Statistics Assignment Solutions | Desklib
VerifiedAdded on 2023/06/04
|8
|1150
|326
AI Summary
This document contains solutions to statistics assignments covering topics such as frequency descriptions, ANOVA tables, regression equations and more. The solutions are provided by experts and are suitable for students in higher education. The document also includes references for further reading.
Contribute Materials
Your contribution can guide someone’s learning journey. Share your
documents today.
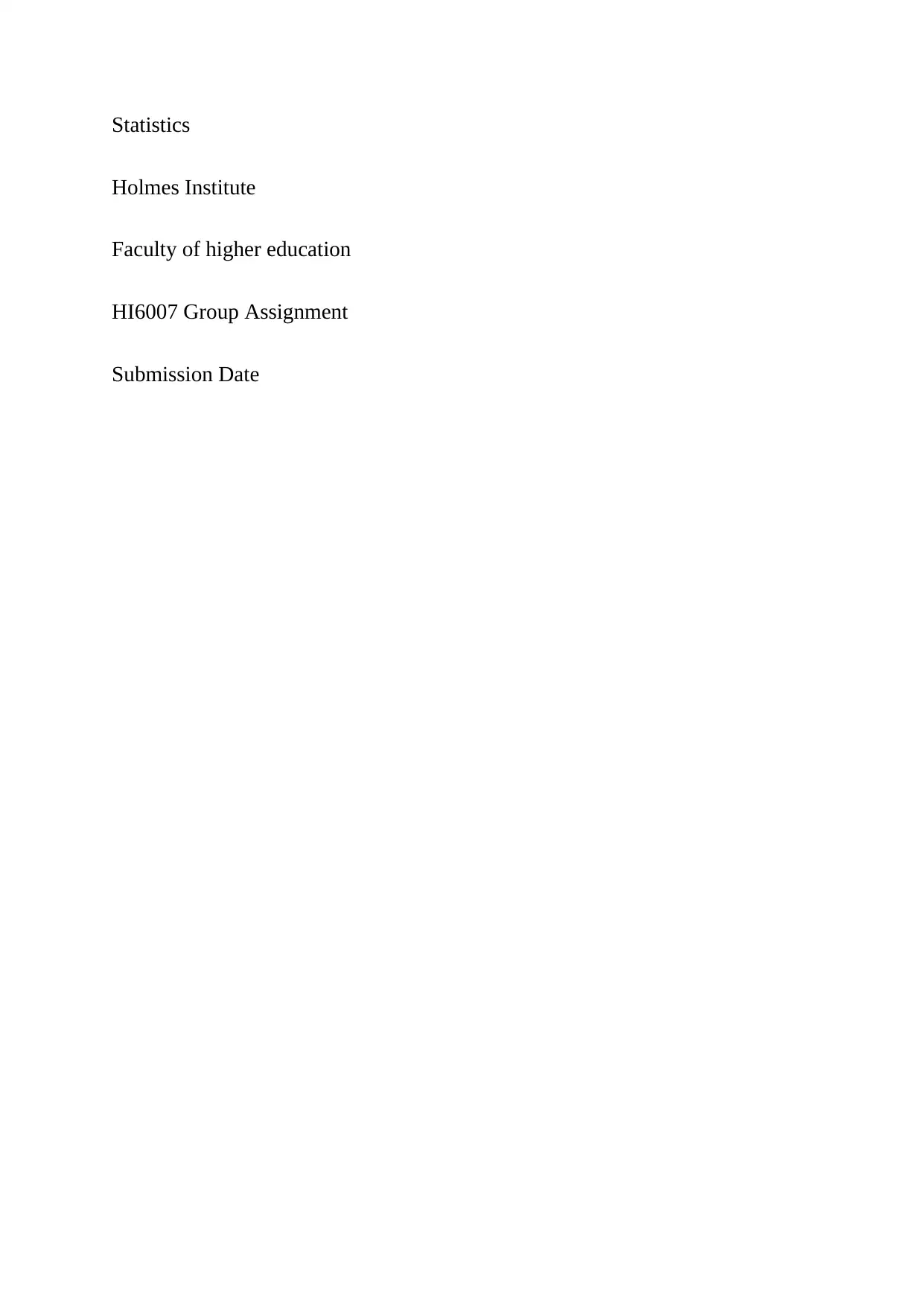
Statistics
Holmes Institute
Faculty of higher education
HI6007 Group Assignment
Submission Date
Holmes Institute
Faculty of higher education
HI6007 Group Assignment
Submission Date
Secure Best Marks with AI Grader
Need help grading? Try our AI Grader for instant feedback on your assignments.
