Economics Study Material and Solved Assignments
VerifiedAdded on 2022/12/16
|11
|1720
|198
AI Summary
This document provides study material and solved assignments for Economics. It covers topics such as stationarity, serial correlation, linear probability model, fixed effect model, instrumental variable, simultaneous equation, and more. The document also includes references for further reading.
Contribute Materials
Your contribution can guide someone’s learning journey. Share your
documents today.
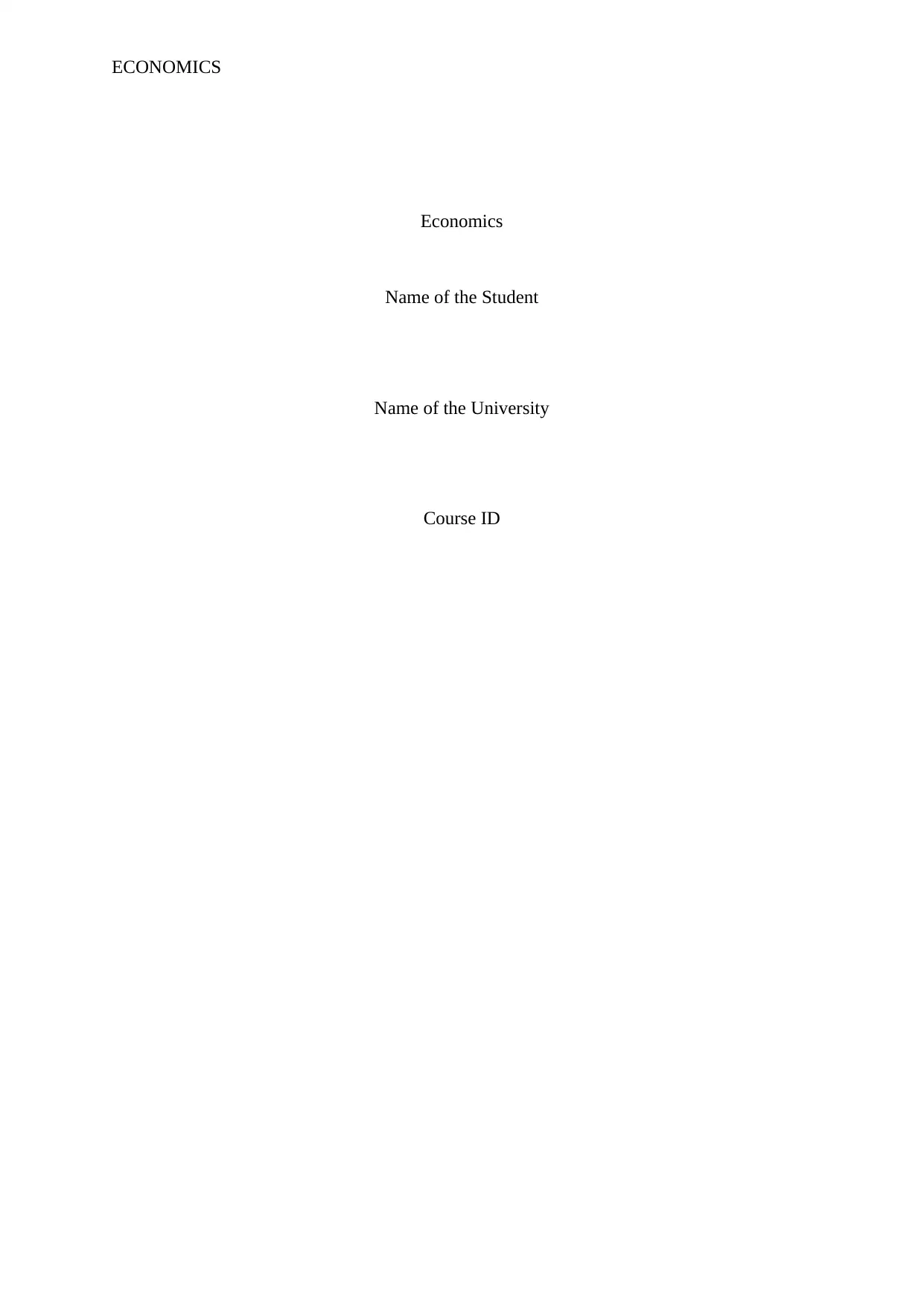
ECONOMICS
Economics
Name of the Student
Name of the University
Course ID
Economics
Name of the Student
Name of the University
Course ID
Secure Best Marks with AI Grader
Need help grading? Try our AI Grader for instant feedback on your assignments.
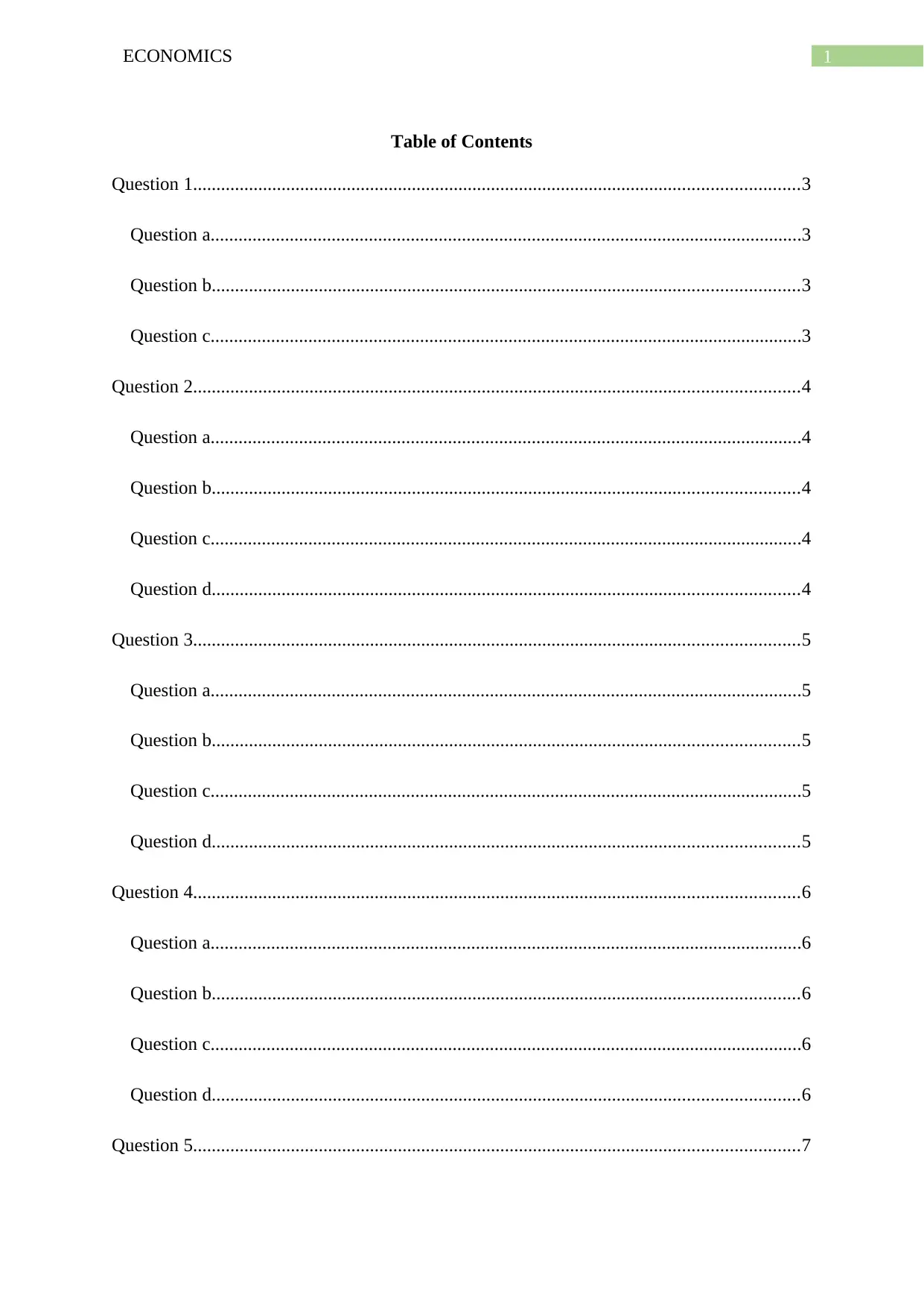
1ECONOMICS
Table of Contents
Question 1..................................................................................................................................3
Question a...............................................................................................................................3
Question b..............................................................................................................................3
Question c...............................................................................................................................3
Question 2..................................................................................................................................4
Question a...............................................................................................................................4
Question b..............................................................................................................................4
Question c...............................................................................................................................4
Question d..............................................................................................................................4
Question 3..................................................................................................................................5
Question a...............................................................................................................................5
Question b..............................................................................................................................5
Question c...............................................................................................................................5
Question d..............................................................................................................................5
Question 4..................................................................................................................................6
Question a...............................................................................................................................6
Question b..............................................................................................................................6
Question c...............................................................................................................................6
Question d..............................................................................................................................6
Question 5..................................................................................................................................7
Table of Contents
Question 1..................................................................................................................................3
