Business Processing Engineering
VerifiedAdded on 2023/06/11
|10
|2442
|494
AI Summary
This paper discusses the impact of cognitive computing on Business Process Management (BPM) and explores how it will transform the BPM ecosystem in fundamental ways. It also lays out a framework for understanding how cognitive computing will impact BPM over the next several years, including cognitive process abstractions, cognitive process learning, and cognitive process enablement. The paper concludes by discussing the research challenges that cognitive computing brings to BPM and the most important research themes that are most central to the BPM community.
Contribute Materials
Your contribution can guide someone’s learning journey. Share your
documents today.

Running head: BUSINESS PROCESSING ENGINEERING
Business Processing Engineering
[Name of the Student]
[Name of the University]
[Author note]
Business Processing Engineering
[Name of the Student]
[Name of the University]
[Author note]
Secure Best Marks with AI Grader
Need help grading? Try our AI Grader for instant feedback on your assignments.
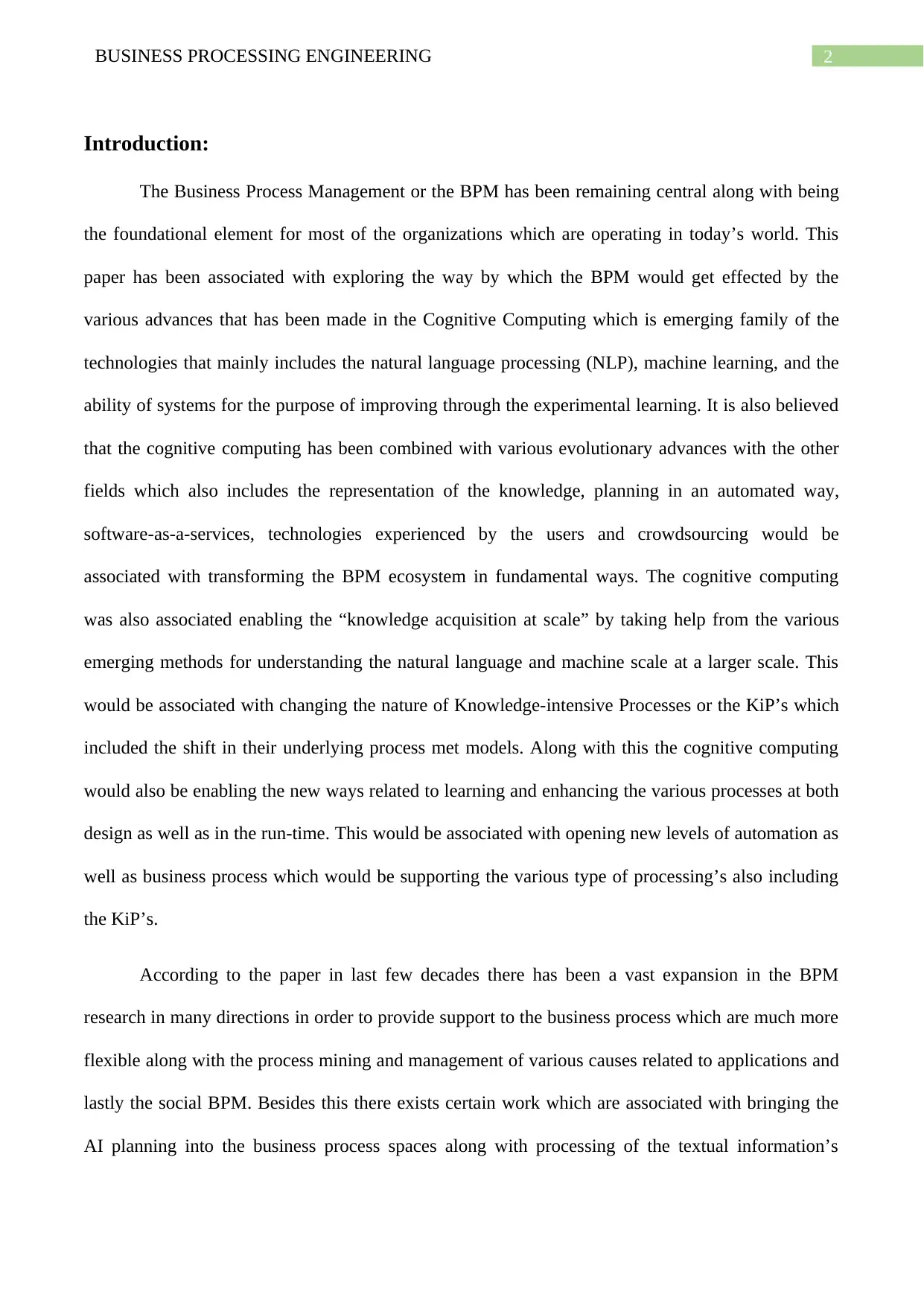
2BUSINESS PROCESSING ENGINEERING
Introduction:
The Business Process Management or the BPM has been remaining central along with being
the foundational element for most of the organizations which are operating in today’s world. This
paper has been associated with exploring the way by which the BPM would get effected by the
various advances that has been made in the Cognitive Computing which is emerging family of the
technologies that mainly includes the natural language processing (NLP), machine learning, and the
ability of systems for the purpose of improving through the experimental learning. It is also believed
that the cognitive computing has been combined with various evolutionary advances with the other
fields which also includes the representation of the knowledge, planning in an automated way,
software-as-a-services, technologies experienced by the users and crowdsourcing would be
associated with transforming the BPM ecosystem in fundamental ways. The cognitive computing
was also associated enabling the “knowledge acquisition at scale” by taking help from the various
emerging methods for understanding the natural language and machine scale at a larger scale. This
would be associated with changing the nature of Knowledge-intensive Processes or the KiP’s which
included the shift in their underlying process met models. Along with this the cognitive computing
would also be enabling the new ways related to learning and enhancing the various processes at both
design as well as in the run-time. This would be associated with opening new levels of automation as
well as business process which would be supporting the various type of processing’s also including
the KiP’s.
According to the paper in last few decades there has been a vast expansion in the BPM
research in many directions in order to provide support to the business process which are much more
flexible along with the process mining and management of various causes related to applications and
lastly the social BPM. Besides this there exists certain work which are associated with bringing the
AI planning into the business process spaces along with processing of the textual information’s
Introduction:
The Business Process Management or the BPM has been remaining central along with being
the foundational element for most of the organizations which are operating in today’s world. This
paper has been associated with exploring the way by which the BPM would get effected by the
various advances that has been made in the Cognitive Computing which is emerging family of the
technologies that mainly includes the natural language processing (NLP), machine learning, and the
ability of systems for the purpose of improving through the experimental learning. It is also believed
that the cognitive computing has been combined with various evolutionary advances with the other
fields which also includes the representation of the knowledge, planning in an automated way,
software-as-a-services, technologies experienced by the users and crowdsourcing would be
associated with transforming the BPM ecosystem in fundamental ways. The cognitive computing
was also associated enabling the “knowledge acquisition at scale” by taking help from the various
emerging methods for understanding the natural language and machine scale at a larger scale. This
would be associated with changing the nature of Knowledge-intensive Processes or the KiP’s which
included the shift in their underlying process met models. Along with this the cognitive computing
would also be enabling the new ways related to learning and enhancing the various processes at both
design as well as in the run-time. This would be associated with opening new levels of automation as
well as business process which would be supporting the various type of processing’s also including
the KiP’s.
