Analysis of International Flight Traffic in Australia
VerifiedAdded on 2023/04/23
|11
|2383
|237
AI Summary
This report analyzes the performance of Australian airports catering to international flights. It includes analysis of two datasets, hypothesis testing, and graphical summaries. The report concludes that Brisbane and Sydney have superior performance in comparison to Melbourne based on the underlying traffic distribution in Dataset 1. Also, the hypothesis test could not find any relevant relationship between the airports and airlines.
Contribute Materials
Your contribution can guide someone’s learning journey. Share your
documents today.
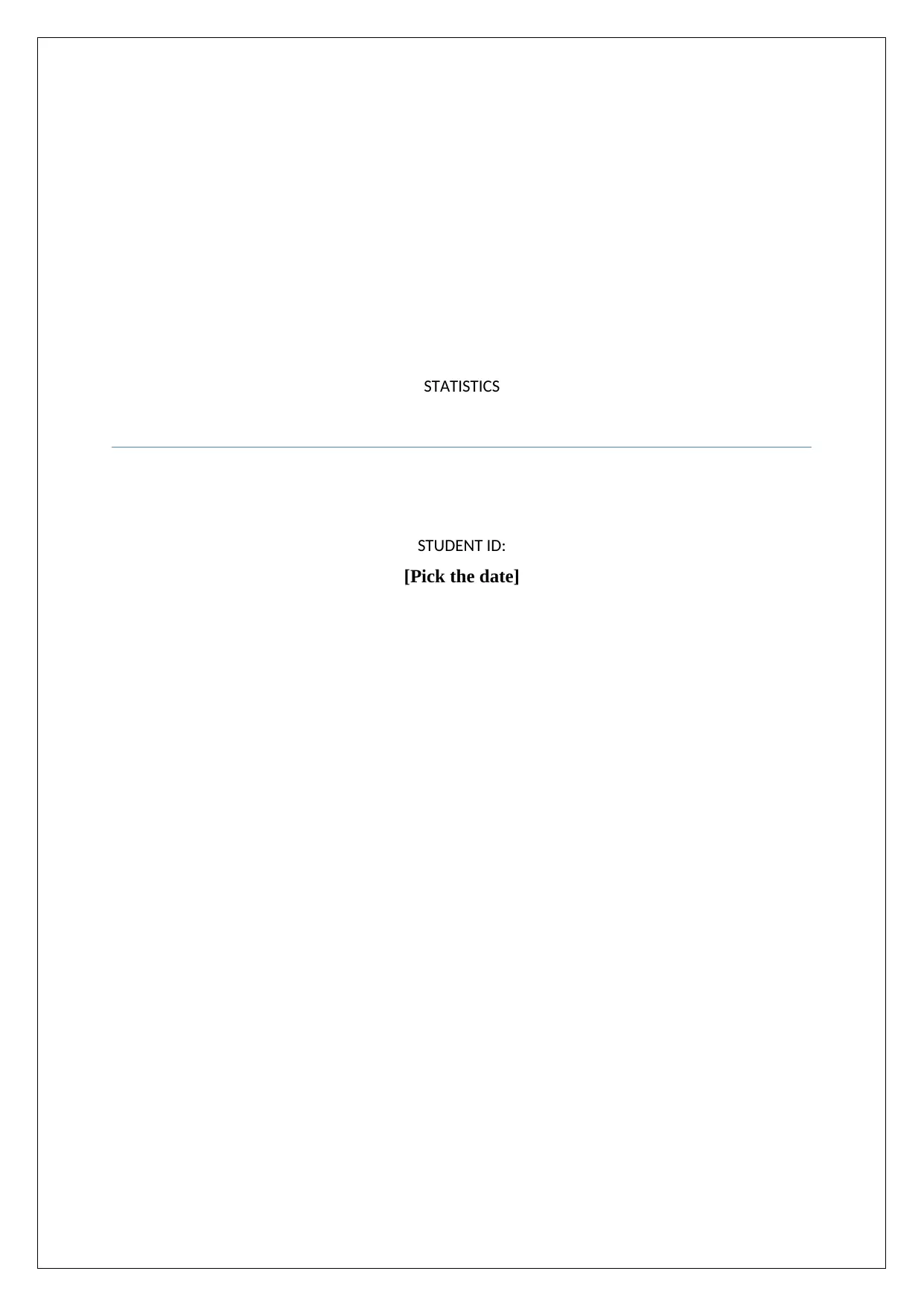
STATISTICS
STUDENT ID:
[Pick the date]
STUDENT ID:
[Pick the date]
Secure Best Marks with AI Grader
Need help grading? Try our AI Grader for instant feedback on your assignments.
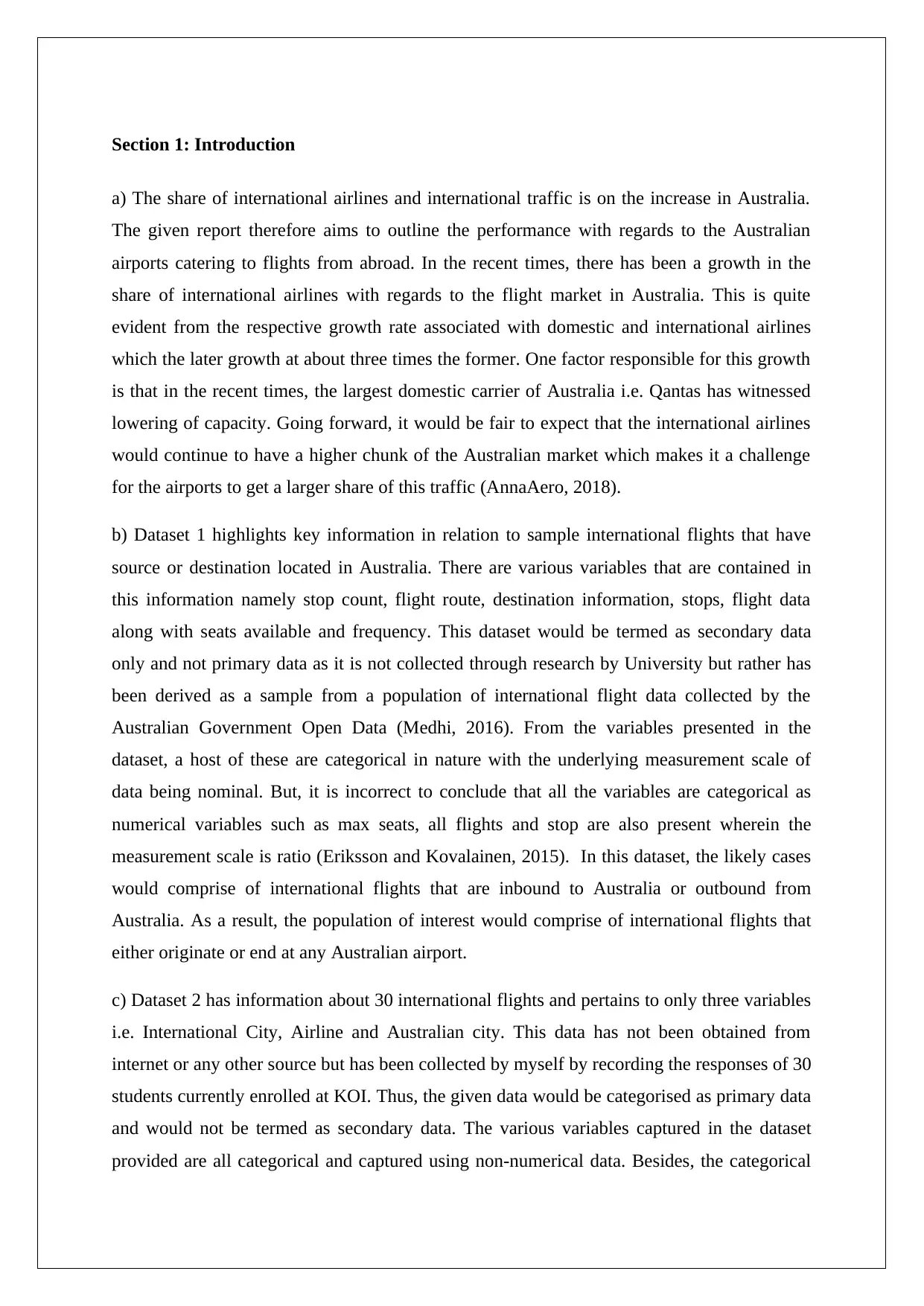
Section 1: Introduction
a) The share of international airlines and international traffic is on the increase in Australia.