Question a...............................................................................................................................3
Question b..............................................................................................................................3
Question c...............................................................................................................................3
Question 2..................................................................................................................................4
Question a...............................................................................................................................4
Question b..............................................................................................................................4
Question c...............................................................................................................................4
Question d..............................................................................................................................4
Question 3..................................................................................................................................5
Question a...............................................................................................................................5
Question b..............................................................................................................................5
Question c...............................................................................................................................5
Question d..............................................................................................................................5
Question 4..................................................................................................................................6
Question a...............................................................................................................................6
Question b..............................................................................................................................6
Question c...............................................................................................................................6
Question d..............................................................................................................................6
Question 5..................................................................................................................................7
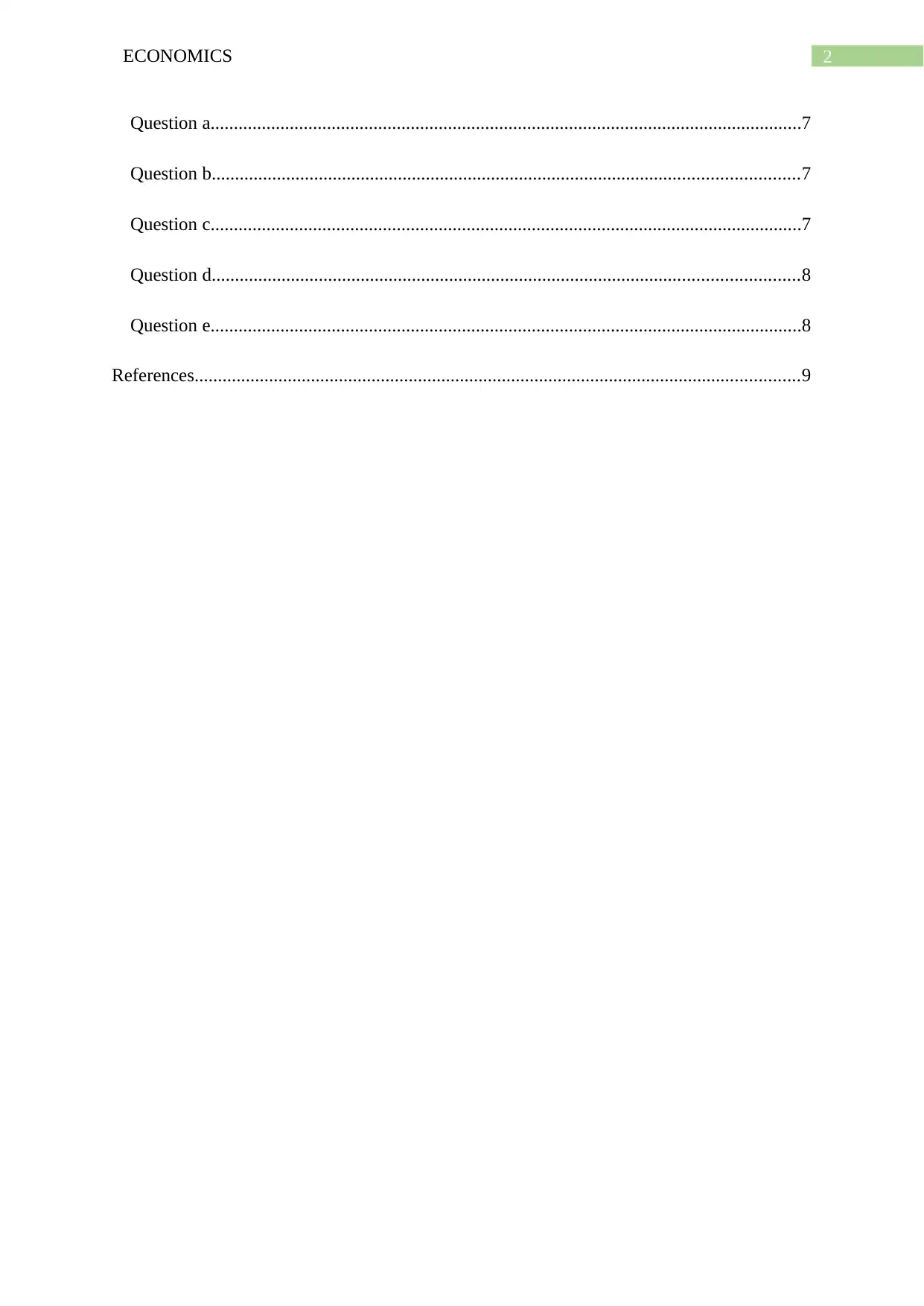
2ECONOMICS
Question a...............................................................................................................................7
Question b..............................................................................................................................7
Question c...............................................................................................................................7
Question d..............................................................................................................................8
Question e...............................................................................................................................8
References..................................................................................................................................9
Question a...............................................................................................................................7
Question b..............................................................................................................................7
