Market Research Analysis and Recommendations
VerifiedAdded on  2020/02/24
|16
|3320
|344
AI Summary
This assignment focuses on analyzing market research data presented in several tables. The tables cover various aspects such as customer quality perception, brand image, preferred sales channels (direct purchase vs. sales representative), and the relationship between these factors and customer recommendations. Students are expected to interpret the data presented in these tables, calculating marginal probabilities and drawing conclusions about customer preferences and market trends.
Contribute Materials
Your contribution can guide someone’s learning journey. Share your
documents today.
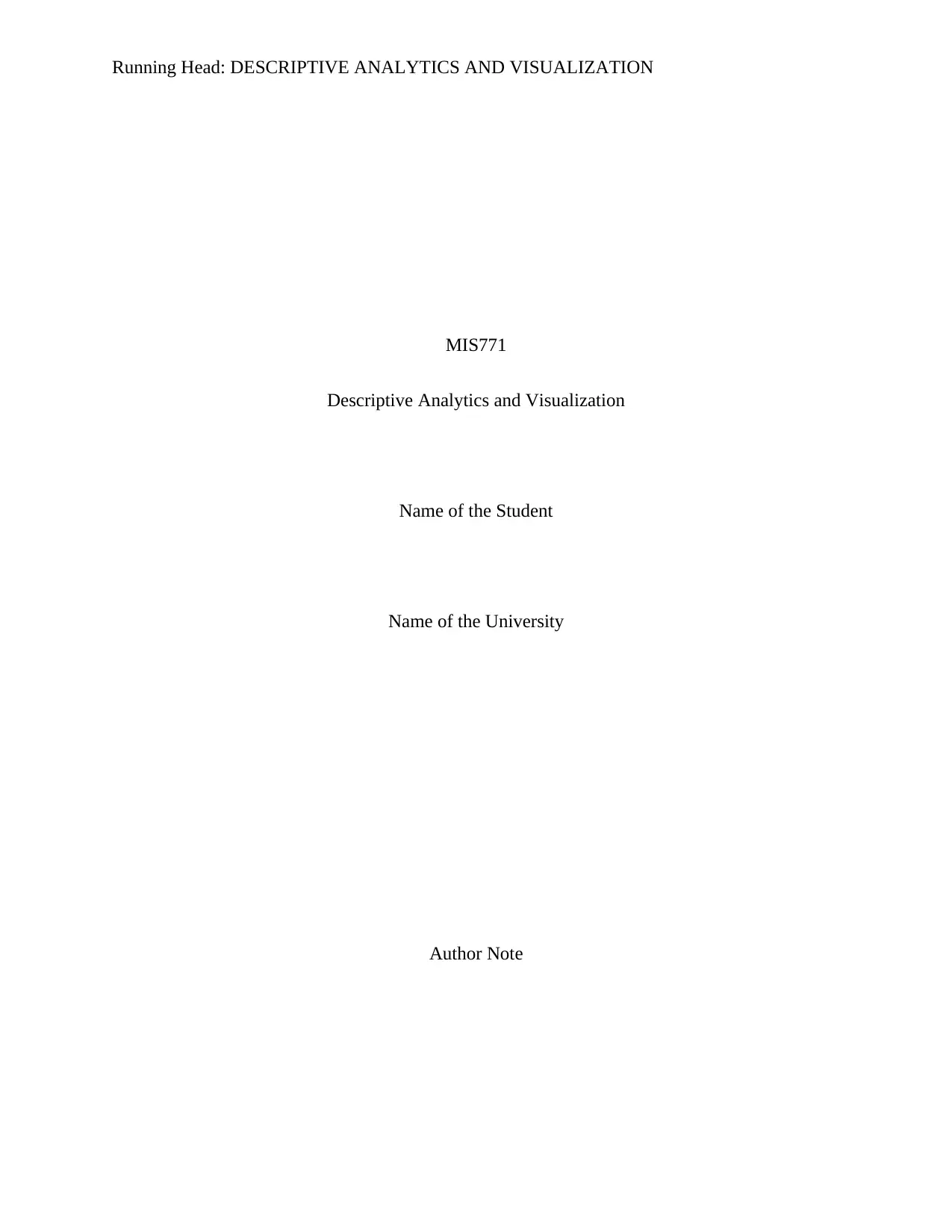
Running Head: DESCRIPTIVE ANALYTICS AND VISUALIZATION
MIS771
Descriptive Analytics and Visualization
Name of the Student
Name of the University
Author Note
MIS771
Descriptive Analytics and Visualization
Name of the Student
Name of the University
Author Note
Secure Best Marks with AI Grader
Need help grading? Try our AI Grader for instant feedback on your assignments.

1DESCRIPTIVE ANALYTICS AND VISUALIZATION
Table of Contents
1. Introduction..................................................................................................................................2
2. Collection of Data........................................................................................................................3
3. Description of the Data................................................................................................................3
4. Analysis of the Data.....................................................................................................................3
4.1 Dependent Variable Analysis................................................................................................3
4.2 Factors Influencing Repurchasing Intention..........................................................................5
4.2.1 Variable Identification....................................................................................................5
4.2.2 Prediction of Repurchasing Intention.............................................................................7
4.2.3 Different Analysis...........................................................................................................8
4.3 Furphy Recommendation.......................................................................................................9
4.4 Analysis based on Time Series............................................................................................11
5. Recommendations and Conclusion............................................................................................11
REFERENCES..............................................................................................................................13
APPENDICES...............................................................................................................................14
Table of Contents
1. Introduction..................................................................................................................................2
2. Collection of Data........................................................................................................................3
3. Description of the Data................................................................................................................3
4. Analysis of the Data.....................................................................................................................3
4.1 Dependent Variable Analysis................................................................................................3
4.2 Factors Influencing Repurchasing Intention..........................................................................5
4.2.1 Variable Identification....................................................................................................5
4.2.2 Prediction of Repurchasing Intention.............................................................................7
4.2.3 Different Analysis...........................................................................................................8
4.3 Furphy Recommendation.......................................................................................................9
4.4 Analysis based on Time Series............................................................................................11
5. Recommendations and Conclusion............................................................................................11
REFERENCES..............................................................................................................................13
APPENDICES...............................................................................................................................14

2DESCRIPTIVE ANALYTICS AND VISUALIZATION
1. Introduction
Furphy Beer is a micro-brewery company born in Australia. The company is a new
company with less than 15 years of experience in the brewing ale. The production and sales of
the company is limited in the city of Melbourne and reginal Victoria. The sales and production is
increasing rapidly in all the other parts of Australia. In the last two years significant growth in
the production and sales have been noticed. The demand of the company has been increasing so
much that in the year 2016 they decided to increase the production to 3 million litres per year in
order to cope up with the increasing demand of the product.