The given report therefore aims to outline the performance with regards to the Australian
airports catering to flights from abroad. In the recent times, there has been a growth in the
share of international airlines with regards to the flight market in Australia. This is quite
evident from the respective growth rate associated with domestic and international airlines
which the later growth at about three times the former. One factor responsible for this growth
is that in the recent times, the largest domestic carrier of Australia i.e. Qantas has witnessed
lowering of capacity. Going forward, it would be fair to expect that the international airlines
would continue to have a higher chunk of the Australian market which makes it a challenge
for the airports to get a larger share of this traffic (AnnaAero, 2018).
b) Dataset 1 highlights key information in relation to sample international flights that have
source or destination located in Australia. There are various variables that are contained in
this information namely stop count, flight route, destination information, stops, flight data
along with seats available and frequency. This dataset would be termed as secondary data
only and not primary data as it is not collected through research by University but rather has
been derived as a sample from a population of international flight data collected by the
Australian Government Open Data (Medhi, 2016). From the variables presented in the
dataset, a host of these are categorical in nature with the underlying measurement scale of
data being nominal. But, it is incorrect to conclude that all the variables are categorical as
numerical variables such as max seats, all flights and stop are also present wherein the
measurement scale is ratio (Eriksson and Kovalainen, 2015). In this dataset, the likely cases
would comprise of international flights that are inbound to Australia or outbound from
Australia. As a result, the population of interest would comprise of international flights that
either originate or end at any Australian airport.
c) Dataset 2 has information about 30 international flights and pertains to only three variables
i.e. International City, Airline and Australian city. This data has not been obtained from
internet or any other source but has been collected by myself by recording the responses of 30
students currently enrolled at KOI. Thus, the given data would be categorised as primary data
and would not be termed as secondary data. The various variables captured in the dataset
provided are all categorical and captured using non-numerical data. Besides, the categorical
a) The share of international airlines and international traffic is on the increase in Australia.
The given report therefore aims to outline the performance with regards to the Australian
airports catering to flights from abroad. In the recent times, there has been a growth in the
share of international airlines with regards to the flight market in Australia. This is quite
evident from the respective growth rate associated with domestic and international airlines
which the later growth at about three times the former. One factor responsible for this growth
is that in the recent times, the largest domestic carrier of Australia i.e. Qantas has witnessed
lowering of capacity. Going forward, it would be fair to expect that the international airlines
would continue to have a higher chunk of the Australian market which makes it a challenge
for the airports to get a larger share of this traffic (AnnaAero, 2018).
b) Dataset 1 highlights key information in relation to sample international flights that have
source or destination located in Australia. There are various variables that are contained in
this information namely stop count, flight route, destination information, stops, flight data
along with seats available and frequency. This dataset would be termed as secondary data
only and not primary data as it is not collected through research by University but rather has
been derived as a sample from a population of international flight data collected by the
Australian Government Open Data (Medhi, 2016). From the variables presented in the
dataset, a host of these are categorical in nature with the underlying measurement scale of
data being nominal. But, it is incorrect to conclude that all the variables are categorical as
numerical variables such as max seats, all flights and stop are also present wherein the
measurement scale is ratio (Eriksson and Kovalainen, 2015). In this dataset, the likely cases
would comprise of international flights that are inbound to Australia or outbound from
Australia. As a result, the population of interest would comprise of international flights that
either originate or end at any Australian airport.
c) Dataset 2 has information about 30 international flights and pertains to only three variables
i.e. International City, Airline and Australian city. This data has not been obtained from
internet or any other source but has been collected by myself by recording the responses of 30
students currently enrolled at KOI. Thus, the given data would be categorised as primary data
and would not be termed as secondary data. The various variables captured in the dataset
provided are all categorical and captured using non-numerical data. Besides, the categorical
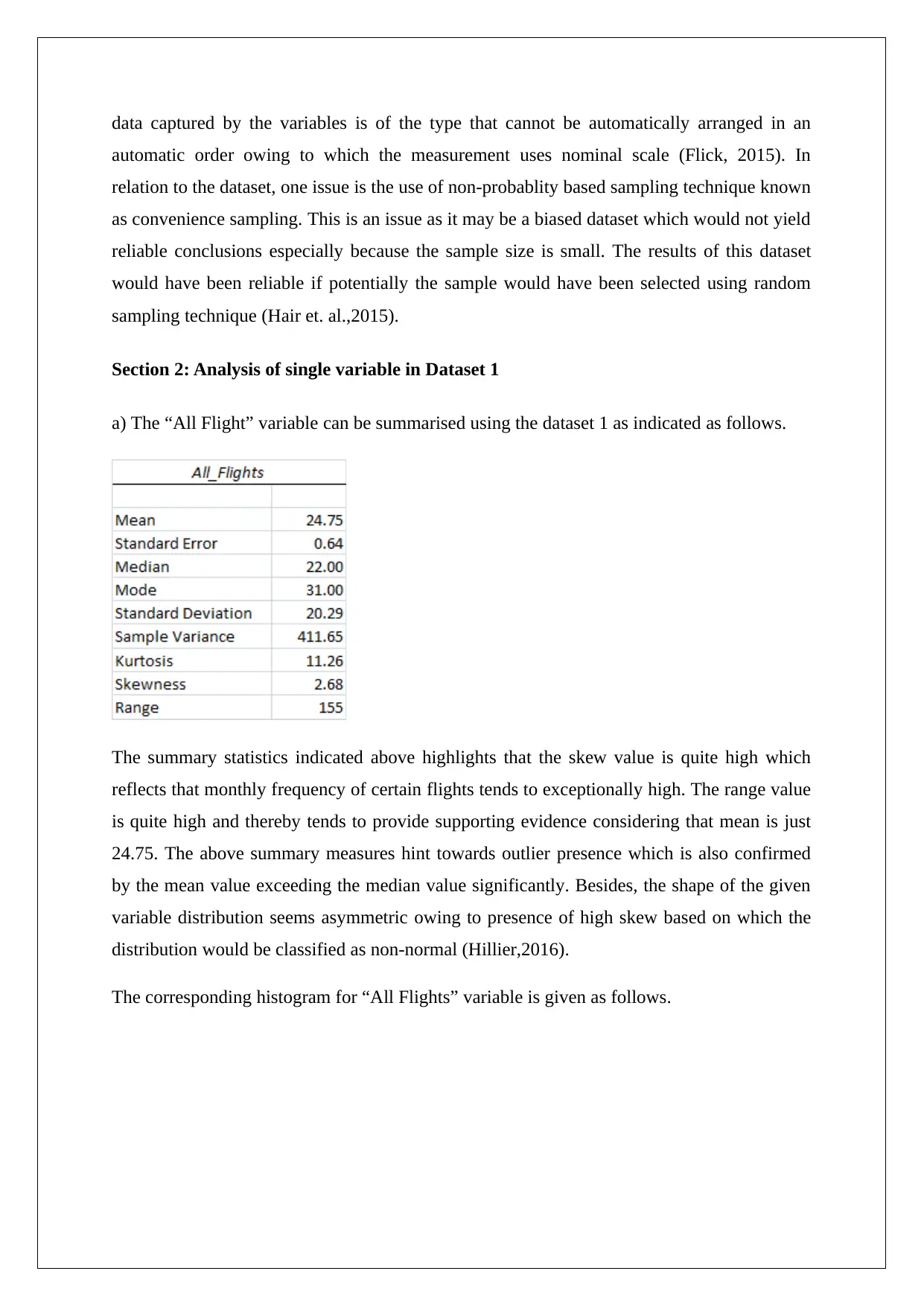
data captured by the variables is of the type that cannot be automatically arranged in an
automatic order owing to which the measurement uses nominal scale (Flick, 2015). In
relation to the dataset, one issue is the use of non-probablity based sampling technique known
as convenience sampling. This is an issue as it may be a biased dataset which would not yield
reliable conclusions especially because the sample size is small. The results of this dataset
would have been reliable if potentially the sample would have been selected using random
sampling technique (Hair et. al.,2015).