Question c...............................................................................................................................7
Question d..............................................................................................................................8
Question e...............................................................................................................................8
References..................................................................................................................................9
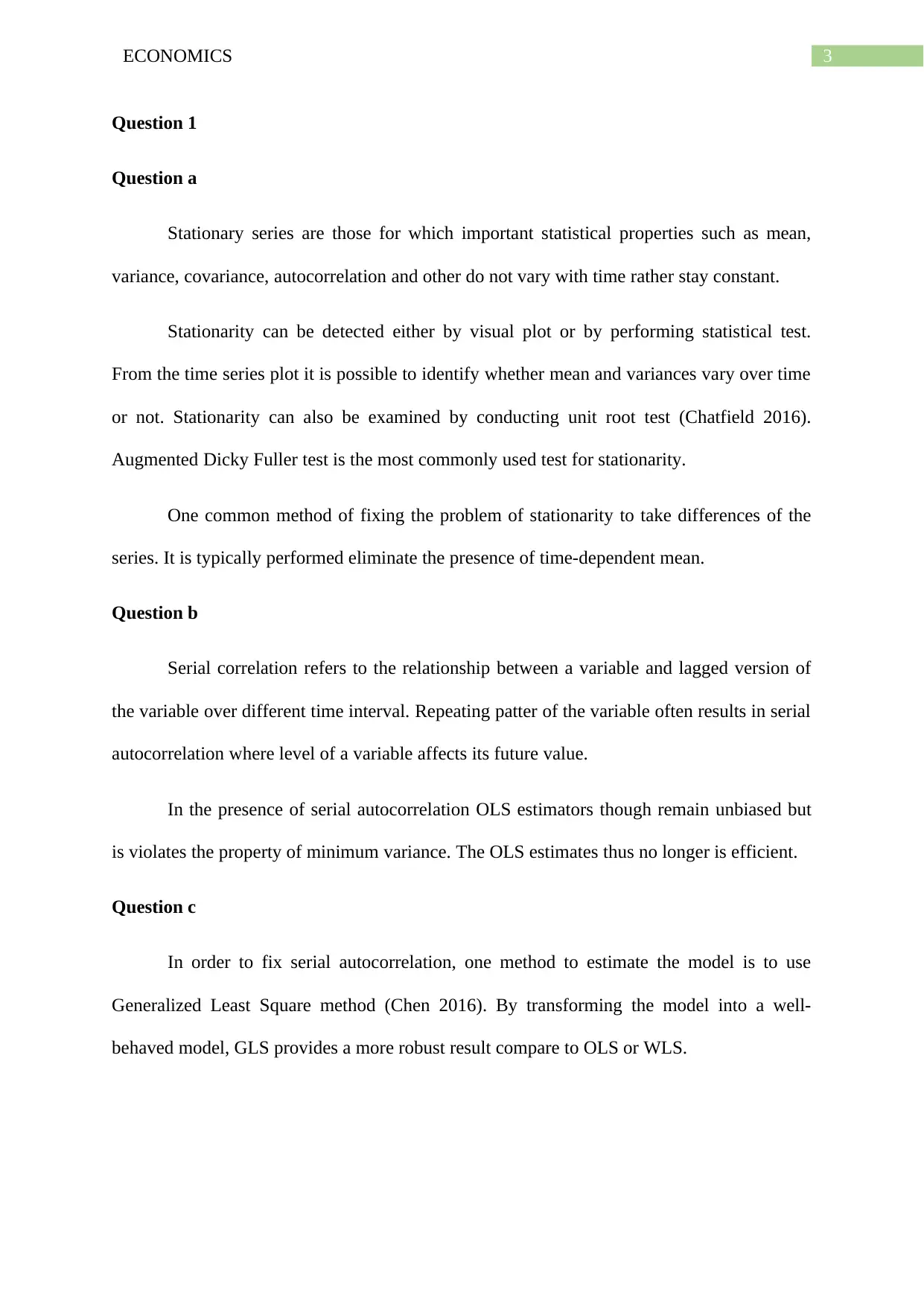
3ECONOMICS
Question 1
Question a
Stationary series are those for which important statistical properties such as mean,
variance, covariance, autocorrelation and other do not vary with time rather stay constant.
Stationarity can be detected either by visual plot or by performing statistical test.
From the time series plot it is possible to identify whether mean and variances vary over time
or not. Stationarity can also be examined by conducting unit root test (Chatfield 2016).
Augmented Dicky Fuller test is the most commonly used test for stationarity.
One common method of fixing the problem of stationarity to take differences of the
series. It is typically performed eliminate the presence of time-dependent mean.
Question b
Serial correlation refers to the relationship between a variable and lagged version of
the variable over different time interval. Repeating patter of the variable often results in serial
autocorrelation where level of a variable affects its future value.
In the presence of serial autocorrelation OLS estimators though remain unbiased but
is violates the property of minimum variance. The OLS estimates thus no longer is efficient.
Question c
In order to fix serial autocorrelation, one method to estimate the model is to use
Generalized Least Square method (Chen 2016). By transforming the model into a well-
behaved model, GLS provides a more robust result compare to OLS or WLS.
Question 1
Question a
Stationary series are those for which important statistical properties such as mean,
variance, covariance, autocorrelation and other do not vary with time rather stay constant.
Stationarity can be detected either by visual plot or by performing statistical test.
From the time series plot it is possible to identify whether mean and variances vary over time
or not. Stationarity can also be examined by conducting unit root test (Chatfield 2016).
Augmented Dicky Fuller test is the most commonly used test for stationarity.
One common method of fixing the problem of stationarity to take differences of the
series. It is typically performed eliminate the presence of time-dependent mean.
Question b
Serial correlation refers to the relationship between a variable and lagged version of
the variable over different time interval. Repeating patter of the variable often results in serial
autocorrelation where level of a variable affects its future value.
In the presence of serial autocorrelation OLS estimators though remain unbiased but
is violates the property of minimum variance. The OLS estimates thus no longer is efficient.
Question c
In order to fix serial autocorrelation, one method to estimate the model is to use
Generalized Least Square method (Chen 2016). By transforming the model into a well-
behaved model, GLS provides a more robust result compare to OLS or WLS.
Secure Best Marks with AI Grader
Need help grading? Try our AI Grader for instant feedback on your assignments.
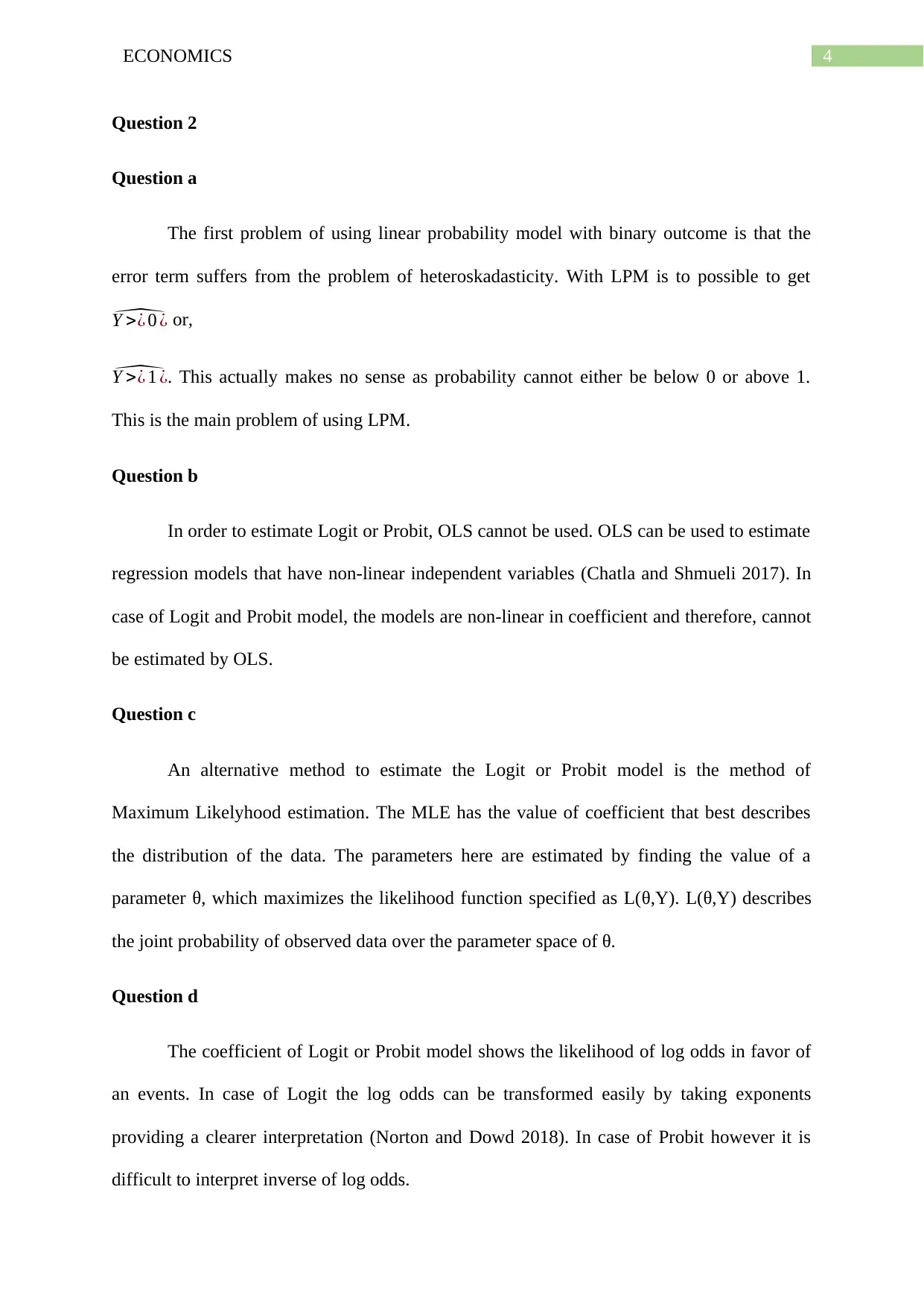
4ECONOMICS
Question 2
Question a
The first problem of using linear probability model with binary outcome is that the
error term suffers from the problem of heteroskadasticity. With LPM is to possible to get
^Y >¿ 0 ¿ or,
^Y >¿ 1 ¿. This actually makes no sense as probability cannot either be below 0 or above 1.