There are two market segments, which buy the Furphy pale ale. One is pubs, bars and
restaurants and the other is bottleshops. The beer produced by Furphy are sold in these two
markets either directly or with the help of sales representatives.
The company has experienced huge success in their operations and financial turnovers in
the previous two years. Then also, the company can sense a change in the business climate in the
upcoming five years. This can be the outcome of the high popularity of craft beer and
microbrewery culture in Victoria and its surrounding regions. Thus, with the increase in the
competition, the company has felt the necessity of building a strong relationship with the
customers. Thus, to understand and identify the factors that are responsible to build a strong
customer relationship the company has appointed a market research company named Beautiful
Data. The have been asked to conduct a large scale survey of the clients of the company to have
a clear understanding of the clients characteristics and their repurchasing intentions.
1. Introduction
Furphy Beer is a micro-brewery company born in Australia. The company is a new
company with less than 15 years of experience in the brewing ale. The production and sales of
the company is limited in the city of Melbourne and reginal Victoria. The sales and production is
increasing rapidly in all the other parts of Australia. In the last two years significant growth in
the production and sales have been noticed. The demand of the company has been increasing so
much that in the year 2016 they decided to increase the production to 3 million litres per year in
order to cope up with the increasing demand of the product.
There are two market segments, which buy the Furphy pale ale. One is pubs, bars and
restaurants and the other is bottleshops. The beer produced by Furphy are sold in these two
markets either directly or with the help of sales representatives.
The company has experienced huge success in their operations and financial turnovers in
the previous two years. Then also, the company can sense a change in the business climate in the
upcoming five years. This can be the outcome of the high popularity of craft beer and
microbrewery culture in Victoria and its surrounding regions. Thus, with the increase in the
competition, the company has felt the necessity of building a strong relationship with the
customers. Thus, to understand and identify the factors that are responsible to build a strong
customer relationship the company has appointed a market research company named Beautiful
Data. The have been asked to conduct a large scale survey of the clients of the company to have
a clear understanding of the clients characteristics and their repurchasing intentions.

3DESCRIPTIVE ANALYTICS AND VISUALIZATION
2. Collection of Data
To meet the criteria provided by Furphy, the market research company Beautiful Data
made contacts with Furphy’s clients and asked them to fill up an online survey. The survey
questionnaire contained various factors necessary for the calculation. The data on the past years
sales in the four quarters per year have been collected from the information stored and compiled
through Furphy’s datamart.
3. Description of the Data
The data collected from 200 clients of the company has 9 different perceptions of the
customers or clients. These perceptions have been recorded in a scale of 1 – 10. Other variables
give qualitative information about the outcomes of the purchases by the clients and their business
relationship with the respective clients. The analysis has been done using the MS Excel software.
4. Analysis of the Data
4.1 Dependent Variable Analysis
The dependent variables that has to be analyzed and predicted with the data collected are
intention of the customers in repurchasing Furphy’s products and recommending the products of
the company Furphy to others. The test results show that most of the ratings are between 7 and 8.
Thus, the product from the customers has received high ratings. Hence, the product is
repurchased by the clients. The clients have a high probability of repurchasing the product. Table
1 gives the repurchasing intentions of the clients. This is shown diagrammatically in figure 1.
2. Collection of Data
To meet the criteria provided by Furphy, the market research company Beautiful Data
made contacts with Furphy’s clients and asked them to fill up an online survey. The survey
questionnaire contained various factors necessary for the calculation. The data on the past years
sales in the four quarters per year have been collected from the information stored and compiled
through Furphy’s datamart.
3. Description of the Data
The data collected from 200 clients of the company has 9 different perceptions of the
customers or clients. These perceptions have been recorded in a scale of 1 – 10. Other variables
give qualitative information about the outcomes of the purchases by the clients and their business
relationship with the respective clients. The analysis has been done using the MS Excel software.
4. Analysis of the Data
4.1 Dependent Variable Analysis
The dependent variables that has to be analyzed and predicted with the data collected are
intention of the customers in repurchasing Furphy’s products and recommending the products of
the company Furphy to others. The test results show that most of the ratings are between 7 and 8.
Thus, the product from the customers has received high ratings. Hence, the product is
repurchased by the clients. The clients have a high probability of repurchasing the product. Table
1 gives the repurchasing intentions of the clients. This is shown diagrammatically in figure 1.
Secure Best Marks with AI Grader
Need help grading? Try our AI Grader for instant feedback on your assignments.

4DESCRIPTIVE ANALYTICS AND VISUALIZATION
Table 1: Repurchasing intentions of Clients
Row Labels Count of Repurchase_Int
4-5 1
5-6 4
6-7 29
7-8 87
8-9 64
9-10 15
Grand Total 200
4-5 5-6 6-7 7-8 8-9 9-101 4
29
87
64
15
Total
Total
Figure 1: Repurchase ratings by customers
Another dependent that has been considered is the recommendation given by the clients
towards purchasing the product. The table 2 shows the results of the opinions given by the
customers. It shows clearly that the beer from the brand Furphy is highly recommended by the
customers. However, it can also be observed that the percentages of recommending the brand
and not recommending the brand are close to each other. Thus, it is clear from here that there are
a lot of clients of the company who do not recommend the brand. Hence, if Furphy wants to
survive in the market of competition with the increasing market of craft beer and another
Table 1: Repurchasing intentions of Clients
Row Labels Count of Repurchase_Int
4-5 1
5-6 4
6-7 29
7-8 87
8-9 64
9-10 15
Grand Total 200
4-5 5-6 6-7 7-8 8-9 9-101 4
29
87
64
15
Total
Total
Figure 1: Repurchase ratings by customers
Another dependent that has been considered is the recommendation given by the clients
towards purchasing the product. The table 2 shows the results of the opinions given by the
customers. It shows clearly that the beer from the brand Furphy is highly recommended by the
customers. However, it can also be observed that the percentages of recommending the brand
and not recommending the brand are close to each other. Thus, it is clear from here that there are
a lot of clients of the company who do not recommend the brand. Hence, if Furphy wants to
survive in the market of competition with the increasing market of craft beer and another

5DESCRIPTIVE ANALYTICS AND VISUALIZATION
brewery rising in the locality, it is necessary for Furphy to identify the factors that are holding its
prime clients from recommending the brand to others. The distribution is shown in figure 2.