Section 2: Analysis of single variable in Dataset 1
a) The “All Flight” variable can be summarised using the dataset 1 as indicated as follows.
The summary statistics indicated above highlights that the skew value is quite high which
reflects that monthly frequency of certain flights tends to exceptionally high. The range value
is quite high and thereby tends to provide supporting evidence considering that mean is just
24.75. The above summary measures hint towards outlier presence which is also confirmed
by the mean value exceeding the median value significantly. Besides, the shape of the given
variable distribution seems asymmetric owing to presence of high skew based on which the
distribution would be classified as non-normal (Hillier,2016).
The corresponding histogram for “All Flights” variable is given as follows.
automatic order owing to which the measurement uses nominal scale (Flick, 2015). In
relation to the dataset, one issue is the use of non-probablity based sampling technique known
as convenience sampling. This is an issue as it may be a biased dataset which would not yield
reliable conclusions especially because the sample size is small. The results of this dataset
would have been reliable if potentially the sample would have been selected using random
sampling technique (Hair et. al.,2015).
Section 2: Analysis of single variable in Dataset 1
a) The “All Flight” variable can be summarised using the dataset 1 as indicated as follows.
The summary statistics indicated above highlights that the skew value is quite high which
reflects that monthly frequency of certain flights tends to exceptionally high. The range value
is quite high and thereby tends to provide supporting evidence considering that mean is just
24.75. The above summary measures hint towards outlier presence which is also confirmed
by the mean value exceeding the median value significantly. Besides, the shape of the given
variable distribution seems asymmetric owing to presence of high skew based on which the
distribution would be classified as non-normal (Hillier,2016).
The corresponding histogram for “All Flights” variable is given as follows.
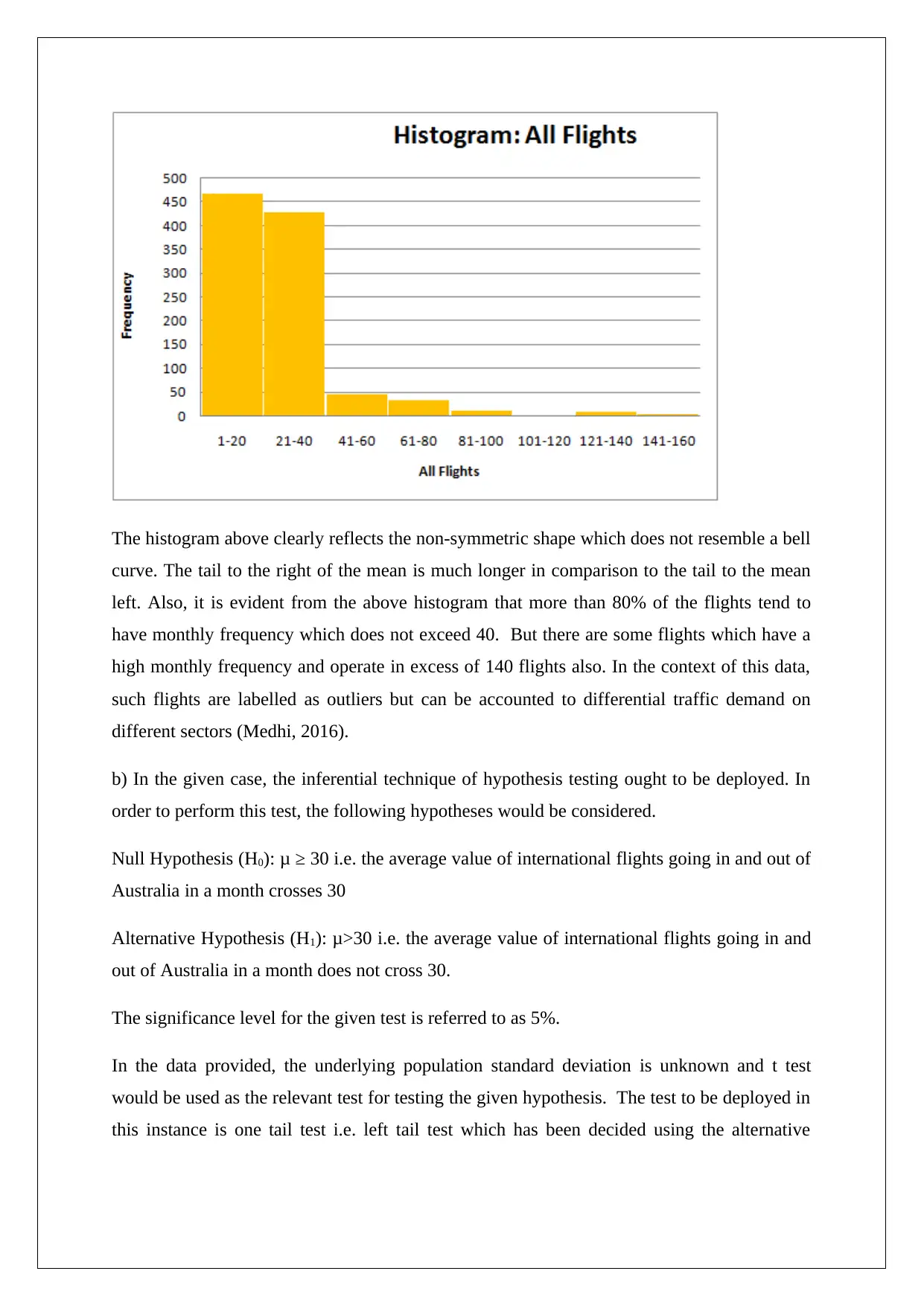
The histogram above clearly reflects the non-symmetric shape which does not resemble a bell
curve. The tail to the right of the mean is much longer in comparison to the tail to the mean
left. Also, it is evident from the above histogram that more than 80% of the flights tend to
have monthly frequency which does not exceed 40. But there are some flights which have a
high monthly frequency and operate in excess of 140 flights also. In the context of this data,
such flights are labelled as outliers but can be accounted to differential traffic demand on
different sectors (Medhi, 2016).
b) In the given case, the inferential technique of hypothesis testing ought to be deployed. In
order to perform this test, the following hypotheses would be considered.
Null Hypothesis (H0): μ ≥ 30 i.e. the average value of international flights going in and out of
Australia in a month crosses 30
Alternative Hypothesis (H1): μ>30 i.e. the average value of international flights going in and
out of Australia in a month does not cross 30.
The significance level for the given test is referred to as 5%.
In the data provided, the underlying population standard deviation is unknown and t test
would be used as the relevant test for testing the given hypothesis. The test to be deployed in
this instance is one tail test i.e. left tail test which has been decided using the alternative
curve. The tail to the right of the mean is much longer in comparison to the tail to the mean
left. Also, it is evident from the above histogram that more than 80% of the flights tend to
have monthly frequency which does not exceed 40. But there are some flights which have a
high monthly frequency and operate in excess of 140 flights also. In the context of this data,
such flights are labelled as outliers but can be accounted to differential traffic demand on
different sectors (Medhi, 2016).
b) In the given case, the inferential technique of hypothesis testing ought to be deployed. In
order to perform this test, the following hypotheses would be considered.
Null Hypothesis (H0): μ ≥ 30 i.e. the average value of international flights going in and out of
Australia in a month crosses 30
Alternative Hypothesis (H1): μ>30 i.e. the average value of international flights going in and
out of Australia in a month does not cross 30.
The significance level for the given test is referred to as 5%.