This is the main problem of using LPM.
Question b
In order to estimate Logit or Probit, OLS cannot be used. OLS can be used to estimate
regression models that have non-linear independent variables (Chatla and Shmueli 2017). In
case of Logit and Probit model, the models are non-linear in coefficient and therefore, cannot
be estimated by OLS.
Question c
An alternative method to estimate the Logit or Probit model is the method of
Maximum Likelyhood estimation. The MLE has the value of coefficient that best describes
the distribution of the data. The parameters here are estimated by finding the value of a
parameter θ, which maximizes the likelihood function specified as L(θ,Y). L(θ,Y) describes
the joint probability of observed data over the parameter space of θ.
Question d
The coefficient of Logit or Probit model shows the likelihood of log odds in favor of
an events. In case of Logit the log odds can be transformed easily by taking exponents
providing a clearer interpretation (Norton and Dowd 2018). In case of Probit however it is
difficult to interpret inverse of log odds.
Question 2
Question a
The first problem of using linear probability model with binary outcome is that the
error term suffers from the problem of heteroskadasticity. With LPM is to possible to get
^Y >¿ 0 ¿ or,
^Y >¿ 1 ¿. This actually makes no sense as probability cannot either be below 0 or above 1.
This is the main problem of using LPM.
Question b
In order to estimate Logit or Probit, OLS cannot be used. OLS can be used to estimate
regression models that have non-linear independent variables (Chatla and Shmueli 2017). In
case of Logit and Probit model, the models are non-linear in coefficient and therefore, cannot
be estimated by OLS.
Question c
An alternative method to estimate the Logit or Probit model is the method of
Maximum Likelyhood estimation. The MLE has the value of coefficient that best describes
the distribution of the data. The parameters here are estimated by finding the value of a
parameter θ, which maximizes the likelihood function specified as L(θ,Y). L(θ,Y) describes
the joint probability of observed data over the parameter space of θ.
Question d
The coefficient of Logit or Probit model shows the likelihood of log odds in favor of
an events. In case of Logit the log odds can be transformed easily by taking exponents
providing a clearer interpretation (Norton and Dowd 2018). In case of Probit however it is
difficult to interpret inverse of log odds.
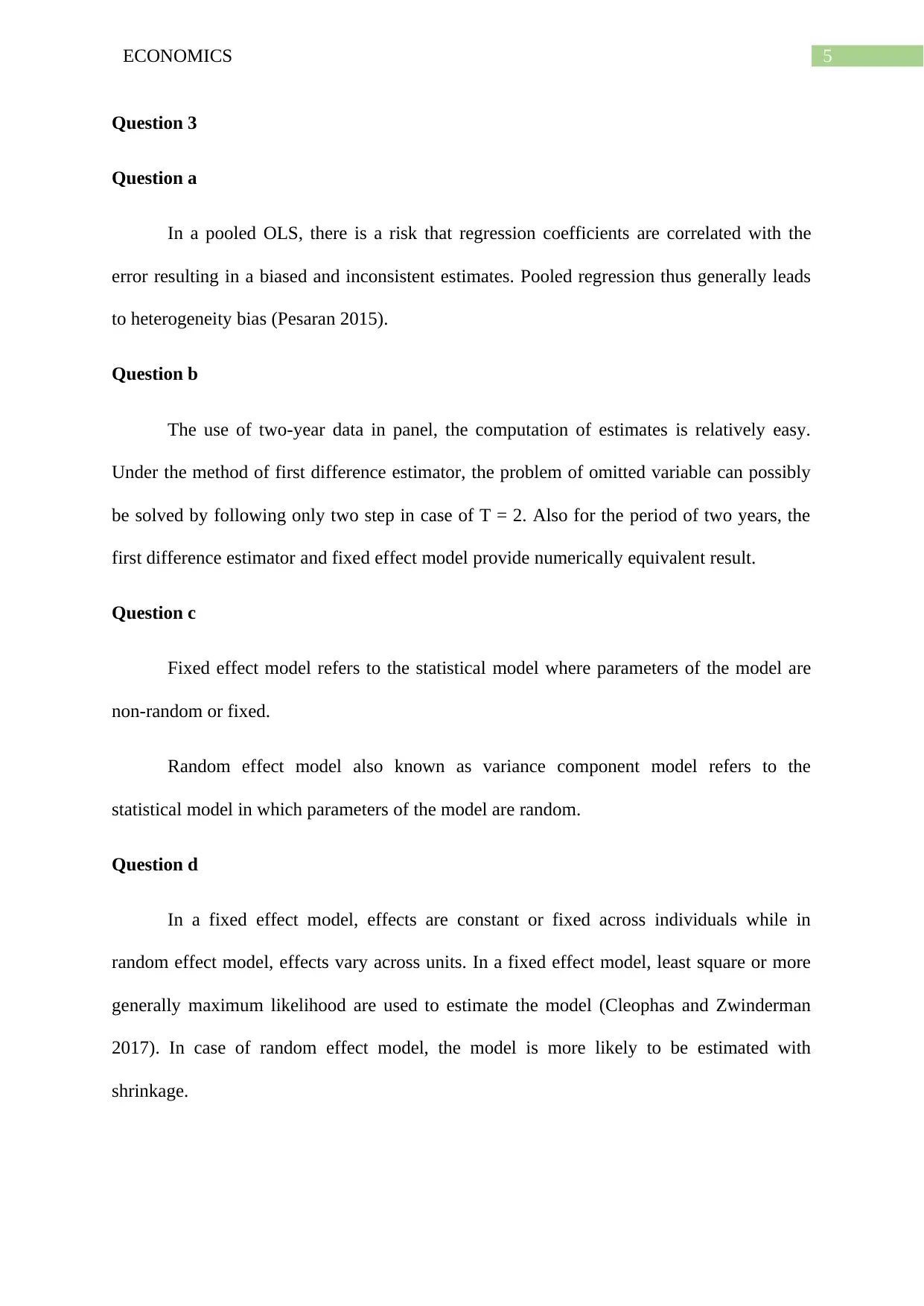
5ECONOMICS
Question 3
Question a
In a pooled OLS, there is a risk that regression coefficients are correlated with the
error resulting in a biased and inconsistent estimates. Pooled regression thus generally leads
to heterogeneity bias (Pesaran 2015).