According to the paper in last few decades there has been a vast expansion in the BPM
research in many directions in order to provide support to the business process which are much more
flexible along with the process mining and management of various causes related to applications and
lastly the social BPM. Besides this there exists certain work which are associated with bringing the
AI planning into the business process spaces along with processing of the textual information’s
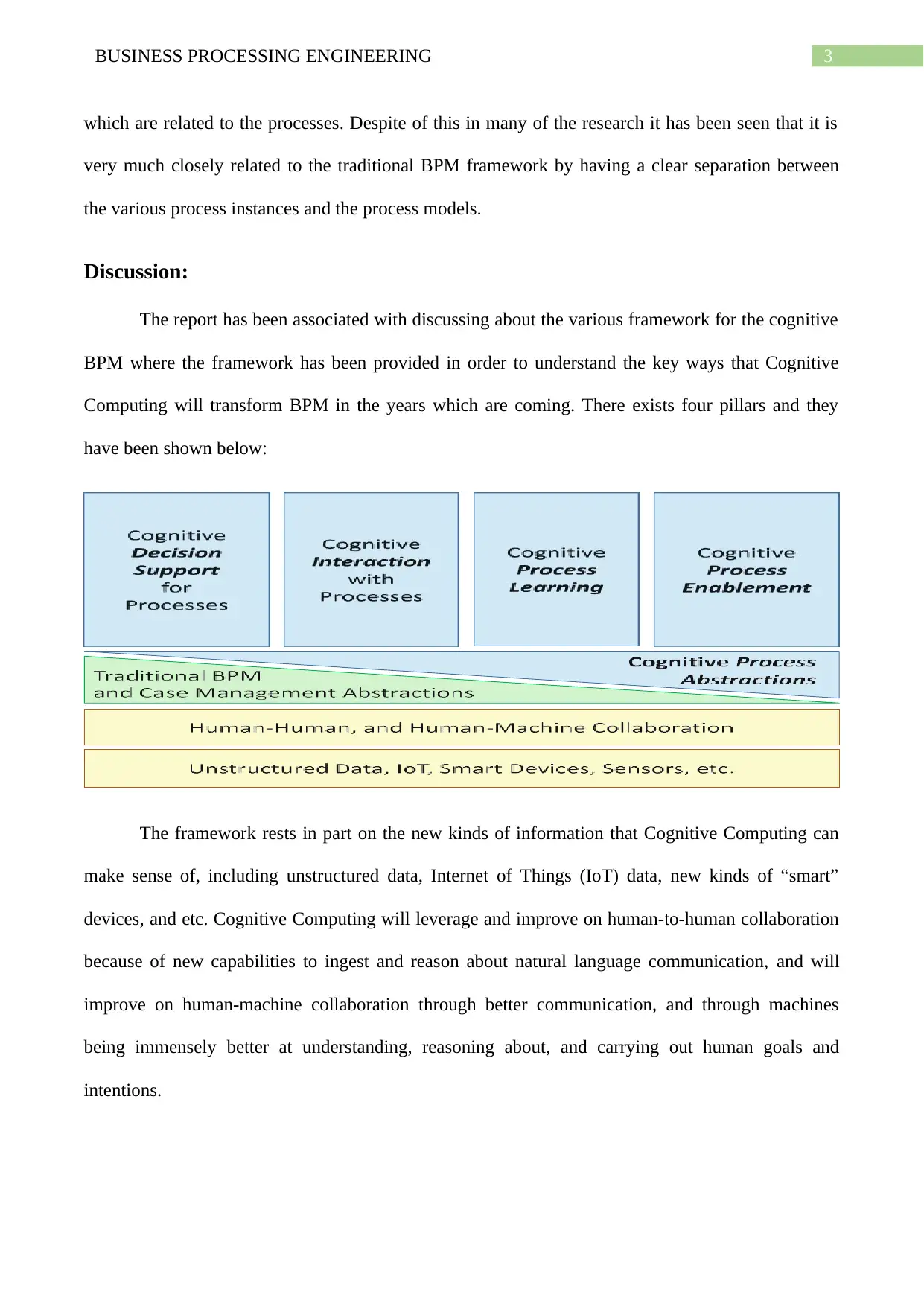
3BUSINESS PROCESSING ENGINEERING
which are related to the processes. Despite of this in many of the research it has been seen that it is
very much closely related to the traditional BPM framework by having a clear separation between
the various process instances and the process models.
Discussion:
The report has been associated with discussing about the various framework for the cognitive
BPM where the framework has been provided in order to understand the key ways that Cognitive
Computing will transform BPM in the years which are coming. There exists four pillars and they
have been shown below:
The framework rests in part on the new kinds of information that Cognitive Computing can
make sense of, including unstructured data, Internet of Things (IoT) data, new kinds of “smart”
devices, and etc. Cognitive Computing will leverage and improve on human-to-human collaboration
because of new capabilities to ingest and reason about natural language communication, and will
improve on human-machine collaboration through better communication, and through machines
being immensely better at understanding, reasoning about, and carrying out human goals and
intentions.
which are related to the processes. Despite of this in many of the research it has been seen that it is
very much closely related to the traditional BPM framework by having a clear separation between
the various process instances and the process models.
Discussion:
The report has been associated with discussing about the various framework for the cognitive
BPM where the framework has been provided in order to understand the key ways that Cognitive
Computing will transform BPM in the years which are coming. There exists four pillars and they
have been shown below:
The framework rests in part on the new kinds of information that Cognitive Computing can
make sense of, including unstructured data, Internet of Things (IoT) data, new kinds of “smart”
devices, and etc. Cognitive Computing will leverage and improve on human-to-human collaboration
because of new capabilities to ingest and reason about natural language communication, and will
improve on human-machine collaboration through better communication, and through machines
being immensely better at understanding, reasoning about, and carrying out human goals and
intentions.
