(PDF) An introduction to statistical modelling
VerifiedAdded on 2021/05/31
|12
|2406
|48
AI Summary
Contribute Materials
Your contribution can guide someone’s learning journey. Share your
documents today.
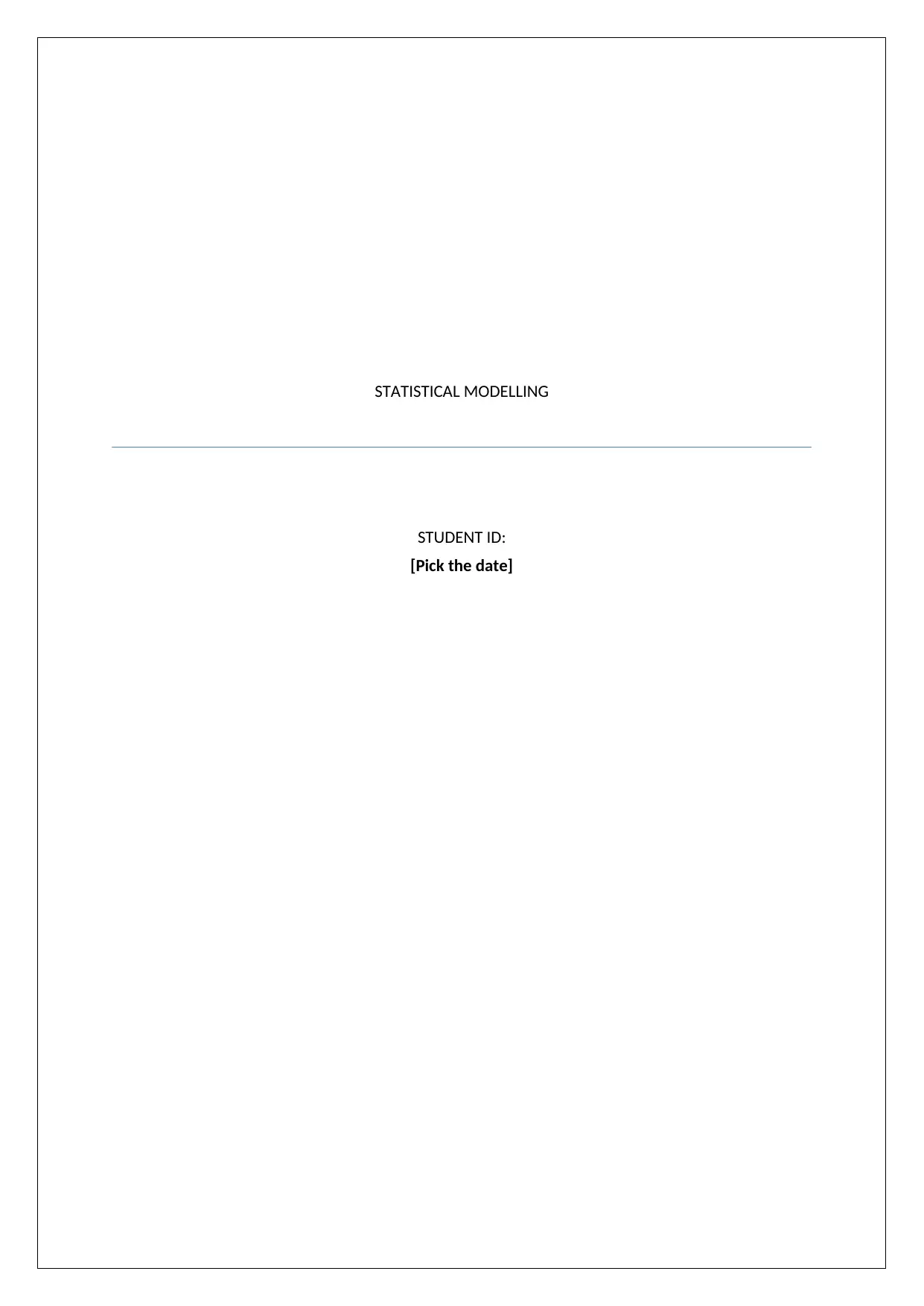
STATISTICAL MODELLING
STUDENT ID:
[Pick the date]
STUDENT ID:
[Pick the date]
Secure Best Marks with AI Grader
Need help grading? Try our AI Grader for instant feedback on your assignments.
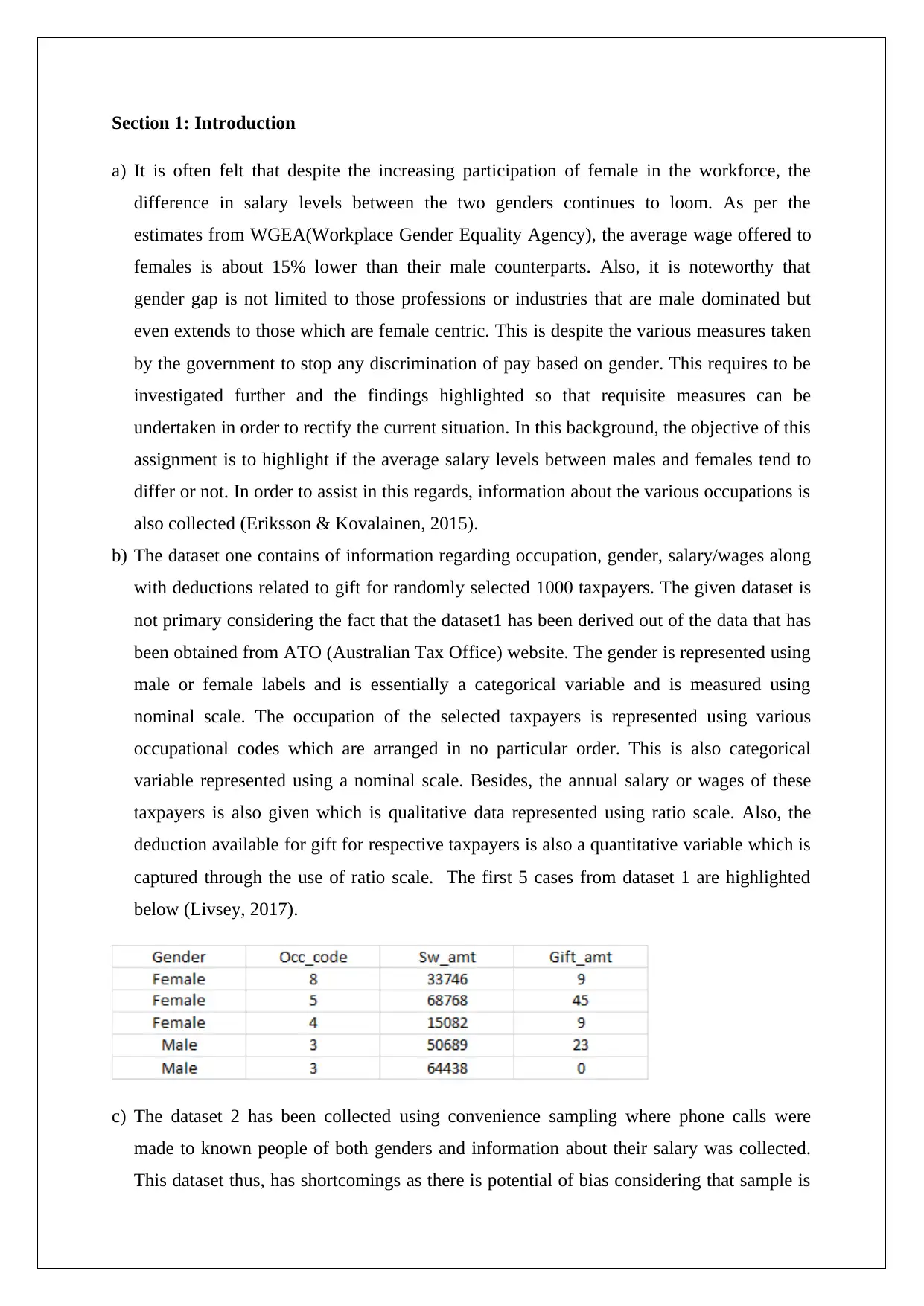
Section 1: Introduction
a) It is often felt that despite the increasing participation of female in the workforce, the
difference in salary levels between the two genders continues to loom. As per the
estimates from WGEA(Workplace Gender Equality Agency), the average wage offered to
females is about 15% lower than their male counterparts. Also, it is noteworthy that
gender gap is not limited to those professions or industries that are male dominated but
even extends to those which are female centric. This is despite the various measures taken
by the government to stop any discrimination of pay based on gender. This requires to be
investigated further and the findings highlighted so that requisite measures can be
undertaken in order to rectify the current situation. In this background, the objective of this
assignment is to highlight if the average salary levels between males and females tend to
differ or not. In order to assist in this regards, information about the various occupations is
also collected (Eriksson & Kovalainen, 2015).
