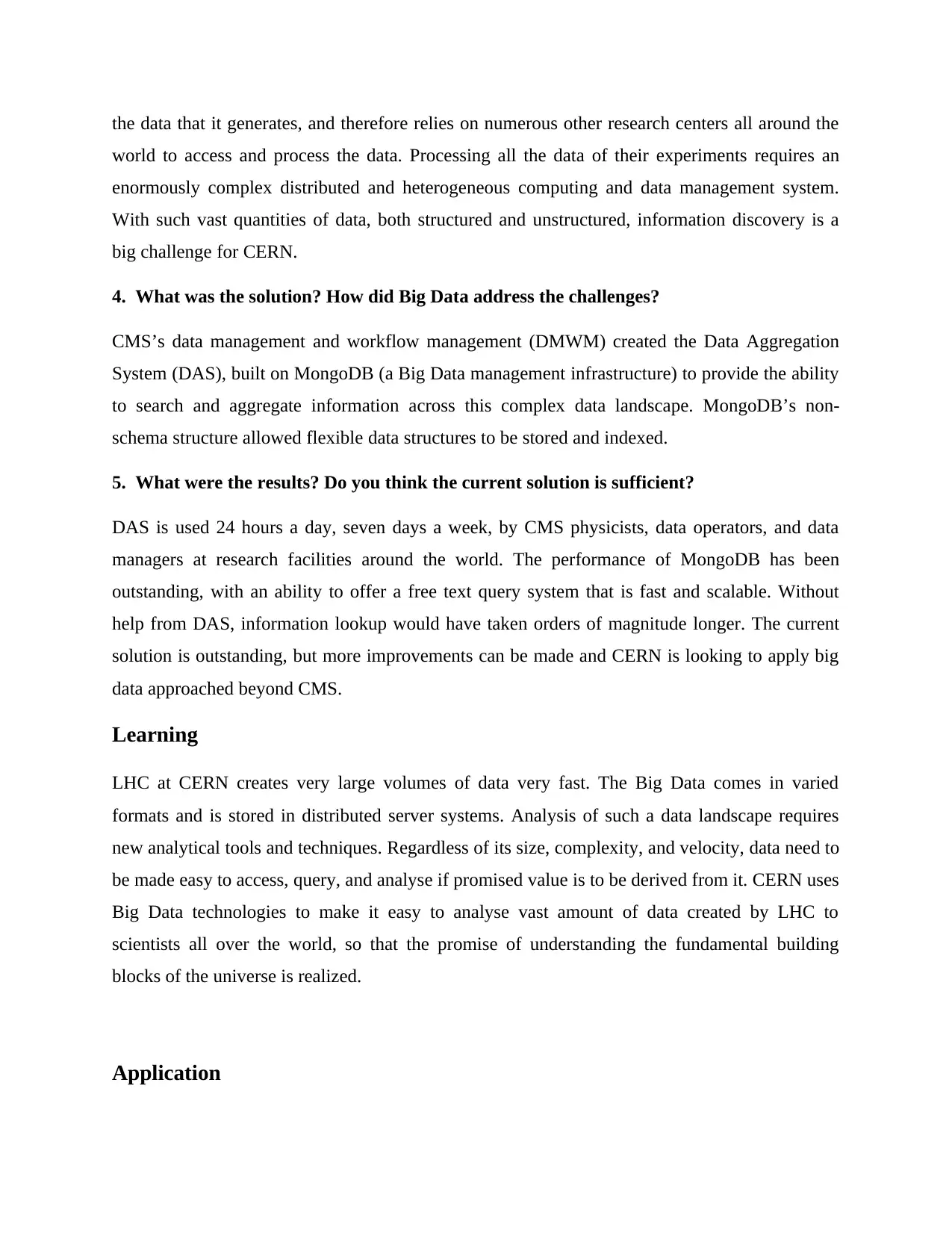
the data that it generates, and therefore relies on numerous other research centers all around the
world to access and process the data. Processing all the data of their experiments requires an
enormously complex distributed and heterogeneous computing and data management system.
With such vast quantities of data, both structured and unstructured, information discovery is a
big challenge for CERN.
4. What was the solution? How did Big Data address the challenges?
CMS’s data management and workflow management (DMWM) created the Data Aggregation
System (DAS), built on MongoDB (a Big Data management infrastructure) to provide the ability
to search and aggregate information across this complex data landscape. MongoDB’s non-
schema structure allowed flexible data structures to be stored and indexed.
5. What were the results? Do you think the current solution is sufficient?
DAS is used 24 hours a day, seven days a week, by CMS physicists, data operators, and data
managers at research facilities around the world. The performance of MongoDB has been
outstanding, with an ability to offer a free text query system that is fast and scalable. Without
help from DAS, information lookup would have taken orders of magnitude longer. The current
solution is outstanding, but more improvements can be made and CERN is looking to apply big
data approached beyond CMS.
Learning
LHC at CERN creates very large volumes of data very fast. The Big Data comes in varied
formats and is stored in distributed server systems. Analysis of such a data landscape requires
new analytical tools and techniques. Regardless of its size, complexity, and velocity, data need to
be made easy to access, query, and analyse if promised value is to be derived from it. CERN uses
Big Data technologies to make it easy to analyse vast amount of data created by LHC to
scientists all over the world, so that the promise of understanding the fundamental building
blocks of the universe is realized.
Application
world to access and process the data. Processing all the data of their experiments requires an
enormously complex distributed and heterogeneous computing and data management system.
With such vast quantities of data, both structured and unstructured, information discovery is a
big challenge for CERN.
4. What was the solution? How did Big Data address the challenges?
CMS’s data management and workflow management (DMWM) created the Data Aggregation
System (DAS), built on MongoDB (a Big Data management infrastructure) to provide the ability
to search and aggregate information across this complex data landscape. MongoDB’s non-
schema structure allowed flexible data structures to be stored and indexed.
5. What were the results? Do you think the current solution is sufficient?
DAS is used 24 hours a day, seven days a week, by CMS physicists, data operators, and data
managers at research facilities around the world. The performance of MongoDB has been
outstanding, with an ability to offer a free text query system that is fast and scalable. Without
help from DAS, information lookup would have taken orders of magnitude longer. The current
solution is outstanding, but more improvements can be made and CERN is looking to apply big
data approached beyond CMS.
Learning
LHC at CERN creates very large volumes of data very fast. The Big Data comes in varied
formats and is stored in distributed server systems. Analysis of such a data landscape requires
new analytical tools and techniques. Regardless of its size, complexity, and velocity, data need to
be made easy to access, query, and analyse if promised value is to be derived from it. CERN uses
Big Data technologies to make it easy to analyse vast amount of data created by LHC to
scientists all over the world, so that the promise of understanding the fundamental building
blocks of the universe is realized.