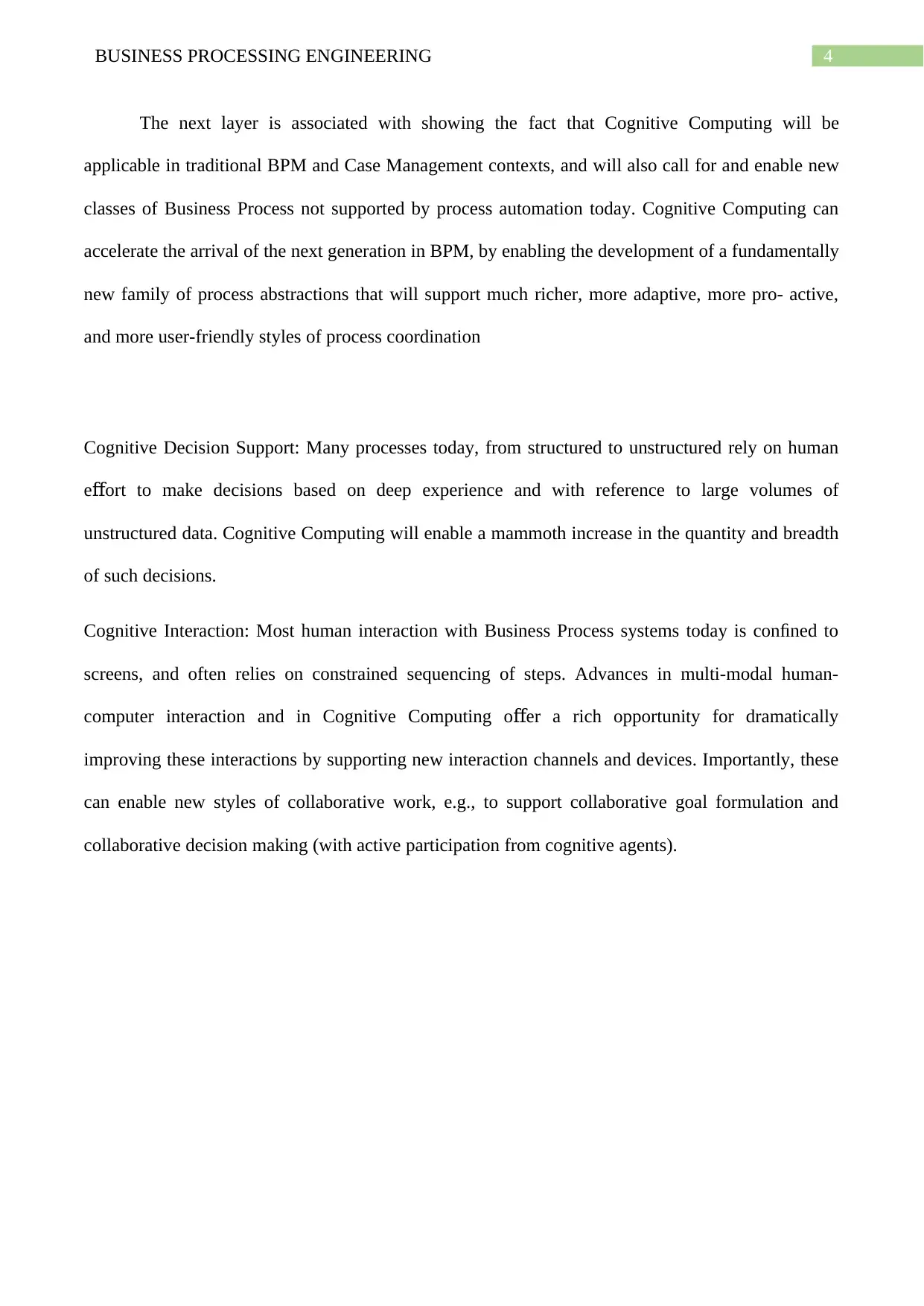
4BUSINESS PROCESSING ENGINEERING
The next layer is associated with showing the fact that Cognitive Computing will be
applicable in traditional BPM and Case Management contexts, and will also call for and enable new
classes of Business Process not supported by process automation today. Cognitive Computing can
accelerate the arrival of the next generation in BPM, by enabling the development of a fundamentally
new family of process abstractions that will support much richer, more adaptive, more pro- active,
and more user-friendly styles of process coordination
Cognitive Decision Support: Many processes today, from structured to unstructured rely on human
e ort to make decisions based on deep experience and with reference to large volumes offf
unstructured data. Cognitive Computing will enable a mammoth increase in the quantity and breadth
of such decisions.
Cognitive Interaction: Most human interaction with Business Process systems today is confined to
screens, and often relies on constrained sequencing of steps. Advances in multi-modal human-
computer interaction and in Cognitive Computing o er a rich opportunity for dramaticallyff
improving these interactions by supporting new interaction channels and devices. Importantly, these
can enable new styles of collaborative work, e.g., to support collaborative goal formulation and
collaborative decision making (with active participation from cognitive agents).
The next layer is associated with showing the fact that Cognitive Computing will be
applicable in traditional BPM and Case Management contexts, and will also call for and enable new
classes of Business Process not supported by process automation today. Cognitive Computing can
accelerate the arrival of the next generation in BPM, by enabling the development of a fundamentally
new family of process abstractions that will support much richer, more adaptive, more pro- active,
and more user-friendly styles of process coordination
Cognitive Decision Support: Many processes today, from structured to unstructured rely on human
e ort to make decisions based on deep experience and with reference to large volumes offf
unstructured data. Cognitive Computing will enable a mammoth increase in the quantity and breadth
of such decisions.
Cognitive Interaction: Most human interaction with Business Process systems today is confined to
screens, and often relies on constrained sequencing of steps. Advances in multi-modal human-
computer interaction and in Cognitive Computing o er a rich opportunity for dramaticallyff
improving these interactions by supporting new interaction channels and devices. Importantly, these
can enable new styles of collaborative work, e.g., to support collaborative goal formulation and
collaborative decision making (with active participation from cognitive agents).
Secure Best Marks with AI Grader
Need help grading? Try our AI Grader for instant feedback on your assignments.