Question b
The use of two-year data in panel, the computation of estimates is relatively easy.
Under the method of first difference estimator, the problem of omitted variable can possibly
be solved by following only two step in case of T = 2. Also for the period of two years, the
first difference estimator and fixed effect model provide numerically equivalent result.
Question c
Fixed effect model refers to the statistical model where parameters of the model are
non-random or fixed.
Random effect model also known as variance component model refers to the
statistical model in which parameters of the model are random.
Question d
In a fixed effect model, effects are constant or fixed across individuals while in
random effect model, effects vary across units. In a fixed effect model, least square or more
generally maximum likelihood are used to estimate the model (Cleophas and Zwinderman
2017). In case of random effect model, the model is more likely to be estimated with
shrinkage.
Question 3
Question a
In a pooled OLS, there is a risk that regression coefficients are correlated with the
error resulting in a biased and inconsistent estimates. Pooled regression thus generally leads
to heterogeneity bias (Pesaran 2015).
Question b
The use of two-year data in panel, the computation of estimates is relatively easy.
Under the method of first difference estimator, the problem of omitted variable can possibly
be solved by following only two step in case of T = 2. Also for the period of two years, the
first difference estimator and fixed effect model provide numerically equivalent result.
Question c
Fixed effect model refers to the statistical model where parameters of the model are
non-random or fixed.
Random effect model also known as variance component model refers to the
statistical model in which parameters of the model are random.
Question d
In a fixed effect model, effects are constant or fixed across individuals while in
random effect model, effects vary across units. In a fixed effect model, least square or more
generally maximum likelihood are used to estimate the model (Cleophas and Zwinderman
2017). In case of random effect model, the model is more likely to be estimated with
shrinkage.
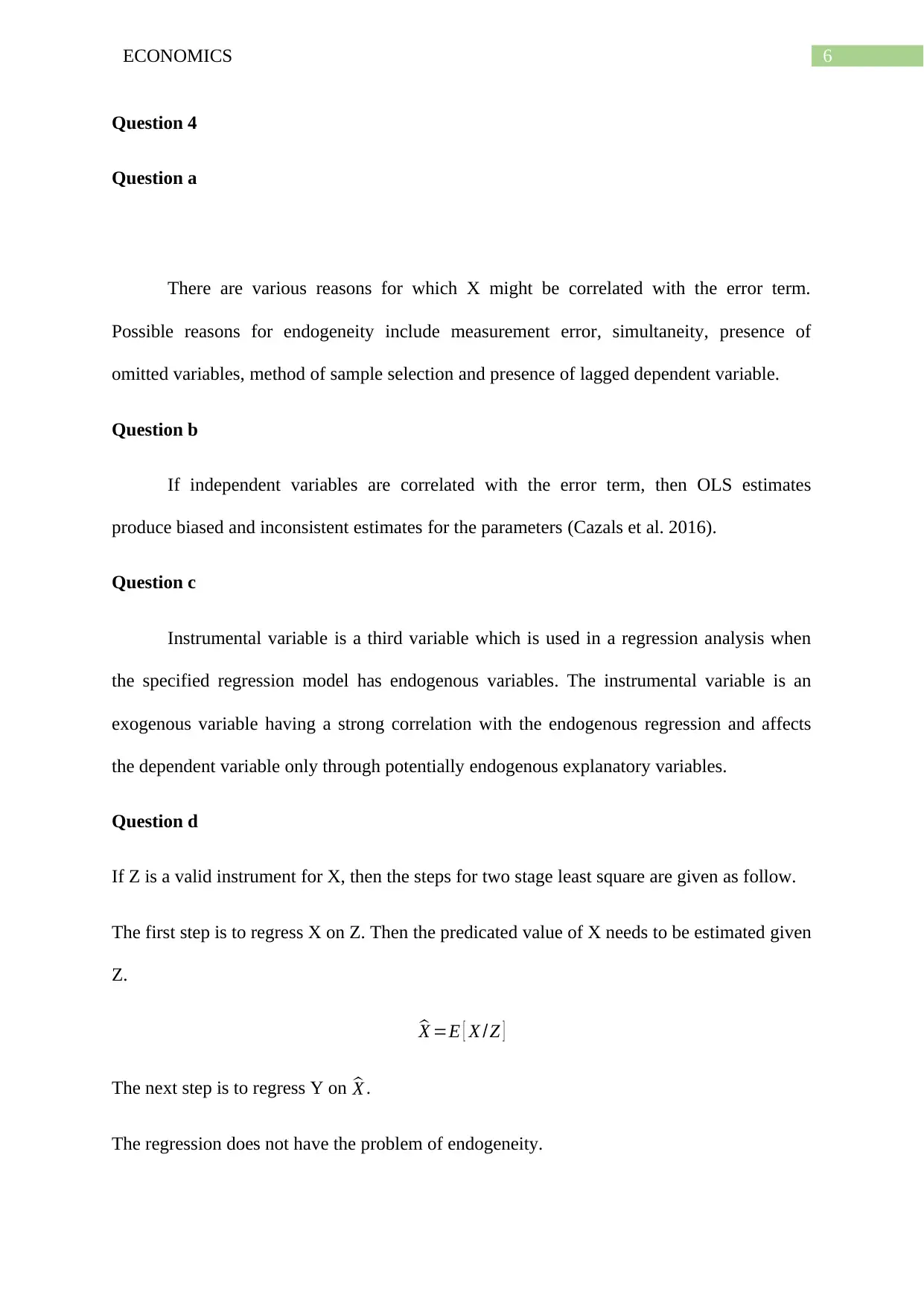
6ECONOMICS
Question 4
Question a
There are various reasons for which X might be correlated with the error term.
Possible reasons for endogeneity include measurement error, simultaneity, presence of
omitted variables, method of sample selection and presence of lagged dependent variable.
Question b
If independent variables are correlated with the error term, then OLS estimates
produce biased and inconsistent estimates for the parameters (Cazals et al. 2016).
Question c
Instrumental variable is a third variable which is used in a regression analysis when
the specified regression model has endogenous variables. The instrumental variable is an
exogenous variable having a strong correlation with the endogenous regression and affects
the dependent variable only through potentially endogenous explanatory variables.
Question d
If Z is a valid instrument for X, then the steps for two stage least square are given as follow.
The first step is to regress X on Z. Then the predicated value of X needs to be estimated given
Z.
^X =E [ X /Z ]
The next step is to regress Y on ^X .
The regression does not have the problem of endogeneity.
Question 4
Question a
There are various reasons for which X might be correlated with the error term.
Possible reasons for endogeneity include measurement error, simultaneity, presence of
omitted variables, method of sample selection and presence of lagged dependent variable.
Question b
If independent variables are correlated with the error term, then OLS estimates
produce biased and inconsistent estimates for the parameters (Cazals et al. 2016).
Question c
Instrumental variable is a third variable which is used in a regression analysis when
the specified regression model has endogenous variables. The instrumental variable is an
exogenous variable having a strong correlation with the endogenous regression and affects
the dependent variable only through potentially endogenous explanatory variables.