Application
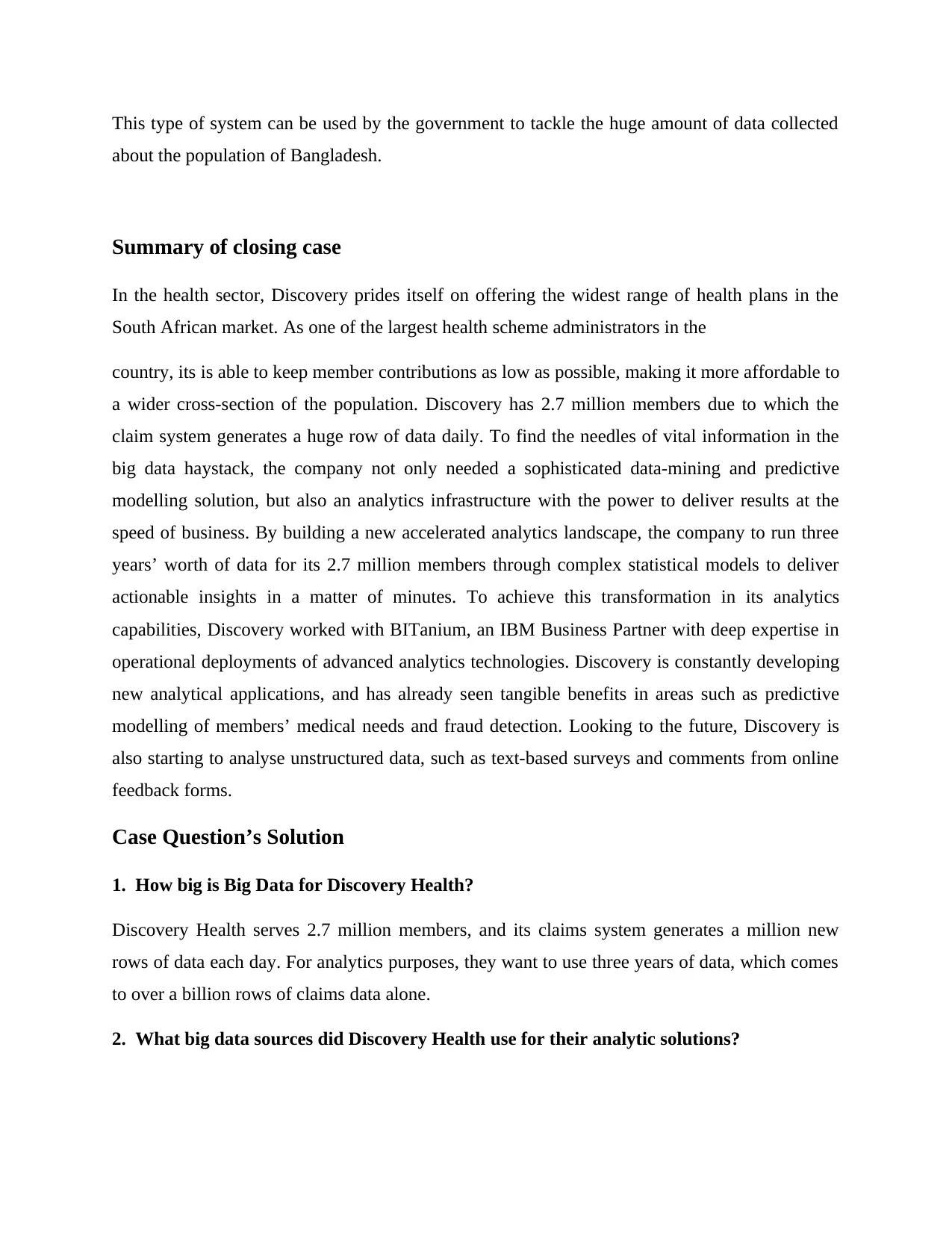
This type of system can be used by the government to tackle the huge amount of data collected
about the population of Bangladesh.
Summary of closing case
In the health sector, Discovery prides itself on offering the widest range of health plans in the
South African market. As one of the largest health scheme administrators in the
country, its is able to keep member contributions as low as possible, making it more affordable to
a wider cross-section of the population. Discovery has 2.7 million members due to which the
claim system generates a huge row of data daily. To find the needles of vital information in the
big data haystack, the company not only needed a sophisticated data-mining and predictive
modelling solution, but also an analytics infrastructure with the power to deliver results at the
speed of business. By building a new accelerated analytics landscape, the company to run three
years’ worth of data for its 2.7 million members through complex statistical models to deliver
actionable insights in a matter of minutes. To achieve this transformation in its analytics
capabilities, Discovery worked with BITanium, an IBM Business Partner with deep expertise in
operational deployments of advanced analytics technologies. Discovery is constantly developing
new analytical applications, and has already seen tangible benefits in areas such as predictive
modelling of members’ medical needs and fraud detection. Looking to the future, Discovery is
also starting to analyse unstructured data, such as text-based surveys and comments from online
feedback forms.
Case Question’s Solution
1. How big is Big Data for Discovery Health?
Discovery Health serves 2.7 million members, and its claims system generates a million new
rows of data each day. For analytics purposes, they want to use three years of data, which comes
to over a billion rows of claims data alone.
2. What big data sources did Discovery Health use for their analytic solutions?
about the population of Bangladesh.
Summary of closing case
In the health sector, Discovery prides itself on offering the widest range of health plans in the
South African market. As one of the largest health scheme administrators in the
country, its is able to keep member contributions as low as possible, making it more affordable to
a wider cross-section of the population. Discovery has 2.7 million members due to which the
claim system generates a huge row of data daily. To find the needles of vital information in the
big data haystack, the company not only needed a sophisticated data-mining and predictive
modelling solution, but also an analytics infrastructure with the power to deliver results at the
speed of business. By building a new accelerated analytics landscape, the company to run three
years’ worth of data for its 2.7 million members through complex statistical models to deliver
actionable insights in a matter of minutes. To achieve this transformation in its analytics
capabilities, Discovery worked with BITanium, an IBM Business Partner with deep expertise in
operational deployments of advanced analytics technologies. Discovery is constantly developing
new analytical applications, and has already seen tangible benefits in areas such as predictive
modelling of members’ medical needs and fraud detection. Looking to the future, Discovery is
also starting to analyse unstructured data, such as text-based surveys and comments from online
feedback forms.
Case Question’s Solution
1. How big is Big Data for Discovery Health?
Discovery Health serves 2.7 million members, and its claims system generates a million new
rows of data each day. For analytics purposes, they want to use three years of data, which comes
to over a billion rows of claims data alone.
