Hypothesis Test on a Sample and the Real Population
VerifiedAdded on 2023/04/20
|12
|1753
|379
AI Summary
This article discusses the process of conducting a hypothesis test on a sample and comparing it to the real population. It includes a literature review, explanation of hypothesis testing, difference in sample and population results, type 1 and type 2 errors, and conclusion and recommendations.
Contribute Materials
Your contribution can guide someone’s learning journey. Share your
documents today.
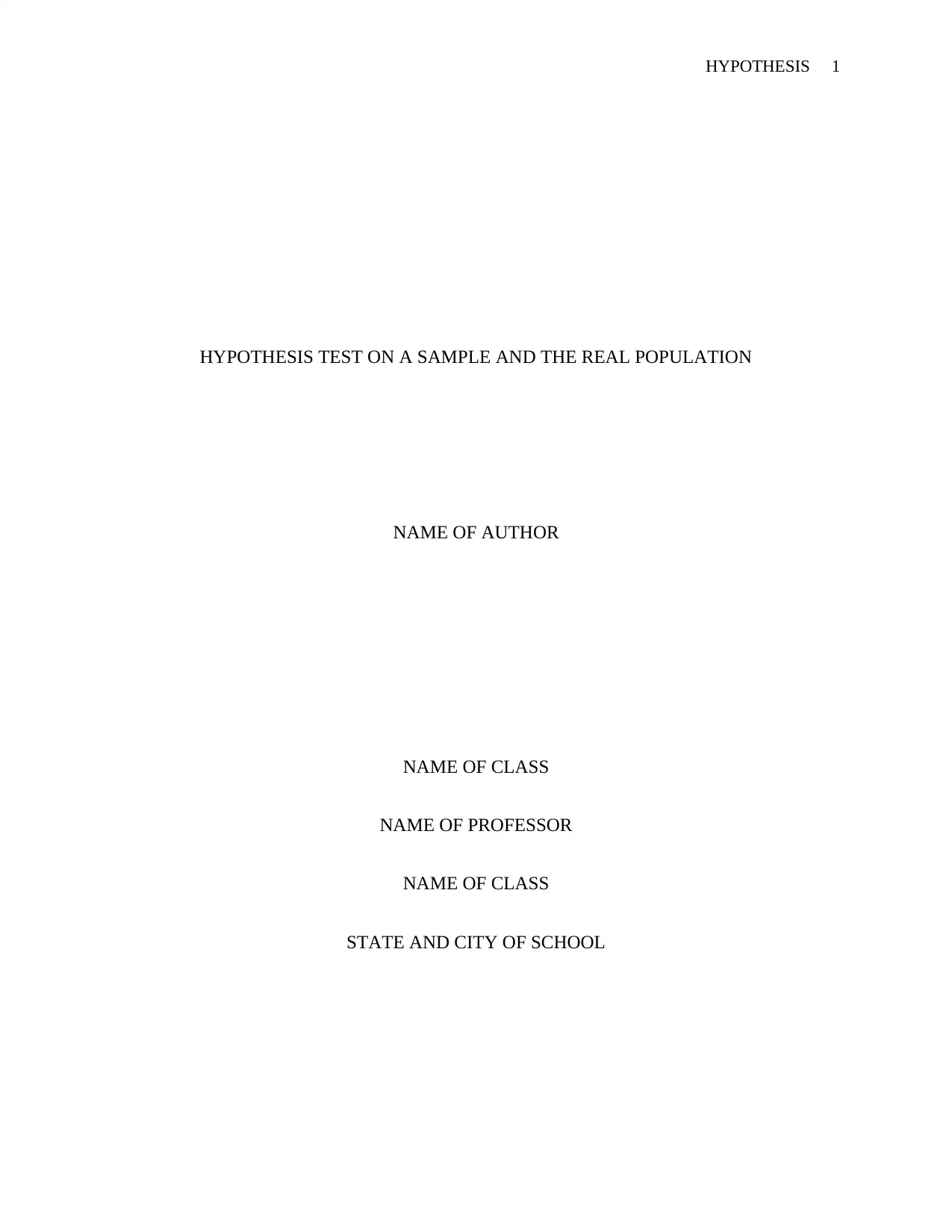
HYPOTHESIS 1
HYPOTHESIS TEST ON A SAMPLE AND THE REAL POPULATION
NAME OF AUTHOR
NAME OF CLASS
NAME OF PROFESSOR
NAME OF CLASS
STATE AND CITY OF SCHOOL
HYPOTHESIS TEST ON A SAMPLE AND THE REAL POPULATION
NAME OF AUTHOR
NAME OF CLASS
NAME OF PROFESSOR
NAME OF CLASS
STATE AND CITY OF SCHOOL
Secure Best Marks with AI Grader
Need help grading? Try our AI Grader for instant feedback on your assignments.