b) The dataset one contains of information regarding occupation, gender, salary/wages along
with deductions related to gift for randomly selected 1000 taxpayers. The given dataset is
not primary considering the fact that the dataset1 has been derived out of the data that has
been obtained from ATO (Australian Tax Office) website. The gender is represented using
male or female labels and is essentially a categorical variable and is measured using
nominal scale. The occupation of the selected taxpayers is represented using various
occupational codes which are arranged in no particular order. This is also categorical
variable represented using a nominal scale. Besides, the annual salary or wages of these
taxpayers is also given which is qualitative data represented using ratio scale. Also, the
deduction available for gift for respective taxpayers is also a quantitative variable which is
captured through the use of ratio scale. The first 5 cases from dataset 1 are highlighted
below (Livsey, 2017).
c) The dataset 2 has been collected using convenience sampling where phone calls were
made to known people of both genders and information about their salary was collected.
This dataset thus, has shortcomings as there is potential of bias considering that sample is
a) It is often felt that despite the increasing participation of female in the workforce, the
difference in salary levels between the two genders continues to loom. As per the
estimates from WGEA(Workplace Gender Equality Agency), the average wage offered to
females is about 15% lower than their male counterparts. Also, it is noteworthy that
gender gap is not limited to those professions or industries that are male dominated but
even extends to those which are female centric. This is despite the various measures taken
by the government to stop any discrimination of pay based on gender. This requires to be
investigated further and the findings highlighted so that requisite measures can be
undertaken in order to rectify the current situation. In this background, the objective of this
assignment is to highlight if the average salary levels between males and females tend to
differ or not. In order to assist in this regards, information about the various occupations is
also collected (Eriksson & Kovalainen, 2015).
b) The dataset one contains of information regarding occupation, gender, salary/wages along
with deductions related to gift for randomly selected 1000 taxpayers. The given dataset is
not primary considering the fact that the dataset1 has been derived out of the data that has
been obtained from ATO (Australian Tax Office) website. The gender is represented using
male or female labels and is essentially a categorical variable and is measured using
nominal scale. The occupation of the selected taxpayers is represented using various
occupational codes which are arranged in no particular order. This is also categorical
variable represented using a nominal scale. Besides, the annual salary or wages of these
taxpayers is also given which is qualitative data represented using ratio scale. Also, the
deduction available for gift for respective taxpayers is also a quantitative variable which is
captured through the use of ratio scale. The first 5 cases from dataset 1 are highlighted
below (Livsey, 2017).
c) The dataset 2 has been collected using convenience sampling where phone calls were
made to known people of both genders and information about their salary was collected.
This dataset thus, has shortcomings as there is potential of bias considering that sample is
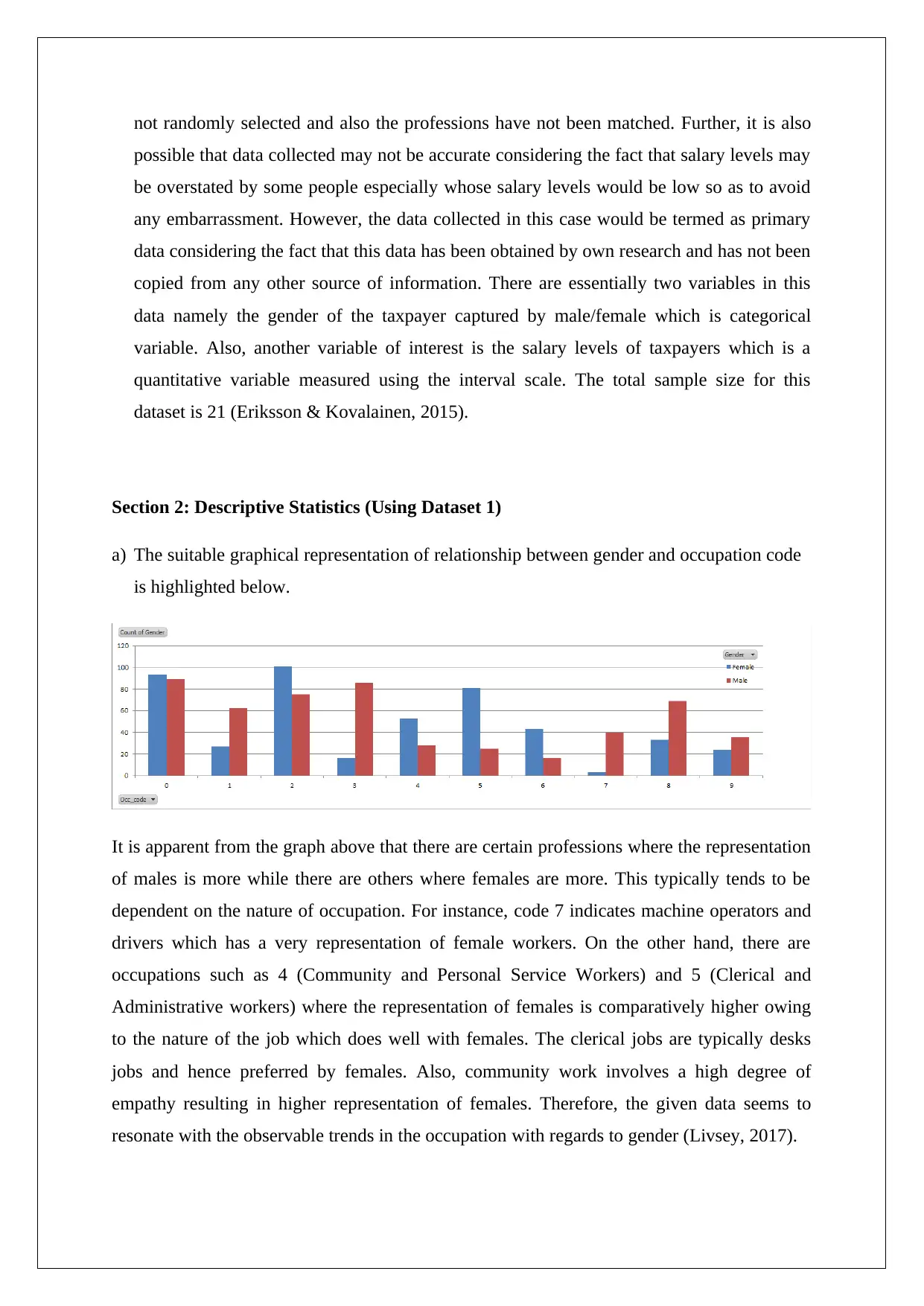
not randomly selected and also the professions have not been matched. Further, it is also
possible that data collected may not be accurate considering the fact that salary levels may
be overstated by some people especially whose salary levels would be low so as to avoid
any embarrassment. However, the data collected in this case would be termed as primary
data considering the fact that this data has been obtained by own research and has not been
copied from any other source of information. There are essentially two variables in this
data namely the gender of the taxpayer captured by male/female which is categorical
variable. Also, another variable of interest is the salary levels of taxpayers which is a
quantitative variable measured using the interval scale. The total sample size for this
dataset is 21 (Eriksson & Kovalainen, 2015).
Section 2: Descriptive Statistics (Using Dataset 1)
a) The suitable graphical representation of relationship between gender and occupation code
is highlighted below.
It is apparent from the graph above that there are certain professions where the representation
of males is more while there are others where females are more. This typically tends to be
dependent on the nature of occupation. For instance, code 7 indicates machine operators and
drivers which has a very representation of female workers. On the other hand, there are
occupations such as 4 (Community and Personal Service Workers) and 5 (Clerical and
Administrative workers) where the representation of females is comparatively higher owing
to the nature of the job which does well with females. The clerical jobs are typically desks
jobs and hence preferred by females. Also, community work involves a high degree of
empathy resulting in higher representation of females. Therefore, the given data seems to
resonate with the observable trends in the occupation with regards to gender (Livsey, 2017).
possible that data collected may not be accurate considering the fact that salary levels may
be overstated by some people especially whose salary levels would be low so as to avoid
any embarrassment. However, the data collected in this case would be termed as primary
data considering the fact that this data has been obtained by own research and has not been
copied from any other source of information. There are essentially two variables in this
data namely the gender of the taxpayer captured by male/female which is categorical
variable. Also, another variable of interest is the salary levels of taxpayers which is a
quantitative variable measured using the interval scale. The total sample size for this
dataset is 21 (Eriksson & Kovalainen, 2015).