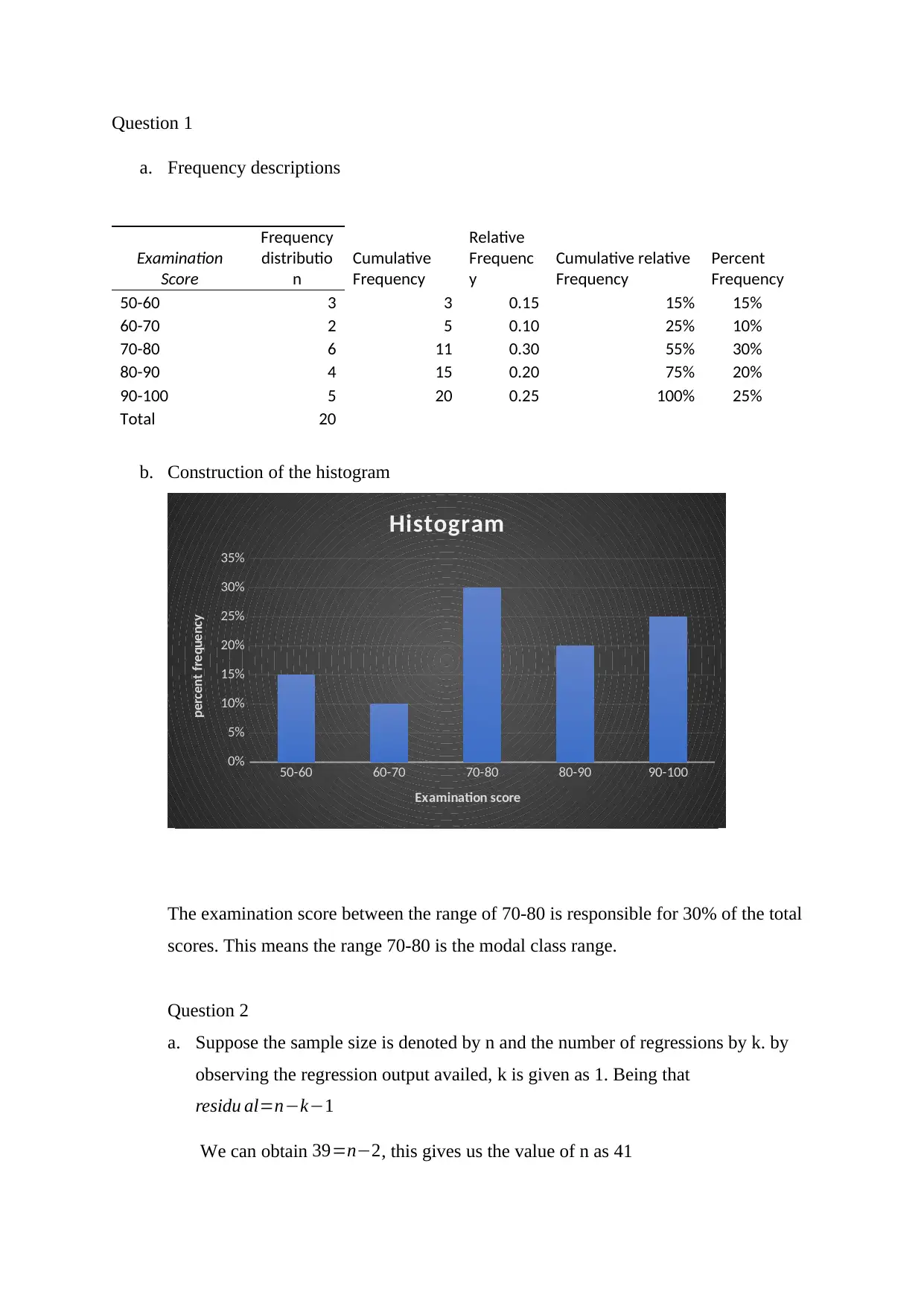
Question 1
a. Frequency descriptions
Examination
Score
Frequency
distributio
n
Cumulative
Frequency
Relative
Frequenc
y
Cumulative relative
Frequency
Percent
Frequency
50-60 3 3 0.15 15% 15%
60-70 2 5 0.10 25% 10%
70-80 6 11 0.30 55% 30%
80-90 4 15 0.20 75% 20%
90-100 5 20 0.25 100% 25%
Total 20
b. Construction of the histogram
50-60 60-70 70-80 80-90 90-100
0%
5%
10%
15%
20%
25%
30%
35%
Histogram
Examination score
percent frequency
The examination score between the range of 70-80 is responsible for 30% of the total
scores. This means the range 70-80 is the modal class range.
Question 2
a. Suppose the sample size is denoted by n and the number of regressions by k. by
observing the regression output availed, k is given as 1. Being that
residu al=n−k−1
We can obtain 39=n−2, this gives us the value of n as 41
a. Frequency descriptions
Examination
Score
Frequency
distributio
n
Cumulative
Frequency
Relative
Frequenc
y
Cumulative relative
Frequency
Percent
Frequency
50-60 3 3 0.15 15% 15%
60-70 2 5 0.10 25% 10%
70-80 6 11 0.30 55% 30%
80-90 4 15 0.20 75% 20%
90-100 5 20 0.25 100% 25%
Total 20
b. Construction of the histogram
50-60 60-70 70-80 80-90 90-100
0%
5%
10%
15%
20%
25%
30%
35%
Histogram
Examination score
percent frequency
The examination score between the range of 70-80 is responsible for 30% of the total
scores. This means the range 70-80 is the modal class range.