Question d
If Z is a valid instrument for X, then the steps for two stage least square are given as follow.
The first step is to regress X on Z. Then the predicated value of X needs to be estimated given
Z.
^X =E [ X /Z ]
The next step is to regress Y on ^X .
The regression does not have the problem of endogeneity.
Paraphrase This Document
Need a fresh take? Get an instant paraphrase of this document with our AI Paraphraser
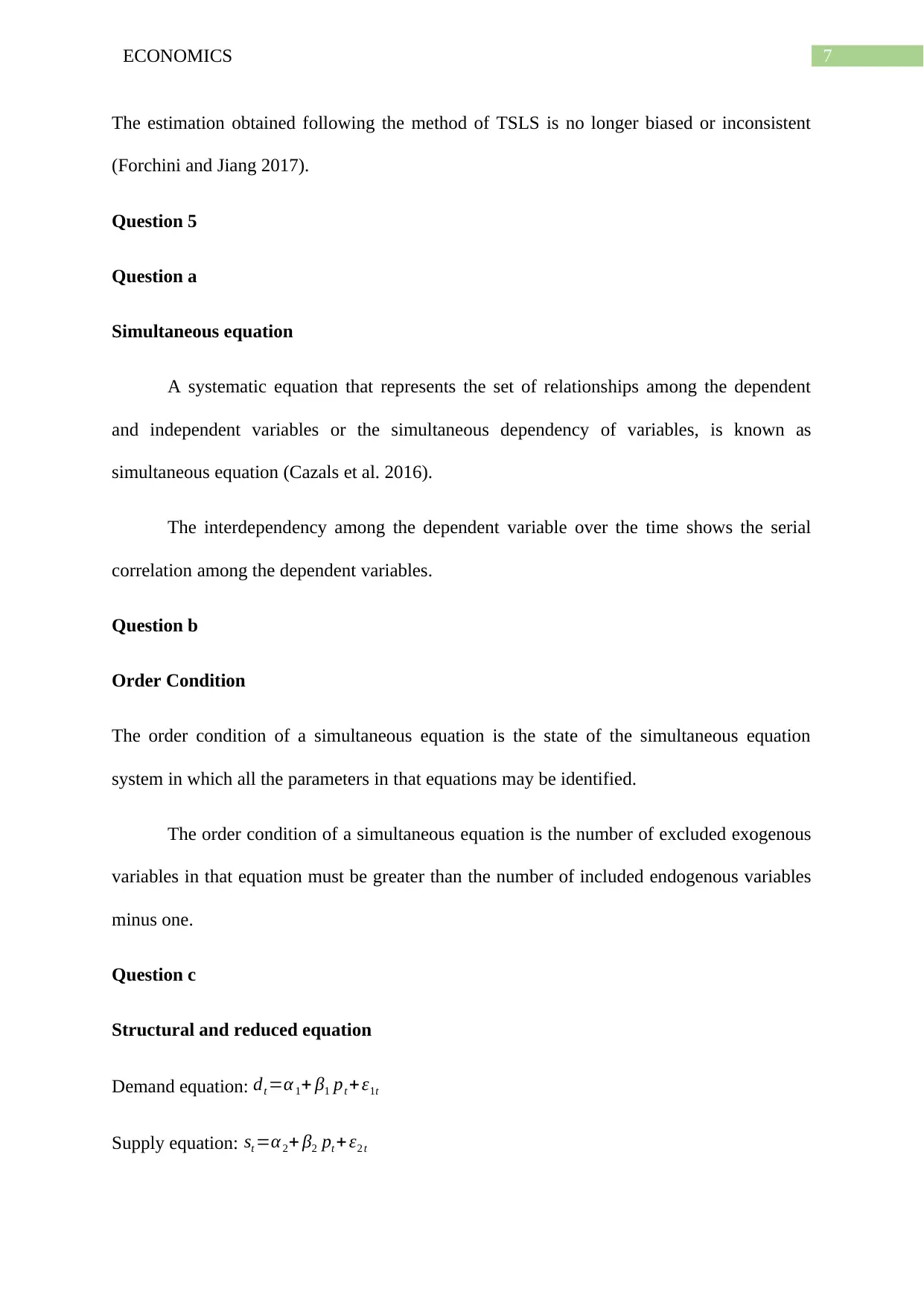
7ECONOMICS
The estimation obtained following the method of TSLS is no longer biased or inconsistent
(Forchini and Jiang 2017).
Question 5
Question a
Simultaneous equation
A systematic equation that represents the set of relationships among the dependent
and independent variables or the simultaneous dependency of variables, is known as
simultaneous equation (Cazals et al. 2016).
The interdependency among the dependent variable over the time shows the serial
correlation among the dependent variables.
Question b
Order Condition
The order condition of a simultaneous equation is the state of the simultaneous equation
system in which all the parameters in that equations may be identified.
The order condition of a simultaneous equation is the number of excluded exogenous
variables in that equation must be greater than the number of included endogenous variables
minus one.
Question c
Structural and reduced equation
Demand equation: dt =α 1+ β1 pt + ε1t
Supply equation: st =α 2+ β2 pt + ε2 t
The estimation obtained following the method of TSLS is no longer biased or inconsistent
(Forchini and Jiang 2017).
Question 5
Question a
Simultaneous equation
A systematic equation that represents the set of relationships among the dependent
and independent variables or the simultaneous dependency of variables, is known as
simultaneous equation (Cazals et al. 2016).
The interdependency among the dependent variable over the time shows the serial
correlation among the dependent variables.
Question b
Order Condition
The order condition of a simultaneous equation is the state of the simultaneous equation
system in which all the parameters in that equations may be identified.
The order condition of a simultaneous equation is the number of excluded exogenous
variables in that equation must be greater than the number of included endogenous variables
minus one.