Section 2: Descriptive Statistics (Using Dataset 1)
a) The suitable graphical representation of relationship between gender and occupation code
is highlighted below.
It is apparent from the graph above that there are certain professions where the representation
of males is more while there are others where females are more. This typically tends to be
dependent on the nature of occupation. For instance, code 7 indicates machine operators and
drivers which has a very representation of female workers. On the other hand, there are
occupations such as 4 (Community and Personal Service Workers) and 5 (Clerical and
Administrative workers) where the representation of females is comparatively higher owing
to the nature of the job which does well with females. The clerical jobs are typically desks
jobs and hence preferred by females. Also, community work involves a high degree of
empathy resulting in higher representation of females. Therefore, the given data seems to
resonate with the observable trends in the occupation with regards to gender (Livsey, 2017).
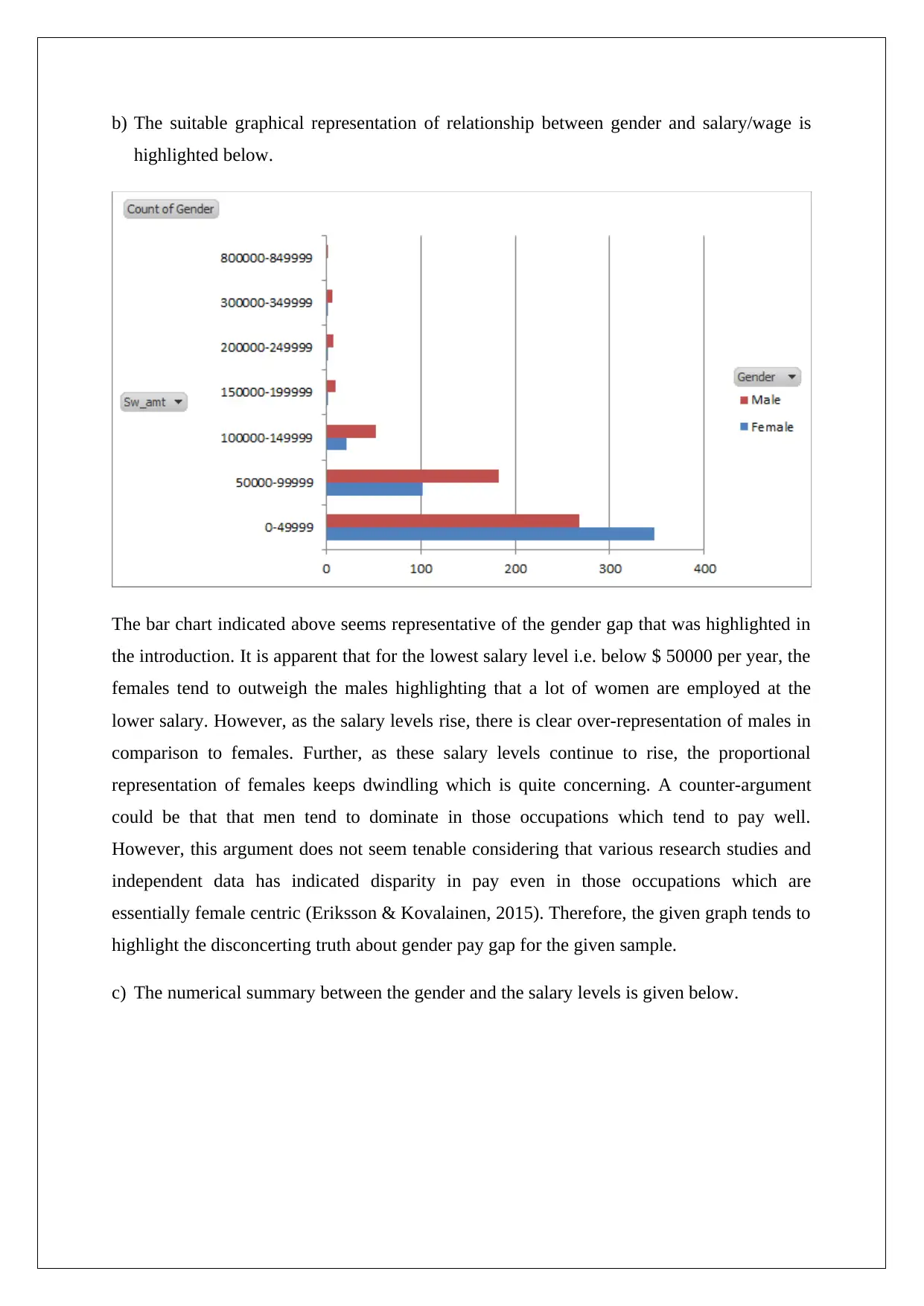
b) The suitable graphical representation of relationship between gender and salary/wage is
highlighted below.
The bar chart indicated above seems representative of the gender gap that was highlighted in
the introduction. It is apparent that for the lowest salary level i.e. below $ 50000 per year, the
females tend to outweigh the males highlighting that a lot of women are employed at the
lower salary. However, as the salary levels rise, there is clear over-representation of males in
comparison to females. Further, as these salary levels continue to rise, the proportional
representation of females keeps dwindling which is quite concerning. A counter-argument
could be that that men tend to dominate in those occupations which tend to pay well.
However, this argument does not seem tenable considering that various research studies and
independent data has indicated disparity in pay even in those occupations which are
essentially female centric (Eriksson & Kovalainen, 2015). Therefore, the given graph tends to
highlight the disconcerting truth about gender pay gap for the given sample.
c) The numerical summary between the gender and the salary levels is given below.
highlighted below.
The bar chart indicated above seems representative of the gender gap that was highlighted in
the introduction. It is apparent that for the lowest salary level i.e. below $ 50000 per year, the
females tend to outweigh the males highlighting that a lot of women are employed at the
lower salary. However, as the salary levels rise, there is clear over-representation of males in
comparison to females. Further, as these salary levels continue to rise, the proportional
representation of females keeps dwindling which is quite concerning. A counter-argument
could be that that men tend to dominate in those occupations which tend to pay well.
However, this argument does not seem tenable considering that various research studies and
independent data has indicated disparity in pay even in those occupations which are
essentially female centric (Eriksson & Kovalainen, 2015). Therefore, the given graph tends to
highlight the disconcerting truth about gender pay gap for the given sample.
c) The numerical summary between the gender and the salary levels is given below.