In the data provided, the underlying population standard deviation is unknown and t test
would be used as the relevant test for testing the given hypothesis. The test to be deployed in
this instance is one tail test i.e. left tail test which has been decided using the alternative
Secure Best Marks with AI Grader
Need help grading? Try our AI Grader for instant feedback on your assignments.
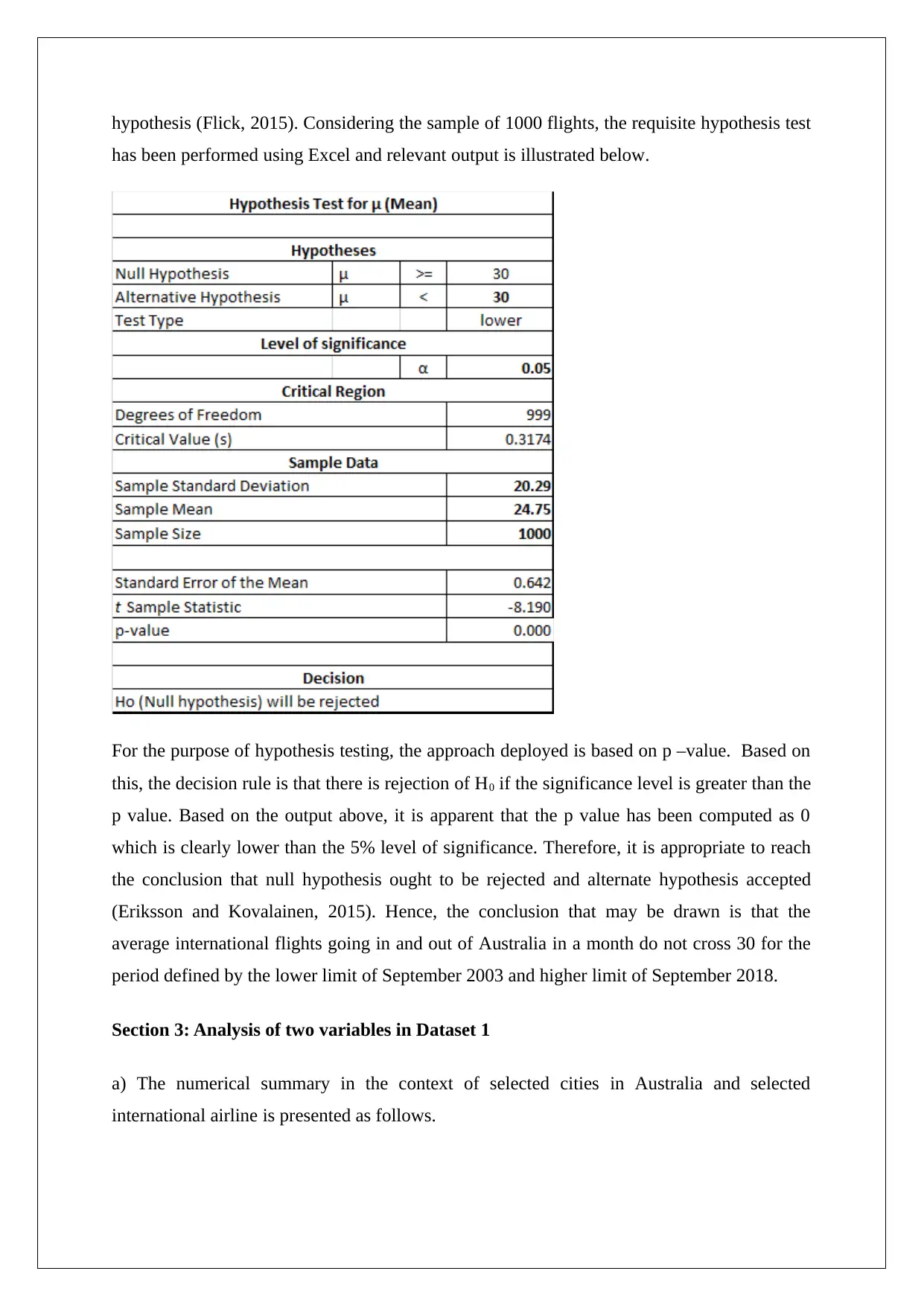
hypothesis (Flick, 2015). Considering the sample of 1000 flights, the requisite hypothesis test
has been performed using Excel and relevant output is illustrated below.
For the purpose of hypothesis testing, the approach deployed is based on p –value. Based on
this, the decision rule is that there is rejection of H0 if the significance level is greater than the
p value. Based on the output above, it is apparent that the p value has been computed as 0
which is clearly lower than the 5% level of significance. Therefore, it is appropriate to reach
the conclusion that null hypothesis ought to be rejected and alternate hypothesis accepted
(Eriksson and Kovalainen, 2015). Hence, the conclusion that may be drawn is that the
average international flights going in and out of Australia in a month do not cross 30 for the
period defined by the lower limit of September 2003 and higher limit of September 2018.
Section 3: Analysis of two variables in Dataset 1
a) The numerical summary in the context of selected cities in Australia and selected
international airline is presented as follows.
has been performed using Excel and relevant output is illustrated below.
For the purpose of hypothesis testing, the approach deployed is based on p –value. Based on
this, the decision rule is that there is rejection of H0 if the significance level is greater than the
p value. Based on the output above, it is apparent that the p value has been computed as 0
which is clearly lower than the 5% level of significance. Therefore, it is appropriate to reach
the conclusion that null hypothesis ought to be rejected and alternate hypothesis accepted
(Eriksson and Kovalainen, 2015). Hence, the conclusion that may be drawn is that the
average international flights going in and out of Australia in a month do not cross 30 for the
period defined by the lower limit of September 2003 and higher limit of September 2018.
Section 3: Analysis of two variables in Dataset 1
a) The numerical summary in the context of selected cities in Australia and selected
international airline is presented as follows.
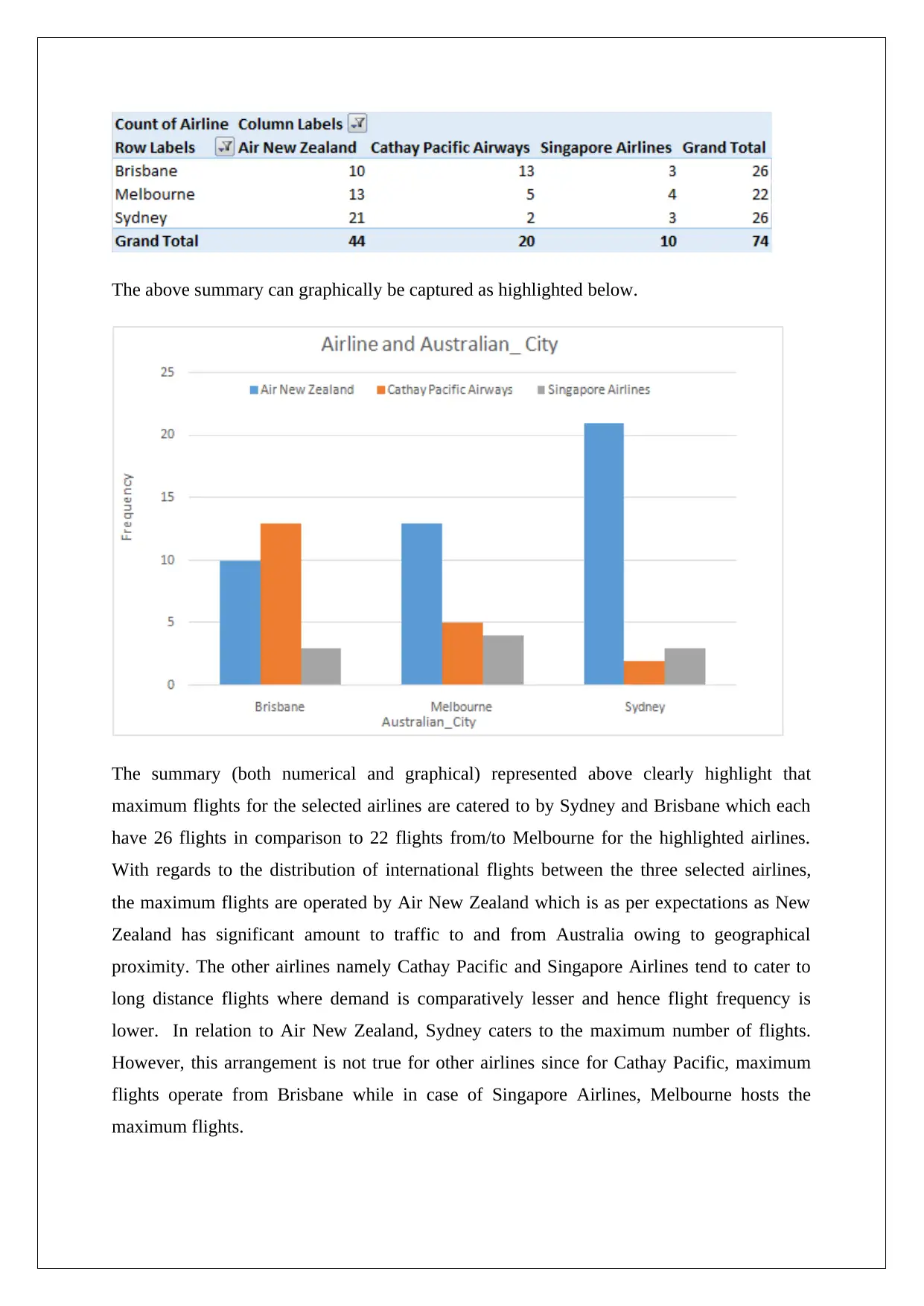
The above summary can graphically be captured as highlighted below.