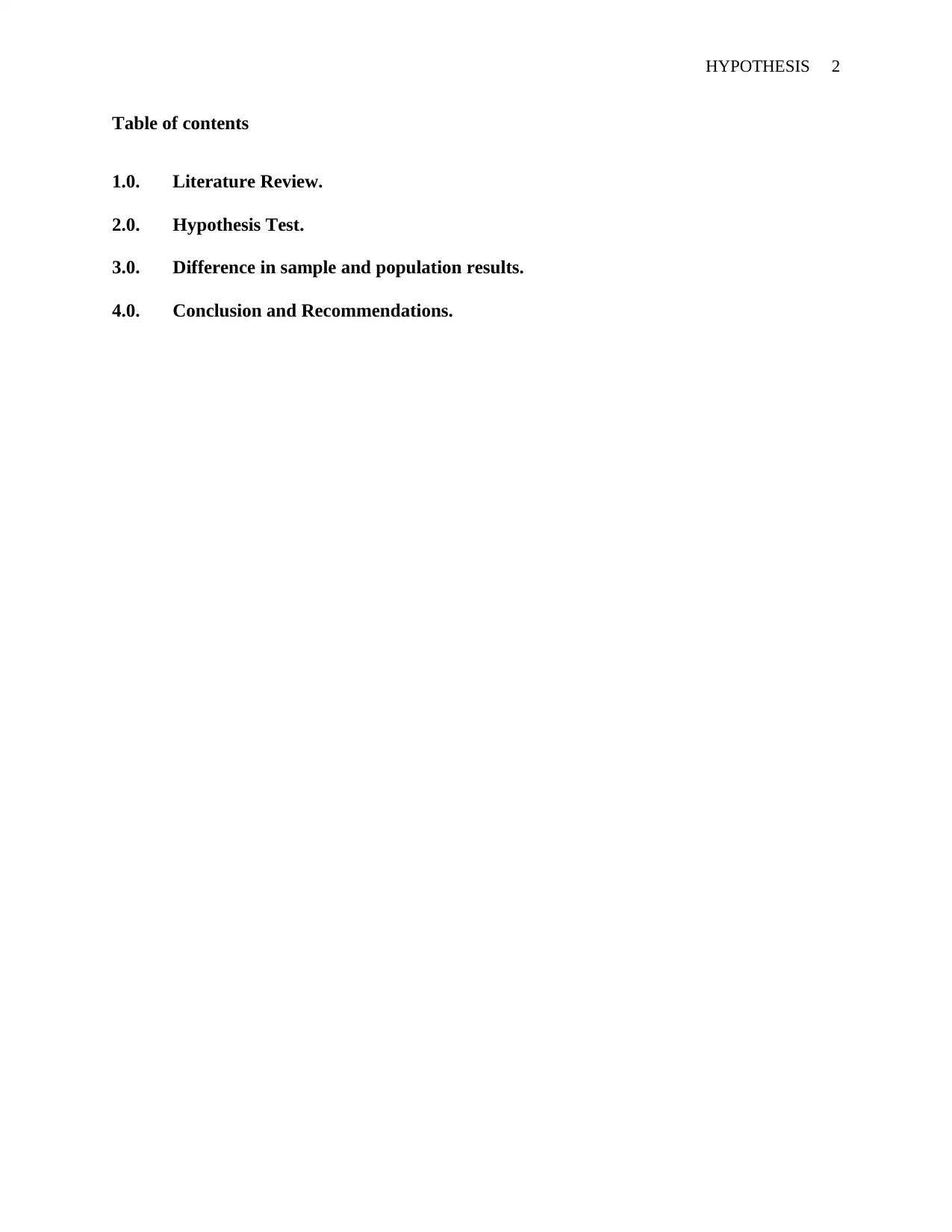
HYPOTHESIS 2
Table of contents
1.0. Literature Review.
2.0. Hypothesis Test.
3.0. Difference in sample and population results.
4.0. Conclusion and Recommendations.
Table of contents
1.0. Literature Review.
2.0. Hypothesis Test.
3.0. Difference in sample and population results.
4.0. Conclusion and Recommendations.
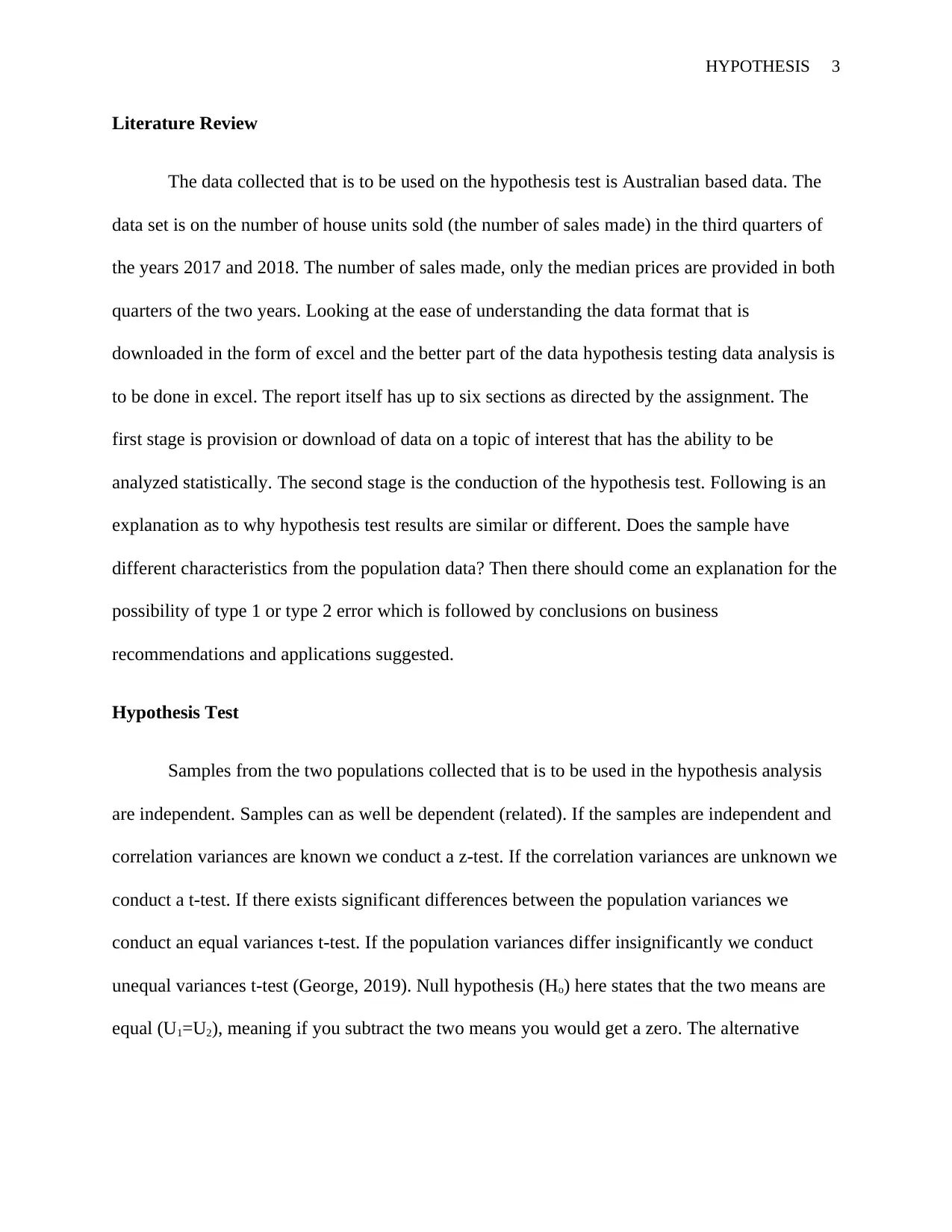
HYPOTHESIS 3
Literature Review
The data collected that is to be used on the hypothesis test is Australian based data. The
data set is on the number of house units sold (the number of sales made) in the third quarters of
the years 2017 and 2018. The number of sales made, only the median prices are provided in both
quarters of the two years. Looking at the ease of understanding the data format that is
downloaded in the form of excel and the better part of the data hypothesis testing data analysis is
to be done in excel. The report itself has up to six sections as directed by the assignment. The
first stage is provision or download of data on a topic of interest that has the ability to be
analyzed statistically. The second stage is the conduction of the hypothesis test. Following is an
explanation as to why hypothesis test results are similar or different. Does the sample have
different characteristics from the population data? Then there should come an explanation for the
possibility of type 1 or type 2 error which is followed by conclusions on business
recommendations and applications suggested.
Hypothesis Test
Samples from the two populations collected that is to be used in the hypothesis analysis
are independent. Samples can as well be dependent (related). If the samples are independent and
correlation variances are known we conduct a z-test. If the correlation variances are unknown we
conduct a t-test. If there exists significant differences between the population variances we
conduct an equal variances t-test. If the population variances differ insignificantly we conduct
unequal variances t-test (George, 2019). Null hypothesis (Ho) here states that the two means are
equal (U1=U2), meaning if you subtract the two means you would get a zero. The alternative
Literature Review
The data collected that is to be used on the hypothesis test is Australian based data. The
data set is on the number of house units sold (the number of sales made) in the third quarters of
the years 2017 and 2018. The number of sales made, only the median prices are provided in both
quarters of the two years. Looking at the ease of understanding the data format that is
downloaded in the form of excel and the better part of the data hypothesis testing data analysis is
to be done in excel. The report itself has up to six sections as directed by the assignment. The
first stage is provision or download of data on a topic of interest that has the ability to be
analyzed statistically. The second stage is the conduction of the hypothesis test. Following is an
explanation as to why hypothesis test results are similar or different. Does the sample have
different characteristics from the population data? Then there should come an explanation for the
possibility of type 1 or type 2 error which is followed by conclusions on business
recommendations and applications suggested.