2. What big data sources did Discovery Health use for their analytic solutions?
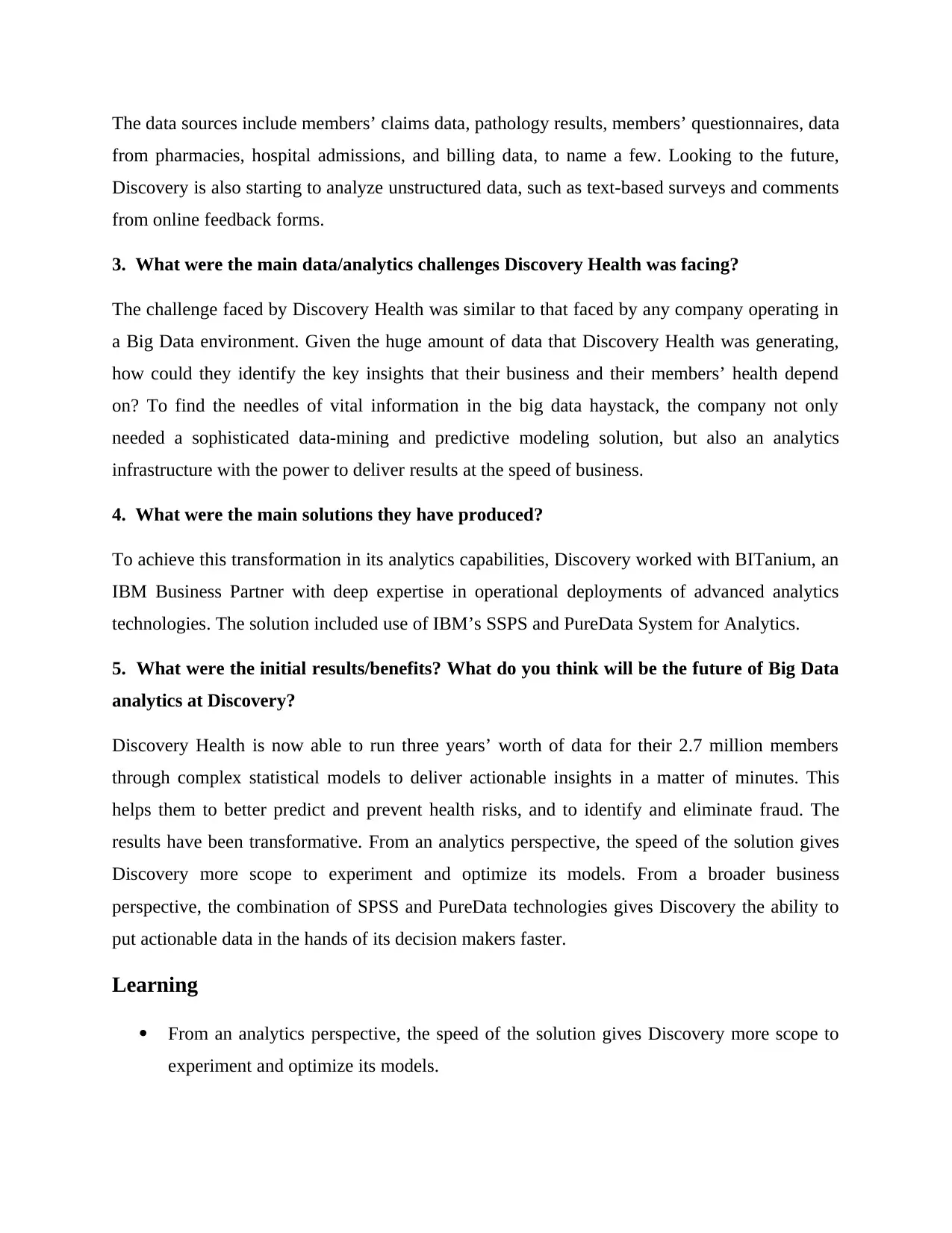
The data sources include members’ claims data, pathology results, members’ questionnaires, data
from pharmacies, hospital admissions, and billing data, to name a few. Looking to the future,
Discovery is also starting to analyze unstructured data, such as text-based surveys and comments
from online feedback forms.
3. What were the main data/analytics challenges Discovery Health was facing?
The challenge faced by Discovery Health was similar to that faced by any company operating in
a Big Data environment. Given the huge amount of data that Discovery Health was generating,
how could they identify the key insights that their business and their members’ health depend
on? To find the needles of vital information in the big data haystack, the company not only
needed a sophisticated data-mining and predictive modeling solution, but also an analytics
infrastructure with the power to deliver results at the speed of business.
4. What were the main solutions they have produced?
To achieve this transformation in its analytics capabilities, Discovery worked with BITanium, an
IBM Business Partner with deep expertise in operational deployments of advanced analytics
technologies. The solution included use of IBM’s SSPS and PureData System for Analytics.
5. What were the initial results/benefits? What do you think will be the future of Big Data
analytics at Discovery?
Discovery Health is now able to run three years’ worth of data for their 2.7 million members
through complex statistical models to deliver actionable insights in a matter of minutes. This
helps them to better predict and prevent health risks, and to identify and eliminate fraud. The
results have been transformative. From an analytics perspective, the speed of the solution gives
Discovery more scope to experiment and optimize its models. From a broader business
perspective, the combination of SPSS and PureData technologies gives Discovery the ability to
put actionable data in the hands of its decision makers faster.
Learning
From an analytics perspective, the speed of the solution gives Discovery more scope to
experiment and optimize its models.
from pharmacies, hospital admissions, and billing data, to name a few. Looking to the future,
Discovery is also starting to analyze unstructured data, such as text-based surveys and comments
from online feedback forms.
3. What were the main data/analytics challenges Discovery Health was facing?
The challenge faced by Discovery Health was similar to that faced by any company operating in
a Big Data environment. Given the huge amount of data that Discovery Health was generating,
how could they identify the key insights that their business and their members’ health depend
on? To find the needles of vital information in the big data haystack, the company not only
needed a sophisticated data-mining and predictive modeling solution, but also an analytics
infrastructure with the power to deliver results at the speed of business.
4. What were the main solutions they have produced?
To achieve this transformation in its analytics capabilities, Discovery worked with BITanium, an
IBM Business Partner with deep expertise in operational deployments of advanced analytics
technologies. The solution included use of IBM’s SSPS and PureData System for Analytics.
5. What were the initial results/benefits? What do you think will be the future of Big Data
analytics at Discovery?