Paraphrase This Document
Need a fresh take? Get an instant paraphrase of this document with our AI Paraphraser
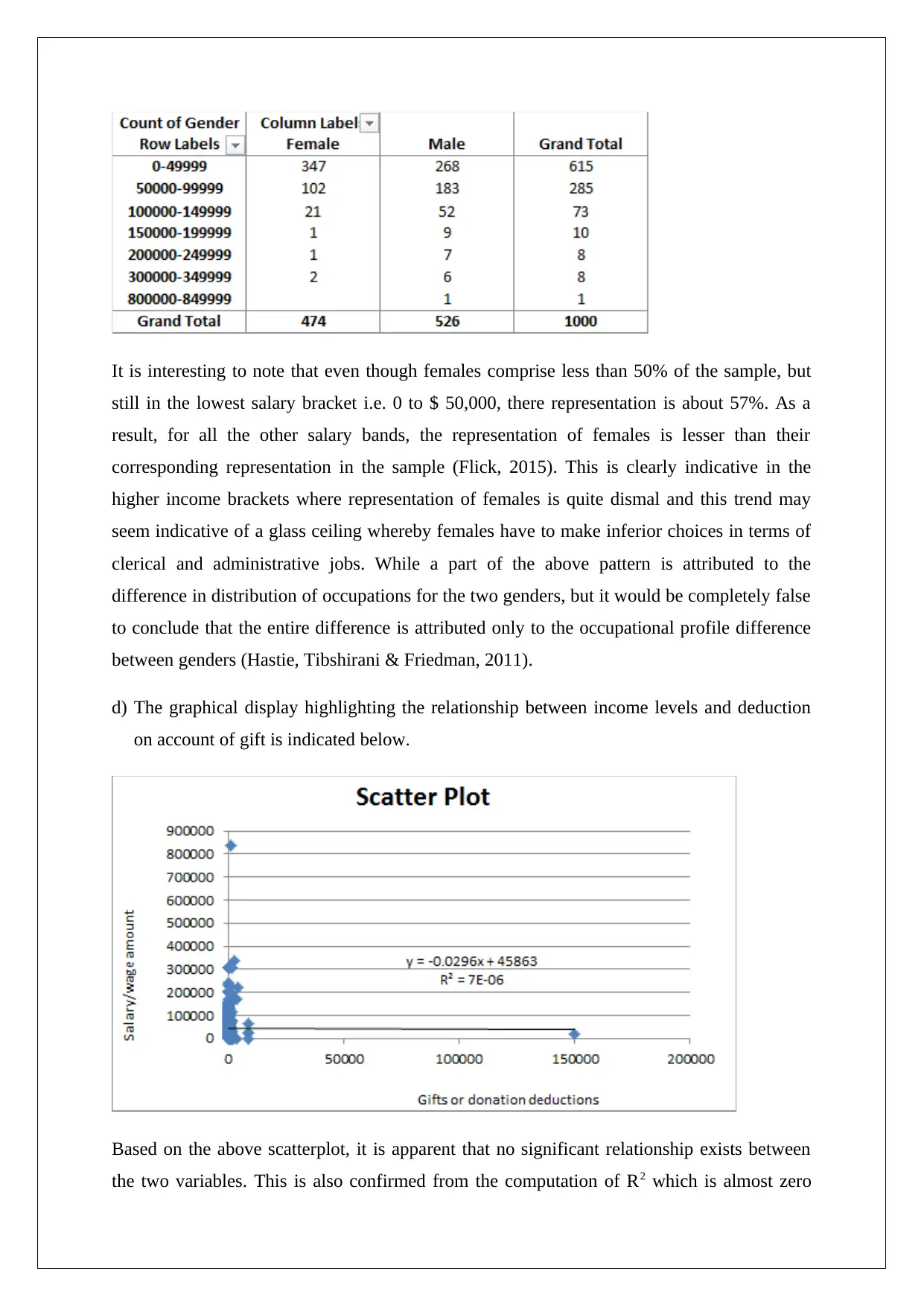
It is interesting to note that even though females comprise less than 50% of the sample, but
still in the lowest salary bracket i.e. 0 to $ 50,000, there representation is about 57%. As a
result, for all the other salary bands, the representation of females is lesser than their
corresponding representation in the sample (Flick, 2015). This is clearly indicative in the
higher income brackets where representation of females is quite dismal and this trend may
seem indicative of a glass ceiling whereby females have to make inferior choices in terms of
clerical and administrative jobs. While a part of the above pattern is attributed to the
difference in distribution of occupations for the two genders, but it would be completely false
to conclude that the entire difference is attributed only to the occupational profile difference
between genders (Hastie, Tibshirani & Friedman, 2011).
d) The graphical display highlighting the relationship between income levels and deduction
on account of gift is indicated below.
Based on the above scatterplot, it is apparent that no significant relationship exists between
the two variables. This is also confirmed from the computation of R2 which is almost zero
still in the lowest salary bracket i.e. 0 to $ 50,000, there representation is about 57%. As a
result, for all the other salary bands, the representation of females is lesser than their
corresponding representation in the sample (Flick, 2015). This is clearly indicative in the
higher income brackets where representation of females is quite dismal and this trend may
seem indicative of a glass ceiling whereby females have to make inferior choices in terms of
clerical and administrative jobs. While a part of the above pattern is attributed to the
difference in distribution of occupations for the two genders, but it would be completely false
to conclude that the entire difference is attributed only to the occupational profile difference
between genders (Hastie, Tibshirani & Friedman, 2011).
d) The graphical display highlighting the relationship between income levels and deduction
on account of gift is indicated below.
Based on the above scatterplot, it is apparent that no significant relationship exists between
the two variables. This is also confirmed from the computation of R2 which is almost zero
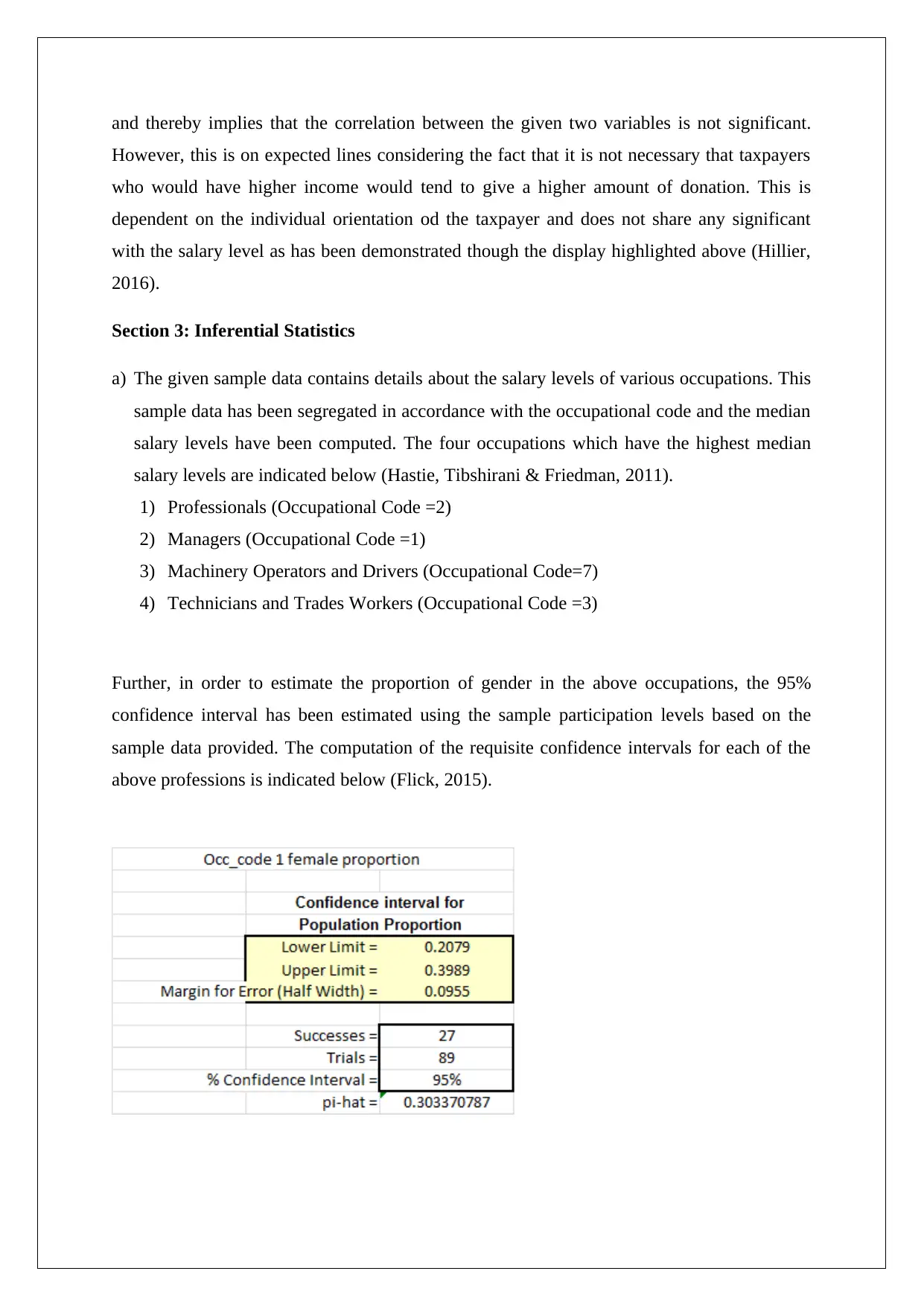
and thereby implies that the correlation between the given two variables is not significant.
However, this is on expected lines considering the fact that it is not necessary that taxpayers
who would have higher income would tend to give a higher amount of donation. This is
dependent on the individual orientation od the taxpayer and does not share any significant
with the salary level as has been demonstrated though the display highlighted above (Hillier,
2016).
Section 3: Inferential Statistics
a) The given sample data contains details about the salary levels of various occupations. This
sample data has been segregated in accordance with the occupational code and the median
salary levels have been computed. The four occupations which have the highest median
salary levels are indicated below (Hastie, Tibshirani & Friedman, 2011).