Hypothesis Test
Samples from the two populations collected that is to be used in the hypothesis analysis
are independent. Samples can as well be dependent (related). If the samples are independent and
correlation variances are known we conduct a z-test. If the correlation variances are unknown we
conduct a t-test. If there exists significant differences between the population variances we
conduct an equal variances t-test. If the population variances differ insignificantly we conduct
unequal variances t-test (George, 2019). Null hypothesis (Ho) here states that the two means are
equal (U1=U2), meaning if you subtract the two means you would get a zero. The alternative
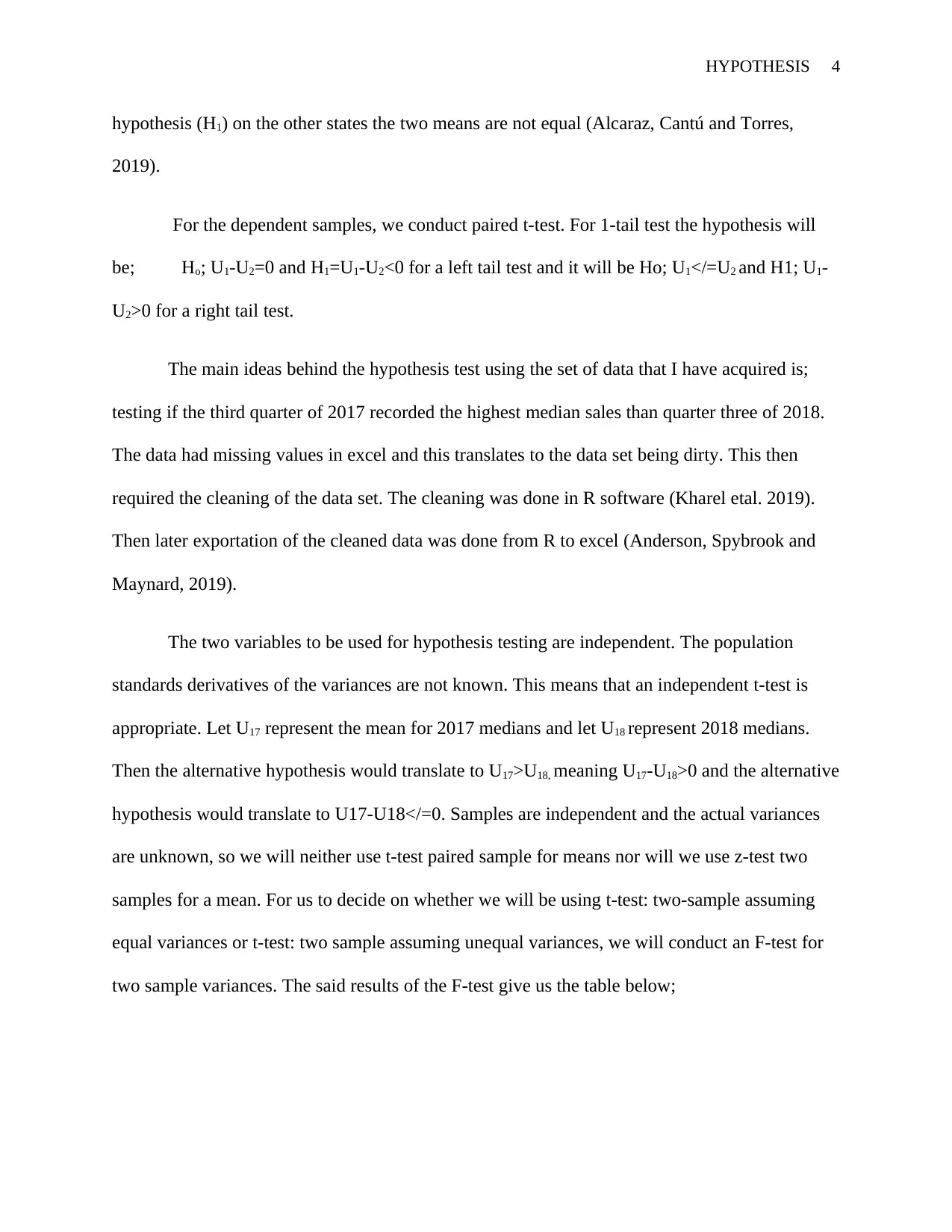
HYPOTHESIS 4
hypothesis (H1) on the other states the two means are not equal (Alcaraz, Cantú and Torres,
2019).
For the dependent samples, we conduct paired t-test. For 1-tail test the hypothesis will
be; Ho; U1-U2=0 and H1=U1-U2<0 for a left tail test and it will be Ho; U1</=U2 and H1; U1-
U2>0 for a right tail test.
The main ideas behind the hypothesis test using the set of data that I have acquired is;
testing if the third quarter of 2017 recorded the highest median sales than quarter three of 2018.
The data had missing values in excel and this translates to the data set being dirty. This then
required the cleaning of the data set. The cleaning was done in R software (Kharel etal. 2019).
Then later exportation of the cleaned data was done from R to excel (Anderson, Spybrook and
Maynard, 2019).
The two variables to be used for hypothesis testing are independent. The population
standards derivatives of the variances are not known. This means that an independent t-test is
appropriate. Let U17 represent the mean for 2017 medians and let U18 represent 2018 medians.
Then the alternative hypothesis would translate to U17>U18, meaning U17-U18>0 and the alternative
hypothesis would translate to U17-U18</=0. Samples are independent and the actual variances
are unknown, so we will neither use t-test paired sample for means nor will we use z-test two
samples for a mean. For us to decide on whether we will be using t-test: two-sample assuming
equal variances or t-test: two sample assuming unequal variances, we will conduct an F-test for
two sample variances. The said results of the F-test give us the table below;
hypothesis (H1) on the other states the two means are not equal (Alcaraz, Cantú and Torres,
2019).
For the dependent samples, we conduct paired t-test. For 1-tail test the hypothesis will
be; Ho; U1-U2=0 and H1=U1-U2<0 for a left tail test and it will be Ho; U1</=U2 and H1; U1-
U2>0 for a right tail test.
The main ideas behind the hypothesis test using the set of data that I have acquired is;
testing if the third quarter of 2017 recorded the highest median sales than quarter three of 2018.
The data had missing values in excel and this translates to the data set being dirty. This then
required the cleaning of the data set. The cleaning was done in R software (Kharel etal. 2019).
Then later exportation of the cleaned data was done from R to excel (Anderson, Spybrook and
Maynard, 2019).
The two variables to be used for hypothesis testing are independent. The population
standards derivatives of the variances are not known. This means that an independent t-test is
appropriate. Let U17 represent the mean for 2017 medians and let U18 represent 2018 medians.