Question 2
a. Suppose the sample size is denoted by n and the number of regressions by k. by
observing the regression output availed, k is given as 1. Being that
residu al=n−k−1
We can obtain 39=n−2, this gives us the value of n as 41
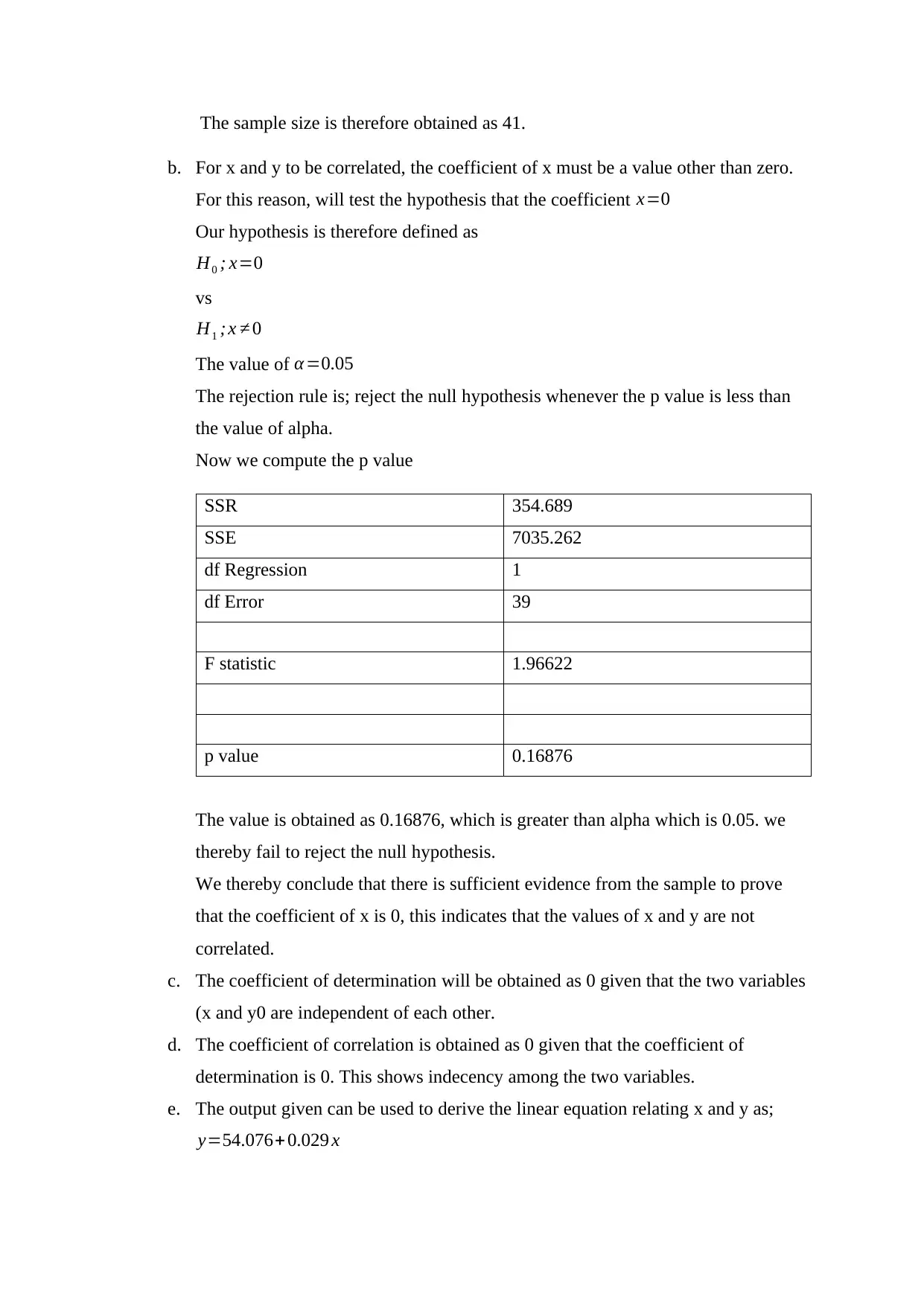
The sample size is therefore obtained as 41.
b. For x and y to be correlated, the coefficient of x must be a value other than zero.
For this reason, will test the hypothesis that the coefficient x=0
Our hypothesis is therefore defined as
H0 ; x=0
vs
H1 ; x ≠ 0
The value of α =0.05
The rejection rule is; reject the null hypothesis whenever the p value is less than
the value of alpha.
Now we compute the p value
SSR 354.689
SSE 7035.262
df Regression 1
df Error 39
F statistic 1.96622
p value 0.16876
The value is obtained as 0.16876, which is greater than alpha which is 0.05. we
thereby fail to reject the null hypothesis.
We thereby conclude that there is sufficient evidence from the sample to prove
that the coefficient of x is 0, this indicates that the values of x and y are not
correlated.
c. The coefficient of determination will be obtained as 0 given that the two variables
(x and y0 are independent of each other.
d. The coefficient of correlation is obtained as 0 given that the coefficient of
determination is 0. This shows indecency among the two variables.
e. The output given can be used to derive the linear equation relating x and y as;
y=54.076+ 0.029 x
b. For x and y to be correlated, the coefficient of x must be a value other than zero.
For this reason, will test the hypothesis that the coefficient x=0
Our hypothesis is therefore defined as
H0 ; x=0
vs
H1 ; x ≠ 0
The value of α =0.05
The rejection rule is; reject the null hypothesis whenever the p value is less than
the value of alpha.
Now we compute the p value
SSR 354.689
SSE 7035.262
df Regression 1
df Error 39
F statistic 1.96622
p value 0.16876
The value is obtained as 0.16876, which is greater than alpha which is 0.05. we
thereby fail to reject the null hypothesis.
We thereby conclude that there is sufficient evidence from the sample to prove
that the coefficient of x is 0, this indicates that the values of x and y are not
correlated.
c. The coefficient of determination will be obtained as 0 given that the two variables
(x and y0 are independent of each other.
d. The coefficient of correlation is obtained as 0 given that the coefficient of
determination is 0. This shows indecency among the two variables.