Table 2: Recommendations given by Clients
Row Labels Count of Recommend
No 99
Yes 101
Grand Total 200
50%51%
Total
No
Yes
Figure 2: Percentage of clients recommending Furphy
4.2 Factors Influencing Repurchasing Intention
It is important to identify the potential variables that are responsible in influencing the
repurchasing intention of Furphy beer. To identify the variable regression analysis has been done
on the complete dataset.
4.2.1 Variable Identification
The data collected by the market research company from the clients of Furphy beer
involves a lot of variables. All of the variables might not be significant to predict the
brewery rising in the locality, it is necessary for Furphy to identify the factors that are holding its
prime clients from recommending the brand to others. The distribution is shown in figure 2.
Table 2: Recommendations given by Clients
Row Labels Count of Recommend
No 99
Yes 101
Grand Total 200
50%51%
Total
No
Yes
Figure 2: Percentage of clients recommending Furphy
4.2 Factors Influencing Repurchasing Intention
It is important to identify the potential variables that are responsible in influencing the
repurchasing intention of Furphy beer. To identify the variable regression analysis has been done
on the complete dataset.
4.2.1 Variable Identification
The data collected by the market research company from the clients of Furphy beer
involves a lot of variables. All of the variables might not be significant to predict the

6DESCRIPTIVE ANALYTICS AND VISUALIZATION
repurchasing intentions of the product. In order to identify which are the significant variables the
regression is necessary to run and check the p-values of the variables involved (Draper and
Smith 2014). The regression has been run at ninety-five percent level of significance. The
variables with the p-value higher than 0.05 (95 percent level of significance) are termed as
insignificant variables and the variables with the p-value lower than 0.05 are termed as
significant variables (Montgomery, Peck and Vining 2015). Regression analysis is the most
important analysis in this case as with the help of this analysis only the significant variables can
be identified (Chatterjee and Hadi 2015). Analysis of variance (ANOVA) technique can be used
to check whether all the independent variables overall signify the dependent variable (Kleinbaum
et al.2015). The ANOVA table (Table 3) shows that the significance value is less than 0.05.
Thus, the independent variables that have been taken into consideration for this regression
analysis has an overall impact in predicting the dependent variable repurchasing intention of the
clients.
Table 3: ANOVA involving all Independent Variables
df SS MS F Significance F
Regression 13 74.616 5.740 12.685 1.08754E-19
Residual 186 84.159 0.452
Total 199 158.775
Table 5 shows the regression coefficients and the p-values of the independent variables
individually. From the table, it can be seen that the variables that mostly influence the purchasing
intention of the customers are
ï‚· Loyalty of the customers that is the length of the time the particular customer has been
buying from Furphy
 Distribution Channel that is how the Furphy’s products are sold to the customers.
repurchasing intentions of the product. In order to identify which are the significant variables the
regression is necessary to run and check the p-values of the variables involved (Draper and
Smith 2014). The regression has been run at ninety-five percent level of significance. The
variables with the p-value higher than 0.05 (95 percent level of significance) are termed as
insignificant variables and the variables with the p-value lower than 0.05 are termed as
significant variables (Montgomery, Peck and Vining 2015). Regression analysis is the most
important analysis in this case as with the help of this analysis only the significant variables can
be identified (Chatterjee and Hadi 2015). Analysis of variance (ANOVA) technique can be used
to check whether all the independent variables overall signify the dependent variable (Kleinbaum
et al.2015). The ANOVA table (Table 3) shows that the significance value is less than 0.05.
Thus, the independent variables that have been taken into consideration for this regression
analysis has an overall impact in predicting the dependent variable repurchasing intention of the
clients.
Table 3: ANOVA involving all Independent Variables
df SS MS F Significance F
Regression 13 74.616 5.740 12.685 1.08754E-19
Residual 186 84.159 0.452
Total 199 158.775
Table 5 shows the regression coefficients and the p-values of the independent variables
individually. From the table, it can be seen that the variables that mostly influence the purchasing
intention of the customers are
ï‚· Loyalty of the customers that is the length of the time the particular customer has been
buying from Furphy
 Distribution Channel that is how the Furphy’s products are sold to the customers.