Discovery Health is now able to run three years’ worth of data for their 2.7 million members
through complex statistical models to deliver actionable insights in a matter of minutes. This
helps them to better predict and prevent health risks, and to identify and eliminate fraud. The
results have been transformative. From an analytics perspective, the speed of the solution gives
Discovery more scope to experiment and optimize its models. From a broader business
perspective, the combination of SPSS and PureData technologies gives Discovery the ability to
put actionable data in the hands of its decision makers faster.
Learning
From an analytics perspective, the speed of the solution gives Discovery more scope to
experiment and optimize its models.
Secure Best Marks with AI Grader
Need help grading? Try our AI Grader for instant feedback on your assignments.
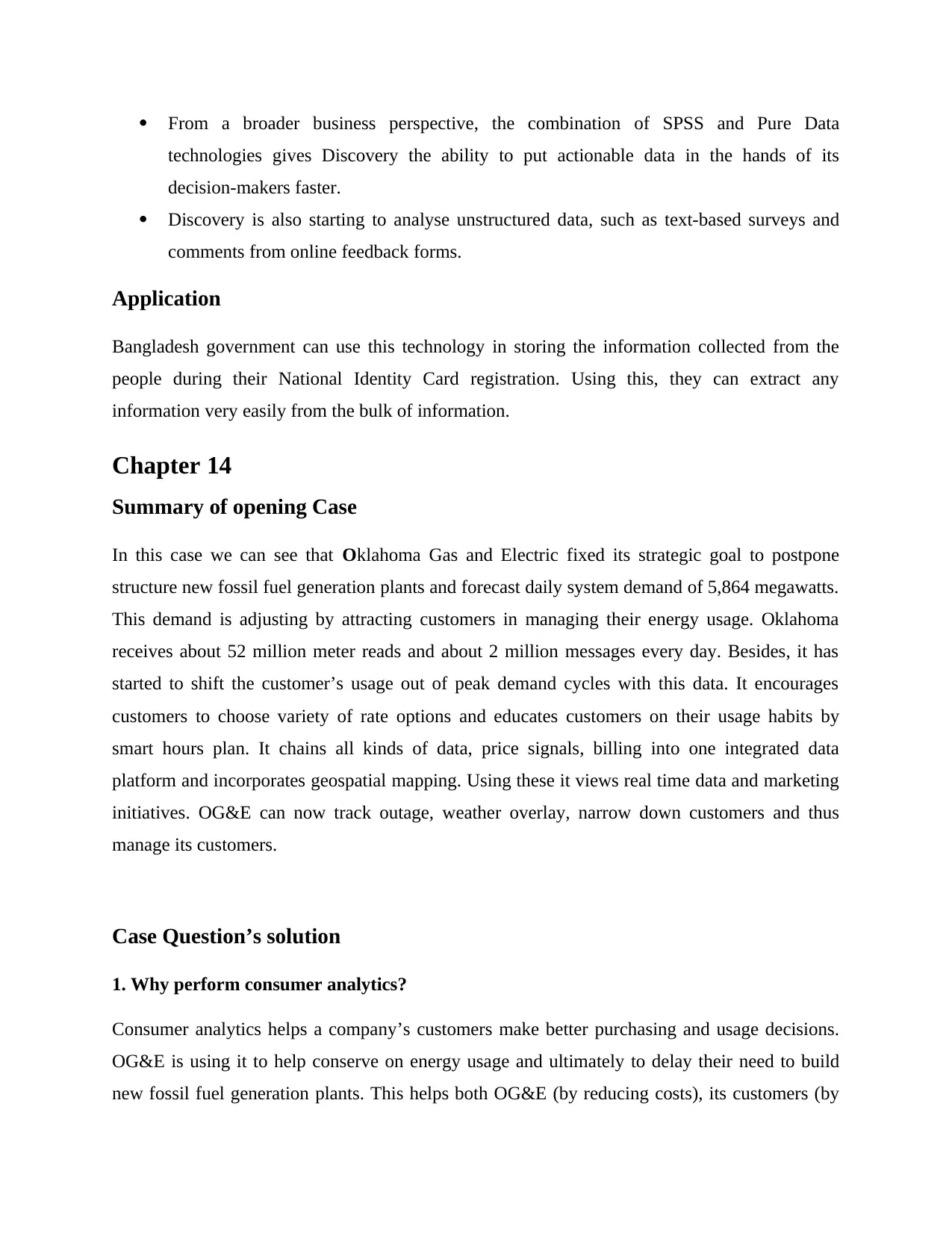
From a broader business perspective, the combination of SPSS and Pure Data
technologies gives Discovery the ability to put actionable data in the hands of its
decision-makers faster.
Discovery is also starting to analyse unstructured data, such as text-based surveys and
comments from online feedback forms.
Application
Bangladesh government can use this technology in storing the information collected from the
people during their National Identity Card registration. Using this, they can extract any
information very easily from the bulk of information.
Chapter 14
Summary of opening Case
In this case we can see that Oklahoma Gas and Electric fixed its strategic goal to postpone
structure new fossil fuel generation plants and forecast daily system demand of 5,864 megawatts.
This demand is adjusting by attracting customers in managing their energy usage. Oklahoma
receives about 52 million meter reads and about 2 million messages every day. Besides, it has
started to shift the customer’s usage out of peak demand cycles with this data. It encourages
customers to choose variety of rate options and educates customers on their usage habits by
smart hours plan. It chains all kinds of data, price signals, billing into one integrated data
platform and incorporates geospatial mapping. Using these it views real time data and marketing
initiatives. OG&E can now track outage, weather overlay, narrow down customers and thus
manage its customers.
Case Question’s solution
1. Why perform consumer analytics?
Consumer analytics helps a company’s customers make better purchasing and usage decisions.
OG&E is using it to help conserve on energy usage and ultimately to delay their need to build
new fossil fuel generation plants. This helps both OG&E (by reducing costs), its customers (by
technologies gives Discovery the ability to put actionable data in the hands of its
decision-makers faster.
Discovery is also starting to analyse unstructured data, such as text-based surveys and
comments from online feedback forms.
Application
Bangladesh government can use this technology in storing the information collected from the
people during their National Identity Card registration. Using this, they can extract any
information very easily from the bulk of information.
Chapter 14
Summary of opening Case
In this case we can see that Oklahoma Gas and Electric fixed its strategic goal to postpone
structure new fossil fuel generation plants and forecast daily system demand of 5,864 megawatts.
This demand is adjusting by attracting customers in managing their energy usage. Oklahoma
receives about 52 million meter reads and about 2 million messages every day. Besides, it has
started to shift the customer’s usage out of peak demand cycles with this data. It encourages
customers to choose variety of rate options and educates customers on their usage habits by
smart hours plan. It chains all kinds of data, price signals, billing into one integrated data
platform and incorporates geospatial mapping. Using these it views real time data and marketing
initiatives. OG&E can now track outage, weather overlay, narrow down customers and thus
manage its customers.