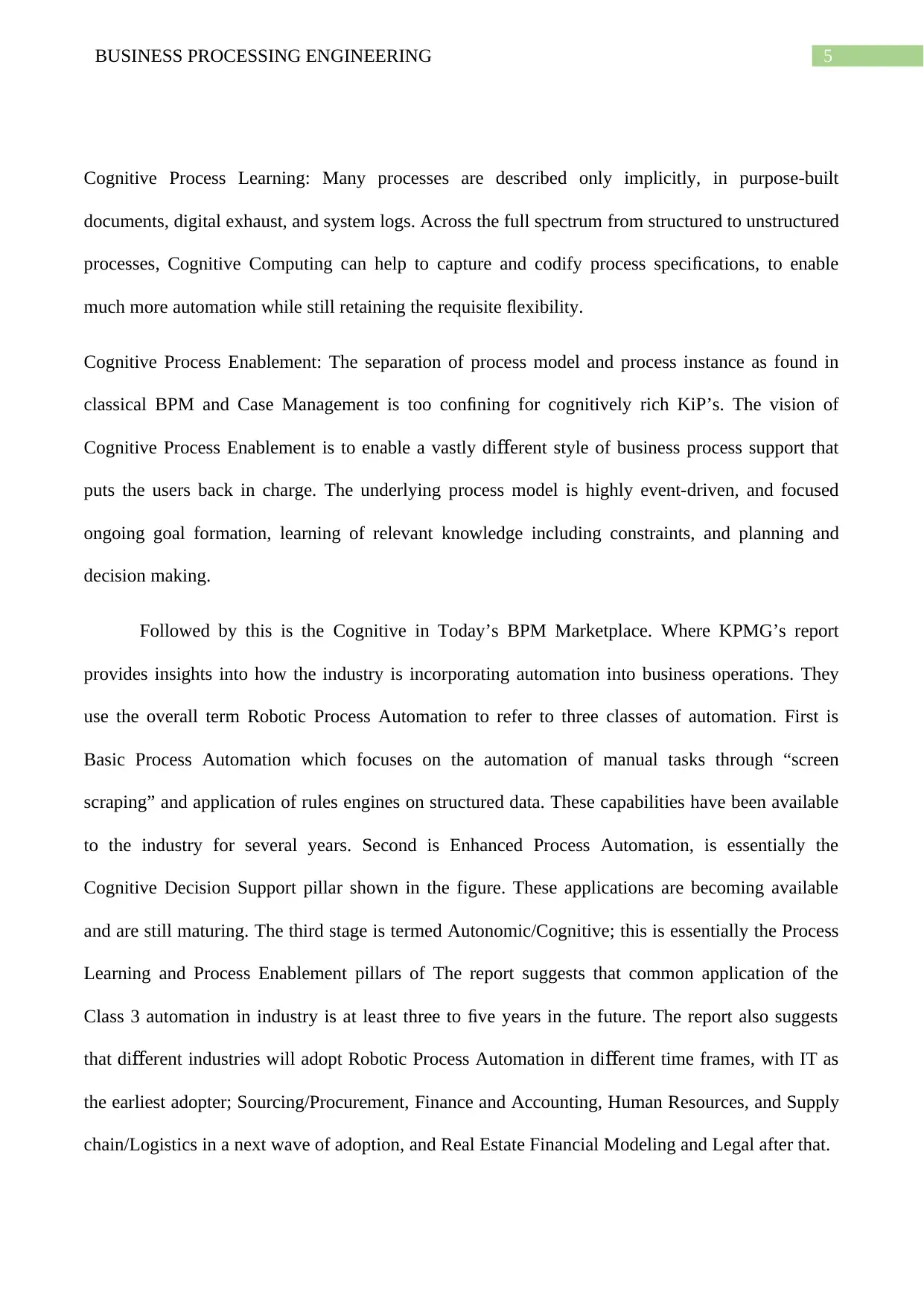
5BUSINESS PROCESSING ENGINEERING
Cognitive Process Learning: Many processes are described only implicitly, in purpose-built
documents, digital exhaust, and system logs. Across the full spectrum from structured to unstructured
processes, Cognitive Computing can help to capture and codify process specifications, to enable
much more automation while still retaining the requisite flexibility.
Cognitive Process Enablement: The separation of process model and process instance as found in
classical BPM and Case Management is too confining for cognitively rich KiP’s. The vision of
Cognitive Process Enablement is to enable a vastly di erent style of business process support thatff
puts the users back in charge. The underlying process model is highly event-driven, and focused
ongoing goal formation, learning of relevant knowledge including constraints, and planning and
decision making.
Followed by this is the Cognitive in Today’s BPM Marketplace. Where KPMG’s report
provides insights into how the industry is incorporating automation into business operations. They
use the overall term Robotic Process Automation to refer to three classes of automation. First is
Basic Process Automation which focuses on the automation of manual tasks through “screen
scraping” and application of rules engines on structured data. These capabilities have been available
to the industry for several years. Second is Enhanced Process Automation, is essentially the
Cognitive Decision Support pillar shown in the figure. These applications are becoming available
and are still maturing. The third stage is termed Autonomic/Cognitive; this is essentially the Process
Learning and Process Enablement pillars of The report suggests that common application of the
Class 3 automation in industry is at least three to five years in the future. The report also suggests
that di erent industries will adopt Robotic Process Automation in di erent time frames, with IT asff ff
the earliest adopter; Sourcing/Procurement, Finance and Accounting, Human Resources, and Supply
chain/Logistics in a next wave of adoption, and Real Estate Financial Modeling and Legal after that.
Cognitive Process Learning: Many processes are described only implicitly, in purpose-built
documents, digital exhaust, and system logs. Across the full spectrum from structured to unstructured
processes, Cognitive Computing can help to capture and codify process specifications, to enable
much more automation while still retaining the requisite flexibility.
Cognitive Process Enablement: The separation of process model and process instance as found in
classical BPM and Case Management is too confining for cognitively rich KiP’s. The vision of
Cognitive Process Enablement is to enable a vastly di erent style of business process support thatff
puts the users back in charge. The underlying process model is highly event-driven, and focused
ongoing goal formation, learning of relevant knowledge including constraints, and planning and
decision making.
Followed by this is the Cognitive in Today’s BPM Marketplace. Where KPMG’s report
provides insights into how the industry is incorporating automation into business operations. They
use the overall term Robotic Process Automation to refer to three classes of automation. First is
Basic Process Automation which focuses on the automation of manual tasks through “screen
scraping” and application of rules engines on structured data. These capabilities have been available
to the industry for several years. Second is Enhanced Process Automation, is essentially the
Cognitive Decision Support pillar shown in the figure. These applications are becoming available
and are still maturing. The third stage is termed Autonomic/Cognitive; this is essentially the Process
Learning and Process Enablement pillars of The report suggests that common application of the
Class 3 automation in industry is at least three to five years in the future. The report also suggests
that di erent industries will adopt Robotic Process Automation in di erent time frames, with IT asff ff
the earliest adopter; Sourcing/Procurement, Finance and Accounting, Human Resources, and Supply
chain/Logistics in a next wave of adoption, and Real Estate Financial Modeling and Legal after that.