Paraphrase This Document
Need a fresh take? Get an instant paraphrase of this document with our AI Paraphraser
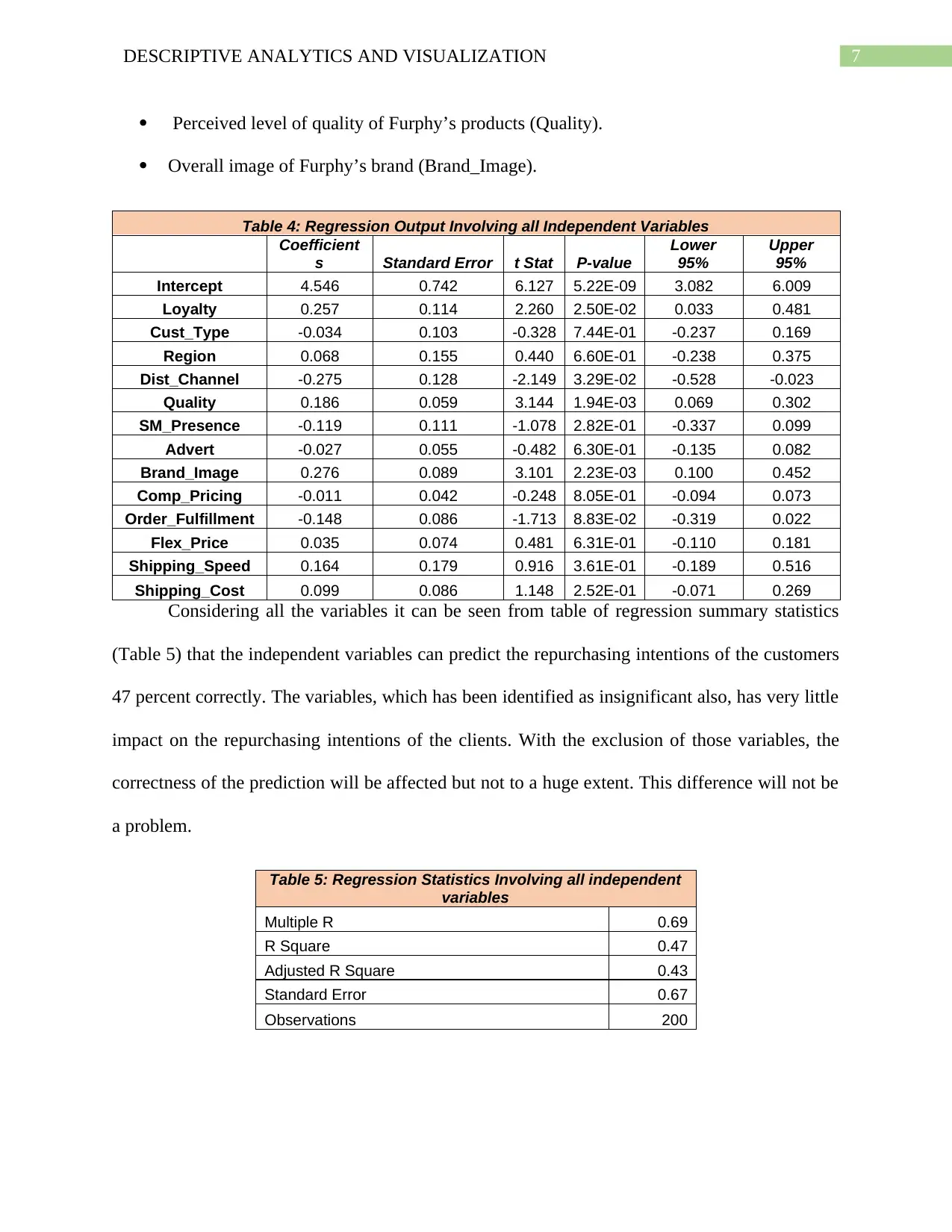
7DESCRIPTIVE ANALYTICS AND VISUALIZATION
 Perceived level of quality of Furphy’s products (Quality).
 Overall image of Furphy’s brand (Brand_Image).
Table 4: Regression Output Involving all Independent Variables
Coefficient
s Standard Error t Stat P-value
Lower
95%
Upper
95%
Intercept 4.546 0.742 6.127 5.22E-09 3.082 6.009
Loyalty 0.257 0.114 2.260 2.50E-02 0.033 0.481
Cust_Type -0.034 0.103 -0.328 7.44E-01 -0.237 0.169
Region 0.068 0.155 0.440 6.60E-01 -0.238 0.375
Dist_Channel -0.275 0.128 -2.149 3.29E-02 -0.528 -0.023
Quality 0.186 0.059 3.144 1.94E-03 0.069 0.302
SM_Presence -0.119 0.111 -1.078 2.82E-01 -0.337 0.099
Advert -0.027 0.055 -0.482 6.30E-01 -0.135 0.082
Brand_Image 0.276 0.089 3.101 2.23E-03 0.100 0.452
Comp_Pricing -0.011 0.042 -0.248 8.05E-01 -0.094 0.073
Order_Fulfillment -0.148 0.086 -1.713 8.83E-02 -0.319 0.022
Flex_Price 0.035 0.074 0.481 6.31E-01 -0.110 0.181
Shipping_Speed 0.164 0.179 0.916 3.61E-01 -0.189 0.516
Shipping_Cost 0.099 0.086 1.148 2.52E-01 -0.071 0.269
Considering all the variables it can be seen from table of regression summary statistics
(Table 5) that the independent variables can predict the repurchasing intentions of the customers
47 percent correctly. The variables, which has been identified as insignificant also, has very little
impact on the repurchasing intentions of the clients. With the exclusion of those variables, the
correctness of the prediction will be affected but not to a huge extent. This difference will not be
a problem.
Table 5: Regression Statistics Involving all independent
variables
Multiple R 0.69
R Square 0.47
Adjusted R Square 0.43
Standard Error 0.67
Observations 200
 Perceived level of quality of Furphy’s products (Quality).
 Overall image of Furphy’s brand (Brand_Image).
Table 4: Regression Output Involving all Independent Variables
Coefficient
s Standard Error t Stat P-value
Lower
95%
Upper
95%
Intercept 4.546 0.742 6.127 5.22E-09 3.082 6.009
Loyalty 0.257 0.114 2.260 2.50E-02 0.033 0.481
Cust_Type -0.034 0.103 -0.328 7.44E-01 -0.237 0.169
Region 0.068 0.155 0.440 6.60E-01 -0.238 0.375
Dist_Channel -0.275 0.128 -2.149 3.29E-02 -0.528 -0.023
Quality 0.186 0.059 3.144 1.94E-03 0.069 0.302
SM_Presence -0.119 0.111 -1.078 2.82E-01 -0.337 0.099
Advert -0.027 0.055 -0.482 6.30E-01 -0.135 0.082
Brand_Image 0.276 0.089 3.101 2.23E-03 0.100 0.452
Comp_Pricing -0.011 0.042 -0.248 8.05E-01 -0.094 0.073
Order_Fulfillment -0.148 0.086 -1.713 8.83E-02 -0.319 0.022
Flex_Price 0.035 0.074 0.481 6.31E-01 -0.110 0.181
Shipping_Speed 0.164 0.179 0.916 3.61E-01 -0.189 0.516
Shipping_Cost 0.099 0.086 1.148 2.52E-01 -0.071 0.269
Considering all the variables it can be seen from table of regression summary statistics
(Table 5) that the independent variables can predict the repurchasing intentions of the customers
47 percent correctly. The variables, which has been identified as insignificant also, has very little
impact on the repurchasing intentions of the clients. With the exclusion of those variables, the
correctness of the prediction will be affected but not to a huge extent. This difference will not be
a problem.