Then the alternative hypothesis would translate to U17>U18, meaning U17-U18>0 and the alternative
hypothesis would translate to U17-U18</=0. Samples are independent and the actual variances
are unknown, so we will neither use t-test paired sample for means nor will we use z-test two
samples for a mean. For us to decide on whether we will be using t-test: two-sample assuming
equal variances or t-test: two sample assuming unequal variances, we will conduct an F-test for
two sample variances. The said results of the F-test give us the table below;
Paraphrase This Document
Need a fresh take? Get an instant paraphrase of this document with our AI Paraphraser
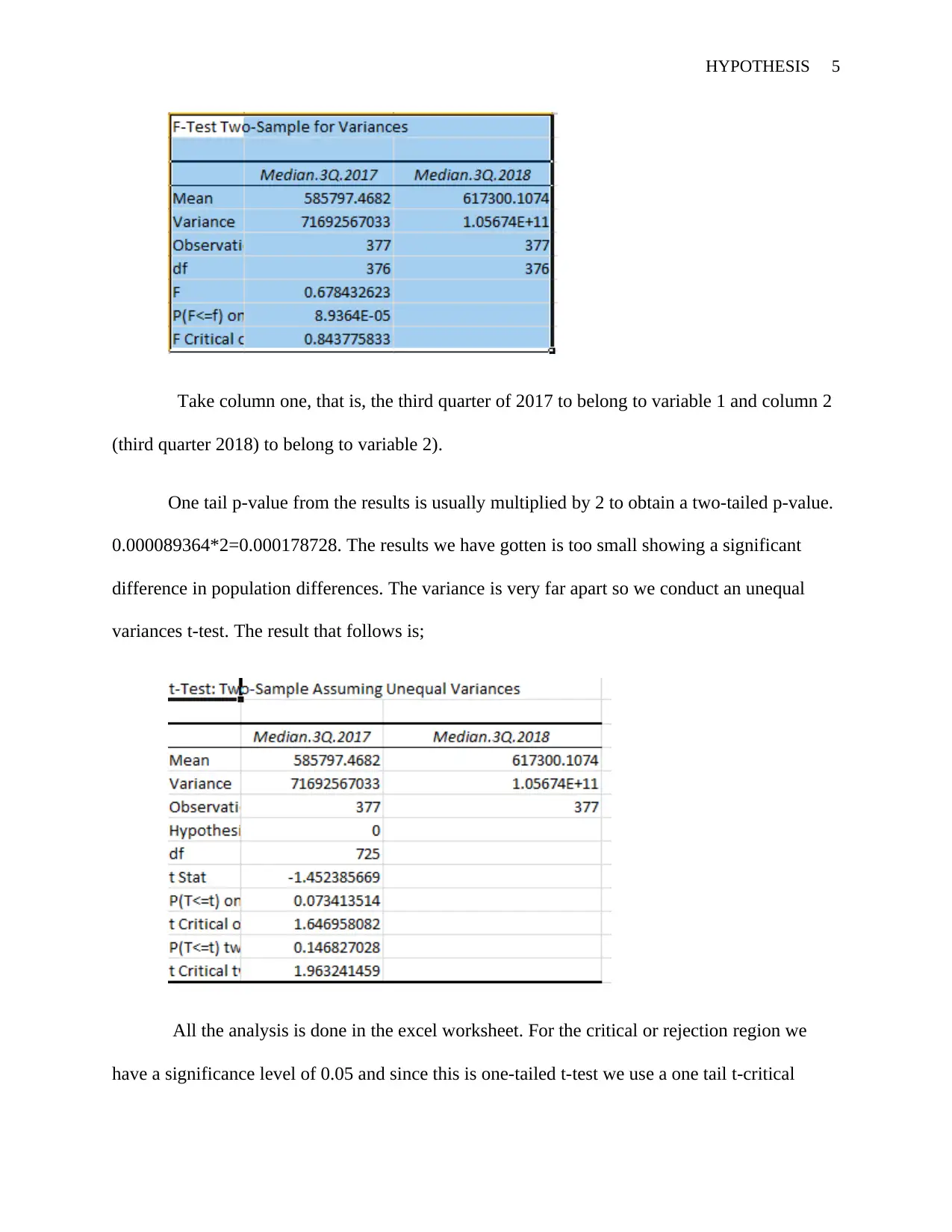
HYPOTHESIS 5
Take column one, that is, the third quarter of 2017 to belong to variable 1 and column 2
(third quarter 2018) to belong to variable 2).
One tail p-value from the results is usually multiplied by 2 to obtain a two-tailed p-value.
0.000089364*2=0.000178728. The results we have gotten is too small showing a significant
difference in population differences. The variance is very far apart so we conduct an unequal
variances t-test. The result that follows is;
All the analysis is done in the excel worksheet. For the critical or rejection region we
have a significance level of 0.05 and since this is one-tailed t-test we use a one tail t-critical
Take column one, that is, the third quarter of 2017 to belong to variable 1 and column 2
(third quarter 2018) to belong to variable 2).
One tail p-value from the results is usually multiplied by 2 to obtain a two-tailed p-value.
0.000089364*2=0.000178728. The results we have gotten is too small showing a significant
difference in population differences. The variance is very far apart so we conduct an unequal
variances t-test. The result that follows is;
All the analysis is done in the excel worksheet. For the critical or rejection region we
have a significance level of 0.05 and since this is one-tailed t-test we use a one tail t-critical
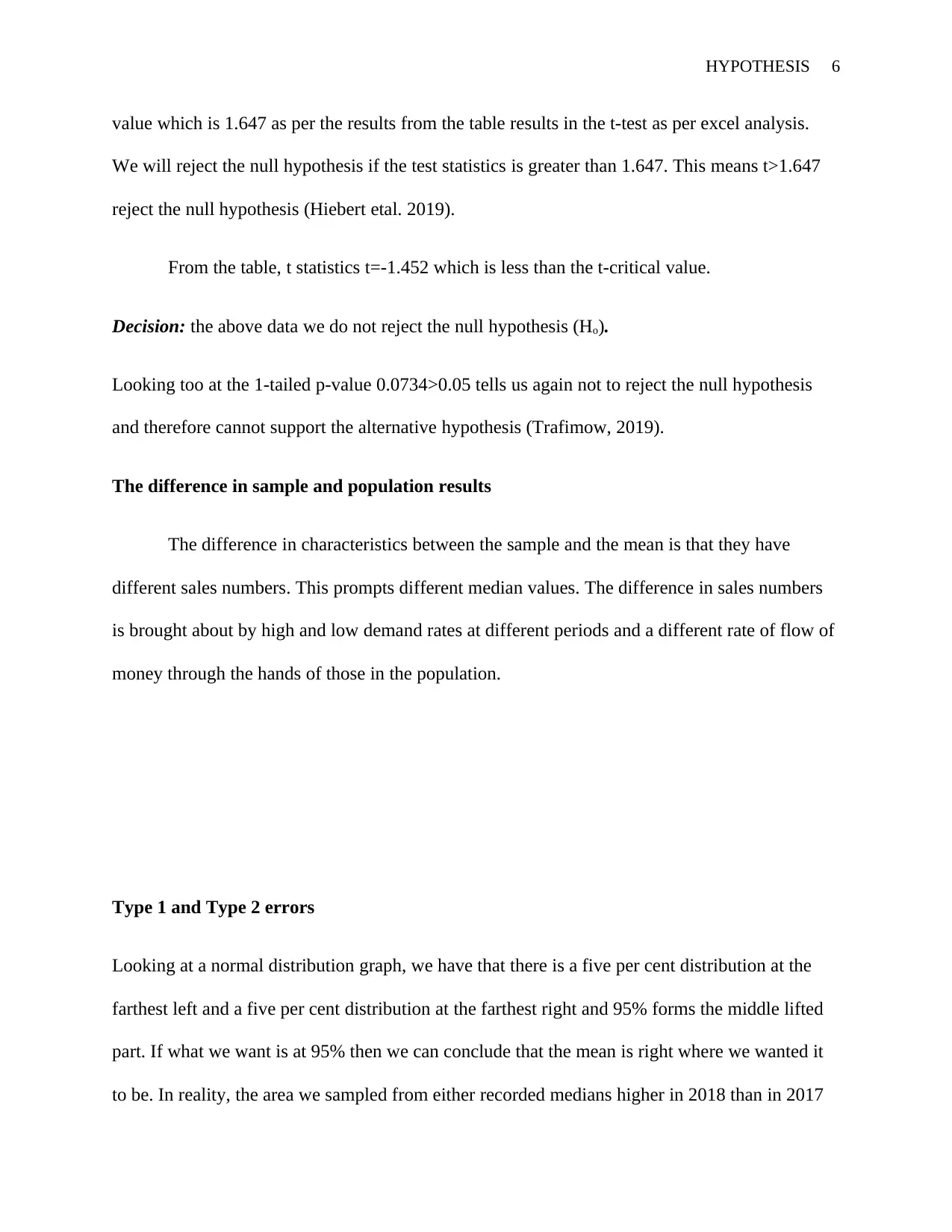
HYPOTHESIS 6
value which is 1.647 as per the results from the table results in the t-test as per excel analysis.
We will reject the null hypothesis if the test statistics is greater than 1.647. This means t>1.647
reject the null hypothesis (Hiebert etal. 2019).
From the table, t statistics t=-1.452 which is less than the t-critical value.
Decision: the above data we do not reject the null hypothesis (Ho).
Looking too at the 1-tailed p-value 0.0734>0.05 tells us again not to reject the null hypothesis
and therefore cannot support the alternative hypothesis (Trafimow, 2019).