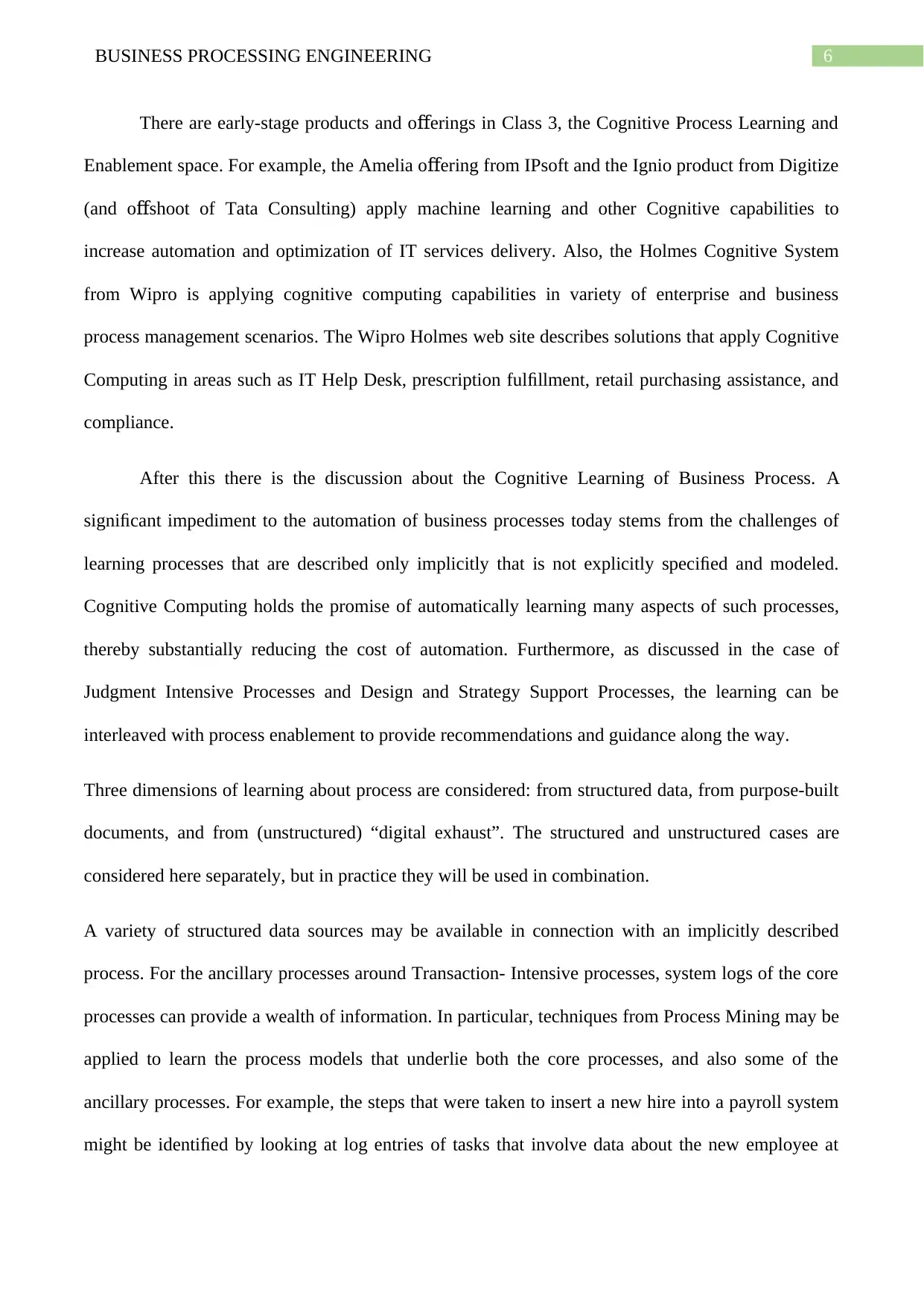
6BUSINESS PROCESSING ENGINEERING
There are early-stage products and o erings in Class 3, the Cognitive Process Learning andff
Enablement space. For example, the Amelia o ering from IPsoft and the Ignio product from Digitizeff
(and o shoot of Tata Consulting) apply machine learning and other Cognitive capabilities toff
increase automation and optimization of IT services delivery. Also, the Holmes Cognitive System
from Wipro is applying cognitive computing capabilities in variety of enterprise and business
process management scenarios. The Wipro Holmes web site describes solutions that apply Cognitive
Computing in areas such as IT Help Desk, prescription fulfillment, retail purchasing assistance, and
compliance.
After this there is the discussion about the Cognitive Learning of Business Process. A
significant impediment to the automation of business processes today stems from the challenges of
learning processes that are described only implicitly that is not explicitly specified and modeled.
Cognitive Computing holds the promise of automatically learning many aspects of such processes,
thereby substantially reducing the cost of automation. Furthermore, as discussed in the case of
Judgment Intensive Processes and Design and Strategy Support Processes, the learning can be
interleaved with process enablement to provide recommendations and guidance along the way.
Three dimensions of learning about process are considered: from structured data, from purpose-built
documents, and from (unstructured) “digital exhaust”. The structured and unstructured cases are
considered here separately, but in practice they will be used in combination.
A variety of structured data sources may be available in connection with an implicitly described
process. For the ancillary processes around Transaction- Intensive processes, system logs of the core
processes can provide a wealth of information. In particular, techniques from Process Mining may be
applied to learn the process models that underlie both the core processes, and also some of the
ancillary processes. For example, the steps that were taken to insert a new hire into a payroll system
might be identified by looking at log entries of tasks that involve data about the new employee at
There are early-stage products and o erings in Class 3, the Cognitive Process Learning andff
Enablement space. For example, the Amelia o ering from IPsoft and the Ignio product from Digitizeff
(and o shoot of Tata Consulting) apply machine learning and other Cognitive capabilities toff
increase automation and optimization of IT services delivery. Also, the Holmes Cognitive System
from Wipro is applying cognitive computing capabilities in variety of enterprise and business
process management scenarios. The Wipro Holmes web site describes solutions that apply Cognitive
Computing in areas such as IT Help Desk, prescription fulfillment, retail purchasing assistance, and
compliance.
After this there is the discussion about the Cognitive Learning of Business Process. A
significant impediment to the automation of business processes today stems from the challenges of
learning processes that are described only implicitly that is not explicitly specified and modeled.
Cognitive Computing holds the promise of automatically learning many aspects of such processes,
thereby substantially reducing the cost of automation. Furthermore, as discussed in the case of
Judgment Intensive Processes and Design and Strategy Support Processes, the learning can be
interleaved with process enablement to provide recommendations and guidance along the way.
Three dimensions of learning about process are considered: from structured data, from purpose-built
documents, and from (unstructured) “digital exhaust”. The structured and unstructured cases are
considered here separately, but in practice they will be used in combination.