e. The output given can be used to derive the linear equation relating x and y as;
y=54.076+ 0.029 x
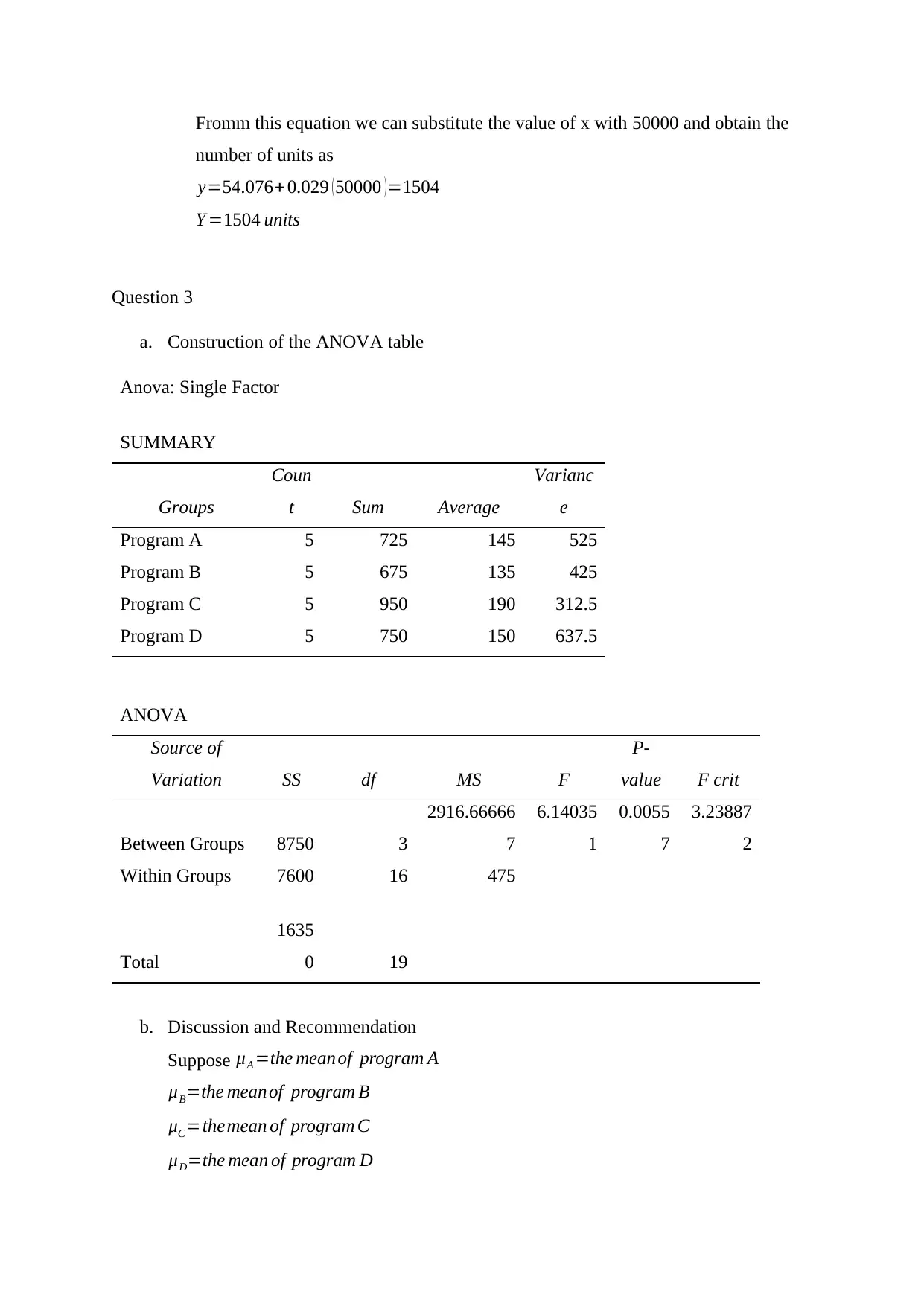
Fromm this equation we can substitute the value of x with 50000 and obtain the
number of units as
y=54.076+0.029 (50000 )=1504
Y =1504 units
Question 3
a. Construction of the ANOVA table
Anova: Single Factor
SUMMARY
Groups
Coun
t Sum Average
Varianc
e
Program A 5 725 145 525
Program B 5 675 135 425
Program C 5 950 190 312.5
Program D 5 750 150 637.5
ANOVA
Source of
Variation SS df MS F
P-
value F crit
Between Groups 8750 3
2916.66666
7
6.14035
1
0.0055
7
3.23887
2
Within Groups 7600 16 475
Total
1635
0 19
b. Discussion and Recommendation
Suppose μA =the meanof program A
μB=the meanof program B
μC=themean of program C
μD=the mean of program D
number of units as
y=54.076+0.029 (50000 )=1504
Y =1504 units
Question 3
a. Construction of the ANOVA table
Anova: Single Factor
SUMMARY
Groups
Coun
t Sum Average
Varianc
e
Program A 5 725 145 525
Program B 5 675 135 425
Program C 5 950 190 312.5
Program D 5 750 150 637.5
ANOVA
Source of
Variation SS df MS F
P-
value F crit
Between Groups 8750 3
2916.66666
7
6.14035
1
0.0055
7
3.23887
2
Within Groups 7600 16 475
Total
1635
0 19
b. Discussion and Recommendation
Suppose μA =the meanof program A
μB=the meanof program B
μC=themean of program C
μD=the mean of program D
Secure Best Marks with AI Grader
Need help grading? Try our AI Grader for instant feedback on your assignments.