The difference in sample and population results
The difference in characteristics between the sample and the mean is that they have
different sales numbers. This prompts different median values. The difference in sales numbers
is brought about by high and low demand rates at different periods and a different rate of flow of
money through the hands of those in the population.
Type 1 and Type 2 errors
Looking at a normal distribution graph, we have that there is a five per cent distribution at the
farthest left and a five per cent distribution at the farthest right and 95% forms the middle lifted
part. If what we want is at 95% then we can conclude that the mean is right where we wanted it
to be. In reality, the area we sampled from either recorded medians higher in 2018 than in 2017
value which is 1.647 as per the results from the table results in the t-test as per excel analysis.
We will reject the null hypothesis if the test statistics is greater than 1.647. This means t>1.647
reject the null hypothesis (Hiebert etal. 2019).
From the table, t statistics t=-1.452 which is less than the t-critical value.
Decision: the above data we do not reject the null hypothesis (Ho).
Looking too at the 1-tailed p-value 0.0734>0.05 tells us again not to reject the null hypothesis
and therefore cannot support the alternative hypothesis (Trafimow, 2019).
The difference in sample and population results
The difference in characteristics between the sample and the mean is that they have
different sales numbers. This prompts different median values. The difference in sales numbers
is brought about by high and low demand rates at different periods and a different rate of flow of
money through the hands of those in the population.
Type 1 and Type 2 errors
Looking at a normal distribution graph, we have that there is a five per cent distribution at the
farthest left and a five per cent distribution at the farthest right and 95% forms the middle lifted
part. If what we want is at 95% then we can conclude that the mean is right where we wanted it
to be. In reality, the area we sampled from either recorded medians higher in 2018 than in 2017
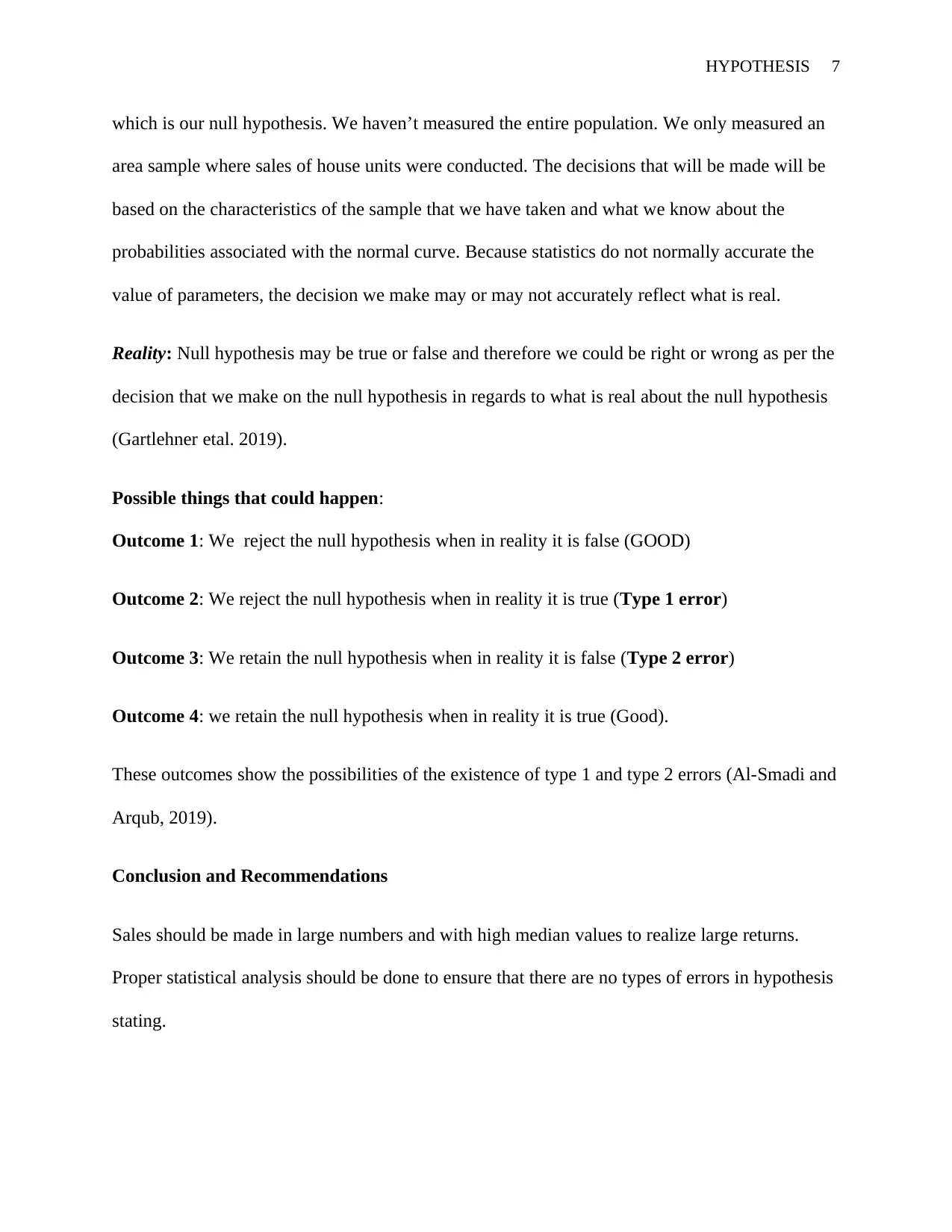
HYPOTHESIS 7
which is our null hypothesis. We haven’t measured the entire population. We only measured an
area sample where sales of house units were conducted. The decisions that will be made will be
based on the characteristics of the sample that we have taken and what we know about the
probabilities associated with the normal curve. Because statistics do not normally accurate the
value of parameters, the decision we make may or may not accurately reflect what is real.
Reality: Null hypothesis may be true or false and therefore we could be right or wrong as per the
decision that we make on the null hypothesis in regards to what is real about the null hypothesis
(Gartlehner etal. 2019).
Possible things that could happen:
Outcome 1: We reject the null hypothesis when in reality it is false (GOOD)
Outcome 2: We reject the null hypothesis when in reality it is true (Type 1 error)
Outcome 3: We retain the null hypothesis when in reality it is false (Type 2 error)
Outcome 4: we retain the null hypothesis when in reality it is true (Good).
These outcomes show the possibilities of the existence of type 1 and type 2 errors (Al-Smadi and
Arqub, 2019).
Conclusion and Recommendations
Sales should be made in large numbers and with high median values to realize large returns.
Proper statistical analysis should be done to ensure that there are no types of errors in hypothesis
stating.
which is our null hypothesis. We haven’t measured the entire population. We only measured an
area sample where sales of house units were conducted. The decisions that will be made will be
based on the characteristics of the sample that we have taken and what we know about the
probabilities associated with the normal curve. Because statistics do not normally accurate the
value of parameters, the decision we make may or may not accurately reflect what is real.
Reality: Null hypothesis may be true or false and therefore we could be right or wrong as per the
decision that we make on the null hypothesis in regards to what is real about the null hypothesis
(Gartlehner etal. 2019).
Possible things that could happen:
Outcome 1: We reject the null hypothesis when in reality it is false (GOOD)
Outcome 2: We reject the null hypothesis when in reality it is true (Type 1 error)
Outcome 3: We retain the null hypothesis when in reality it is false (Type 2 error)
Outcome 4: we retain the null hypothesis when in reality it is true (Good).
These outcomes show the possibilities of the existence of type 1 and type 2 errors (Al-Smadi and
Arqub, 2019).
Conclusion and Recommendations
Sales should be made in large numbers and with high median values to realize large returns.
Proper statistical analysis should be done to ensure that there are no types of errors in hypothesis
stating.
Secure Best Marks with AI Grader
Need help grading? Try our AI Grader for instant feedback on your assignments.