A variety of structured data sources may be available in connection with an implicitly described
process. For the ancillary processes around Transaction- Intensive processes, system logs of the core
processes can provide a wealth of information. In particular, techniques from Process Mining may be
applied to learn the process models that underlie both the core processes, and also some of the
ancillary processes. For example, the steps that were taken to insert a new hire into a payroll system
might be identified by looking at log entries of tasks that involve data about the new employee at
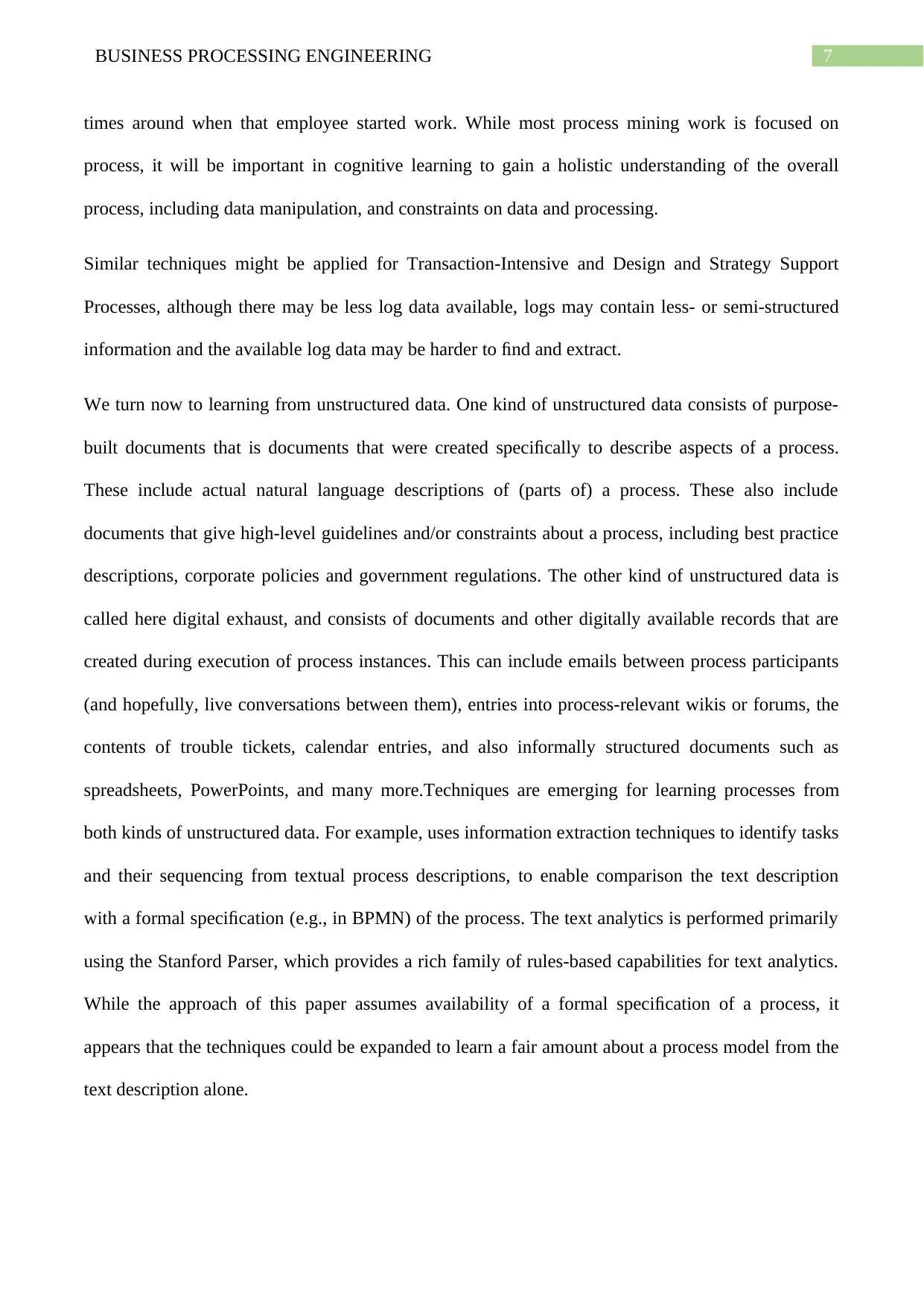
7BUSINESS PROCESSING ENGINEERING
times around when that employee started work. While most process mining work is focused on
process, it will be important in cognitive learning to gain a holistic understanding of the overall
process, including data manipulation, and constraints on data and processing.
Similar techniques might be applied for Transaction-Intensive and Design and Strategy Support
Processes, although there may be less log data available, logs may contain less- or semi-structured
information and the available log data may be harder to find and extract.
We turn now to learning from unstructured data. One kind of unstructured data consists of purpose-
built documents that is documents that were created specifically to describe aspects of a process.
These include actual natural language descriptions of (parts of) a process. These also include
documents that give high-level guidelines and/or constraints about a process, including best practice
descriptions, corporate policies and government regulations. The other kind of unstructured data is
called here digital exhaust, and consists of documents and other digitally available records that are
created during execution of process instances. This can include emails between process participants
(and hopefully, live conversations between them), entries into process-relevant wikis or forums, the
contents of trouble tickets, calendar entries, and also informally structured documents such as
spreadsheets, PowerPoints, and many more.Techniques are emerging for learning processes from
both kinds of unstructured data. For example, uses information extraction techniques to identify tasks
and their sequencing from textual process descriptions, to enable comparison the text description
with a formal specification (e.g., in BPMN) of the process. The text analytics is performed primarily
using the Stanford Parser, which provides a rich family of rules-based capabilities for text analytics.
While the approach of this paper assumes availability of a formal specification of a process, it
appears that the techniques could be expanded to learn a fair amount about a process model from the
text description alone.
times around when that employee started work. While most process mining work is focused on
process, it will be important in cognitive learning to gain a holistic understanding of the overall
process, including data manipulation, and constraints on data and processing.
Similar techniques might be applied for Transaction-Intensive and Design and Strategy Support
Processes, although there may be less log data available, logs may contain less- or semi-structured
information and the available log data may be harder to find and extract.
We turn now to learning from unstructured data. One kind of unstructured data consists of purpose-
built documents that is documents that were created specifically to describe aspects of a process.
These include actual natural language descriptions of (parts of) a process. These also include
documents that give high-level guidelines and/or constraints about a process, including best practice
descriptions, corporate policies and government regulations. The other kind of unstructured data is
called here digital exhaust, and consists of documents and other digitally available records that are
created during execution of process instances. This can include emails between process participants
(and hopefully, live conversations between them), entries into process-relevant wikis or forums, the
contents of trouble tickets, calendar entries, and also informally structured documents such as
spreadsheets, PowerPoints, and many more.Techniques are emerging for learning processes from
both kinds of unstructured data. For example, uses information extraction techniques to identify tasks
and their sequencing from textual process descriptions, to enable comparison the text description
with a formal specification (e.g., in BPMN) of the process. The text analytics is performed primarily
using the Stanford Parser, which provides a rich family of rules-based capabilities for text analytics.
While the approach of this paper assumes availability of a formal specification of a process, it
appears that the techniques could be expanded to learn a fair amount about a process model from the
text description alone.