Case Question’s solution
1. Why perform consumer analytics?
Consumer analytics helps a company’s customers make better purchasing and usage decisions.
OG&E is using it to help conserve on energy usage and ultimately to delay their need to build
new fossil fuel generation plants. This helps both OG&E (by reducing costs), its customers (by
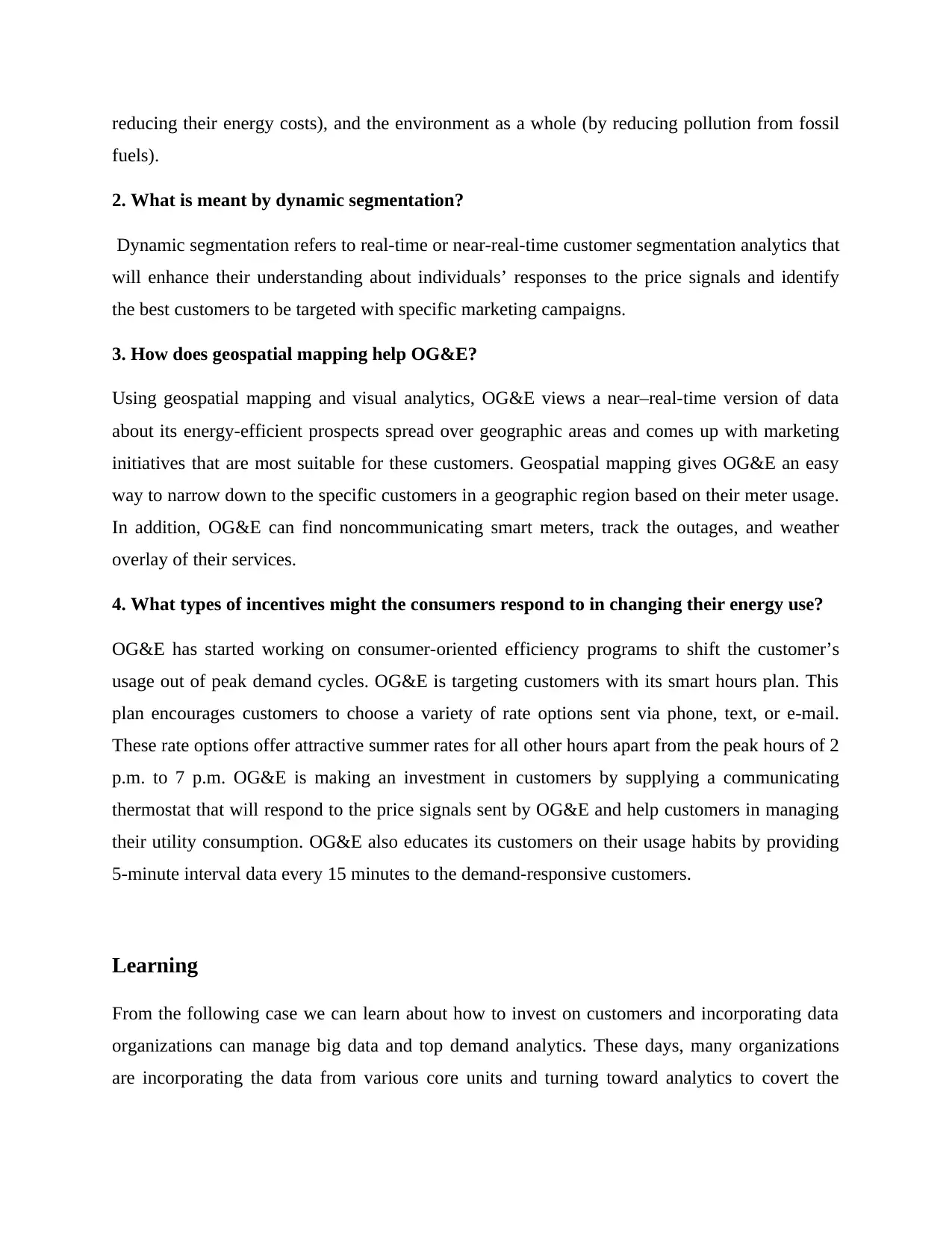
reducing their energy costs), and the environment as a whole (by reducing pollution from fossil
fuels).
2. What is meant by dynamic segmentation?
Dynamic segmentation refers to real-time or near-real-time customer segmentation analytics that
will enhance their understanding about individuals’ responses to the price signals and identify
the best customers to be targeted with specific marketing campaigns.
3. How does geospatial mapping help OG&E?
Using geospatial mapping and visual analytics, OG&E views a near–real-time version of data
about its energy-efficient prospects spread over geographic areas and comes up with marketing
initiatives that are most suitable for these customers. Geospatial mapping gives OG&E an easy
way to narrow down to the specific customers in a geographic region based on their meter usage.
In addition, OG&E can find noncommunicating smart meters, track the outages, and weather
overlay of their services.
4. What types of incentives might the consumers respond to in changing their energy use?
OG&E has started working on consumer-oriented efficiency programs to shift the customer’s
usage out of peak demand cycles. OG&E is targeting customers with its smart hours plan. This
plan encourages customers to choose a variety of rate options sent via phone, text, or e-mail.
These rate options offer attractive summer rates for all other hours apart from the peak hours of 2
p.m. to 7 p.m. OG&E is making an investment in customers by supplying a communicating
thermostat that will respond to the price signals sent by OG&E and help customers in managing
their utility consumption. OG&E also educates its customers on their usage habits by providing
5-minute interval data every 15 minutes to the demand-responsive customers.
Learning
From the following case we can learn about how to invest on customers and incorporating data
organizations can manage big data and top demand analytics. These days, many organizations
are incorporating the data from various core units and turning toward analytics to covert the
fuels).
2. What is meant by dynamic segmentation?
Dynamic segmentation refers to real-time or near-real-time customer segmentation analytics that
will enhance their understanding about individuals’ responses to the price signals and identify
the best customers to be targeted with specific marketing campaigns.
3. How does geospatial mapping help OG&E?
Using geospatial mapping and visual analytics, OG&E views a near–real-time version of data
about its energy-efficient prospects spread over geographic areas and comes up with marketing
initiatives that are most suitable for these customers. Geospatial mapping gives OG&E an easy
way to narrow down to the specific customers in a geographic region based on their meter usage.
In addition, OG&E can find noncommunicating smart meters, track the outages, and weather
overlay of their services.
4. What types of incentives might the consumers respond to in changing their energy use?
OG&E has started working on consumer-oriented efficiency programs to shift the customer’s
usage out of peak demand cycles. OG&E is targeting customers with its smart hours plan. This
plan encourages customers to choose a variety of rate options sent via phone, text, or e-mail.