The summary (both numerical and graphical) represented above clearly highlight that
maximum flights for the selected airlines are catered to by Sydney and Brisbane which each
have 26 flights in comparison to 22 flights from/to Melbourne for the highlighted airlines.
With regards to the distribution of international flights between the three selected airlines,
the maximum flights are operated by Air New Zealand which is as per expectations as New
Zealand has significant amount to traffic to and from Australia owing to geographical
proximity. The other airlines namely Cathay Pacific and Singapore Airlines tend to cater to
long distance flights where demand is comparatively lesser and hence flight frequency is
lower. In relation to Air New Zealand, Sydney caters to the maximum number of flights.
However, this arrangement is not true for other airlines since for Cathay Pacific, maximum
flights operate from Brisbane while in case of Singapore Airlines, Melbourne hosts the
maximum flights.
The summary (both numerical and graphical) represented above clearly highlight that
maximum flights for the selected airlines are catered to by Sydney and Brisbane which each
have 26 flights in comparison to 22 flights from/to Melbourne for the highlighted airlines.
With regards to the distribution of international flights between the three selected airlines,
the maximum flights are operated by Air New Zealand which is as per expectations as New
Zealand has significant amount to traffic to and from Australia owing to geographical
proximity. The other airlines namely Cathay Pacific and Singapore Airlines tend to cater to
long distance flights where demand is comparatively lesser and hence flight frequency is
lower. In relation to Air New Zealand, Sydney caters to the maximum number of flights.
However, this arrangement is not true for other airlines since for Cathay Pacific, maximum
flights operate from Brisbane while in case of Singapore Airlines, Melbourne hosts the
maximum flights.
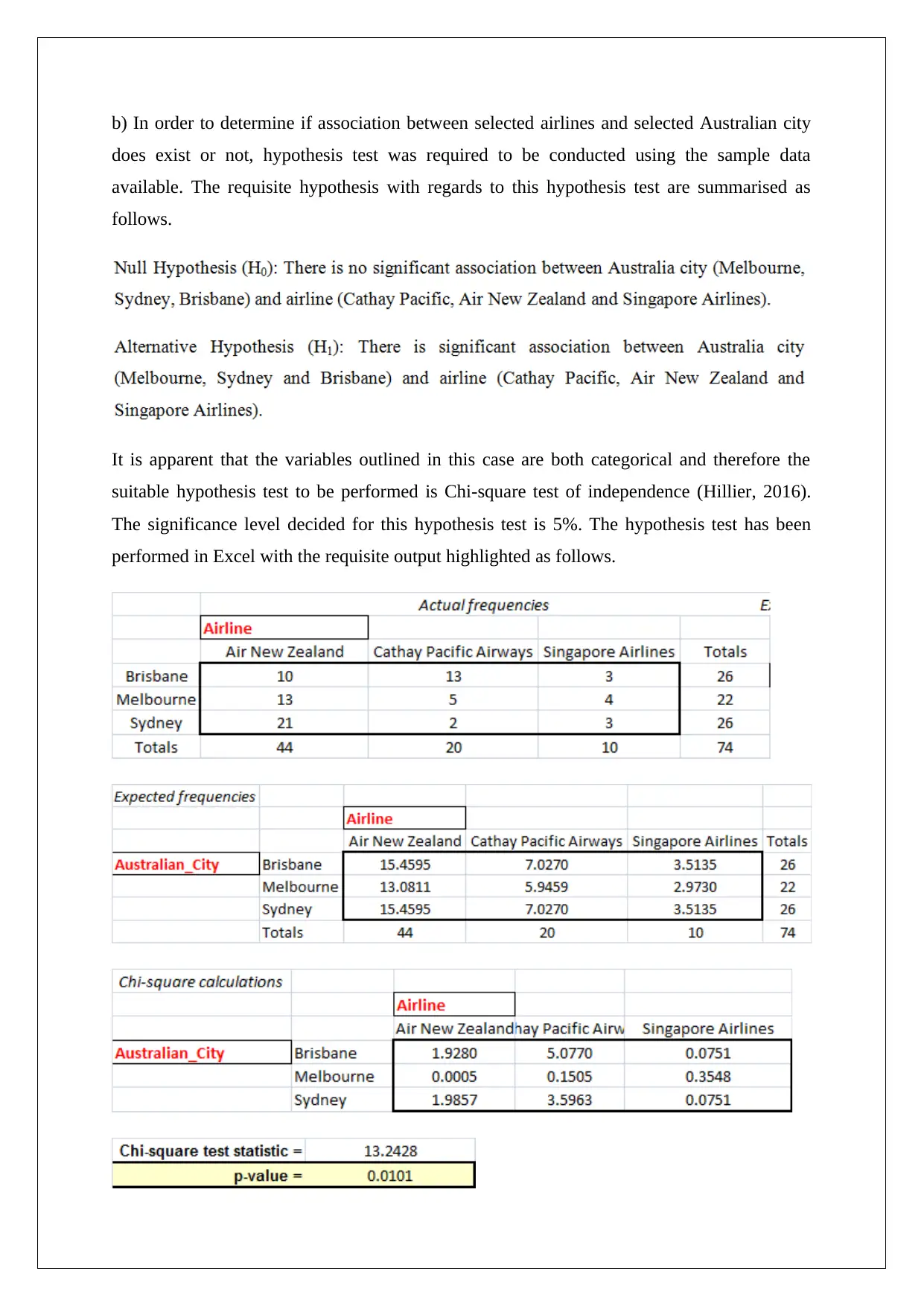
b) In order to determine if association between selected airlines and selected Australian city
does exist or not, hypothesis test was required to be conducted using the sample data
available. The requisite hypothesis with regards to this hypothesis test are summarised as
follows.
It is apparent that the variables outlined in this case are both categorical and therefore the
suitable hypothesis test to be performed is Chi-square test of independence (Hillier, 2016).
The significance level decided for this hypothesis test is 5%. The hypothesis test has been
performed in Excel with the requisite output highlighted as follows.
does exist or not, hypothesis test was required to be conducted using the sample data
available. The requisite hypothesis with regards to this hypothesis test are summarised as
follows.
It is apparent that the variables outlined in this case are both categorical and therefore the
suitable hypothesis test to be performed is Chi-square test of independence (Hillier, 2016).