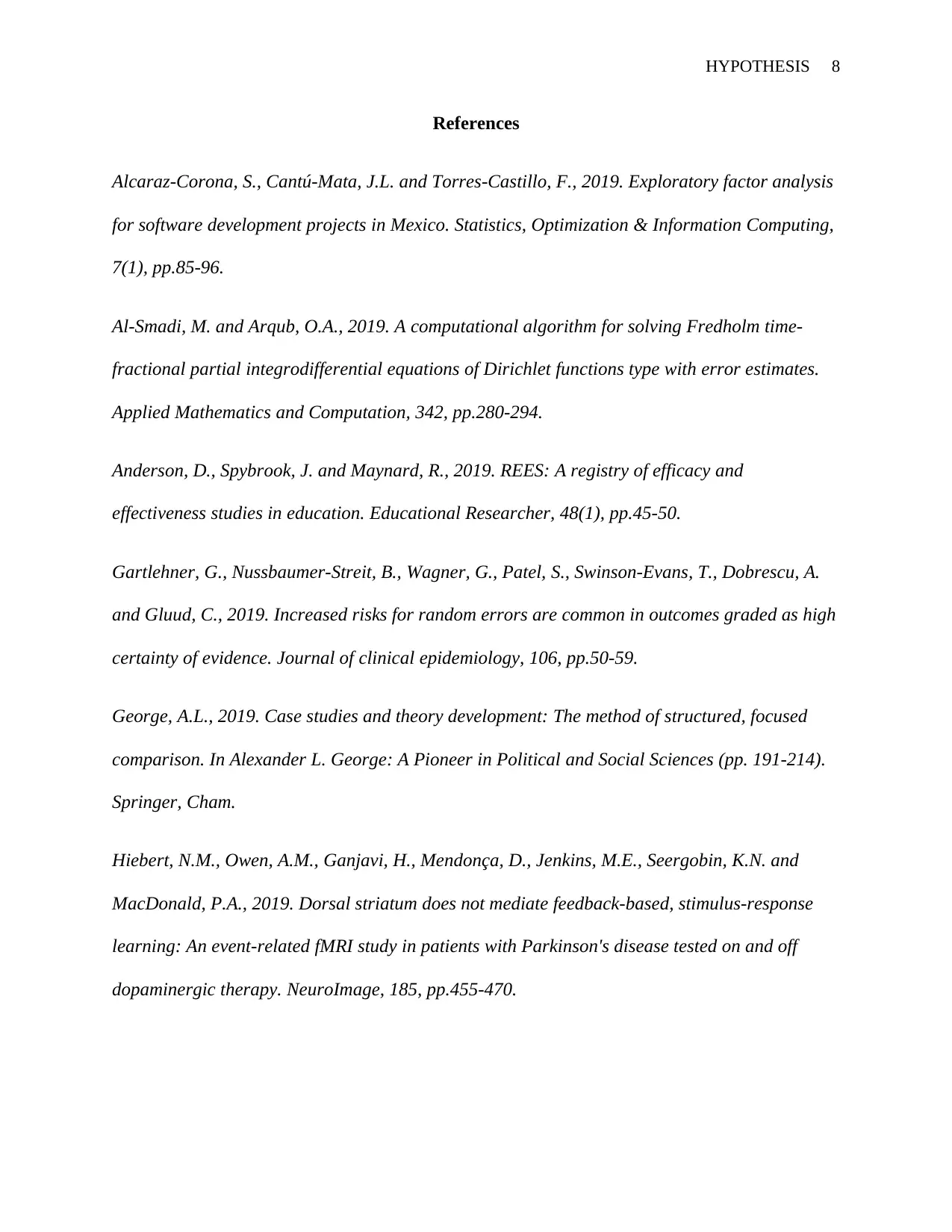
HYPOTHESIS 8
References
Alcaraz-Corona, S., Cantú-Mata, J.L. and Torres-Castillo, F., 2019. Exploratory factor analysis
for software development projects in Mexico. Statistics, Optimization & Information Computing,
7(1), pp.85-96.
Al-Smadi, M. and Arqub, O.A., 2019. A computational algorithm for solving Fredholm time-
fractional partial integrodifferential equations of Dirichlet functions type with error estimates.
Applied Mathematics and Computation, 342, pp.280-294.
Anderson, D., Spybrook, J. and Maynard, R., 2019. REES: A registry of efficacy and
effectiveness studies in education. Educational Researcher, 48(1), pp.45-50.
Gartlehner, G., Nussbaumer-Streit, B., Wagner, G., Patel, S., Swinson-Evans, T., Dobrescu, A.
and Gluud, C., 2019. Increased risks for random errors are common in outcomes graded as high
certainty of evidence. Journal of clinical epidemiology, 106, pp.50-59.
George, A.L., 2019. Case studies and theory development: The method of structured, focused
comparison. In Alexander L. George: A Pioneer in Political and Social Sciences (pp. 191-214).
Springer, Cham.
Hiebert, N.M., Owen, A.M., Ganjavi, H., Mendonça, D., Jenkins, M.E., Seergobin, K.N. and
MacDonald, P.A., 2019. Dorsal striatum does not mediate feedback-based, stimulus-response
learning: An event-related fMRI study in patients with Parkinson's disease tested on and off
dopaminergic therapy. NeuroImage, 185, pp.455-470.
References
Alcaraz-Corona, S., Cantú-Mata, J.L. and Torres-Castillo, F., 2019. Exploratory factor analysis
for software development projects in Mexico. Statistics, Optimization & Information Computing,
7(1), pp.85-96.
Al-Smadi, M. and Arqub, O.A., 2019. A computational algorithm for solving Fredholm time-
fractional partial integrodifferential equations of Dirichlet functions type with error estimates.
Applied Mathematics and Computation, 342, pp.280-294.
Anderson, D., Spybrook, J. and Maynard, R., 2019. REES: A registry of efficacy and
effectiveness studies in education. Educational Researcher, 48(1), pp.45-50.
Gartlehner, G., Nussbaumer-Streit, B., Wagner, G., Patel, S., Swinson-Evans, T., Dobrescu, A.
and Gluud, C., 2019. Increased risks for random errors are common in outcomes graded as high
certainty of evidence. Journal of clinical epidemiology, 106, pp.50-59.
George, A.L., 2019. Case studies and theory development: The method of structured, focused
comparison. In Alexander L. George: A Pioneer in Political and Social Sciences (pp. 191-214).
Springer, Cham.
Hiebert, N.M., Owen, A.M., Ganjavi, H., Mendonça, D., Jenkins, M.E., Seergobin, K.N. and
MacDonald, P.A., 2019. Dorsal striatum does not mediate feedback-based, stimulus-response
learning: An event-related fMRI study in patients with Parkinson's disease tested on and off
dopaminergic therapy. NeuroImage, 185, pp.455-470.