1) Professionals (Occupational Code =2)
2) Managers (Occupational Code =1)
3) Machinery Operators and Drivers (Occupational Code=7)
4) Technicians and Trades Workers (Occupational Code =3)
Further, in order to estimate the proportion of gender in the above occupations, the 95%
confidence interval has been estimated using the sample participation levels based on the
sample data provided. The computation of the requisite confidence intervals for each of the
above professions is indicated below (Flick, 2015).
However, this is on expected lines considering the fact that it is not necessary that taxpayers
who would have higher income would tend to give a higher amount of donation. This is
dependent on the individual orientation od the taxpayer and does not share any significant
with the salary level as has been demonstrated though the display highlighted above (Hillier,
2016).
Section 3: Inferential Statistics
a) The given sample data contains details about the salary levels of various occupations. This
sample data has been segregated in accordance with the occupational code and the median
salary levels have been computed. The four occupations which have the highest median
salary levels are indicated below (Hastie, Tibshirani & Friedman, 2011).
1) Professionals (Occupational Code =2)
2) Managers (Occupational Code =1)
3) Machinery Operators and Drivers (Occupational Code=7)
4) Technicians and Trades Workers (Occupational Code =3)
Further, in order to estimate the proportion of gender in the above occupations, the 95%
confidence interval has been estimated using the sample participation levels based on the
sample data provided. The computation of the requisite confidence intervals for each of the
above professions is indicated below (Flick, 2015).
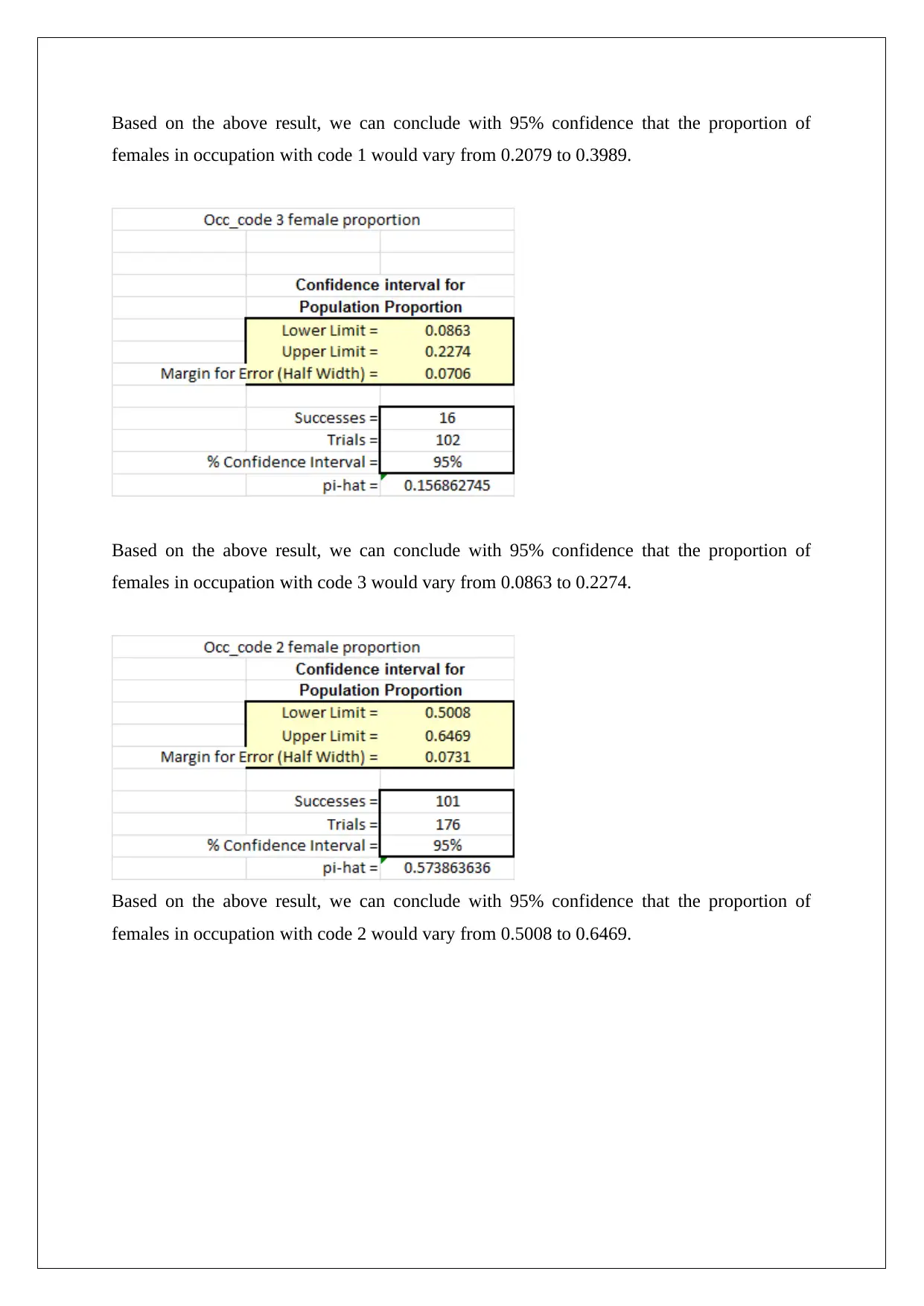
Based on the above result, we can conclude with 95% confidence that the proportion of
females in occupation with code 1 would vary from 0.2079 to 0.3989.
Based on the above result, we can conclude with 95% confidence that the proportion of
females in occupation with code 3 would vary from 0.0863 to 0.2274.
Based on the above result, we can conclude with 95% confidence that the proportion of
females in occupation with code 2 would vary from 0.5008 to 0.6469.
females in occupation with code 1 would vary from 0.2079 to 0.3989.
Based on the above result, we can conclude with 95% confidence that the proportion of
females in occupation with code 3 would vary from 0.0863 to 0.2274.
Based on the above result, we can conclude with 95% confidence that the proportion of
females in occupation with code 2 would vary from 0.5008 to 0.6469.
Secure Best Marks with AI Grader
Need help grading? Try our AI Grader for instant feedback on your assignments.
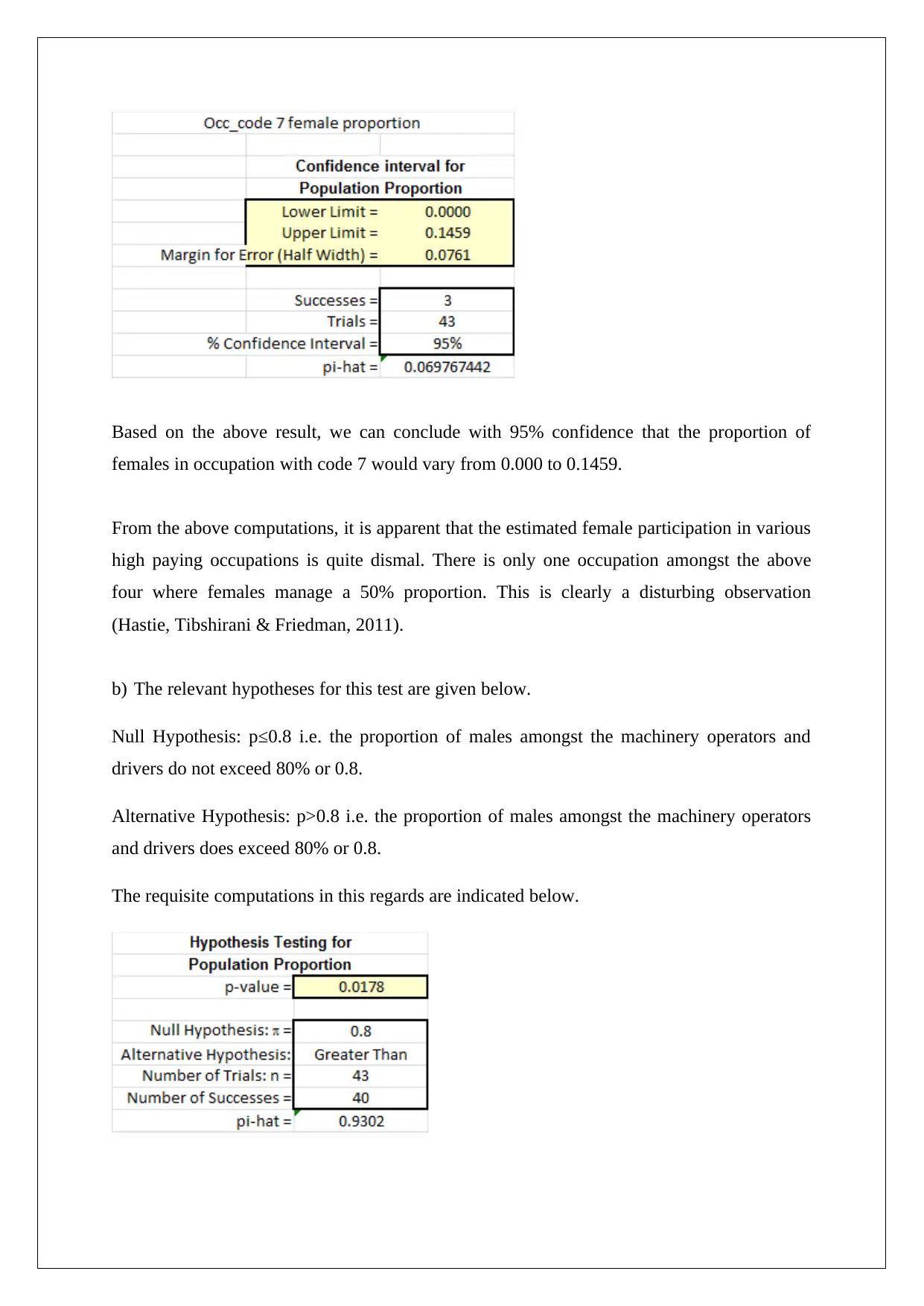
Based on the above result, we can conclude with 95% confidence that the proportion of
females in occupation with code 7 would vary from 0.000 to 0.1459.