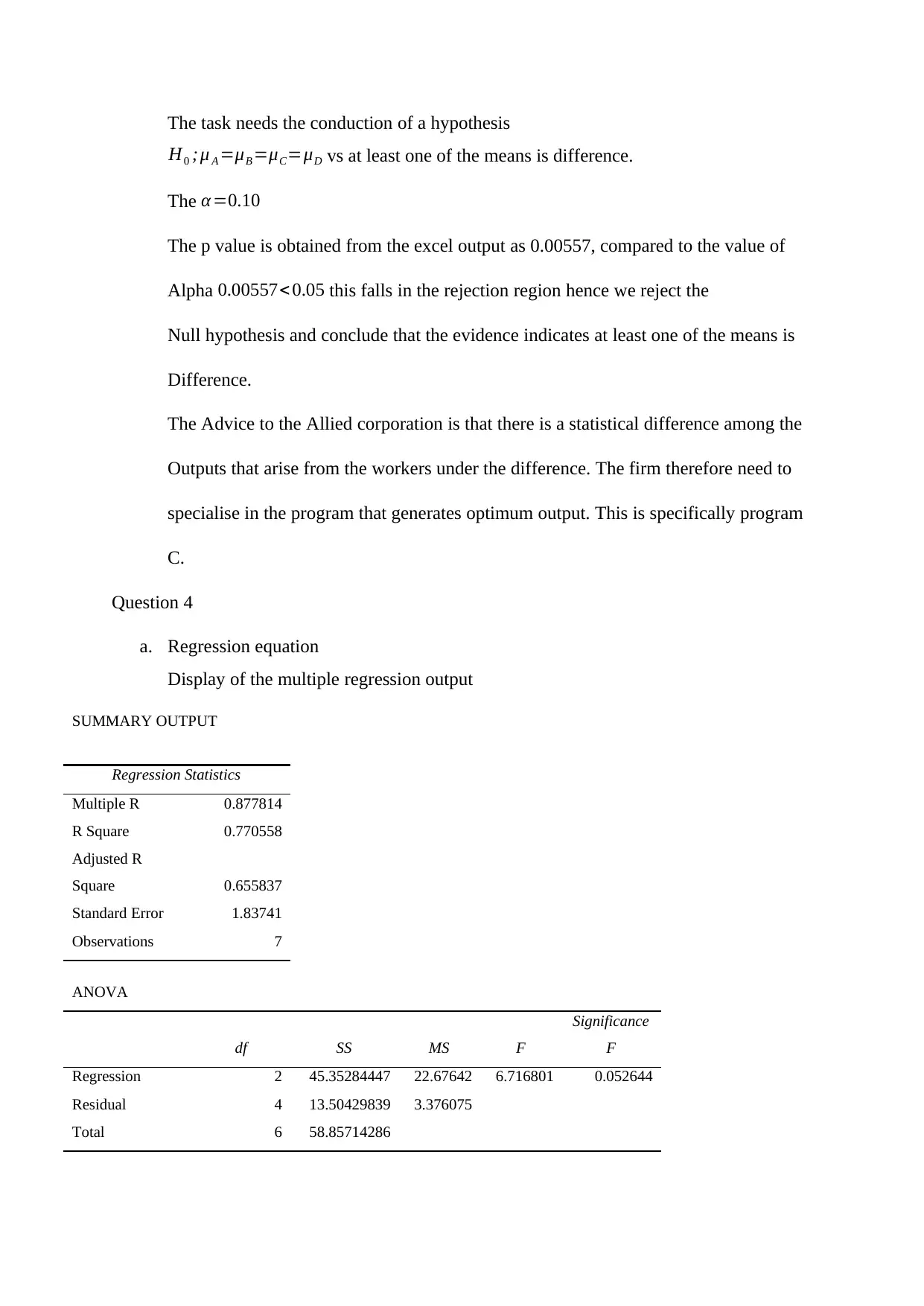
The task needs the conduction of a hypothesis
H0 ;μ A =μB =μC=μD vs at least one of the means is difference.
The α =0.10
The p value is obtained from the excel output as 0.00557, compared to the value of
Alpha 0.00557< 0.05 this falls in the rejection region hence we reject the
Null hypothesis and conclude that the evidence indicates at least one of the means is
Difference.
The Advice to the Allied corporation is that there is a statistical difference among the
Outputs that arise from the workers under the difference. The firm therefore need to
specialise in the program that generates optimum output. This is specifically program
C.
Question 4
a. Regression equation
Display of the multiple regression output
SUMMARY OUTPUT
Regression Statistics
Multiple R 0.877814
R Square 0.770558
Adjusted R
Square 0.655837
Standard Error 1.83741
Observations 7
ANOVA
df SS MS F
Significance
F
Regression 2 45.35284447 22.67642 6.716801 0.052644
Residual 4 13.50429839 3.376075
Total 6 58.85714286
H0 ;μ A =μB =μC=μD vs at least one of the means is difference.
The α =0.10
The p value is obtained from the excel output as 0.00557, compared to the value of
Alpha 0.00557< 0.05 this falls in the rejection region hence we reject the
Null hypothesis and conclude that the evidence indicates at least one of the means is
Difference.
The Advice to the Allied corporation is that there is a statistical difference among the
Outputs that arise from the workers under the difference. The firm therefore need to
specialise in the program that generates optimum output. This is specifically program
C.