Table 5: Regression Statistics Involving all independent
variables
Multiple R 0.69
R Square 0.47
Adjusted R Square 0.43
Standard Error 0.67
Observations 200

8DESCRIPTIVE ANALYTICS AND VISUALIZATION
4.2.2 Prediction of Repurchasing Intention
To predict the intention to repurchase Furphy Beer, regression analysis has been done
again with the significant variables only. From the analysis, it is clear that the identified variables
can predict 44 percent (R Square value, Table 6) of the repurchasing intention of the customers
to buy the beer (Cameron and Trivedi 2013). The predicted value or rating for the repurchasing
intention can be given by the following relationship. The relationship is obtained from table 7.
Repurchasing Intention = 5.31 + (0.42 * Loyalty) – (0.29 * Distribution Channel) + (0.12 *
Quality) + (0.21 * Brand Image).
Table 6: Regression Statistics of the significant
independent variables
Multiple R 0.664
R Square 0.441
Adjusted R Square 0.430
Standard Error 0.675
Observations 200
Table 7: Regression Output Involving Significant Variables
Coefficients Standard Error t Stat P-value Lower 95% Upper 95%
Intercept 5.309 0.566 9.380 1.73E-17 4.193 6.425
Loyalty 0.416 0.076 5.483 1.29E-07 0.267 0.566
Dist_Channel -0.286 0.111 -2.566 0.011041 -0.506 -0.066
Quality 0.106 0.049 2.161 0.031911 0.009 0.203
Brand_Image 0.215 0.047 4.558 9.11E-06 0.122 0.308
4.2.3 Different Analysis
The manager of the research team has conducted a different analysis. From the analysis,
it is observed that repurchasing units significantly depend on the perception about beer quality.
Another related findings is that there is a common tendency among the customers to link brand
image with quality of the product. Therefore, these two variables should be used for a good
4.2.2 Prediction of Repurchasing Intention
To predict the intention to repurchase Furphy Beer, regression analysis has been done
again with the significant variables only. From the analysis, it is clear that the identified variables
can predict 44 percent (R Square value, Table 6) of the repurchasing intention of the customers
to buy the beer (Cameron and Trivedi 2013). The predicted value or rating for the repurchasing
intention can be given by the following relationship. The relationship is obtained from table 7.
Repurchasing Intention = 5.31 + (0.42 * Loyalty) – (0.29 * Distribution Channel) + (0.12 *
Quality) + (0.21 * Brand Image).
Table 6: Regression Statistics of the significant
independent variables
Multiple R 0.664
R Square 0.441
Adjusted R Square 0.430
Standard Error 0.675
Observations 200
Table 7: Regression Output Involving Significant Variables
Coefficients Standard Error t Stat P-value Lower 95% Upper 95%
Intercept 5.309 0.566 9.380 1.73E-17 4.193 6.425
Loyalty 0.416 0.076 5.483 1.29E-07 0.267 0.566
Dist_Channel -0.286 0.111 -2.566 0.011041 -0.506 -0.066
Quality 0.106 0.049 2.161 0.031911 0.009 0.203
Brand_Image 0.215 0.047 4.558 9.11E-06 0.122 0.308
4.2.3 Different Analysis
The manager of the research team has conducted a different analysis. From the analysis,
it is observed that repurchasing units significantly depend on the perception about beer quality.
Another related findings is that there is a common tendency among the customers to link brand
image with quality of the product. Therefore, these two variables should be used for a good

9DESCRIPTIVE ANALYTICS AND VISUALIZATION
prediction of repurchasing intention. The regression analysis shows that perception about quality
of beer and brand image explained 34% variation in repurchasing intention (Table 8). As
explanatory variables both quality and brand image are statistically significant. There P values
are less than 0.05 (Table 9). The estimated relationship of repurchasing intention with that of
quality and brand image are given as-
Repurchasing Intentions = 3.59 + (0.31 * Quality) + (0.31 * Brand Image)
Table 8: Regression Statistics of Manager Identified
Independent Variables
Multiple R 0.584
R Square 0.341
Adjusted R Square 0.334
Standard Error 0.729
Observations 200
Table 9: Regression Output Involving Manager Identified Variables
Coefficient
s
Standard
Error t Stat P-value Lower 95% Upper 95%
Intercept
3.58749267
7 0.407503955
8.80357757
6
6.78306E-
16 2.78386269
4.39112266
3
Quality
0.30941394
9 0.037618814
8.22497879
1
2.60564E-
14
0.23522667
6
0.38360122
2
Brand_Imag
e
0.31154603
8 0.046100345 6.75799795
1.54395E-
10
0.22063251
6 0.40245956
4.3 Furphy Recommendation
Next agenda of the tram manager is to recommend Furphy. There are clients who are
neutral about delivery speed of Furphy depending on the quality and images of the brand. There
are also clients who make purchase either directly or through sales representatives. Special
attention has been given to these groups of clients.
prediction of repurchasing intention. The regression analysis shows that perception about quality
of beer and brand image explained 34% variation in repurchasing intention (Table 8). As
explanatory variables both quality and brand image are statistically significant. There P values
are less than 0.05 (Table 9). The estimated relationship of repurchasing intention with that of
quality and brand image are given as-
Repurchasing Intentions = 3.59 + (0.31 * Quality) + (0.31 * Brand Image)
Table 8: Regression Statistics of Manager Identified
Independent Variables
Multiple R 0.584
R Square 0.341
Adjusted R Square 0.334
Standard Error 0.729
Observations 200
Table 9: Regression Output Involving Manager Identified Variables
Coefficient
s
Standard
Error t Stat P-value Lower 95% Upper 95%
Intercept
3.58749267
7 0.407503955
8.80357757
6
6.78306E-
16 2.78386269
4.39112266
3
Quality
0.30941394
9 0.037618814
8.22497879
1
2.60564E-
14
0.23522667
6
0.38360122
2
Brand_Imag
e
0.31154603
8 0.046100345 6.75799795
1.54395E-
10
0.22063251
6 0.40245956
4.3 Furphy Recommendation
Next agenda of the tram manager is to recommend Furphy. There are clients who are
neutral about delivery speed of Furphy depending on the quality and images of the brand. There
are also clients who make purchase either directly or through sales representatives. Special
attention has been given to these groups of clients.