Question c
Structural and reduced equation
Demand equation: dt =α 1+ β1 pt + ε1t
Supply equation: st =α 2+ β2 pt + ε2 t
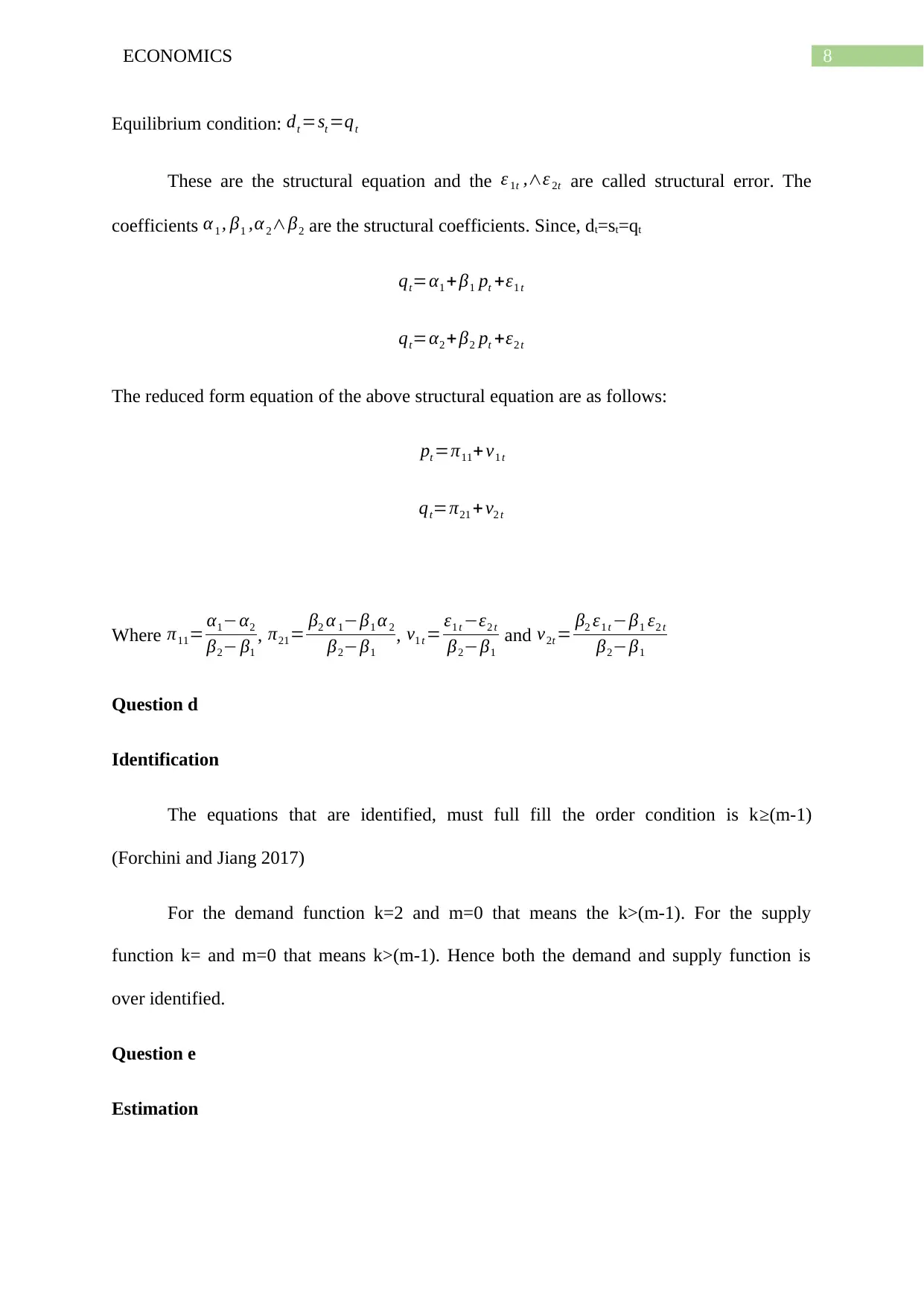
8ECONOMICS
Equilibrium condition: dt =st =qt
These are the structural equation and the ε 1t ,∧ε 2t are called structural error. The
coefficients α 1 , β1 ,α2∧β2 are the structural coefficients. Since, dt=st=qt
qt=α1 + β1 pt +ε1 t
qt=α2 + β2 pt +ε2 t
The reduced form equation of the above structural equation are as follows:
pt =π11+v1 t
qt=π21 + v2 t
Where π11= α1−α2
β2− β1
, π21= β2 α 1−β1 α 2
β2−β1
, v1 t = ε1 t −ε2 t
β2−β1
and v2t = β2 ε1 t −β1 ε2 t
β2−β1
Question d
Identification
The equations that are identified, must full fill the order condition is k≥(m-1)
(Forchini and Jiang 2017)
For the demand function k=2 and m=0 that means the k>(m-1). For the supply
function k= and m=0 that means k>(m-1). Hence both the demand and supply function is
over identified.
Question e
Estimation
Equilibrium condition: dt =st =qt
These are the structural equation and the ε 1t ,∧ε 2t are called structural error. The
coefficients α 1 , β1 ,α2∧β2 are the structural coefficients. Since, dt=st=qt
qt=α1 + β1 pt +ε1 t
qt=α2 + β2 pt +ε2 t
The reduced form equation of the above structural equation are as follows:
pt =π11+v1 t
qt=π21 + v2 t
Where π11= α1−α2
β2− β1
, π21= β2 α 1−β1 α 2
β2−β1
, v1 t = ε1 t −ε2 t
β2−β1
and v2t = β2 ε1 t −β1 ε2 t
β2−β1
Question d
Identification
The equations that are identified, must full fill the order condition is k≥(m-1)
(Forchini and Jiang 2017)
For the demand function k=2 and m=0 that means the k>(m-1). For the supply
function k= and m=0 that means k>(m-1). Hence both the demand and supply function is
over identified.
Question e
Estimation
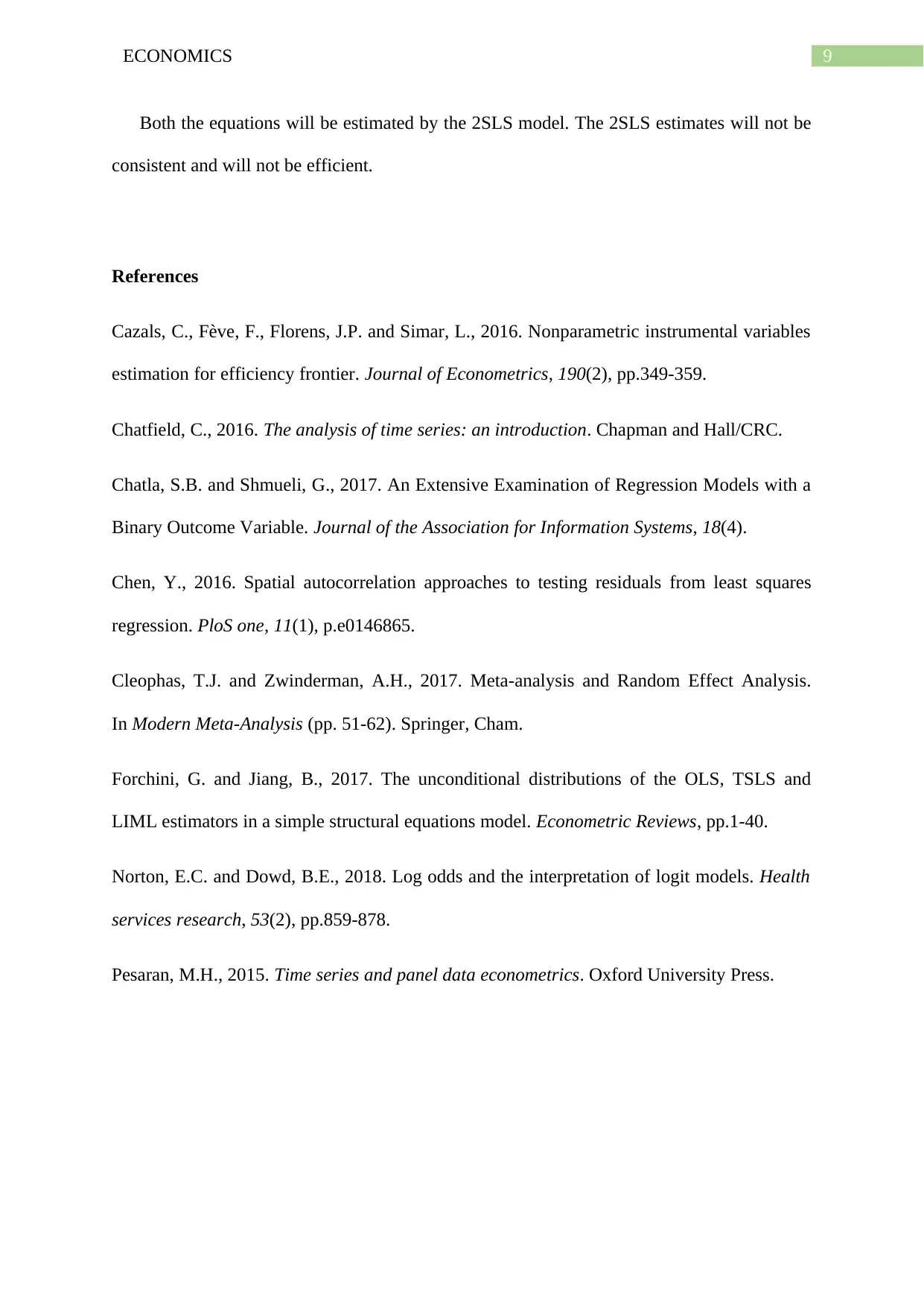
9ECONOMICS
Both the equations will be estimated by the 2SLS model. The 2SLS estimates will not be
consistent and will not be efficient.