Question 4
a. Regression equation
Display of the multiple regression output
SUMMARY OUTPUT
Regression Statistics
Multiple R 0.877814
R Square 0.770558
Adjusted R
Square 0.655837
Standard Error 1.83741
Observations 7
ANOVA
df SS MS F
Significance
F
Regression 2 45.35284447 22.67642 6.716801 0.052644
Residual 4 13.50429839 3.376075
Total 6 58.85714286
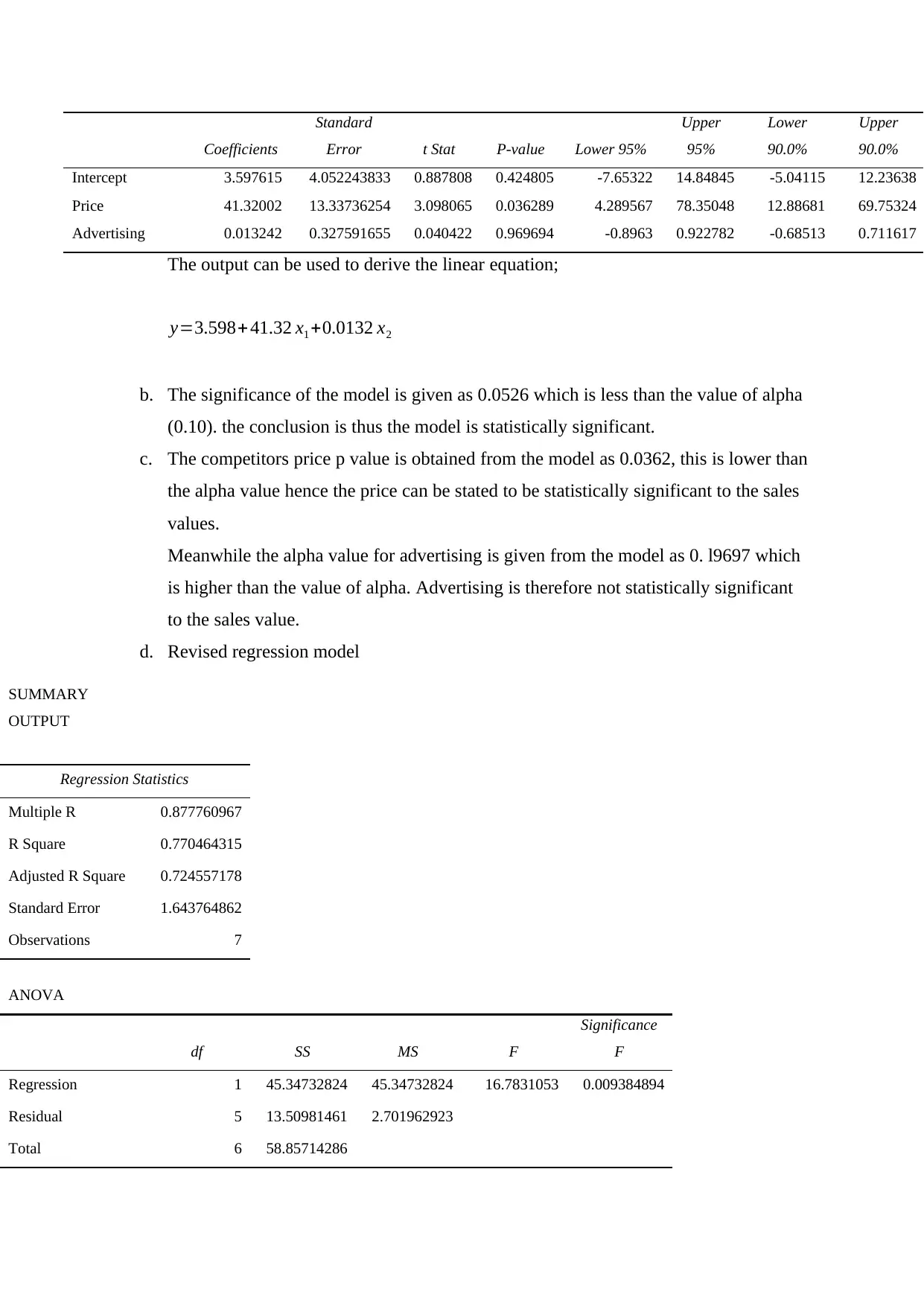
Coefficients
Standard
Error t Stat P-value Lower 95%
Upper
95%
Lower
90.0%
Upper
90.0%
Intercept 3.597615 4.052243833 0.887808 0.424805 -7.65322 14.84845 -5.04115 12.23638
Price 41.32002 13.33736254 3.098065 0.036289 4.289567 78.35048 12.88681 69.75324
Advertising 0.013242 0.327591655 0.040422 0.969694 -0.8963 0.922782 -0.68513 0.711617
The output can be used to derive the linear equation;
y=3.598+ 41.32 x1 +0.0132 x2
b. The significance of the model is given as 0.0526 which is less than the value of alpha
(0.10). the conclusion is thus the model is statistically significant.
c. The competitors price p value is obtained from the model as 0.0362, this is lower than
the alpha value hence the price can be stated to be statistically significant to the sales
values.
Meanwhile the alpha value for advertising is given from the model as 0. l9697 which
is higher than the value of alpha. Advertising is therefore not statistically significant
to the sales value.
d. Revised regression model
SUMMARY
OUTPUT
Regression Statistics
Multiple R 0.877760967
R Square 0.770464315
Adjusted R Square 0.724557178
Standard Error 1.643764862
Observations 7
ANOVA
df SS MS F
Significance
F
Regression 1 45.34732824 45.34732824 16.7831053 0.009384894
Residual 5 13.50981461 2.701962923
Total 6 58.85714286
Standard
Error t Stat P-value Lower 95%
Upper
95%
Lower
90.0%
Upper
90.0%
Intercept 3.597615 4.052243833 0.887808 0.424805 -7.65322 14.84845 -5.04115 12.23638
Price 41.32002 13.33736254 3.098065 0.036289 4.289567 78.35048 12.88681 69.75324
Advertising 0.013242 0.327591655 0.040422 0.969694 -0.8963 0.922782 -0.68513 0.711617
The output can be used to derive the linear equation;
y=3.598+ 41.32 x1 +0.0132 x2
b. The significance of the model is given as 0.0526 which is less than the value of alpha
(0.10). the conclusion is thus the model is statistically significant.
c. The competitors price p value is obtained from the model as 0.0362, this is lower than
the alpha value hence the price can be stated to be statistically significant to the sales
values.