The significance level decided for this hypothesis test is 5%. The hypothesis test has been
performed in Excel with the requisite output highlighted as follows.
Paraphrase This Document
Need a fresh take? Get an instant paraphrase of this document with our AI Paraphraser
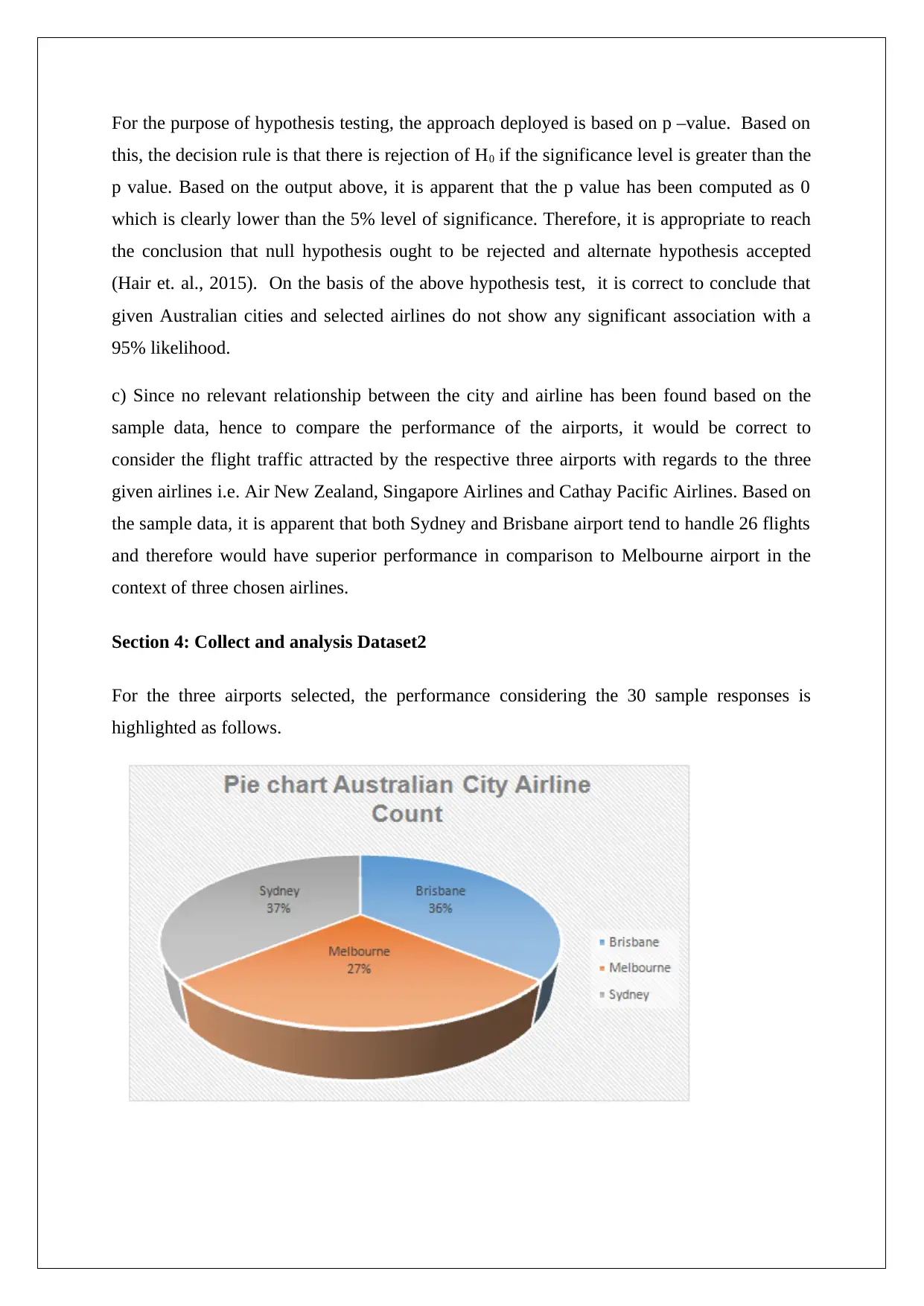
For the purpose of hypothesis testing, the approach deployed is based on p –value. Based on
this, the decision rule is that there is rejection of H0 if the significance level is greater than the
p value. Based on the output above, it is apparent that the p value has been computed as 0
which is clearly lower than the 5% level of significance. Therefore, it is appropriate to reach
the conclusion that null hypothesis ought to be rejected and alternate hypothesis accepted
(Hair et. al., 2015). On the basis of the above hypothesis test, it is correct to conclude that
given Australian cities and selected airlines do not show any significant association with a
95% likelihood.
c) Since no relevant relationship between the city and airline has been found based on the
sample data, hence to compare the performance of the airports, it would be correct to
consider the flight traffic attracted by the respective three airports with regards to the three
given airlines i.e. Air New Zealand, Singapore Airlines and Cathay Pacific Airlines. Based on
the sample data, it is apparent that both Sydney and Brisbane airport tend to handle 26 flights
and therefore would have superior performance in comparison to Melbourne airport in the
context of three chosen airlines.
Section 4: Collect and analysis Dataset2
For the three airports selected, the performance considering the 30 sample responses is
highlighted as follows.
this, the decision rule is that there is rejection of H0 if the significance level is greater than the
p value. Based on the output above, it is apparent that the p value has been computed as 0
which is clearly lower than the 5% level of significance. Therefore, it is appropriate to reach
the conclusion that null hypothesis ought to be rejected and alternate hypothesis accepted
(Hair et. al., 2015). On the basis of the above hypothesis test, it is correct to conclude that
given Australian cities and selected airlines do not show any significant association with a
95% likelihood.
c) Since no relevant relationship between the city and airline has been found based on the
sample data, hence to compare the performance of the airports, it would be correct to
consider the flight traffic attracted by the respective three airports with regards to the three
given airlines i.e. Air New Zealand, Singapore Airlines and Cathay Pacific Airlines. Based on
the sample data, it is apparent that both Sydney and Brisbane airport tend to handle 26 flights
and therefore would have superior performance in comparison to Melbourne airport in the
context of three chosen airlines.
Section 4: Collect and analysis Dataset2
For the three airports selected, the performance considering the 30 sample responses is
highlighted as follows.