Secure Best Marks with AI Grader
Need help grading? Try our AI Grader for instant feedback on your assignments.

10DESCRIPTIVE ANALYTICS AND VISUALIZATION
An important determinant of this is the ratings given by clients. To make the analysis
simple, ratings are rounded off to get the nearest whole number. Clients who have given a 5
ratings are collected to confirm the likely for Furphy recommendation. The likely hood of
recommendation is 29 percent for the clients who are neutral about Furphy’s delivery speed as
shown from table 12.
Perception of clients varied with different levels of product quality. With this varying
level, the likely hood for recommending Furphy to other clients is 0.505, as stated in table 14.
From the table it is seen that the lowest recorded rating is 6. Therefore, from the rating statistics
it is clearly evident that the product delivered by Furphy is quite satisfactory and is of a good
quality product.
The likely hood for different brand images is represented in tale 16. The brand images are
categorized in three groups positive brand image, neutral brand image and negative brand image.
1 percent of the clients having neutral brand preference recommend Furphy’s product. Clients
with positive and negative brand image constitutes likely hood of 31% and 18.5% respectively.
These are the recommendation statistics for clients influencing others toward making purchase of
Furphy’s beer brand.
The tendency for recommendation by customers who directly purchase the product or
purchase with intermediation by the sales representatives is shown in table 18. It is seen that
those who directly purchase the product have a higher tendency for recommendation as
compared to those purchase the product with sales representative. Percentage of customers
recommending Furphy to others in directly purchasing group is 34% while that for buyers with
sales representative is 16.5 percent.
An important determinant of this is the ratings given by clients. To make the analysis
simple, ratings are rounded off to get the nearest whole number. Clients who have given a 5
ratings are collected to confirm the likely for Furphy recommendation. The likely hood of
recommendation is 29 percent for the clients who are neutral about Furphy’s delivery speed as
shown from table 12.
Perception of clients varied with different levels of product quality. With this varying
level, the likely hood for recommending Furphy to other clients is 0.505, as stated in table 14.
From the table it is seen that the lowest recorded rating is 6. Therefore, from the rating statistics
it is clearly evident that the product delivered by Furphy is quite satisfactory and is of a good
quality product.
The likely hood for different brand images is represented in tale 16. The brand images are
categorized in three groups positive brand image, neutral brand image and negative brand image.
1 percent of the clients having neutral brand preference recommend Furphy’s product. Clients
with positive and negative brand image constitutes likely hood of 31% and 18.5% respectively.
These are the recommendation statistics for clients influencing others toward making purchase of
Furphy’s beer brand.
The tendency for recommendation by customers who directly purchase the product or
purchase with intermediation by the sales representatives is shown in table 18. It is seen that
those who directly purchase the product have a higher tendency for recommendation as
compared to those purchase the product with sales representative. Percentage of customers
recommending Furphy to others in directly purchasing group is 34% while that for buyers with
sales representative is 16.5 percent.

11DESCRIPTIVE ANALYTICS AND VISUALIZATION
Furphy’s beer brand is quite popular in the state. Mixed responses are obtained from the
clients purchasing the products. There are both positive and negative ratings obtained from the
clients. Despite negative and neutral return from customers, there is an overall good image for
the product. Recommendations come from all the groups of customers. The Customers
recommend the product to others irrespective of their ratings
4.4 Analysis based on Time Series
A time series analysis is made for foresting sales of Furphy in the next quarter. Using
sales data until 2017, prediction is made for the tear 2018. Quarterly moving average method
(Granger and Newbold 2014) has been used to predict quarterly sales in 2018. When past data
points are available then moving average methods are suitable for making forecast (Brockwell
and Davis 2016). The trend obtained from the data is likely to depict a clear trend for forecast
(Box et al. 2015). Moreover, moving average method is widely used because of simplicity in
calculation and easy interpretation (Montgomery, Jennings and Kulahci 2015). The predicted
sale in first quarter of 2018 is 1699.40 litres per ale. An increase in quarterly sales prediction is
found for the next quarter. In the second quarter of 2018, the expected sale is 1714 litres per ale.
This trend declines in the third and fourth quarter of 2018. Forecasted sales for the third and
fourth quarter of 2018 are 1656.66 litres per ale and 1688.71 litres per ale.
5. Recommendations and Conclusion
The paper summarizes sales and ratings of the Furohy product with reference to
repurchase unit. Most people using the product have given high rating for the product. The
significant variables affecting repurchase units are distribution channel, loyalty of the customers
towards product, brand image and perceived product quality. The adjusted R square for the
regression analysis is 0.44. This implies the explanatory variables taken in the regression
Furphy’s beer brand is quite popular in the state. Mixed responses are obtained from the
clients purchasing the products. There are both positive and negative ratings obtained from the
clients. Despite negative and neutral return from customers, there is an overall good image for
the product. Recommendations come from all the groups of customers. The Customers
recommend the product to others irrespective of their ratings
4.4 Analysis based on Time Series
A time series analysis is made for foresting sales of Furphy in the next quarter. Using
sales data until 2017, prediction is made for the tear 2018. Quarterly moving average method
(Granger and Newbold 2014) has been used to predict quarterly sales in 2018. When past data
points are available then moving average methods are suitable for making forecast (Brockwell
and Davis 2016). The trend obtained from the data is likely to depict a clear trend for forecast
(Box et al. 2015). Moreover, moving average method is widely used because of simplicity in
calculation and easy interpretation (Montgomery, Jennings and Kulahci 2015). The predicted
sale in first quarter of 2018 is 1699.40 litres per ale. An increase in quarterly sales prediction is
found for the next quarter. In the second quarter of 2018, the expected sale is 1714 litres per ale.
This trend declines in the third and fourth quarter of 2018. Forecasted sales for the third and
fourth quarter of 2018 are 1656.66 litres per ale and 1688.71 litres per ale.