Meanwhile the alpha value for advertising is given from the model as 0. l9697 which
is higher than the value of alpha. Advertising is therefore not statistically significant
to the sales value.
d. Revised regression model
SUMMARY
OUTPUT
Regression Statistics
Multiple R 0.877760967
R Square 0.770464315
Adjusted R Square 0.724557178
Standard Error 1.643764862
Observations 7
ANOVA
df SS MS F
Significance
F
Regression 1 45.34732824 45.34732824 16.7831053 0.009384894
Residual 5 13.50981461 2.701962923
Total 6 58.85714286
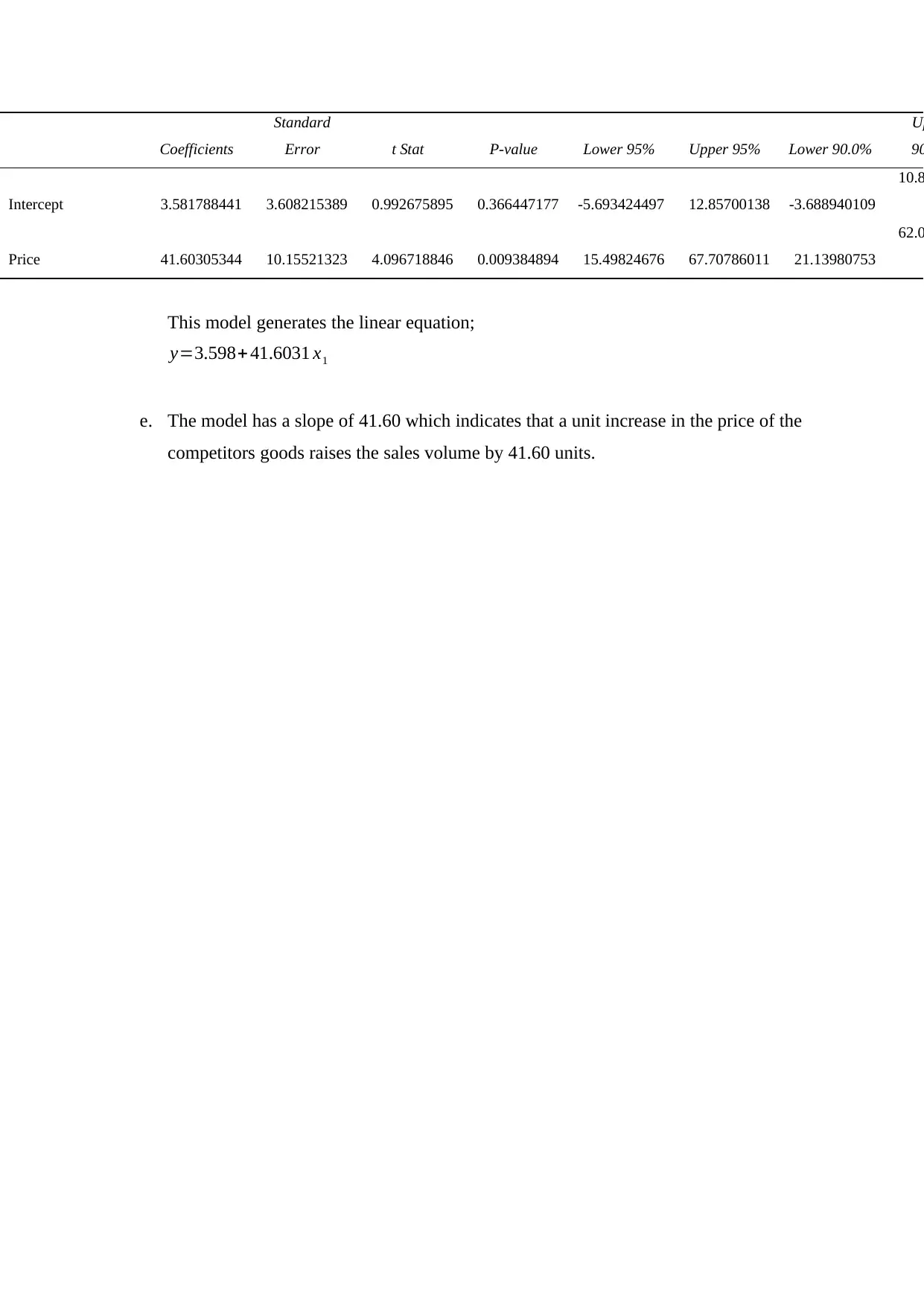
Coefficients
Standard
Error t Stat P-value Lower 95% Upper 95% Lower 90.0%
Up
90
Intercept 3.581788441 3.608215389 0.992675895 0.366447177 -5.693424497 12.85700138 -3.688940109
10.8
Price 41.60305344 10.15521323 4.096718846 0.009384894 15.49824676 67.70786011 21.13980753
62.0
This model generates the linear equation;
y=3.598+ 41.6031 x1
e. The model has a slope of 41.60 which indicates that a unit increase in the price of the
competitors goods raises the sales volume by 41.60 units.
Standard
Error t Stat P-value Lower 95% Upper 95% Lower 90.0%
Up
90
Intercept 3.581788441 3.608215389 0.992675895 0.366447177 -5.693424497 12.85700138 -3.688940109
10.8
Price 41.60305344 10.15521323 4.096718846 0.009384894 15.49824676 67.70786011 21.13980753
62.0
This model generates the linear equation;
y=3.598+ 41.6031 x1
e. The model has a slope of 41.60 which indicates that a unit increase in the price of the
competitors goods raises the sales volume by 41.60 units.