These rate options offer attractive summer rates for all other hours apart from the peak hours of 2
p.m. to 7 p.m. OG&E is making an investment in customers by supplying a communicating
thermostat that will respond to the price signals sent by OG&E and help customers in managing
their utility consumption. OG&E also educates its customers on their usage habits by providing
5-minute interval data every 15 minutes to the demand-responsive customers.
Learning
From the following case we can learn about how to invest on customers and incorporating data
organizations can manage big data and top demand analytics. These days, many organizations
are incorporating the data from various core units and turning toward analytics to covert the
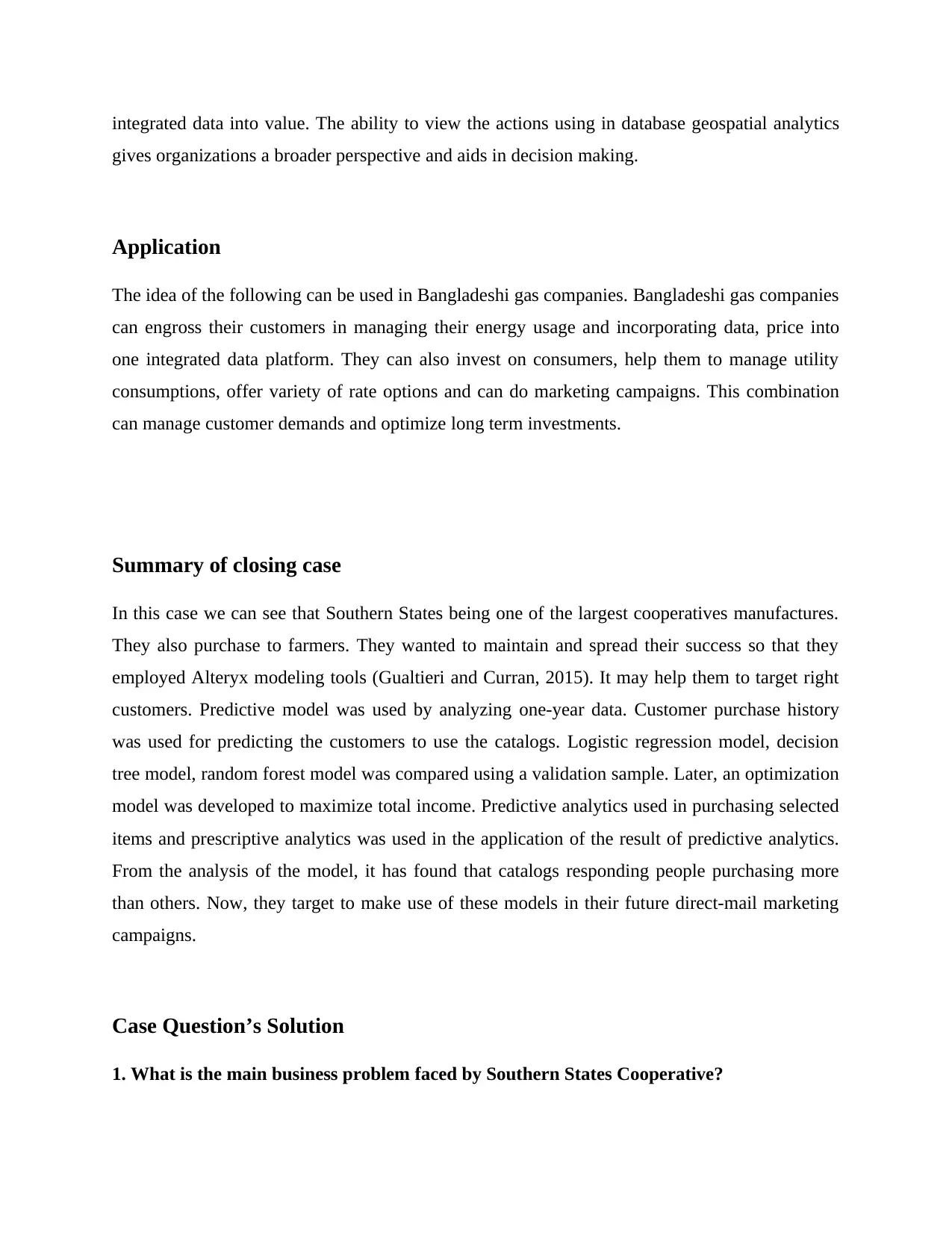
integrated data into value. The ability to view the actions using in database geospatial analytics
gives organizations a broader perspective and aids in decision making.
Application
The idea of the following can be used in Bangladeshi gas companies. Bangladeshi gas companies
can engross their customers in managing their energy usage and incorporating data, price into
one integrated data platform. They can also invest on consumers, help them to manage utility
consumptions, offer variety of rate options and can do marketing campaigns. This combination
can manage customer demands and optimize long term investments.
Summary of closing case
In this case we can see that Southern States being one of the largest cooperatives manufactures.
They also purchase to farmers. They wanted to maintain and spread their success so that they
employed Alteryx modeling tools (Gualtieri and Curran, 2015). It may help them to target right
customers. Predictive model was used by analyzing one-year data. Customer purchase history
was used for predicting the customers to use the catalogs. Logistic regression model, decision
tree model, random forest model was compared using a validation sample. Later, an optimization
model was developed to maximize total income. Predictive analytics used in purchasing selected
items and prescriptive analytics was used in the application of the result of predictive analytics.
From the analysis of the model, it has found that catalogs responding people purchasing more
than others. Now, they target to make use of these models in their future direct-mail marketing
campaigns.
Case Question’s Solution
1. What is the main business problem faced by Southern States Cooperative?
gives organizations a broader perspective and aids in decision making.
Application
The idea of the following can be used in Bangladeshi gas companies. Bangladeshi gas companies
can engross their customers in managing their energy usage and incorporating data, price into
one integrated data platform. They can also invest on consumers, help them to manage utility
consumptions, offer variety of rate options and can do marketing campaigns. This combination
can manage customer demands and optimize long term investments.
Summary of closing case
In this case we can see that Southern States being one of the largest cooperatives manufactures.
They also purchase to farmers. They wanted to maintain and spread their success so that they
employed Alteryx modeling tools (Gualtieri and Curran, 2015). It may help them to target right
customers. Predictive model was used by analyzing one-year data. Customer purchase history
was used for predicting the customers to use the catalogs. Logistic regression model, decision
tree model, random forest model was compared using a validation sample. Later, an optimization
model was developed to maximize total income. Predictive analytics used in purchasing selected
items and prescriptive analytics was used in the application of the result of predictive analytics.
From the analysis of the model, it has found that catalogs responding people purchasing more
than others. Now, they target to make use of these models in their future direct-mail marketing
campaigns.