5. Recommendations and Conclusion
The paper summarizes sales and ratings of the Furohy product with reference to
repurchase unit. Most people using the product have given high rating for the product. The
significant variables affecting repurchase units are distribution channel, loyalty of the customers
towards product, brand image and perceived product quality. The adjusted R square for the
regression analysis is 0.44. This implies the explanatory variables taken in the regression

12DESCRIPTIVE ANALYTICS AND VISUALIZATION
analysis explained nearly 44% variation in repurchase unit. Again, brand quality and image
predicts 34% of the repurchasing intensity. As per recommendation is concerned, people with
positive ratings recommends the product most. Finally, forecast is made for future sales.
Predicted sales is higher for first two quarters of 2018 while for later two quarters sales is
predicted to be declined slightly.
Furphy should consider some policy changes to increase its sales and improve ratings.
For repurchasing units the significant variables are distribution channel, brand image and quality
and customer loyalty. Improvement of these aspects can lead to an improvement in sales of the
concerned company. Once the company improves distribution channels or customer loyalty those
giving a neutral rating or negative ratings currently might give a positive response. With increase
in positive responses, more people will recommend the product to others. This will further
enhance sales.
analysis explained nearly 44% variation in repurchase unit. Again, brand quality and image
predicts 34% of the repurchasing intensity. As per recommendation is concerned, people with
positive ratings recommends the product most. Finally, forecast is made for future sales.
Predicted sales is higher for first two quarters of 2018 while for later two quarters sales is
predicted to be declined slightly.
Furphy should consider some policy changes to increase its sales and improve ratings.
For repurchasing units the significant variables are distribution channel, brand image and quality
and customer loyalty. Improvement of these aspects can lead to an improvement in sales of the
concerned company. Once the company improves distribution channels or customer loyalty those
giving a neutral rating or negative ratings currently might give a positive response. With increase
in positive responses, more people will recommend the product to others. This will further
enhance sales.
Paraphrase This Document
Need a fresh take? Get an instant paraphrase of this document with our AI Paraphraser

13DESCRIPTIVE ANALYTICS AND VISUALIZATION
REFERENCES
Box, G.E., Jenkins, G.M., Reinsel, G.C. and Ljung, G.M., 2015. Time series analysis:
forecasting and control. John Wiley & Sons.
Brockwell, P.J. and Davis, R.A., 2013. Time series: theory and methods. Springer Science &
Business Media.
Cameron, A.C. and Trivedi, P.K., 2013. Regression analysis of count data (Vol. 53). Cambridge
university press.
Chatfield, C., 2016. The analysis of time series: an introduction. CRC press.
Chatterjee, S. and Hadi, A.S., 2015. Regression analysis by example. John Wiley & Sons.
Draper, N.R. and Smith, H., 2014. Applied regression analysis. John Wiley & Sons.
Granger, C.W.J. and Newbold, P., 2014. Forecasting economic time series. Academic Press.
Kleinbaum, D., Kupper, L., Nizam, A. and Rosenberg, E., 2013. Applied regression analysis and
other multivariable methods. Nelson Education.
Montgomery, D.C., Jennings, C.L. and Kulahci, M., 2015. Introduction to time series analysis
and forecasting. John Wiley & Sons.
Montgomery, D.C., Peck, E.A. and Vining, G.G., 2015. Introduction to linear regression
analysis. John Wiley & Sons.
Nelson, E., 2016. Radically Elementary Probability Theory.(AM-117) (Vol. 117). Princeton
University Press.
REFERENCES
Box, G.E., Jenkins, G.M., Reinsel, G.C. and Ljung, G.M., 2015. Time series analysis:
forecasting and control. John Wiley & Sons.
Brockwell, P.J. and Davis, R.A., 2013. Time series: theory and methods. Springer Science &
Business Media.
Cameron, A.C. and Trivedi, P.K., 2013. Regression analysis of count data (Vol. 53). Cambridge
university press.
Chatfield, C., 2016. The analysis of time series: an introduction. CRC press.
Chatterjee, S. and Hadi, A.S., 2015. Regression analysis by example. John Wiley & Sons.
Draper, N.R. and Smith, H., 2014. Applied regression analysis. John Wiley & Sons.
Granger, C.W.J. and Newbold, P., 2014. Forecasting economic time series. Academic Press.
Kleinbaum, D., Kupper, L., Nizam, A. and Rosenberg, E., 2013. Applied regression analysis and
other multivariable methods. Nelson Education.
Montgomery, D.C., Jennings, C.L. and Kulahci, M., 2015. Introduction to time series analysis
and forecasting. John Wiley & Sons.
Montgomery, D.C., Peck, E.A. and Vining, G.G., 2015. Introduction to linear regression
analysis. John Wiley & Sons.
Nelson, E., 2016. Radically Elementary Probability Theory.(AM-117) (Vol. 117). Princeton
University Press.