From the above computations, it is apparent that the estimated female participation in various
high paying occupations is quite dismal. There is only one occupation amongst the above
four where females manage a 50% proportion. This is clearly a disturbing observation
(Hastie, Tibshirani & Friedman, 2011).
b) The relevant hypotheses for this test are given below.
Null Hypothesis: p≤0.8 i.e. the proportion of males amongst the machinery operators and
drivers do not exceed 80% or 0.8.
Alternative Hypothesis: p>0.8 i.e. the proportion of males amongst the machinery operators
and drivers does exceed 80% or 0.8.
The requisite computations in this regards are indicated below.
females in occupation with code 7 would vary from 0.000 to 0.1459.
From the above computations, it is apparent that the estimated female participation in various
high paying occupations is quite dismal. There is only one occupation amongst the above
four where females manage a 50% proportion. This is clearly a disturbing observation
(Hastie, Tibshirani & Friedman, 2011).
b) The relevant hypotheses for this test are given below.
Null Hypothesis: p≤0.8 i.e. the proportion of males amongst the machinery operators and
drivers do not exceed 80% or 0.8.
Alternative Hypothesis: p>0.8 i.e. the proportion of males amongst the machinery operators
and drivers does exceed 80% or 0.8.
The requisite computations in this regards are indicated below.
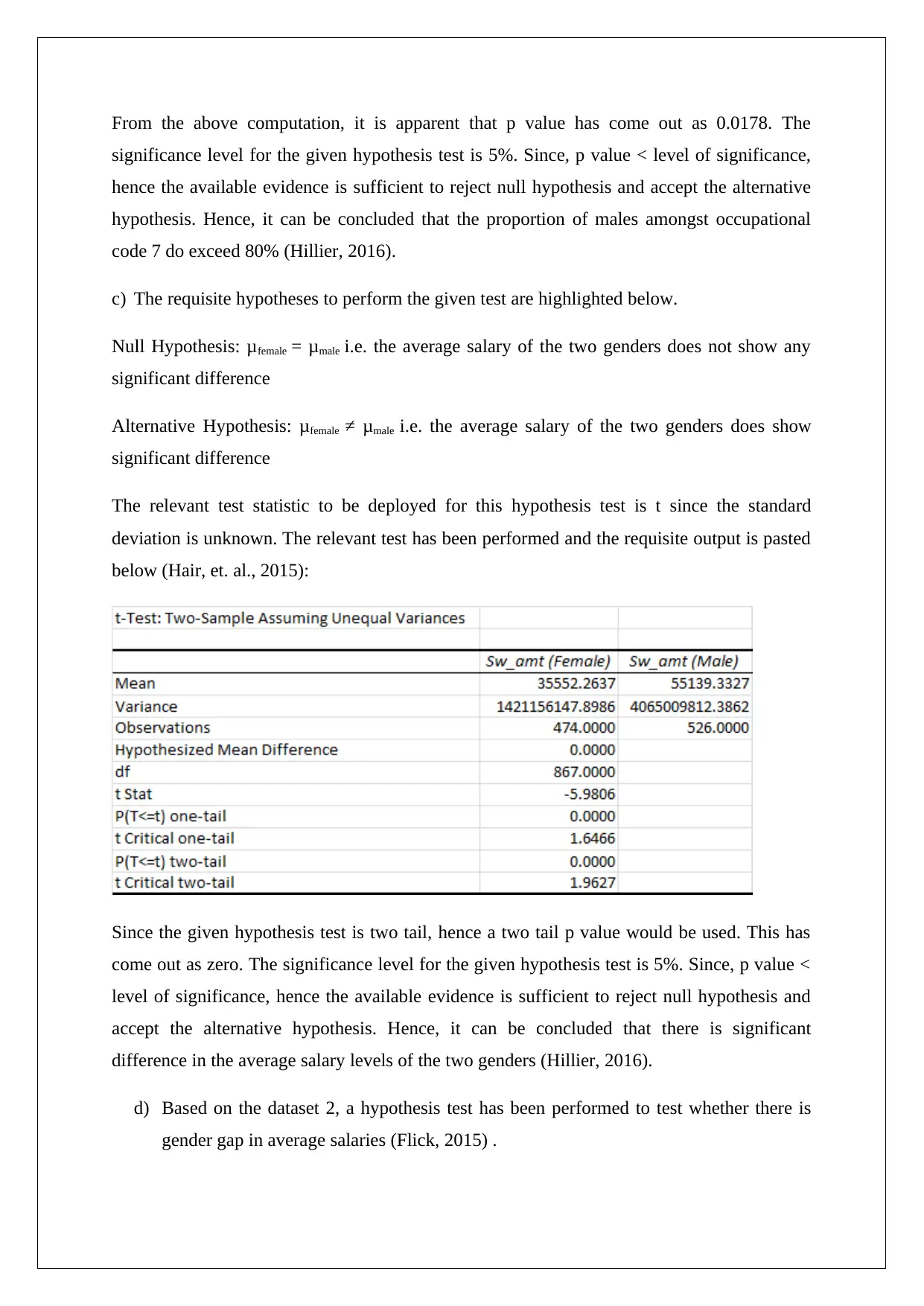
From the above computation, it is apparent that p value has come out as 0.0178. The
significance level for the given hypothesis test is 5%. Since, p value < level of significance,
hence the available evidence is sufficient to reject null hypothesis and accept the alternative
hypothesis. Hence, it can be concluded that the proportion of males amongst occupational
code 7 do exceed 80% (Hillier, 2016).
c) The requisite hypotheses to perform the given test are highlighted below.
Null Hypothesis: μfemale = μmale i.e. the average salary of the two genders does not show any
significant difference
Alternative Hypothesis: μfemale ≠ μmale i.e. the average salary of the two genders does show
significant difference
The relevant test statistic to be deployed for this hypothesis test is t since the standard
deviation is unknown. The relevant test has been performed and the requisite output is pasted
below (Hair, et. al., 2015):
Since the given hypothesis test is two tail, hence a two tail p value would be used. This has
come out as zero. The significance level for the given hypothesis test is 5%. Since, p value <
level of significance, hence the available evidence is sufficient to reject null hypothesis and
accept the alternative hypothesis. Hence, it can be concluded that there is significant
difference in the average salary levels of the two genders (Hillier, 2016).
d) Based on the dataset 2, a hypothesis test has been performed to test whether there is
gender gap in average salaries (Flick, 2015) .
significance level for the given hypothesis test is 5%. Since, p value < level of significance,
hence the available evidence is sufficient to reject null hypothesis and accept the alternative
hypothesis. Hence, it can be concluded that the proportion of males amongst occupational
code 7 do exceed 80% (Hillier, 2016).
c) The requisite hypotheses to perform the given test are highlighted below.