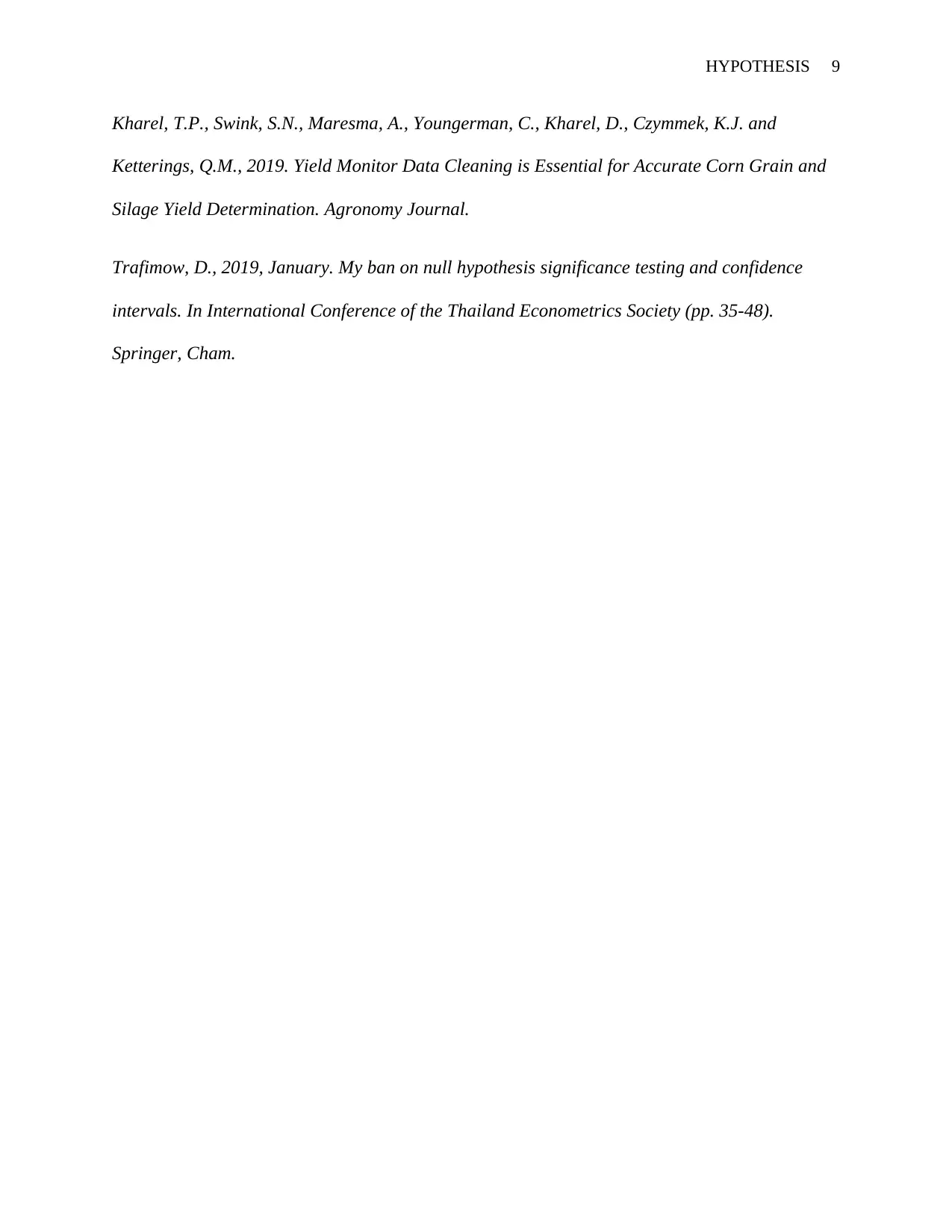
HYPOTHESIS 9
Kharel, T.P., Swink, S.N., Maresma, A., Youngerman, C., Kharel, D., Czymmek, K.J. and
Ketterings, Q.M., 2019. Yield Monitor Data Cleaning is Essential for Accurate Corn Grain and
Silage Yield Determination. Agronomy Journal.
Trafimow, D., 2019, January. My ban on null hypothesis significance testing and confidence
intervals. In International Conference of the Thailand Econometrics Society (pp. 35-48).
Springer, Cham.
Kharel, T.P., Swink, S.N., Maresma, A., Youngerman, C., Kharel, D., Czymmek, K.J. and
Ketterings, Q.M., 2019. Yield Monitor Data Cleaning is Essential for Accurate Corn Grain and
Silage Yield Determination. Agronomy Journal.
Trafimow, D., 2019, January. My ban on null hypothesis significance testing and confidence