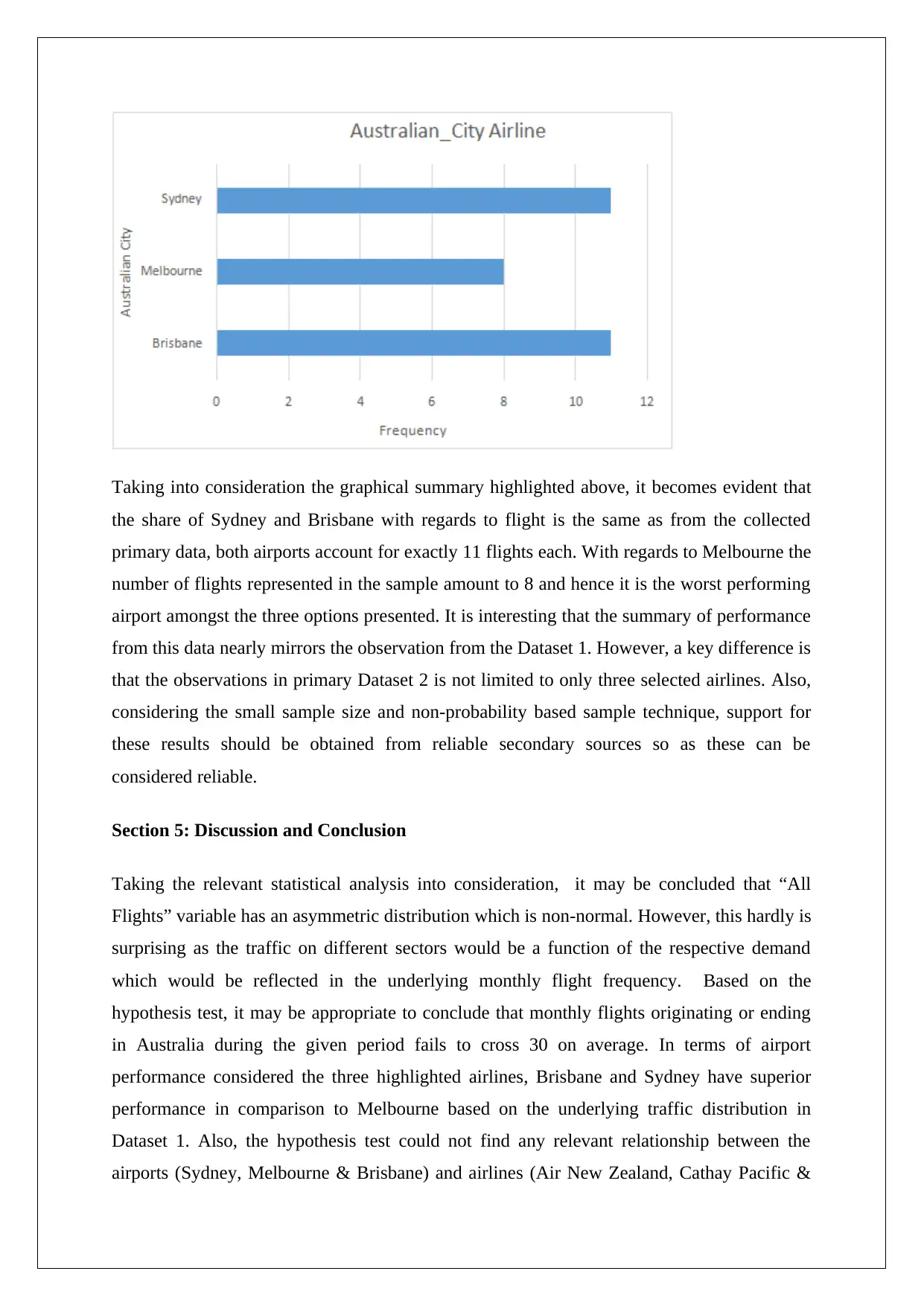
Taking into consideration the graphical summary highlighted above, it becomes evident that
the share of Sydney and Brisbane with regards to flight is the same as from the collected
primary data, both airports account for exactly 11 flights each. With regards to Melbourne the
number of flights represented in the sample amount to 8 and hence it is the worst performing
airport amongst the three options presented. It is interesting that the summary of performance
from this data nearly mirrors the observation from the Dataset 1. However, a key difference is
that the observations in primary Dataset 2 is not limited to only three selected airlines. Also,
considering the small sample size and non-probability based sample technique, support for
these results should be obtained from reliable secondary sources so as these can be
considered reliable.
Section 5: Discussion and Conclusion
Taking the relevant statistical analysis into consideration, it may be concluded that “All
Flights” variable has an asymmetric distribution which is non-normal. However, this hardly is
surprising as the traffic on different sectors would be a function of the respective demand
which would be reflected in the underlying monthly flight frequency. Based on the
hypothesis test, it may be appropriate to conclude that monthly flights originating or ending
in Australia during the given period fails to cross 30 on average. In terms of airport
performance considered the three highlighted airlines, Brisbane and Sydney have superior
performance in comparison to Melbourne based on the underlying traffic distribution in
Dataset 1. Also, the hypothesis test could not find any relevant relationship between the
airports (Sydney, Melbourne & Brisbane) and airlines (Air New Zealand, Cathay Pacific &
the share of Sydney and Brisbane with regards to flight is the same as from the collected
primary data, both airports account for exactly 11 flights each. With regards to Melbourne the
number of flights represented in the sample amount to 8 and hence it is the worst performing
airport amongst the three options presented. It is interesting that the summary of performance
from this data nearly mirrors the observation from the Dataset 1. However, a key difference is
that the observations in primary Dataset 2 is not limited to only three selected airlines. Also,
considering the small sample size and non-probability based sample technique, support for
these results should be obtained from reliable secondary sources so as these can be
considered reliable.
Section 5: Discussion and Conclusion
Taking the relevant statistical analysis into consideration, it may be concluded that “All
Flights” variable has an asymmetric distribution which is non-normal. However, this hardly is
surprising as the traffic on different sectors would be a function of the respective demand
which would be reflected in the underlying monthly flight frequency. Based on the
hypothesis test, it may be appropriate to conclude that monthly flights originating or ending
in Australia during the given period fails to cross 30 on average. In terms of airport
performance considered the three highlighted airlines, Brisbane and Sydney have superior
performance in comparison to Melbourne based on the underlying traffic distribution in
Dataset 1. Also, the hypothesis test could not find any relevant relationship between the
airports (Sydney, Melbourne & Brisbane) and airlines (Air New Zealand, Cathay Pacific &
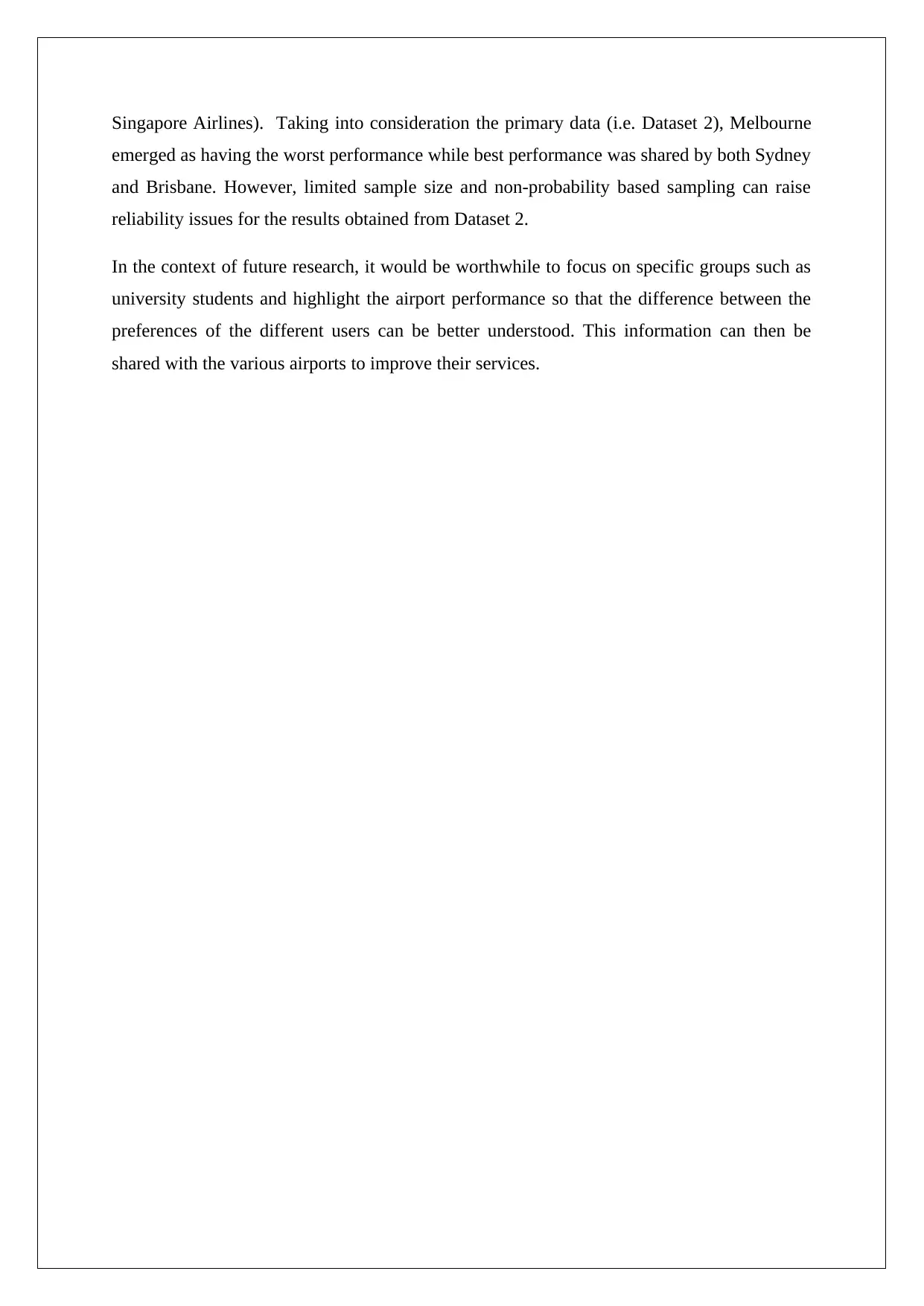
Singapore Airlines). Taking into consideration the primary data (i.e. Dataset 2), Melbourne
emerged as having the worst performance while best performance was shared by both Sydney
and Brisbane. However, limited sample size and non-probability based sampling can raise
reliability issues for the results obtained from Dataset 2.