Paraphrase This Document
Need a fresh take? Get an instant paraphrase of this document with our AI Paraphraser

8BUSINESS PROCESSING ENGINEERING
An emerging sub-area that is gaining attention is to apply text analytics to government regulations to
extract rules and constraints on processes to ensure compliance. This combines both statistical and
rules-based approaches to NLP: first, statistically-based techniques are used to classify sentences that
are deemed to hold regulatory information; second, these are processed using a rules-based approach,
and Rules relating to both industry-specific terminologies and an industry-specific ontology are also
used.
Conclusion:
This paper has laid out a framework for understanding how Cognitive Computing will impact the
practice of BPM over the next several years, and focused primarily on emerging perspectives for
cognitive process abstractions, cognitive process learning, and cognitive process enablement. Our
findings are relevant to the full spectrum of business processes, from Transaction-Intensive, to
Judgment-Intensive, to Design and Strategy Support.
This paper describes many of the research challenges that Cognitive Computing brings to BPM. We
conclude by reiterating the most important of the research themes that are most central to the BPM
community.
Automatic Learning about Business Process. This learning will be at “design time” (e.g., from
purpose-built documents, historical digital exhaust, and system logs), and at “run time” (e.g., from
asserted requirements and goals, fresh digital exhaust including human collaborations, and the
process instance history). The learning needs to be geared towards process automation and
enhancement, including semi-automated Project Management, pro-active knowledge acquisition, and
guiding of human activities.
Embracing Flexibility: The Plan-Act-Learn cycle. A new kind of process meta- model is needed for
KiP’s in the context of “knowledge acquisition at scale”. We have proposed to base this on the Plan-
Act-Learn cycle. But there is a huge distance between this high-level proposal and a robust
An emerging sub-area that is gaining attention is to apply text analytics to government regulations to
extract rules and constraints on processes to ensure compliance. This combines both statistical and
rules-based approaches to NLP: first, statistically-based techniques are used to classify sentences that
are deemed to hold regulatory information; second, these are processed using a rules-based approach,
and Rules relating to both industry-specific terminologies and an industry-specific ontology are also
used.
Conclusion:
This paper has laid out a framework for understanding how Cognitive Computing will impact the
practice of BPM over the next several years, and focused primarily on emerging perspectives for
cognitive process abstractions, cognitive process learning, and cognitive process enablement. Our
findings are relevant to the full spectrum of business processes, from Transaction-Intensive, to
Judgment-Intensive, to Design and Strategy Support.
This paper describes many of the research challenges that Cognitive Computing brings to BPM. We
conclude by reiterating the most important of the research themes that are most central to the BPM
community.
Automatic Learning about Business Process. This learning will be at “design time” (e.g., from
purpose-built documents, historical digital exhaust, and system logs), and at “run time” (e.g., from
asserted requirements and goals, fresh digital exhaust including human collaborations, and the
process instance history). The learning needs to be geared towards process automation and
enhancement, including semi-automated Project Management, pro-active knowledge acquisition, and
guiding of human activities.
Embracing Flexibility: The Plan-Act-Learn cycle. A new kind of process meta- model is needed for
KiP’s in the context of “knowledge acquisition at scale”. We have proposed to base this on the Plan-
Act-Learn cycle. But there is a huge distance between this high-level proposal and a robust
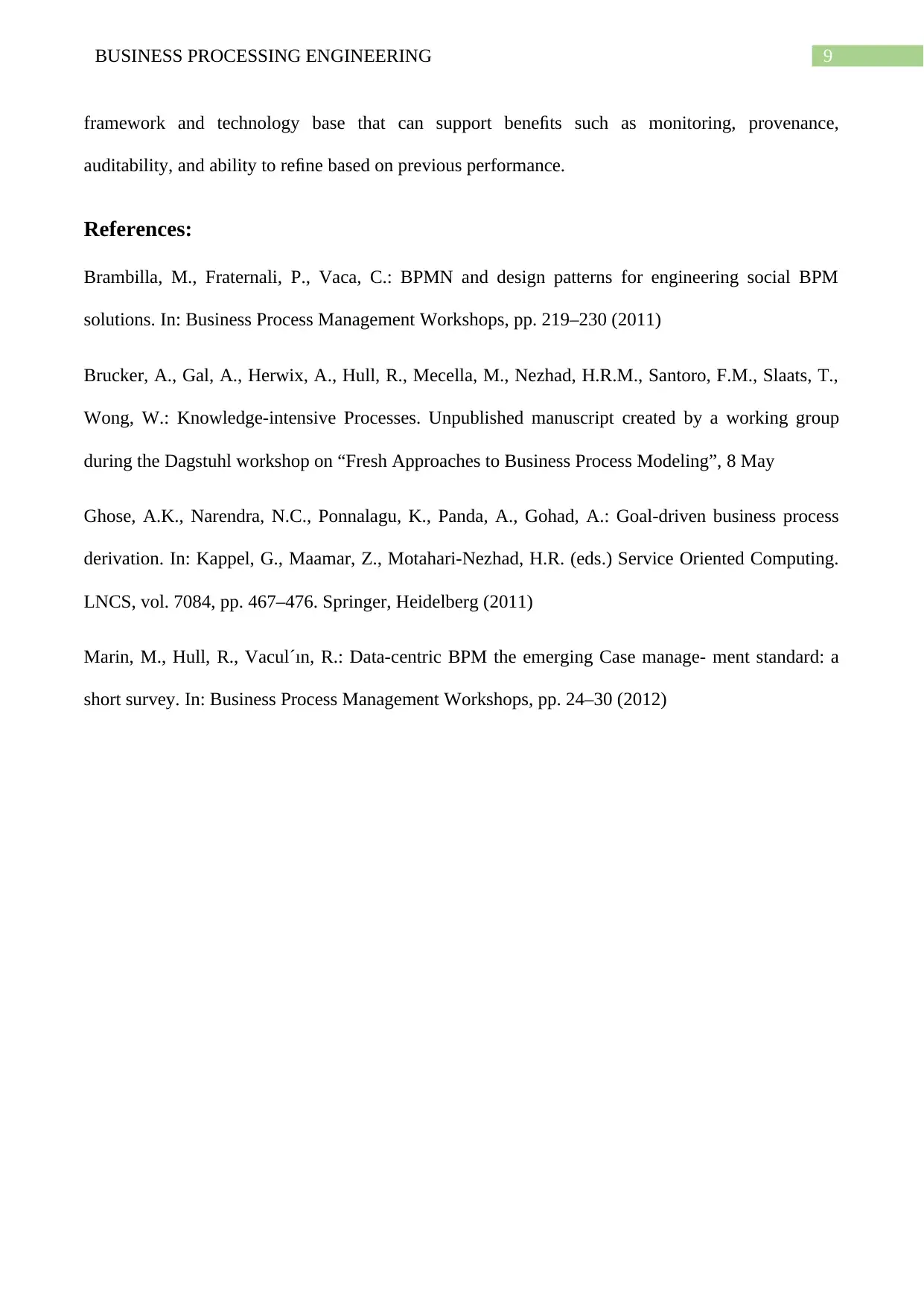
9BUSINESS PROCESSING ENGINEERING
framework and technology base that can support benefits such as monitoring, provenance,
auditability, and ability to refine based on previous performance.