intervals. In International Conference of the Thailand Econometrics Society (pp. 35-48).
Springer, Cham.
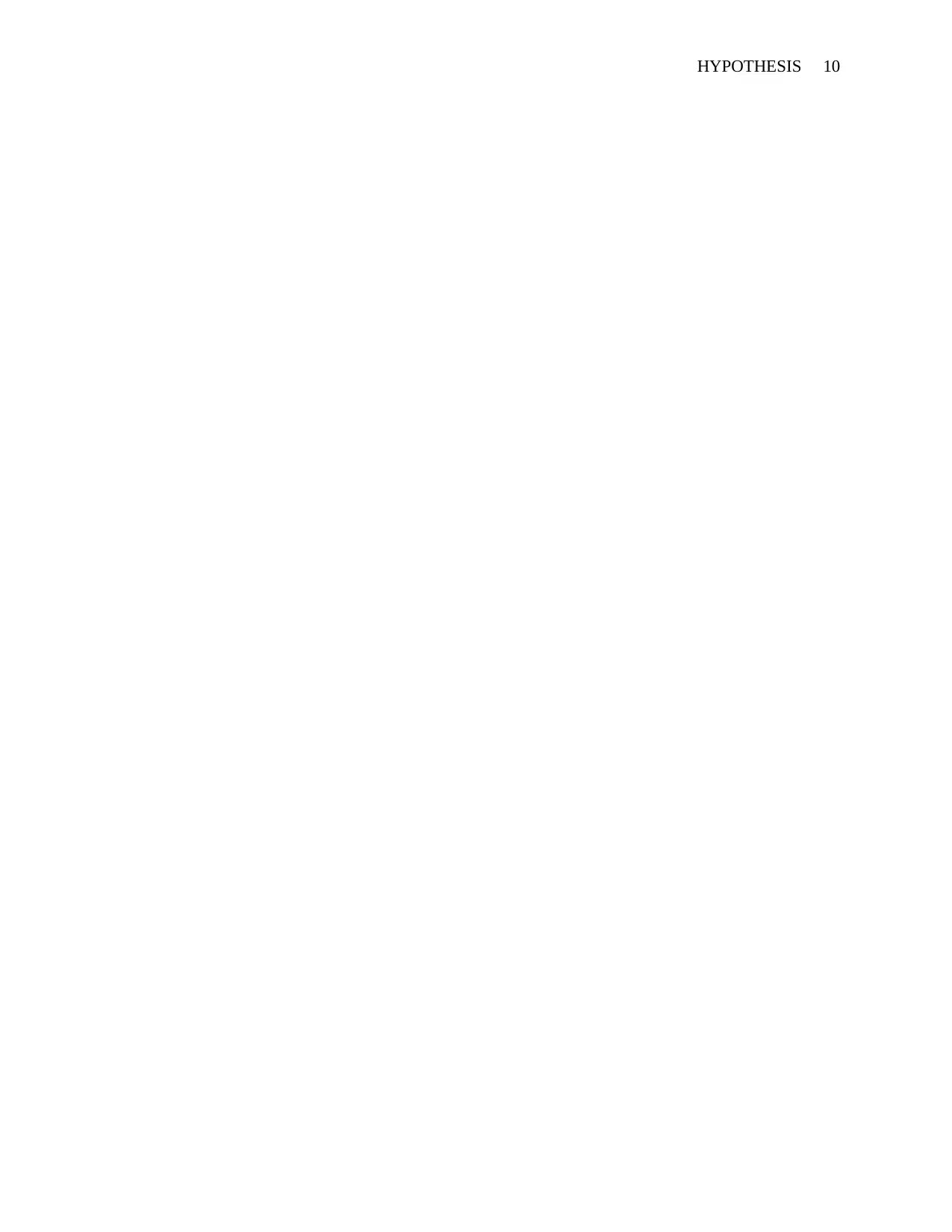
HYPOTHESIS 10
Paraphrase This Document
Need a fresh take? Get an instant paraphrase of this document with our AI Paraphraser
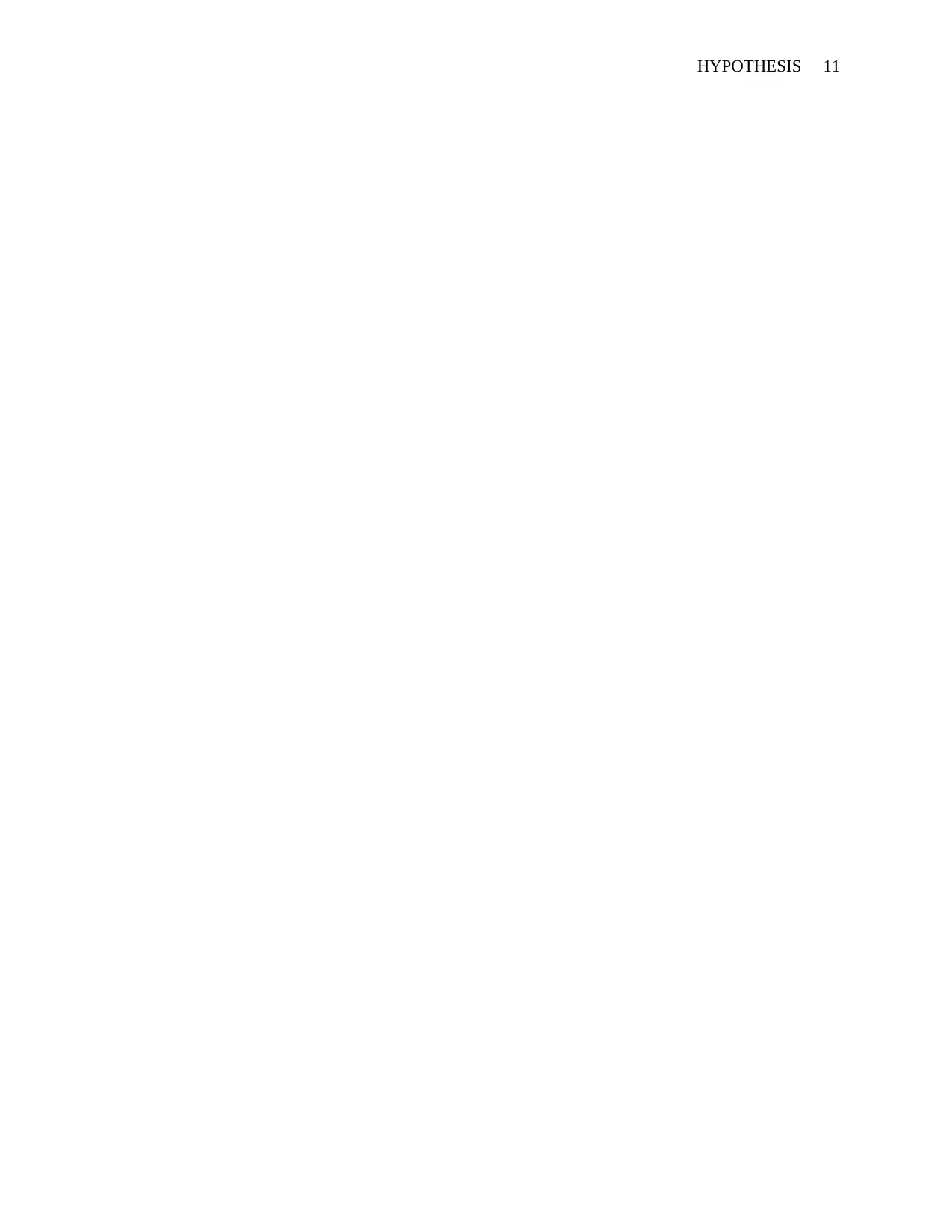
HYPOTHESIS 11
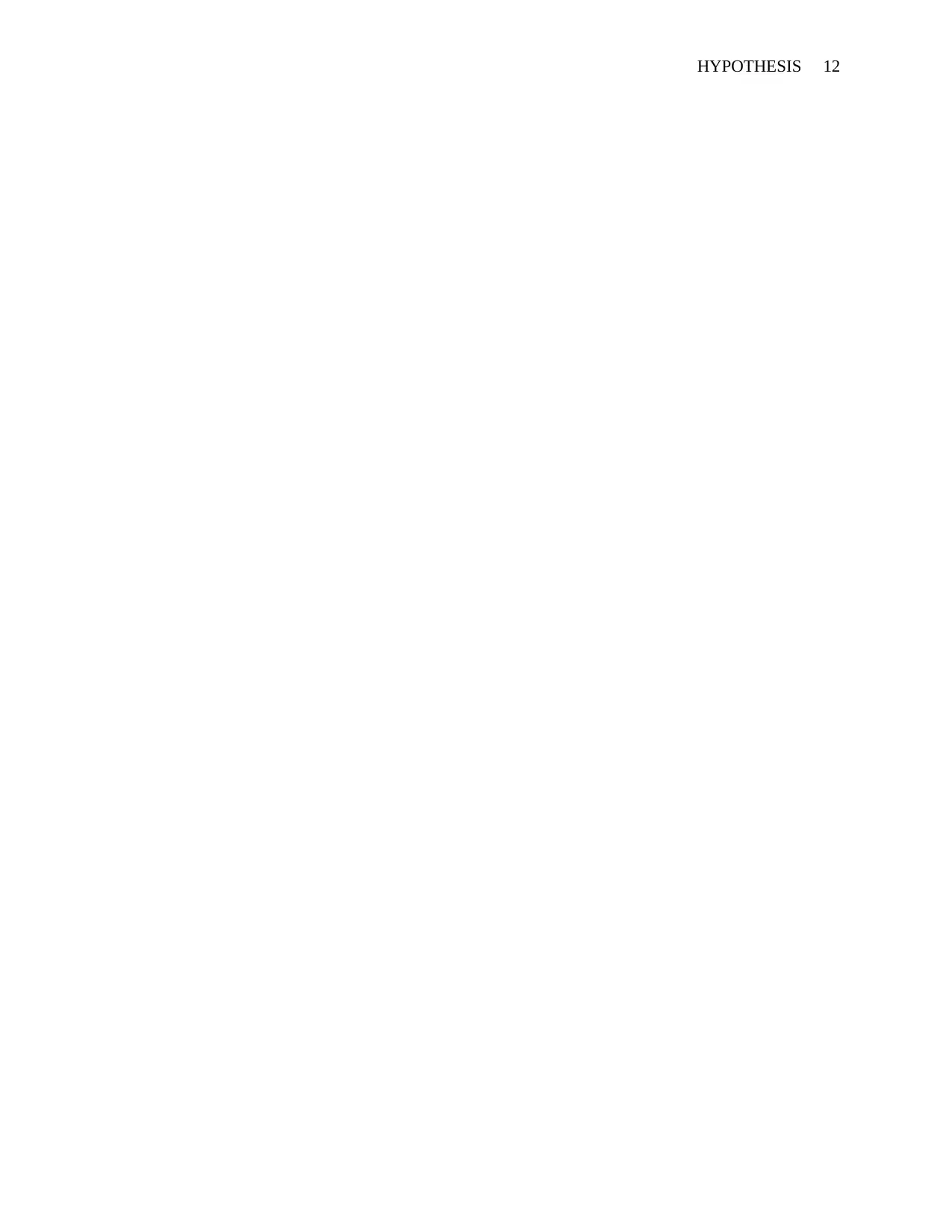
HYPOTHESIS 12
1 out of 12
Related Documents
![[object Object]](/_next/image/?url=%2F_next%2Fstatic%2Fmedia%2Flogo.6d15ce61.png&w=640&q=75)
Your All-in-One AI-Powered Toolkit for Academic Success.
+13062052269
info@desklib.com
Available 24*7 on WhatsApp / Email
Unlock your academic potential
© 2024 | Zucol Services PVT LTD | All rights reserved.