In the context of future research, it would be worthwhile to focus on specific groups such as
university students and highlight the airport performance so that the difference between the
preferences of the different users can be better understood. This information can then be
shared with the various airports to improve their services.
emerged as having the worst performance while best performance was shared by both Sydney
and Brisbane. However, limited sample size and non-probability based sampling can raise
reliability issues for the results obtained from Dataset 2.
In the context of future research, it would be worthwhile to focus on specific groups such as
university students and highlight the airport performance so that the difference between the
preferences of the different users can be better understood. This information can then be
shared with the various airports to improve their services.
Secure Best Marks with AI Grader
Need help grading? Try our AI Grader for instant feedback on your assignments.
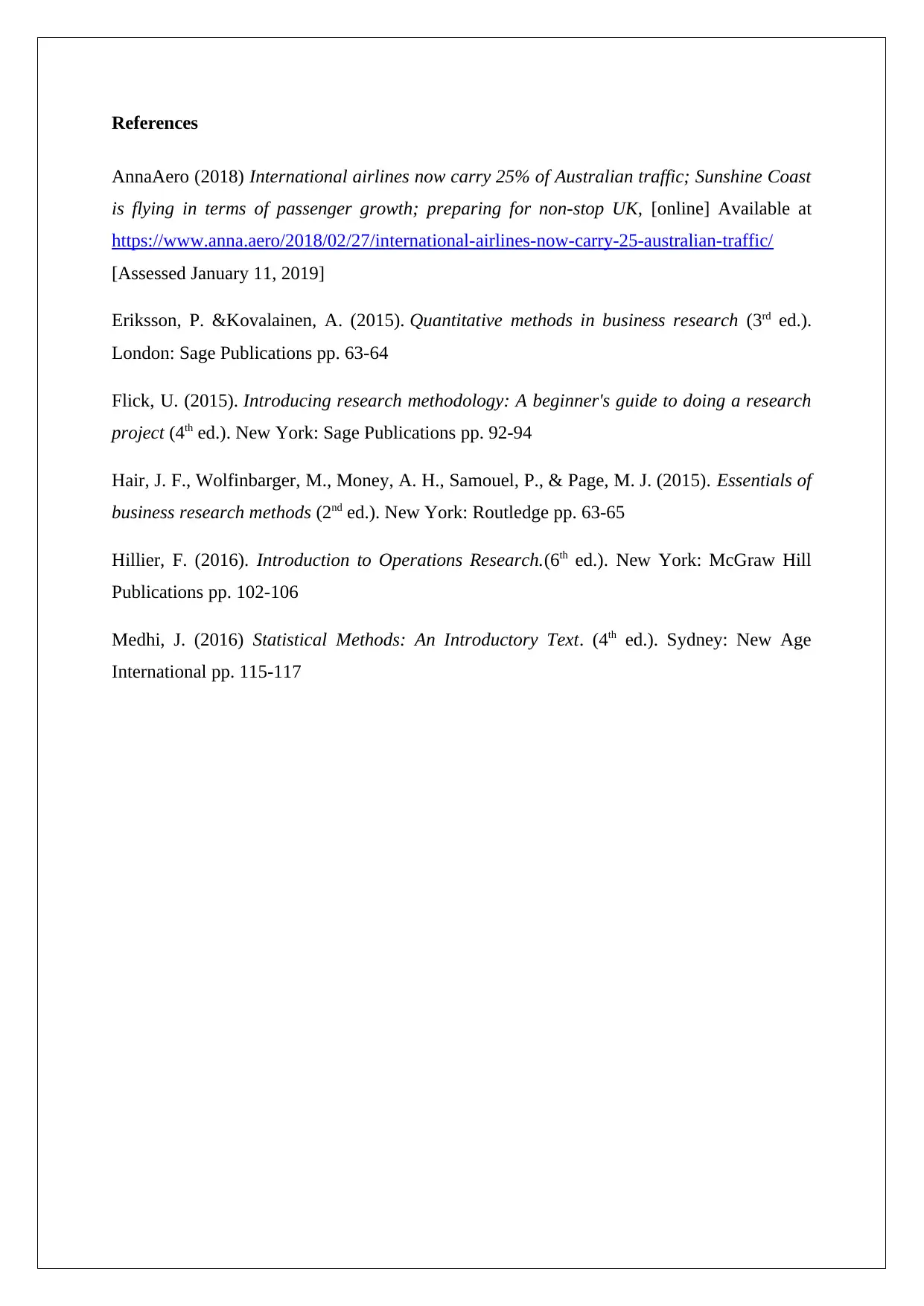
References
AnnaAero (2018) International airlines now carry 25% of Australian traffic; Sunshine Coast
is flying in terms of passenger growth; preparing for non-stop UK, [online] Available at
https://www.anna.aero/2018/02/27/international-airlines-now-carry-25-australian-traffic/
[Assessed January 11, 2019]
Eriksson, P. &Kovalainen, A. (2015). Quantitative methods in business research (3rd ed.).
London: Sage Publications pp. 63-64
Flick, U. (2015). Introducing research methodology: A beginner's guide to doing a research
project (4th ed.). New York: Sage Publications pp. 92-94
Hair, J. F., Wolfinbarger, M., Money, A. H., Samouel, P., & Page, M. J. (2015). Essentials of
business research methods (2nd ed.). New York: Routledge pp. 63-65
Hillier, F. (2016). Introduction to Operations Research.(6th ed.). New York: McGraw Hill
Publications pp. 102-106
Medhi, J. (2016) Statistical Methods: An Introductory Text. (4th ed.). Sydney: New Age
International pp. 115-117
AnnaAero (2018) International airlines now carry 25% of Australian traffic; Sunshine Coast
is flying in terms of passenger growth; preparing for non-stop UK, [online] Available at
https://www.anna.aero/2018/02/27/international-airlines-now-carry-25-australian-traffic/
[Assessed January 11, 2019]
Eriksson, P. &Kovalainen, A. (2015). Quantitative methods in business research (3rd ed.).
London: Sage Publications pp. 63-64
Flick, U. (2015). Introducing research methodology: A beginner's guide to doing a research
project (4th ed.). New York: Sage Publications pp. 92-94
Hair, J. F., Wolfinbarger, M., Money, A. H., Samouel, P., & Page, M. J. (2015). Essentials of
business research methods (2nd ed.). New York: Routledge pp. 63-65
Hillier, F. (2016). Introduction to Operations Research.(6th ed.). New York: McGraw Hill
Publications pp. 102-106
Medhi, J. (2016) Statistical Methods: An Introductory Text. (4th ed.). Sydney: New Age
International pp. 115-117
1 out of 11
Related Documents
![[object Object]](/_next/image/?url=%2F_next%2Fstatic%2Fmedia%2Flogo.6d15ce61.png&w=640&q=75)
Your All-in-One AI-Powered Toolkit for Academic Success.
+13062052269
info@desklib.com
Available 24*7 on WhatsApp / Email
Unlock your academic potential
© 2024 | Zucol Services PVT LTD | All rights reserved.