Null Hypothesis: μfemale = μmale i.e. the average salary of the two genders does not show any
significant difference
Alternative Hypothesis: μfemale ≠ μmale i.e. the average salary of the two genders does show
significant difference
The relevant test statistic to be deployed for this hypothesis test is t since the standard
deviation is unknown. The relevant test has been performed and the requisite output is pasted
below (Hair, et. al., 2015):
Since the given hypothesis test is two tail, hence a two tail p value would be used. This has
come out as zero. The significance level for the given hypothesis test is 5%. Since, p value <
level of significance, hence the available evidence is sufficient to reject null hypothesis and
accept the alternative hypothesis. Hence, it can be concluded that there is significant
difference in the average salary levels of the two genders (Hillier, 2016).
d) Based on the dataset 2, a hypothesis test has been performed to test whether there is
gender gap in average salaries (Flick, 2015) .
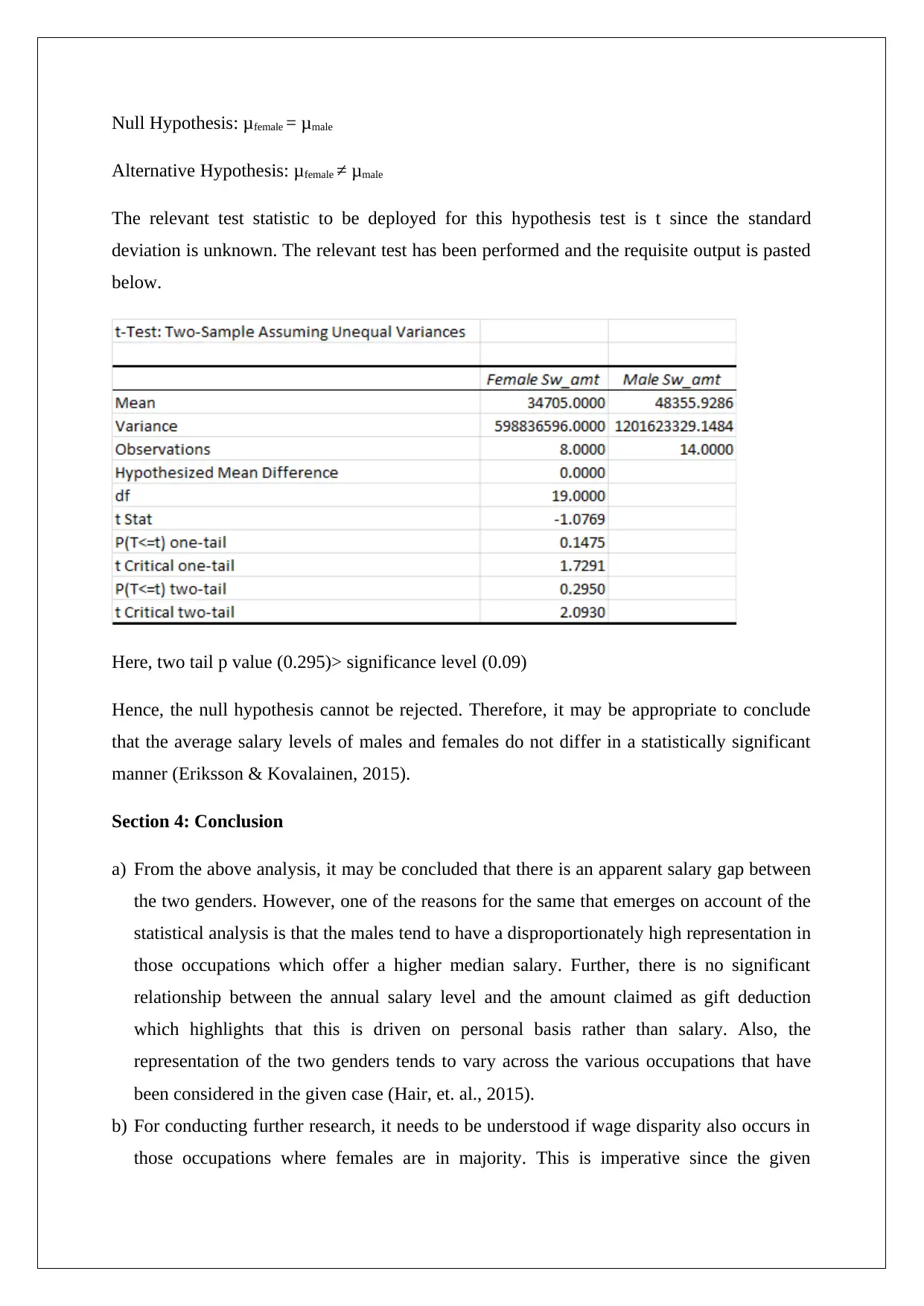
Null Hypothesis: μfemale = μmale
Alternative Hypothesis: μfemale ≠ μmale
The relevant test statistic to be deployed for this hypothesis test is t since the standard
deviation is unknown. The relevant test has been performed and the requisite output is pasted
below.
Here, two tail p value (0.295)> significance level (0.09)
Hence, the null hypothesis cannot be rejected. Therefore, it may be appropriate to conclude
that the average salary levels of males and females do not differ in a statistically significant
manner (Eriksson & Kovalainen, 2015).
Section 4: Conclusion
a) From the above analysis, it may be concluded that there is an apparent salary gap between
the two genders. However, one of the reasons for the same that emerges on account of the
statistical analysis is that the males tend to have a disproportionately high representation in
those occupations which offer a higher median salary. Further, there is no significant
relationship between the annual salary level and the amount claimed as gift deduction
which highlights that this is driven on personal basis rather than salary. Also, the
representation of the two genders tends to vary across the various occupations that have
been considered in the given case (Hair, et. al., 2015).
b) For conducting further research, it needs to be understood if wage disparity also occurs in
those occupations where females are in majority. This is imperative since the given
Alternative Hypothesis: μfemale ≠ μmale
The relevant test statistic to be deployed for this hypothesis test is t since the standard
deviation is unknown. The relevant test has been performed and the requisite output is pasted
below.
Here, two tail p value (0.295)> significance level (0.09)
Hence, the null hypothesis cannot be rejected. Therefore, it may be appropriate to conclude
that the average salary levels of males and females do not differ in a statistically significant
manner (Eriksson & Kovalainen, 2015).
Section 4: Conclusion
a) From the above analysis, it may be concluded that there is an apparent salary gap between
the two genders. However, one of the reasons for the same that emerges on account of the
statistical analysis is that the males tend to have a disproportionately high representation in
those occupations which offer a higher median salary. Further, there is no significant
relationship between the annual salary level and the amount claimed as gift deduction
which highlights that this is driven on personal basis rather than salary. Also, the
representation of the two genders tends to vary across the various occupations that have
been considered in the given case (Hair, et. al., 2015).