14DESCRIPTIVE ANALYTICS AND VISUALIZATION
APPENDICES
Table 10: ANOVA of the Significant Variables
df SS MS F Significance F
Regression 4 70.02649616 17.50662 38.46591 1.03018E-23
Residual 195 88.74850384 0.455121
Total 199 158.775
Table 11: ANOVA of the Manager Identified Variables
df SS MS F Significance F
Regression 2 54.089 27.045 50.893 1.52046E-18
Residual 197 104.686 0.531
Total 199 158.775
Table 12: Neutral Shipping Speed
Recommendation Frequency Probability
Yes 58 0.29
No 26 0.13
Table 13
Recommendation Quality Total
6 7 8 9 10
Yes 11 8 12 22 48 101
No 11 30 24 22 12 99
Total 22 38 36 44 60 200
Table 14
Recommendation Marginal Probability of Quality Total
6 7 8 9 10
Yes 0.055 0.04 0.06 0.11 0.24 0.505
No 0.055 0.15 0.12 0.11 0.06 0.495
Total 0.11 0.19 0.18 0.22 0.3 1
Table 15
APPENDICES
Table 10: ANOVA of the Significant Variables
df SS MS F Significance F
Regression 4 70.02649616 17.50662 38.46591 1.03018E-23
Residual 195 88.74850384 0.455121
Total 199 158.775
Table 11: ANOVA of the Manager Identified Variables
df SS MS F Significance F
Regression 2 54.089 27.045 50.893 1.52046E-18
Residual 197 104.686 0.531
Total 199 158.775
Table 12: Neutral Shipping Speed
Recommendation Frequency Probability
Yes 58 0.29
No 26 0.13
Table 13
Recommendation Quality Total
6 7 8 9 10
Yes 11 8 12 22 48 101
No 11 30 24 22 12 99
Total 22 38 36 44 60 200
Table 14
Recommendation Marginal Probability of Quality Total
6 7 8 9 10
Yes 0.055 0.04 0.06 0.11 0.24 0.505
No 0.055 0.15 0.12 0.11 0.06 0.495
Total 0.11 0.19 0.18 0.22 0.3 1
Table 15
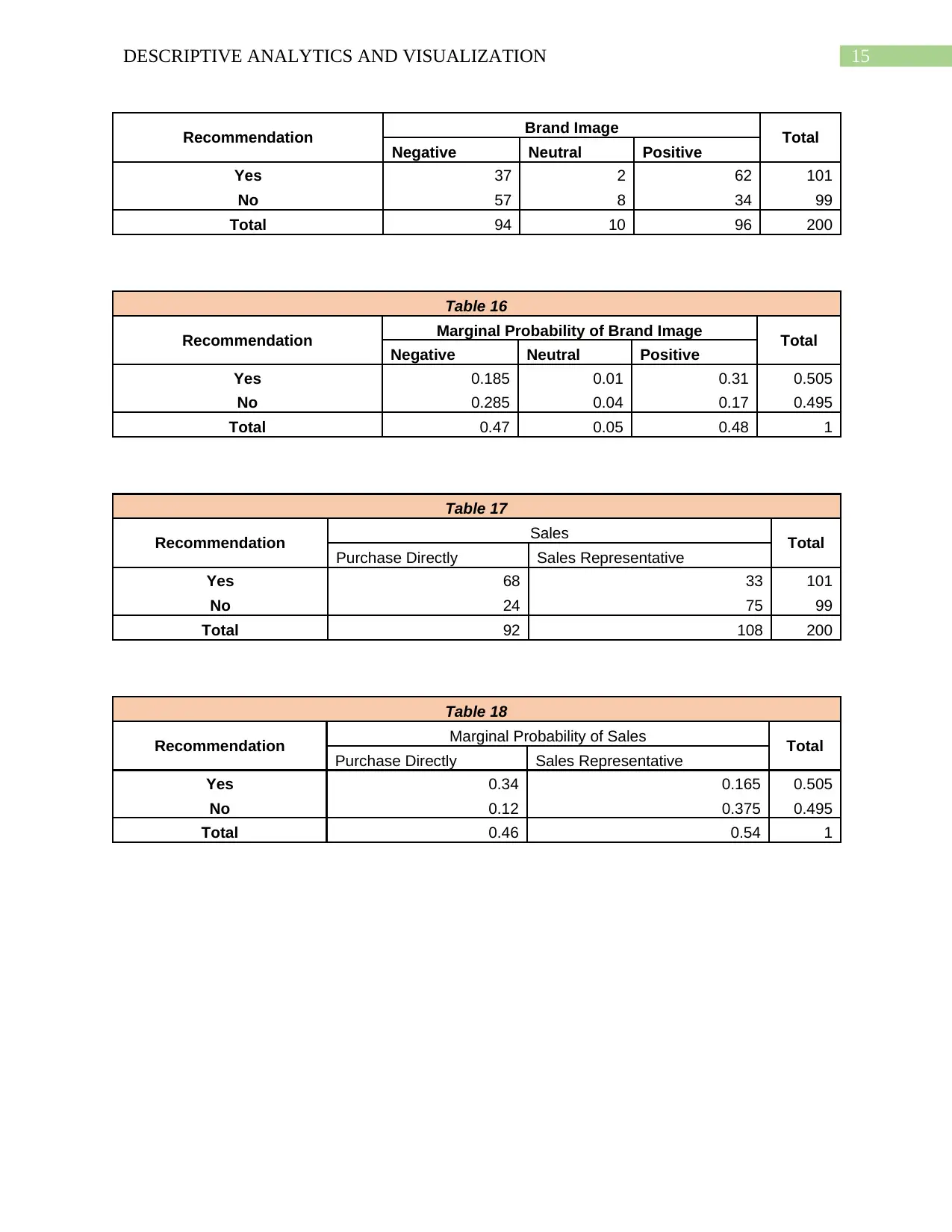
15DESCRIPTIVE ANALYTICS AND VISUALIZATION
Recommendation Brand Image Total
Negative Neutral Positive
Yes 37 2 62 101
No 57 8 34 99
Total 94 10 96 200
Table 16
Recommendation Marginal Probability of Brand Image Total
Negative Neutral Positive
Yes 0.185 0.01 0.31 0.505
No 0.285 0.04 0.17 0.495
Total 0.47 0.05 0.48 1
Table 17
Recommendation Sales Total
Purchase Directly Sales Representative
Yes 68 33 101
No 24 75 99
Total 92 108 200
Table 18
Recommendation Marginal Probability of Sales Total
Purchase Directly Sales Representative
Yes 0.34 0.165 0.505
No 0.12 0.375 0.495
Total 0.46 0.54 1
Recommendation Brand Image Total
Negative Neutral Positive
Yes 37 2 62 101
No 57 8 34 99
Total 94 10 96 200
Table 16
Recommendation Marginal Probability of Brand Image Total
Negative Neutral Positive
Yes 0.185 0.01 0.31 0.505
No 0.285 0.04 0.17 0.495
Total 0.47 0.05 0.48 1
Table 17
Recommendation Sales Total
Purchase Directly Sales Representative
Yes 68 33 101
No 24 75 99
Total 92 108 200
Table 18
Recommendation Marginal Probability of Sales Total
Purchase Directly Sales Representative
Yes 0.34 0.165 0.505
No 0.12 0.375 0.495
Total 0.46 0.54 1
1 out of 16
Related Documents
![[object Object]](/_next/image/?url=%2F_next%2Fstatic%2Fmedia%2Flogo.6d15ce61.png&w=640&q=75)
Your All-in-One AI-Powered Toolkit for Academic Success.
 +13062052269
info@desklib.com
Available 24*7 on WhatsApp / Email
Unlock your academic potential
© 2024  |  Zucol Services PVT LTD  |  All rights reserved.