References:
Brambilla, M., Fraternali, P., Vaca, C.: BPMN and design patterns for engineering social BPM
solutions. In: Business Process Management Workshops, pp. 219–230 (2011)
Brucker, A., Gal, A., Herwix, A., Hull, R., Mecella, M., Nezhad, H.R.M., Santoro, F.M., Slaats, T.,
Wong, W.: Knowledge-intensive Processes. Unpublished manuscript created by a working group
during the Dagstuhl workshop on “Fresh Approaches to Business Process Modeling”, 8 May
Ghose, A.K., Narendra, N.C., Ponnalagu, K., Panda, A., Gohad, A.: Goal-driven business process
derivation. In: Kappel, G., Maamar, Z., Motahari-Nezhad, H.R. (eds.) Service Oriented Computing.
LNCS, vol. 7084, pp. 467–476. Springer, Heidelberg (2011)
Marin, M., Hull, R., Vacul´ın, R.: Data-centric BPM the emerging Case manage- ment standard: a
short survey. In: Business Process Management Workshops, pp. 24–30 (2012)
framework and technology base that can support benefits such as monitoring, provenance,
auditability, and ability to refine based on previous performance.
References:
Brambilla, M., Fraternali, P., Vaca, C.: BPMN and design patterns for engineering social BPM
solutions. In: Business Process Management Workshops, pp. 219–230 (2011)
Brucker, A., Gal, A., Herwix, A., Hull, R., Mecella, M., Nezhad, H.R.M., Santoro, F.M., Slaats, T.,
Wong, W.: Knowledge-intensive Processes. Unpublished manuscript created by a working group
during the Dagstuhl workshop on “Fresh Approaches to Business Process Modeling”, 8 May
Ghose, A.K., Narendra, N.C., Ponnalagu, K., Panda, A., Gohad, A.: Goal-driven business process
derivation. In: Kappel, G., Maamar, Z., Motahari-Nezhad, H.R. (eds.) Service Oriented Computing.
LNCS, vol. 7084, pp. 467–476. Springer, Heidelberg (2011)
Marin, M., Hull, R., Vacul´ın, R.: Data-centric BPM the emerging Case manage- ment standard: a
short survey. In: Business Process Management Workshops, pp. 24–30 (2012)
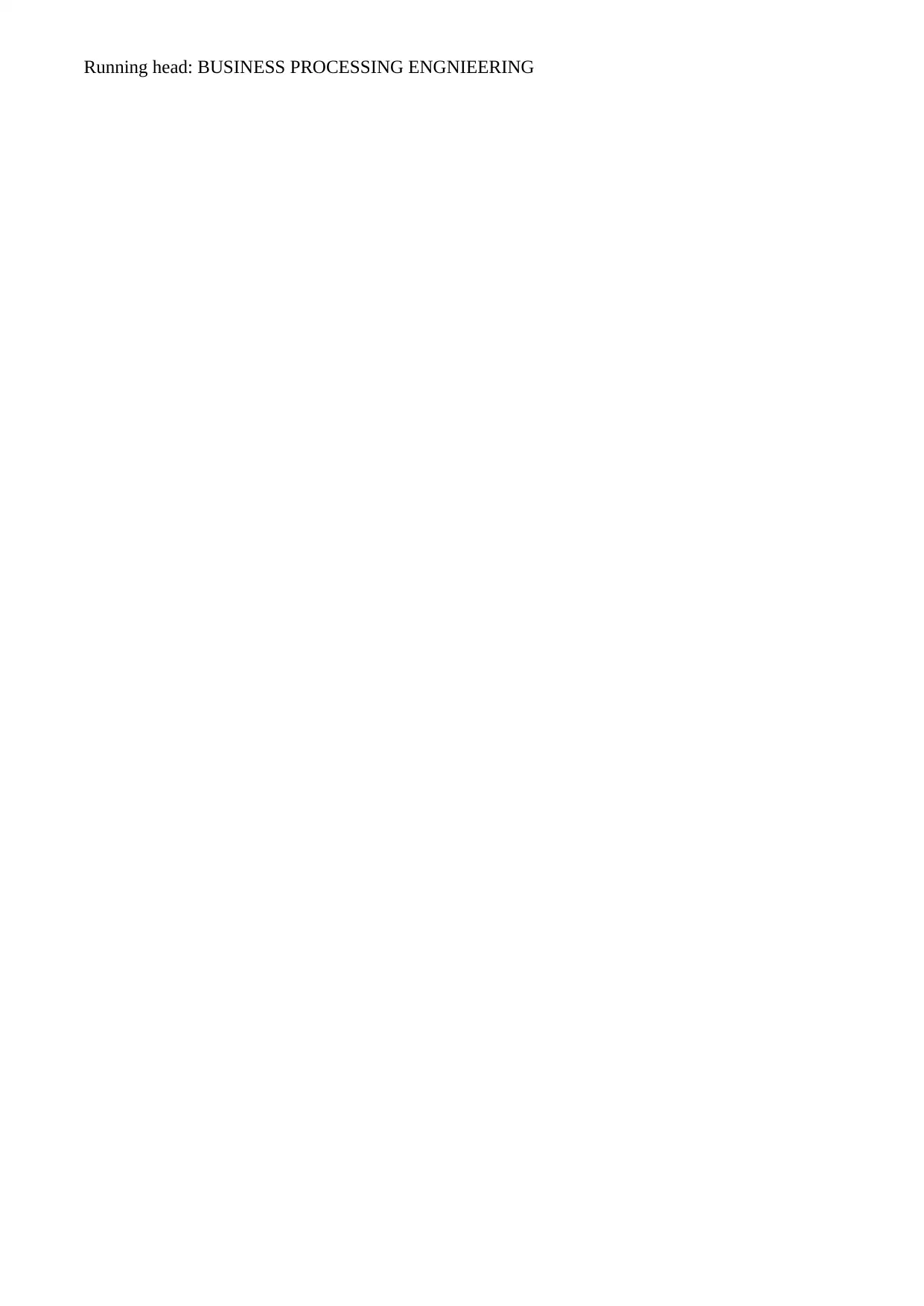
Running head: BUSINESS PROCESSING ENGNIEERING
1 out of 10
Related Documents
![[object Object]](/_next/image/?url=%2F_next%2Fstatic%2Fmedia%2Flogo.6d15ce61.png&w=640&q=75)
Your All-in-One AI-Powered Toolkit for Academic Success.
+13062052269
info@desklib.com
Available 24*7 on WhatsApp / Email
Unlock your academic potential
© 2024 | Zucol Services PVT LTD | All rights reserved.