Paraphrase This Document
Need a fresh take? Get an instant paraphrase of this document with our AI Paraphraser
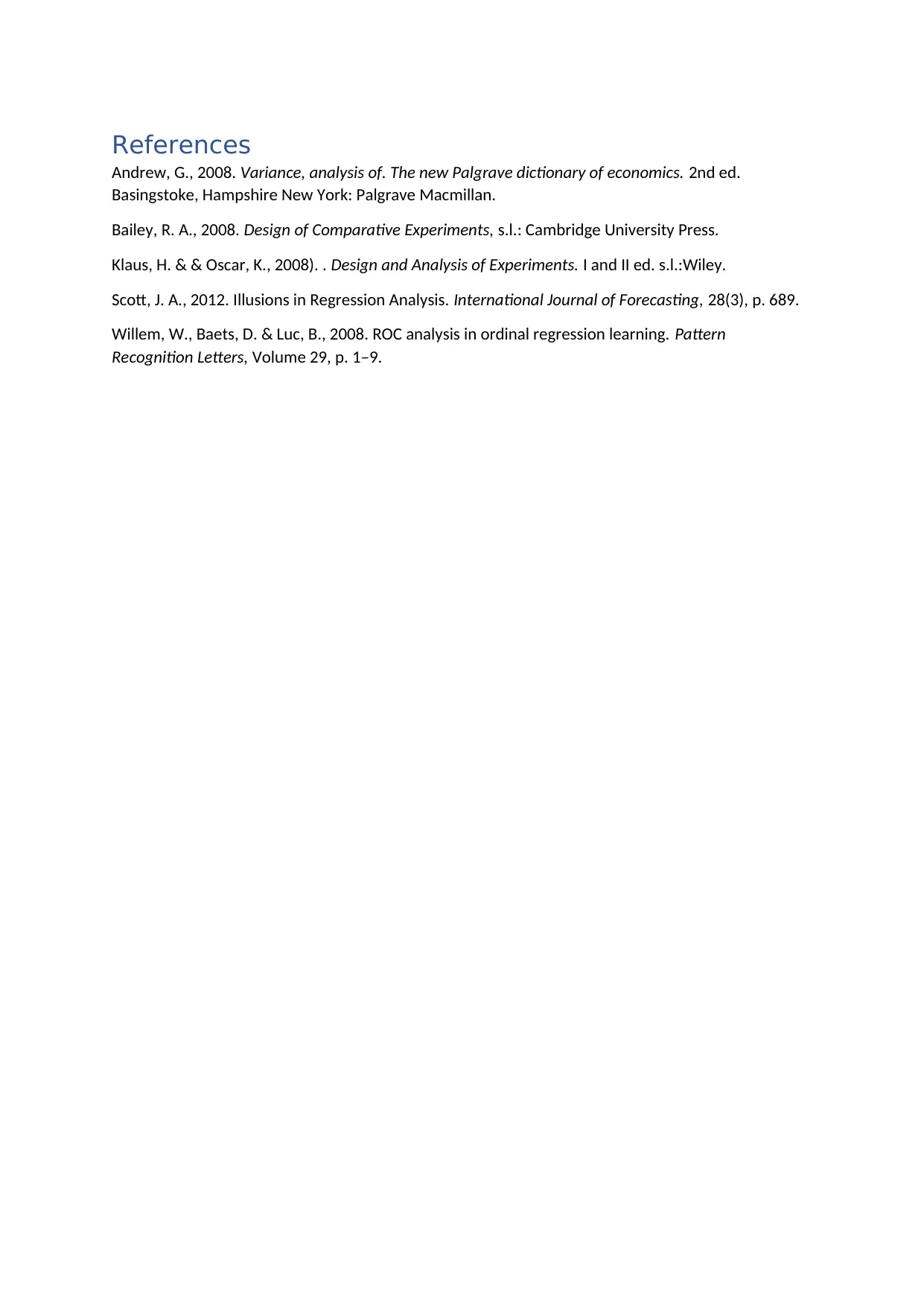
References
Andrew, G., 2008. Variance, analysis of. The new Palgrave dictionary of economics. 2nd ed.
Basingstoke, Hampshire New York: Palgrave Macmillan.
Bailey, R. A., 2008. Design of Comparative Experiments, s.l.: Cambridge University Press.
Klaus, H. & & Oscar, K., 2008). . Design and Analysis of Experiments. I and II ed. s.l.:Wiley.
Scott, J. A., 2012. Illusions in Regression Analysis. International Journal of Forecasting, 28(3), p. 689.
Willem, W., Baets, D. & Luc, B., 2008. ROC analysis in ordinal regression learning. Pattern
Recognition Letters, Volume 29, p. 1–9.
Andrew, G., 2008. Variance, analysis of. The new Palgrave dictionary of economics. 2nd ed.
Basingstoke, Hampshire New York: Palgrave Macmillan.
Bailey, R. A., 2008. Design of Comparative Experiments, s.l.: Cambridge University Press.
Klaus, H. & & Oscar, K., 2008). . Design and Analysis of Experiments. I and II ed. s.l.:Wiley.
Scott, J. A., 2012. Illusions in Regression Analysis. International Journal of Forecasting, 28(3), p. 689.
Willem, W., Baets, D. & Luc, B., 2008. ROC analysis in ordinal regression learning. Pattern
Recognition Letters, Volume 29, p. 1–9.
1 out of 8
Related Documents
![[object Object]](/_next/image/?url=%2F_next%2Fstatic%2Fmedia%2Flogo.6d15ce61.png&w=640&q=75)
Your All-in-One AI-Powered Toolkit for Academic Success.
+13062052269
info@desklib.com
Available 24*7 on WhatsApp / Email
Unlock your academic potential
© 2024 | Zucol Services PVT LTD | All rights reserved.