References
Cazals, C., Fève, F., Florens, J.P. and Simar, L., 2016. Nonparametric instrumental variables
estimation for efficiency frontier. Journal of Econometrics, 190(2), pp.349-359.
Chatfield, C., 2016. The analysis of time series: an introduction. Chapman and Hall/CRC.
Chatla, S.B. and Shmueli, G., 2017. An Extensive Examination of Regression Models with a
Binary Outcome Variable. Journal of the Association for Information Systems, 18(4).
Chen, Y., 2016. Spatial autocorrelation approaches to testing residuals from least squares
regression. PloS one, 11(1), p.e0146865.
Cleophas, T.J. and Zwinderman, A.H., 2017. Meta-analysis and Random Effect Analysis.
In Modern Meta-Analysis (pp. 51-62). Springer, Cham.
Forchini, G. and Jiang, B., 2017. The unconditional distributions of the OLS, TSLS and
LIML estimators in a simple structural equations model. Econometric Reviews, pp.1-40.
Norton, E.C. and Dowd, B.E., 2018. Log odds and the interpretation of logit models. Health
services research, 53(2), pp.859-878.
Pesaran, M.H., 2015. Time series and panel data econometrics. Oxford University Press.
Both the equations will be estimated by the 2SLS model. The 2SLS estimates will not be
consistent and will not be efficient.
References
Cazals, C., Fève, F., Florens, J.P. and Simar, L., 2016. Nonparametric instrumental variables
estimation for efficiency frontier. Journal of Econometrics, 190(2), pp.349-359.
Chatfield, C., 2016. The analysis of time series: an introduction. Chapman and Hall/CRC.
Chatla, S.B. and Shmueli, G., 2017. An Extensive Examination of Regression Models with a
Binary Outcome Variable. Journal of the Association for Information Systems, 18(4).
Chen, Y., 2016. Spatial autocorrelation approaches to testing residuals from least squares
regression. PloS one, 11(1), p.e0146865.
Cleophas, T.J. and Zwinderman, A.H., 2017. Meta-analysis and Random Effect Analysis.
In Modern Meta-Analysis (pp. 51-62). Springer, Cham.
Forchini, G. and Jiang, B., 2017. The unconditional distributions of the OLS, TSLS and
LIML estimators in a simple structural equations model. Econometric Reviews, pp.1-40.
Norton, E.C. and Dowd, B.E., 2018. Log odds and the interpretation of logit models. Health
services research, 53(2), pp.859-878.
Pesaran, M.H., 2015. Time series and panel data econometrics. Oxford University Press.
Secure Best Marks with AI Grader
Need help grading? Try our AI Grader for instant feedback on your assignments.
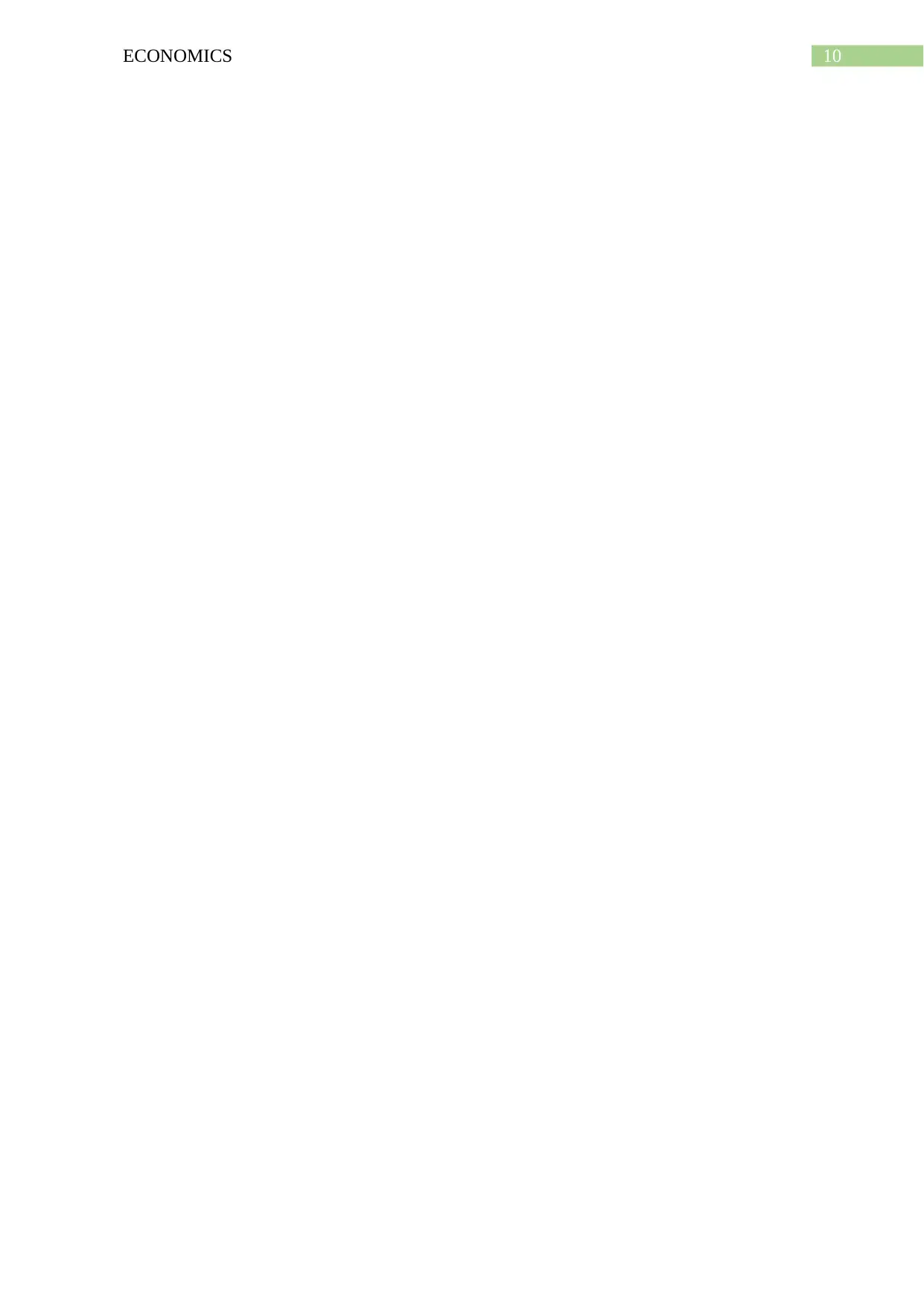
10ECONOMICS
1 out of 11
Related Documents
![[object Object]](/_next/image/?url=%2F_next%2Fstatic%2Fmedia%2Flogo.6d15ce61.png&w=640&q=75)
Your All-in-One AI-Powered Toolkit for Academic Success.
+13062052269
info@desklib.com
Available 24*7 on WhatsApp / Email
Unlock your academic potential
© 2024 | Zucol Services PVT LTD | All rights reserved.