Case Question’s Solution
1. What is the main business problem faced by Southern States Cooperative?
Paraphrase This Document
Need a fresh take? Get an instant paraphrase of this document with our AI Paraphraser
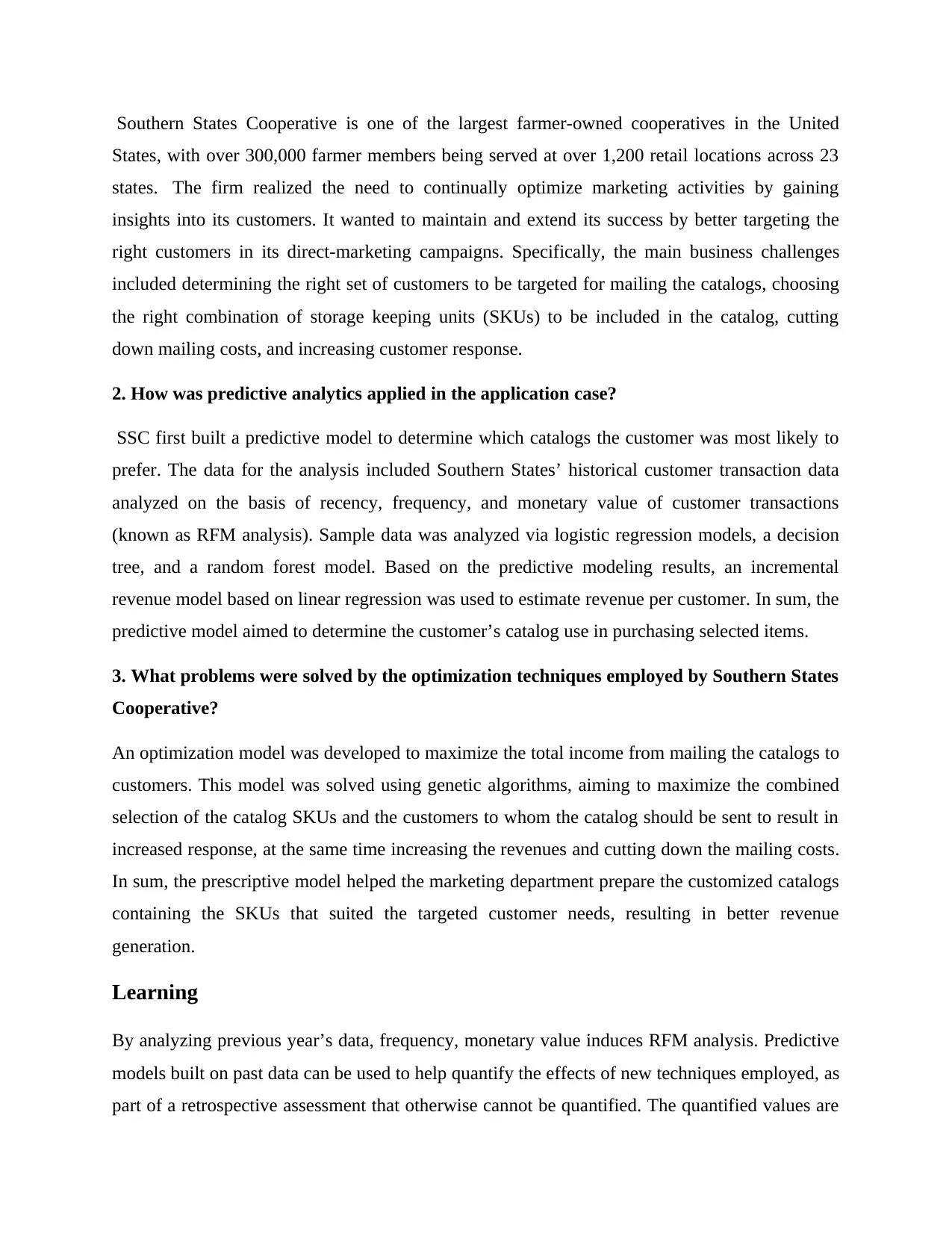
Southern States Cooperative is one of the largest farmer-owned cooperatives in the United
States, with over 300,000 farmer members being served at over 1,200 retail locations across 23
states. The firm realized the need to continually optimize marketing activities by gaining
insights into its customers. It wanted to maintain and extend its success by better targeting the
right customers in its direct-marketing campaigns. Specifically, the main business challenges
included determining the right set of customers to be targeted for mailing the catalogs, choosing
the right combination of storage keeping units (SKUs) to be included in the catalog, cutting
down mailing costs, and increasing customer response.
2. How was predictive analytics applied in the application case?
SSC first built a predictive model to determine which catalogs the customer was most likely to
prefer. The data for the analysis included Southern States’ historical customer transaction data
analyzed on the basis of recency, frequency, and monetary value of customer transactions
(known as RFM analysis). Sample data was analyzed via logistic regression models, a decision
tree, and a random forest model. Based on the predictive modeling results, an incremental
revenue model based on linear regression was used to estimate revenue per customer. In sum, the
predictive model aimed to determine the customer’s catalog use in purchasing selected items.
3. What problems were solved by the optimization techniques employed by Southern States
Cooperative?
An optimization model was developed to maximize the total income from mailing the catalogs to
customers. This model was solved using genetic algorithms, aiming to maximize the combined
selection of the catalog SKUs and the customers to whom the catalog should be sent to result in
increased response, at the same time increasing the revenues and cutting down the mailing costs.
In sum, the prescriptive model helped the marketing department prepare the customized catalogs
containing the SKUs that suited the targeted customer needs, resulting in better revenue
generation.
Learning
By analyzing previous year’s data, frequency, monetary value induces RFM analysis. Predictive
models built on past data can be used to help quantify the effects of new techniques employed, as
part of a retrospective assessment that otherwise cannot be quantified. The quantified values are
States, with over 300,000 farmer members being served at over 1,200 retail locations across 23
states. The firm realized the need to continually optimize marketing activities by gaining
insights into its customers. It wanted to maintain and extend its success by better targeting the
right customers in its direct-marketing campaigns. Specifically, the main business challenges
included determining the right set of customers to be targeted for mailing the catalogs, choosing
the right combination of storage keeping units (SKUs) to be included in the catalog, cutting
down mailing costs, and increasing customer response.
2. How was predictive analytics applied in the application case?
SSC first built a predictive model to determine which catalogs the customer was most likely to
prefer. The data for the analysis included Southern States’ historical customer transaction data
analyzed on the basis of recency, frequency, and monetary value of customer transactions
(known as RFM analysis). Sample data was analyzed via logistic regression models, a decision
tree, and a random forest model. Based on the predictive modeling results, an incremental
revenue model based on linear regression was used to estimate revenue per customer. In sum, the
predictive model aimed to determine the customer’s catalog use in purchasing selected items.