b) For conducting further research, it needs to be understood if wage disparity also occurs in
those occupations where females are in majority. This is imperative since the given
Paraphrase This Document
Need a fresh take? Get an instant paraphrase of this document with our AI Paraphraser
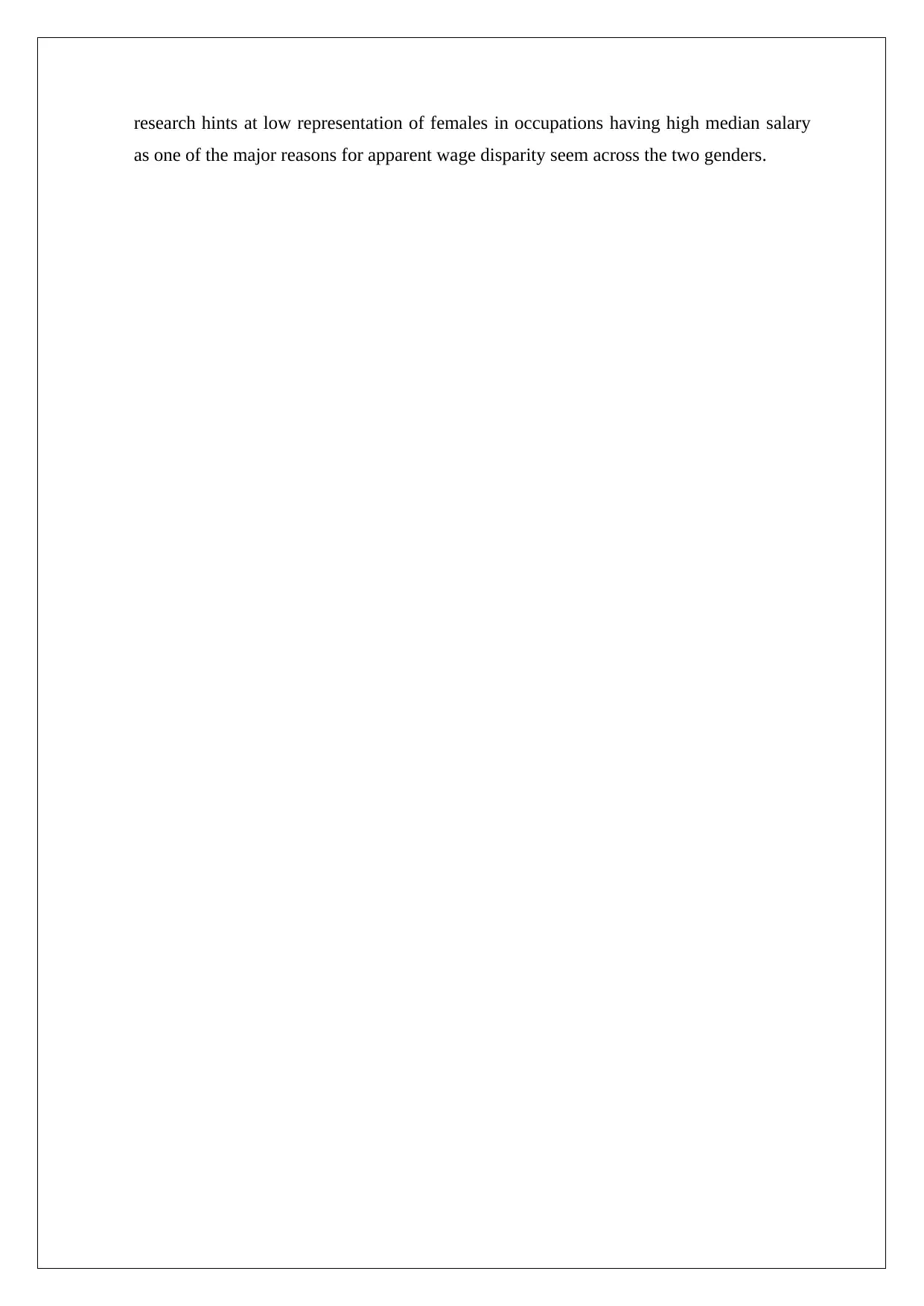
research hints at low representation of females in occupations having high median salary
as one of the major reasons for apparent wage disparity seem across the two genders.
as one of the major reasons for apparent wage disparity seem across the two genders.
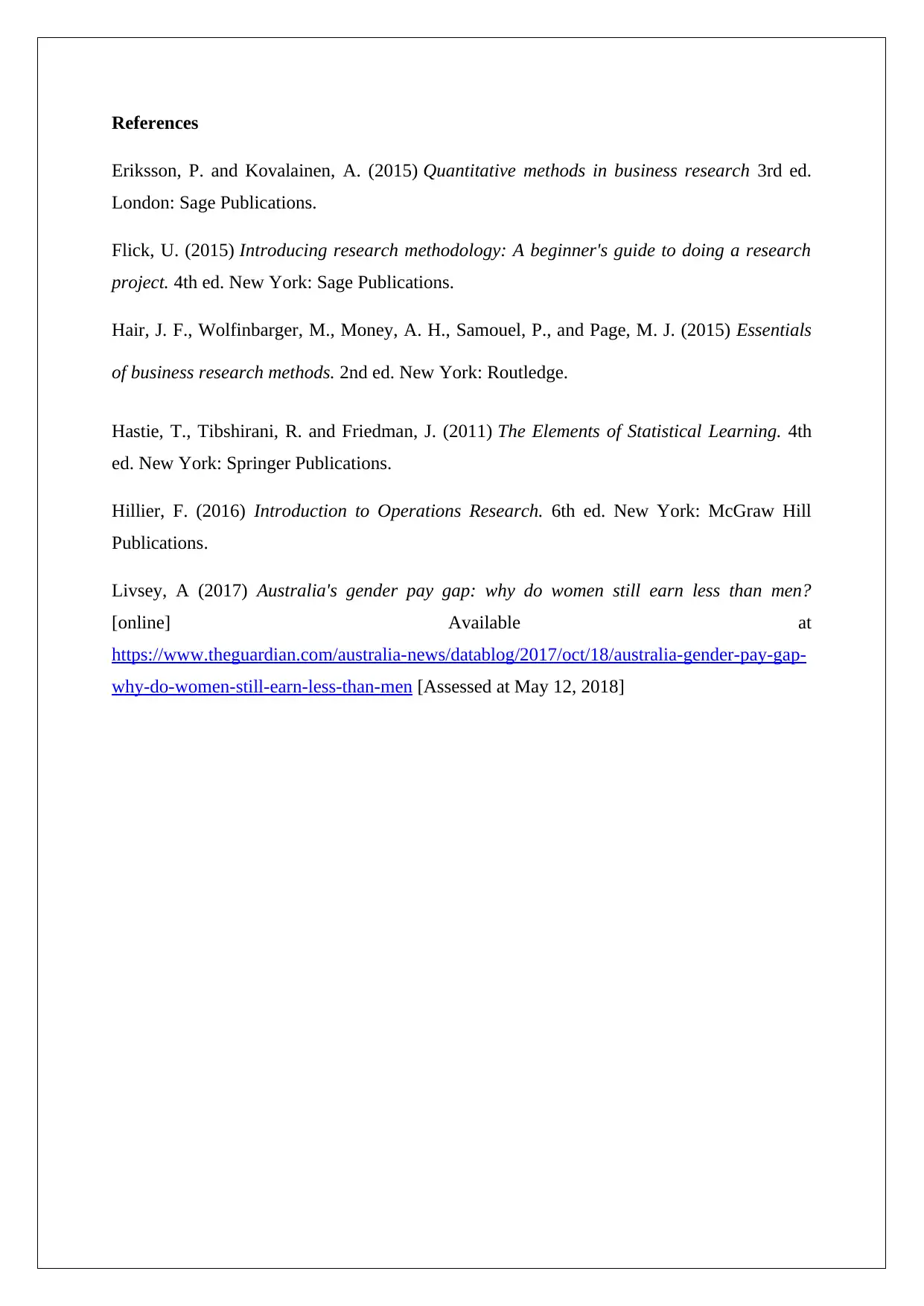
References
Eriksson, P. and Kovalainen, A. (2015) Quantitative methods in business research 3rd ed.
London: Sage Publications.
Flick, U. (2015) Introducing research methodology: A beginner's guide to doing a research
project. 4th ed. New York: Sage Publications.
Hair, J. F., Wolfinbarger, M., Money, A. H., Samouel, P., and Page, M. J. (2015) Essentials
of business research methods. 2nd ed. New York: Routledge.
Hastie, T., Tibshirani, R. and Friedman, J. (2011) The Elements of Statistical Learning. 4th
ed. New York: Springer Publications.
Hillier, F. (2016) Introduction to Operations Research. 6th ed. New York: McGraw Hill
Publications.
Livsey, A (2017) Australia's gender pay gap: why do women still earn less than men?
[online] Available at
https://www.theguardian.com/australia-news/datablog/2017/oct/18/australia-gender-pay-gap-
why-do-women-still-earn-less-than-men [Assessed at May 12, 2018]
Eriksson, P. and Kovalainen, A. (2015) Quantitative methods in business research 3rd ed.
London: Sage Publications.
Flick, U. (2015) Introducing research methodology: A beginner's guide to doing a research
project. 4th ed. New York: Sage Publications.
Hair, J. F., Wolfinbarger, M., Money, A. H., Samouel, P., and Page, M. J. (2015) Essentials
of business research methods. 2nd ed. New York: Routledge.
Hastie, T., Tibshirani, R. and Friedman, J. (2011) The Elements of Statistical Learning. 4th
ed. New York: Springer Publications.
Hillier, F. (2016) Introduction to Operations Research. 6th ed. New York: McGraw Hill
Publications.
Livsey, A (2017) Australia's gender pay gap: why do women still earn less than men?
[online] Available at
https://www.theguardian.com/australia-news/datablog/2017/oct/18/australia-gender-pay-gap-
why-do-women-still-earn-less-than-men [Assessed at May 12, 2018]
1 out of 12
Related Documents
![[object Object]](/_next/image/?url=%2F_next%2Fstatic%2Fmedia%2Flogo.6d15ce61.png&w=640&q=75)
Your All-in-One AI-Powered Toolkit for Academic Success.
+13062052269
info@desklib.com
Available 24*7 on WhatsApp / Email
Unlock your academic potential
© 2024 | Zucol Services PVT LTD | All rights reserved.