3. What problems were solved by the optimization techniques employed by Southern States
Cooperative?
An optimization model was developed to maximize the total income from mailing the catalogs to
customers. This model was solved using genetic algorithms, aiming to maximize the combined
selection of the catalog SKUs and the customers to whom the catalog should be sent to result in
increased response, at the same time increasing the revenues and cutting down the mailing costs.
In sum, the prescriptive model helped the marketing department prepare the customized catalogs
containing the SKUs that suited the targeted customer needs, resulting in better revenue
generation.
Learning
By analyzing previous year’s data, frequency, monetary value induces RFM analysis. Predictive
models built on past data can be used to help quantify the effects of new techniques employed, as
part of a retrospective assessment that otherwise cannot be quantified. The quantified values are
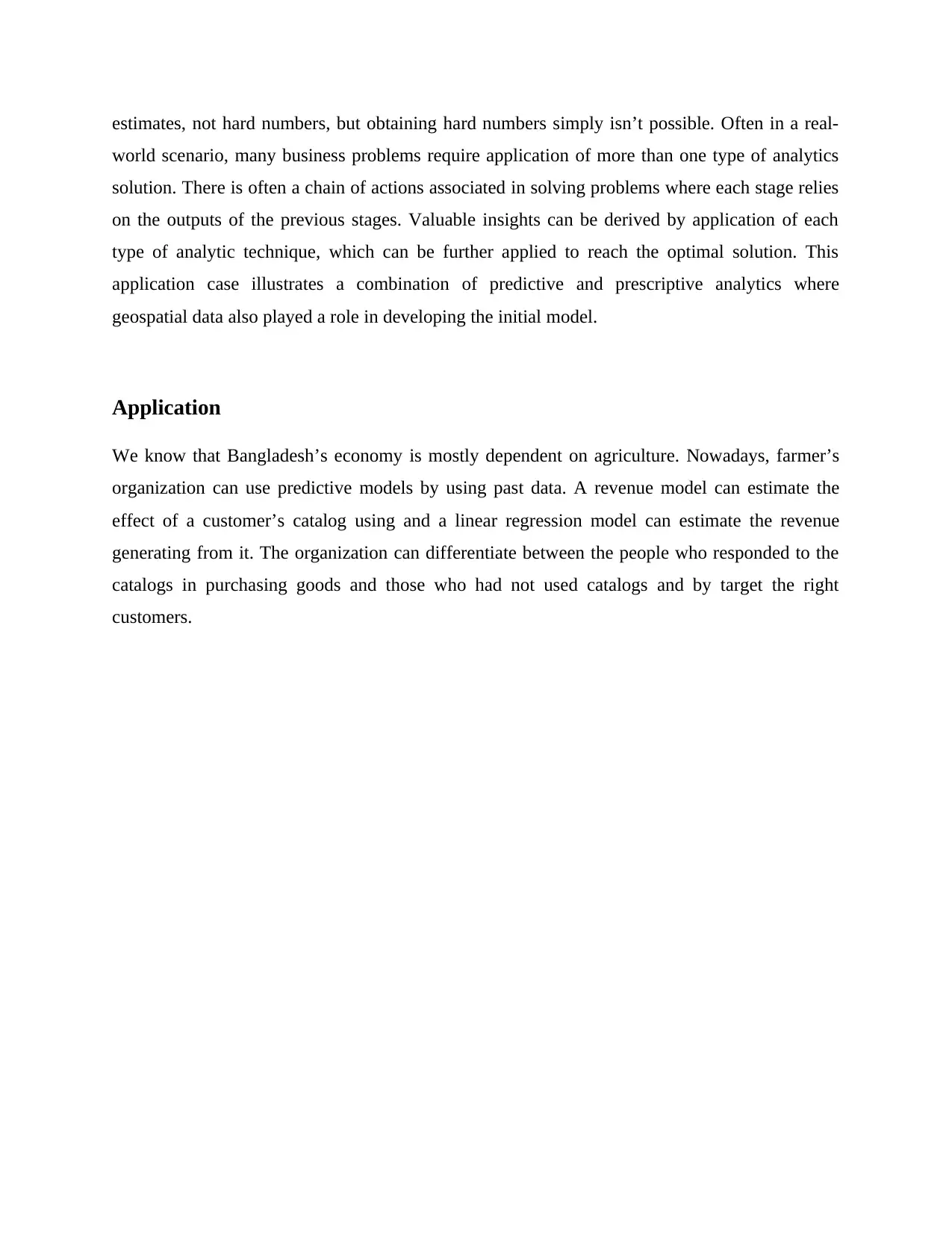
estimates, not hard numbers, but obtaining hard numbers simply isn’t possible. Often in a real-
world scenario, many business problems require application of more than one type of analytics
solution. There is often a chain of actions associated in solving problems where each stage relies
on the outputs of the previous stages. Valuable insights can be derived by application of each
type of analytic technique, which can be further applied to reach the optimal solution. This
application case illustrates a combination of predictive and prescriptive analytics where
geospatial data also played a role in developing the initial model.
Application
We know that Bangladesh’s economy is mostly dependent on agriculture. Nowadays, farmer’s
organization can use predictive models by using past data. A revenue model can estimate the
effect of a customer’s catalog using and a linear regression model can estimate the revenue
generating from it. The organization can differentiate between the people who responded to the
catalogs in purchasing goods and those who had not used catalogs and by target the right
customers.
world scenario, many business problems require application of more than one type of analytics
solution. There is often a chain of actions associated in solving problems where each stage relies
on the outputs of the previous stages. Valuable insights can be derived by application of each
type of analytic technique, which can be further applied to reach the optimal solution. This
application case illustrates a combination of predictive and prescriptive analytics where
geospatial data also played a role in developing the initial model.
Application
We know that Bangladesh’s economy is mostly dependent on agriculture. Nowadays, farmer’s
organization can use predictive models by using past data. A revenue model can estimate the
effect of a customer’s catalog using and a linear regression model can estimate the revenue
generating from it. The organization can differentiate between the people who responded to the
catalogs in purchasing goods and those who had not used catalogs and by target the right
customers.
1 out of 39
Related Documents
![[object Object]](/_next/image/?url=%2F_next%2Fstatic%2Fmedia%2Flogo.6d15ce61.png&w=640&q=75)
Your All-in-One AI-Powered Toolkit for Academic Success.
+13062052269
info@desklib.com
Available 24*7 on WhatsApp / Email
Unlock your academic potential
© 2024 | Zucol Services PVT LTD | All rights reserved.